the Creative Commons Attribution 4.0 License.
the Creative Commons Attribution 4.0 License.
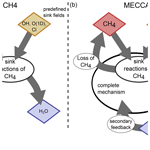
Methane chemistry in a nutshell – the new submodels CH4 (v1.0) and TRSYNC (v1.0) in MESSy (v2.54.0)
Franziska Winterstein
Patrick Jöckel
Climate projections including chemical feedbacks rely on state-of-the-art chemistry–climate models (CCMs). Of particular importance is the role of methane (CH4) for the budget of stratospheric water vapour (SWV), which has an important climate impact. However, simulations with CCMs are, due to the large number of involved chemical species, computationally demanding, which limits the simulation of sensitivity studies.
To allow for sensitivity studies and ensemble simulations with a reduced demand for computational resources, we introduce a simplified approach to simulate the core of methane chemistry in form of the new Modular Earth Submodel System (MESSy) submodel CH4. It involves an atmospheric chemistry mechanism reduced to the sink reactions of CH4 with predefined fields of the hydroxyl radical (OH), excited oxygen (O(1D)), and chlorine (Cl), as well as photolysis and the reaction products limited to water vapour (H2O). This chemical production of H2O is optionally fed back onto the specific humidity (q) of the connected general circulation model (GCM), to account for the impact onto SWV and its effect on radiation and stratospheric dynamics.
The submodel CH4 is further capable of simulating the four most prevalent CH4 isotopologues for carbon and hydrogen (CH4 and CH3D, as well as 12CH4 and 13CH4). Furthermore, the production of deuterated water vapour (HDO) is, similar to the production of H2O in the CH4 oxidation, optionally passed back to the isotopological hydrological cycle simulated by the submodel H2OISO, using the newly developed auxiliary submodel TRSYNC. Moreover, the simulation of a user-defined number of diagnostic CH4 age and emission classes is possible, the output of which can be used for offline inverse optimization techniques.
The presented approach combines the most important chemical hydrological feedback including the isotopic signatures with the advantages concerning the computational simplicity of a GCM, in comparison to a full-featured CCM.
- Article
(3175 KB) -
Supplement
(2635 KB) - BibTeX
- EndNote
It is beyond question that methane (CH4) is a strong greenhouse gas (GHG), with an estimated global warming potential (GWP) of 34 times that of carbon dioxide (CO2) on a 100-year horizon (IPCC, 2013). Therefore, most general circulation models (GCMs) include the effect of the radiative forcing of CH4. However, the effect of CH4 is underrepresented by only using its direct radiative impact and not accounting for the water vapour (H2O) produced by the oxidation of CH4 due to a set-up without chemistry. Especially in the stratosphere, this additional H2O (stratospheric water vapour, SWV) influences, among other factors, the radiative forcing, stratospheric temperature, and ozone (O3) chemistry (Stenke and Grewe, 2005; Tian et al., 2009; Solomon et al., 2010; Revell et al., 2012; Winterstein et al., 2019). The inclusion of production of H2O by CH4 requires a chemical mechanism as provided by chemistry–climate models (CCMs). Current state-of-the-art CCMs include a vast amount of chemical species and reactions. By extending the chemical mechanisms, it is intended to achieve an increase in accuracy of the atmospheric chemistry representation. At the same time, however, the computational demands increase. Although available computational power increases at a certain rate, the availability and capacity of high-performance computers is a limiting factor for sensitivity studies in climate projection simulations with CCMs. It is hence advisable to recognize both of the main effects of CH4, namely its radiative forcing and its impact on SWV, while at the same time keeping computational demands low. Therefore, our approach to simulate CH4 includes both effects and is able to use predefined reaction partners of CH4, which reduces computational cost to a minimum.
An early version of the simplified CH4 chemistry submodel (CH4) has been described by Eichinger et al. (2015a). The present version has been updated and extended by the additional features for simulating age and emission classes and isotopologues.
Section 1.1 and 1.2 introduce the sources and sinks of CH4 and CH4 isotopologues and their fractionation effects, respectively. In Sect. 2, we briefly present the Modular Earth Submodel System and describe the concept of the CH4 submodel in Sect. 3. Two additional options of the CH4 submodel are explained in the subsequent Sect. 3.1 and 3.2. The coupling to the hydrological cycle with the submodel TRSYNC is introduced in Sect. 4. We show three example applications using the newly presented submodels in Sect. 5 and end with a short summary. Parts of the paper are based on the PhD thesis of the first author (Frank, 2018).
1.1 Sources and sinks of CH4
Methane is a GHG emitted by both natural and anthropogenic sources at the Earth's surface. There are basically no known chemical sources of CH4 in the free atmosphere.
In CCMs usually predefined lower boundary conditions instead of emission fluxes are used to describe atmospheric CH4. This approach is mainly employed due to two major problems. (1) The lifetime of CH4 is on an order of magnitude of 10 years, but its exact value is still unknown and subject to uncertainties. However, CH4 is an important precursor of the Ox-HOx chemistry in CCMs. For this reason, in most CCM set-ups CH4 is prescribed at the lower model boundary to achieve a realistic CH4 burden independent of the simulated lifetime. (2) Despite large ongoing efforts, current emission inventories are still subject to large uncertainties, as top-down and bottom-up inventories differ significantly (e.g. EDGAR or Saunois et al., 2016). This mismatch indicates the dilemma that there are a lot of open questions with respect to both the magnitude of sources and the sinks of CH4.
Methane is removed from the atmosphere mainly by three photochemical reactions:
and is also depleted by photolysis:
with Reactions (R1)–(R3) taken from Sander et al. (2011) and Reaction (R4) taken from Sander et al. (2014).
About 92 % of the atmospheric CH4 removal happens in the troposphere. The largest part is thereby the reaction with the hydroxyl radical (OH) (>90 % of the tropospheric sink), while the rest is attributed to the reaction with chlorine (Cl) in the marine boundary layer (MBL, Kirschke et al., 2013). About 8 % of CH4 is depleted in the stratosphere by the reactions with OH, excited oxygen (O(1D)), and Cl and through photolysis (IPCC, 2013).
Another sink of CH4 is the so-called soil loss at the Earth's surface. CH4 is either depleted by CH4-consuming bacteria (methanotrophs) or it is removed from the air by diffusive transport into the soil, which is mostly influenced by soil water content (King, 1997). Globally, the soil loss accounts for approximately 4 % of the total CH4 sink (IPCC, 2013).
1.2 Isotopologues of CH4
A powerful and common method in the investigation of the CH4 budget is the study of CH4 isotopologues. Production and removal of CH4 both cause fractionation effects, which lead to distinct isotopological signals in the atmosphere. These isotopic signatures potentially provide additional insights into the role of specific CH4 sources and depleting reactions and are already widely used in the context of CH4 (Hein et al., 1997; Fletcher et al., 2004; Monteil et al., 2011; Rigby et al., 2012; Nisbet et al., 2016; Schaefer et al., 2016).
Fundamentally, the stable isotopologues of CH4 form with respect to the most abundant stable isotopes of hydrogen and carbon. The stable isotopes of hydrogen are 1H and 2H (deuterium, D), and for carbon the stable isotopes are carbon-12 (12C) and carbon-13 (13C). This results in the first-order stable isotopologues 12CH4, 13CH4, and CH3D. The corresponding sink reactions are shown in the Supplement (Sect. S1). The relative abundances of higher substituted and mixed isotopologues (e.g. CH2D2 or 13CH3D) are less than 0.0007 % (compared to 0.0616 % of CH3D) (Stolper et al., 2014) and hence neglected.
The chemical fractionation is based on the fact that isotopologues of the same molecule have different rate coefficients; i.e. they react with different speed or probability. This difference in rate coefficients is described as the so-called kinetic isotope effect (KIE) and becomes apparent during the chemical reaction of a specific molecule X:
with XL being its light (major) and XH its heavy (minor) isotopologue. E and denote the reaction partner(s) and product(s), respectively. The value of the KIE is thereby defined as the ratio of the rate coefficients kL and kH (Bigeleisen, 2005), and its inverse is called the fractionation factor α:
The KIEs of the sink reactions of CH4 have been determined by Saueressig et al. (1995, 1996, 2001), and Crowley et al. (1999), among others, in laboratory measurements (see Table 1). Since the KIEs of CH4 isotopologues are partly temperature dependent, the KIEs are described by two parameters, A and B, and are calculated as
with T being the temperature in K.
Saueressig et al. (2001)Saueressig et al. (2001)Saueressig et al. (1995)Crowley et al. (1999)Saueressig et al. (2001)Saueressig et al. (2001)Saueressig et al. (1996)Table 1Temperature-dependent KIEs of the sink reactions of CH4 described as . The KIEs are valid in the given temperature range (T, in K).
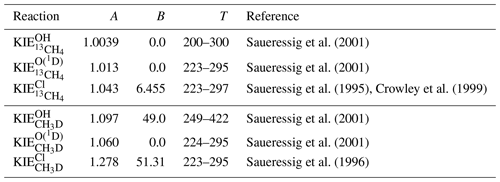
The largest KIE and therefore strongest fractionation effect is found for the reaction with Cl, which especially influences the isotopic composition of CH4 in the middle and upper stratosphere (Saueressig et al., 1996; Bergamaschi et al., 1996). Conversely, the reaction with O(1D) shows the lowest KIE, which furthermore does not show any temperature dependence. The KIE of the reaction with OH is temperature dependent with respect to deuterated methane (CH3D) but not with respect to methane containing 13C (13CH4) (Saueressig et al., 2001). Nair et al. (2005) estimated the rate coefficients of the photodissociation of CH4 and its major isotopologues for the planet Mars, which results in a calculated KIE=1.005 for CH3D and a negligible isotopic fractionation for the 13C isotopologue (Nixon et al., 2012). There is, especially for deuterium, a non-negligible fractionation during the soil-loss for CH4 (Snover and Quay, 2000; Maxfield et al., 2008). An average value for the overall soil loss is estimated as KIE and KIE (Snover and Quay, 2000; Holmgren, 2006; Maxfield et al., 2008).
The framework of the Modular Earth Submodel System (MESSy, used in the second version MESSy2, Jöckel et al., 2010) is based on the idea of modularizing a climate model in such a way that single components can be switched on and off independently, depending on the desired set-up, meeting the demands of current Earth system modelling in terms of flexibility and computational performance. The modularization enables the user to pick suitable submodels or easily expand the model with new ones. Presented here are the submodel CH4 and the auxiliary submodel TRacer SYNChronization (TRSYNC), which are implemented based on this framework.
For the application examples of the new submodels, MESSy is used together with the fifth-generation European Centre Hamburg general circulation model (ECHAM5, Roeckner et al., 2006). The ECHAM/MESSy Atmospheric Chemistry (EMAC) model is a numerical chemistry and climate simulation system that includes sub-models describing tropospheric and middle atmosphere processes and their interaction with oceans, land, and human influences (Jöckel et al., 2010). EMAC (ECHAM5 version 5.3.02, MESSy version 2.54, Jöckel et al., 2010, 2016) is applied in the given examples in the T42L90MA-resolution, i.e. with a spherical truncation of T42, which corresponds to a quadratic Gaussian grid of approx. 2.8 by 2.8∘ in latitude and longitude and includes 90 vertical hybrid pressure levels from the Earth surface up to 0.01 hPa. MESSy allows the configuration of EMAC in several operational modes. The two basic ones are the GCM set-up without chemistry and the CCM set-up with fully interactive chemistry, using, among other components, the Module Efficiently Calculating the Chemistry of the Atmosphere (MECCA, Sander et al., 2005) and the SCAVenging (SCAV, Tost et al., 2006) submodel to represent the chemical kinetics of EMAC in the gas phase and aqueous phase, respectively. They define the underlying chemical reaction mechanisms in troposphere, stratosphere, and lower mesosphere. MECCA and SCAV provide comprehensive mechanisms, combining state-of-the-art reactions and rate coefficients. The kinetic chemistry tagging technique (MECCA_TAG, Gromov et al., 2010) enables the user to tag selected chemical elements, without modifying the underlying standard chemical mechanism of MECCA. It can be applied for simulating isotopologues of trace gases with respect to selected isotopes. In order to do so, rare and abundant isotopologues of the species of interest (e.g. those containing atomic hydrogen, H) are created in an extended set of reactions in the same chemical mechanism.
MESSy and its application in EMAC has been used in multiple studies (see the special issue of Atmospheric Chemistry and Physics https://www.atmos-chem-phys.net/special_issue22.html, last access: 18 January 2021) and includes several submodels from contributing institutions. Further information on EMAC, MESSy, and its submodels can be found in Jöckel et al. (2010, 2016), on the website https://www.messy-interface.org/ (last access: 18 January 2021), or in the accompanying papers documenting the specific submodels.
The MESSy submodel CH4 aims to close the gap between the operational modes of EMAC as a GCM without chemistry and as a CCM with the comprehensive chemical mechanisms of MECCA and SCAV. The basic concept of the submodel is to limit the chemical mechanism to the loss processes of methane and use predefined fields of the reaction partners OH, O(1D), and Cl to reduce the computational demands.
The sink Reactions (R1)–(R4) in the CH4 submodel are parameterized as follows:
where [X] denotes the mixing ratio of species X in mol mol−1, cair is the concentration of air in cm−3, kR is the reaction rate coefficient of reaction R in cm3 s−1, and is the photolysis rate of CH4 in s−1.
The prescribed fields are taken either from existing simulation results with detailed chemistry, or from other data sources (e.g. reanalyses or projections). If CH4 is included in an EMAC CCM simulation (which is possible in the MESSy framework), the CH4 submodel can also be coupled to the reactant fields, which are calculated online during the same simulation by the chemical mechanism (i.e. MECCA). Although this does not reduce computational requirements, such a simulation configuration can be used, for example, if output of one of the additional options of the CH4 submodel (age and emission classes or isotopologues) is desired. In that case a second CH4 tracer is treated and oxidized by the reactants provided by the kinetic solver of the comprehensive chemical mechanism. The same applies for the photolysis rate of CH4, which can be prescribed as gridded data provided offline or calculated online by the submodel JVAL (Sander et al., 2014). In either case, the CH4 submodel does not alter the reactant educts. Hence there is no feedback onto the CH4 sink by the submodel. In the case of coupling to MECCA via the educts, the coupling is one-way only.
Figure 1 visualizes the conceptual differences between the MESSy submodel CH4 (left) and a CCM simulation with MECCA (right). MECCA simulates the entire chemical mechanism and therefore also includes the feedback onto the reaction partners (depicted in yellow) of CH4. Additionally, there is also a secondary feedback by the products from the CH4 sink reactions (e.g. H2O and HO2, depicted in blue), as the subsequent chemical processes are influenced by the products from the CH4 oxidation. Conversely, the CH4 submodel uses the prescribed fields of the reactant species to calculate the CH4 loss. This loss is included in the master tracer of the CH4 submodel (the present CH4 is reduced) but does not feed back onto the sink fields or any other chemical species. The only exception is H2O, in the case when the hydrological feedback of CH4 oxidation is switched on. GCMs include CH4 foremost for its radiative impact as a greenhouse gas but also for its influence on SWV (e.g. Monge-Sanz et al., 2013; ECMWF, 2007; Austin et al., 2007; Boville et al., 2001; Mote, 1995). The CH4 submodel is therefore equipped with an optional feedback onto H2O to account for part of the secondary climate feedback of CH4. It is thereby assumed that two molecules of H2O are produced per oxidized CH4 molecule (le Texier et al., 1988), which is, however, only a rough approximation, as analysed by Frank et al. (2018). The approximation of two molecules H2O per oxidized CH4 molecule overestimates the H2O production in the lower stratosphere and underestimates the production in the upper stratosphere. It also does not account for the chemical loss of H2O in the mesosphere.
Note that soil loss is not explicitly included in the CH4 submodel, since the concept of dry deposition is already part of the EMAC submodel DDEP (Kerkweg et al., 2006a). An example of how to use DDEP to simulate the soil loss of CH4 is included in the Supplement to this paper.
The submodel CH4, with its four sink reactions of CH4, is considerably computationally cheaper compared to a fully interactive chemistry simulation using MECCA, which represents (depending on the chosen set-up) several hundred reactions (e.g. more than 300 in the base simulations of the Earth System Chemistry integrated Modelling (ESCiMo) project Jöckel et al., 2016). For example, a reference set-up with MECCA requires about 250 node-h1 per simulated year, while a set-up with the CH4 submodel without MECCA requires only 30 node-h per year. These numbers are calculated for simulations conducted on the high-performance computer (HPC) Mistral at the German Climate Computing Center (Deutsches Klimarechenzentrum, DKRZ).
The presented framework of the reduced CH4 chemistry is applicable since CH4 is only reduced and not produced in the free atmosphere. Therefore, the discretization of the four reactions where CH4 is involved is sufficient to represent the chemical loss of CH4. Nevertheless, in order to achieve meaningful simulation results with the CH4 submodel, some prerequisites have to be met. Since the educts (OH, O(1D), and Cl) are prescribed, there is no feedback on them. Thus, very large variations in CH4 mixing ratio, which would in reality influence the CH4 sink (Winterstein et al., 2019), are not representable by the CH4 submodel.
Furthermore, the set-up with the CH4 submodel also lacks any feedback on O3. In the atmosphere, the O3 chemistry is influenced by changes in OH (reduced by CH4), H2O (produced by CH4) and temperature (influenced by the radiatively active CH4). The CH4 submodel alters H2O and with that influences the radiation budget and hence the temperature; however, there is no feedback on O3 when the set-up does not include any other chemical mechanism. In a set-up where the CH4 submodel is not used in parallel to MECCA, O3 time series or climatologies are usually prescribed for the radiation scheme.
The first simulations using the CH4 submodel are presented in studies by Eichinger et al. (2015a, b), it was included in the simulations of the ESCiMo project (Jöckel et al., 2016), and it has been used for the CH4 forecast system presented by Nickl et al. (2020).
3.1 Option I: age and emission classes
The CH4 submodel includes an option for simulating age and emission classes. These classes, which can be specified by the user via a namelist, enable a precise distinction between CH4 source sectors and/or regions (emission classes), as well as further insight into the CH4 distribution over time (age classes). The term “emission class” thereby denotes a CH4-like tracer defined by the CH4 submodel. The assignment of specific emission fluxes (sectors and regions) to the tracers of the emission classes is handled by the submodel OFFEMIS (Kerkweg et al., 2006b). In our present example application these classes are subject to emissions being a combination of an emission sector (like wetlands, biomass burning, anthropogenic sources, etc.) and a region (e.g. continents or countries). One tracer, for example, thus traces anthropogenic CH4 emitted from Africa, as shown in Sect. 5.1. These additional diagnostic tracers are transported identically to the master CH4 tracer of the CH4 submodel and also experience the same sink reactions.
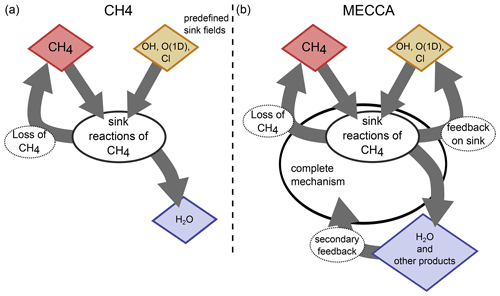
Figure 1Sketch visualizing the concepts of parameterizing CH4 sink reactions in the MESSy submodels CH4 (a) and MECCA (b). The red species is the core species CH4. The chemical mechanism in CH4 is reduced to the sink reactions of CH4 and optionally includes the feedback to H2O (blue) only. In MECCA a complete chemical mechanism is included, which among others feeds back onto H2O and other products of the CH4 sink reactions, including the reaction partners (educts) of CH4. The reaction partners (yellow) in the CH4 submodel are prescribed and not changed by the CH4 submodel.
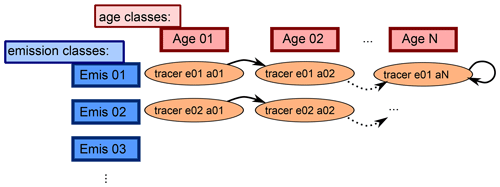
Figure 2Sketch showing the advancing of the age classes in the CH4 submodel. Each tracer represents one specific emission and age class. After the defined length of time, the age classes proceed to the next “older” age class. The last class represents the background CH4, where the CH4 is only subject to transport and the chemically defined sink reactions but is not propagated to an older age class, which is indicated by the circled arrow.
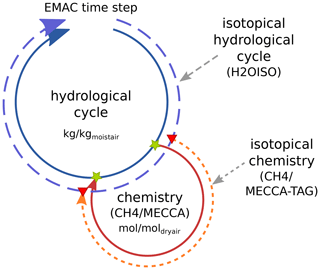
Figure 3Sketch showing the coupling of the hydrological cycle and the chemistry (either CH4 or MECCA) with respect to H2O isotopologues in one time step of EMAC. Green stars indicate the points of the hydrological cycle, where (1) the current value of the water vapour master tracer is taken and (2) the chemical tendencies are added onto the water vapour master tracer. Red triangles indicate the synchronization points of the corresponding isotopical tracers by the TRSYNC submodel. Synchronization of the isotopological cycles also accounts for the appropriate unit conversion and adds the tendency of chemical processes to the fractionation in the physical hydrological cycle.
The time period represented by one age class can be chosen by the user. How the age and emission classes evolve over time is depicted in Fig. 2. CH4 of each emission class is propagated through a specific number of age classes. The emitted CH4 of a specific emission class is added to the tracer that corresponds to the first age class. After the selected time span it moves to the next “older” age class until it reaches the oldest. The oldest age class represents the background, since CH4 does not proceed further.
Which age evolving method is applied is also selectable. The CH4 submodel offers three options: (1) CH4 is passed on in one step after a user-defined time span, (2) CH4 is continuously passed on with respect to an user-defined time span, and (3) CH4 is passed on monthly with fixed time lag.
We define the state vector for emission class i and age classes 1 to N as follows:
The first two options are implemented according to
with Δfi being the tendency of fi, Δt being the time step length, and M being a matrix defining the ageing step according to the chosen option. For option (1) this matrix looks like
This moves the current values of one age class tracer after a user-defined time span to the next older one. This option is not consistent with a Leapfrog time stepping using an Asselin filter and might cause numerical oscillations and negative values. It was implemented solely for testing purposes during development, but it is not recommended for real applications. The ageing step matrix M for option (2) is M′:
with and being the user-defined time span indicating the binning width of the age class. This option carries out a quasi-continuous update of the age classes, as it moves a fraction (α) of the current age class to the next at every time step.
The third option is implemented for usage by a fixed-lag Kalman filter for inverse optimization. With this option, one age class represents 1 month, and at the end of 1 month all CH4 of one age class moves to the next. This option is specifically implemented to be consistent with the Leapfrog time stepping (cf. option 1). A preliminary application of the concept of using the age and emission classes for an inverse optimization using the fixed-lag Kalman filter has been shown by Frank (2018).
In order to reduce numerical errors, the age and emission classes are continuously constrained (i.e. in each model time step) to sum up to the master tracer and are scaled appropriately if the sum deviates. This procedure is done to avoid the accumulation of such numerical errors, which mainly arise from small non-linearities in the large-scale advection scheme. The magnitude therefore depends on the applied advection scheme but is usually on the order of the floating point precision.
3.2 Option II: isotopologues
In addition to solving the basic CH4 kinetics, the submodel CH4 also
allows for the simulation of CH4 isotopologues, which are a potent
diagnostic measure in the source and sink attribution. The submodel CH4 is
able to simulate the abundant and first-order rare isotopologues and defines
these as tracers additional to the master tracer. Higher substituted
isotopologues are neglected. The user can choose whether isotopologues are
simulated with respect to carbon (12CH4
and 13CH4), hydrogen (CH4 containing 1H isotopes only and CH3D), or both. The abundant (with 12C or 1H
isotopes only) and rare (with 13C or D) isotopologues are thereby
simulated in parallel. During the simulation care is taken that each
isotopologue family sums up to the master CH4 tracer of the CH4
submodel (CH4_fx
). The isotopic signatures of CH4 emission sources
are included by splitting the emission fluxes into an abundant and a rare
fraction. This is handled via the OFFEMIS namelist (Kerkweg et al., 2006b; see the
example namelists in the Supplement).
The rate coefficients of the CH4 isotopologues with their reaction partners are adjusted with respect to the KIE factors, e.g.
and are similar for reactions with O(1D), Cl, and photolysis. The applied reaction partners are thereby the same as those used for the master tracer.
To a certain extent the oxidation of CH3D produces deuterated water vapour (HDO). If the feedback of CH4 oxidation onto the hydrological cycle and the simulation of D-containing isotopologues is switched on in the CH4 submodel, an additional tracer for HDO is created by the submodel and filled by the produced HDO from CH3D oxidation. There are two options available: (1) one oxidized CH3D produces one HDO molecule, or (2) the tendency of the HDO tracer is calculated by Eq. (9) (Eichinger et al., 2015a):
with Mair and MHDO being the molar masses of air (28.987 g mol−1) and HDO (19.02 g mol−1), respectively. This empirical equation accounts for the D, which stays in molecular hydrogen (HD) as it builds up to an equilibrium with HDO via the HOx cycle.
In EMAC three different submodels are included that deal with isotopologues of H2O in the vapour phase: (1) the CH4 submodel presented here, (2) MECCA_TAG, and (3) H2O ISOtopologues (H2OISO, Eichinger et al., 2015a). CH4 and MECCA_TAG include the chemical fractionations, while H2OISO is responsible for the physical fractionations in the hydrological cycle of the underlying GCM. All three create independent tracers of H2O isotopologues, which need to be synchronized to be able to combine physical and chemical fractionation effects of H2O and its isotopologues. The chemical fractionation is thereby considered either from MECCA_TAG or from CH4, although both submodels can be concurrently included in a simulation and compute the isotopic fractionation independently.
In principle, if EMAC is applied in GCM mode, only the master hydrological cycle is present (see Fig. 3, inner blue circle). Adding MECCA or CH4 to the set-up expands the model into a CCM or a simple “CH4-only” CCM, respectively (solid red circle). The chemistry submodels use water vapour as a chemical tracer (first green star) and calculate the contribution from CH4 oxidation (second green star). This chemical feedback onto water vapour was already implemented as an option in previous EMAC versions. By including the isotopological submodels into the set-up, H2OISO duplicates the hydrological cycle for the water isotopologues and CH4 or MECCA_TAG creates the chemical tracers of the water isotopologues (outer dashed circles). This results in several physical and chemical H2O isotopologue tracers. While the master chemical process adds its feedback directly to the specific humidity of the hydrological cycle (there is no need for a chemical water tracer), the synchronization of the physical isotopological tracers in the isotopic hydrological cycle (H2OISO) and the chemical isotopological tracers (CH4 or MECCA_TAG) is done via the new auxiliary submodel TRSYNC. In brief, TRSYNC guarantees that the physical H2O tracers (including their isotopologues) also receive the correct tendencies of the corresponding chemical tracers. Since isotopological water vapour tracers of MECCA_TAG and the HDO tracer created by CH4 are transported in EMAC in the same way as every other tracer, they are subject to some of the physical processes but not to all hydrological fractionation effects. Thus, at the first synchronization point the chemical tracer is synchronized to represent the current value of the physical tracer. In the following, chemical tendencies including fractionation effects are calculated and are added via the second synchronization point to the physical tracer. By doing so, chemical and physical fractionation processes are strictly separated and the tendencies of the chemical tracers represent the chemical tendencies in addition to the previous physical fractionations in the current time step.
Water vapour in the physical hydrological cycle (regarding ECHAM5 and H2OISO) is defined in units of kilograms of the tracer per kilogram of moist air (), while the chemical tracers are defined in units of . This also holds for the corresponding isotopologue tracers. Parameterizations of physical processes in ECHAM5 are by design formulated with specific humidity (per moist air). Conversely, chemical reactions are necessarily calculated with species concentrations. This requires the individual chemical and physical isotopologue tracers, which have, for the sake of correct process formulations, distinct units, and motivated the development of the auxiliary submodel TRSYNC in order to be able to synchronize these tracers accordingly in the same way for CH4 and MECCA_TAG.
In addition to that, the application of MECCA_TAG creates the basis to investigate various other isotopes in the interactive chemical mechanism. While CH4 feedbacks onto H2O with respect to hydrogen isotopes only, MECCA_TAG can also be used to simulate oxygen isotopes (16O, 17O and 18O) in the chemical mechanism. It is therefore also possible to couple MECCA_TAG with oxygen isotopes to the corresponding oxygen-related isotopologue tracers in H2OISO. Last but not least, for MECCA_TAG tracer names are not standardized. Therefore, the namelist of the submodel TRSYNC can be adjusted according to the actual tracer names used in MECCA_TAG.
The following examples are simulations carried out with EMAC in a GCM-like mode, including the newly presented CH4 and TRSYNC submodels. Other involved MESSy submodels are OFFEMIS (Kerkweg et al., 2006b) and DDEP (Kerkweg et al., 2006a). OFFEMIS manages the emissions of CH4 from prescribed sources. It reads predefined fields with emission data and adds these fluxes to the chemical tracers. DDEP simulates the dry deposition for gases and aerosols and is used in the present context to simulate the soil loss of CH4, which is not done in the CH4 submodel itself.
Monthly mean sink fields are used in the simulation set-up in the examples below. Higher frequencies are technically possible; this would, however, increase the computational demands due to the larger amount of data read from disk. Monthly mean fields smooth the diurnal cycle, which is especially strong in OH. However, in order to investigate long-term global trends of CH4, which has a tropospheric lifetime of 8–10 years, variations on timescales of less than 1 month are negligible, and monthly mean fields are assumed to suffice for such applications. Furthermore, in these examples photolysis rates are calculated by the submodel JVAL in the presented examples, but predefined data can be used as well.
The H2OISO submodel (Eichinger, 2014; Eichinger et al., 2015a) simulates the stable water isotopologues with respect to H and D, as well as 16O, 17O, and 18O. Overall, it represents a second hydrological cycle, which includes water isotopologues in their three phases: gas, liquid, and ice. H2OISO accounts for fractionation processes during phase transitions in large-scale and convective clouds, during vertical diffusion, and during evaporation from the ocean (evaporation from soil, the biosphere, and snow are not considered to have a significant fractionation).
We simulated the years 1989 to 2012 and applied a specified dynamics set-up to represent the reanalysed meteorology of this time. Specified dynamics means here that the prognostic variables divergence, vorticity, temperature, and (logarithm of) surface pressure are nudged by Newtonian relaxation towards ECMWF ERA-Interim reanalysis data (Dee et al., 2011).
5.1 Application of the CH4 submodel for inverse optimization of CH4 emission inventories
Current estimates of CH4 emission inventories still include large uncertainties. In order to reduce these, new estimates of inventories must be able to represent temporal and spatial resolutions in greater detail (e.g. seasonal cycle, distinct regions). One statistical method to estimate CH4 emission strengths is the fixed-lag Kalman filter, which performs an inverse optimization of the emission inventory by comparing simulated and observed mixing ratios of a trace gas (see, e.g. Bruhwiler et al., 2005). This “offline” inversion algorithm requires data from a forward simulation, including temporal and spatial information of the simulated CH4 tracer.
In order to provide the necessary data, the CH4 submodel with the option of age and emission classes is applied. The combination of chosen regions and emission sectors in this example results in 48 emission classes altogether. These 48 emission classes are simulated with 5 age classes for ages up to 1, 2, 3, 4, and ≥5 months since emission release. Figure 4 exemplarily shows the evolution of a single emission class (i.e. anthropogenic emissions in Africa) from age class to age class. Figure 4a shows the emissions of the year 2000 in g(CH4) . Figure 4b–f show the age classes in ascending order and display the distribution of the CH4 mixing ratio onto the five age classes in January 2000 (the simulation has started in 1989). In the fourth age class the CH4 from anthropogenic African sources is almost evenly distributed, mostly in the Northern Hemisphere (NH). Eventually, the fifth age class (i.e. the last age class) shows the accumulated background of all CH4 from anthropogenic African sources. An a priori emission inventory is applied in the example situation.
Overall, the temporal evolution of the age classes in Fig. 4 confirms that the five age classes in this set-up sufficiently track the spread of CH4 towards a fairly uniform distribution, which is a prerequisite for a successful application of the inverse optimization method.
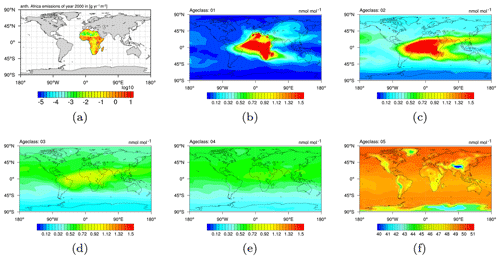
Figure 4(a) Anthropogenic emissions in Africa (taken from EDGARv4.2 2010 fast-track database; Olivier and Janssens-Maenhout, 2012). (b–f) Methane as a pressure-weighted column up to 200 hPa of anthropogenic origin from Africa, distributed into five age classes, i.e. up to 1, 2, 3, and 4, and ≥5 months after emission release. As an example, all age classes of January 2000 after the simulation has run for 12 years are shown.
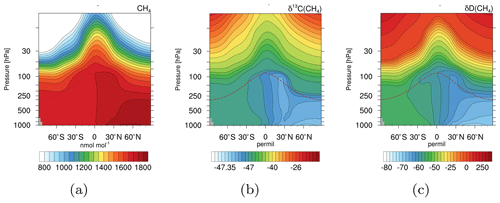
Figure 5Zonal mean climatologies of 2000–2009 for CH4 in nmol mol−1 (a), δ13C(CH4) in ‰ (b), and δD(CH4) in ‰ (c) of the simulation with EMAC and the CH4 submodel. The dashed brown lines indicate the height of the climatological tropopause.
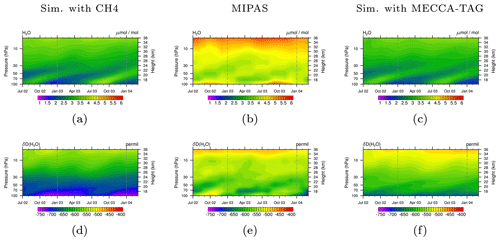
Figure 6Tropical stratospheric tape recorder signal of H2O (a, b, c) and δD(H2O) (d, e, f) in MIPAS data (b, e) and the simulations with the CH4 submodel (a, d) and the MECCA_TAG submodel (c, f) in the time period July 2002 to March 2004. Simulation data are averaged monthly, zonally, and over the tropics between 23∘ S–23∘ N and are displayed between 100 and 1 hPa. The dashed grey lines are included for eye guidance in the comparison of the tape recorder signal.
5.2 Simulating CH4 isotopologues
We further present a simulation using the CH4 submodel, which includes all four CH4 isotopologues. For this simulation, we applied a global a posteriori emission inventory provided by Dominik Brunner (personal communication, 2017) and a set of isotopic emission signatures prepared from data from the literature (see Table S1 in the Supplement).
Figure 5 shows zonal mean climatologies (2000–2009) of CH4 in nmol mol−1 and the corresponding isotopic signature in ‰. The isotopologues are displayed in the δ notation with respect to the reference isotope ratios Vienna PeeDee Belemnite (VPDB) for 13CH4, and Vienna Standard Mean Ocean Water (VSMOW) for CH3D, respectively. In the troposphere the NH is isotopically depleted compared to the Southern Hemisphere (SH). Most isotopically light emissions, for example from wetlands and rice, are located in the NH, while isotopically heavy sources like biomass burning are mostly located in the SH. This results in the prevalent tropospheric north–south gradient. In the stratosphere CH4 becomes isotopically enriched towards higher altitudes. When CH4 is ascending in the atmosphere it is exposed to oxidation. Due to fractionation processes, heavy CH4 isotopologues are unfavoured and therefore accumulate in the remaining CH4.
Our simulation results compare well to observations. For example, isotopic observations from the National Oceanic and Atmospheric Administration/Earth System Research Laboratory (NOAA/ESRL) sampling sites (White et al., 2016, 2017) and airborne samples taken during the Comprehensive Observation Network for TRace gases by AIrLiner (CONTRAIL) project (Umezawa et al., 2012) verify the north–south gradient (shown Sect. S2.1 and S2.2 in the Supplement). The values of the signature of 13C in CH4 (δ13C(CH4)), for example, are within the uncertainty of the CONTRAIL observations. The signature of D in CH4 (δD(CH4)) is isotopologically depleted in D compared to the CONTRAIL observations; however, it still captures the gradient well. The vertical gradient (i.e. isotopical enrichment in the stratosphere) can be verified by comparing it with balloon-borne observations by Röckmann et al. (2011). Our simulation results are thereby within the local and temporal uncertainties (shown in Sect. S2.3 in the Supplement). Note that an optimization with respect to source signatures is yet to be made and requires an optimized emission inventory. However, the capturing of the respective gradients indicates that the isotopical fractionation is sufficiently implemented.
5.3 Coupling of the CH4 isotopologues to the isotopological hydrological cycle
The previously shown results were achieved with the CH4 submodel, including the option to simulate CH4 isotopologues. The produced HDO (by oxidation of CH3D) is connected via the TRSYNC submodel to the isotopological hydrological cycle represented by the H2OISO submodel. We carried out an additional simulation in which we applied MECCA and MECCA_TAG to simulate the atmospheric chemistry and the CH4 isotopologues instead of the CH4 submodel. In this simulation TRSYNC likewise connects the produced HDO to the isotopological water tracers of H2OISO.
In Fig. 6 we compare the results obtained with submodel CH4 (left) and those obtained with the submodel MECCA_TAG (right) to vertical profiles of H2O and HDO (middle) provided by the Michelson Interferometer for Passive Atmospheric Sounding (MIPAS) instrument mounted on the ENVIronmental SATellite (ENVISAT, Steinwagner et al., 2007; Lossow et al., 2011). The ENVISAT satellite is on a sun-synchronous orbit around the Earth, completing the circuit 14 times a day. The presented observational and simulated data comprise the time period July 2002 to March 2004. The vertical range of the observations extends from 6 to 68 km (i.e. approx. the range 100–1 hPa) with a vertical resolution of 3–8 km. Simulation and observation data are monthly and zonally averaged over the tropics. Similar to the conclusions of Eichinger et al. (2015a) it is observed that the EMAC model underestimates the H2O mixing ratio (see Figs. 6a and c). This is associated with a too cold tropopause in EMAC, where a temperature bias of −2 to −6 K is detected in the upper troposphere, as long as the mean temperature is excluded from the nudging procedure defining the specified dynamics set-up (Jöckel et al., 2016). This reduces the H2O transported into the stratosphere since more gas-phase H2O freezes and sediments. Comparing Fig. 6d with f indicates a better agreement concerning the signature of D in H2O (δD(H2O)) in the simulation using the submodel MECCA_TAG with the MIPAS observations, which suggests that although the absolute H2O and HDO mixing ratios are not met, the relative composition is well represented. The differences in HDO in the simulation with the CH4 submodel compared to the one with the MECCA_TAG submodel and MIPAS are potentially caused by (1) the Eq. (9) from Eichinger et al. (2015a) used in the simulation using the CH4 submodel, which possibly does not capture important fractionation processes in the oxidation chain of CH3D, and (2) the HD produced in the troposphere and propagating into the stratosphere, which is not included in the simplified chemistry but represents an additional source of HDO. For an accurate simulation of stratospheric HDO this source also needs to be considered in future simulations.
The submodel CH4 provides a reduced chemical set-up focusing on the CH4 sink reactions using predefined data of reaction partners and optionally includes the feedback on SWV. This reduces the computational demands of sensitivity simulations of climate projections without neglecting the main source of chemically induced SWV.
We present two additional options of the CH4 submodel. The age and emission classes allow the inverse optimization of emission inventories using a fixed-lag Kalman filter. The simulation of CH4 isotopologues provides further insight into the variability and distribution of CH4 from its source (via emission signatures and fractionation effects) to its sink (coupling to the isotopic content of H2O). The latter is implemented in form of the new submodel TRSYNC, which takes care of the correct and time-integration-conform synchronization of the various H2O isotopologue tracers in the model.
Example use cases show specific applications of the CH4 submodel and the coupling to the isotopological hydrological cycle via the TRSYNC submodel, which is especially helpful for the closure of the isotopic content in SWV.
The Modular Earth Submodel System (MESSy) is continuously further developed and applied by a consortium of institutions. The usage of MESSy and access to the source code is licensed to all affiliates of institutions that are members of the MESSy Consortium. Institutions can become a member of the MESSy Consortium by signing the MESSy Memorandum of Understanding. More information can be found on the MESSy Consortium website (http://www.messy-interface.org, last access: 18 January 2021). The new submodels presented in this paper have been implemented based on MESSy v2.53.0 and have been available since v2.54.0. The exact code version used to produce the examples is archived at the German Climate Computing Center (Deutsches Klimarechenzentrum, DKRZ) and can be made available to members of the MESSy community upon request.
The supplement related to this article is available online at: https://doi.org/10.5194/gmd-14-661-2021-supplement.
FW and PJ worked on the development of the CH4 and TRSYNC submodels and wrote the manuscript.
The authors declare that they have no conflict of interest.
The model simulations have been performed at the German Climate Computing Centre (DKRZ) through support from the Bundesministerium für Bildung und Forschung (BMBF). We further thank Theresa Klausner and two anonymous reviewers for their supportive comments on the manuscript.
The DLR project KliSAW (Klimarelevanz von atmosphärischen Spurengasen, Aerosolen und Wolken) and the Helmholtz-Gemeinschaft e.V. (HGF) “Project Advanced Earth System Modelling Capacity (ESM)” provided the financial basis for the presented model developments.
The article processing charges for this open-access publication were covered by a Research Centre of the Helmholtz Association.
This paper was edited by Fiona O'Connor and reviewed by two anonymous referees.
Austin, J., Wilson, J., Li, F., and Vömel, H.: Evolution of Water Vapor Concentrations and Stratospheric Age of Air in Coupled Chemistry-Climate Model Simulations, B. Am. Meteorol. Soc., 64, 905–921, https://doi.org/10.1175/JAS3866.1, 2007. a
Bergamaschi, P., Brühl, C., Brenninkmeijer, C. A. M., Saueressig, G., Crowley, J. N., Grooß, J. U., Fischer, H., and Crutzen, P. J.: Implications of the large carbon kinetic isotope effect in the reaction CH4 + Cl for the 13C∕12C ratio of stratospheric CH4, Geophys. Res. Lett., 23, 2227–2230, https://doi.org/10.1029/96GL02139, 1996. a
Bigeleisen, J.: Isotope Effects in Chemistry and Biology, chap. 01, Theoretical Basis of Isotope Effects from an Autobiographical Perspective, Taylor and Taylor and Francis Group, LLC, 1–40, https://doi.org/10.1201/9781420028027, 2005. a
Boville, B. A., Kiehl, J. T., Rasch, P. J., and Bryan, F. O.: Improvements to the NCAR CSM-1 for Transient Climate Simulations, J. Climate, 14, 164–179, https://doi.org/10.1175/1520-0442(2001)014<0164:ITTNCF>2.0.CO;2, 2001. a
Bruhwiler, L. M. P., Michalak, A. M., Peters, W., Baker, D. F., and Tans, P.: An improved Kalman Smoother for atmospheric inversions, Atmos. Chem. Phys., 5, 2691–2702, https://doi.org/10.5194/acp-5-2691-2005, 2005. a
Crowley, J. N., Saueressig, G., Bergamaschi, P., Fischer, H., and Harris, G. W.: Carbon kinetic isotope effect in the reaction CH4+Cl: a relative rate study using FTIR spectroscopy, Chem. Phys. Lett., 303, 268–274, https://doi.org/10.1016/S0009-2614(99)00243-2, 1999. a, b
Dee, D. P., Uppala, S. M., Simmons, A. J., Berrisford, P., Poli, P., Kobayashi, S., Andrae, U., Balmaseda, M. A., Balsamo, G., Bauer, P., Bechtold, P., Beljaars, A. C. M., van de Berg, L., Bidlot, J., Bormann, N., Delsol, C., Dragani, R., Fuentes, M., Geer, A. J., Haimberger, L., Healy, S. B., Hersbach, H., Hólm, E. V., Isaksen, L., Kållberg, P., Köhler, M., Matricardi, M., McNally, A. P., Monge-Sanz, B. M., Morcrette, J.-J., Park, B.-K., Peubey, C., de Rosnay, P., Tavolato, C., Thépaut, J.-N., and Vitart, F.: The ERA-Interim reanalysis: configuration and performance of the data assimilation system, Q. J. Roy. Meteor. Soc., 137, 553–597, https://doi.org/10.1002/qj.828, https://doi.org/10.1002/qj.828, 2011. a
ECMWF: IFS DOCUMENTATION – Cy31r1, Part IV: Physical Processes, available at: https://www.ecmwf.int/sites/default/files/elibrary/2007/9221-part-iv-physical-processes.pdf (last access: 18 January 2021), 2007. a
Eichinger, R.: Investigation of stratospheric water vapour by means of the simulation of water isotopologues, PhD thesis, Ludwig Maximilian Universität München, München, 2014. a
Eichinger, R., Jöckel, P., Brinkop, S., Werner, M., and Lossow, S.: Simulation of the isotopic composition of stratospheric water vapour Part 1: Description and evaluation of the EMAC model, Atmos. Chem. Phys., 15, 5537–5555, https://doi.org/10.5194/acp-15-5537-2015, 2015a. a, b, c, d, e, f, g
Eichinger, R., Jöckel, P., and Lossow, S.: Simulation of the isotopic composition of stratospheric water vapour Part 2: Investigation of HDO∕H2O variations, Atmos. Chem. Phys., 15, 7003–7015, https://doi.org/10.5194/acp-15-7003-2015, 2015b. 2015b. a
Fletcher, M., E., S., Tans, P. P., Bruhwiler, L. M., Miller, J. B., and Heimann, M.: CH4 sources estimated from atmospheric observations of CH4 and its 13C∕12C isotopic ratios: 2. Inverse modeling of CH4 fluxes from geographical regions, Global Biogeochem. Cy., 18, 1–15, https://doi.org/10.1029/2004GB002224, 2004. a
Frank, F.: Atmospheric methane and its isotopic composition in a changing climate: A modelling study, PhD thesis, Ludwigs Maximillian Universität München, München, 2018. a, b
Frank, F., Jöckel, P., Gromov, S., and Dameris, M.: Investigating the yield of H2O and H2 from methane oxidation in the stratosphere, Atmos. Chem. Phys., 18, 9955–9973, https://doi.org/10.5194/acp-18-9955-2018, 2018. a
Gromov, S., Jöckel, P., Sander, R., and Brenninkmeijer, C. A. M.: A kinetic chemistry tagging technique and its application to modelling the stable isotopic composition of atmospheric trace gases, Geosci. Model Dev., 3, 337–364, https://doi.org/10.5194/gmd-3-337-2010, 2010. a
Hein, R., Crutzen, P. J., and Heimann, M.: An inverse modeling approach to investigate the global atmospheric methane cycle, Global Biogeochem. Cy., 11, 43–76, 1997. a
Holmgren, P.: Global land use area change matrix, Working Paper 134, Forest Resources Assessment Programme, Food and Agriculture Organization of the United Nations, Rome, Italy, 2006. a
IPCC: Climate Change 2013: The Physical Science Basis. Contribution of Working Group I to the Fifth Assessment Report of the Intergovernmental Panel on Climate Change, Cambridge University Press, Cambridge, UK and New York, NY, USA, https://doi.org/10.1017/CBO9781107415324, 2013. a, b, c
Jöckel, P., Kerkweg, A., Pozzer, A., Sander, R., Tost, H., Riede, H., Baumgaertner, A., Gromov, S., and Kern, B.: Development cycle 2 of the Modular Earth Submodel System (MESSy2), Geosci. Model Dev., 3, 717–752, https://doi.org/10.5194/gmd-3-717-2010, 2010. a, b, c, d
Jöckel, P., Tost, H., Pozzer, A., Kunze, M., Kirner, O., Brenninkmeijer, C. A. M., Brinkop, S., Cai, D. S., Dyroff, C., Eckstein, J., Frank, F., Garny, H., Gottschaldt, K.-D., Graf, P., Grewe, V., Kerkweg, A., Kern, B., Matthes, S., Mertens, M., Meul, S., Neumaier, M., Nützel, M., Oberländer-Hayn, S., Ruhnke, R., Runde, T., Sander, R., Scharffe, D., and Zahn, A.: Earth System Chemistry integrated Modelling (ESCiMo) with the Modular Earth Submodel System (MESSy) version 2.51, Geosci. Model Dev., 9, 1153–1200, https://doi.org/10.5194/gmd-9-1153-2016, 2016. a, b, c, d, e
Kerkweg, A., Buchholz, J., Ganzeveld, L., Pozzer, A., Tost, H., and Jöckel, P.: Technical Note: An implementation of the dry removal processes DRY DEPosition and SEDImentation in the Modular Earth Submodel System (MESSy), Atmos. Chem. Phys., 6, 4617–4632, https://doi.org/10.5194/acp-6-4617-2006, 2006a. a, b
Kerkweg, A., Sander, R., Tost, H., and Jöckel, P.: Technical note: Implementation of prescribed (OFFLEM), calculated (ONLEM), and pseudo-emissions (TNUDGE) of chemical species in the Modular Earth Submodel System (MESSy), Atmos. Chem. Phys., 6, 3603–3609, https://doi.org/10.5194/acp-6-3603-2006, 2006b. a, b, c
King, G.: Responses of atmospheric methane consumption by soils to global climate change, Glob. Change Biol., 3, 351–362, https://doi.org/10.1046/j.1365-2486.1997.00090.x, 1997. a
Kirschke, S., Bousquet, P., Ciais, P., Saunois, M., Canadell, J. G., Dlugokencky, E. J., Bergamaschi, P., Bergmann, D., Blake, D. R., Bruhwiler, L., Cameron-Smith, P., Castaldi, S., Chevallier, F., Feng, L., Fraser, A., Heimann, M., Hodson, E. L., Houweling, S., Josse, B., Fraser, P. J., Krummel, P. B., Lamarque, J.-F., Langenfelds, R. L., Le Quèrè, C., Naik, V., O'Doherty, S., Palmer, P. I., Pison, I., Plummer, D., Poulter, B., Prinn, R. G., Rigby, M., Ringeval, B., Santini, M., Schmidt, M., Shindell, D. T., Simpson, I. J., Spahni, R., Steele, L. P., Strode, S. A., Sudo, K., Szopa, S., van der Werf, G. R., Voulgarakis, A., van Weele, M., Weiss, R. F., Williams, J. E., and Zeng, G.: Three decades of global methane sources and sinks, Nat. Geosci., 6, 813–823, https://doi.org/10.1038/ngeo1955, 2013. a
le Texier, H., Solomon, S., and Garcia, R. R.: The role of molecular hydrogen and methane oxidation in the water vapour budget of the stratosphere, Q. J. Roy. Meteor. Soc., 114, 281–295, https://doi.org/10.1002/qj.49711448002, 1988. a
Lossow, S., Steinwagner, J., Urban, J., Dupuy, E., Boone, C. D., Kellmann, S., Linden, A., Kiefer, M., Grabowski, U., Glatthor, N., Höpfner, M., Röckmann, T., Murtagh, D. P., Walker, K. A., Bernath, P. F., von Clarmann, T., and Stiller, G. P.: Comparison of HDO measurements from Envisat/MIPAS with observations by Odin/SMR and SCISAT/ACE-FTS, Atmos. Meas. Tech., 4, 1855–1874, https://doi.org/10.5194/amt-4-1855-2011, 2011. a
Maxfield, P. J., Evershed, R. P., and Hornibrook, E. R. C.: Physical and Biological Controls on the In Situ Kinetic Isotope Effect Associated with Oxidation of Atmospheric CH4 in Mineral Soils, Environ. Sci. Technol., 42, 7824–7830, https://doi.org/10.1021/es800544q, 2008. a, b
Monge-Sanz, B. M., Chipperfield, M. P., Untch, A., Morcrette, J.-J., Rap, A., and Simmons, A. J.: On the uses of a new linear scheme for stratospheric methane in global models: water source, transport tracer and radiative forcing, Atmos. Chem. Phys., 13, 9641–9660, https://doi.org/10.5194/acp-13-9641-2013, 2013. a
Monteil, G., Houweling, S., Dlugockenky, E. J., Maenhout, G., Vaughn, B. H., White, J. W. C., and Rockmann, T.: Interpreting methane variations in the past two decades using measurements of CH4 mixing ratio and isotopic composition, Atmos. Chem. Phys., 11, 9141–9153, https://doi.org/10.5194/acp-11-9141-2011, 2011. a
Mote, P.: The annual cycle of stratospheric water vapor in a general circulation model, J. Geophys. Res., 100, 7363–7379, https://doi.org/10.1029/94JD03301, 1995. a
Nair, H., Summers, M. E., Miller, C. E., and Yung, Y. L.: Isotopic fractionation of methane in the martian atmosphere, ICARUS, 175, 32–35, https://doi.org/10.1016/j.icarus.2004.10.018, 2005. a
Nickl, A.-L., Mertens, M., Roiger, A., Fix, A., Amediek, A., Fiehn, A., Gerbig, C., Galkowski, M., Kerkweg, A., Klausner, T., Eckl, M., and Jöckel, P.: Hindcasting and forecasting of regional methane from coal mine emissions in the Upper Silesian Coal Basin using the online nested global regional chemistryclimate model MECO(n) (MESSy v2.53), Geosci. Model Dev., 13, 1925–1943, https://doi.org/10.5194/gmd-13-1925-2020, 2020. a
Nisbet, E. G., Dlugokencky, E. J., Manning, M. R., Lowry, D., Fisher, R. E., France, J. L., Michel, S. E., Miller, J. B., White, J. W. C., Vaughn, B., Bousquet, P., Pyle, J. A., Warwick, N. J., Cain, M., Brownlow, R., Zazzeri, G., Lanoisellé, M., Manning, A. C., Gloor, E., Worthy, D. E. J., Brunke, E.-G., Labuschangne, C., W., W. E., and Ganesan, A. L.: Rising atmospheric methane: 2007–2014 growth and isotopic shift, Global Biogeochem. Cy., 30, 1356–1370, https://doi.org/10.1002/2016GB00540, 2016. a
Nixon, C. A., Temelso, B., Vinatier, S., Teanby, N. A., B'ezard, B., Achterberg, R. K., Mandt, K. E., Sherrill, C. D., Irwin, P. G. J., Jennings, D. E., Romani, P. N., Coustenis, A., and Flasar, F. M.: Isotopic ratios in titan's methane: measurements and modeling, Astrophys. J., 749, 159, https://doi.org/10.1088/0004-637X/749/2/159, 2012. a
Olivier, J. and Janssens-Maenhout, G.: CO2 Emissions from Fuel Combustion 2012, chap. Part III, Greenhouse-Gas Emissions, p. 540, International Energy Agency, https://doi.org/10.1787/co2_fuel-2012-en, 2012. a
Revell, L. E., Bodeker, G. E., Huck, P. E., Williamson, B. E., and Rozanov, E.: The sensitivity of stratospheric ozone changes through the 21st century to N2O and CH4, Atmos. Chem. Phys., 12, 11309–11317, https://doi.org/10.5194/acp-12-11309-2012, 2012. a
Rigby, M., Manning, A. J., and Prinn, R. G.: The value of high-frequency high-precision methane isotopologue measurements for source and sink estimation, J. Geophys. Res., 117, D12312, https://doi.org/10.1029/2011JD017384, 2012. a
Röckmann, T., Brass, M., Borchers, R., and Engel, A.: The isotopic composition of methane in the stratosphere: high-altitude balloon sample measurements, Atmos. Chem. Phys., 11, 13287–13304, https://doi.org/10.5194/acp-11-13287-2011, 2011. a
Roeckner, E., Brokopf, R., Esch, M., Giorgetta, M., Hagemann, S., Kornblueh, L., Manzini, E., Schlese, U., and Schulzweida, U.: Sensitivity of Simulated Climate to Horizontal and Vertical Resolution in the ECHAM5 Atmosphere Model, B. Am. Meteorol. Soc., 19, 3771–3791, 2006. a
Sander, R., Kerkweg, A., Jöckel, P., and Lelieveld, J.: Technical note: The new comprehensive atmospheric chemistry module MECCA, Atmos. Chem. Phys., 5, 445–450, https://doi.org/10.5194/acp-5-445-2005, 2005. a
Sander, R., Jöckel, P., Kirner, O., Kunert, A. T., Landgraf, J., and Pozzer, A.: The photolysis module JVAL-14, compatible with the MESSy standard, and the JVal PreProcessor (JVPP), Geosci. Model Dev., 7, 2653–2662, https://doi.org/10.5194/gmd-7-2653-2014, 2014. a, b
Sander, S. P., Abbatt, J., Barker, J. R., Burkholder, J. B., Friedl, R. R., Golden, D. M., Huie, R. E., Kolb, C. E., Kurylo, M. J., Moortgat, G. K., Orkin, V. L., and Wine, P. H.: Chemical Kinetics and Photochemical Data for Use in Atmospheric Studies, Evaluation No. 17, JPL Publication 10-6, Jet Propulsion Laboratory, https://doi.org/10.1002/kin.550171010, 2011. a
Saueressig, G., Bergamaschi, P., Crowley, J. N., and Fischer, H.: Carbon kinetic isotope effect in the reaction of CH4 with Cl atoms, Geophys. Res. Lett., 22, 1225–1228, 1995. a, b
Saueressig, G., Bergamaschi, P., Crowley, J., and Fischer, H.: D/H kinetic isotope effect in the reaction CH4 + Cl, Geophys. Res. Lett., 23, 3619–3622, 1996. a, b, c
Saueressig, G., Crowley, J. N., Bergamaschi, P., Brühl, C., Brenninkmeijer, C. A. M., and Fischer, H.: Carbon 13 and D kinetic isotope effects in the reactions of CH4 with O1(D) and OH: New laboratory measurements and their implications for the isotopic composition of stratospheric methane, J. Geophys. Res., 106, 23127–23138, 2001. a, b, c, d, e, f
Saunois, M., Bousquet, P., Poulter, B., Peregon, A., Ciais, P., Canadell, J. G., Dlugokencky, E. J., Etiope, G., Bastviken, D., Houweling, S., Janssens-Maenhout, G., Tubiello, F. N., Castaldi, S., Jackson, R. B., Alexe, M., Arora, V. K., Beerling, D. J., Bergamaschi, P., Blake, D. R., Brailsford, G., Brovkin, V., Bruhwiler, L., Crevoisier, C., Crill, P., Covey, K., Curry, C., Frankenberg, C., Gedney, N., Höglund-Isaksson, L., Ishizawa, M., Ito, A., Joos, F., Kim, H.-S., Kleinen, T., Krummel, P., Lamarque, J.-F., Langenfelds, R., Locatelli, R., Machida, T., Maksyutov, S., McDonald, K. C., Marshall, J., Melton, J. R., Morino, I., Naik, V., O'Doherty, S., Parmentier, F.-J. W., Patra, P. K., Peng, C., Peng, S., Peters, G. P., Pison, I., Prigent, C., Prinn, R., Ramonet, M., Riley, W. J., Saito, M., Santini, M., Schroeder, R., Simpson, I. J., Spahni, R., Steele, P., Takizawa, A., Thornton, B. F., Tian, H., Tohjima, Y., Viovy, N., Voulgarakis, A., van Weele, M., van der Werf, G. R., Weiss, R., Wiedinmyer, C., Wilton, D. J., Wiltshire, A., Worthy, D., Wunch, D., Xu, X., Yoshida, Y., Zhang, B., Zhang, Z., and Zhu, Q.: The global methane budget 2000–2012, Earth Syst. Sci. Data, 8, 697–751, https://doi.org/10.5194/essd-8-697-2016, 2016. a
Schaefer, H., Fletcher, S. E. M., Veidt, C., Lassey, K. R., Brailsford, G. W., Bromley, T. M., Dlugokencky, E. J., Michel, S. E., Miller, J. B., Levin, I., Lowe, D. C., Martin, R. J., Vaughn, B. H., and White, J. W. C.: A 21st century shift from fossil-fuel to biogenic methane emissions indicated by 13CH4, Science, 352, 80–84, https://doi.org/10.1126/science.aad2705, 2016. a
Snover, A. and Quay, P.: Hydrogen and carbon kinetic isotope effects during soil uptake of atmospheric methane and a temperate grassland, Global Biogeochem. Cy., 14, 25–39, 2000. a, b
Solomon, S., Rosenlof, K. H., Portmann, R. W., Daniel, J. S., Davis, S. M., Sanford, T. J., and Plattner, G.-K.: Contributions of Stratospheric Water Vapor to Decadal Changes in the Rate of Global Warming, Science, 327, 1219–1223, https://doi.org/10.1126/science.1182488, 2010. a
Steinwagner, J., Milz, M., von Clarmann, T., Glatthor, N., Grabowski, U., Höpfner, M., Stiller, G. P., and Röckmann, T.: HDO measurements with MIPAS, Atmos. Chem. Phys., 7, 2601–2615, https://doi.org/10.5194/acp-7-2601-2007, 2007. a
Stenke, A. and Grewe, V.: Simulation of stratospheric water vapor trends: impact on stratospheric ozone chemistry, Atmos. Chem. Phys., 5, 1257–1272, https://doi.org/10.5194/acp-5-1257-2005, 2005. a
Stolper, D., Sessions, A., Ferreira, A., Neto, E. S., Schimmelmann, A., Shusta, S., Valentine, D., and Eiler, J.: Combined C–D and D–D clumping in methane: Methods and preliminary results, Geochim. Cosmochim. Ac., 126, 169–191, https://doi.org/10.1016/j.gca.2013.10.045, 2014. a
Tian, W., Chipperfield, M. P., and Lü, D.: Impact of increasing stratospheric water vapor on ozone depletion and temperature change, Adv. Atmos. Sci., 26, 423–437, https://doi.org/10.1007/s00376-009-0423-3, 2009. a
Tost, H., Jöckel, P., Kerkweg, A., Sander, R., and Lelieveld, J.: Technical note: A new comprehensive SCAVenging submodel for global atmospheric chemistry modelling, Atmos. Chem. Phys., 6, 565–574, https://doi.org/10.5194/acp-6-565-2006, 2006. a
Umezawa, T., Machida, T., Ishijima, K., Matsueda, H., Sawa, Y., Patra, P. K., Aoki, S., and Nakazawa, T.: Carbon and hydrogen isotopic ratios of atmospheric methane in the upper troposphere over the Western Pacific, Atmos. Chem. Phys., 12, 8095–8113, https://doi.org/10.5194/acp-12-8095-2012, 2012. a
White, J., Vaughn, B., and Michel, S.: University of Colorado, Institute of Arctic and Alpine Research (INSTAAR), Stable Isotopic Composition of Atmospheric Methane (2H) from the NOAA ESRL Carbon Cycle Cooperative Global Air Sampling Network, 2005–2009, Version: 2016-04-26, available at: ftp://aftp.cmdl.noaa.gov/data/trace_gases/ch4h2/flask/ (last access: 18 January 2021), 2016. a
White, J., Vaughn, B., and Michel, S.: University of Colorado, Institute of Arctic and Alpine Research (INSTAAR), Stable Isotopic Composition of Atmospheric Methane (13C) from the NOAA ESRL Carbon Cycle Cooperative Global Air Sampling Network, 1998–2016, Version: 2018-01-31, ftp://aftp.cmdl.noaa.gov/data/trace_gases/ch4c13/flask/ (last access: 18 January 2021), 2017. a
Winterstein, F., Tanalski, F., Jöckel, P., Dameris, M., and Ponater, M.: Implication of strongly increased atmospheric methane concentrations for chemistryclimate connections, Atmos. Chem. Phys., 19, 7151–7163, https://doi.org/10.5194/acp-19-7151-2019, 2019. a, b
node-h: required wall-clock hours times applied HPC nodes.
- Abstract
- Introduction
- The Modular Earth Submodel System (MESSy)
- The submodel CH4
- Coupling to the hydrological cycle with the new submodel TRSYNC
- Example applications
- Summary
- Code and data availability
- Author contributions
- Competing interests
- Acknowledgements
- Financial support
- Review statement
- References
- Supplement
- Abstract
- Introduction
- The Modular Earth Submodel System (MESSy)
- The submodel CH4
- Coupling to the hydrological cycle with the new submodel TRSYNC
- Example applications
- Summary
- Code and data availability
- Author contributions
- Competing interests
- Acknowledgements
- Financial support
- Review statement
- References
- Supplement