the Creative Commons Attribution 4.0 License.
the Creative Commons Attribution 4.0 License.
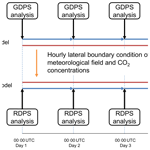
The Canadian atmospheric transport model for simulating greenhouse gas evolution on regional scales: GEM–MACH–GHG v.137-reg
Saroja M. Polavarapu
Douglas Chan
Michael Neish
In this study, we present the development of a regional atmospheric transport model for greenhouse gas (GHG) simulation based on an operational weather forecast model and a chemical transport model at Environment and Climate Change Canada (ECCC), with the goal of improving our understanding of the high-spatiotemporal-resolution interaction between the atmosphere and surface GHG fluxes over Canada and the United States. The regional model uses 10 km×10 km horizontal grid spacing and 80 vertical levels spanning the ground to 0.1 hPa. The lateral boundary conditions of meteorology and tracers are provided by the global transport model used for GHG simulation at ECCC. The performance of the regional model and added benefit of the regional model over our lower-resolution global models is investigated in terms of modelled CO2 concentration and meteorological forecast quality for multiple seasons in 2015. We find that our regional model has the capability to simulate the high spatial (horizontal and vertical) and temporal scales of atmospheric CO2 concentrations based on comparisons to surface and aircraft observations. In addition, the bias and standard deviation of forecast error in boreal summer are reduced by the regional model. Better representation of model topography in the regional model results in improved simulation of the CO2 diurnal cycle compared to the global model at Walnut Grove, California. The new regional model will form the basis of a flux inversion system that estimates regional-scale fluxes of GHGs over Canada.
- Article
(13644 KB) - Full-text XML
-
Supplement
(956 KB) - BibTeX
- EndNote
The works published in this journal are distributed under the Creative Commons Attribution 4.0 License. This licence does not affect the Crown copyright work, which is reusable under the Open Government Licence (OGL). The Creative Commons Attribution 4.0 License and the OGL are interoperable and do not conflict with, reduce or limit each other.
© Crown copyright 2020
The global mean atmospheric carbon dioxide (CO2) concentration or mixing ratio (in mole fractions of dry air) has been increasing since the industrial revolution, mainly due to anthropogenic emissions into the atmosphere, while terrestrial and oceanic uptake moderate the increase in CO2 in the atmosphere (Canadell et al., 2007; Le Quéré et al., 2009). Apart from this global increase, information about each component affecting the global carbon budget and its uncertainties is estimated and updated regularly at the global scale using a wide range of methods and data (Le Quéré et al., 2009, 2018). Since the ocean CO2 sink has been increasing constantly in line with the increased CO2 in the atmosphere (Le Quéré et al., 2018), the interannual variability of CO2 growth in the atmosphere is primarily attributed to that of terrestrial fluxes. Recently, the mean annual atmospheric CO2 growth rate reached a record high, mainly due to the impact of the El Niño–Southern Oscillation on the interannual variability of biospheric fluxes (Buchwitz et al., 2018) and increased net biospheric respiration in the tropics (Liu et al., 2017). Globally, increased CO2 and temperature are positively or negatively associated with terrestrial uptake by enhancing photosynthesis or respiration (Fernández-Martínez et al., 2018). Regionally, however, the carbon balance of Canadian boreal forest and its impact on the global carbon budget are highly uncertain, and the ecosystems of Canada are vulnerable to a changing climate (Kurz et al., 2013; Bush and Lemmen, 2019). Therefore, correctly accounting for biospheric fluxes over Canada is important for understanding both the global and regional carbon cycles.
Surface sources and sinks of CO2 can be estimated through inverse modelling using atmospheric CO2 concentrations as a constraint to adjust prior fluxes so as to minimise the difference between the modelled CO2 concentrations and observed values (Ciais et al., 2010). Many atmospheric inversion studies have been conducted to quantify surface CO2 fluxes on both global and regional scales (Tans et al., 1990; Gurney et al., 2002; Peters et al., 2007; Lauvaux et al., 2008, 2012b). Although there is a consensus of estimated fluxes at the global scale, significant discrepancies among different inversion system results still exist, especially in partitioning terrestrial fluxes at continental scales (Peylin et al., 2013; Crowell et al., 2019), due to the contribution of atmospheric transport model errors and prescribed fossil fuel emissions (Peylin et al., 2011; Gaubert et al., 2019).
In an atmospheric inversion of CO2, the transport model plays a key role in transforming the surface CO2 flux information into atmospheric CO2 concentrations and can be used as a verification tool for estimated surface CO2 fluxes (Ciais et al., 2010; Nisbet and Weiss, 2010; Bergamaschi et al., 2018). The errors caused by an imperfect transport model can introduce biases and uncertainties into estimated fluxes during the inversion process (Law et al., 1996; Gloor et al., 1999; Engelen et al., 2002; Houweling et al., 2010; Chevallier et al., 2010, 2014; Locatelli et al., 2013). Such errors may arise from a variety of sources: model formulation, meteorological fields and representativeness errors. Model formulation errors may arise from processes associated with parameterisations of vertical mixing within the planetary boundary layer (PBL) (Lauvaux and Davis, 2014), vertical mixing between the PBL and the free troposphere (Stephens et al., 2007), isentropic transport (Parazoo et al., 2012; Barnes et al., 2016), synoptic-scale variations due to advection and convection (Parazoo et al., 2008), and mid-latitude storm tracks (Parazoo et al., 2011). In fact, the impact of synoptic and mesoscale transport on the variability of CO2 is comparable with that of surface fluxes (Chan et al., 2004). Since an atmospheric transport model is driven by meteorology, uncertainties in meteorological models and observations are another important source of error in the transport of tracers (Liu et al., 2011; Miller et al., 2015; Polavarapu et al., 2016). Finally, representation error is also a source of errors in inversions. The mismatch between coarse-resolution transport model simulations and observations from the real CO2 field impacts the ability to resolve the sub-grid-scale variability of CO2. In particular, unresolved synoptic and mesoscale processes increase representation error (Engelen et al., 2002).
The sparseness of the CO2 observation network used in inversions is another major contributing factor to the uncertainty in estimated fluxes. Increasing the density of the surface observation network is beneficial for reducing uncertainty and improving the accuracy of retrieved fluxes in the context of both global (Bruhwiler et al., 2011) and regional inverse modelling (Lauvaux et al., 2012a; Schuh et al., 2013). Since a number of new measurement sites have been established over Canada and the US in recent decades (e.g. Worthy et al., 2005; Andrews et al., 2014; Bush et al., 2019), it should now be possible to obtain optimised fluxes on finer spatial scales and with reduced uncertainties. However, in order to better interpret information from spatially dense observation networks which contain information on strongly varying biospheric fluxes and strong sources of anthropogenic emissions, a high-resolution atmospheric transport model capable of capturing these signals is needed.
Resolving the fine-scale spatial and temporal variability of CO2 generated by heterogeneous land surface and complex topography, which is not resolved by the typical grid sizes of global models, is the primary motivation for regional-scale inverse modelling. Increased horizontal resolution could alleviate transport and representation errors and thus improve simulations of synoptic variations of CO2 concentrations (Patra et al., 2008; Remaud et al., 2018). Indeed, Gerbig et al. (2003) suggested that in order to resolve spatial variations of CO2 in the PBL over a continent, a horizontal grid spacing no larger than 30 km is required. In addition, Pillai et al. (2011) showed that a maximum horizontal resolution of 12 km is required to represent the variability of CO2 concentrations, especially over mountainous or complex terrain. To this end, several studies focusing on forward CO2 simulation at regional scales were carried out using different models and configurations over various regions of interest. One approach to simulate the atmospheric CO2 concentration at finer spatial and temporal resolution is using zooming or nested domains within a global model (Krol et al., 2005; Lin et al., 2018). Another option is to use a regional atmospheric transport model. Various kinds of regional-scale modelling studies have been conducted for the mid-continental region of North America (Díaz-Isaac et al., 2014), southwest France (Ahmadodv et al., 2007, 2009), western Europe (Kretschmer et al., 2014) and East Asia (Ballav et al., 2012). By increasing horizontal and vertical resolutions, regional models have an advantage over global models in terms of simulating CO2 concentrations, as shown by intercomparison experiments (Geels et al., 2007; Pillai et al., 2010; Díaz-Isaac et al., 2014).
At Environment and Climate Change Canada (ECCC), a carbon assimilation system (EC-CAS) is under development in order to estimate surface greenhouse gas (GHG) states and fluxes. To this end, a GHG forward modelling system which includes coupled meteorology and a tracer transport model with full model physics, namely GEM–MACH–GHG (Polavarapu et al., 2016), has been developed. GEM–MACH–GHG is based on an operational weather forecast model, the Global Environmental Multiscale (GEM) model at the Canadian Meteorological Centre (CMC) (Côté et al., 1998a, b; Girard et al., 2014), and a chemical transport model with complete tropospheric chemistry, the GEM-Modelling Air quality and Chemistry (GEM–MACH) model (Moran et al., 2010; Robichaud and Ménard, 2014; Makar et al., 2015), although the tropospheric chemistry module is not used in GEM–MACH–GHG simulations. GEM–MACH–GHG with 0.9∘ horizontal grid spacing is capable of simulating CO2 concentrations over the globe acceptably well in comparison with in situ and surface-based column-averaged CO2 observations. GEM–MACH–GHG was also used to investigate the uncertainty of CO2 transport across different global transport models (Polavarapu et al., 2018) and was tested with the Canadian Land Surface Scheme and Canadian Terrestrial Ecosystem Model (CLASS-CTEM) in order to eventually consistently simulate the atmosphere–land exchange of CO2 over the globe (Badawy et al., 2018). While a limited-area version of the GEM model exists for operational weather and air quality forecasting, the ability to simulate GHGs on a regional model domain over a continental region had not been developed before now.
In this paper, in order to obtain a better understanding of the variability of the atmospheric CO2 concentration at finer spatiotemporal scales over a continental region during a relatively long time period, a regional-scale atmospheric transport model for GHG simulation based on GEM–MACH–GHG is developed and tested. As a first step, CO2 simulations with a 10 km grid spacing are performed for the year 2015 on a domain covering most of Canada and the US. The performance of the new model is investigated using meteorological and CO2 concentration observations. In addition, the added benefit of the regional model over the global model in terms of CO2 simulation as well as weather forecasts is investigated. The article is organised as follows. A description of the model, data and methodologies used in this study is provided in Sect. 2. In Sect. 3 the performance of the regional model is assessed in terms of its meteorological forecast and CO2 simulation capability through comparisons with global model results. The benefit of higher horizontal resolution is investigated in Sect. 4, followed by a discussion of the results and a conclusion in Sect. 5.
2.1 Model description
2.1.1 GEM–MACH–GHG
GEM–MACH–GHG (Polavarapu et al., 2016) is a global GHG transport model, coupled with the meteorological model, wherein tracers are transported every time step. The horizontal resolution of the model is 0.9∘ using a globally uniform latitude–longitude grid (400×200 grid points), and there are 80 vertical levels spanning the surface to 0.1 hPa. For meteorology and tracer transport, a semi-Lagrangian advection scheme is used. Additionally, a global mass fixer was implemented for the transport of tracers in order to conserve the global mass of CO2 during model forecasts. The Kain and Fritsch (Kain and Fritsch, 1990; Kain, 2004) scheme was implemented for the convective transport of tracers through deep convection. More details about the model can be found in Polavarapu et al. (2016). While CO2 is regarded as an inert trace gas in the model, methane (CH4) and carbon monoxide (CO) utilise a simple parameterised climate chemistry. Specifically, the full troposphere chemistry package employed in GEM–MACH is replaced by simple hydroxide reactions related to oxidations of CH4 and CO in the atmosphere, along with the conversion of CH4 to CO.
As the operational version of GEM is updated periodically, model parameters are invariably tuned to optimise the performance of the model. In the previous configuration of GEM–MACH–GHG used in Polavarapu et al. (2016), thermal eddy diffusivity values within the PBL calculated by GEM were overridden to enhance vertical mixing of the CO2 concentration. A minimum value of 10 m2 s−1 was imposed within the PBL to prevent too little vertical mixing of CO2 in boreal summer because low values resulted in spuriously low CO2 concentrations on model levels near the surface in daytime when the magnitude of biospheric flux sinks is great (Polavarapu et al., 2016). In contrast, the lower limit imposed in the operational version of GEM–MACH is 0.1 m2 s−1, which was also empirically chosen for air quality applications. In this study, we use a more recent version of GEM which has better vertical mixing within the PBL than the version used in the previous study. This improvement allowed us to revise the thermal eddy diffusivity minimum imposed with the PBL to 1 from 10 m2 s−1 for all simulations (with both global and regional models) conducted in this study because the previous value resulted in CO2 concentrations that are too low at model levels near the surface over snow-covered regions, e.g. Alberta and Saskatchewan, in boreal winter. The impact of the revised value in summer daytime is minimal, and some improvements are found in nighttime, making the diurnal cycle of modelled CO2 concentrations more realistic overall.
Results from the global model with 0.9∘ horizontal grid spacing are used as the reference experiment for the verification of the newly developed regional model. However, to provide lateral boundary conditions (LBCs) of the CO2 concentration and meteorology to the regional model, a higher horizontal resolution of 0.45∘ (800×400 grid points) is needed to avoid numerical instability in meteorological forecasts caused by a drastic change in spatial resolution at the lateral boundary of the regional model domain. The original configuration is somewhat coarse to be used as LBCs for our regional model with 10 km horizontal resolution. Therefore, we also run the global model with a 0.45∘ horizontal grid spacing. All other configurations except horizontal grid spacing are the same as those used in the coarse-resolution (0.9∘) global model.
2.1.2 Extension to regional domain
For the regional model simulation, a rotated latitude–longitude map projection with approximately 10 km horizontal grid spacing and a hybrid vertical coordinate is used. The domain of the regional model covers most of Canada and the US, as shown in Fig. 1, and consists of 528 by 708 grid points. The number of vertical levels is the same as in the global model as described in Sect. 2.1.1, namely 80 levels spanning the atmosphere from the surface to 0.1 hPa. Since the number of grid points is also almost 5 times greater than that used in the 0.9∘ global model, the new regional model is more expensive to run. The physics packages used in the regional model are similar to those of the global model and GEM–MACH, and they include radiation (Li and Barker, 2005), boundary layer mixing (Bélair et al., 1999), shallow (Bélair et al., 2005) and deep convection (Kain and Fritsch, 1990; Kain, 2004), orographic gravity wave drag (McFarlane, 1987), and nonorographic gravity wave drag (Hines, 1997a, b) schemes. More details are provided in Mailhot et al. (1998).
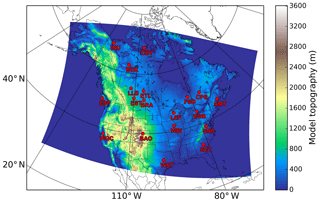
Figure 1Model topography of the regional model with 10 km horizontal grid spacing and CO2 measurement sites used in this study (red dot with site code).
For a simulation with tagged tracers to distinguish each component of CO2, e.g. those associated with biospheric, ocean and fossil fuel fluxes, a transport model should have the ability to simulate consistent masses across different components. In other words, the mass of the total CO2 field should exactly equal the sum of the tagged CO2 species, both globally and locally. This property is also required for estimating surface fluxes through Bayesian synthesis inversion (e.g. Enting, 2002). As already described in Polavarapu et al. (2016), the semi-Lagrangian advection scheme implemented in GEM alters mass slightly during model integration. The magnitude of the change for short-range forecasts is negligible, but this is not the case for the lengthy simulations of inert trace gases such as CO2. To compensate for mass losses of tracers, a mass conservation scheme (Bermejo and Conde, 2002) and a shape-preserving locally mass-conserving scheme (Sørensen et al., 2013) were applied to tracer fields. At the lateral boundaries of the regional model domain, a mass restoration scheme (Aranami et al., 2015 scheme) is applied. These schemes, however, can make mixing ratios across multiple tracers inconsistent since they correct for global mass changes in local regions where tracer gradients are large. Since each tagged component has a rather different spatial structure and gradients from the total CO2 field, the mass fixes made to the individual tagged variables need not be consistent with that made to the total field. As a result, the sum of each component may not equal the total CO2 concentration field. To address this issue, the monotonicity and mass conservation schemes applied during the advection step are turned off in the regional model. The impact of the configuration on the total mass of CO2 within the regional model domain was compared with the total mass of CO2 from an experiment using mass-related schemes turned on. The results show that there is no significant difference between two configurations in terms of the total mass of CO2 in the whole model domain or modelled CO2 concentrations at the lowest model level in which most surface-based in situ observation sites are located (not shown). This occurs because the majority of tracer mass is injected into the regional model domain through its lateral boundaries. The mass of CO2 from the surface flux is small compared to the total mass of CO2 in the atmosphere of the regional model domain, and the signal of surface fluxes exits the lateral boundaries during model integration before they reach the upper levels of the atmosphere (e.g. upper troposphere and stratosphere). Therefore, in the regional model, we obtain perfect “additivity” of the tagged components, with a negligible loss of mass.
2.2 Surface flux
In this study, the optimised CO2 fluxes from NOAA's CarbonTracker version CT2016 (Peters et al., 2007, with updates documented at http://carbontracker.noaa.gov, last access: 14 January 2020) were used as surface CO2 fluxes for CO2 simulations. The temporal resolution of the surface flux is 3 h. Because of their ready availability and careful validation, many studies aimed at global to regional to urban scales have used optimised fluxes from CarbonTracker for forward CO2 simulations (Houweling et al., 2010; Ballav et al., 2012; Díaz-Isaac et al., 2014; Polavarapu et al., 2016; Li et al., 2017; Wu et al., 2018).
The original CT2016 flux product is available with 1∘ by 1∘ horizontal grid spacing. However, the global and regional models have different horizontal grid spacing. Thus, fluxes are re-gridded to GEM's grids with 0.9∘, 0.45∘ and 10 km horizontal spacing grid, respectively, in a mass conservative way. In addition, one more redistribution method is applied to re-gridded fluxes on the 10 km grid. This process applies a land–sea mask to the regional model grid in order to avoid unphysical modelled CO2 concentrations caused by the different behaviour of vertical mixing over land and water grid cells. Because coarse-resolution fluxes do not contain all the information needed for high-resolution grid cells, considering only the size of a grid cell in re-gridding is insufficient because it would lead to modelled CO2 concentrations that are too low or too high relative to observed CO2 concentrations in regions of strong surface CO2 fluxes. With respect to fossil fuel emissions, for example, dynamic consistency is one of the important factors in regional-scale CO2 concentration simulations, in particular along coastal margins (Zhang et al., 2014). Hence, biospheric and fossil fuel flux components on water grid cells in which the fraction of land is less than 30 %, including lakes and oceans, are redistributed into land grid cells (within a radius of 30 grid points) in order to simulate realistic CO2 concentrations along coastlines in the regional model domain while minimising the impact of redistributed surface fluxes on CO2 simulation and while conserving the total mass of surface fluxes within the regional model domain.
2.3 Observations
Modelled CO2 concentrations are verified against observations from ObsPack (Masarie et al., 2014), which is maintained and provided by NOAA. For surface measurement sites, not all observations available in the regional model domain are used in the evaluation. The following selection criteria are applied: (1) sites were used to infer optimised CO2 fluxes in CT2016. Thus, we can expect that optimised surface fluxes from CT2016 provide information about sources and sinks consistent with observed CO2 concentrations at those sites so that differences in model simulation results may be attributable to model error. (2) Sites have continuous measurements (e.g. hourly data), as we want verify the results for all forecast hours. (3) Sites have no periods of missing data longer than 1 month (except for ESP – see Table 1 for a full list of station abbreviations – which has no data in January 2015), so results can be obtained for all seasons during the experimental period. As a result, 19 measurement sites (11 sites in Canada and 8 tower sites in the US) are selected as shown in Fig. 1 and listed in Table 1. For aircraft profiles of CO2, measurement sites available over Canada and the US in the year 2015 were selected.
2.4 Experiment design
Three experiments are performed as listed in Table 2. GLB90 is the reference experiment using the global model with 0.9∘ horizontal grid spacing. GLB45, which uses the global model with 0.45∘ horizontal grid spacing, is carried out to provide LBCs to the regional model. LAM is the regional model run with 10 km horizontal grid spacing. The simulation period is 1 year for 2015. In the analysis, the first 10 d of simulations are regarded as a spin-up period and discarded.
Figure 2 depicts the global and regional model cycles. In each forecast, the weather forecast and CO2 transport by forecasted wind fields are performed simultaneously at every time step. For the initial condition (IC) of meteorological fields for the two global models at the beginning of every cycle, the operational global analysis products from the global deterministic prediction system (GDPS; Buehner et al., 2015), whose horizontal resolution is roughly 25 km on a regular latitude–longitude grid or, as of 15 December 2015, a yin–yang grid (Qaddouri and Lee, 2011), are used. The archived data are interpolated to our low-resolution grids and topographies of GLB90 and GLB45 separately. For the IC of meteorological fields for the regional model, the operational regional analysis products from the regional deterministic prediction system (RDPS; Fillion et al., 2010; Caron et al., 2015) are used. The regional model grid is a subset of the model domain of the operational RDPS with the same horizontal resolution, sharing grid points on the same latitudes and longitudes. Therefore, it is not necessary to perform a horizontal interpolation at the start of every cycle. Also, a spin-up period for the meteorological forecast is unnecessary. Both operational global and regional meteorological analyses are produced four times per day with a 6 h assimilation window centred on the analysis time. We only use analyses produced at 00:00 UTC every day as an IC of meteorology. Thus, a 24 h weather forecast is produced during each 24 h CO2 cycle, and these forecasts are replaced by new analyses at every 00:00 UTC, with the exception of microphysics tracers which are retained to allow a hot start for the hydrometeor fields (Milbrandt et al., 2016). On the other hand, the mass of CO2 in a model grid volume is kept during cycles without replacements. The 24 h forecast of CO2 from the previous cycle is used as the IC of the CO2 field for the next cycle at 00:00 UTC. This is combined with the updated meteorological analysis for a complete initial state for the coupled model. Such 24 h forecast cycles are also used in other global model systems (e.g. Agustí-Panareda et al., 2014; Ott et al., 2015).
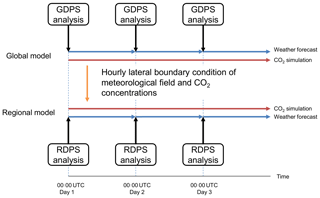
Figure 2Schematic diagram of GEM–MACH–GHG global and regional forward model cycles. Meteorological analyses are from CMC's operational global deterministic prediction system (GDPS) and regional deterministic prediction system (RDPS). Global and regional 24 h weather forecasts start at 00:00 UTC each day with operational analyses, while CO2 concentrations are kept during cycles. The global forward model provides the lateral boundary condition of meteorological and CO2 fields to the regional forward model every hour.
The IC of 3-D atmospheric CO2 concentrations at the beginning of all three experiments is taken from CT2016 CO2 concentrations at 00:00 UTC on 1 January 2015. The LBC of CO2 concentrations for the regional model are obtained from GLB45 and include hourly meteorological and CO2 fields.
One more possible configuration is using operational RDPS forecasts as meteorological LBCs for the regional model, which is similar to the configuration of the operational regional GEM–MACH (Moran et al., 2010). We tested this configuration and compared modelled CO2 concentrations with the LAM experiment's modelled CO2 concentrations. A negligible difference was found (not shown), and therefore we decided not to include that configuration in this study because our purpose is to develop an integrated global–regional forward modelling framework for GHG simulations as shown in Fig. 2.
2.5 Sampling method and metrics
In order to evaluate the performance of CO2 simulations and meteorological forecasts, a series of metrics are used as described below. Modelled or forecasted values are sampled at the observed location by applying horizontal and vertical interpolation to model fields rather than selecting the nearest grid point to measurement locations and selecting the time step closest to the observed time. To sample modelled CO2 concentrations, the sampling height above the ground level (or the model surface level) is considered to determine the altitude for vertical interpolation instead of using the actual sampling height above sea level (i.e. the sum of the altitude of an observation site plus intake height). Coarse-horizontal-resolution models cannot resolve the complex topography well (e.g. mountain regions) around some measurement sites. As a result, the altitude of model topography may be far above or below the actual height of a measurement site. If the height of a model-sampled observation is erroneously placed in the PBL (free troposphere) as a result of coarse model topography, this can result in an unphysical diurnal cycle of CO2 that is too strong (too weak) compared to observed values (see Agustí-Panareda et al., 2019). From a comparison of the two sampling methods, it was found that the LAM experiment is not sensitive to the vertical sampling method, as expected, because it can resolve actual topography well thanks to the higher spatial resolution, but the GLB90 experiment is sensitive to the method at a number of measurement sites (not shown). Thus, in order to reduce the topography mismatch problem in the coarse-resolution global model and investigate the impact of higher horizontal resolution without this problem, the method using intake height is used to help the global model capture the behaviour of the PBL variations with time and height. A detailed discussion of the vertical sampling methods in connection with horizontal resolution is found in Agustí-Panareda et al. (2019).
To analyse our results, including CO2 and meteorology, the bias and standard deviation of forecast error (SD) are used.
The bias is defined as
where N indicates the number of observations, Mi indicates modelled CO2 concentration or meteorological forecast, and Oi indicates the corresponding observation. The SD is defined as
where Xi=Mi-Oi and the overbar refers to the bias of the quantity.
To calculate the amplitude of the CO2 diurnal cycle (or other frequencies) for a measurement site or grid point, we use a discrete Fourier transform (DFT) technique. The linear trend in hourly CO2 time series from a specific location is removed first, and then the DFT technique is applied to the detrended CO2 time series to extract the amplitude of CO2 variability across temporal scales, from synoptic to diurnal to sub-daily scales, as discussed in Sect. 4.4.
3.1 Evaluation of meteorological fields
Before considering CO2 simulation results, weather forecasts from the three experiments are verified against observations over the regional model domain and are compared with each other. The motivation for doing this is two-fold: (1) to check that the meteorological forecasts from our regional model have not drifted away from the operational forecasts, which have been produced and maintained by the CMC for many decades, and (2) to compare the regional model results with the global model results. The first check is necessary because the configuration for weather prediction and the GEM model version used in this study are different from what was used to produce the operational forecast in 2015. For example, LBCs in RDPS were obtained from a global model forecast using a 33 km horizontal grid spacing (Caron et al., 2015) (or with a 25 km horizontal grid spacing as of 15 December 2015), with a different model domain extent and vertical coordinate. As shown by Polavarapu et al. (2016), the performance of the weather forecast by the global model is already well evaluated. The uncertainty of 24 h weather forecasts in the global model corresponding to the GLB90 experiment in this study is comparable with those of reanalyses provided by three operational centres; the monthly and zonal means of fields in 2009 and 2010 are within an acceptable range on global scales. Thus, in this section, we focus on the regional model results and on differences between experiments in the regional domain.
Figure 3 shows the bias and SD of the 24 h forecast error for the three experiments for vertical levels from 1000 to 10 hPa in July 2015. The same numbers of North American radiosonde observations are used in each of the three sets of verifications, and these are indicated on the right of each panel. The statistical significance of the differences using a T test for the means or an F test for the standard deviations at the 95 % confidence level was computed but is not shown explicitly. However, the discussion below uses this information in that we only mention results that are statistically significant. The three experiments show good agreement with observations in terms of bias and SD. For zonal wind, there are quite small differences among experiments, and the scores remain within the range of operational forecasts (not shown), except at 925 and 850 hPa where biases in the GLB90 and GLB45 experiments are slightly better than those in the LAM experiment (Fig. 3a). For wind speed, unlike the zonal wind, forecasts in the LAM experiment are better than those from the GLB90 experiment for levels from 925 to 50 hPa and better than those in the GLB45 experiment for levels from 300 to 70 hPa and at 700 hPa (Fig. 3b). For geopotential height, the forecasts in the LAM experiment are better than those in the GLB90 and GLB45 experiments from 400 to 10 hPa where relatively large positive biases in the GLB90 and GLB45 experiments exist. In addition, the SD in the LAM experiment is better at all vertical levels except a few levels (Fig. 3c). For temperature, the forecasts in the LAM experiment are better than those in the GLB90 and GLB45 experiments from 1000 to 70 hPa, with the exception of 150 hPa (Fig. 3d).
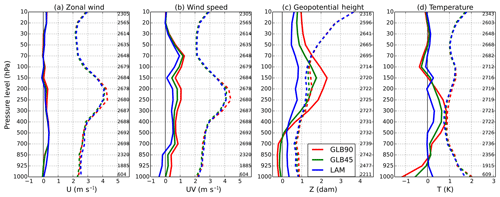
Figure 3The bias (solid line) and standard error (dashed line) of (a) zonal wind (m s−1), (b) wind speed (m s−1), (c) geopotential height (dam) and (d) temperature (K) from the GLB90 (red), GLB45 (green) and LAM (blue) experiments based on a comparison the 24 h forecasts against North American radiosondes for July 2015. The numbers on the left side of each panel denote the pressure level. The numbers on the right side of each panel denote the number of observations used in the statistics at each pressure level.
The scores for December 2015 are shown in Fig. 4. The differences in bias and SD among experiments are smaller than those in July. In addition, patterns in the reduction of bias and SD from coarse horizontal resolution to higher horizontal resolution can be seen much more clearly than in July, which means that the values in the GLB45 experiment are located between those of the GLB90 and LAM experiments. For zonal wind, there are quite small differences in bias and SD among experiments, as was the case in July (Fig. 4a). For wind speed, forecasts in the LAM experiment are better than those in the GLB90 experiment from 850 to 150 hPa as well as those in the GLB45 experiment at 500 and 400 hPa but not better at 925 hPa (Fig. 4b). For geopotential height, both bias and SD in the LAM experiment are better than those in the GLB90 experiment at most pressure levels except from 700 to 400 hPa, while the LAM experiment is better than GLB45 from 1000 and 925 hPa (Fig. 4c). For temperature, the bias in the LAM experiment is better than that in the GLB90 and GLB45 experiments from 925 to 250 hPa (Fig. 4d).
It is also worth considering how our meteorological forecasts compare to those of other systems. Agustí-Panareda et al. (2019) show root mean square errors (RMSEs) of vector wind for January and July 2014 from 1 d forecasts from the Copernicus Atmosphere Monitoring Service (CAMS). Our RMSE scores computed using the data from Figs. 3 and 4 for wind speed are shown in Table S1 in the Supplement, and these can be compared to their Fig. 4. Our LAM scores are lower than those of the 9 km CAMS at all heights in January and July. However, this is not a fair comparison since their scores are for a global domain, whereas we consider the North American domain, and their values are for 2014 but ours are for 2015. Nevertheless, the comparability of the scores further suggests that our LAM is performing well in terms of 24 h meteorological forecasts.
The number of available observations at 1000 hPa is much smaller than that of other pressure levels (see the numbers on right side of each panel in Figs. 3 and 4); because the typical altitudes of many sites are above the level corresponding to 1000 hPa and surface pressures may be below 1000 hPa depending on the synoptic situation, there is little confidence in the verification at this level by means of radiosondes. A better approach to rigorously investigate the performance of weather forecasts at lower levels is to use surface observations because of their much greater numbers (in both space and time). Therefore, weather forecasts in the three experiments are also verified against observations near the surface. Figure 5 shows the bias and SD of sea level pressure, 2 m temperature and 10 m wind speed as well as the Heidke skill score (HSS) (Wilks, 2006) of 10 m wind direction for July 2015. The SD of sea level pressure in the LAM experiment is lower than those in the GLB90 and GLB45 experiments, while the bias of sea level pressure in the LAM experiment is slightly lower than those of the GLB90 and GLB45 experiments. However, the difference between the LAM and the GLB90 and GLB45 biases does not exceed 0.5 hPa (Fig. 5a). The SDs of 2 m temperature and 10 m wind speed in the LAM experiment are smaller than those in the GLB90 and GLB45 experiments for all forecast hours (Fig. 5b and c), which implies that the error of forecasts from the LAM experiment fluctuates less than those of the GLB90 and GLB45 experiments. Also, the better results of the LAM experiment in 10 m wind direction is evident in the higher HSS of the LAM experiment (Fig. 5d). Higher HSS means a better forecast of wind direction. In addition, the root mean square errors (RMSEs) of variables in the LAM experiment are lower than those of the GLB90 and GLB45 experiments (not shown).
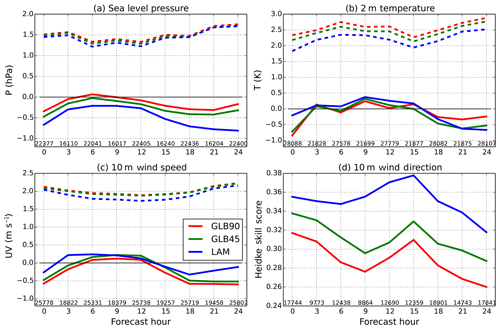
Figure 5The bias (solid line) and standard error (dashed line) of (a) sea level pressure (hPa), (b) 2 m temperature (K), (c) 10 m wind speed (m s−1) and (d) Heidke skill score of 10 m wind direction from the GLB90 (red), GLB45 (green) and LAM (blue) experiments based on a comparison of forecasts against surface-based stations over North America for July 2015. The numbers at the bottom of each panel denote the number of observations used in the statistics at each forecast hour.
In December 2015, the better forecasts in the LAM experiment compared to those of the GLB90 and GLB45 experiments can be seen more clearly (Fig. 6). The bias and SD of each variable in the LAM experiment are lower than those of the GLB90 and GLB45 experiments at most forecast hours (Fig. 6a–c), and higher HSS values of 10 m wind direction are evident at all forecast hours (Fig. 6d).
In summary, the LAM experiment produces reasonable meteorological forecasts in comparison with meteorological observations and better results relative to the GLB90 and GLB45 experiments, in particular at surface levels which are important for correctly capturing the flow of CO2 affected by surface fluxes and boundary layer mixing, with reductions in both bias and SD. Since forecasted meteorological fields are used to transport CO2 in each simulation individually, better CO2 simulations in the LAM experiment can be expected in the verification of modelled CO2 concentrations.
3.2 Evaluation of CO2 fields
The CO2 fields in the LAM and other experiments are investigated in terms of the monthly bias and SD of daily afternoon (12:00–16:00 LST) modelled CO2 concentrations at the measurement sites shown in Fig. 1 and listed in Table 1 (Fig. 7). Daily afternoon time was selected because this is what CT2016 used to estimate surface CO2 fluxes. Also, CT2016 results are included as a reference since this is what all our model experiments used as input fluxes. In general, bias and SD in summer are larger than in other seasons at most sites except BAO, SCT, WGC and WKT. Better results in CT2016 than in all three experiments at many sites can be seen, especially in June to October. Surface CO2 fluxes from CT2016 were inferred by minimising the difference between observations and forecasts of TM5 (Krol et al., 2005), which is the transport model used in CT2016. CT2016 fluxes thus contain an imprint of TM5's transport so that when GEM–MACH–GHG is forced with CT2016 fluxes, the mismatch of transport between the two models is evident (Polavarapu et al., 2016). As a result, larger biases in the three experiments relative to CT2016 at the abovementioned sites may be expected due to the discrepancies of modelled CO2 concentrations between CT2016 and GEM–MACH–GHG. In contrast, the three models show similar biases to each other except at ESP and WGC, especially in boreal summer. Since the LAM experiment uses LBCs of CO2 concentrations from the GLB45 experiment, information about the large-scale transport of the CO2 concentration is reflected in LAM experiment.
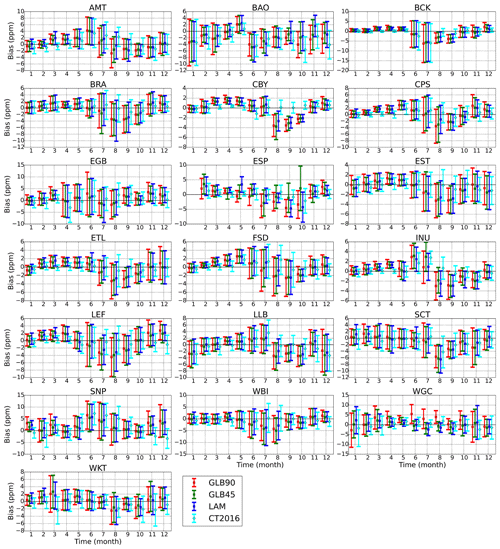
Figure 7Monthly mean bias of daily afternoon averaged (12:00–16:00 LST) CO2 concentrations from the GLB90 (red), GLB45 (green) and LAM (blue) experiments as well as CT2016 (cyan) at all measurement sites used in this study.
Now we consider the seasonal variation of the performance of the LAM experiment over the regional (North American) domain. The seasonal bias and SD of modelled CO2 concentrations in the LAM experiment are shown in Fig. 8 based on daily afternoon CO2 concentrations. In boreal winter (DJF) and spring (MAM), there are mainly positive biases at most Canadian sites, while SDs are small relative to other sites in the US. The magnitude of surface CO2 fluxes in those seasons over Canada is quite small (not shown), and thus bias at the Canadian sites contributes little to the overestimation of CO2 concentrations. This result suggests that biases included implicitly in the LBC of CO2 concentrations provided by the GLB45 experiment are more important in determining the biases in the regional model domain in those seasons. Four sites (BRA, EST, ETL and LLB) in Alberta and Saskatchewan show relatively large SD in DJF due to local influences of surface fluxes trapped within a shallow boundary layer by low temperatures. On the other hand, in boreal summer (JJA), large SD can be seen with negative biases at most sites. The large biases and SD in JJA may be attributed to errors in terrestrial CO2 fluxes within the regional model domain. As shown in Fig. 7, the GLB90 and GLB45 experiments underestimate CO2 concentrations over northern sites in JJA. That is also reflected in the underestimation of CO2 concentrations in the LAM experiment because the same surface fluxes are used in the simulations. Finally, in boreal autumn (SON), both biases and SD show moderate values between MAM and JJA. Negative biases at northern sites in the LAM experiment are partially due to biases in the LBCs obtained from the GLB45 experiment. In summary, the performance of the regional model partially depends on biases in the global model which provides the LBC, and the relative importance of these biases varies with season. In this regard, the use of CT2016 posterior fluxes to drive our global models exacerbates such biases. However, in the future when our global models provide their own flux estimates, such biases may be reduced. Furthermore, the need for a better understanding of the relative role of initial and boundary conditions and surface fluxes in controlling CO2 distributions within the regional model domain is evident. This is the subject of our future work.
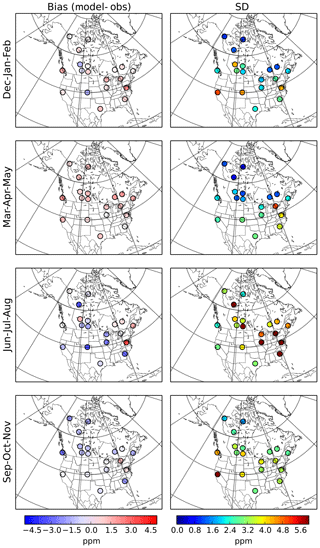
Figure 8Mean (left column) and standard deviation (right column) of the residuals between modelled CO2 from the LAM experiment and observed CO2 concentrations (modelled – observed) at each observation site over January to February and December 2015 (first row), March to May 2015 (second row), June to August 2015 (third row), and September to November 2015 (fourth row).
Since our regional model requires more computational resources (due to the greater number of grid points and shorter time step) than our global (GLB90) model, it is important to consider the added benefit of the higher horizontal resolution for CO2 simulations. In this section, modelled CO2 concentrations from the three experiments are analysed from the perspective of the spatial patterns and vertical profiles of CO2 concentrations as well as the reproducibility of temporal patterns against atmospheric CO2 observations.
4.1 Spatial patterns of surface CO2 concentrations
Biases between two experiments are compared pairwise for four seasons (Fig. 9). Three comparisons are shown because three experiments are conducted. Since bias can have both positive and negative values, absolute bias is used in the calculation. Blue (red) means that the higher-horizontal-resolution model simulated smaller (larger) absolute bias compared to the coarser one. In DJF, the GLB45 and LAM experiments are better than the GLB90 experiment. However, the LAM experiment is not better than the GLB45 experiment at US sites except WGC and BAO. In MAM, the differences among the experiments are the smallest, except at ESP near the west coast of North America. Differences between the LAM and GLB45 experiments at northern Canadian sites are quite small in DJF and MAM, which is associated with weak surface CO2 fluxes in those seasons. In JJA, the reduction in bias resulting from the higher-horizontal-resolution model can be seen clearly, and the magnitude of the reduction is higher, probably due to better weather simulation (less transport error) as shown in Figs. 3, 4, 5 and 6. The LAM experiment shows better results than both the GLB90 and GLB45 experiments at most sites. On the other hand, the GLB45 experiment is not better than the GLB90 experiment except at a few sites. This nonlinearity of the improvement with increased resolution is consistent with the results of Agustí-Panareda et al. (2019), although their conclusions are based on RMSE rather than absolute bias. In SON, the results are similar to those in JJA; namely, the LAM experiment is better than both the GLB90 and GLB45 experiments at most sites except ESP. At many sites, the benefit of finer grid spacing is evident, but higher horizontal grid spacing does not always guarantee a lower magnitude of bias for all sites. Part of the reason that improvement is not clear at certain sites in Fig. 9 may be due to the focus on afternoon mean values. For example, if we consider higher-temporal-frequency output (i.e. hourly residuals), LAM is better than GLB90 and GLB45 even at ESP in November (Fig. S1 in the Supplement).
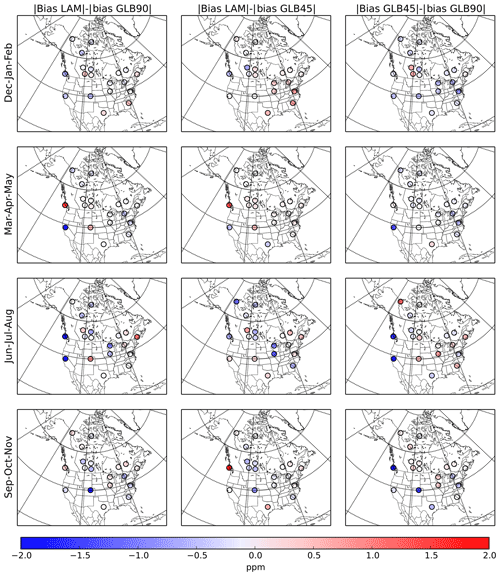
Figure 9The difference in absolute mean bias between GLB90 and LAM (first column), GLB45 and LAM (second column), and GLB90 and GLB45 (third column) over January to February and December 2015 (first row), March to May 2015 (second row), June to August 2015 (third row), and September to November 2015 (fourth row).
Figure 10 shows the differences in SD between two experiments. The spatial pattern of differences in SD is different from that of bias. More blue dots are evident, indicating that the SD of the higher-horizontal-resolution model is smaller than that of the coarser-horizontal-resolution model at most sites. Specifically, the LAM experiment shows better results than both the GLB90 and GLB45 experiments in DJF. As shown in Fig. 6c and d, better forecasts of 10 m wind speed and direction at screen level in the LAM experiment should help to reduce SD in the LAM experiment relative to the two global model experiments. In MAM, the impact of horizontal resolution is very small at most sites except near the southern boundary of the regional model domain due to the weak magnitude of the surface CO2 flux in this season. The ratio between CO2 concentrations resulting from surface CO2 fluxes within the regional model domain and background CO2 concentrations from the GLB45 experiment shows that the contribution of the surface CO2 flux is the lowest in MAM due to the small magnitude of the surface CO2 flux (not shown). In JJA, on the other hand, the magnitude of the difference is larger than in other seasons in both positive and negative directions. Finally, in SON, the improvement due to finer grid spacing can be seen.
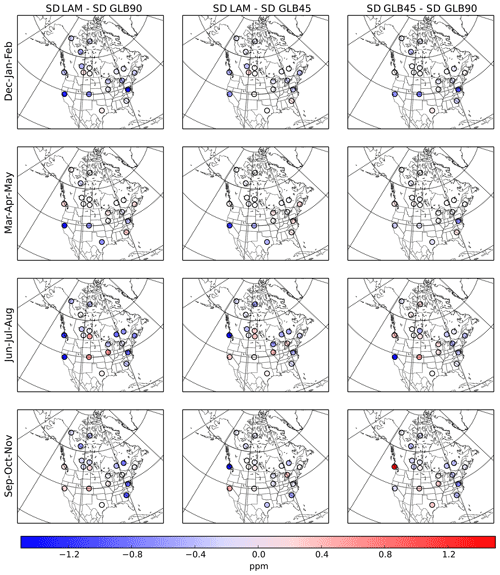
Figure 10The difference in the standard deviation of the residuals between modelled CO2 and observed CO2 concentrations (modelled – observed) between GLB90 and LAM (first column), GLB45 and LAM (second column), and GLB90 and GLB45 (third column) over January to February and December 2015 (first row), March to May 2015 (second row), June to August 2015 (third row), and September to November 2015 (fourth row).
In summary, the differences in bias and SD between experiments provide evidence of improvement in CO2 simulations due to finer horizontal resolution and better wind forecasts near the surface in the LAM experiment. The pattern of the differences is strongly associated with the spatial and seasonal patterns of the magnitude of surface CO2 fluxes used in the simulations.
4.2 Vertical profile of CO2 concentrations
We now consider the quality of modelled CO2 concentrations in the free troposphere. Observed profiles of CO2 can reveal the signatures of vertical mixing, so they can be used to measure the performance of transport models (Lin et al., 2006). The seasonal bias and SD of vertical profiles of modelled CO2 concentrations against NOAA aircraft profiles (Sweeney et al., 2015) over sites in Canada and the US inside the regional model domain are shown in Fig. 11. Modelled CO2 concentrations are sampled at the exact location and height of observations by applying vertical and horizontal interpolation to 3-D model fields at a time step close to the observed time. Then, averages over all profiles of modelled and observed values for a season are binned into 1 km thick layers. The three experiments generally overestimate CO2 concentrations in DJF (at 1000 m) and MAM and underestimate them in JJA and SON, which is consistent with the comparisons against surface CO2 measurement sites shown in Fig. 8. The magnitude of the bias does not exceed about 2 ppm in any altitude or season, and it decreases with altitude. Profiles of CO2 concentrations near the ground are difficult to simulate due to the strong influence of surface fluxes (Geels et al., 2007). The range of the biases in the three experiments is similar to that seen in our previous study (Polavarapu et al., 2016) as is the direction of the biases. Specifically, the bias changes sign with height, with positive biases at low altitudes and negative biases in DJF and MAM. In JJA and SON, the bias remains negative at almost all heights. The LAM experiment generally has the smallest biases for all seasons and altitudes, in particular below 4000 m in JJA when the influence of surface CO2 fluxes is significant through active vertical mixing. Lower wind speeds in boreal summer compared to other seasons causes the accumulation of surface fluxes over North America in the lower 4000 m (Sweeney et al., 2015). Reduced bias and SD of forecasted temperature profiles in the LAM experiment in July (Fig. 3d) may help to improve vertical advection in the LAM simulations relative to the global model experiments through improved buoyancy calculations. This may explain why the LAM experiment has a better ability to simulate vertical profiles of CO2.
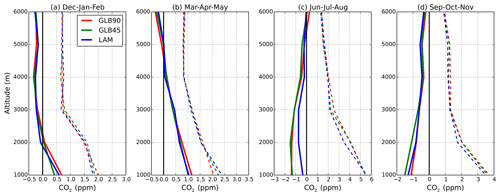
Figure 11Comparison of profiles of modelled CO2 concentrations from the GLB90 (red), GLB45 (green) and LAM (blue) experiments to NOAA aircraft observations for (a) January to February and December 2015, (b) March to May 2015, (c) June to August 2015, and (d) September to November 2015. Solid line denotes mean bias and dashed line denotes standard error. The sites used are as follows: Briggsdale, Colorado; Cape May, New Jersey; Dahlen, North Dakota; Estevan Point, British Columbia; East Trout Lake, Saskatchewan; Homer, Illinois; Park Falls, Wisconsin; Worcester, Massachusetts; Poker Flat, Alaska; Charleston, South Carolina; Southern Great Plains, Oklahoma; Sinton, Texas; Trinidad Head, California; West Branch, Iowa.
4.3 Temporal patterns
We evaluate modelled CO2 concentrations at various temporal scales including synoptic variability and the diurnal cycle. First, the synoptic variability of modelled CO2 concentrations is analysed. Figure 12 shows Taylor diagrams (Taylor, 2011) of modelled CO2 concentrations in the afternoon compared with observations. Since the domain of the LAM experiment covers a variety of geographic regions across Canada and the US, including mountain, continental and coastal sites, the synoptic variability of CO2 is not expected to be captured well at all sites. In DJF, the variability of modelled CO2 concentrations in the LAM experiment is closer to the observed variability than that captured in the GLB90 and GLB45 experiments, in accordance with the decreased SD seen in Fig. 10 (Fig. 12a). In MAM, the variability of modelled CO2 is scattered, with relatively lower correlations than other seasons (Fig. 12b). In general, due to the onset of the growing season in MAM, transport models tend to produce lower correlations with observed CO2 (Geels et al., 2004; Pillai et al., 2011; Agustí-Panareda et al., 2014). In JJA, despite having larger biases than other seasons (Fig. 7), correlations are quite reasonable, mostly lying between 0.6 and 0.95 (Fig. 12c). However, the variability in the CO2 concentrations tends to be overestimated. This could be mainly due to the large uncertainty in biospheric fluxes (Patra et al., 2008). Also, the range of correlations is the biggest – between approximately 0 and 0.95. In SON, the synoptic variability of CO2 is well captured by all experiments (Fig. 12d). Many sites have correlations higher than 0.9, standard deviations similar to observed variability and the least normalised RMSE (the distance from the reference point on the x axis) relative to other seasons. We expect the LAM experiment to produce higher correlations and smaller normalised RMSEs, as well as normalised standard deviations approaching 1. Indeed, the LAM experiment tends to simulate the observed variability of CO2 well, and it produces a smaller normalised RMSE relative to the GLB90 and GLB45 experiments to some extent, although the results vary according to site, and each experiment shows similar seasonal patterns which are driven by the weather forecasts and surface fluxes.
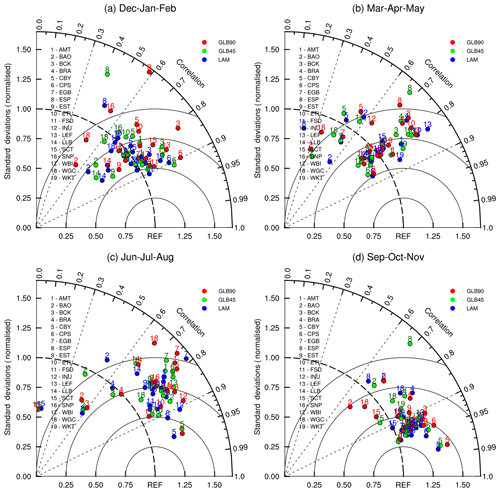
Figure 12Taylor diagram showing correlations and normalised standard deviations between daily afternoon modelled CO2 concentrations and observed CO2 concentrations from three models, GLB90 (red), GLB45 (green) and LAM (blue), over (a) January to February and December 2015, (b) March to May 2015, (c) June to August 2015, and (d) September to November 2015.
Thus far, modelled CO2 concentrations in the afternoon (12:00–16:00 LST) have been analysed. Henceforth, data at all times of the day and night are retained. Figure 13 shows the mean diurnal cycle of modelled and observed CO2 concentrations for July and December at WGC where the most significant differences among the three experiments are observed. In general, the three experiments simulate similar CO2 diurnal cycles for other sites (not shown). Data are available for three sampling levels at WGC in 2015. CT2016 is included as well for comparison purposes, but only results at the highest sampling level are shown because only observations at this level were used in the inversion in CT2016. The LAM experiment captures the CO2 diurnal cycle well, but the GLB90 and GLB45 experiments and CT2016 do not, especially in July (Fig. 13a, c and e). At the sampling level of 483 m, the GLB90 experiment overestimates morning CO2 concentrations, and CT2016 overestimates nighttime CO2 concentrations in July, while the GLB45 and LAM experiments capture the diurnal cycle (Fig. 13a) relatively well. This level (483 m) has a comparatively weak diurnal cycle because it is mostly decoupled from the surface at night, and daytime enhancements are significantly diluted relative to lower levels. At lower sampling levels of 91 and 30 m, both the GLB90 and GLB45 experiments overestimate nighttime CO2 concentrations in July, whereas the LAM experiment captures both day and nighttime CO2 concentrations well (Fig. 13c and e). This greater sensitivity to model resolution at night was also seen by Agustí-Panareda et al. (2019). WGC is located in a valley between two mountain ranges. The model topographies of GLB90 and GLB45 do not resolve this geography well due to their coarse horizontal resolutions. In contrast, the LAM experiment resolves the actual topography around the WGC site well relative to the two global models. In daytime, CO2 concentrations are well simulated in the LAM and GLB45 experiments due to the strong vertical mixing (Fig. 13a, c and e). In contrast, accumulated CO2 in nighttime still remains in the afternoon time in the GLB90 experiment, leading to an overestimation of CO2 in the afternoon at all sampling levels. In December, the LAM experiment simulates the CO2 concentration and its standard deviation slightly better at all sampling levels, while the GLB90 and GLB45 experiments underestimate CO2 concentrations (Fig. 13b, d and f).
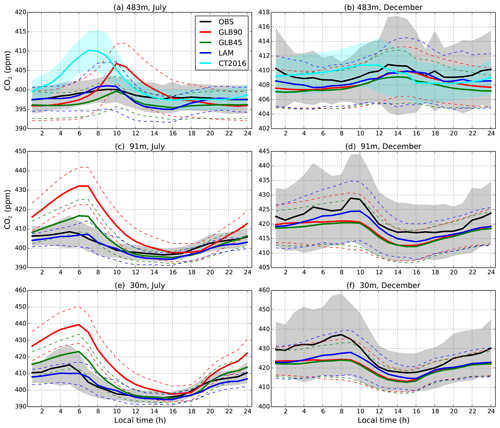
Figure 13Mean diurnal cycle of observed CO2 concentrations (black) and modelled CO2 concentrations from the GLB90 (red), GLB45 (green) and LAM (blue) experiments at WGC (Walnut Grove, California) for intake heights of (a) 483 m for July 2015, (b) 483 m for December 2015, (c) 91 m for July 2015, (d) 91 m for December 2015, (e) 30 m for July 2015 and (f) 30 m for December 2015. The grey (cyan) shaded region indicates 1 standard deviation above and below observed (CT2016) CO2 concentrations, while the dashed lines indicate the same for modelled CO2 concentrations. Note that CT2016 results are only available at 483 m.
In order to analyse CO2 time series across various temporal scales beyond the diurnal cycle, the DFT method explained in Sect. 2.5 is applied to hourly CO2 time series. Figure 14 shows the amplitude of hourly CO2 concentration time series across different temporal scales from 2 h to 92 d for the period from June to August 2015 at the LEF and WGC sites. Unfortunately, not all sites have hourly observations without missing values for the year 2015. These two sites have hourly data available for 3 months from June to August 2015 without missing values and fortunately reveal different properties. Thus, they were selected to illustrate the impact of increased horizontal resolution on CO2 simulations on the timescales captured by the models. At LEF, one sampling level, 396 m, satisfies our constraint of no missing data, and at WGC two sampling levels, 483 and 91 m, meet this constraint. At the LEF site, the three experiments capture the signals well across all temporal scales in observed CO2 time series, including synoptic and diurnal variations (Fig. 14a and b). The topography mismatch of the GLB90 and GLB45 experiments is relatively small at the LEF site. The intake height of measurements at LEF is 396 m above the ground at which laminar flow is more dominant than turbulent flow in nighttime so that the respiration signal from the surface does not reach the free troposphere and synoptic variability is more dominant (Davis et al., 2003; Wang et al., 2007). As a result, the differences in amplitude in the three experiments are less than about 0.8 ppm for all temporal periods (Fig. 14b). At the WGC site, on the other hand, as already shown in Fig. 13, the GLB90 experiment simulates diurnal cycles of CO2 that are too strong at lower sampling heights in July, which can also be clearly seen in Fig. 14c–f, with the largest overestimation at the lowest sampling level (Fig. 14e and f). However, it is not just the diurnal cycle of CO2 from June to August that is overestimated in the GLB90 experiment. Periods from sub-diurnal to longer day periods are also overestimated (Fig. 14c–f). Furthermore, while GLB45 performs better than GBL90, it also overestimates the diurnal cycle amplitudes and longer timescales at 483 and 91 m. Hence, the larger mismatch of topography results not only in inaccurate daily timescales but also other scales such as synoptic scales longer than 4 d. A similar result was also found for time periods of 92 to 300 d (Fig. S2). The amplitude of the diurnal cycle can also be computed in model space to illustrate its spatial variability as a function of model resolution (Fig. S3). With the same prescribed fluxes, greater spatial heterogeneity in the diurnal cycle amplitude occurs with increased resolution. However, the validation of these finer spatial scales requires a dense observation network and is not possible at present.
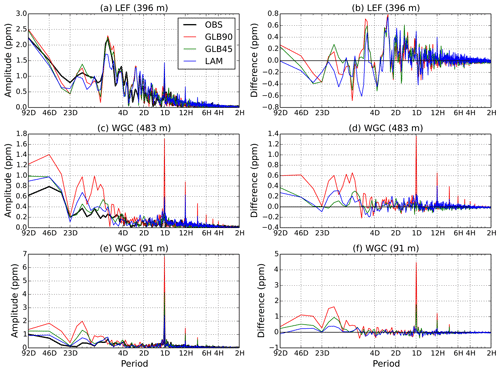
Figure 14The amplitude of hourly time series of observed CO2 (black) and modelled CO2 concentrations from the GLB90 (red), GLB45 (green) and LAM (blue) experiments across temporal scales from 2 h to 92 d at (a) LEF (intake height of 396 m) and (b) their differences, (c) WGC (intake height of 483 m) and (d) their differences, and (e) WGC (intake height of 91 m) and (f) their differences.
We have developed a regional atmospheric transport model for GHG simulation as an extension of GEM–MACH–GHG, which is ECCC's global atmospheric transport model for GHG simulation. The regional model shares much of the configuration of the global model, while its model domain is focused on Canada and the US. One gain from using the same vertical coordinate in both the regional and global models is that there is consistency at the lateral boundaries of the regional model domain. CO2 simulations using the same surface CO2 fluxes from CT2016 are performed with three configurations of two global models and one regional model in order to assess whether the newly developed regional model is working properly and to assess the benefit of the regional model over the global model in terms of weather forecasts and CO2 simulations. In a given experiment, a series of 24 h forecasts is replaced by operational analyses every cycle and used to transport CO2 every time step, whereas transported CO2 fields are not replaced but are kept during each 1-year simulation.
Meteorological forecasts in the three experiments are verified against North American radiosondes and surface observations at the screen level. All experiments show acceptable ranges of bias and SD compared to observations. Overall, meteorological forecasts in the regional model show better results than both global models, especially in wind speed and direction at the screen level, which are both of particular importance for CO2 transport near the surface. We demonstrate the improvement of weather forecasts with increasing horizontal resolution, which is most apparent in boreal winter. In addition, good-quality meteorological forecasts in the global model are also required for providing meteorological LBCs to the regional model with reduced errors at large scales. Indeed, the GLB45 experiment can provide good-quality meteorological LBCs to the regional model every hour, which is more frequent than when using reanalyses that are available at 3 h or 6 h intervals.
While the meteorological forecasts from the higher-resolution regional model are demonstrably better than those of the coarser-resolution global models, demonstrating improved CO2 simulations with higher resolution is more challenging. For example, the impact of biases in the LBCs provided by the GLB45 experiment on CO2 simulations near the Arctic region in the regional model is large, especially in boreal spring. In a regional-scale inverse modelling system, estimated fluxes within the regional model domain are strongly influenced by the inflow of CO2 from the global transport model through the lateral boundary (Schuh et al., 2010). Because LBCs of CO2 include information on sources and sinks outside the regional model domain, correct information at the lateral boundary is important to determine the sources and sinks in the regional model domain (Gerbig et al., 2003). As discussed in Polavarapu et al. (2016), GEM has different transport behaviour from the transport model used in CarbonTracker, in particular over the Arctic region, as seen in time series of CO2 concentrations and column-averaged CO2. Thus, our models are not expected to perform better than CT2016 because we use surface CO2 fluxes inferred by an inversion framework using a different transport model which has different transport behaviour. That is why our focus in this work is on the comparison of our regional and global models. We are able to find some benefits of our regional model over our global model when looking at the diurnal cycle of CO2 concentrations for particular sites at which large topography mismatches exist, e.g. WGC. Our global models did not capture diurnal cycles well, while our regional model did. This is a promising result because it suggests that using nighttime data in an inversion to estimate nighttime fluxes (e.g. Lauvaux et al., 2008) may be beneficial if a high-resolution model is used. Currently, a GHG state estimation system using GEM–MACH–GHG and ECCC's operational ensemble Kalman filter data assimilation system (Houtekamer et al., 2014) is under development. When posterior fluxes become available from our global model, this will alleviate the issue of model transport error mismatches with CarbonTracker. However, we will still have transport error, which is one of the biggest sources of posterior uncertainties in an inversion (Schuh et al., 2019). To address this issue, we plan to use multiple sources of meteorology to better account for transport error in posterior flux and uncertainty estimates.
The regional model produces lower SDs of CO2 at surface measurement sites, in line with its lower SD of meteorological forecasts. With respect to aircraft CO2 profile comparisons, clear improvement of the profiles of modelled CO2 in the LAM experiment occurs at altitudes lower than 4000 m in boreal summer. Although the regional model domain is vast enough to include most of Canada and the US so as to be able to estimate national- to provincial-scale surface GHG fluxes at finer spatial resolution via inverse modelling in the future, it is not easy to obtain better results everywhere. For example, at the ESP site located on the coastline of Vancouver Island, British Columbia, the LAM experiment does not have a lower bias of modelled CO2 than the GLB90 and GLB45 experiments in MAM and SON. Nonetheless, the overall performance of CO2 simulations by the regional model is better than our global models. It is well known that only afternoon CO2 concentrations are typically used in inversions due to the difficulty in capturing boundary layer evolution in most global transport models (Law et al., 2008; Patra et al., 2008). Noticeable improvement in reproducing the CO2 diurnal cycle by the regional model can be seen at WGC, which is located in complex terrain. Reduced topographic mismatch in the finer-horizontal-resolution model is the major driving force behind reduced sampling and representation error. This effect is not limited to just the diurnal cycle but also occurs for the synoptic variability of CO2 at the level where large-scale motions are dominant, and even more so at lower sampling levels near the surface. In addition, the potential benefit of reproducing detailed diurnal cycles over regions with the complex terrains hypothesised here is consistent with the findings of Agustí-Panareda et al. (2019).
Previous studies comparing high- and low-horizontal-resolution transport models for CO2 simulations concluded that some advantages can be attained by using higher horizontal resolution (Geels et al., 2007; Pillai et al., 2010; Díaz-Isaac et al., 2014). For example, a better resolved amplitude and phase of the short-term variability of CO2 (Geels et al., 2007), reduced representation errors (Pillai et al., 2010), and smaller-scale structures of modelled CO2 that are more sensitive to the distribution of CO2 fluxes (Díaz-Isaac et al., 2014) were attained by using higher spatial resolution in the transport model. Indeed, we also find similar results as mentioned above, but these advantages from the regional model experiment are not obtained at every observation site (Figs. 9 and 10). Basically, increasing horizontal resolution gives some positive impact to some extent, but it generally has a mixed impact in this study. Part of the reason may be due to the fact that our models are variants of the same model but with different grid spacing and/or domain. Furthermore, the same coarse-resolution surface fluxes were used with all models and this limits the potential for improvement (Remaud et al., 2018). In addition, the global model configurations used in this study already have relatively higher horizontal resolutions (0.9 and 0.45∘) compared to other coarse-resolution global transport models (e.g. Geels et al., 2007), and they all use the same number of (80) vertical levels as the regional model. Another major difference is that our global model is not an offline transport model which generally uses reanalyses as a meteorological driver for transport. Instead we take advantage of operational analyses to initialise weather forecasts every day and produce weather forecasts at every model time step. A major limitation in validating the overall improved ability to capture fine spatial scales may simply be the current sparsity of verifying observations of CO2. With vastly greater numbers of verifying observations, the meteorological simulations are demonstrably better with increased resolution. Since the regional model can better simulate the spatial heterogeneity of the diurnal cycle of CO2 in model space (Fig. S3), better observational density is needed to validate the performance of CO2 simulations in the regional model in more detail.
While this work has focused on the benefit of our higher-resolution regional model over our global model for CO2 simulation, both models are “online” in that the meteorology is coupled to the tracer transport every time step. An interesting question that was not addressed here is the impact of increased horizontal resolution in the context of an “offline” transport model which ingests meteorological analyses or reanalyses from another model (e.g. Kjellström et al., 2002; Geels et al., 2004, 2007). Additional errors then arise due to spatial and temporal interpolation from another model grid to the offline model grid.
A limitation of this study is the use of coarse-resolution surface CO2 fluxes in conjunction with the fine horizontal grid spacing of the regional model. For better simulation of CO2, not only high-quality meteorological forcing but also high-resolution prescribed surface fluxes are demanded (Locatelli et al., 2015). Higher-spatial- and temporal-resolution fluxes could lead to better simulation of CO2 concentrations (Feng et al., 2016; Lin et al., 2018) if the fluxes have correct space and time information about the distribution of sources and sinks of CO2 fluxes. The challenge is in obtaining high-spatial- and temporal-resolution surface fluxes that are accurate. One way to deal with this issue is to model biogenic fluxes explicitly at the same horizontal resolution as the transport model (e.g. Agustí-Panareda et al., 2019). Indeed, this is an avenue we plan to investigate in the future. Preliminary investigations with a high-resolution anthropogenic flux product revealed improved comparisons to observations at some sites but degradation at other sites. For that reason, we chose to start an investigation of the regional model by using fluxes with the same resolution as the global model and limiting the potential benefit of high resolution for improved meteorological depictions.
The LBCs of CO2 from the global model play an important role, as shown in Fig. 8, dominating the bias in the regional model when the magnitude of the surface flux is weak. In addition, the LBCs of meteorology also play an important role in CO2 simulations. For example, the meteorological IC and LBC contribute to the variability of daytime CO2 in the PBL (Díaz-Isaac et al., 2018). Thus, there is a need to better understand the relative importance of initial conditions, boundary conditions and surface fluxes for the performance of the regional model in order to better characterise these components of CO2 model error within the regional domain. Indeed, the predictability of CO2 on the regional domain and the relative role of initial and boundary conditions and surface fluxes in model error are topics currently under investigation.
There are a number of extensions to this work that are envisioned. For example, the newly developed regional model is not limited to CO2 simulations but also includes other greenhouse gases such as CH4. Thus, a separate validation of the regional model's ability to simulate CH4 is planned. The regional model can also be utilised to provide information (e.g. IC and LBC) to urban-scale forward or inverse modelling systems (e.g. Feng et al., 2016; Pugliese et al., 2018; Ishizawa et al., 2019). Lastly, and most importantly, an inverse modelling system for estimating surface CO2 fluxes is being developed using the new regional GHG transport model to better understand the carbon cycle in Canada at finer spatial and temporal scales.
The GEM–MACH–GHG model source code is publicly available at https://doi.org/10.5281/zenodo.3246556 (Neish et al., 2019) under the GNU Lesser General Public License version 2.1 (LGPL v2.1) or ECCC's Atmospheric Science and Technology licence version 3. The model output data are available at http://crd-data-donnees-rdc.ec.gc.ca/CCMR/pub/2019_Kim_GMD_Canadian_atmospheric_transport_model_for_simulating_greenhouse_gas_evolution_on_regional_scales/ (Kim and Neish, 2020).
The supplement related to this article is available online at: https://doi.org/10.5194/gmd-13-269-2020-supplement.
JK and MN developed model code. JK designed and carried out the experiments. All authors participated in the analysis of the results. The paper was prepared with contributions from all authors.
The authors declare that they have no conflict of interest.
We are grateful to Colm Sweeney (NOAA ESRL) for providing the NOAA aircraft profiles and to Ken Masarie of the NOAA Global Monitoring Division in Boulder, Colorado, for compiling ObsPack. The National Oceanic and Atmospheric Administration (NOAA) North American Carbon Program has funded the NOAA/ESRL Global Greenhouse Gas Reference Network Aircraft program. CarbonTracker CT2016 results were provided by NOAA ESRL, Boulder, Colorado, USA, from the website at http://carbontracker.noaa.gov. The ObsPack data (obspack_co2_1_GLOBALVIEWplus_v3.1_2017_10_18) were obtained for 2015 from https://doi.org/10.15138/G3T055 (ObsPack, 2017). We would like to thank Doug Worthy of the Atmospheric Science and Technology Directorate, Environment and Climate Change Canada, for developing and maintaining ECCC's greenhouse gas measurement network and for providing the CO2 concentration measurement data. We thank Marc L. Fischer for useful comments on the paper. Data collection at the WGC site was partially supported by the California Air Resources Board through work at the Lawrence Berkeley National Laboratory, operating under U.S. Department of Energy contract no. DE-AC02-05CH11231. We thank Monique Tanguay and Felix Vogel for their careful internal review.
This paper was edited by Axel Lauer and reviewed by two anonymous referees.
Agustí-Panareda, A., Massart, S., Chevallier, F., Boussetta, S., Balsamo, G., Beljaars, A., Ciais, P., Deutscher, N. M., Engelen, R., Jones, L., Kivi, R., Paris, J.-D., Peuch, V.-H., Sherlock, V., Vermeulen, A. T., Wennberg, P. O., and Wunch, D.: Forecasting global atmospheric CO2, Atmos. Chem. Phys., 14, 11959–11983, https://doi.org/10.5194/acp-14-11959-2014, 2014.
Agustí-Panareda, A., Diamantakis, M., Massart, S., Chevallier, F., Muñoz-Sabater, J., Barré, J., Curcoll, R., Engelen, R., Langerock, B., Law, R. M., Loh, Z., Morguí, J. A., Parrington, M., Peuch, V.-H., Ramonet, M., Roehl, C., Vermeulen, A. T., Warneke, T., and Wunch, D.: Modelling CO2 weather – why horizontal resolution matters, Atmos. Chem. Phys., 19, 7347–7376, https://doi.org/10.5194/acp-19-7347-2019, 2019.
Ahmadov R., Gerbig, C., Kretschmer, R., Koerner, S., Neininger, B., Dolman, A. J., and Sarrat, C.: Mesoscale covariance of transport and CO2 fluxes: Evidence from observations and simulations using the WRF-VPRM coupled atmosphere-biosphere model, J. Geophys. Res., 112, D22107, https://doi.org/10.1029/2007JD008552, 2007.
Ahmadov, R., Gerbig, C., Kretschmer, R., Körner, S., Rödenbeck, C., Bousquet, P., and Ramonet, M.: Comparing high resolution WRF-VPRM simulations and two global CO2 transport models with coastal tower measurements of CO2, Biogeosciences, 6, 807–817, https://doi.org/10.5194/bg-6-807-2009, 2009.
Andrews, A. E., Kofler, J. D., Trudeau, M. E., Williams, J. C., Neff, D. H., Masarie, K. A., Chao, D. Y., Kitzis, D. R., Novelli, P. C., Zhao, C. L., Dlugokencky, E. J., Lang, P. M., Crotwell, M. J., Fischer, M. L., Parker, M. J., Lee, J. T., Baumann, D. D., Desai, A. R., Stanier, C. O., De Wekker, S. F. J., Wolfe, D. E., Munger, J. W., and Tans, P. P.: CO2, CO, and CH4 measurements from tall towers in the NOAA Earth System Research Laboratory's Global Greenhouse Gas Reference Network: instrumentation, uncertainty analysis, and recommendations for future high-accuracy greenhouse gas monitoring efforts, Atmos. Meas. Tech., 7, 647–687, https://doi.org/10.5194/amt-7-647-2014, 2014.
Aranami, K., Davies, T., and Wood, N.: A mass restoration scheme for limited-area models with semi-Lagrangian advection, Q. J. Roy. Meteor. Soc., 141, 1795–1803, https://doi.org/10.1002/qj.2482, 2015.
Badawy, B., Polavarapu, S., Jones, D. B. A., Deng, F., Neish, M., Melton, J. R., Nassar, R., and Arora, V. K.: Coupling the Canadian Terrestrial Ecosystem Model (CTEM v. 2.0) to Environment and Climate Change Canada's greenhouse gas forecast model (v.107-glb), Geosci. Model Dev., 11, 631–663, https://doi.org/10.5194/gmd-11-631-2018, 2018.
Ballav, S., Patra, P. K., Takigawa, M., Ghosh, S., De, U. K., Maksyutov, S., Murayama, S., Mukai, H., and Hashimoto, S.: Simulation of CO2 Concentration over East Asia Using the Regional Transport Model WRF-CO2, J. Meteorol. Soc. Jpn., 90, 959–976, https://doi.org/10.2151/jmsj.2012-607, 2012.
Barnes, E. A., Parazoo, N., Orbe, C., and Denning, A. S.: Isentropic transport and the seasonal cycle amplitude of CO2, J. Geophys. Res.-Atmos., 121, 8106–8124, https://doi.org/10.1002/2016JD025109, 2016.
Bélair, S., Mailhot, J., Strapp, J. W., and MacPherson, J. I.: An Examination of Local versus Nonlocal Aspects of a TKE-Based Boundary Layer Scheme in Clear Convective Conditions, J. Appl. Meteorol., 38, 1499–1518, https://doi.org/10.1175/1520-0450(1999)038<1499:AEOLVN>2.0.CO;2, 1999.
Bélair, S., Mailhot, J., Girard, C., and Vaillancourt, P.: Boundary layer and shallow cumulus clouds in a medium-range forecast of a large-scale weather system, Mon. Weather Rev., 133, 1938–1960, https://doi.org/10.1175/MWR2958.1, 2005.
Bergamaschi, P., Danila, A., Weiss, R. F., Ciais, P., Thompson, R. L., Brunner, D., Levin, I., Meijer, Y., Chevallier, F., Janssens-Maenhout, G., Bovensmann, H., Crisp, D., Basu, S., Dlugokencky, E., Engelen, R., Gerbig, C., Günther, D., Hammer, S., Henne, S., Houweling, S., Karstens, U., Kort, E., Maione, M., Manning, A. J., Miller, J., Montzka, S., Pandey, S., Peters, W., Peylin, P., Pinty, B., Ramonet, M., Reimann, S., Röckmann, T., Schmidt, M., Strogies, M., Sussams, J., Tarasova, O., van Aardenne, J., Vermeulen, A. T., and Vogel, F.: Atmospheric monitoring and inverse modelling for verification of greenhouse gas inventories, Publications Office of the European Union, Luxembourg, https://doi.org/10.2760/759928, 2018.
Bermejo, R. and Conde, J.: A conservative quasi-monotone semi-Lagrangian scheme, Mon. Weather Rev. 130, 423–430, 2002.
Bruhwiler, L. M. P., Michalak, A. M., and Tans, P. P.: Spatial and temporal resolution of carbon flux estimates for 1983–2002, Biogeosciences, 8, 1309–1331, https://doi.org/10.5194/bg-8-1309-2011, 2011.
Buchwitz, M., Reuter, M., Schneising, O., Noël, S., Gier, B., Bovensmann, H., Burrows, J. P., Boesch, H., Anand, J., Parker, R. J., Somkuti, P., Detmers, R. G., Hasekamp, O. P., Aben, I., Butz, A., Kuze, A., Suto, H., Yoshida, Y., Crisp, D., and O'Dell, C.: Computation and analysis of atmospheric carbon dioxide annual mean growth rates from satellite observations during 2003–2016, Atmos. Chem. Phys., 18, 17355–17370, https://doi.org/10.5194/acp-18-17355-2018, 2018.
Buehner, M., McTaggart-Cowan, R., Beaulne, A., Charette, C., Garand, L., Heilliette, S., Lapalme, E., Laroche, S., Macpherson, S. R., Morneau, J., and Zadra, A.: Implementation of Deterministic Weather Forecasting Systems based on Ensemble-Variational Data Assimilation at Environment Canada. Part I: The Global System, Mon. Weather Rev., 143, 2532–2559, https://doi.org/10.1175/MWR-D-14-00354.1, 2015.
Bush, E. and Lemmen, D. S. (Eds.): Canada's Changing Climate Report, Government of Canada, Ottawa, Canada, 444 pp., 2019.
Bush, E., Gillett, N., Watson, E., Fyfe, J., Vogel, F., and Swart, N.: Understanding Observed Global Climate Change, Chapter 2 in: Canada's Changing Climate Report, edited by: Bush, E. and Lemmen, D. S., Government of Canada, Ottawa, Canada, 24–72, 2019.
Canadell, J. G., Le Quéré, C., Raupach, M. R., Field, C. B., Buitenhuis, E. T., Ciais, P., Conway, T. J., Gillett, N. P., Houghton, R. A., and Marland, G.: Contributions to accelerating atmospheric CO2 growth from economic activity, carbon intensity, and efficiency of natural sinks, P. Natl. Acad. Sci. USA, 104, 18866–18870, https://doi.org/10.1073/pnas.0702737104, 2007.
Caron, J.-F., Milewski, T., Buehner, M., Fillion, L., Reszka, M., Macpherson, S., and St-James, J.: Implementation of deterministic weather forecasting systems based on ensemble–variational data assimilation at Environment Canada. Part II: The regional system, Mon. Weather Rev., 143, 2560–2580, https://doi.org/10.1175/MWR-D-14-00353.1, 2015.
Chan, D., Yuen, C. W., Higuchi, K., Shashkov, A., Liu, J., Chen, J., and Worthy, D.: On the CO2 exchange between the atmosphere and the biosphere: the role of synoptic and mesoscale processes, Tellus B, 56, 194–212, https://doi.org/10.3402/tellusb.v56i3.16424, 2004.
Chevallier, F., Feng, L., Bösch, H., Palmer, P. I., and Rayner, P. J.: On the impact of transport model errors for the estimation of CO2 surface fluxes from GOSAT observations, Geophys. Res. Lett., 37, L21803, https://doi.org/10.1029/2010GL044652, 2010.
Chevallier, F., Palmer, P. I., Feng, L., Boesch, H., O'Dell, C. W., and Bousquet, P.: Toward robust and consistent regional CO2 flux estimates from in situ and spaceborne measurements of atmospheric CO2, Geophys. Res. Lett., 41, 1065–1070, https://doi.org/10.1002/2013GL058772, 2014.
Ciais, P., Rayner, P., Chevallier, F., Bousquet, P., Logan, M., Peylin, P., and Ramonet, M.: Atmospheric inversions for estimating CO2 fluxes: methods and perspectives, Climatic Change, 103, 69–92, https://doi.org/10.1007/s10584-010-9909-3, 2010.
Côté, J., Gravel, S., Méthot, A., Patoine, A., Roch, M., and Staniforth, A.: The operational CMC–MRB Global Environmental Multiscale (GEM) model. Part I: Design considerations and formulation, Mon. Weather Rev., 126, 1373–1395, https://doi.org/10.1175/1520-0493(1998)126<1373:TOCMGE>2.0.CO;2, 1998a.
Côté, J., Desmarais, J.-G., Gravel, S., Méthot, A., Patoine, A., Roch, M., and Staniforth, A.: The Operational CMC/MRB Global Environment Multiscale (GEM) Model. Part II: Results, Mon. Weather Rev., 126, 1397–1418, 1998b.
Crowell, S., Baker, D., Schuh, A., Basu, S., Jacobson, A. R., Chevallier, Crowell, S., Baker, D., Schuh, A., Basu, S., Jacobson, A. R., Chevallier, F., Liu, J., Deng, F., Feng, L., McKain, K., Chatterjee, A., Miller, J. B., Stephens, B. B., Eldering, A., Crisp, D., Schimel, D., Nassar, R., O'Dell, C. W., Oda, T., Sweeney, C., Palmer, P. I., and Jones, D. B. A.: The 2015–2016 carbon cycle as seen from OCO-2 and the global in situ network, Atmos. Chem. Phys., 19, 9797–9831, https://doi.org/10.5194/acp-19-9797-2019, 2019.
Davis, K. J., Bakwin, P. S., Yi, C., Berger, B. W., Zhao, C., Teclaw, R. M., and Isebrands, J. G.: The annual cycles of CO2 and H2O exchange over a northern mixed forest as observed from a very tall tower, Glob. Change Biol., 9, 1278–1293, https://doi.org/10.1046/j.1365-2486.2003.00672.x, 2003.
Díaz-Isaac, L. I., Lauvaux, T., Davis, K. J., Miles, N. L., Richardson, S. J., Jacobson, A. R., and Andrews, A. E.: Model-data comparison of MCI field campaign atmospheric CO2 mole fractions, J. Geophys. Res.-Atmos. 119, 10536–10551, https://doi.org/10.1002/2014JD021593, 2014.
Díaz-Isaac, L. I., Lauvaux, T., and Davis, K. J.: Impact of physical parameterizations and initial conditions on simulated atmospheric transport and CO2 mole fractions in the US Midwest, Atmos. Chem. Phys., 18, 14813–14835, https://doi.org/10.5194/acp-18-14813-2018, 2018.
Engelen, R. J., Denning, A. S., Gurney, K. R., and TransCom3 modelers: On error estimation in atmospheric CO2 inversions, J. Geophys. Res., 107, 4635, https://doi.org/10.1029/2002JD002195, 2002.
Enting, I. G.: Inverse Problems in Atmospheric Constituent Transport, Cambridge University Press, Edward Tipping, Centre for Ecology & Hydrology, Windermere Series, Cambridge Atmospheric and Space Science Series, ISBN 9780521018081, 2002.
Feng, S., Lauvaux, T., Newman, S., Rao, P., Ahmadov, R., Deng, A., Díaz-Isaac, L. I., Duren, R. M., Fischer, M. L., Gerbig, C., Gurney, K. R., Huang, J., Jeong, S., Li, Z., Miller, C. E., O'Keeffe, D., Patarasuk, R., Sander, S. P., Song, Y., Wong, K. W., and Yung, Y. L.: Los Angeles megacity: a high-resolution land–atmosphere modelling system for urban CO2 emissions, Atmos. Chem. Phys., 16, 9019–9045, https://doi.org/10.5194/acp-16-9019-2016, 2016.
Fernández-Martínez, M., Sardans, J., Chevallier, F., Ciais, P., Obersteiner, M., Vicca, S., Canadell, J. G., Bastos, A., Friedlingstein, P., Sitch, S., Piao, S. L., Janssens, I. A., and Peñuelas, J.: Global trends in carbon sinks and their relationships with CO2 and temperature, Nat. Clim. Change, 9, 73–79, https://doi.org/10.1038/s41558-018-0367-7, 2018.
Fillion, L., Tanguay, M., Lapalme, E., Denis, B., Desgagné, M., Lee, V., Ek, N., Liu, Z., Lajoie, M., Caron, J.-F., and Pagé, C.: The Canadian regional data assimilation and forecasting system, Weather Forecast., 25, 1645–1669, 2010.
Gaubert, B., Stephens, B. B., Basu, S., Chevallier, F., Deng, F., Kort, E. A., Patra, P. K., Peters, W., Rödenbeck, C., Saeki, T., Schimel, D., Van der Laan-Luijkx, I., Wofsy, S., and Yin, Y.: Global atmospheric CO2 inverse models converging on neutral tropical land exchange, but disagreeing on fossil fuel and atmospheric growth rate, Biogeosciences, 16, 117–134, https://doi.org/10.5194/bg-16-117-2019, 2019.
Geels, C., Doney, S., Dargaville, R., Brandt, J., and Christensen, J.: Investigating the sources of synoptic variability in atmospheric CO2 measurements over the Northern Hemisphere continents: a regional model study, Tellus B, 56, 35–50, 2004.
Geels, C., Gloor, M., Ciais, P., Bousquet, P., Peylin, P., Vermeulen, A. T., Dargaville, R., Aalto, T., Brandt, J., Christensen, J. H., Frohn, L. M., Haszpra, L., Karstens, U., Rödenbeck, C., Ramonet, M., Carboni, G., and Santaguida, R.: Comparing atmospheric transport models for future regional inversions over Europe – Part 1: mapping the atmospheric CO2 signals, Atmos. Chem. Phys., 7, 3461–3479, https://doi.org/10.5194/acp-7-3461-2007, 2007.
Gerbig, C., Lin, J. C., Wofsy, S. C., Daube, B. C., Andrews, A. E., Stephens, B. B., Bakwin, P. S., and Grainger, C. A.: Toward constraining regional-scale fluxes of CO2 with atmospheric observations over a continent: 1. Observed spatial variability from airborne platforms, J. Geophys. Res., 108, 4756, https://doi.org/10.1029/2002JD003018, 2003.
Girard, C., Plante, A., Desgagné, M., McTaggart-Cowan, R., Côté, J., Charron, M., Gravel, S., Lee, V., Patoine, A., Qaddouri, A., Roch, M., Spacek, L., Tanguay, M., Vaillancourt, P., and Zadra, A.: Staggered Vertical Discretization of the Canadian Environmental Multiscale (GEM) model using a coordinate of the loghydrostatic-pressure type, Mon. Weather Rev., 142, 1183–1196, 2014.
Gloor, M., Fan, S.-M., Pacala, S., Sarmiento, J., and Ramonet, M.: A model-based evaluation of inversions of atmospheric transport, using annual mean mixing ratios, as a tool to monitor fluxes of nonreactive trace substances like CO2 on a continental scale, J. Geophys. Res., 104, 14245, https://doi.org/10.1029/1999JD900132, 1999.
Gurney, K. R., Law, R. M., Denning, A. S., Rayner, P. J., Baker, D., Bousquet, P., Bruhwiler, L., Chen, Y.-H., Ciais, P., Fan, S., Fung, I. Y., Gloor, M., Heimann, M., Higuchi, K., John, J., Maki, T., Maksyutov, S., Masari, K., Peylin, P., Prather, M., Pak, B. C., Randerson, J., Sarmiento, J., Taguchi, S., Takahashi, T., and Yuen, C.-W.: Towards robust regional estimates of CO2 sources and sinks using atmospheric transport models, Nature, 415, 626–630, 2002.
Hines, C. O.: Doppler-spread parameterization of gravity wave momentum deposition in the middle atmosphere. Part 1: Basic formulation, J. Atmos. Sol.-Terr. Phys., 59, 371–386, 1997a.
Hines, C. O.: Doppler-spread parameterization of gravity-wave momentum deposition in the middle atmosphere. Part 2: Broad and quasi monochromatic spectra, and implementation, J. Atmos. Sol.-Terr. Phys., 59, 387–400, 1997b.
Houtekamer, P. L., Deng, X., Mitchell, H. L., Baek, S.-J., and Gagnon, N.: Higher Resolution in an Operational Ensemble Kalman Filter, Mon. Weather Rev., 142, 1143–1162, https://doi.org/10.1175/MWR-D-13-00138.1, 2014.
Houweling, S., Aben, I., Breon, F.-M., Chevallier, F., Deutscher, N., Engelen, R., Gerbig, C., Griffith, D., Hungershoefer, K., Macatangay, R., Marshall, J., Notholt, J., Peters, W., and Serrar, S.: The importance of transport model uncertainties for the estimation of CO2 sources and sinks using satellite measurements, Atmos. Chem. Phys., 10, 9981–9992, https://doi.org/10.5194/acp-10-9981-2010, 2010.
Ishizawa, M., Chan, D., Worthy, D., Chan, E., Vogel, F., and Maksyutov, S.: Analysis of atmospheric CH4 in Canadian Arctic and estimation of the regional CH4 fluxes, Atmos. Chem. Phys., 19, 4637–4658, https://doi.org/10.5194/acp-19-4637-2019, 2019.
Kain, J. S.: The Kain-Fritsch convective parameterization: an update, J. Appl. Meteorol., 43, 170–181, 2004.
Kain, J. S. and Fritsch, J. M.: A one-dimensional entraining/detraining plume model and its application in convective parameterizations, J. Atmos. Sci., 47, 2784–2802, 1990.
Kim, J. and Neish, M.: Model output data of The Canadian atmospheric transport model for simulating greenhouse gas evolution on regional scales: GEM-MACH-GHG v.137-reg, available at: http://crd-data-donnees-rdc.ec.gc.ca/CCMR/pub/2019_Kim_GMD_Canadian_atmospheric_transport_model_for_simulating_greenhouse_gas_evolution_on_regional_scales/, last access: 14 January 2020.
Kjellström, E., Holmén, K., Eneroth, K., and Engardt, M.: Summertime Siberian CO2 simulations with the regional transport model MATCH: a feasibility study of carbon uptake calculations from EUROSIB data, Tellus, 54B, 834–849, https://doi.org/10.3402/tellusb.v54i5.16733, 2002.
Kretschmer, R., Gerbig, C., Karstens, U., Biavati, G., Vermeulen, A., Vogel, F., Hammer, S., and Totsche, K. U.: Impact of optimized mixing heights on simulated regional atmospheric transport of CO2, Atmos. Chem. Phys., 14, 7149–7172, https://doi.org/10.5194/acp-14-7149-2014, 2014.
Krol, M., Houweling, S., Bregman, B., van den Broek, M., Segers, A., van Velthoven, P., Peters, W., Dentener, F., and Bergamaschi, P.: The two-way nested global chemistry-transport zoom model TM5: algorithm and applications, Atmos. Chem. Phys., 5, 417–432, https://doi.org/10.5194/acp-5-417-2005, 2005.
Kurz, W. A., Shaw, C. H., Boisvenue, C., Stinson, G., Metsaranta, J., Leckie, D., Dyk, A., and Smyth, C.: Carbon in Canada's boreal forecast – A synthesis, Environ. Rev., 21, 260–292, https://doi.org/10.1139/er-2013-0041, 2013.
Lauvaux, T. and Davis, K. J.: Planetary boundary layer errors in mesoscale inversions of column-integrated CO2 measurements, J. Geophys. Res.-Atmos., 119, 490–508, https://doi.org/10.1002/2013jd020175, 2014.
Lauvaux, T., Uliasz, M., Sarrat, C., Chevallier, F., Bousquet, P., Lac, C., Davis, K. J., Ciais, P., Denning, A. S., and Rayner, P. J.: Mesoscale inversion: first results from the CERES campaign with synthetic data, Atmos. Chem. Phys., 8, 3459–3471, https://doi.org/10.5194/acp-8-3459-2008, 2008.
Lauvaux, T., Schuh, A. E., Bocquet, M., Wu, L., Richardson, S., Miles, N., and Davis, K. J.: Network design for mesoscale inversions of CO2 sources and sinks, Tellus B, 64, 17980, https://doi.org/10.3402/tellusb.v64i0.17980, 2012a.
Lauvaux, T., Schuh, A. E., Uliasz, M., Richardson, S., Miles, N., Andrews, A. E., Sweeney, C., Diaz, L. I., Martins, D., Shepson, P. B., and Davis, K. J.: Constraining the CO2 budget of the corn belt: exploring uncertainties from the assumptions in a mesoscale inverse system, Atmos. Chem. Phys., 12, 337–354, https://doi.org/10.5194/acp-12-337-2012, 2012b.
Law, R. M., Rayner, P. J., Denning, A. S., Erickson, D., Fung, I. Y., Heimann, M., Piper, S. C., Romonet, M., Taguchi, S., Taylor, J. A., Trudinger, C. M., and Watterson, I. G.: Variations in modeled atmospheric transport of carbon dioxide and the consequences for CO2 inversions, Global Biogeochem. Cy., 10, 783–796, 1996.
Law, R. M., Peters, W., Rödenbeck, C., Aulagnier, C., Baker, I., Bergmann, D. J., Bousquet, P., Brandt, J., Bruhwiler, L., Cameron-Smith, P. J., Christensen, J. H., Delage, F., Denning, A. S., Fan, S., Geels, C., Houweling, S., Imasu, R., Karstens, U., Kawa, S. R., Kleist, J., Krol, M. C., Lin, S.-J., Lokupitiya, R., Maki, T., Maksyutov, S., Niwa, Y., Onishi, R., Parazoo, N., Patra, P. K., Pieterse, G., Rivier, L., Satoh, M., Serrar, S., Taguchi, S., Takigawa, M., Vautard, R., Vermeulen, A. T., and Zhu, Z.: TransCom model simulations of hourly atmospheric CO2: Experimental overview and diurnal cycle results for 2002, Global Biogeochem. Cy., 22, GB3009, https://doi.org/10.1029/2007GB003050, 2008.
Le Quéré, C., Raupach, M. R., Canadell, J. G., Marland, G., Bopp, L., Ciais, P., Conway, T. J., Doney, S. C., Feely, R. A., Foster, P., Friedlingstein, P., Gurney, K., Houghton, R. A., House, J. I., Huntingford, C., Levy, P. E., Lomas, M. R., Majkut, J., Metzl, N., Ometto, J. P., Peters, G. P., Prentice, I. C., Randerson, J. T., Running, S. W., Sarmiento, J. L., Schuster, U., Sitch, S., Takahashi, T., Viovy, N., van der Werf, G. R., and Woodward, F. I.: Trends in the sources and sinks of carbon dioxide, Nat. Geosci., 2, 831–836, https://doi.org/10.1038/NGEO689, 2009.
Le Quéré, C., Andrew, R. M., Friedlingstein, P., Sitch, S., Hauck, J., Pongratz, J., Pickers, P. A., Korsbakken, J. I., Peters, G. P., Canadell, J. G., Arneth, A., Arora, V. K., Barbero, L., Bastos, A., Bopp, L., Chevallier, F., Chini, L. P., Ciais, P., Doney, S. C., Gkritzalis, T., Goll, D. S., Harris, I., Haverd, V., Hoffman, F. M., Hoppema, M., Houghton, R. A., Hurtt, G., Ilyina, T., Jain, A. K., Johannessen, T., Jones, C. D., Kato, E., Keeling, R. F., Goldewijk, K. K., Landschützer, P., Lefèvre, N., Lienert, S., Liu, Z., Lombardozzi, D., Metzl, N., Munro, D. R., Nabel, J. E. M. S., Nakaoka, S., Neill, C., Olsen, A., Ono, T., Patra, P., Peregon, A., Peters, W., Peylin, P., Pfeil, B., Pierrot, D., Poulter, B., Rehder, G., Resplandy, L., Robertson, E., Rocher, M., Rödenbeck, C., Schuster, U., Schwinger, J., Séférian, R., Skjelvan, I., Steinhoff, T., Sutton, A., Tans, P. P., Tian, H., Tilbrook, B., Tubiello, F. N., van der Laan-Luijkx, I. T., van der Werf, G. R., Viovy, N., Walker, A. P., Wiltshire, A. J., Wright, R., Zaehle, S., and Zheng, B.: Global Carbon Budget 2018, Earth Syst. Sci. Data, 10, 2141–2194, https://doi.org/10.5194/essd-10-2141-2018, 2018.
Li, J. and Barker, H. W.: A radiation algorithm with correlated k-distribution. Part I: local thermal equilibrium, J. Atmos. Sci., 62, 286–309, 2005.
Li, R., Zhang, M., Chen, L., Kou, X., and Skorokhod, A.: CMAQ simulation of atmospheric CO2 concentration in East Asia: Comparison with GOSAT observations and ground measurements, Atmos. Environ., 160, 176–185, 2017.
Lin, J. C., Gerbig, C., Wofsy, S. C., Daube, B. C., Matross, D. M., Chow, V. Y., Gottlieb, E., Andrews, A. E., Pathmathevan, M., and Munger, J. W.: What have we learned from intensive atmospheric sampling field programmes of CO2?, Tellus B, 58, 331–343, https://doi.org/10.1111/j.1600-0889.2006.00202.x, 2006.
Lin, X., Ciais, P., Bousquet, P., Ramonet, M., Yin, Y., Balkanski, Y., Cozic, A., Delmotte, M., Evangeliou, N., Indira, N. K., Locatelli, R., Peng, S., Piao, S., Saunois, M., Swathi, P. S., Wang, R., Yver-Kwok, C., Tiwari, Y. K., and Zhou, L.: Simulating CH4 and CO2 over South and East Asia using the zoomed chemistry transport model LMDz-INCA, Atmos. Chem. Phys., 18, 9475–9497, https://doi.org/10.5194/acp-18-9475-2018, 2018.
Liu, J., Fung, I., Kalnay, E., and Kang, J.-S.: CO2 transport uncertainties from the uncertainties in meteorological fields, Geophys. Res. Lett., 38, L12808, https://doi.org/10.1029/2011GL047213, 2011.
Liu, J., Bowman, K. W., Schimel, D. S., Parazoo, N. C., Jiang, Z., Lee, M., Bloom, A. A., Wunch, D., Frankenberg, C., Sun, Y., O'Dell, C. W., Gurney, K. R., Menemenlis, D., Gierach, M., Crisp, D., and Eldering, A.: Contrasting carbon cycle responses of the tropical continents to the 2015–2016 El Niño, Science, 358, eaam5690, https://doi.org/10.1126/science.aam5690, 2017.
Locatelli, R., Bousquet, P., Chevallier, F., Fortems-Cheney, A., Szopa, S., Saunois, M., Agusti-Panareda, A., Bergmann, D., Bian, H., Cameron-Smith, P., Chipperfield, M. P., Gloor, E., Houweling, S., Kawa, S. R., Krol, M., Patra, P. K., Prinn, R. G., Rigby, M., Saito, R., and Wilson, C.: Impact of transport model errors on the global and regional methane emissions estimated by inverse modelling, Atmos. Chem. Phys., 13, 9917–9937, https://doi.org/10.5194/acp-13-9917-2013, 2013.
Locatelli, R., Bousquet, P., Hourdin, F., Saunois, M., Cozic, A., Couvreux, F., Grandpeix, J.-Y., Lefebvre, M.-P., Rio, C., Bergamaschi, P., Chambers, S. D., Karstens, U., Kazan, V., van der Laan, S., Meijer, H. A. J., Moncrieff, J., Ramonet, M., Scheeren, H. A., Schlosser, C., Schmidt, M., Vermeulen, A., and Williams, A. G.: Atmospheric transport and chemistry of trace gases in LMDz5B: evaluation and implications for inverse modelling, Geosci. Model Dev., 8, 129–150, https://doi.org/10.5194/gmd-8-129-2015, 2015.
Maihot, J., Bélair, S., Benoit, R., Bilodeau, B., Delage, Y., Fillion, L., Garand, L., Girard, C., and Tremblay, A.: Scientific description of RPN physics library – Version 3.6, Atmospheric Environment Service Tech. Rep., 188 pp., available at: http://collaboration.cmc.ec.gc.ca/science/rpn/physics/physic98.pdf (last access: 13 February 2019), 1998.
Makar, P. A., Gong, W., Milbrandt, J., Hogrefe., C., Zhang, Y., Curci, G., Žabkar, R., Im, U., Balzarini, A., Baró, R., Bianconi, R., Cheung, P., Forkel, R., Gravel, S., Hirtl, M., Honzak, L., Hou, A., Jiménez-Guerrero, P., Langer, M., Moran, M. D., Pabla, B., Pérez, J. L., Pirovano, G., San José, R., Tuccella, P., Werhahn, J., Zhang, J., and Galmarini, S.: Feedbacks between air pollution and weather, Part 1: Effects on weather, Atmos. Environ., 115, 442–469, https://doi.org/10.1016/j.atmosenv.2014.12.003, 2015.
Masarie, K. A., Peters, W., Jacobson, A. R., and Tans, P. P.: ObsPack: a framework for the preparation, delivery, and attribution of atmospheric greenhouse gas measurements, Earth Syst. Sci. Data, 6, 375–384, https://doi.org/10.5194/essd-6-375-2014, 2014.
McFarlane, N. A.: The effect of orographically excited gravity wave drag on the circulation of the lower stratosphere and troposphere, J. Atmos. Sci., 44, 1775–1800, https://doi.org/10.1175/1520-0469(1987)044<1775:TEOOEG>2.0.CO;2, 1987.
Milbrandt, J. A., Bélair, S., Faucher, M., Vallée, M., Carrera, M. L., and Glazer, A.: The Pan-Canadian High Resolution (2.5 km) Deterministic Prediction System, Weather Forecast., 31, 1791–1816, https://doi.org/10.1175/WAF-D-16-0035.1, 2016.
Miller, S. M., Hayek, M. N., Andrews, A. E., Fung, I., and Liu, J.: Biases in atmospheric CO2 estimates from correlated meteorology modeling errors, Atmos. Chem. Phys., 15, 2903–2914, https://doi.org/10.5194/acp-15-2903-2015, 2015.
Moran, M. D., Ménard, S., Talbot, D., Huang, P., Makar, P. A., Gong, W., Landry, H., Gravel, S., Gong, S., Crevier, L.-P., Kallaur, A., and Sassi, M.: Particulate-matter forecasting with GEM-MACH15, a new Canadian air-quality forecast model, in: Air Pollution Modelling and Its Application XX, edited by: Steyn, D. G. and Rao, S. T., Springer, Dordrecht, 289–292, 2010.
Neish, M., Tanguay, M., Semeniuk, K., Polavarapu, S. M., DeGrandpre, J., Girard, C., Qaddouri, A., Gravel, S., Chan, D., Ren, S., and GEM-MACH development team: GEM-MACH-GHG revision 137, Zenodo, https://doi.org/10.5281/zenodo.3246556, 2019.
Nisbet, E. and Weiss, R.: Top-down versus bottom-up, Science, 328, 1241–1243, https://doi.org/10.1126/science.1189936, 2010.
ObsPack: Cooperative Global Atmospheric Data Integration Project; Multi-laboratory compilation of atmospheric carbon dioxide data for the period 1957–2016; obspack_co2_1_GLOBALVIEWplus_v3.1_2017_10_18; NOAA Earth System Research Laboratory, Global Monitoring Division, https://doi.org/10.15138/G3T055, 2017.
Ott, L. E., Pawson, S., Collatz, G. J., Gregg, W. W., Menemenlis, D., Brix, H., Rousseaux, C. S., Bowman, K. W., Liu, J., Eldering, A., Gunson, M. R., and Kawa, S. R.: Assessing the magnitude of CO2 flux uncertainty in atmospheric CO2 records using products from NASA's Carbon Monitoring Flux Pilot Project, J. Geophys. Res.-Atmos., 120, 734–765, https://doi.org/10.1002/2014JD022411, 2015.
Parazoo, N. C., Denning, A. S., Kawa, S. R., Corbin, K. D., Lokupitiya, R. S., and Baker, I. T.: Mechanisms for synoptic variations of atmospheric CO2 in North America, South America and Europe, Atmos. Chem. Phys., 8, 7239–7254, https://doi.org/10.5194/acp-8-7239-2008, 2008.
Parazoo, N. C., Denning, A. S., Berry, J. A., Wolf, A., Randall, D. A., Kawa, S. R., Pauluis, O., and Doney, S. C.: Moist synoptic transport of CO2 along the mid-latitude storm track, Geophys. Res. Lett., 38, L09804, https://doi.org/10.1029/2011GL047238, 2011.
Parazoo, N. C., Denning, A. S., Kawa, S. R., Pawson, S., and Lokupitiya, R.: CO2 flux estimation errors associated with moist atmospheric processes, Atmos. Chem. Phys., 12, 6405–6416, https://doi.org/10.5194/acp-12-6405-2012, 2012.
Patra, P. K., Law, R. M., Peters, W., Rödenbeck, C., Takigawa, M., Aulagnier, C., Baker, I., Bergmann, D. J., Bousquet, P., Brandt, J., Bruhwiler, L., Cameron-Smith, P. J., Christensen, J. H., Delage, F., Denning, A. S., Fan, S., Geels, C., Houweling, S., Imasu, R., Karstens, U., Kawa, S. R., Kleist, J., Krol, M. C., Lin, S.-J., Lokupitiya, R., Maki, T., Maksyutov, S., Niwa, Y., Onishi, R., Parazoo, N., Pieterse, G., Rivier, L., Satoh, M., Serrar, S., Taguchi, S., Vautard, R., Vermeulen, A. T., and Zhu, Z.: TransCom model simulations of hourly atmospheric CO2: Analysis of synoptic-scale variations for the period 2002–2003, Global Biogeochem. Cy., 22, GB4013, https://doi.org/10.1029/2007GB003081, 2008.
Peters, W., Jacobson, A. R., Sweeney, C., Andrews, A. E., Conway, T. J., Masarie, K., Miller, J. B., Bruhwiler, L. M. P., Pétron, G., Hirsch, A. I.,Worthy, D. E. J., van der Werf, G. R., Randerson, J. T., Wennberg, P. O., Krol, M. C., and Tans, P. P.: An atmospheric perspective on North American carbon dioxide exchange: CarbonTracker, P. Natl. Acad. Sci. USA, 104, 18925–18930, 2007.
Peylin, P., Houweling, S., Krol, M. C., Karstens, U., Rödenbeck, C., Geels, C., Vermeulen, A., Badawy, B., Aulagnier, C., Pregger, T., Delage, F., Pieterse, G., Ciais, P., and Heimann, M.: Importance of fossil fuel emission uncertainties over Europe for CO2 modeling: model intercomparison, Atmos. Chem. Phys., 11, 6607-6622, https://doi.org/10.5194/acp-11-6607-2011, 2011.
Peylin, P., Law, R. M., Gurney, K. R., Chevallier, F., Jacobson, A. R., Maki, T., Niwa, Y., Patra, P. K., Peters, W., Rayner, P. J., Rödenbeck, C., van der Laan-Luijkx, I. T., and Zhang, X.: Global atmospheric carbon budget: results from an ensemble of atmospheric CO2 inversions, Biogeosciences, 10, 6699–6720, https://doi.org/10.5194/bg-10-6699-2013, 2013.
Pillai, D., Gerbig, C., Marshall, J., Ahmadov, R., Kretschmer, R., Koch, T., and Karstens, U.: High resolution modeling of CO2 over Europe: implications for representation errors of satellite retrievals, Atmos. Chem. Phys., 10, 83–94, https://doi.org/10.5194/acp-10-83-2010, 2010.
Pillai, D., Gerbig, C., Ahmadov, R., Rödenbeck, C., Kretschmer, R., Koch, T., Thompson, R., Neininger, B., and Lavrié, J. V.: High-resolution simulations of atmospheric CO2 over complex terrain – representing the Ochsenkopf mountain tall tower, Atmos. Chem. Phys., 11, 7445–7464, https://doi.org/10.5194/acp-11-7445-2011, 2011.
Polavarapu, S. M., Neish, M., Tanguay, M., Girard, C., de Grandpré, J., Semeniuk, K., Gravel, S., Ren, S., Roche, S., Chan, D., and Strong, K.: Greenhouse gas simulations with a coupled meteorological and transport model: the predictability of CO2, Atmos. Chem. Phys., 16, 12005–12038, https://doi.org/10.5194/acp-16-12005-2016, 2016.
Polavarapu, S. M., Deng, F., Byrne, B., Jones, D. B. A., and Neish, M.: A comparison of posterior atmospheric CO2 adjustments obtained from in situ and GOSAT constrained flux inversions, Atmos. Chem. Phys., 18, 12011–12044, https://doi.org/10.5194/acp-18-12011-2018, 2018.
Pugliese, S. C., Murphy, J. G., Vogel, F. R., Moran, M. D., Zhang, J., Zheng, Q., Stroud, C. A., Ren, S., Worthy, D., and Broquet, G.: High-resolution quantification of atmospheric CO2 mixing ratios in the Greater Toronto Area, Canada, Atmos. Chem. Phys., 18, 3387–3401, https://doi.org/10.5194/acp-18-3387-2018, 2018.
Qaddouri, A. and Lee, V.: The Canadian Global Environmental Multiscale model on the Yin-Yang grid system, Q. J. Roy. Meteor. Soc., 137, 1913–1926, https://doi.org/10.1002/qj.873, 2011.
Remaud, M., Chevallier, F., Cozic, A., Lin, X., and Bousquet, P.: On the impact of recent developments of the LMDz atmospheric general circulation model on the simulation of CO2 transport, Geosci. Model Dev., 11, 4489–4513, https://doi.org/10.5194/gmd-11-4489-2018, 2018.
Robichaud, A. and Ménard, R.: Multi-year objective analyses of warm season ground-level ozone and PM2.5 over North America using real-time observations and Canadian operational air quality models, Atmos. Chem. Phys., 14, 1769–1800, https://doi.org/10.5194/acp-14-1769-2014, 2014.
Schuh, A. E., Denning, A. S., Corbin, K. D., Baker, I. T., Uliasz, M., Parazoo, N., Andrews, A. E., and Worthy, D. E. J.: A regional high-resolution carbon flux inversion of North America for 2004, Biogeosciences, 7, 1625–1644, https://doi.org/10.5194/bg-7-1625-2010, 2010.
Schuh, A. E., Lauvaux, T., West, T. O., Denning, A. S., Davis, K. J., Miles, N., Richardson, S., Uliasz, M., Lokupitiya, E., Cooley, D., Andrews, A., and Ogle, S.: Evaluating atmospheric CO2 inversions at multiple scales over a highly-inventoried agricultural landscape, Glob. Change Biol., 19, 1424–1439, https://doi.org/10.1111/gcb.12141, 2013.
Schuh, A. E., Jacobson, A. R., Basu, S., Weir, B., Baker, D., Bowman, K., Chevallier, F., Crowell, S., Davis, K. J., Deng, F., Denning, S., Feng, L., Jones, D., Liu, J., and Palmer, P. I.: Quantifying the Impact of Atmospheric Transport Uncertainty on CO2 Surface Flux Estimates, Global Biogeochem. Cy., 33, 2018GB006086, https://doi.org/10.1029/2018GB006086, 2019.
Sørensen, B., Kaas, E., and Korsholm, U. S.: A mass-conserving and multi-tracer efficient transport scheme in the online integrated Enviro-HIRLAM model, Geosci. Model Dev., 6, 1029–1042, https://doi.org/10.5194/gmd-6-1029-2013, 2013.
Stephens, B. B., Gurney, K. R., Tans, P. P., Sweeney, C., Peters, W., Bruhwiler, L., Ciais, P., Ramonet, M., Bousquet, P., Nakazawa, T., Aoki, S., Machida, T., Inoue, G., Vinnichenko, N., Lloyd, J., Jordan, A., Heimann, M., Shibistova, O., Langenfelds, R. L., Steele, L. P., Francey, R. J., and Denning, A. S.: Weak northern and strong tropical land carbon uptake from vertical profiles of atmospheric CO2, Science, 316, 1732–1735, https://doi.org/10.1126/science.1137004, 2007.
Sweeney, C., Karion, A., Wolter, S., Newberger, T., Guenther, D., Higgs, J. A., Andrews, A. E., Lang, P. M., Neff, D., Dlugokencky, E., Miller, J. B., Montzka, S. A., Miller, B. R., Masarie, K. A., Biraud, S. C., Novelli, P. C., Crotwell, M., Crotwell, A. M., Thoning, K., and Tans, P. P.: Seasonal climatology of CO2 across North America from aircraft measurements in the NOAA/ESRL Global Greenhouse Gas Reference Network, J. Geophys. Res.-Atmos., 120, 5155–5190, https://doi.org/10.1002/2014JD022591, 2015.
Tans, P. P., Fung, I. Y., and Takahashi, T.: Observational Contrains on the Global Atmospheric CO2 Budget, Science, 247, 1431–1438, https://doi.org/10.1126/science.247.4949.1431, 1990.
Taylor, K. E.: Summarizing multiple aspects of model performance in a single diagram, J. Geophys. Res.-Atmos., 106, 7183–7192, 2001.
Wang, J. W., Denning, A. S., Lu, L., Baker, I. T., Corbin, K. D., and Davis, K. J.: Observations and simulations of synoptic, regional, and local variations in atmospheric CO2, J. Geophys. Res., 112, D04108, https://doi.org/10.1029/2006JD007410, 2007.
Wilks, D. S.: Statistical methods in the atmospheric sciences, Academic Press, 2006.
Worthy, D. E., Platt, J. A., Kessler, R., Ernst, M., Audette, C., and Racki, S.: An update on the Canadian GHG measurement program, in: Report of the 12th WMO/IAEA Meeting of Experts on Carbon Dioxide Concentration and Related Tracer Measurement Techniques, Toronto, Canada, September 2003, edited by: Worthy, D. and Huang, L., World Meteorological Organization Global Atmosphere Watch, Report 162, 220–231, 2005.
Wu, D., Lin, J. C., Fasoli, B., Oda, T., Ye, X., Lauvaux, T., Yang, E. G., and Kort, E. A.: A Lagrangian approach towards extracting signals of urban CO2 emissions from satellite observations of atmospheric column CO2 (XCO2): X-Stochastic Time-Inverted Lagrangian Transport model (“X-STILT v1”), Geosci. Model Dev., 11, 4843–4871, https://doi.org/10.5194/gmd-11-4843-2018, 2018.
Zhang, X., Gurney, K. R., Rayner, P., Liu, Y., and Asefi-Najafabady, S.: Sensitivity of simulated CO2 concentration to regridding of global fossil fuel CO2 emissions, Geosci. Model Dev., 7, 2867–2874, https://doi.org/10.5194/gmd-7-2867-2014, 2014.