the Creative Commons Attribution 4.0 License.
the Creative Commons Attribution 4.0 License.
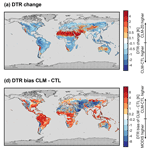
Impacts of a revised surface roughness parameterization in the Community Land Model 5.1
Ronny Meier
Gordon B. Bonan
David M. Lawrence
Xiaolong Hu
Gregory Duveiller
Catherine Prigent
Sonia I. Seneviratne
The roughness of the land surface (z0) is a key property, exerting significant influence on the amount of near-surface turbulent activity and consequently the turbulent exchange of energy, water, momentum, and chemical species between the land and the atmosphere. Variations in z0 are substantial across different types of land cover, ranging from typically less than 1 mm over fresh snow or sand deserts up to more than 1 m over urban areas or forests. In this study, we revise the parameterizations and parameter choices related to z0 in the Community Land Model 5.1 (CLM), the land component of the Community Earth System Model (CESM). We propose a number modifications for z0 in CLM, guided by observational data. Most importantly, we find that the observations support an increase in z0 for all types of forests and a decrease in the momentum z0 for bare soil, snow, glaciers, and crops. We then assess the effect of those modifications in land-only and land–atmosphere coupled simulations. With the revised parameterizations, diurnal variations of the land surface temperature (LST) are dampened in forested regions and are amplified over warm deserts. These changes mitigate model biases compared to MODerate resolution Imaging Spectroradiometer (MODIS) remote sensing observations. The changes in LST are generally stronger during the day than at night. For example, the LST increases by 5.1 K at 13:30 local solar time but only by 0.6 K at 01:30 during boreal summer across the entire Sahara. The induced changes in the diurnal variability of near-surface air temperatures are generally of the opposite sign and of smaller magnitude. Near-surface winds accelerate in areas where the momentum z0 was lowered, such as the Sahara, the Middle East, and Antarctica, and decelerate in regions with forests. Overall, this study finds that the current representation of z0 in CLM is not in agreement with observational constraints for several types of land cover. The proposed model modifications are shown to considerably alter the simulated climate in terms of temperatures and wind speed at the land surface.
- Article
(20029 KB) - Full-text XML
- BibTeX
- EndNote
The land surface interacts in numerous ways with the atmosphere. Among the most relevant interactions is the turbulent exchange of sensible heat, water vapor, momentum, and chemical species at the land–atmosphere interface. Turbulence above the land surface occurs due to the retardation of moving air by friction and due to the buoyancy created by surface heating from solar irradiance (Bonan, 2019). The intensity of the turbulence generated by friction is determined by the amount and shape of obstacles on land in concert with atmospheric conditions. In land models, the turbulent exchange with the atmosphere is commonly represented through the Monin–Obukhov similarity theory (MOST). A key parameter in MOST is the aerodynamic or momentum surface roughness, z0m. A rough surface, such as an urban environment or a forest, is characterized by a higher z0m and therefore induces more turbulence for a given wind speed than a smooth surface, such as a snow field or a lake. Similar surface roughness parameters exist for the exchange of scalars (e.g., temperature and water vapor). Observed values of z0m over land span more than 4 orders of magnitude with values ranging from a few tenths of a millimeter over fresh snow (Brock et al., 2006) or bare soil (Prigent et al., 2005) to several meters over forests (Hu et al., 2020) or urban areas (Kanda et al., 2013).
The momentum surface roughness is defined as the height above the displacement height (d) where the average wind speed extrapolates to zero under neutral conditions. The displacement height accounts for the fact that large roughness elements, such as trees or buildings, may shift the logarithmic wind speed profile (which occurs under neutral conditions) upwards, such that mean wind speed extrapolates to zero at the height z0m+d rather than z0m. Similarly, the sensible heat (z0h) and the latent heat (z0q) roughness lengths are defined as the heights above d where the air temperature and the specific humidity reach their respective surface value under neutral conditions. In the surface sublayer, a thin layer of air directly adjacent to the surface of typically 10−3 to 10−1 m thickness, water vapor and heat are transported solely through molecular diffusion, while momentum exchange is also facilitated by pressure fluctuations that are induced by the presence of roughness elements (Zeng and Dickinson, 1998). As a result, the turbulent exchange of sensible and latent heat between the land and the atmosphere is generally less efficient than the exchange of momentum. Accordingly, z0h and z0q are often much smaller than z0m (Yang et al., 2002, 2008; Hu et al., 2020).
In the field, z0 is commonly estimated through four main methods. The first approach is to measure the vertical wind speed profile and link these measurements to z0m using the equation below (e.g., Greeley et al., 1997; Brock et al., 2006; Marticorena et al., 2006; Nakai et al., 2008; Hugenholtz et al., 2013; Kanda et al., 2013; Nield et al., 2013; Fitzpatrick et al., 2019). The wind speed profile is logarithmic under neutral conditions over a plain surface:
where u(z) is the mean wind speed profile, z is the height above the surface, u* is the friction velocity, and κ is the von Karman constant (=0.4). This approach can also be used to estimate z0h and z0q through measurements of the temperature and specific humidity profiles. A second method is to use eddy covariance measurements of the momentum, the sensible heat, and latent heat fluxes, which can then be used to deduce the z0m, z0h, and z0q that conform best with the measured fluxes according to MOST (e.g., Maurer et al., 2013; Li et al., 2015; Hu et al., 2020). A third method involves using measurements of the microtopography to link z0m to small-scale variations of the height of the surface (e.g., Brock et al., 2006; Weligepolage et al., 2012; Hugenholtz et al., 2013; Fitzpatrick et al., 2019; van Tiggelen et al., 2021). The final approach uses remote sensing observations of either backscattering at the land surface or the surface reflectance to serve as a proxy for microtopography to estimate z0m (e.g., Greeley et al., 1997; Marticorena et al., 2004; Prigent et al., 2005, 2012; Stilla et al., 2020). This latter approach requires some in situ measurements of z0m to establish a relationship between the remotely sensed proxy and z0m.
The surface roughness plays a central role for atmospheric dynamics (Sud et al., 1988; Vautard et al., 2010; Wever, 2012), energy fluxes at the land surface, and thereby temperatures at the land surface (Zeng and Dickinson, 1998; Zeng and Wang, 2007). Several studies have linked deficiencies of various models to a misrepresentation of z0 (Chen et al., 2010; Subin et al., 2012; Zeng et al., 2012; Wang et al., 2014; Trigo et al., 2015; Xu et al., 2016; Wang et al., 2019). The aerodynamic z0 also affects the simulated mineral dust emissions (Menut et al., 2013), which absorb and reflect solar radiation and thereby alter temperatures at the land surface (Claquin et al., 1998; Miller and Tegen, 1998; Klose et al., 2021). Further, alterations in z0 due to de-, re-, and afforestation represent an important contribution to the biogeophysical effect of such land cover changes (Davin and de Noblet-Ducoudré, 2010; Lee et al., 2011; Burakowski et al., 2018; Belušić et al., 2019; Laguë et al., 2019; Winckler et al., 2019). Adequate parameterizations of z0 are therefore not only crucial to realistically simulate climate and weather but also to understand the biogeophysical effects of land cover changes.
In this study, we revise the representation of z0 in the Community Land Model version 5.1 (CLM; Lawrence et al., 2019), which is the land surface model of the Community Earth System Model (CESM; Danabasoglu et al., 2020). Our endeavors are motivated by an underestimation of diurnal variations in land surface temperature over arid and semi-arid regions in CLM/CESM (Zeng et al., 2012; Wang et al., 2014; Meier et al., 2019) as well as a seasonal cycle of z0 for broadleaf deciduous forests that appears to be in opposition to observational data, as will be shown in the next section. In Sect. 2, we compare the representation of z0 for each land cover type in CLM to observational data and parameterizations that have been proposed in the literature. Based on this comparison, we introduce five modifications to CLM: (1) a new parameterization of the vegetation z0 based on Raupach (1992) with optimized parameters to match the data collected in Hu et al. (2020) for different types of vegetation; (2) new globally constant z0m values for bare soil, snow, and glaciers based on field measurements; (3) inclusion of the parameterization of Yang et al. (2008) for z0h and z0q over bare soil, snow, and glaciers; (4) use of a spatially explicit z0m input field for bare soil based on the data of Prigent et al. (2005); and (5) inclusion of the Brock et al. (2006) parameterization of z0m for snow that is based on accumulated snowmelt. The latter two modifications replace the globally constant z0m values for bare soil and snow and may therefore be activated individually by the model user. To assess the impact of these modifications, we then conduct land-only and land–atmosphere coupled simulations, as described in Sect. 3, and evaluate them as described in Sect. 4. Section 5 describes how our modifications of z0 affect temperatures at the land surface and wind speed. We also confront the default and modified model configuration with MODerate resolution Imaging Spectroradiometer (MODIS) remote sensing observations of diurnal variations in the land surface temperature (LST) and the sensitivity of LST to a conversion of vegetation to bare land, based on the approach of Duveiller et al. (2018).
2.1 General description of CESM and CLM
The Community Earth System Model is a state-of-the-art Earth system model, which is widely applied in the field of climate science and has contributed to multiple multi-model intercomparison projects. Version 2 was released in June 2018 (Danabasoglu et al., 2020), followed by several incremental releases to version 2.1.2, which is used in this study. The development of CESM is coordinated and led by the National Center for Atmospheric Research (NCAR). However, a number of additional universities and research institutes contribute to the development of CESM. It is publicly available and well documented to facilitate this community effort (https://www.cesm.ucar.edu/models/cesm2/, last access: 21 February 2022). CESM comprises prognostic components for the atmosphere, ocean, land, sea ice, land ice, rivers, and waves. Besides these prognostic components, an observed data version exists for most components. In these versions, the coupling fields of the respective components are prescribed from recent observational data instead of running this component prognostically. CESM therefore allows us to flexibly disable or enable coupling of model components depending on the application.
The Community Land Model is the land component of CESM. It comprehensively represents the surface energy fluxes, the surface hydrology, and optionally the biogeochemical cycles for carbon and nitrogen (Lawrence et al., 2018, 2019). Up to five different land units may exist in each grid cell: (naturally) vegetated, lakes, urban, glaciers, and crops. A land unit tile can be further divided into different columns (e.g., rainfed and irrigated for crops) and patches (e.g., different types of natural vegetation). Bare soil, which can be found frequently in arid regions, is treated as a patch of natural vegetation. These patches of natural vegetation are called plant functional types (PFTs). Vegetation is simulated by a big-leaf approach (Sellers et al., 1986), distinguishing between sunlit and shaded leaves. The vegetation phenology can either be prescribed from remote-sensing-based data (satellite phenology), which are used in this study, or computed prognostically from the vegetation carbon pools, if the biogeochemical cycle is activated.
CLM5 distinguishes between vegetation, bare soil, snow, glacier ice, lakes, and urban areas in its parameterization of z0 (Lawrence et al., 2018). In the following sections, we describe the current representation of z0 in CLM and our proposed modifications, which we justify by comparison to the literature. As mentioned, z0m, z0h, and z0q correspond to the surface roughness for momentum, sensible heat, and latent heat, respectively. The land cover type is specified after a comma using “v”, “b”, “s”, “i”, and “g” for vegetated, bare soil, snow, ice (glaciers), and any type of ground (bare soil, snow, or ice), respectively (e.g., z0h,b is the sensible heat surface roughness of bare soil). Note that z0,v in CLM represents the aerodynamic roughness length for the turbulent exchange between the canopy air space and the free atmosphere. There is thus no distinction between z0m,v, z0h,v, and z0q,v, as this exchange occurs above the surface sublayer. However, there are additional resistances between the leaves/ground and the canopy air space to account for the surface resistance of the sensible and latent heat fluxes. A list of the symbols and abbreviations used in this study is provided in Table A2.
2.2 Vegetation
The current representation of z0,v and d was developed by Zeng and Wang (2007) and links these properties to the vegetation height (htop), the exposed leaf area index (LAI; i.e., the one-sided leaf area above the snow, if there is any), and the exposed stem area index (SAI; i.e., the one-sided stem and dead leaf area above the snow) as follows (Eqs. 2.5.125–127 in Lawrence et al., 2018):
where VAI is the vegetation area index defined as the sum of LAI and SAI, Rz0m and Rd are the ratios of z0,v and d to the canopy height for values of VAI exceeding the critical value VAIcr=2 m2 m−2, z0m,g is the momentum surface roughness of the ground (see Sect. 2.3–2.5), V is a fractional weight, and β=1. Rz0m is set to 0.075 for broadleaf evergreen trees, to 0.055 for other trees, and to 0.12 for grass, crops, and shrubs, while Rd is 0.67 for all trees and 0.68 for grass, crops, and shrubs. With this implementation, increases almost linearly with VAI before plateauing at the constant value Rz0m beyond VAIcr (red curves in the left column of Fig. 1).
Observations find a first-order linear relation between htop and z0,v as well as d (Tanner and Pelton, 1960). It is therefore common practice to normalize z0,v by htop when determining whether other vegetation properties influence z0,v (Shaw and Pereira, 1982; Yang and Friedl, 2003; Zhou et al., 2006; Nakai et al., 2008; Maurer et al., 2015). Proposed parameterizations hence frequently link and to other structural properties of the vegetation such as LAI, stand density, and/or crown width (Choudhury and Monteith, 1988; Raupach, 1992, 1994; Yang and Friedl, 2003; Nakai et al., 2008; Bingöl, 2019). For crops, z0,v exhibits a distinct seasonal cycle in the extratropics, with low values during winter, when crops are absent (blue and turquoise lines in Fig. 1m; Hu et al., 2020; Young et al., 2021). On the other hand, z0,v remains relatively high during the dormant phase if parts of the vegetation such as the stem and branches of trees persist throughout the year (Fig. 1b, d, and f; Dolman, 1986; Hu et al., 2020). In the case of broadleaf deciduous forests, there are even several studies that find a decrease in z0,v for higher values of LAI, probably because the dense canopies during the growing season shelter the branches and trunks of trees from the atmospheric flow (Nakai et al., 2008; Maurer et al., 2013; Young et al., 2021).
Hu et al. (2020) provide z0,v estimates for an extensive collection of FLUXNET sites, which offers an unprecedented opportunity to reconcile z0,v values observed in the field and the z0,v parameterizations in models. Here, we use an updated version of this data set for comparison to z0,v in CLM, which is subsequently referred to as Hu20. This updated version includes more FLUXNET sites than the original publication. Hu20 estimates daily z0,v values at a total of 113 FLUXNET sites by minimizing the following cost function J:
where is the measured friction velocity in the field and the estimated friction velocity according to MOST:
where u is the wind speed measured at the instrument height, zm, d is the displacement height estimated as two-thirds of htop, Ψm is the stability correction function for momentum transfer, and L is the Obukhov length scale. We divide the sites in Hu20 into the following six vegetation types: needleleaf forest, evergreen broadleaf forest, deciduous broadleaf forest, shrubland, grassland, and cropland. We make a number of additional suitability checks of the already quality-checked data, before using the data of Hu20 for comparison to CLM: (1) we exclude z0,v values that deviate by more than 2 standard deviations from the mean z0,v at the respective site; (2) we exclude z0,v values when htop=0, because we scale z0,v by htop in the next step; (3) we exclude sites that are not representative of the respective vegetation type according to a visual inspection on Google Maps (e.g., a sparse plantation); and (4) we remove sites with thin forest by excluding forest sites with a htop below 5 m and/or a maximum fractional vegetation cover below 0.8. Finally, we assign the forest sites designated as mixed forest to the most abundant type of forest according the species composition as described in the respective publication. In addition to the mean annual cycle of z0,v, we also consider the relationship between the VAI and in Hu20 to evaluate and revise the current parameterization in CLM. Hu20 provides LAI information but not SAI. Therefore, we extract from our CESM control simulation (Sect. 3) the monthly simulated SAI for the respective PFT and location, multiply it by the mean LAI at the site, and divide it by the mean LAI in CESM to estimate the SAI. Then, we collect all the estimates for the mentioned vegetation types, bin them into VAI bins of 0.2 m2 m−2, and compute the median in each bin (black points in Fig. 1). Finally, bins with fewer than 20 data samples are removed prior to the optimization process.
Compared to this data set, the current version of CLM overestimates the z0,v of crops and underestimates the z0,v for all other types of vegetation, in particular for forests (compare red and turquoise lines in Fig. 1). Further, CLM produces low values of z0,v in the absence of leaves for broadleaf deciduous forests, resulting in an annual cycle of z0,v that is in contradiction to Hu20 (Fig. 1f) and other observational studies (Nakai et al., 2008; Maurer et al., 2013; Young et al., 2021). Hu20 exhibits a peak in for intermediate values of VAI for most types of vegetation (left column of Fig. 1). The current parameterization for in CLM does not capture such behavior, with increasing monotonically with VAI before plateauing at a constant value (red lines in Fig. 1).
We therefore optimize the z0,v parameterization of Raupach (1992) and Raupach (1994), which is subsequently called Ra92, for the binned in Hu20. Ra92 was chosen over other proposed parameterizations for z0,v, because it (1) is appropriate for a broad range of vegetation densities (Raupach, 1992, 1994), (2) exhibits a similar shape for the relation between z0,v and the LAI as found by machine learning algorithms in Hu20, and (3) requires only htop and the single-sided area of all canopy elements as inputs describing the vegetation structure, which are both already present in CLM. Ra92 parameterizes the ratio of z0,v and htop as follows:
Here, Ψh is the roughness sublayer influence function, which is computed in Raupach (1994) as
where cw is a constant larger than 1 (Raupach, 1992). The ratio of the wind speed at canopy height, Uh, and u* is derived from an implicit function of the roughness density, λ:
Here, CS represents the drag coefficient of the ground in the absence of vegetation, CR is the drag coefficient of an isolated roughness element (plant), c is an empirical constant, and λmax is the maximum λ, above which becomes constant. The λmax is set to the value of λ for which Eq. (9) in the absence of λmax would have its minimum. Equation (9) can be written as
where .
X and thereby Uh/u* can be found iteratively:
and
We update X until it changes by less than from one iteration to the next during the optimization of Ra92 and in the implementation in CLM. As proposed in Raupach (1994), λ is set to half the total single-sided area of all canopy elements, here VAI. For numerical stability, VAI cannot be lower than m2 m−2 when computing λ:
For d, we use the parameterization proposed in Raupach (1994), which replaces Eq. (3):
where cd1=7.5. We then optimize the values of the parameters cw, CS, CR, and c so that they minimize the root-mean-square deviation (RMSD) in comparison to the median values in the different bins of VAI for each vegetation type. When computing the RMSD, we weight by the number of sites that contribute to the respective bins. We do not optimize cd1 because CLM exhibits little sensitivity to d and the effect of cd1 on z0,v is similar to that of CR and cw. The optimization is completed via a brute-force approach by testing 40 different values of the four fitted parameters over their realistic ranges and with the precision as shown on the bottom of Table 1. To assure numerical stability, we only test parameter combinations for which CS≤10 CR holds. Thus, we test a total of 1.312×106 () parameter combinations for each type of vegetation. The resultant fits of are depicted as orange lines in the left column of Fig. 1 and the parameter values in Table 1.
The optimized Ra92 parameterizations improve the mean annual cycle of z0,v for all vegetation types (compare orange to red lines in the right column of Fig. 1 in reference to turquoise lines). Notably, the z0,v of forests and shrubland, which was underestimated by the default z0,v parameterization, increases considerably. Further, the z0,v of crops is decreased by roughly a factor of 2. The z0,v of deciduous broadleaf forests decreases with higher VAI values in the data of Hu20. This relation is captured with the updated z0,v parameterization, resulting in a seasonal minimum of z0,v during summer as observed in the field. Given these clear improvements, the new parameterization of z0,v is added to the model code following Eqs. (7) to (13). The four parameters that were optimized for the different vegetation types are added to the parameter file of CLM/CESM and read in by the model at the start of a simulation. Besides these four parameters, λmax is also treated as a PFT-specific parameter in the revised model version. This is done to avoid requiring that the model compute for the full range of possible VAI values to find the minimum of every time z0,v is updated.
Table 1Fitted parameter values for Ra92. From left to right, vegetation type, number of sites from Hu20 assigned to this vegetation type, total number of daily z0,v estimates used to fit Ra92, and optimized values for CS, CR, c, cw, and the maximum VAI are shown. Below, tested range and precision used when fitting parameters of Ra92 are shown.
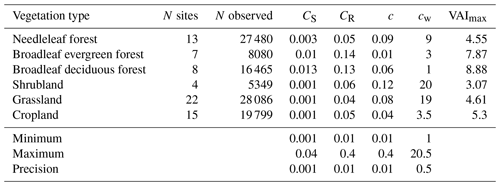
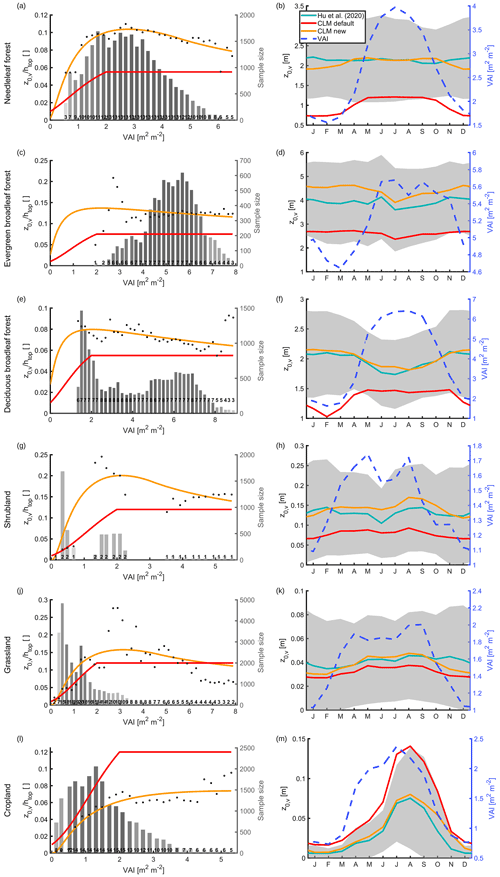
Figure 1Left column: median of Hu20 in VAI bins as black dots, red line the default z0,v parameterization of CLM, and orange line the optimized Ra92 parameterization. Height of gray bars shows the sample size in the respective bin and numbers at the bottom of the bars the number of sites that contributed to the respective bin. The darkness of the bars increases with an increasing fraction of total sites that are present in respective bin. Right column: monthly mean z0,v in Hu20 (turquoise), with default parameterization of CLM (red) and with optimized Ra92 parameterization (orange). Gray shading shows mean of Hu20 ± 1 standard deviation and dotted blue line mean seasonal cycle of VAI. Note that the data of sites south of 30∘ S were shifted by 6 months. (a–b) Needleleaf forests, (c–d) evergreen broadleaf forests, (e–f) deciduous broadleaf forests, (g–h) shrubland, (j–k) grassland, and (l–m) cropland.
2.3 Bare soil
CLM5 currently prescribes a z0m,b of 0.01 m (Lawrence et al., 2018). As mentioned above, z0h,b and z0q,b differ from z0m,b because scalar fluxes are not affected by the pressure fluctuations that are induced by the presence of the roughness elements. In CLM5, this is accounted for after Zeng and Dickinson (1998):
where a=0.13 and ν is the kinematic viscosity of air ( m2 s−1). Note that this equation is also used to compute z0h and z0q over snow and ice.
Observed z0m values over bare soil exhibit a wide range from m to m but are frequently around 0.001 m (Greeley et al., 1997; Callot et al., 2000; Marticorena et al., 2004, 2006; Hugenholtz et al., 2013; Nield et al., 2013). The default value of 0.01 m is within the observed range but lies at the upper end of it. To better determine the distribution of observed values, we synthesize z0m,b observed values reported in the literature (Fig. 2). Specifically, we use the data compiled in Table 1 of Prigent et al. (2005), sites S8 and S9 in Table 6, as well as the data compiled in Table 7 of Marticorena et al. (2006), and the reported values in Hugenholtz et al. (2013) and Nield et al. (2013), making sure that no value is counted twice. When a range is reported, we compute the average of this range (e.g., 0.001–0.005 m would be included as 0.003 m). We confirm that the 0.01 m value used in CLM is near the maximum among the observations from the literature (Fig. 2). Therefore, we replace the current value with the median value from our literature synthesis ( m).
Several remote-sensing-based data sets exist for z0m,b, which could potentially be used as an alternative to using one globally constant z0m,b value (e.g., Marticorena et al., 2004; Prigent et al., 2005, 2012; Stilla et al., 2020). We therefore additionally implement the input of a spatially explicit z0m,b based on the data of Prigent et al. (2005), which we subsequently refer to as Pr05. Unlike other data sets, Pr05 also covers warm deserts other than the Sahara. Pr05 employs observations of the backscattering coefficient from the European Remote Sensing Satellite (ERS) scatterometer, calibrated on quality in situ and geomorphological z0m,b estimates, to derive monthly mean z0m,b in arid and semi-arid regions for an equal-area grid of 0.25∘ resolution at the Equator. To derive a spatially continuous input field for CLM, we collect the monthly data from all grid cells in Pr05 that fall within a focal grid cell in our simulations. We use the 25th percentile of the corresponding monthly data that fall within the focal grid cell as a temporally constant input for our simulations assuming that the temporal evolution in Pr05 results purely from the seasonality of vegetation (which is represented by the vegetation patches described in the previous section). The 25th percentile is chosen because vegetation normally exhibits a higher z0m than the ground. For grid cells without observations in Pr05 we use the area-weighted global mean of all the grid cells that contain data ( m). We replace values of z0m,b that fall below m with this value for numerical stability. The z0m,b values in Pr05 are at the lower side of in situ observations with values as low as m. This might originate from the fact that Pr05 focuses on desert regions by excluding z0m,b values above m, while some in situ sites might exhibit a locally higher z0m,b due to the presence of rocks or sparse vegetation elements.
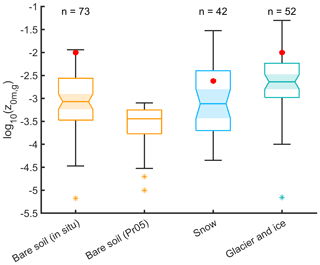
Figure 2Boxplot of the decimal logarithm in in situ observations of z0m,b (left), z0m,s (second from right), and z0m,i (right). The value of n corresponds to the number of sites. Second boxplot from left shows z0m,b in remote-sensing-based data of Prigent et al. (2005). Stars correspond to outliers, which are more than 1.5 times the interquartile range away from the box. Red dots show the current value in CLM5.
Next, we focus on the formulation of z0h,b and z0q,b. Yang et al. (2008) assessed the performance of seven different parameterizations for the ratio of , including Eq. (14), at several bare soil sites. The formulations of Owen and Thomson (1963) and a revised version of Yang et al. (2002) performed best among the tested parameterizations. Further, exhibits distinct diurnal variations, which is reproduced best by the latter parameterization. The parameterization of Zeng and Dickinson (1998), on the other hand, overestimates strongly, particularly during the day. Similarly, Chen et al. (2010) implemented and tested several parameterizations of in the Noah LSM, confirming the good performance of the formulation proposed in Yang et al. (2008), which is subsequently called Ya08. In particular, the Ya08 parameterization reduced the underestimation of daytime LSTs of Noah in arid regions (Chen et al., 2011). Similar biases to those for Noah exist in CLM3.5, which could be improved by decreasing (Zeng et al., 2012).
Overall, there is clear evidence that the parameterization of z0h,b and most likely also z0q,b applied currently in CLM5 is in disagreement with observations. We therefore employ Ya08 for the parameterization of z0h,b and z0q,b:
Here, β=7.2 and T* is the frictional temperature defined as , where SH is the sensible heat flux, ρ is the air density, and cp is the specific heat of air at constant pressure. We have also tested the formulation of after Owen and Thomson (1963) in CLM and found no major difference to the model version using Ya08. Ya08 is also used in the revised version of CLM to compute the z0h and z0q of snow and ice, which will be described in more detail in the next two sections.
2.4 Snow
The current z0 representation for snow is similar to the one of bare soil except that a globally constant z0m,s value of 0.0024 m is used instead of 0.01 m. We here focus on z0m,s, as the modifications of z0h,s and z0q,s were described in the previous section. For a comparison of z0m,s, we collect the data compiled and measured with the wind profile method for snow in Brock et al. (2006) as well as the measured values in Fitzpatrick et al. (2019) and van Tiggelen et al. (2021), applying the same procedure for reported ranges as for bare soil. Again, the default value of 0.0024 m lies in the higher range of observed values, although less drastically than for bare soil (Fig. 2). Therefore, we replace the globally constant value for z0m,s with the median value from the literature synthesis ( m).
Field observations show that z0m,s increases as snow melting proceeds due to the formation of melting ponds (Brock et al., 2006; Fitzpatrick et al., 2019). Brock et al. (2006) propose the following parameterization of z0m,s as a function of accumulated snowmelt to account for this relationship (solid line in Fig. 3):
where ln (z0m,s) is the natural logarithm of z0m,s in millimeters, b1 and b2 are empirical constants, and Ma is the accumulated snowmelt in meters water equivalent. For application in CLM, we compute the constants b1 and b2 such that the parameterization will pass through the 10th percentile of the data displayed in Fig. 2 as Ma=0 m and approaches the 90th percentile as Ma goes towards infinity, arriving at b1=1.4 and (dashed line in Fig. 3). Ma should decrease when fresh snow falls on snow that was previously melting. Therefore, we update Ma for snow that already existed at the previous time step as follows:
where and are the accumulated snowmelt at the current time step and previous time step, respectively, is the freshly fallen snow, and is the melted snow, all in meters water equivalent.
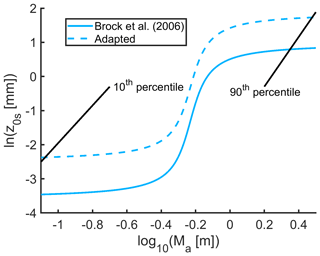
Figure 3Parameterization of z0m,s as a function of accumulated snowmelt since snowfall of Brock et al. (2006) (solid line) and parameterization with adapted constants, such that it passes through the 10th and 90th percentiles of data displayed in Fig. 2 (dashed line).
2.5 Glaciers
The surface roughness of ice sheets and glaciers is currently the same as that for bare soil in CLM. It should be noted that the surface properties of land ice play a somewhat subordinate role in CLM, since ice is generally covered by snow. As with snow, we employ the z0m,i observations of Brock et al. (2006), Fitzpatrick et al. (2019), and van Tiggelen et al. (2021) for a comparison to CLM (Fig. 2). The z0m of land ice tends to be higher than for bare soil or snow. Still, the current value of 0.01 m in CLM is on the upper end of the synthesized field observations. Accordingly, we decrease this globally constant value to m, the median among the collected field observations.
2.6 Lakes
The current lake model in CLM, the Lake, Ice, Snow, and Sediment Simulator (LISSS), was developed by Subin et al. (2012). The z0 parameterization for frozen (potentially snow-covered) lakes is the same as for ice and snow on land. However, the z0m,i was decreased in the lake model to 0.001 m. For unfrozen lakes, z0m, z0h, and z0q is parameterized as follows:
and
where α=0.1, c is the effective Charnock coefficient (for details check Lawrence et al., 2018), g is the acceleration of gravity, Pr=0.71 is the molecular Prandt number for air, R0 is the near-surface atmospheric roughness Reynolds number, and Sc=0.66 is the molecular Schmidt number for water in air. The resultant z0m values over open water lie typically in the range of to m.
Subin et al. (2012) demonstrated the added value of the z0 formulations described above compared to prescribing a constant value in LISSS. The WRF lake model also profited from an introduction of this parameterization (Xu et al., 2016; Wang et al., 2019). Li et al. (2015) find the dependence of z0m, z0h, and z0q on wind speed in LISSS is not ideal for a lake over the Tibetan Plateau. However, the simulated values are generally of reasonable magnitude compared to the observed values. We conclude that there is no clear evidence for a need to revise the z0 parameterization of LISSS. Therefore, we retain the current formulations for z0 over lakes. We do, however, adopt the revisions for the z0 of frozen lakes, consistent with the modifications for snow and ice on land described in Sect. 2.4 and 2.5.
2.7 Urban areas
In the urban module of CLM, z0 and d are parameterized after Macdonald et al. (1998) as a function of the canyon height, H, the plan area index, λp, and the frontal area index λf (for more details see Oleson et al., 2008, 2010):
and
where α=4.43 is an empirical coefficient and CD is the depth-integrated mean drag coefficient for surface-mounted cubes in a shear flow. As for vegetation, this z0 corresponds to the aerodynamic z0 for the exchange between the urban canopy and the atmosphere. Again, there are additional resistance for the exchange of water vapor and energy between the surface of the different elements in the urban environment and the urban canopy air.
Variations of among urban environments are considerable (e.g., Kanda et al., 2013). The parameterization of Macdonald et al. (1998) generally lies comfortably within the spread of estimates (Grimmond and Oke, 1999; Nakayama et al., 2011; Kanda et al., 2013). We therefore conclude that there is no justification to revise the representation of z0 and d in the urban module of CLM.
2.8 Resulting changes in surface roughness
Here, we present the impact on z0 due to the proposed set of CLM modifications in land-only simulations, which will be described in the next section. The introduction of Ra92 leads to an increase in z0,v for the forest PFTs (Fig. 4a and c). In particular, the z0,v of forests increase by more than an order of magnitude during winter in some locations because the z0,v of deciduous trees is raised considerably for low values of VAI (Fig. 1e). Changes in z0,v for grassland and cropland PFTs generally exhibit no clear pattern, with the exception of a pronounced reduction in z0,v in the northern high latitudes during winter (Fig. 4b and d).
The z0m,g decreases by more than an order of magnitude in most regions due to the changes in z0m,b, z0m,s, and z0m,i (Fig. 5a and d). In some coastal areas of Greenland, z0m,g increases slightly, as enough snowmelt accumulates to reach the higher end of the Brock et al. (2006) parameterization for z0m,s. The z0h,g and z0q,g now exhibit a distinct diurnal cycle following the introduction of Ya08. They decrease at daytime in low latitudes and during summer in the midlatitudes, while they increase under stable conditions that are often present in high latitudes and at night. In fact, the concurrent decrease of z0m,g and increases of z0h,g frequently result in a distinctly larger z0h,g than z0m,g (not shown). This is in contradiction to field observations, which normally find higher values of z0m,g than for z0h,g (Yang et al., 2002, 2008).
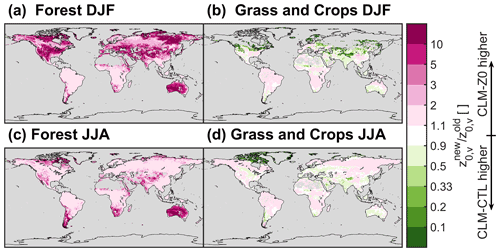
Figure 4Ratio of new vegetation surface roughness (z0,v; in CLM-Z0) divided by old z0,v (in CLM-CTL). Panels (a) and (c) show the ratio of average z0,v across forest plant functional types, and (b) and (d) show the ratio across grassland and cropland plant functional types. (a, b) Boreal winter (DJF) and (c, d) boreal summer (JJA). Data are masked if vegetation is buried completely by snow.
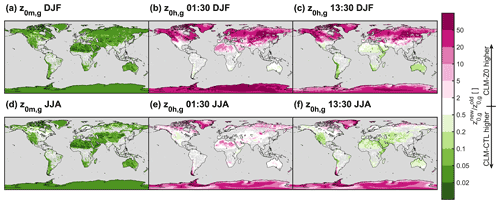
Figure 5Ratio of new ground surface roughness (z0,g) divided by old z0,g. Panels (a) and (d) show the momentum surface roughness, (b) and (e) surface roughness of scalars at 01:30 local solar time, and (c) and (f) surface roughness of scalars at 13:30 local solar time. (a–c) Boreal winter (DJF) and (d–f) boreal summer (JJA).
We present results from two sets of simulations: (1) land-only simulations using CLM version 5.1 forced by the GSWP3 reanalysis data (Dirmeyer et al., 2006; Kim, 2014) and (2) land–atmosphere simulations with CESM version 2.1.2. For each simulation, we conduct a 50-year spinup followed by a 15-year analysis period using a near present-day climatological configuration. The vegetation phenology is prescribed from satellite observations in all simulations (SP mode in CLM). The different patches of vegetation are placed on separated soil columns to suppress lateral exchange of energy and water among them (Schultz et al., 2017; Meier et al., 2018) and biomass heat storage was activated to enable removal of the stability cap of the Monin–Obukhov stability parameter (Swenson et al., 2019; Meier et al., 2019). We additionally implement a new history file averaging flag that enables retrieval of model output at a specified local solar time. This allows us to determine the model state at a specific local solar time, for example at the time of MODIS LST observations at 01:30 and 13:30, without archiving data at all model time steps. For each configuration we conduct one control simulation with the existing representation of z0 in CLM and a simulation in which we apply the updates for z0 previously described.
For the CLM simulations, we use the component configuration set “I2000Clm51Sp”. These simulations are run at 0.5∘ resolution. For the atmospheric forcing we cycle through the GSWP3 data of 1998–2012. The resulting simulations are called CLM-CTL and CLM-Z0 subsequently. In addition, a series of CLM land-only experiments are presented in Appendix A1 to assess the effect of the individual modifications. Table A1 provides an overview of all CLM simulations.
The CESM simulations are run in the configuration “F2000climo” at 0.9∘ × 1.25∘ resolution. This configuration couples CLM version 5.0 with the atmospheric model CAM version 6.0. The ocean is prescribed in F2000climo from the Hadley Centre Sea Ice and Sea Surface Temperature (HadISST) v1.1 data set (i.e., it is run in data mode; Hurrell et al., 2008). For the prescribed sea surface temperature forcing, we cycle through the data of 1998–2012 instead of using the data from 2000 only, as is normally the case in F2000climo. This is done to introduce more interannual variability. We refer to the CESM simulations as CESM-CTL and CESM-Z0.
4.1 Reference data sets
We consult two observation-based data sets to assess the impact of the imposed modifications in CLM-Z0 and CESM-Z0 on model performance in terms of LST. First, we use observations of the MODIS system, which is installed on the low-Earth-orbit satellites, Terra and Aqua, to evaluate diurnal variations of the LST at grid cell level. These instruments provide LST estimates at a resolution of 1 km at approximately 01:30 and 13:30 local solar time at the Equator, based on the longwave radiation emitted by the land surface. We employ data from 2003–2012 of the product MYD11C3 version 6 (Wan et al., 2015), which has a native resolution of 0.05∘. From these data, we compute a multi-year monthly climatology as described in Meier et al. (2019) at 0.5∘ resolution. For comparison to the CESM simulations, we regrid this climatology to 0.9∘ × 1.25∘ with first-order conservative remapping of the Climate Data Operators (CDO) library. We output the LST in the model simulations at 01:30/13:30 and use only model output from 2003–2012 for a consistent comparison with MODIS. Further, we apply a cloud masking to the model output as described below.
In addition to comparing LST directly at grid cell level, we also evaluate the local LST difference between bare soil and vegetation. To extract such information from the MODIS LST observations, we repeat the space-for-time substitution approach as in Duveiller et al. (2018) to relate the LST to the land cover type. For the LST, we again employ monthly MYD11C3 data both at daytime (13:30) and during nighttime (01:30). The land cover fractions are based on the ESA Climate Change Initiative Land Cover project (ESA, 2017). We aggregate all land cover types that involve vegetation to form the vegetated land cover class, while we use the bare land class as bare soil. Then, we conduct a multiple linear regression between the MODIS LST observations and the land cover fractions within moving windows of 5 by 5 pixels for each month in 2008–2012 (see Duveiller et al., 2018, 2021, for details). The slope of this multiple linear regression between the vegetated land cover class and bare land forms the estimated LST sensitivity for a conversion between these two land cover types. With this procedure, we retrieve a monthly observation-based estimate of the LST sensitivity to a conversion of vegetation to bare soil at 0.25∘ resolution, along with an estimate of the uncertainty associated with the regression. For comparison to the CLM simulations, we compute the multi-year monthly average at 0.5∘ resolution, weighting all grid cells that fall into the focal location–month combination by area and by 1 over the uncertainty estimate of the respective value. The resultant reference data set is called Du18. In CLM, we compute the subgrid difference in the variable of interest of the bare soil patch minus all vegetation patches (including crops) within a grid cell as described in more detail in Meier et al. (2018). We only use cloud-masked LST data for 2008–2012, which was output at 01:30 and 13:30 local solar time.
4.2 Computation of LST and cloud masking
The LST in CLM is computed based on the leaf temperature (Tleaf) and the temperature of the ground (Tgrnd):
where the vegetation emissivity, ev, is a function of the VAI:
Here, corresponds to the average inverse optical depth for longwave radiation, which is set to 1 in CLM (Eq. 4.20 in Lawrence et al., 2018). Tgrnd is a function of the snow temperature (Tsnow), the temperature of the top soil layer (Tsoil), the temperature of the surface water (), the fraction of the ground covered by snow (fsnow), and the fraction of the ground covered by surface water ():
MODIS can observe the LST only under clear-sky conditions (Wan et al., 2015). We therefore remove cloudy conditions in our model output when confronting it with MODIS. For the CESM simulations, we can filter for clear-sky conditions directly from the total cloud cover model output. To do so, we output the total cloud coverage and the variables of interest at daily temporal resolution. In the postprocessing, we then remove days with an average total cloud coverage above 50 %. It is more complex to exclude cloudy days in the land-only CLM simulations, since the GSWP3 forcing does not include information on cloud coverage (Kim, 2014). We therefore mask for cloudy days based on the incoming shortwave radiation. This is done through a comparison to the daily incoming solar radiation at the top of the atmosphere according to Berger (1978), WTOA, which is a function of latitude and the day of the year. However, solar radiation passing through the atmosphere can be altered even under clear-sky conditions, for example, because of aerosols (IPCC, 2013). Therefore, we derive a climatology of the incoming solar radiation at the surface under clear-sky conditions, , based on WTOA in an iterative procedure:
-
A multiplicative factor, C, is optimized, such that it minimizes the sum-squared deviation to the daily incoming solar radiation forcing of GSWP3 at a given location:
-
Incoming solar radiation values below 80 % of are removed for the next iteration, unless the current fit is based on less than 200 values (the iteration starts with values).
-
This iteration is stopped if the sum-squared deviation of to the remaining daily incoming solar radiation forcing of GSWP3 improves by less than 10 W2 m−4.
With this procedure, we estimate for each land point. We then remove days where the daily incoming solar radiation lies below 20 W m−2 or below 90 % of in the postprocessing of the model output of the CLM simulations. Figure 6 illustrates this clear-sky masking for four grid cells. Note that this cloud-masking procedure is not perfect because it effectively ignores clouds at night and does not distinguish between cloud types, which affect the incoming shortwave radiation at the surface differently (L'Ecuyer et al., 2019). Also, it results in data gaps during the polar night, because no incoming shortwave radiation is available for the cloud-masking procedure.
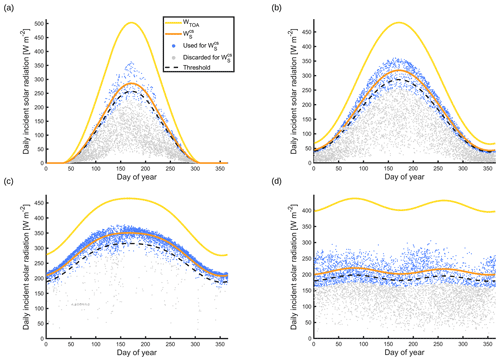
Figure 6Examples of cloud masking based on incoming shortwave radiation at (a) 73.25∘ N/11.75∘ E, (b) 53.25∘ N/11.75∘ E, (c) 23.25∘ N/11.75∘ E, and (d) 3.25∘ N/11.75∘ E. The yellow line shows daily incoming solar radiation at the top of atmosphere according to Berger (1978), the orange line shows fitted incoming shortwave radiation at the surface under clear-sky conditions, the blue dots show daily incoming solar radiation values in GSWP3 included to make this fit, the gray points show daily incoming solar radiation values in GSWP3 removed because they are below 80 % of the last fit of , and the dashed black line shows the threshold of 90 % of above which days are considered to have clear-sky conditions.
4.3 Significance testing
The CESM simulations exhibit a considerable degree of interannual variability. Therefore, we conduct a statistical test to assess whether the identified seasonal differences between CESM-Z0 and CESM-CTL are significant. First, we conduct a one-sample Student's t test at 5 % confidence level for the sample of seasonal mean differences between CESM-Z0 and CESM-CTL for each grid cell and season. This test in isolation is inappropriate when applied to a spatially autocorrelated field, as clustered areas can appear erroneously significant (Wilks, 2016). Thus, we control the false discovery rate as proposed in Wilks (2016) using a confidence level of 10 % ( %), which is appropriate for data with a moderate to strong spatial autocorrelation. In addition, we include the last 30 years of the spinup period for some variables to corroborate the presented results.
We first focus on the LST response at 01:30/13:30 local solar time in the land-only CLM simulations in Sect. 5.1. We also compare the simulated diurnal variations in LST to MODIS observation as well as the subgrid LST difference between bare soil and vegetation to Du18. In Sect. 5.2 we focus on the results from the CESM land–atmosphere simulations. Initially, the focus is again on the LST (Sect. 5.2.1) and additionally the air temperature at the bottom of the atmosphere (Sect. 5.2.2). Afterwards, we present alterations in wind speed. Note that we present a number of sensitivity experiments in Appendix A1, where we assess the influence of the different z0 modifications individually. Further, we conduct an energy balance decomposition after Luyssaert et al. (2014) in Appendix A2 to link the changes in LST described in this section to individual energy fluxes.
5.1 LST response in land-only simulations
At 13:30, the LST increases substantially in warm desert regions (Fig. 7a and c). This warming originates mainly from the reduction in z0m,g, while the introduction of the Ya08 formulation for z0h,g and z0q,g produces only a small impact (Appendix A1). The reduced z0m,g inhibits the exchange of sensible heat with the atmosphere (Fig. A2). The solar radiation absorbed by the land surface in desert regions is therefore transferred less efficiently to the atmosphere in CLM-Z0. Consequently, the land surface warms and maintains its energy balance through emission of more longwave radiation and a higher ground heat flux (Fig. A2). Accordingly, the induced warming is higher during the summer season, when the solar irradiance is highest. On the other hand, the reduction in z0m,g decreases the LST in the cold deserts, in particular during the winter season. This is again the result of a reduced sensible heat flux, which is however generally directed from the warmer atmosphere to the land surface in those regions. In vegetated areas, the increased z0,v of forests enhances the turbulent transport of energy away from the land surface (Fig. A2), producing a cooling of the daytime LST.
The LST response at 01:30 is generally considerably weaker than the daytime effect (Fig. 7b and d). Conditions in the surface layer are more commonly stable at night, inhibiting the turbulent energy exchange between the land and the atmosphere. Also, there is no strong energy input to the land surface in the form shortwave radiation at night. Therefore, our modifications of z0 produce a weaker effect. Interestingly, the pronounced daytime warming effect in the warm deserts translates into the night through the energy stored in the soils (Fig. A3). In contrast, the increase in z0,v of forests warms the land surface at night in particular during summer by increasing the sensible heat flux towards the land. Thus, the LST response at 01:30 over vegetation opposes the daytime response in sign, unlike in desert regions. This is likely the case, because the LST in CLM is linked tightly to the leaf temperature, which exhibits a smaller thermal inertia than the ground (Eq. 23). Consequently, the alterations in LST change sign diurnally in regions dominated by vegetation, while the sign remains the same over regions dominated by bare soil.
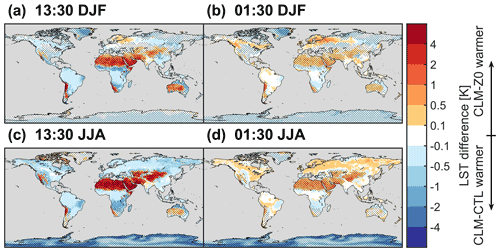
Figure 7LST difference between CLM-Z0 and CLM-CTL. (a, c) LST difference at 13:30 local solar time and (b, d) difference at 01:30 local solar time. (a, b) Boreal winter (DJF) and (c, d) boreal summer (JJA). The stippling shows areas dominated by bare soil with a seasonal average VAI below 0.5 m2 m−2. Note the non-linear color scale.
Overall, the modified z0 amplifies the diurnal temperature range (DTR; here defined as the LST difference between 13:30 and 01:30 local solar time) in desert regions and dampens the DTR in regions with forests (Fig. 8a). This links back to previous studies that found an overestimation of the DTR in desert regions and an underestimation over forests in CLM compared to remote sensing observations (Zeng et al., 2012; Meier et al., 2019). This tendency prevails in the current version (5.1) of CLM (Fig. 8d). The modifications of z0 in CLM-Z0 alleviate these biases in most regions with the notable exception of the southern half of the Sahara, where the reduced z0m,g in CLM-Z0 frequently overcompensates an only slight underestimation of the LST DTR in CLM-CTL (Fig. 8b, c, e, and f).
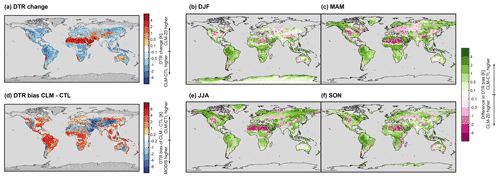
Figure 8Panel (a) shows the difference in LST DTR of CLM-Z0 minus CLM-CTL and panel (d) bias in LST DTR of CLM-CTL compared to MODIS remote sensing observations. The stippling in those panels shows areas with an average VAI below 0.5 m2 m−2. To the right, the changes in the LST DTR bias between CLM-Z0 and CLM-CTL in boreal winter (b), spring (c), summer (e), and autumn (d) are shown. CLM data are cloud masked based on the incoming shortwave radiation. Note the non-linear color scale.
The modifications in CLM-Z0 also affect the sensitivity of the LST to land cover. Here, we compare the LST response to a conversion of vegetated land to bare soil, as estimated in Du18, to the subgrid LST difference between the vegetated tiles and the bare soil tiles in CLM. This land cover transition is likely relevant for the biogeophysical response to desertification, which has become more common over the last decades (IPCC, 2019). Overall, Du18 observes an increase in LST at 13:30 over bare soils compared to vegetation with the exception of latitudes exceeding 40∘ N/S during the colder months (Fig. 9a). CLM-CTL exhibits a lower daytime LST over bare soil tiles than over vegetated tiles in most regions (Fig. 9b). CLM-Z0 captures the LST increase for a conversion of vegetation to bare soil at 13:30 in most cases (Fig. 9c). However, the signal is considerably stronger than in Du18, resulting in a higher RMSE for this simulation than in CLM-CTL. At night, the modifications in CLM-Z0 further amplify a positive bias in the LST difference between bare soil and vegetation in CLM-CTL in comparison to Du18 (Fig. 9e–h). For the DTR, Du18 finds an amplification over bare land compared to vegetation for most latitude–month combinations, with the exception of the high latitudes during winter (Fig. 9j). CLM-CTL on the other hand mostly exhibits a lower DTR over bare soil than over vegetation (Fig. 9k). This bias is mitigated to some extent in CLM-Z0, even though a dampening of the DTR often persists in the northern midlatitudes (Fig. 9l). Overall, the imposed alterations in z0 do not result in a clear and consistent improvement of the LST response to a conversion between vegetation and bare soil but clearly do alter this sensitivity. Some discrepancies between Du18 and the CLM simulations might also arise from the absence of atmospheric feedbacks in the subgrid approach, which are used to diagnose the land cover sensitivity in CLM (note that the subgrid approach would still neglected atmospheric feedbacks in the CESM simulations; for more information see Chen and Dirmeyer, 2020). In addition, the cloud masking based on the incoming solar radiation could potentially introduce mismatches between CLM and Du18, in particular at night. Further, preferential occurrence of clouds over vegetation or bare soil could introduce biases in Du18. In fact, a recent study observed increased low-level cloud cover over forests compared to short vegetation, using a similar methodology as in Du18 (Duveiller et al., 2021).
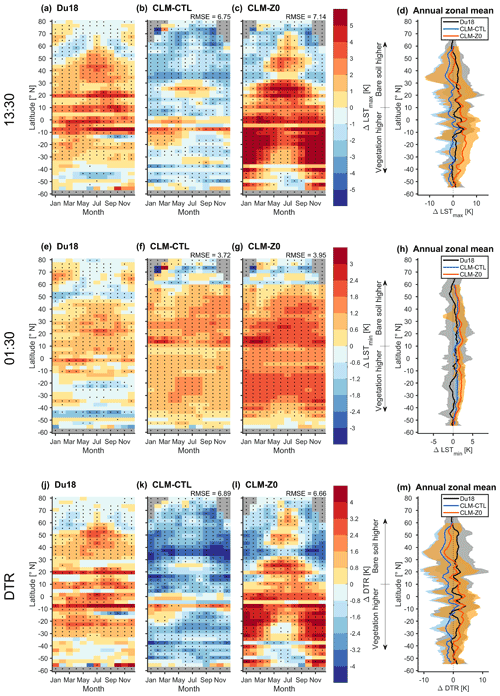
Figure 9LST sensitivity in Du18 and CLM to conversion of vegetation to bare land. (a–d) LST difference between bare soil minus vegetated land at 13:30 local solar time (ΔLSTmax). Seasonal and latitudinal variations of (ΔLSTmax) in (a) the observation-based estimate of Du18, (b) CLM-CTL, and (c) CLM-Z0. Points with a mean which is significantly different from 0 in a two-sided t test at the 95 % confidence level are marked with a black dot. All data from the 2008–2012 analysis period corresponding to a given latitude and a given month are pooled to derive the sample set for the test. The numbers next to the titles are the area-weighted spatiotemporal root-mean-squared deviation of the respective simulation against Du18. Panel (d) shows the zonal annual mean of Du18 (black, range between the 10th and 90th percentiles in gray), CLM-CTL (blue, range between the 10th and 90th percentiles in blue), and CLM-Z0 (red, range between the 10th and 90th percentiles in orange). Note that on this subfigure results have been smoothed latitudinally with a simple moving average over 4∘. CLM data are cloud masked based on the incoming shortwave radiation. Panels (e–h) are the same for the LST difference at 01:30 local solar time and panels (j–m) for the diurnal temperature range.
5.2 Effect in land–atmosphere coupled simulations
In the preceding section, we assessed the impact of the proposed changes to z0 in land-only simulations. However, the resultant changes in land turbulent fluxes may also affect the atmosphere. In this section, we therefore evaluate the impact of the z0 modifications in land–atmosphere simulations.
5.2.1 LST response
At low latitudes, the LST at 13:30 in CESM-Z0 increases over the deserts and decreases in most regions with dense vegetation, similar to the land-only simulations (Fig. 10a and b). However, the daytime warming in deserts is stronger in the coupled simulations (Fig. 7). It therefore appears that atmospheric feedbacks trigger an additional warming of the land in these regions. Indeed, we find an increase in incoming shortwave radiation accompanied by a reduction in cloud cover, which is most notable over the Sahara and the Middle East (Fig. 10e–h and Figs. A4 and A1). Previous studies have found that an increase in the sensible heat flux favors cloud coverage (Khanna et al., 2017; Bosman et al., 2019). It is therefore possible that the reduction in cloud coverage over desert regions in CESM-Z0 is a byproduct of the lower sensible heat flux in this simulation. Over the northern midlatitudes and high latitudes, an increase in cloud cover during summer coincides with a reduction in daytime LSTs due to less incoming shortwave radiation (Fig. A4). The LST response at night is often weaker but of the same sign as the daytime signal in CESM, similar to the land-only simulations (Fig. 10c and d). However, no distinct nighttime warming emerges over midlatitude forests during the summer season at night in the coupled simulations (compare Figs. 7d and 10d). In the mid- and high latitudes, changes in LST often exhibit a similar spatial pattern to surface air temperature changes, which are discussed in more detail in the next section (compare Figs. 10 and A6). In particular, the warming of the LST during winter over Alaska and western Canada appears to be related to more incoming longwave radiation at the surface (Fig. A1), which could be the result of warmer atmospheric temperatures in this region (Fig. A6a).
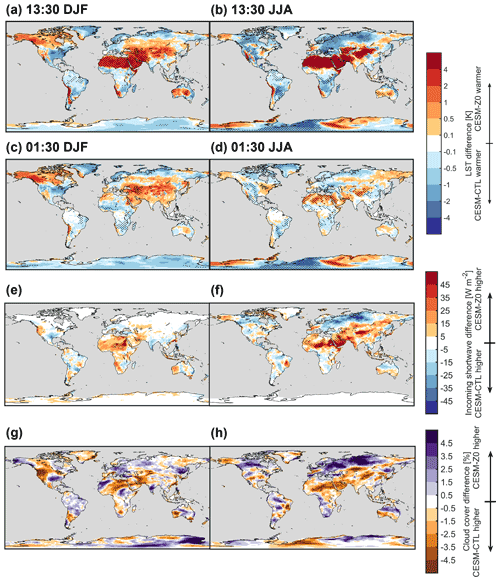
Figure 10LST difference between CESM-Z0 and CESM-CTL at (a–b) 13:30 local solar time and (c–d) 01:30 local solar time. Panels (e) and (f) show the difference in incoming shortwave radiation at 13:30 local solar time between CESM-Z0 and CESM-CTL, and (g) and (h) show the difference in daily average total cloud cover. The stippling shows areas with a difference that is statistically significant different from 0 in a two-sided t test at the 95 % confidence level with a controlled false discovery rate. The left column shows boreal winter (DJF) and the right column boreal summer (JJA). Note the non-linear color scale for panels (a–d).
Compared to the MODIS observations, CESM-CTL underestimates the DTR over arid and semi-arid areas even more than CLM-CTL (Fig. 11d). On the other hand, the DTR is overestimated over most regions with dense vegetation and regions with permanent ice sheets. Again, the reduced z0m,g amplifies the DTR in warm desert regions producing an improved agreement with the remote sensing observations (Fig. 11). Apart from these regions, the results are more mixed. Still, there is a clear improvement over the northern midlatitudes during boreal summer. Yet, the alterations of z0 in CESM-Z0 do not entirely alleviate the existing biases in the LST DTR of CESM (Fig. A7). The remaining biases may not only originate from deficiencies in the land model itself but could also be related to atmospheric processes such as radiative transfer in the air column.
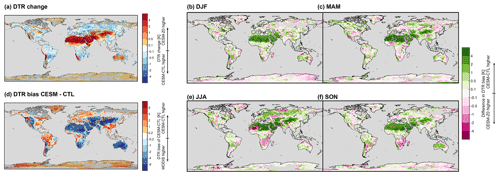
Figure 11As Fig. 8 but for land–atmosphere coupled simulations CESM-Z0 and CESM-CTL. CESM data are cloud masked.
5.2.2 Response in surface air temperature and comparison to LST
The altered surface energy fluxes also affect air temperatures at the bottom of the atmospheric column (TBOT). The difference in daily average TBOT between CESM-Z0 and CESM-CTL exhibits considerable interannual variability. Therefore, we included the last 30 years of the spinup period to corroborate the results shown in Fig. 12a and b. Figure A6 depicts the average TBOT response for the analysis period and the last 30 years of the spinup period separately. Even when including these additional years, some pronounced features, such as the wintertime warming of average TBOT over north Asia, are still not statistically significant. Nevertheless, the wintertime average TBOT increases considerably in many regions in the Northern Hemisphere, showing a similar spatial pattern to that of the LST response (Fig. 12a). This is linked to more incoming longwave radiation (Fig. A1). On the other hand, the increase in z0,v decreases the summertime TBOT in those regions (Fig. 12b). This can be explained by lower incoming shortwave radiation in CESM-Z0 compared to CESM-CTL (Fig. A4) as a result of higher total cloud coverage (Fig. 10e). Consequently, less energy is available close to the land surface in CESM-Z0, cooling both the LST and TBOT. At low latitudes, TBOT decreases mostly over the rainforests. Interestingly, CESM-Z0 also often exhibits a lower average TBOT over the Sahara in particular during boreal winter, thus opposing the LST response in sign. Further, there is a distinct band where TBOT warms in JJA over the Sahel region, while it cools both just north and south of this region (Fig. 12b).
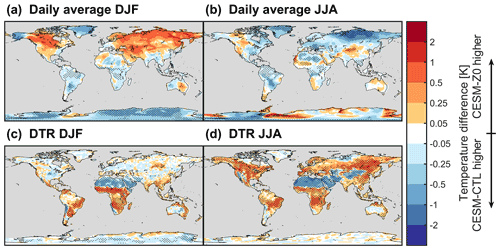
Figure 12(a–b) Seasonal average difference in air temperature at the bottom of the atmospheric column (TBOT) between CESM-Z0 and CESM-CTL using data from the last 30 years of the spinup period and data from the analysis period (15 years). (c–d) Difference in TBOT DTR. The stippling shows areas with a difference that is statistically significant different from 0 in a two-sided t test at the 95 % confidence level with a controlled false discovery rate. (a, c) Boreal winter (DJF) and (b, d) boreal summer (JJA). Note the non-linear color scale.
The effect on the DTR of TBOT in CESM-Z0 opposes the effect on the LST DTR in sign, which is most visible in Africa (compare Fig. 12c and d to Fig. 11a). Where z0 is decreased, less energy is transferred from the land into the atmosphere under unstable surface layer conditions (which are frequently present during day) and from the atmosphere to the land surface under stable conditions (frequently present at night). Consequently, the DTR at the land surface (LST) is amplified, while the DTR is dampened in the atmosphere above. This dipole between the DTR response of LST and TBOT to alterations in z0 was previously found also in the context of deforestation in CESM (Chen and Dirmeyer, 2019) and in a number of regional climate models (Breil et al., 2020).
Figure 13 displays how the response of the DTR in LST and TBOT scale with the change in z0m for latitudes between 30∘ N/S. The DTR in LST for the individual vegetation patches decreases linearly with the logarithm of the ratio between the z0,v in CESM-Z0 and the z0,v in CESM-CTL , with a slope of −3 K (when using the decimal logarithm; Fig. 13a). In other words, a 10-fold increase in z0,v dampens the LST DTR by 3 K. At grid cell level, the LST DTR exhibits a similar dependence on the change in z0m between CESM-Z0 and CESM-CTL, if z0m changes by no more than a factor of 3, as visible by values between −0.5 to 0.5 on the x axis in Fig. 13b. For stronger reductions in z0m over desert regions the amplification of the LST DTR saturates at approximately 4 K (values below −0.5 on the x axis). It therefore appears that the distinct linear relation of the LST DTR to at PFT level does not hold at grid cell level for strong reductions in z0m. This effect might originate from several factors. First, smaller changes in z0m between CESM-Z0 and CESM-CTL occur over vegetation, while the strong reductions occur over bare soil (compare Figs. 4 to 5a and d). It might therefore be that the LST reacts more strongly to alterations of z0,v than to alterations of z0m,g due to the smaller thermal inertia of vegetation compared to soils. Second, different types of land cover with varying changes in z0m are mixed at the grid cell level. For some PFT patches, z0,v increases by more than an order of magnitude (i.e., ), which is never the case for entire grid cells. We therefore cannot establish from our model experiments how the DTR would react to increases in z0m by an order of magnitude at grid cell level. Third, our sensitivity experiments in Appendix A2 show that the reductions of z0m,g in combination with the alterations z0,v amplify the response of the LST DTR over vegetation, compared to a simulation were only z0,v changed. And fourth, the sensitivity experiments indicate that the introduction of Ya08 for z0h,g and z0q,g moderates the effect of the decreased z0m,g over the Sahara on the LST DTR.
Again, the dipole between the LST DTR response and the TBOT DTR response can be observed when comparing panels (b) and (c) in Fig. 13. The two variables are clearly mirrored in sign. However, the response in TBOT DTR is considerably weaker than the one of LST. This is likely owed to the differing nature of these two variables. The LST is computed from the temperatures of the leaves and the ground and is therefore tightly linked to the energy redistribution at the land surface. In contrast, TBOT is affected not only by the energy redistribution at the land surface but also by lateral and vertical mixing of air masses. This mixing may explain why the TBOT DTR response is generally weaker than the LST DTR response.
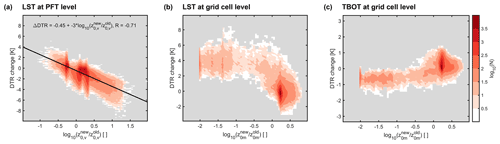
Figure 13(a) Density plot of change in multi-year monthly mean LST DTR at the PFT level of CESM-Z0 minus CESM-CTL versus the decimal logarithm of the ratio of z0,v in CESM-Z0 divided by z0,v in CESM-CTL. Bin size on the x axis is 0.05, and on the y axis it is 0.1 K. Color scale on the far right shows the decimal logarithm of the number of tiles that fall within the respective bin. Multi-year monthly mean data of all PFTs excluding bare soil between 30∘ N/S were used to generate this figure. (b–c) The same for the LST DTR (b) and TBOT DTR (c) at grid cell level and the maximum of z0m,g and z0,v. Bin size on the y axis in panel (c) is 0.05 K. The black line in panel (a) shows the linear fit with its formula and the Pearson correlation coefficient (R) above. Note the differing ranges of the y axis for the different panels.
5.2.3 Response of surface wind speed
The changes in z0m affect the drag exerted by the land, and thus the wind speed, at least close to the land surface. The wind speed at the lowest atmospheric level increases notably in CESM-Z0 over desert regions, where z0m was lowered, such as the Sahara, Greenland, or Antarctica (Fig. 14a and d). The remaining land mass is dominated by reductions in surface wind speed, consistent with the increases in z0,v that were introduced for most vegetation types in CESM-Z0. These alterations of surface wind speed decay relatively abruptly with height and are only rarely significant at a height of 1.1 km (Fig. 14b and e). Even over the Sahara, where wind speeds close to the surface increase considerably, this signal disappears about 2.5 km above the surface (Fig. 14f).
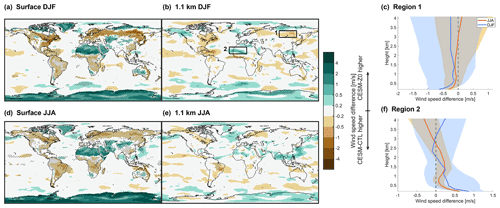
Figure 14Seasonal mean wind speed difference of CESM-Z0 minus CESM-CTL at lowest atmospheric level (a, d) and approximately 1.1 km above sea level (b, e). Top row shows boreal winter (DJF) and bottom row boreal summer (JJA). The stippling shows areas with a difference that is statistically significant different from 0 in a two-sided t test at the 95 % confidence level with a controlled false discovery rate. Note the non-linear color scale. Panels (c) and (f) show the profile of area-weighted mean wind speed difference in DJF (blue) and JJA (red) in regions 1 (c) and 2 (f), which are marked in panel (b). The line depicts the median wind speed difference across all seasonal means and shading shows the range between the 10th and 90th percentiles. Height is calculated assuming a surface pressure of 1013.2 hPa, a surface air temperature of 288.15 K, and a constant lapse rate of 6.5 K km−1. Data from the last 30 years of the spinup period and data from the analysis period (15 years) were used for this figure.
In this study, we have compared the representation of z0 in CLM to observations and parameterizations that exist in the literature, conducted revisions of CLM when clearly supported by this comparison, and assessed the impact of these revisions on simulated temperatures and wind speed. Specifically, we introduced the parameterization proposed by Raupach (1992) for the z0,v, where parameter choices were optimized to match the observational data of Hu et al. (2020). The z0 of forests is increased considerably with this new parameterization, while z0 for crops is decreased. Further, the revised z0 of broadleaf deciduous forests exhibits a minimum during the growing season as observed in the field. Based on our literature synthesis, the globally constant values for z0m,b, z0m,s, and z0m,i are reduced from to m, from to m, and from to m, respectively. Alternatively, the spatially explicit z0m,b input field from Prigent et al. (2005) can be activated in the revised model version. Similarly, the user may activate the parameterization of Brock et al. (2006) for z0m,s as a function of accumulated snowmelt. Finally, we replace the parameterization of Zeng and Dickinson (1998) for z0h,g and z0q,g with the parameterization of Yang et al. (2008). Overall, our proposed modifications increase z0m in most areas dominated by vegetation, while z0m is decreased considerably in desert regions.
The decrease of z0m,g is found to warm the land surface in warm deserts during the day and, to a lesser extent, also at night. The LST decreases over the cold deserts in particular during the winter season. The impact of the raised z0,v varies diurnally, with a cooling effect during day and a warming effect at night. In land–atmosphere coupled simulations, the daytime warming of LST over warm deserts is amplified compared to land-only simulations due to a decrease in cloud cover leading to an increase in incoming solar radiation. Overall, the proposed model modifications reduce biases in the LST DTR compared to MODIS both over warm deserts, where the DTR is underestimated, and in regions dominated by forests, where the DTR tends to be overestimated. Also, the revisions of z0 alter the local LST response to a conversion of vegetation to bare land, which could be relevant for the simulated biogeophysical effect of desertification. The sensitivity of the LST at 13:30 and the DTR improves in CLM-Z0, while the nighttime sensitivity deteriorates compared to Du18. The response in the TBOT DTR in CESM opposes the sign of the LST DTR response, with an amplification in forested regions and a dampening over warm deserts. Surface wind speeds increase over desert areas, while they decrease in regions with forests. These alterations in surface wind speed typically disappear beyond approximately 1 km above the land surface.
Overall, our results highlight the importance of z0 for the exchange of energy, water, and momentum between the land surface and the atmosphere and through that for surface temperatures and wind speed. Beyond these, there are several potential impacts we did not explore in this study. For example, we did not evaluate how these changes might affect the exchange of greenhouse gases between the land and the atmosphere, be it directly through alterations of the turbulent exchange of such gases or indirectly through biogeophysical effects that affect biogeochemical processes such as photosynthesis or respiration. Further, the resultant increase in surface wind speed in arid and semi-arid regions are likely to affect mineral dust emissions and might thereby alter dust aerosol loading in the atmosphere (Csavina et al., 2014; Wu et al., 2019).
Even though our revisions of z0 oftentimes improve the simulated LST DTR compared to MODIS, some considerable biases persist. Such biases are at least partly related to inadequate properties of the land surface other than z0. For example, the surface emissivity varies considerably across different types of land cover (Jin and Liang, 2006). Values as low as 0.9 are observed over the Sahara, which is much lower than the prescribed value of 0.96 for soils used in CLM and might therefore affect simulated LSTs compared to MODIS (Jin and Liang, 2006). Next, z0h,g and z0q,g frequently exceed z0m,g in the revised model, since the formulation of Yang et al. (2008) often increases z0h,g and z0q,g while z0m,g is decreased. Field studies on the other hand only rarely observe lower values for z0m,g than z0h,g and z0q,g. This behavior highlights a potential drawback of the formulation of Yang et al. (2008), which decouples z0h,g and z0q,g to some extent from z0m,g. This feature is unique compared to most other formulations in the literature that link z0h,g and z0q,g directly to z0m,g. Nevertheless, the two modifications in combination improved the diurnal LST variability compared to MODIS over most desert areas. For vegetation, several development activities are underway within CLM to improve the diurnal variability of temperatures and surface fluxes. Bonan et al. (2018) replace the big-leaf approach in CLM with a multi-layer canopy and introduce a roughness sublayer parameterization for tall canopies. The latter modification could ultimately replace z0,v entirely. Further, the recent addition of biomass heat storage to CLM improved the realism of simulated energy fluxes and LSTs over forests (Swenson et al., 2019; Meier et al., 2019). Finally, some discrepancies between our simulations and MODIS could also be related to the atmospheric forcing fields that CLM receives, be it from the GSWP3 reanalysis data in the case of the land-only simulations or from the atmospheric component of CESM for the coupled simulations.
While observations of z0 provide valuable information for model development, the assumptions within the model world can differ from the assumptions made to estimate z0 in the field. For example, the formulations for the stability correction functions in Hu20 differ from the ones used in CLM. Consequently, CLM would produce slightly different turbulent fluxes than measured in the field, even if conditions are exactly the same. We would like to highlight that the current approach in CLM of dividing grid cells into tiles of differing land covers does not specify how the different land covers are situated within this cell. For example, CLM treats a savanna covered by sparse trees and grasses the same as one large forest next to a grassland landscape (given that the two types of vegetation and the area fraction covered by each vegetation type are roughly the same). But in terms of z0 and other surface properties these two landscapes differ. It might therefore be necessary to further refine the tile approach in CLM, such that these two landscapes may be distinguished. In CLM, the ecosystem demography model FATES resolves this issue to some extent (Fisher et al., 2015). However, our updates of z0,v after Ra92 are not yet implemented in this version of the model.
We would like to emphasize the value of z0 observations for this work but also for other efforts of model and parameterization development. Several decades of observations of z0 allow for better constraint of models and a better understanding of how z0 is influenced by conditions at the land surface. Yet, knowledge gaps remain, for example for ice sheets. In situ observations indicate that z0m,i varies substantially, likely related to variations in the structure of the ice (Brock et al., 2006; Fitzpatrick et al., 2019). However, the surface structure of the ice is not explicitly simulated in Earth system models. Remote-sensing-based data of z0m,i over the ice sheets might be a good solution to capture such spatial variations in z0m,i, similar to what already exists for z0m,b (e.g., Prigent et al., 2005). In urban environments, z0 is not only closely linked to mean building height and the density of buildings but also to the variability of the building height (Nakayama et al., 2011; Kanda et al., 2013). If a global data set of variability of building heights becomes available, it could therefore be considered as an additional input variable to compute z0 in the urban module of CLM. For vegetation, we focused on the z0 for the exchange between the canopy air space and the free atmosphere, but did not consider the conductances for sensible and latent heat between the leaves/ground and the canopy air space. Hu et al. (2020) and the recent study of Young et al. (2021) focus both on z0h,v alongside z0m,v. Future studies could therefore develop a framework to confront the leaf surface conductance for sensible heat in CLM with such observational constraints of z0h,v.
A1 Sensitivity tests to isolate contributions from individual modifications
Besides CLM-CTL and CLM-Z0, we run a number of additional 15-year simulations, which are summarized in Table A1, to better understand the importance of the individual modifications introduced in CLM-Z0. First of all, we run a simulation, CLM-Z0C, that follows the same protocol as CLM-Z0 but with the median values for z0m,b and z0m,s depicted in Fig. 2 instead of using the spatially explicit data of Prigent et al. (2005) and the parameterization of Brock et al. (2006), respectively. This simulation uses the initial conditions from the spinup of CLM-Z0. Additionally, we start three simulations starting from the initial conditions of CLM-CTL that only utilize a subset of the modifications described in the Sect. 2. CLM-VEG uses only the parameterization of Raupach (1992) for z0,v but preserves the default for z0 otherwise. In CLM-Z0M, we introduce all the modifications related to z0m but retain the formulation of Zeng and Dickinson (1998) for z0h,g and z0q,g. CLM-Ya08 on the other hand applies the formulation of Yang et al. (2008) for z0h,g and z0q,g and uses the default representation of z0m. For this analysis, we use the years 1998–2002 as an additional spinup period and only analyze 2003–2012.
Zeng and Wang (2007)Zeng and Dickinson (1998)Raupach (1992)Prigent et al. (2005)Brock et al. (2006)Yang et al. (2008)Raupach (1992)Yang et al. (2008)Raupach (1992)Zeng and Dickinson (1998)Raupach (1992)Prigent et al. (2005)Brock et al. (2006)Zeng and Dickinson (1998)Zeng and Wang (2007)Yang et al. (2008)Table A1Overview of CLM simulations. Columns from left to right show the name of simulation, parameterization for z0,v, z0m,b z0m,s, choice of z0m,i, parameterization for z0h,g and z0q,g, and initial conditions used. Parameterizations and data sets that are marked with a asterisk were modified before including them in CLM.

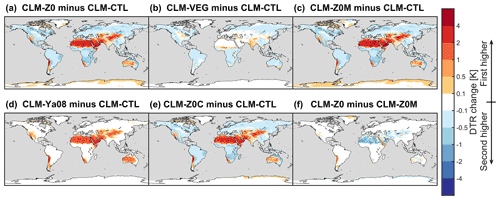
Figure A1Difference in LST DTR over 2003–2012 for (a) CLM-Z0 minus CLM-CTL, (b) CLM-VEG minus CLM-CTL, (c) CLM-Z0M minus CLM-CTL, (d) CLM-Ya08 minus CLM-CTL, (e) CLM-Z0C minus CLM-CTL, and (f) CLM-Z0 minus CLM-Z0M.
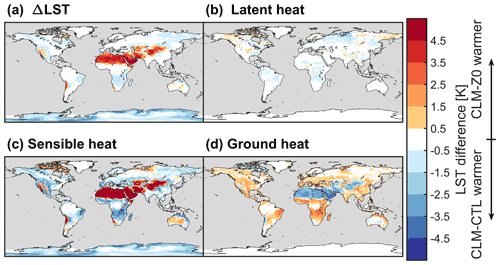
Figure A2Energy balance decomposition for change in LST at 13:30 local solar time in boreal summer of CLM-Z0 minus CLM-CTL. (a) Change in LST, (b) contribution from change in latent heat, (c) contribution from change in sensible heat, and (d) contribution from change in ground heat flux. Note that some terms are not shown because they are zero in offline simulations (incoming radiation terms) or because they are small (albedo and the imbalance term).
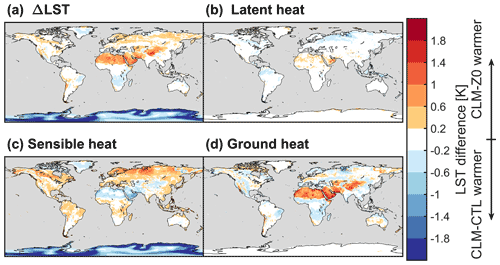
Figure A3As Fig. A2 but at 01:30 local solar time.
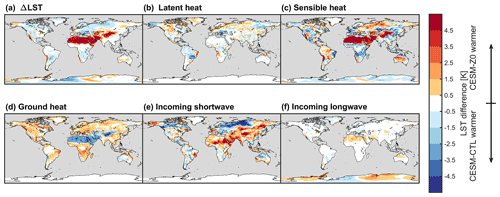
Figure A4Energy balance decomposition for change in LST at 13:30 local solar time in boreal summer of CESM-Z0 minus CESM-CTL. (a) Change in LST, (b) contribution from change in latent heat, (c) contribution from change in sensible heat, (d) contribution from change in ground heat flux, (e) contribution from change in incoming shortwave radiation, and (f) contribution from change in incoming longwave radiation. Note that the albedo and the imbalance term are not shown because they are small.
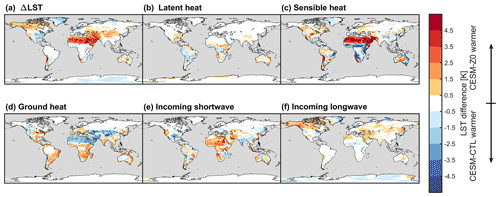
Figure A5As Fig. A4 but for boreal winter.
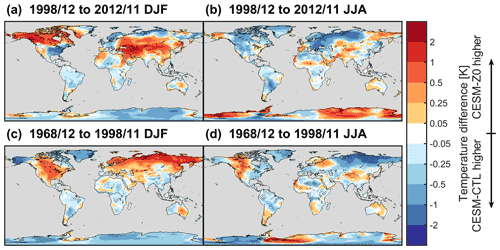
Figure A6As Fig. 12a and b but using data of the analysis period only (a, b, December 1998 to November 2012) and using data from the last 30 years of the spinup period (c, d, December 1968 to November 1998).
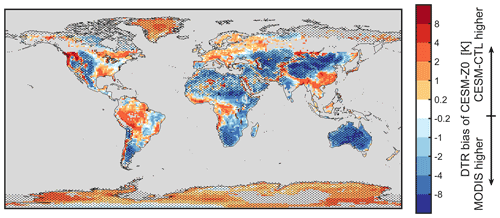
Figure A7As Fig. 11d but for CESM-Z0.
Table A2List of abbreviations and symbols used in this study. Symbols that only appear in one equation are not listed.
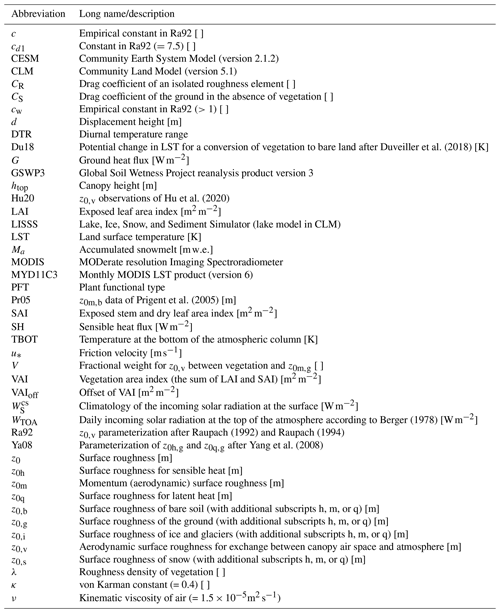
Here, we compare the effect on the annual mean LST DTR of the different sensitivity experiments in comparison to CLM-CTL. The alterations in z0,v alone introduced in CLM-VEG decrease the DTR in regions dominated by forests (where the z0,v is increased) and increase it in regions with a considerable amount of crops (for which z0,v is decreased; Fig. A1b). Interestingly, the response in forested regions is often weaker in CLM-VEG than in CLM-Z0 or even reversed in sign in central North America (Fig. A1a). The full signal strength in regions dominated by vegetation only emerges, when the alterations of z0m,g are introduced in CLM-Z0M (Fig. A1c). It appears that a decrease in z0m,g under a closed canopy dampens the LST DTR more than an increase in z0,v in isolation. The opposite is the case over warm desert areas. Somewhat unexpected, the amplifications of diurnal variations in LST over arid and semi-arid regions is moderated when Ya08 is introduced in CLM-Z0 compared CLM-Z0M over most of the Sahara, the Middle East, and the Himalaya (Fig. A1f). On the other hand, the introduction of the Ya08 parameterization for z0h,g and z0q,g with the default z0m,g in CLM-Ya08 enhances the LST DTR (Fig. A1d). Ya08 therefore amplifies the diurnal LST variability for relatively large values of z0m,g (which are used in CLM-Ya08 and CLM-CTL), while it dampens this variability for small z0m,g values (which are used in CLM-Z0M and CLM-Z0) compared to the parameterization of Zeng and Dickinson (1998). The globally constant z0m,b in CLM-Z0C is larger than the spatially explicit data in Pr05 (Fig. 2). Also, z0m,s is higher in CLM-Z0C over most regions than in CLM-Z0, with the notable exception of some areas of Greenland (not shown). Thus, z0m,g is generally decreased less in CLM-Z0C than in CLM-Z0 in comparison to CLM-CTL. Accordingly, the response in the LST DTR tends to be slightly smaller in magnitude in CLM-Z0C than in CLM-Z0 (Fig. A1a and e). Overall, there is however no major difference between CLM-Z0C and CLM-Z0.
A2 Energy balance decomposition
In this section, we present an energy balance decomposition after Luyssaert et al. (2014) to better understand the contribution of changes in individual energy fluxes to the overall change in LST between CLM/CESM-CTL and CLM/CESM-Z0. Assuming the emissivity of the land surface is equal to 1, the change in LST (ΔLST) is expressed as follows:
where σ is the Stefan–Boltzmann constant, SWin is the incoming shortwave radiation, α is the albedo, LWin is the incoming longwave radiation, LH is the latent heat flux, SH is the sensible heat flux, G is the ground heat flux, and I is the energy imbalance. ΔX corresponds to the difference in variable X between CLM/CESM-Z0 and CLM/CESM-CTL. We take the average of CLM/CESM-Z0 and CLM/CESM-CTL for the variables for which no difference is taken (e.g., SWin for the first term in the brackets). The terms on the right-hand side of Eq. (A1) correspond to the change in LST due to the change in albedo, incoming shortwave radiation, incoming longwave radiation, latent heat, sensible heat, ground heat, and the energy imbalance from left to right.
Figure A2 shows the most important terms of the energy balance decomposition at 13:30 local solar time during boreal summer in the offline simulations. Changes in LST during the day between CLM-CTL and CLM-Z0 are mostly the result of alterations in SH. The contribution from SH is compensated partly by G most of the time. If, for example, the LST increases due to a reduction in SH, part of this energy surplus is compensated by the energy stored in the ground (leading to a warming of the soils below the land surface). The other terms only have a small effect on the overall change in LST. At 01:30 local solar time, ΔLST is again driven by changes in SH in the high latitudes (Fig. A2). At lower latitudes, in particular in the warm deserts, the strong LST response during the day frequently translates into the night through the energy stored in the ground. Over the Sahara, for example, the ground absorbs more energy during the day because SH is reduced, resulting in a warmer LST at night.
For the land–atmosphere coupled simulations, the incoming shortwave and longwave radiation terms become relevant due to atmospheric feedbacks. During boreal summer, increased incoming solar radiation over the Sahara, the Middle East, and the Himalaya amplify the warming from the reduced SH (Fig. A4). The reduction in LST over the northern midlatitudes and high latitudes mostly coincides with less incoming solar radiation. In contrast, the signal in winter is determined by the longwave radiation in those regions (Fig. A5). In particular, the wintertime warming of the LST in the northern part of North America occurs due to more incoming longwave radiation in concert with warmer atmospheric temperatures (Fig. A6a).
The CLM code, the CESM code, Du18, and the estimated climatology of the incoming shortwave radiation at the land surface in GSWP3 under clear-sky conditions are available at https://doi.org/10.3929/ethz-b-000503165 (Meier et al., 2021). MYD11C3 can be downloaded from https://lpdaac.usgs.gov/products/myd11c3v006/ (last access: 23 February 2022; Wan et al., 2015) and Land Cover CCI from http://maps.elie.ucl.ac.be/CCI/viewer/download.php (last access: 23 February 2022; ESA, 2017). For the data from Hu et al. (2020), contact Xiaolong Hu, and for the data from Prigent et al. (2005), Catherine Prigent. Any model output is available upon request from Ronny Meier.
RM and ELD conceptualized the study with help from GBB, DML, and SIS. RM implemented the modifications in the model, with the help of ELD, DML, and GBB. XH provided the vegetation surface roughness observations and helped with their analysis. GD conducted the analysis for the sensitivity of land surface temperatures to desertification. CP provided the data from Prigent et al. (2005). RM conducted the analysis and drafted the manuscript with help from all co-authors. All authors contributed to the interpretation of the results and preparation of the manuscript.
At least one of the (co-)authors is a member of the editorial board of Geoscientific Model Development. The peer-review process was guided by an independent editor, and the authors also have no other competing interests to declare.
Publisher's note: Copernicus Publications remains neutral with regard to jurisdictional claims in published maps and institutional affiliations.
The CESM project is supported primarily by the National Science Foundation. We thank all the scientists, software engineers, and administrators who contributed to the development of CESM2. We thank Wim Thiery for his valuable advice. All graphics were created with MATLAB R2020b.
This research has been supported by the Swiss National Science Foundation (SNSF) through the CLIMPULSE project (http://p3.snf.ch/Project-172715, last access: 23 February 2022; grant no. 200021_172715).
This paper was edited by Jatin Kala and reviewed by two anonymous referees.
Belušić, D., Fuentes-Franco, R., Strandberg, G., and Jukimenko, A.: Afforestation reduces cyclone intensity and precipitation extremes over Europe, Environ. Res. Lett., 14, 074009, https://doi.org/10.1088/1748-9326/ab23b2, 2019. a
Berger, A.: Long-Term Variations of Daily Insolation and Quaternary Climatic Changes, J. Atmos. Sci., 35, 2362–2367, https://doi.org/10.1175/1520-0469(1978)035<2362:LTVODI>2.0.CO;2, 1978. a, b, c
Bingöl, F.: A simplified method on estimation of forest roughness by use of aerial LIDAR data, Energy Sci. Eng., 7, 3274–3282, https://doi.org/10.1002/ese3.496, 2019. a
Bonan, G.: Turbulent Fluxes and Scalar Profiles in the Surface Layer, Cambridge University Press, 80–100, https://doi.org/10.1017/9781107339217.007, 2019. a
Bonan, G. B., Patton, E. G., Harman, I. N., Oleson, K. W., Finnigan, J. J., Lu, Y., and Burakowski, E. A.: Modeling canopy-induced turbulence in the Earth system: a unified parameterization of turbulent exchange within plant canopies and the roughness sublayer (CLM-ml v0), Geosci. Model Dev., 11, 1467–1496, https://doi.org/10.5194/gmd-11-1467-2018, 2018. a
Bosman, P. J. M., van Heerwaarden, C. C., and Teuling, A. J.: Sensible heating as a potential mechanism for enhanced cloud formation over temperate forest, Q. J. Roy. Meteor. Soc., 145, 450–468, https://doi.org/10.1002/qj.3441, 2019. a
Breil, M., Rechid, D., Davin, E., de Noblet-Ducoudré, N., Katragkou, E., Cardoso, R., Hoffmann, P., Jach, L., Soares, P., Sofiadis, G., Strada, S., Strandberg, G., Tölle, M., and Warrach-Sagi, K.: The opposing effects of re/af-forestation on the diurnal temperature cycle at the surface and in the lowest atmospheric model level in the European summer, J. Climate, 33, 1–58, https://doi.org/10.1175/JCLI-D-19-0624.1, 2020. a
Brock, B., Willis, I., and Sharp, M.: Measurement and parameterization of aerodynamic roughness length variations at Haut Glacier d'Arolla, Switzerland, J. Glaciol., 52, 281–297, https://doi.org/10.3189/172756506781828746, 2006. a, b, c, d, e, f, g, h, i, j, k, l, m, n, o
Burakowski, E., Tawfik, A., Ouimette, A., Lepine, L., Novick, K., Ollinger, S., Zarzycki, C., and Bonan, G.: The role of surface roughness, albedo, and Bowen ratio on ecosystem energy balance in the Eastern United States, Agr. Forest Meteorol., 249, 367–376, https://doi.org/10.1016/j.agrformet.2017.11.030, 2018. a
Callot, Y., Marticorena, B., and Bergametti, G.: Geomorphologic approach for modelling the surface features of arid environments in a model of dust emissions: application to the Sahara desert, Geodin. Acta, 13, 245–270, https://doi.org/10.1016/S0985-3111(00)01044-5, 2000. a
Chen, L. and Dirmeyer, P. A.: Differing Responses of the Diurnal Cycle of Land Surface and Air Temperatures to Deforestation, J. Climate, 32, 7067–7079, https://doi.org/10.1175/JCLI-D-19-0002.1, 2019. a
Chen, L. and Dirmeyer, P. A.: Reconciling the disagreement between observed and simulated temperature responses to deforestation, Nat. Commun., 11, 202, https://doi.org/10.1038/s41467-019-14017-0, 2020. a
Chen, Y., Yang, K., Zhou, D., Qin, J., and Guo, X.: Improving the Noah Land Surface Model in Arid Regions with an Appropriate Parameterization of the Thermal Roughness Length, J. Hydrometeorol., 11, 995–1006, https://doi.org/10.1175/2010JHM1185.1, 2010. a, b
Chen, Y., Yang, K., He, J., Qin, J., Shi, J., Du, J., and He, Q.: Improving land surface temperature modeling for dry land of China, J. Geophys. Res.-Atmos., 116, D20104, https://doi.org/10.1029/2011JD015921, 2011. a
Choudhury, B. J. and Monteith, J. L.: A four-layer model for the heat budget of homogeneous land surfaces, Q. J. Roy. Meteor. Soc., 114, 373–398, https://doi.org/10.1002/qj.49711448006, 1988. a
Claquin, T., Schulz, M., Balkanski, Y., and Boucher, O.: Uncertainties in assessing radiative forcing by mineral dust, Tellus B, 50, 491–505, https://doi.org/10.3402/tellusb.v50i5.16233, 1998. a
Csavina, J., Field, J., Félix, O., Corral-Avitia, A. Y., Sáez, A. E., and Betterton, E. A.: Effect of wind speed and relative humidity on atmospheric dust concentrations in semi-arid climates, Sci. Total Environ., 487, 82–90, https://doi.org/10.1016/j.scitotenv.2014.03.138, 2014. a
Danabasoglu, G., Lamarque, J.-F., Bacmeister, J., Bailey, D. A., DuVivier, A. K., Edwards, J., Emmons, L. K., Fasullo, J., Garcia, R., Gettelman, A., Hannay, C., Holland, M. M., Large, W. G., Lauritzen, P. H., Lawrence, D. M., Lenaerts, J. T. M., Lindsay, K., Lipscomb, W. H., Mills, M. J., Neale, R., Oleson, K. W., Otto-Bliesner, B., Phillips, A. S., Sacks, W., Tilmes, S., van Kampenhout, L., Vertenstein, M., Bertini, A., Dennis, J., Deser, C., Fischer, C., Fox-Kemper, B., Kay, J. E., Kinnison, D., Kushner, P. J., Larson, V. E., Long, M. C., Mickelson, S., Moore, J. K., Nienhouse, E., Polvani, L., Rasch, P. J., and Strand, W. G.: The Community Earth System Model Version 2 (CESM2), J. Adv. Model. Earth Sy., 12, e2019MS001916, https://doi.org/10.1029/2019MS001916, 2020. a, b
Davin, E. L. and de Noblet-Ducoudré, N.: Climatic impact of global-scale deforestation: Radiative versus nonradiative processes, J. Climate, 23, 97–112, https://doi.org/10.1175/2009JCLI3102.1, 2010. a
Dirmeyer, P., Gao, X., Zhao, M., Guo, Z., Oki, T., and Hanasaki, N.: GSWP-2: Multimodel Analysis and Implications for Our Perception of the Land Surface, B. Am. Meteorol. Soc., 87, 1381–1398, https://doi.org/10.1175/BAMS-87-10-1381, 2006. a
Dolman, A.: Estimates of roughness length and zero plane displacement for a foliated and non-foliated oak canopy, Agr. Forest Meteorol., 36, 241–248, https://doi.org/10.1016/0168-1923(86)90038-9, 1986. a
Duveiller, G., Hooker, J., and Cescatti, A.: The mark of vegetation change on Earth's surface energy balance, Nat. Commun., 9, https://doi.org/10.1038/s41467-017-02810-8, 2018. a, b, c, d
Duveiller, G., Filipponi, F., Ceglar, A., Bojanowski, J., Alkama, R., and Cescatti, A.: Revealing the widespread potential of forests to increase low level cloud cover, Nat. Commun., 12, https://doi.org/10.1038/s41467-021-24551-5, 2021. a, b
ESA: Land Cover CCI Product User Guide Version 2, Tech. Rep., ESA [data set], http://maps.elie.ucl.ac.be/CCI/viewer/download/ESACCI-LC-Ph2-PUGv2_2.0.pdf (last access: 23 February 2022), 2017. a
Fisher, R. A., Muszala, S., Verteinstein, M., Lawrence, P., Xu, C., McDowell, N. G., Knox, R. G., Koven, C., Holm, J., Rogers, B. M., Spessa, A., Lawrence, D., and Bonan, G.: Taking off the training wheels: the properties of a dynamic vegetation model without climate envelopes, CLM4.5(ED), Geosci. Model Dev., 8, 3593–3619, https://doi.org/10.5194/gmd-8-3593-2015, 2015. a
Fitzpatrick, N., Radić, V., and Menounos, B.: A multi-season investigation of glacier surface roughness lengths through in situ and remote observation, The Cryosphere, 13, 1051–1071, https://doi.org/10.5194/tc-13-1051-2019, 2019. a, b, c, d, e, f
Greeley, R., Blumberg, D. G., McHone, J. F., Dobrovolskis, A., Iversen, J. D., Lancaster, N., Rasmussen, K. R., Wall, S. D., and White, B. R.: Applications of spaceborne radar laboratory data to the study of aeolian processes, J. Geophys. Res.-Planets, 102, 10971–10983, https://doi.org/10.1029/97JE00518, 1997. a, b, c
Grimmond, C. S. B. and Oke, T. R.: Aerodynamic Properties of Urban Areas Derived from Analysis of Surface Form, J. Appl. Meteorol., 38, 1262–1292, https://doi.org/10.1175/1520-0450(1999)038<1262:APOUAD>2.0.CO;2, 1999. a
Hu, X., Shi, L., Lin, L., and Magliulo, V.: Improving surface roughness lengths estimation using machine learning algorithms, Agr. Forest Meteorol., 287, 107956, https://doi.org/10.1016/j.agrformet.2020.107956, 2020. a, b, c, d, e, f, g, h, i, j, k
Hugenholtz, C., Brown, O., and Barchyn, T.: Estimating aerodynamic roughness (z0) from terrestrial laser scanning point cloud data over un-vegetated surfaces, Aeolian Res., 10, 161–169, https://doi.org/10.1016/j.aeolia.2013.03.004, 2013. a, b, c, d
Hurrell, J. W., Hack, J. J., Shea, D., Caron, J. M., and Rosinski, J.: A New Sea Surface Temperature and Sea Ice Boundary Dataset for the Community Atmosphere Model, J. Climate, 21, 5145–5153, https://doi.org/10.1175/2008JCLI2292.1, 2008. a
IPCC: Climate Change 2013: The Physical Science Basis. Contribution of Working Group I to the Fifth Assessment Report of the Intergovernmental Panel on Climate Change, edited by: Stocker, T. F., Qin, D., Plattner, G.-K., Tignor, M., Allen, S. K., Boschung, J., Nauels, A., Xia, Y., Bex, V., and Midgley, P. M., IPCC, ISBN 978-92-9169-138-8, 2013. a
IPCC: Summary for Policymakers. In: Climate Change and Land: an IPCC special report on climate change, desertification, land degradation, sustainable land management, food security, and greenhouse gas fluxes in terrestrial ecosystems, edited by: Shukla, P. R., Skea, J., Calvo Buendia, E., Masson-Delmotte, V., Pörtner, H.-O., Roberts, D. C., Zhai, P., Slade, R., Connors, S., van Diemen, R., Ferrat, M., Haughey, E., Luz, S., Neogi, S., Pathak, M., Petzold, J., Portugal Pereira, J., Vyas, P., Huntley, E., Kissick, K., Belkacemi, M., and Malley, J., in press, https://www.ipcc.ch/srccl/chapter/summary-for-policymakers/ (last access: 18 March 2022), 2019. a
Jin, M. and Liang, S.: An Improved Land Surface Emissivity Parameter for Land Surface Models Using Global Remote Sensing Observations, J. Climate, 19, 2867–2881, https://doi.org/10.1175/JCLI3720.1, 2006. a, b
Kanda, M., Inagaki, A., Miyamoto, T., Gryschka, M., and Raasch, S.: A New Aerodynamic Parametrization for Real UrbanSurfaces, Bound.-Lay. Meteorol., 148, 357–377, https://doi.org/10.1007/s10546-013-9818-x, 2013. a, b, c, d, e
Khanna, J., Medvigy, D., Fueglistaler, S., and Walko, R.: Regional dry-season climate changes due to three decades of Amazonian deforestation, Nat. Clim. Change, 7, 200–204, https://doi.org/10.1038/nclimate3226, 2017. a
Kim, H.: Global Soil Wetness Project Phase 3, http://hydro.iis.u-tokyo.ac.jp/GSWP3/ (last access: 23 February 2022), 2014. a, b
Klose, M., Jorba, O., Gonçalves Ageitos, M., Escribano, J., Dawson, M. L., Obiso, V., Di Tomaso, E., Basart, S., Montané Pinto, G., Macchia, F., Ginoux, P., Guerschman, J., Prigent, C., Huang, Y., Kok, J. F., Miller, R. L., and Pérez García-Pando, C.: Mineral dust cycle in the Multiscale Online Nonhydrostatic AtmospheRe CHemistry model (MONARCH) Version 2.0, Geosci. Model Dev., 14, 6403–6444, https://doi.org/10.5194/gmd-14-6403-2021, 2021. a
Laguë, M. M., Bonan, G. B., and Swann, A. L. S.: Separating the Impact of Individual Land Surface Properties on the Terrestrial Surface Energy Budget in both the Coupled and Uncoupled Land–Atmosphere System, J. Climate, 32, 5725–5744, https://doi.org/10.1175/JCLI-D-18-0812.1, 2019. a
Lawrence, D., Fisher, R., Koven, C., Oleson, K. W., Swenson, S. C., Vertenstein, M., Andre, B., Bonan, G. B., Ghimire, B., Van Kampenhout, L., Kennedy, D., Kluzek, E., Knox, R., Lawrence, P., Li, F., Li, H., Lombardozzi, D., Lu, Y., Perket, J., Riley, W., Sacks, W., Shi, M., Wieder, W., and Xu, C.: Technical Description of version 5.0 of the Community Land Model (CLM), 80307–300, NCAR, https://www.cesm.ucar.edu/models/cesm2/land/ (last access: 19 March 2022), 2018. a, b, c, d, e, f
Lawrence, D. M., Fisher, R. A., Koven, C. D., Oleson, K. W., Swenson, S. C., Bonan, G., Collier, N., Ghimire, B., van Kampenhout, L., Kennedy, D., Kluzek, E., Lawrence, P. J., Li, F., Li, H., Lombardozzi, D., Riley, W. J., Sacks, W. J., Shi, M., Vertenstein, M., Wieder, W. R., Xu, C., Ali, A. A., Badger, A. M., Bisht, G., van den Broeke, M., Brunke, M. A., Burns, S. P., Buzan, J., Clark, M., Craig, A., Dahlin, K., Drewniak, B., Fisher, J. B., Flanner, M., Fox, A. M., Gentine, P., Hoffman, F., Keppel-Aleks, G., Knox, R., Kumar, S., Lenaerts, J., Leung, L. R., Lipscomb, W. H., Lu, Y., Pandey, A., Pelletier, J. D., Perket, J., Randerson, J. T., Ricciuto, D. M., Sanderson, B. M., Slater, A., Subin, Z. M., Tang, J., Thomas, R. Q., Val Martin, M., and Zeng, X.: The Community Land Model Version 5: Description of New Features, Benchmarking, and Impact of Forcing Uncertainty, J. Adv. Model Earth Sy., 11, 4245–4287, https://doi.org/10.1029/2018MS001583, 2019. a, b
L'Ecuyer, T., Hang, Y., Matus, A., and Wang, Z.: Reassessing the Effect of Cloud Type on Earth’s Energy Balance in the Age of Active Spaceborne Observations. Part I: Top of Atmosphere and Surface, J. Climate, 32, 6197–6217, https://doi.org/10.1175/JCLI-D-18-0753.1, 2019. a
Lee, X., Goulden, M. L., Hollinger, D. Y., Barr, A., Black, T. A., Bohrer, G., Bracho, R., Drake, B., Goldstein, A., Gu, L., Katul, G., Kolb, T., Law, B. E., Margolis, L. H., Meyers, T., Monson, R., Munger, W., Oren, R., Paw U, K. T., Richardson, A. D., Schmid, H. P. Staebler, R., Wofsy, S., and Zhao, L.: Observed increase in local cooling effect of deforestation at higher latitude, Nature, 479, 384–387, https://doi.org/10.1038/nature10588, 2011. a
Li, Z., Lyu, S., Zhao, L., Wen, L., Ao, Y., and Wang, S.: Turbulent transfer coefficient and roughness length in a high-altitude lake, Tibetan Plateau, Theor. Appl. Climatol., 124, 723–735, https://doi.org/10.1007/s00704-015-1440-z, 2015. a, b
Luyssaert, S., Jammet, M., Stoy, P. C., Estel, S., Pongratz, J., Ceschia, E., Churkina, G., Don, A., Erb, K.-H., Ferlicoq, M., Gielen, B., Grünwald, T., Houghton, R. A., K., K., Knohl, A., Kolb, T., Kuemmerle, T., Laurila, T., Lohila, A., Loustau, D., McGrath, M. J., Meyfroidt, P., Moors, E. J., Naudts, K., Novick, K., Otto, J., Pilegaard, K., Pio, C. A., Rambal, S., C., R., Ryder, J., Suyker, A. E., Varlagin, A., Wattenback, M., and Dolman, A. J.: Land management and land-cover change have impacts of similar magnitude on surface temperature, Nat. Clim. Change, 4, 389–393, https://doi.org/10.1038/nclimate2196, 2014. a, b
Macdonald, R., Griffiths, R., and Hall, D.: An improved method for the estimation of surface roughness of obstacle arrays, Atmos. Environ., 32, 1857–1864, https://doi.org/10.1016/S1352-2310(97)00403-2, 1998. a, b
Marticorena, B., Chazette, P., Bergametti, G., Dulac, F., and Legrand, M.: Mapping the aerodynamic roughness length of desert surfaces from the POLDER/ADEOS bi-directional reflectance product, Int. J. Remote Sens., 25, 603–626, https://doi.org/10.1080/0143116031000116976, 2004. a, b, c
Marticorena, B., Kardous, M., Bergametti, G., Callot, Y., Chazette, P., Khatteli, H., Le Hégarat-Mascle, S., Maillé, M., Rajot, J.-L., Vidal-Madjar, D., and Zribi, M.: Surface and aerodynamic roughness in arid and semiarid areas and their relation to radar backscatter coefficient, J. Geophys. Res.-Earth, 111, F03017, https://doi.org/10.1029/2006JF000462, 2006. a, b, c
Maurer, K., Hardiman, B., Vogel, C., and Bohrer, G.: Canopy-structure effects on surface roughness parameters: Observations in a Great Lakes mixed-deciduous forest, Agr. Forest Meteorol., 177, 24–34, https://doi.org/10.1016/j.agrformet.2013.04.002, 2013. a, b, c
Maurer, K. D., Bohrer, G., Kenny, W. T., and Ivanov, V. Y.: Large-eddy simulations of surface roughness parameter sensitivity to canopy-structure characteristics, Biogeosciences, 12, 2533–2548, https://doi.org/10.5194/bg-12-2533-2015, 2015. a
Meier, R., Davin, E. L., Lejeune, Q., Hauser, M., Li, Y., Martens, B., Schultz, N. M., Sterling, S., and Thiery, W.: Evaluating and improving the Community Land Model's sensitivity to land cover, Biogeosciences, 15, 4731–4757, https://doi.org/10.5194/bg-15-4731-2018, 2018. a, b
Meier, R., Davin, E. L., Swenson, S. C., Lawrence, D. M., and Schwaab, J.: Biomass heat storage dampens diurnal temperature variations in forests, Environ. Res. Lett., 14, 084026, https://doi.org/10.1088/1748-9326/ab2b4e, 2019. a, b, c, d, e
Meier, R., Duveiller, G., Davin, E. L., Bonan, G. B., Lawrence, D. M., Hu, X., Prigent, C., and Seneviratne, S. I.: Supplementary Material of “Impacts of a Revised Surface Roughness Parameterization in the Community Land Model 5.1”, ETH Zürich [code/data], https://doi.org/10.3929/ethz-b-000503165, 2021. a
Menut, L., Pérez, C., Haustein, K., Bessagnet, B., Prigent, C., and Alfaro, S.: Impact of surface roughness and soil texture on mineral dust emission fluxes modeling, J. Geophys. Res.-Atmos., 118, 6505–6520, https://doi.org/10.1002/jgrd.50313, 2013. a
Miller, R. L. and Tegen, I.: Climate Response to Soil Dust Aerosols, J. Climate, 11, 3247–3267, https://doi.org/10.1175/1520-0442(1998)011<3247:CRTSDA>2.0.CO;2, 1998. a
Nakai, T., Sumida, A., Daikoku, K., Matsumoto, K., Van der Molen, M., Kodama, Y., Kononov, A., Maximov, T., Dolman, H. A., Yabuki, H., Hara, T., and Ohta, T.: Parameterisation of aerodynamic roughness over boreal, cool- and warm-temperate forests, Agr. Forest Meteorol., 148, 1916–1925, https://doi.org/10.1016/j.agrformet.2008.03.009, 2008. a, b, c, d, e
Nakayama, H., Takemi, T., and Nagai, H.: LES Analysis of the Aerodynamic Surface Properties for Turbulent Flows over Building Arrays with Various Geometries, J. Appl. Meteorol. Clim., 50, 1692–1712, https://doi.org/10.1175/2011JAMC2567.1, 2011. a, b
Nield, J. M., King, J., Wiggs, G. F. S., Leyland, J., Bryant, R. G., Chiverrell, R. C., Darby, S. E., Eckardt, F. D., Thomas, D. S. G., Vircavs, L. H., and Washington, R.: Estimating aerodynamic roughness over complex surface terrain, J. Geophys. Res.-Atmos., 118, 12948–12961, https://doi.org/10.1002/2013JD020632, 2013. a, b, c
Oleson, K. W., Bonan, G. B., Feddema, J., Vertenstein, M., and Grimmond, C. S. B.: An Urban Parameterization for a Global Climate Model. Part I: Formulation and Evaluation for Two Cities, J. Appl. Meteorol. Clim., 47, 1038–1060, https://doi.org/10.1175/2007JAMC1597.1, 2008. a
Oleson, K. W., Bonan, G. B., Feddema, J., Vertenstein, M., and Kluzek, E.: Technical description of an urban parameterization for the Community Land Model (CLMU), No. NCAR/TN-480+STR, University Corporation for Atmospheric Research, https://doi.org/10.5065/D6K35RM9, 2010. a
Owen, P. and Thomson, W.: Heat transfer across rough surfaces, J. Fluid Mech., 15, 321–334, 1963. a, b
Prigent, C., Tegen, I., Aires, F., Marticorena, B., and Zribi, M.: Estimation of the aerodynamic roughness length in arid and semi-arid regions over the globe with the ERS scatterometer, J. Geophys. Res.-Atmos., 110, D09205, https://doi.org/10.1029/2004JD005370, 2005. a, b, c, d, e, f, g, h, i, j, k, l, m, n, o
Prigent, C., Jiménez, C., and Catherinot, J.: Comparison of satellite microwave backscattering (ASCAT) and visible/near-infrared reflectances (PARASOL) for the estimation of aeolian aerodynamic roughness length in arid and semi-arid regions, Atmos. Meas. Tech., 5, 2703–2712, https://doi.org/10.5194/amt-5-2703-2012, 2012. a, b
Raupach, M.: Drag and drag partition on rough surfaces, Boundary-Lay. Meteorol., 60, 375–395, https://doi.org/10.1007/BF00155203, 1992. a, b, c, d, e, f, g, h, i, j, k, l
Raupach, M.: Simplified expressions for vegetation roughness length and zero-plane displacement as functions of canopy height and area index, Bound.-Lay. Meteorol., 71, 211–216, https://doi.org/10.1007/BF00709229, 1994. a, b, c, d, e, f, g
Schultz, N. M., Lawrence, P. J., and Lee, X.: Global satellite data highlights the diurnal asymmetry of the surface temperature response to deforestation, J. Geophys. Res.-Biogeo., 122, 903–917, https://doi.org/10.1002/2016JG003653, 2017. a
Sellers, P. J., Mintz, Y., Sud, Y. C., and Dalcher, A.: A Simple Biosphere Model (SIB) for Use within General Circulation Models, J. Atmos. Sci., 43, 505–531, https://doi.org/10.1175/1520-0469(1986)043<0505:ASBMFU>2.0.CO;2, 1986. a
Shaw, R. H. and Pereira, A.: Aerodynamic roughness of a plant canopy: A numerical experiment, Agric. Meteorol., 26, 51–65, https://doi.org/10.1016/0002-1571(82)90057-7, 1982. a
Stilla, D., Zribi, M., Pierdicca, N., Baghdadi, N., and Huc, M.: Desert Roughness Retrieval Using CYGNSS GNSS-R Data, Remote Sens., 12, 743, https://doi.org/10.3390/rs12040743, 2020. a, b
Subin, Z. M., Riley, W. J., and Mironov, D.: An improved lake model for climate simulations: Model structure, evaluation, and sensitivity analyses in CESM1, J. Adv. Model. Earth Sy., 4, M02001, https://doi.org/10.1029/2011MS000072, 2012. a, b, c
Sud, Y. C., Shukla, J., and Mintz, Y.: Influence of Land Surface Roughness on Atmospheric Circulation and Precipitation: A Sensitivity Study with a General Circulation Model, J. Appl. Meteorol. Clim., 27, 1036–1054, https://doi.org/10.1175/1520-0450(1988)027<1036:IOLSRO>2.0.CO;2, 1988. a
Swenson, S. C., Burns, S. P., and Lawrence, D. M.: The Impact of Biomass Heat Storage on the Canopy Energy Balance and Atmospheric Stability in the Community Land Model, J. Adv. Model. Earth Sy., 11, 83–98, https://doi.org/10.1029/2018MS001476, 2019. a, b
Tanner, C. B. and Pelton, W. L.: Potential evapotranspiration estimates by the approximate energy balance method of Penman, J. Geophys. Res., 65, 3391–3413, https://doi.org/10.1029/JZ065i010p03391, 1960. a
Trigo, I. F., Boussetta, S., Viterbo, P., Balsamo, G., Beljaars, A., and Sandu, I.: Comparison of model land skin temperature with remotely sensed estimates and assessment of surface-atmosphere coupling, J. Geophys. Res.-Atmos., 120, 12096–12111, https://doi.org/10.1002/2015JD023812, 2015. a
van Tiggelen, M., Smeets, P. C. J. P., Reijmer, C. H., Wouters, B., Steiner, J. F., Nieuwstraten, E. J., Immerzeel, W. W., and van den Broeke, M. R.: Mapping the aerodynamic roughness of the Greenland Ice Sheet surface using ICESat-2: evaluation over the K-transect, The Cryosphere, 15, 2601–2621, https://doi.org/10.5194/tc-15-2601-2021, 2021. a, b, c
Vautard, R., Cattiaux, J., Yiou, P., Thépaut, J.-N., and Ciais, P.: Northern Hemisphere atmospheric stilling partly attributed to an increase in surface roughness, Nat. Geosci., 3, 756–761, https://doi.org/10.1038/ngeo979, 2010. a
Wan, Z., Hook, S., and Hulley, G.: MYD11C3 MODIS/Aqua Land Surface Temperature/Emissivity Monthly L3 Global 0.05Deg CMG V006, NASA Earth Data [data set], https://doi.org/10.5067/MODIS/MYD11C3.006, 2015. a, b
Wang, F., Ni, G., Riley, W. J., Tang, J., Zhu, D., and Sun, T.: Evaluation of the WRF lake module (v1.0) and its improvements at a deep reservoir, Geosci. Model Dev., 12, 2119–2138, https://doi.org/10.5194/gmd-12-2119-2019, 2019. a, b
Wang, Z., Schaaf, C. B., Strahler, A. H., Chopping, M. J., Román, M. O., Shuai, Y., Woodcock, C. E., Hollinger, D. Y., and Fitzjarrald, D. R.: Evaluation of MODIS albedo product (MCD43A) over grassland, agriculture and forest surface types during dormant and snow-covered periods, Remote Sens. Environ., 140, 60–77, https://doi.org/10.1016/j.rse.2013.08.025, 2014. a, b
Weligepolage, K., Gieske, A., and Su, Z.: Surface roughness analysis of a conifer forest canopy with airborne and terrestrial laser scanning techniques, Int. J. Appl. Earth Obs., 14, 192–203, https://doi.org/10.1016/j.jag.2011.08.014, 2012. a
Wever, N.: Quantifying trends in surface roughness and the effect on surface wind speed observations, J. Geophys. Res.-Atmos., 117, D11104, https://doi.org/10.1029/2011JD017118, 2012. a
Wilks, D. S.: “The Stippling Shows Statistically Significant Grid Points”: How Research Results are Routinely Overstated and Overinterpreted, and What to Do about It, B. Am. Meteorol. Soc., 97, 2263–2273, https://doi.org/10.1175/BAMS-D-15-00267.1, 2016. a, b
Winckler, J., Reick, C. H., Bright, R. M., and Pongratz, J.: Importance of Surface Roughness for the Local Biogeophysical Effects of Deforestation, J. Geophys. Res.-Atmos., 124, 8605–8618, https://doi.org/10.1029/2018JD030127, 2019. a
Wu, M., Liu, X., Yang, K., Luo, T., Wang, Z., Wu, C., Zhang, K., Yu, H., and Darmenov, A.: Modeling Dust in East Asia by CESM and Sources of Biases, J. Geophys. Res.-Atmos., 124, 8043–8064, https://doi.org/10.1029/2019JD030799, 2019. a
Xu, L., Liu, H., Du, Q., and Wang, L.: Evaluation of the WRF-lake model over a highland freshwater lake in southwest China, J. Geophys. Res.-Atmos., 121, 13989–14005, https://doi.org/10.1002/2016JD025396, 2016. a, b
Yang, K., Koike, T., Fujii, H., Tamagawa, K., and Hirose, N.: Improvement of surface flux parametrizations with a turbulence-related length, Q. J. Roy. Meteor. Soc., 128, 2073–2087, https://doi.org/10.1256/003590002320603548, 2002. a, b, c
Yang, K., Koike, T., Ishikawa, H., Kim, J., Li, X., Liu, H., Liu, S., Ma, Y., and Wang, J.: Turbulent Flux Transfer over Bare-Soil Surfaces: Characteristics and Parameterization, J. Appl. Meteorol. Clim., 47, 276–290, https://doi.org/10.1175/2007JAMC1547.1, 2008. a, b, c, d, e, f, g, h, i, j, k, l, m
Yang, R. and Friedl, M.: Determination of Roughness Lengths for Heat and Momentum Over Boreal Forests, Bound.-Lay. Meteorol., 107, 581–603, https://doi.org/10.1023/A:1022880530523, 2003. a, b
Young, A. M., Friedl, M. A., Seyednasrollah, B., Beamesderfer, E., Carrillo, C. M., Li, X., Moon, M., Arain, M. A., Baldocchi, D. D., Blanken, P. D., Bohrer, G., Burns, S. P., Chu, H., Desai, A. R., Griffis, T. J., Hollinger, D. Y., Litvak, M. E., Novick, K., Scott, R. L., Suyker, A. E., Verfaillie, J., Wood, J. D., and Richardson, A. D.: Seasonality in aerodynamic resistance across a range of North American ecosystems, Agr. Forest Meteorol., 310, 108613, https://doi.org/10.1016/j.agrformet.2021.108613, 2021. a, b, c, d
Zeng, X. and Dickinson, R. E.: Effect of Surface Sublayer on Surface Skin Temperature and Fluxes, J. Climate, 11, 537–550, https://doi.org/10.1175/1520-0442(1998)011<0537:EOSSOS>2.0.CO;2, 1998. a, b, c, d, e, f, g, h, i, j
Zeng, X. and Wang, A.: Consistent Parameterization of Roughness Length and Displacement Height for Sparse and Dense Canopies in Land Models, J. Hydrometeorol., 8, 730–737, https://doi.org/10.1175/JHM607.1, 2007. a, b, c, d
Zeng, X., Wang, Z., and Wang, A.: Surface Skin Temperature and the Interplay between Sensible and Ground Heat Fluxes over Arid Regions, J. Hydrometeorol., 13, 1359–1370, https://doi.org/10.1175/JHM-D-11-0117.1, 2012. a, b, c, d
Zhou, Y., Sun, X., Zhu, Z., Zhang, R., Tian, J., Liu, Y., Guan, D.-X., and Yuan, G.: Surface roughness length dynamic over several different surfaces and its effects on modeling fluxes, Sci. China Ser. D, 49, 262–272, https://doi.org/10.1007/s11430-006-8262-x, 2006. a