the Creative Commons Attribution 4.0 License.
the Creative Commons Attribution 4.0 License.
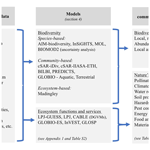
A protocol for an intercomparison of biodiversity and ecosystem services models using harmonized land-use and climate scenarios
HyeJin Kim
Isabel M. D. Rosa
Rob Alkemade
Paul Leadley
George Hurtt
Alexander Popp
Detlef P. van Vuuren
Peter Anthoni
Almut Arneth
Daniele Baisero
Emma Caton
Rebecca Chaplin-Kramer
Louise Chini
Adriana De Palma
Fulvio Di Fulvio
Moreno Di Marco
Felipe Espinoza
Simon Ferrier
Shinichiro Fujimori
Ricardo E. Gonzalez
Maya Gueguen
Carlos Guerra
Mike Harfoot
Thomas D. Harwood
Tomoko Hasegawa
Vanessa Haverd
Petr Havlík
Stefanie Hellweg
Samantha L. L. Hill
Akiko Hirata
Andrew J. Hoskins
Jan H. Janse
Walter Jetz
Justin A. Johnson
Andreas Krause
David Leclère
Ines S. Martins
Tetsuya Matsui
Cory Merow
Michael Obersteiner
Haruka Ohashi
Benjamin Poulter
Andy Purvis
Benjamin Quesada
Carlo Rondinini
Aafke M. Schipper
Richard Sharp
Kiyoshi Takahashi
Wilfried Thuiller
Nicolas Titeux
Piero Visconti
Christopher Ware
Florian Wolf
To support the assessments of the Intergovernmental Science-Policy Platform on Biodiversity and Ecosystem Services (IPBES), the IPBES Expert Group on Scenarios and Models is carrying out an intercomparison of biodiversity and ecosystem services models using harmonized scenarios (BES-SIM). The goals of BES-SIM are (1) to project the global impacts of land-use and climate change on biodiversity and ecosystem services (i.e., nature's contributions to people) over the coming decades, compared to the 20th century, using a set of common metrics at multiple scales, and (2) to identify model uncertainties and research gaps through the comparisons of projected biodiversity and ecosystem services across models. BES-SIM uses three scenarios combining specific Shared Socio-economic Pathways (SSPs) and Representative Concentration Pathways (RCPs) – SSP1xRCP2.6, SSP3xRCP6.0, SSP5xRCP8.6 – to explore a wide range of land-use change and climate change futures. This paper describes the rationale for scenario selection, the process of harmonizing input data for land use, based on the second phase of the Land Use Harmonization Project (LUH2), and climate, the biodiversity and ecosystem services models used, the core simulations carried out, the harmonization of the model output metrics, and the treatment of uncertainty. The results of this collaborative modeling project will support the ongoing global assessment of IPBES, strengthen ties between IPBES and the Intergovernmental Panel on Climate Change (IPCC) scenarios and modeling processes, advise the Convention on Biological Diversity (CBD) on its development of a post-2020 strategic plans and conservation goals, and inform the development of a new generation of nature-centred scenarios.
- Article
(904 KB) - Full-text XML
-
Supplement
(361 KB) - BibTeX
- EndNote
Understanding how anthropogenic activities impact biodiversity and human societies is essential for nature conservation and sustainable development. Land-use and climate change are widely recognized as two of the main drivers of future biodiversity change (Hirsch and CBD, 2010; Maxwell et al., 2016; Sala, 2000; Secretariat of the CBD and UNEP, 2014), with potentially severe impacts on ecosystem services and ultimately human well-being (Cardinale et al., 2012; MEA, 2005). Habitat and land-use changes, resulting from past, present, and future human activities, as well as climate change, have both immediate and long-term impacts on biodiversity and ecosystem services (Graham et al., 2017; Lehsten et al., 2015; Welbergen et al., 2008). Therefore, current and future land-use projections are essential elements for assessing biodiversity and ecosystem change (Titeux et al., 2016, 2017). Climate change has already been observed to have direct and indirect impacts on biodiversity and ecosystems, which are projected to intensify by the end of the century, with potentially severe consequences for species and habitats, and, therefore, also for ecosystem functions and services (Pecl et al., 2017; Settele et al., 2015).
Global environmental assessments, such as the Millennium Ecosystem Assessment (MEA, 2005), the Global Biodiversity Outlooks (GBO), the multiple iterations of the Global Environmental Outlook (GEO), the Intergovernmental Panel on Climate Change (IPCC), and other studies have used scenarios to assess the impact of socio-economic development pathways on land use and climate and their consequences for biodiversity and ecosystem services (Jantz et al., 2015; Pereira et al., 2010). Models are used to quantify the biodiversity and ecosystem services impacts of different scenarios, based on climate and land-use projections from general circulation models (GCMs) and integrated assessment models (IAMs) (Pereira et al., 2010). These models include empirical dose–response models, species–area relationship models, species distribution models and more mechanistic models such as trophic ecosystem models (Pereira et al., 2010; Akçakaya et al., 2015). So far, each of these scenario exercises has been based on a single model or a small number of biodiversity and ecosystem services models, and intermodel comparison and uncertainty analysis have been limited (IPBES, 2016; Leadley et al., 2014). The Expert Group on Scenarios and Models of the Intergovernmental Science-Policy Platform on Biodiversity and Ecosystem Services (IPBES) is addressing this gap by carrying out a biodiversity and ecosystem services model intercomparison with harmonized scenarios, for which this paper lays out the protocol.
Over the past 2 decades, IPCC has fostered the development of global scenarios to inform climate mitigation and adaptation policies. The Representative Concentration Pathways (RCPs) describe different climate futures based on greenhouse gas emissions throughout the 21st century (van Vuuren et al., 2011). These emissions pathways have been converted into climate projections in the most recent Climate Model Inter-comparison Project (CMIP5). In parallel, the climate research community also developed the Shared Socio-economic Pathways (SSPs), which consist of trajectories of future human development with different socio-economic conditions and associated land-use projections (Popp et al., 2017; Riahi et al., 2017). The SSPs can be combined with RCP-based climate projections to explore a range of futures for climate change and land-use change, and they are being used in a wide range of impact modeling intercomparisons (Rosenzweig et al., 2017; van Vuuren et al., 2014). Therefore, the use of the SSP-RCP framework for modeling the impacts on biodiversity and ecosystem services provides an outstanding opportunity to build bridges between the climate, biodiversity and ecosystem services communities; it has been explicitly recommended as a research priority in the IPBES assessment on scenarios and models (IPBES, 2016).
Model intercomparisons bring together different communities of practice for comparable and complementary modeling, in order to improve the comprehensiveness of the subject modeled, and to estimate uncertainties associated with scenarios and models (Frieler et al., 2015). In the last decades, various model intercomparison projects (MIPs) have been initiated to assess the magnitude and uncertainty of climate change impacts. For instance, the Inter-Sectoral Impact Model Intercomparison Project (ISI-MIP) was initiated in 2012 to quantify and synthesize climate change impacts across sectors and scales (Rosenzweig et al., 2017; Warszawski et al., 2014). The ISI-MIP aims to bridge sectors such as agriculture, forestry, fisheries, water, energy, and health with global circulation models, Earth system models (ESMs), and integrated assessment models for more integrated and impact-driven modeling and assessment (Frieler et al., 2017).
Here, we present the methodology used to carry out a BES-SIM in both terrestrial and freshwater ecosystems. The BES-SIM project addresses the following questions. (1) What are the projected magnitudes and spatial distribution of biodiversity and ecosystem services under a range of land-use and climate future scenarios? (2) What is the magnitude of the uncertainties associated with the projections obtained from different scenarios and models? Although independent of the ISI-MIP, the BES-SIM has been inspired by ISI-MIP and other intercomparison projects and was initiated to address the needs of the global assessment of IPBES. We brought together 10 biodiversity models and six ecosystem functions and services models to assess impacts of land-use and climate change scenarios in the coming decades (up to 2070) and to hindcast changes to the last century (to 1900). The modeling approaches differ in several respects concerning how they treat biodiversity and ecosystem services responses to land-use and climate changes, including the use of correlative, deductive, and process-based approaches, and in how they treat spatial-scale and temporal dynamics. We assessed different classes of essential biodiversity variables (EBVs), including species populations, community composition, and ecosystem function, as well as a range of measures on ecosystem services such as food production, pollination, water quantity and quality, climate regulation, soil protection, and pest control (Pereira et al., 2010; Akçakaya et al., 2015). This paper provides an overview of the scenarios, models and metrics used in this intercomparison, and thus a roadmap for further analyses that is envisaged to be integrated into the first global assessment of the IPBES (Fig. 1).
All the models included in BES-SIM used the same set of scenarios with particular combinations of SSPs and RCPs. In the selection of the scenarios, we applied the following criteria: (1) data on projections should be readily available, and (2) the total set should cover a broad range of land-use change and climate change projections. The first criterion entailed the selection of SSP-RCP combinations that are included in the ScenarioMIP protocol as part of CMIP6 (O'Neill et al., 2016), as harmonized data were available for these runs and they form the basis of the CMIP climate simulations. The second criterion implied a selection of scenarios with low and high degrees of climate change and different land-use scenarios within the ScenarioMIP set. Our final selection was SSP1 with RCP2.6 (moderate land-use pressure and low level of climate change) (van Vuuren et al., 2017), SSP3 with RCP6.0 (high land-use pressure and moderately high level of climate change) (Fujimori et al., 2017), and SSP5 with RCP8.5 (medium land-use pressure and very high level of climate change) (Kriegler et al., 2017), thus allowing us to assess a broad range of plausible futures (Table 1). Further, by combining projections of low and high anthropogenic pressure on land use with low and high levels of climate change, we can test these drivers' individual and synergistic impacts on biodiversity and ecosystem services.
Table 1Characteristics of the (a) SSP, (b) RCP and (c) SSPxRCP scenarios simulated in BES-SIM (adapted from Moss et al., 2010; O'Neill et al., 2017; Popp et al., 2017; van Vuuren et al., 2011).
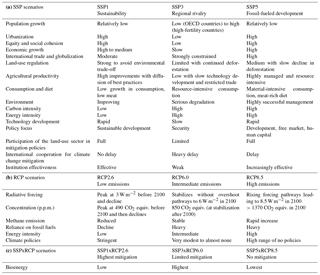
The first scenario (SSP1xRCP2.6) is characterized by a relatively “environmentally friendly world” with low population growth, high urbanization, relatively low demand for animal products, and high agricultural productivity. These factors together lead to a decrease in the land use of around 700 Mha globally over time (mostly pastures). This scenario is also characterized by low air pollution, as policies are introduced to limit the increase in greenhouse gases in the atmosphere, leading to an additional forcing of 2.6 W m−2 before 2100. The second scenario (SSP3xRCP6.0) is characterized by “regional rivalry”, with high population growth, slow economic development, material-intensive consumption, and low food demand per capita. Agricultural land intensification is low, especially due to the very limited transfer of new agricultural technologies to developing countries. This scenario has minimal land-use change regulation, with a large land conversion for human-dominated uses, and a relatively high level of climate change with a radiative forcing of 6.0 W m−2 by 2100. The third scenario (SSP5xRCP8.5) is a world characterized by “strong economic growth” fuelled by fossil fuels, with low population growth, high urbanization, and high food demand per capita but also high agricultural productivity. As a result, there is a modest increase in land use. Air pollution policies are stringent, motivated by local health concerns. This scenario leads to a very high level of climate change with a radiative forcing of 8.5 W m−2 by 2100. Full descriptions of each SSP scenario are provided in Popp et al. (2017) and Riahi et al. (2017). The SSP scenarios excluded elements that have interaction effects with climate change except for SSP1, which focuses on environmental sustainability. Thus, SSPs describe futures where biodiversity is not affected by climate change to allow for the important estimation of the climate change impact on biodiversity (O'Neill et al., 2014).
A consistent set of land-use and climate data was implemented across the models to the extent possible. All models in BES-SIM used the newly released Land Use Harmonization version 2 dataset (LUH2, Hurtt et al., 2018). For the models that require climate data, we selected the climate projections of the past, present, and future from CMIP5/ISIMIP2a (McSweeney and Jones, 2016) and its downscaled version from the WorldClim (Fick and Hijmans, 2017), as well as MAGICC 6.0 (Meinshausen et al., 2011a, b) from the IMAGE model for GLOBIO models (Table 2). A complete list of input datasets and variables used by the models is documented in Table S1 of the Supplement.
3.1 Land-cover and land-use change data
The land-use scenarios provide an assessment of land-use dynamics in response to a range of socio-economic drivers and their consequences for the land system. The IAMs used for modeling land-use scenarios – IMAGE for SSP1/RCP2.6, AIM for SSP3/RCP7.0, and REMIND/MAgPIE for SSP5/RCP8.5 – include different economic and land-use modules for the translation of narratives into consistent quantitative projections across scenarios (Popp et al., 2017). It is important to note that the used land-use scenarios, although driven mostly by the SSP storylines, were projected to be consistent with the paired RCPs and include biofuel deployment to mitigate climate change. The SSP3 is associated with RCP7.0 (SSP3xRCP7.0); however, climate projections (i.e., time series of precipitation and temperature) are currently not available for RCP7.0. Therefore, we chose the closest RCP available, which was RCP6.0, for the standalone use of climate projections, and chose SSP3xRCP6.0 for the land-use projections from the LUH2. In this paper, we refer to this scenario as SSP3xRCP6.0.
The land-use projections from each of the IAMs were harmonized using the LUH2 methodology. LUH2 was developed for CMIP6 and provides a global gridded land-use dataset comprising estimates of historical land-use change (850–2015) and future projections (2015–2100), obtained by integrating and harmonizing land-use history with future projections of different IAMs (Jungclaus et al., 2017; Lawrence et al., 2016; O'Neill et al., 2016). Compared to the first version of the LUH (Hurtt et al., 2011), LUH2 (Hurtt et al., 2018) is driven by the latest SSPs, has a higher spatial resolution (0.25 vs 0.50∘), more detailed land-use transitions (12 versus 5 possible land-use states), and increased data-driven constraints (Heinimann et al., 2017; Monfreda et al., 2008). LUH2 provides over 100 possible transitions per grid cell per year (e.g., crop rotations, shifting cultivation, agricultural changes, wood harvest) and various agricultural management layers (e.g., irrigation, synthetic nitrogen fertilizer, biofuel crops), all with annual time steps. The 12 land states include the separation of primary and secondary natural vegetation into forest and non-forest sub-types, pasture into managed pasture and rangeland, and cropland into multiple crop functional types (C3 annual, C3 perennial, C4 annual, C4 perennial, and N-fixing crops) (Table 2).
Table 3Sources of land-use and climate input data in BES-SIM.
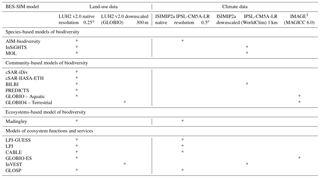
1 All GLOBIO models use MAGICC climate data from the IMAGE model.
For biodiversity and ecosystem services models that rely on discrete, high-resolution land-use data (i.e., the GLOBIO model for terrestrial biodiversity and the InVEST model), the fractional LUH2 data were downscaled to discrete land-use grids (10 arcsec resolution; ∼300 m) with the land-use allocation routine of the GLOBIO4 model. To that end, urban, cropland, pasture, rangeland, and forestry areas from LUH2 were first aggregated across the LUH2 grid cells to the regional level of the IMAGE model, with forestry consisting of the wood harvest from forested cells and non-forested cells with primary vegetation. Next, the totals per region were allocated to 300 m cells with the GLOBIO4 land allocation routine, with specific suitability layers for urban, cropland, pasture, rangeland, and forestry areas. After allocation, cropland was reclassified into three intensity classes (low, medium, high) based on the amount of fertilizer used per grid cell. More details on the downscaling procedure are provided in Supplementary Methods in the Supplement.
3.2 Climate data
GCMs are based on fundamental physical processes (e.g., conservation of energy, mass, and momentum and their interaction with the climate system) and simulate climate patterns of temperature, precipitation, and extreme events on a large scale (Frischknecht et al., 2016). Some GCMs now incorporate elements of Earth's climate system (e.g., atmospheric chemistry, soil and vegetation, land and sea ice, carbon cycle) in Earth system models (GCMs with an interactive carbon cycle), and have dynamically downscaled models with higher-resolution data in regional climate models (RCMs).
A large number of climate datasets are available today from multiple GCMs, but not all GCMs provide projections for all RCPs. In BES-SIM, some models require continuous time-series data. In order to harmonize the climate data to be used across biodiversity and ecosystem services models, we chose the bias-corrected climate projections from CMIP5, which were also adopted by ISIMIP2a (Hempel et al., 2013) or their downscaled versions available from WorldClim (Fick and Hijmans, 2017). Most analyses were carried out using a single GCM, the IPSL-CM5A-LR (Dufresne et al., 2013), since it provides mid-range projections across the five GCMs (HadGEM2-ESGFDL-ESM2M, IPSL-CM5A-LR, MIROC-ESM-CHEM, and NorESM1-M) in ISIMIP2a (Warszawski et al., 2014).
The ISIMIP2a output from the IPSL-CM5A-LR provides 12 climate variables on daily time steps from the pre-industrial period 1951 to 2099 at 0.5∘ resolution (McSweeney and Jones, 2016), of which only a subset was used in this exercise (Table S1). The WorldClim downscaled dataset has 19 bioclimatic variables derived from monthly temperature and rainfall from 1960 to 1990 with multi-year averages for specific points in time (e.g., 2050, 2070) up to 2070. Six models in BES-SIM used the ISIMIP2a dataset and three models used the WorldClim dataset. An exception was made for the GLOBIO models, which used MAGICC 6.0 climate data (Meinshausen et al., 2011a, b) in the IMAGE model framework (Stehfest et al., 2014), to which GLOBIO is tightly connected (Table 3). The variables used from the climate dataset in each model are listed in Table S1.
3.3 Other input data
In addition to the land-use and climate data, most models use additional input data to run their future and past simulations to estimate changes in biodiversity and ecosystem services. For instance, species occurrence data are an integral part of modeling in 6 of 10 biodiversity models, while 2 models rely on estimates of habitat affinity coefficients (e.g., reductions in species richness in a modified habitat relative to the pristine habitat) from the PREDICTS model (Newbold et al., 2016; Purvis et al., 2018). In three dynamic global vegetation models (DGVMs), atmospheric CO2 concentrations, irrigated fraction, and wood harvest estimates are commonly used, while two ecosystem services models rely on topography and soil-type data for soil erosion measures. A full list of model-specific input data is given in Table S1.
Biodiversity and ecosystem services models at the global scale have increased in number and improved considerably over the last decade, especially with the availability of biodiversity data and advancement in statistical modeling tools and methods (IPBES, 2016). In order for a model to be included in BES-SIM, it had either to be published in a peer-reviewed journal or adopt published methodologies, with modifications made to modeling sufficiently documented and accessible for review (Table S2). Sixteen models were included in BES-SIM (Appendix A, details on modeling methods in Table S2). These models were mainly grouped into four classes: species-based, community-based, and ecosystem-based models of biodiversity, and models of ecosystem functions and services. The methodological approaches, the taxonomic or functional groups, the spatial resolution and the output metrics differ across models (Appendix A). All 16 models are spatially explicit, with 15 of them using land-use data as an input and 13 of them requiring climate data. We also used one model, BIOMOD2 (Thuiller, 2004; Thuiller et al., 2009), to assess the uncertainty of climate range projections without the use of land-use data.
4.1 Species-based models of biodiversity
Species-based models aim to predict historical, current, and future potential distribution and abundance of individual species. These can be developed using correlative methods based on species observation and environmental data (Aguirre-Gutiérrez et al., 2013; Guisan and Thuiller, 2005; Guisan and Zimmermann, 2000) as well as expert-based solutions where data limitations exist (Rondinini et al., 2011). Depending on the methodologies employed and the ecological aspects modeled, they can be known as species distribution models, ecological niche models, bioclimatic envelope models, and habitat suitability models (Elith and Leathwick, 2009). Such species-based models have been used to forecast environmental impacts on species distribution and status.
In BES-SIM, four species-based models were included: AIM-biodiversity (Ohashi et al., 2018), InSiGHTS (Rondinini et al., 2011; Visconti et al., 2016), MOL (Jetz et al., 2007; Merow et al., 2013), and BIOMOD2 (Appendix A, Table S2). The first three models project individual species distributions across a large number of species by combining projections of climate impacts on species ranges with projections of land-use impacts on species ranges. AIM-biodiversity uses Global Biodiversity Information Facility (GBIF) species occurrence data on 9025 species across five taxonomic groups (amphibians, birds, mammals, plants, reptiles) to train statistical models for current land use and climate to project future species distributions. InSiGHTS uses species' presence records from regular sampling within species' ranges and pseudo-absence records from regular sampling outside of species' ranges on 2827 species of mammals. MOL uses species land-cover preference information and species presence and absence predictions on 20 833 species of amphibians, birds, and mammals. InSiGHTS and MOL rely on IUCN's range maps as a baseline, which are developed based on expert knowledge of the species habitat preferences and areas of non-occurrence (Fourcade, 2016). Both models use a hierarchical approach with two steps: first, a statistical model trained on current species ranges is used to assess future climate suitability within species ranges; second, a model detailing associations between species and habitat types based on expert opinion is used to assess the impacts of land use in the climate-suitable portion of the species range. BIOMOD2 is an R modeling package that runs up to nine different algorithms (e.g., random forests, logistic regression) of species distribution models using the same data and the same framework. BIOMOD2 included three taxonomic groups (amphibians, birds, mammals) (see Sect. 7 “Uncertainties”).
4.2 Community-based models of biodiversity
Community-based models predict the assemblage of species using environmental data and assess changes in community composition through species presence and abundance (D'Amen et al., 2017). Output variables of community-based models include assemblage-level metrics, such as the proportion of species persisting in a landscape, mean species abundances (number of individuals per species), and compositional similarity (pairwise comparison at the species level) relative to a baseline (typically corresponding to a pristine landscape).
Three models in BES-SIM – cSAR-iDiv (Martins and Pereira, 2017), cSAR-IIASA-ETH (Chaudhary et al., 2015), and BILBI (Hoskins et al., 2018; Ferrier et al., 2004, 2007) – rely on versions of the species–area relationship (SAR) to estimate the proportion of species persisting in human-modified habitats relative to native habitat (i.e., the number of species in the modified landscape divided by the number of species in the native habitat). In its classical form, the SAR describes the relationship between the area of native habitat and the number of species found within that area. The countryside SAR (cSAR) builds on the classic SAR but accounts for the differential use of both human-modified and native habitats by different functional species groups. Both the cSAR-iDiv and cSAR-IIASA-ETH models use habitat affinities (proportion of area of a habitat type that can be effectively used by a species group) to weight the areas of the different habitats in a landscape. The habitat affinities are calibrated from field studies by calculating the change in species richness in a modified habitat relative to the native habitat. The habitat affinities of the cSAR-iDiv model are estimated from the PREDICTS dataset (Hudson et al., 2017, 2016) while the habitat affinities of cSAR-IIASA-ETH come from a previously published database of studies (Chaudhary et al., 2015). The cSAR-iDiv model considers 9853 species for one taxonomic group (birds) in two functional groups (forest species and non-forest species) while cSAR-IIASA-ETH considers a total of 1 911 583 species for five taxonomic groups (amphibians, birds, mammals, plants, reptiles) by ecoregions (these are, however, not 1 911 583 unique species as a species present in two ecoregions will be counted twice). BILBI couples application of the species–area relationship with correlative statistical modeling of continuous spatial turnover patterns in the species composition of communities as a function of environmental variation. Through space-for-time projection of compositional turnover (i.e., change in species), this coupled model enables the effects of both climate change and habitat modification to be considered in estimating the proportion of species persisting for 254 145 vascular plant species globally.
Three community-based models – PREDICTS, GLOBIO Aquatic (Alkemade et al., 2009; Janse et al., 2015), and GLOBIO Terrestrial (Alkemade et al., 2009; Schipper et al., 2016) – estimate a range of assemblage-level metrics based on empirical dose–response relationships between pressure variables (e.g., land-use change and climate change) and biodiversity variables (e.g., species richness or mean species abundance) (Appendix A). PREDICTS uses a hierarchical mixed-effects model to assess how a range of site-level biodiversity metrics respond to land use and related pressures, using a global database of 767 studies, including over 32 000 sites and 51 000 species from a wide range of taxonomic groups (Hudson et al., 2017, 2016). GLOBIO is an integrative modeling framework for aquatic and terrestrial biodiversity that builds upon correlative relationships between biodiversity intactness and pressure variables, established with meta-analyses of biodiversity data retrieved from the literature on a wide range of taxonomic groups.
4.3 Ecosystem-based model of biodiversity
The Madingley model (Harfoot et al., 2014b) is a mechanistic individual-based model of ecosystem structure and function. It encodes a set of fundamental ecological principles to model how individual heterotrophic organisms with a body size greater than 10 µg that feed on other living organisms interact with each other and with their environment. The model is general in the sense that it applies the same set of principles for any ecosystem to which it is applied, and is applicable across scales from local to global. To capture the ecology of all organisms, the model adopts a functional trait-based approach with organisms characterized by a set of categorical traits (feeding mode, metabolic pathway, reproductive strategy, and movement ability), as well as continuous traits (juvenile, adult, and current body mass). Properties of ecological communities emerge from the interactions between organisms, influenced by their environment. The functional diversity of these ecological communities can be calculated, as well as the dissimilarity over space or time between communities (Table S2). Madingley uses three functional groups (trophic levels, metabolic pathways, and reproductive strategies).
Table 4Selected output indicators for intercomparison of biodiversity and ecosystems models. For species diversity change, both proportional changes in species richness (P) and absolute changes (N) are reported. Some models project the α metrics at the level of the grid cell (e.g., species-based and SAR based community models) while others average the local values of the metrics across the grid cell weighted by the area of the different habitats in the cell (e.g., PREDICTS, GLOBIO).
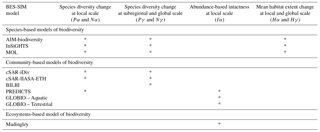
4.4 Models of ecosystem functions and services
In order to measure ecosystem functions and services, three DGVM models – LPJ-GUESS (Lindeskog et al., 2013; Olin et al., 2015; Smith et al., 2014), LPJ (Poulter et al., 2011; Sitch et al., 2003), and CABLE (Haverd et al., 2018) – and three ecosystem services models – InVEST (Sharp et al., 2016), GLOBIO (Alkemade et al., 2009, 2014; Schulp et al., 2012), and GLOSP (Guerra et al., 2016) – were engaged in this model intercomparison. The DGVMs are process-based models that simulate responses of potential natural vegetation and associated biogeochemical and hydrological cycles to changes in climate and atmospheric CO2 and disturbance regimes (Prentice et al., 2007). Processes in anthropogenically managed land (cropland, pastures, and managed forests) are also increasingly being accounted for (Arneth et al., 2017). DGVMs can project changes in future ecosystem states (e.g., type of plant functional trait (PFT), relative distribution of each PFT, biomass, height, leaf area index, water stress), ecosystem functioning (e.g., moderation of climate, processing/filtering of waste and toxicants, provision of food and medicines, modulation of productivity, decomposition, biogeochemical and nutrient flows, energy, matter, water), and habitat structure (i.e., amount, composition, and arrangement of physical matter that describe an ecosystem within a defined location and time); however, DGVMs are limited in capturing species-level biodiversity change because vegetation is represented by a small number of plant functional types (PFTs) (Bellard et al., 2012; Thuiller et al., 2013).
The InVEST suite includes 18 models that map and measure the flow and value of ecosystem goods and services across a landscape or a seascape. They are based on biophysical processes of the structure and function of ecosystems, and they account for both supply and demand. The GLOBIO model estimates ecosystem services based on outputs from the IMAGE model (Stehfest et al., 2014), the PCRaster Global Water Balance global hydrological model (PCR-GLOBWB, van Beek et al., 2011), and the Global Nutrient Model (Beusen et al., 2015). It is based on correlative relationships between ecosystem functions and services, and particular environmental variables (mainly land use), quantified based on literature data. Finally, GLOSP is a 2-D model that estimates the level of global and local soil erosion, and protection using the Universal Soil Loss Equation.
Given the diversity of modeling approaches, a wide range of biodiversity and ecosystem services metrics can be produced by the model set (Table S2). For the biodiversity model intercomparison analysis, three main categories of common output metrics were reported over time: extinctions as absolute change in species richness (N, number of species) or as proportional species richness change (P, % species), abundance-based intactness (I, % intactness), and mean proportional change in suitable habitat extent across species (H, % suitable habitat) (Table 4). These metrics were calculated at two scales: local or grid cell (α scale, i.e., the value of the metric within the smallest spatial unit of BES-SIM which is the grid cell) and regional or global scale (γ scale, i.e., the value of the metric for a set of grid cells comprising a region). For species richness change, some models project the α metrics at the grid cell level (e.g., species-based and SAR-based community models), while others average the local point values of the metrics across the grid cell weighted by the area of the different habitats in the cell (e.g., PREDICTS, GLOBIO). In addition, some models only provided α values while others provided both α and γ values (Table 4). For the models that can project γ metrics, both regional-γ for each IPBES regions (Table 1 in Brooks et al., 2016; UNEP-WCMC, 2015) and a global-γ were reported.
The species diversity change metrics measured as absolute number or percentage change in species richness show species persistence and extinction in a given time and place. Absolute changes in species richness and proportional species richness change are interrelated and may be calculated from reporting species richness over time, as and , where St is the number of species at time t. Most models reported one or both types of species richness metrics (Table 4). The abundance-based intactness (I) measures the mean species abundance in the current community relative to the abundances in a pristine community. This metric is available only for two community-based models: GLOBIO (where intactness is estimated as the arithmetic mean of the abundance ratios of the individual species, whereby ratios >1 are set to 1) and PREDICTS (where intactness is estimated as the ratios of the sum of species abundances). The habitat change (H) measures cell-wise changes in available habitat for the species. It represents the changes in the suitable habitat extent of each species relative to a baseline, i.e., (, where Ei,t is the suitable habitat extent of species i at time t within the unit of analysis. It is reported by averaging across species occurring in each unit of analysis (grid cell, region, or globe), and is provided by the species-level models (i.e., AIM-biodiversity, InSiGHTS, MOL) (Table 4). The baseline year, t0, used to calculate changes for the extinction and habitat extent metrics, was the first year of the simulation (in most cases t0=1900; see Table 5).
For ecosystem functions and services, each model's output metrics were mapped onto the new classification of Nature's Contributions to People (NCP) published by the IPBES scientific community (Díaz et al., 2018). Among the 18 possible NCPs, the combination of models participating in BES-SIM was able to provide measures for 10 NCPs, including regulating metrics on pollination (e.g., proportion of agricultural lands whose pollination needs are met, % agricultural area), climate (e.g., vegetation carbon, total carbon uptake and loss, MgC), water quantity (e.g., monthly runoff, Pg month−1), water quality (e.g., nitrogen and phosphorus leaching, PgN s−1), soil protection (e.g., erosion protection, 0–100 index), hazards (e.g., costal vulnerability, unitless score; flood risk, number of people affected) and detrimental organisms (e.g., fraction of cropland potentially protected by the natural pest relative to all available cropland, km2), and material metrics on bioenergy (e.g., bioenergy–crop production, PgC yr−1), food and feed (e.g., total crop production, 109 KCal) and materials (e.g., wood harvest, KgC) (Table 6). Some of these metrics require careful interpretation in the context of NCPs (e.g., an increase in flood risk can be caused by climate change and/or by a reduction of the capacity of ecosystems to reduce flood risk) and additional translation of increasing or declining measures of ecosystem functions and services (e.g., food and feed, water quantity) into contextually relevant information (i.e., positive or negative impacts) on human well-being and quality of life. Given the disparity of metrics across models within each NCP category, names of the metrics are listed in Table 6, and units, definitions, and methods are provided in Table S3.
The simulations for BES-SIM required a minimum of two outputs from the modeling teams: present (2015) and future (2050). Additionally, a past projection (1900) and a further future projection (2070) were also provided by several modeling teams. Some models projected further into the past and also at multiple time points from the past to the future (Appendix A). Models that simulated a continuous time series of climate change impacts provided 20-year averages around these mid-points to account for inter-annual variability. The models ran simulations at their original spatial resolutions (Appendix A), and upscaled results to 1∘ grid cells using arithmetic means. In order to provide global or regional averages of the α or grid cell metrics, the arithmetic mean values across the cells of the globe or a certain region were calculated, as well as percentiles of those metrics. Both 1∘ rasters and a table with values for each IPBES region and the globe were provided by each modeling team for each output metric.
To measure the individual and synergistic impacts of land-use and climate change on biodiversity and ecosystem services, models accounting for both types of drivers were run three times: with land-use change only, with climate change only, and with both drivers combined. For instance, to measure the impact of land use alone, the projections into 2050 were obtained while retaining climate data constant from the present (2015) to the future (2050). Similarly, to measure the impact of climate change alone, the climate projections into 2050 (or 2070) were obtained while retaining the land-use data constant from the present (2015) to the future (2050). Finally, to measure the impact of land-use and climate change combined, models were run using projections of both land-use and climate change into 2050 (or 2070). When models required continuous climate time-series data to hindcast to 1900, data from years in the time period 1951 to 1960 were randomly selected to fill the data missing for years 1901 to 1950 from the ISIMIP 2a IPSL dataset. Models that used multi-decadal climate averages from WorldClim (i.e., InSiGHTS, BILBI) assumed no climate impacts for 1900.
Reporting uncertainty is a critical component of model intercomparison exercises (IPBES, 2016). Within BES-SIM, uncertainties were explored by each model reporting the mean values of its metrics, and where possible the 25th, 50th, and 75th percentiles based on the parameterization set specific to each model, which can be found in each model's key manuscripts describing the modeling methods. When combining the data provided by the different models, the average and the standard deviations of the common metrics were calculated (e.g., intermodel average and standard deviation of Pγ). In a parallel exercise to inform BES-SIM, the BIOMOD2 model was used in assessing the uncertainty in modeling changes in species ranges arising from using different RCP scenarios, different GCMs, a suite of species distribution modeling algorithms (e.g., random forest, logistic regression), and different species dispersal hypotheses.
The existing SSP and RCP scenarios provide a consistent set of past and future projections of two major drivers of terrestrial and freshwater biodiversity change – land use and climate. However, we acknowledge that these projections have certain limitations. These include limited consideration of biodiversity-specific policies in the storylines (only the SSP1 baseline emphasizes additional biodiversity policies) (O'Neill et al., 2016; Rosa et al., 2017), coarse spatial resolution, and land-use classes that are not sufficiently detailed to fully capture the response of biodiversity to land-use change (Harfoot et al., 2014a; Titeux et al., 2016, 2017). The heterogeneity of models and their methodological approaches, as well as additional harmonization of metrics of ecosystem functions and services (Tables 6, S3), are areas for further work. In the future, it will also be important to capture the uncertainties associated with input data, with a focus on uncertainty in land-use and climate projections resulting from differences among IAMs and GCMs on each scenario (Popp et al., 2017). The gaps identified through BES-SIM and future directions for research and modeling will be published separately, as well as analyses of the results on the model intercomparison and on individual models. As a long-term perspective, BES-SIM is expected to provide critical foundation and insights for the ongoing development of nature-centred, multiscale Nature Futures scenarios (Rosa et al., 2017). Catalyzed by the IPBES Expert Group on Scenarios and Models, this new scenario and modeling framework will shift traditional ways of forecasting impacts of society on nature to more integrative, biodiversity-centred visions and pathways of socio-economic and ecological systems. A future round of BES-SIM could use these biodiversity-centred storylines to project dynamics of biodiversity and ecosystem services and associated consequences for socio-economic development and human well-being. This will help policymakers and practitioners to collectively identify pathways for sustainable futures based on alternative biodiversity management approaches and assist researchers in incorporating the role of biodiversity into socio-economic scenarios.
The output data from this model intercomparison will be downloadable from the website of the IPBES Expert Group on Scenarios and Models in the future (https://www.ipbes.net/deliverables/3c-scenarios-and-modelling, last access: 8 November 2018). The LUH2 land-use data used for model runs are available at http://luh.umd.edu/data.shtml (Hurtt et al., 2017). The climate datasets used in BES-SIM can be downloaded from the respective websites (https://www.isimip.org/outputdata/ (Inter-sectoral Impact Model Intercomparison Project Output Data, 2017), http://worldclim.org/version1, Hijmans et al., 2017).
AIM | Asia-pacific Integrated Model |
BES-SIM | Biodiversity and Ecosystem Services Scenario-based Intercomparison of Models |
BIOMOD | BIOdiversity MODelling |
BILBI | Biogeographic modelling Infrastructure for Large-scale Biodiversity Indicators |
CABLE | Community Atmosphere Biosphere Land Exchange |
CMIP | Climate Model Inter-comparison Project |
cSAR | Countryside Species Area Relationship |
DGVM | Dynamic global vegetation model |
EBV | Essential biodiversity variable |
ESMs | Earth system models |
GBIF | Global Biodiversity Information Facility |
GBO | Global Biodiversity Outlooks |
GCMs | General circulation models |
GEO | Global Environmental Outlook |
GLOBIO | GLObal BIOdiversity |
GLOSP | GLObal Soil Protection |
IAM | Integrated Assessment Models |
IMAGE | Integrated Model to Assess the Global Environment |
InVEST | Integrated Valuation of Ecosystem Services and Tradeoffs |
IPBES | Intergovernmental Science-Policy Platform on Biodiversity and Ecosystem Services |
IPCC | Intergovernmental Panel on Climate Change |
IPSL-CM5A-LR | Institut Pierre-Simon Laplace-Climate Model 5A-Low Resolution |
ISI-MIP | Inter-Sectoral Impact Model Intercomparison Project |
LPJ | Lund-Potsdam-Jena |
LPJ-GUESS | Lund-Potsdam-Jena General Ecosystem Simulator |
LUH2 | Land Use Harmonization Project version 2 |
MA | Millennium Ecosystem Assessment |
MAgPIE | The Model of Agricultural Production and its Impact on the Environment |
MIP | Model Intercomparison Project |
MOL | Map of Life |
NCP | Nature's Contributions to People |
REMIND | Regionalized Model of Investments and Development |
PREDICTS | Projecting Responses of Ecological Diversity In Changing Terrestrial Systems |
RCM | Regional Climate Models |
RCPs | Representative Concentration Pathways |
PCR-GLOBWB | PCRaster Global Water Balance |
SAR | Species–area relationship |
SR | Species richness |
SSPs | Shared Socio-economic Pathways |
The supplement related to this article is available online at: https://doi.org/10.5194/gmd-11-4537-2018-supplement.
All the authors co-designed the study and provided scientific input and technical details on models, scenarios and data necessary to carry out the intermodel comparison and synthesis. HMP, RA, PL, and IMDR led the development of the protocol, and HK led the writing of the manuscript with model-specific text contributions and review comments from all co-authors.
The authors declare that they have no conflict of interest.
HyeJin Kim, Inês Santos Martins, Florian Wolf, Carlos Guerra, and Henrique
M. Pereira are supported by the German Centre for Integrative Biodiversity
Research (iDiv) Halle-Jena-Leipzig, funded by the German Research Foundation
(FZT 118). Isabel Maria Duarte Rosa acknowledges funding from the European
Union's Horizon 2020 research and innovation program under Marie
Sklodowska-Curie grant agreement no. 703862. Paul Leadley is supported by the
LabEx BASC supported by the French “Investment d'Avenir” program (grant
ANR-11-LABX-0034). George C. Hurtt and Louise Parsons Chini gratefully
acknowledge the support of the DOE-SciDAC program (DE SC0012972). Almut
Arneth, Andreas Krause, Benjamin Quesada, and Peter Anthoni acknowledge
support from the Helmholtz Association and its ATMO Programme, and EU FP7
project LUC4C. Andy Purvis, Adriana De Palm, and Samantha L. L. Hill are
supported by the Natural Environment Research Council U.K. (grant number
NE/M014533/1) and by a DIF grant from the Natural History Museum. Rebecca
Chaplin-Kramer and Richard Sharp are supported by private gifts to the
Natural Capital Project. David Leclère, Fulvio Di Fulvio, Petr Havlík,
and Michael Obersteiner are supported by the project IS-WEL-Integrated
Solutions for Water, Energy and Land funding from the Global Environmental
Facility, Washington, USA, coordinated by the United Nations Industrial
Development Organization (UNIDO), UNIDO project no. 140312. Fulvio Di Fulvio
and Michael Obersteiner are supported by the ERC SYNERGY grant project
IMBALANCE-P-Managing Phosphorous limitation in a nitrogen-saturated
Anthropocene, funding from the European Commission, European Research Council
Executive Agency, grant agreement no. 610028. David Leclère and Petr
Havlík are supported by project SIGMA – Stimulating Innovation for Global
Monitoring of Agriculture – and its Impact on the Environment in support of
GEOGLAM, funding from the European Union's FP7 research and innovation
program under the environment area, grant agreement no. 603719. Tomoko
Hasegawa, Haruka Ohashi, Akiko Hirata, Shinichiro Fujimori, Tetsuya Matsui,
and Kiyoshi Takahashi are supported by the Global Environmental Research
(S-14) of the Ministry of the Environment of Japan. Tomoko Hasegawa,
Shinichiro Fujimori, and Kiyoshi Takahashi are supported by Environment
Research and Technology Development Fund 2-1702 of the Environmental
Restoration and Conservation Agency of Japan. Mike Harfoot is supported by a
KR Rasmussen Foundation grant “Modelling the Biodiversity Planetary Boundary
and Embedding Results into Policy” (FP-1503-01714). Vanessa Haverd
acknowledges support from the Earth Systems and Climate Change Hub, funded by
the Australian Government's National Environmental Science program. Cory
Merow acknowledges funding from NSF grant DEB1565046. Finally, we also thank
the following organizations for funding the workshops: the PBL Netherland
Environment Assessment Agency, UNESCO (March 2016), the iDiv German Centre
for Integrative Biodiversity Research (October 2016, October 2017), and the
Zoological Society of London (January 2018).
Edited by: Hisashi Sato
Reviewed by: two anonymous referees
Aguirre-Gutiérrez, J., Carvalheiro, L. G., Polce, C., van Loon, E. E., Raes, N., Reemer, M., Biesmeijer, J. C., and Chapman, M. G. (Eds.): Fit-for-Purpose: Species Distribution Model Performance Depends on Evaluation Criteria – Dutch Hoverflies as a Case Study, PLoS ONE, 8, e63708, https://doi.org/10.1371/journal.pone.0063708, 2013.
Akçakaya, H. R., Pereira, H. M., Canziani, G. A., Mbow, C., Mori, A., Palomo, M. G., Soberoin, J., Thuiller, W., Yachi, S., Ferrier, S., Ninan, K. N., Leadley, P., Alkemade, R., Acosta, L. A., Akçakaya, H. R., Brotons, L., Cheung, W. W. L., Christensen, V., Harhash, K. A., Kabubo-Mariara, J., Lundquist, C., Obersteiner, M., Pereira, H. M., Peterson, G., Pichs-Madruga, R., Ravindranath, N., Rondinini, C., and Wintle, B. A. (Eds.): Improving the rigour and usefulness of scenarios and models through ongoing evaluation and refinement, The methodological assessment report on scenarios and models of biodiversity and ecosystem services, Secretariat of the Intergovernmental Science-Policy Platform for Biodiversity and Ecosystem Services, Bonn, Germany, 2015.
Alkemade, R., van Oorschot, M., Miles, L., Nellemann, C., Bakkenes, M., and ten Brink, B.: GLOBIO3: A Framework to Investigate Options for Reducing Global Terrestrial Biodiversity Loss, Ecosystems, 12, 374–390, https://doi.org/10.1007/s10021-009-9229-5, 2009.
Alkemade, R., Burkhard, B., Crossman, N. D., Nedkov, S., and Petz, K.: Quantifying ecosystem services and indicators for science, policy and practice, Ecol. Indic., 37, 161–162, https://doi.org/10.1016/j.ecolind.2013.11.014, 2014.
Arkema, K. K., Guannel, G., Verutes, G., Wood, S. A., Guerry, A., Ruckelshaus, M., Kareiva, P., Lacayo, M., and Silver, J. M.: Coastal habitats shield people and property from sea-level rise and storms, Nat. Clim. Change, 3, 913–918, https://doi.org/10.1038/nclimate1944, 2013.
Arneth, A., Sitch, S., Pongratz, J., Stocker, B. D., Ciais, P., Poulter, B., Bayer, A. D., Bondeau, A., Calle, L., Chini, L. P., Gasser, T., Fader, M., Friedlingstein, P., Kato, E., Li, W., Lindeskog, M., Nabel, J. E. M. S., Pugh, T. A. M., Robertson, E., Viovy, N., Yue, C., and Zaehle, S.: Historical carbon dioxide emissions caused by land-use changes are possibly larger than assumed, Nat. Geosci., 10, 79–84, https://doi.org/10.1038/ngeo2882, 2017.
Bellard, C., Bertelsmeier, C., Leadley, P., Thuiller, W., and Courchamp, F.: Impacts of climate change on the future of biodiversity: Biodiversity and climate change, Ecol. Lett., 15, 365–377, https://doi.org/10.1111/j.1461-0248.2011.01736.x, 2012.
Beusen, A. H. W., Van Beek, L. P. H., Bouwman, A. F., Mogollón, J. M., and Middelburg, J. J.: Coupling global models for hydrology and nutrient loading to simulate nitrogen and phosphorus retention in surface water – description of IMAGE–GNM and analysis of performance, Geosci. Model Dev., 8, 4045–4067, https://doi.org/10.5194/gmd-8-4045-2015, 2015.
Brooks, T. M., Akçakaya, H. R., Burgess, N. D., Butchart, S. H. M., Hilton-Taylor, C., Hoffmann, M., Juffe-Bignoli, D., Kingston, N., MacSharry, B., Parr, M., Perianin, L., Regan, E. C., Rodrigues, A. S. L., Rondinini, C., Shennan-Farpon, Y., and Young, B. E.: Analysing biodiversity and conservation knowledge products to support regional environmental assessments, Scientific Data, 3, 160007, https://doi.org/10.1038/sdata.2016.7, 2016.
Cardinale, B. J., Duffy, J. E., Gonzalez, A., Hooper, D. U., Perrings, C., Venail, P., Narwani, A., Mace, G. M., Tilman, D., Wardle, D. A., Kinzig, A. P., Daily, G. C., Loreau, M., Grace, J. B., Larigauderie, A., Srivastava, D. S., and Naeem, S.: Biodiversity loss and its impact on humanity, Nature, 486, 59–67, https://doi.org/10.1038/nature11148, 2012.
Chaplin-Kramer, R., Dombeck, E., Gerber, J., Knuth, K. A., Mueller, N. D., Mueller, M., Ziv, G., and Klein, A.-M.: Global malnutrition overlaps with pollinator-dependent micronutrient production, P. R. Soc. B-Biol. Sci., 281, 20141799–20141799, https://doi.org/10.1098/rspb.2014.1799, 2014.
Chaudhary, A., Verones, F., de Baan, L., and Hellweg, S.: Quantifying Land Use Impacts on Biodiversity: Combining Species–Area Models and Vulnerability Indicators, Environ. Sci. Technol., 49, 9987–9995, https://doi.org/10.1021/acs.est.5b02507, 2015.
D'Amen, M., Rahbek, C., Zimmermann, N. E., and Guisan, A.: Spatial predictions at the community level: from current approaches to future frameworks: Methods for community-level spatial predictions, Biol. Rev., 92, 169–187, https://doi.org/10.1111/brv.12222, 2017.
Díaz, S., Pascual, U., Stenseke, M., Martín-López, B., Watson, R. T., Molnár, Z., Hill, R., Chan, K. M. A., Baste, I. A., Brauman, K. A., Polasky, S., Church, A., Lonsdale, M., Larigauderie, A., Leadley, P. W., van Oudenhoven, A. P. E., van der Plaat, F., Schröter, M., Lavorel, S., Aumeeruddy-Thomas, Y., Bukvareva, E., Davies, K., Demissew, S., Erpul, G., Failler, P., Guerra, C. A., Hewitt, C. L., Keune, H., Lindley, S., and Shirayama, Y.: Assessing nature's contributions to people, Science, 359, 270–272, https://doi.org/10.1126/science.aap8826, 2018.
Dufresne, J.-L., Foujols, M.-A., Denvil, S., Caubel, A., Marti, O., Aumont, O., Balkanski, Y., Bekki, S., Bellenger, H., Benshila, R., Bony, S., Bopp, L., Braconnot, P., Brockmann, P., Cadule, P., Cheruy, F., Codron, F., Cozic, A., Cugnet, D., de Noblet, N., Duvel, J.-P., Ethé, C., Fairhead, L., Fichefet, T., Flavoni, S., Friedlingstein, P., Grandpeix, J.-Y., Guez, L., Guilyardi, E., Hauglustaine, D., Hourdin, F., Idelkadi, A., Ghattas, J., Joussaume, S., Kageyama, M., Krinner, G., Labetoulle, S., Lahellec, A., Lefebvre, M.-P., Lefevre, F., Levy, C., Li, Z. X., Lloyd, J., Lott, F., Madec, G., Mancip, M., Marchand, M., Masson, S., Meurdesoif, Y., Mignot, J., Musat, I., Parouty, S., Polcher, J., Rio, C., Schulz, M., Swingedouw, D., Szopa, S., Talandier, C., Terray, P., Viovy, N., and Vuichard, N.: Climate change projections using the IPSL-CM5 Earth System Model: from CMIP3 to CMIP5, Clim. Dynam., 40, 2123–2165, https://doi.org/10.1007/s00382-012-1636-1, 2013.
Elith, J. and Leathwick, J. R.: Species Distribution Models: Ecological Explanation and Prediction Across Space and Time, Annu. Rev. Ecol. Evol. S., 40, 677–697, https://doi.org/10.1146/annurev.ecolsys.110308.120159, 2009.
Ferrier, S., Powell, G. V. N., Richardson, K. S., Manion, G., Overton, J. M., Allnutt, T. F., Cameron, S. E., Mantle, K., Burgess, N. D., Faith, D. P., Lamoreux, J. F., Kier, G., Hijmans, R. J., Funk, V. A., Cassis, G. A., Fisher, B. L., Flemons, P., Lees, D., Lovett, J. C., and Van Rompaey, R. S. A. R.: Mapping More of Terrestrial Biodiversity for Global Conservation Assessment, BioScience, 54, 1101, https://doi.org/10.1641/0006-3568(2004)054[1101:MMOTBF]2.0.CO;2, 2004.
Ferrier, S., Manion, G., Elith, J., and Richardson, K.: Using generalized dissimilarity modelling to analyse and predict patterns of beta diversity in regional biodiversity assessment, Divers. Distrib., 13, 252–264, https://doi.org/10.1111/j.1472-4642.2007.00341.x, 2007.
Fick, S. E. and Hijmans, R. J.: WorldClim 2: new 1-km spatial resolution climate surfaces for global land areas: New climate surface for global land areas, Int. J. Climatol., 37, 4302–4315, https://doi.org/10.1002/joc.5086, 2017.
Fourcade, Y.: Comparing species distributions modelled from occurrence data and from expert-based range maps. Implication for predicting range shifts with climate change, Ecological Informatics, 36, 8–14, https://doi.org/10.1016/j.ecoinf.2016.09.002, 2016.
Frieler, K., Levermann, A., Elliott, J., Heinke, J., Arneth, A., Bierkens, M. F. P., Ciais, P., Clark, D. B., Deryng, D., Döll, P., Falloon, P., Fekete, B., Folberth, C., Friend, A. D., Gellhorn, C., Gosling, S. N., Haddeland, I., Khabarov, N., Lomas, M., Masaki, Y., Nishina, K., Neumann, K., Oki, T., Pavlick, R., Ruane, A. C., Schmid, E., Schmitz, C., Stacke, T., Stehfest, E., Tang, Q., Wisser, D., Huber, V., Piontek, F., Warszawski, L., Schewe, J., Lotze-Campen, H., and Schellnhuber, H. J.: A framework for the cross-sectoral integration of multi-model impact projections: land use decisions under climate impacts uncertainties, Earth Syst. Dynam., 6, 447–460, https://doi.org/10.5194/esd-6-447-2015, 2015.
Frieler, K., Lange, S., Piontek, F., Reyer, C. P. O., Schewe, J., Warszawski, L., Zhao, F., Chini, L., Denvil, S., Emanuel, K., Geiger, T., Halladay, K., Hurtt, G., Mengel, M., Murakami, D., Ostberg, S., Popp, A., Riva, R., Stevanovic, M., Suzuki, T., Volkholz, J., Burke, E., Ciais, P., Ebi, K., Eddy, T. D., Elliott, J., Galbraith, E., Gosling, S. N., Hattermann, F., Hickler, T., Hinkel, J., Hof, C., Huber, V., Jägermeyr, J., Krysanova, V., Marcé, R., Müller Schmied, H., Mouratiadou, I., Pierson, D., Tittensor, D. P., Vautard, R., van Vliet, M., Biber, M. F., Betts, R. A., Bodirsky, B. L., Deryng, D., Frolking, S., Jones, C. D., Lotze, H. K., Lotze-Campen, H., Sahajpal, R., Thonicke, K., Tian, H., and Yamagata, Y.: Assessing the impacts of 1.5 ∘C global warming – simulation protocol of the Inter-Sectoral Impact Model Intercomparison Project (ISIMIP2b), Geosci. Model Dev., 10, 4321–4345, https://doi.org/10.5194/gmd-10-4321-2017, 2017.
Frischknecht, R., Fantke, P., Tschümperlin, L., Niero, M., Antón, A., Bare, J., Boulay, A.-M., Cherubini, F., Hauschild, M. Z., Henderson, A., Levasseur, A., McKone, T. E., Michelsen, O., Canals, L. M., Pfister, S., Ridoutt, B., Rosenbaum, R. K., Verones, F., Vigon, B., and Jolliet, O.: Global guidance on environmental life cycle impact assessment indicators: progress and case study, Int. J. Life Cycle Ass., 21, 429–442, https://doi.org/10.1007/s11367-015-1025-1, 2016.
Fujimori, S., Hasegawa, T., Masui, T., Takahashi, K., Herran, D. S., Dai, H., Hijioka, Y., and Kainuma, M.: SSP3: AIM implementation of Shared Socioeconomic Pathways, Global Environ. Chang., 42, 268–283, https://doi.org/10.1016/j.gloenvcha.2016.06.009, 2017.
Graham, C. T., Wilson, M. W., Gittings, T., Kelly, T. C., Irwin, S., Quinn, J. L., and O'Halloran, J.: Implications of afforestation for bird communities: the importance of preceding land-use type, Biodivers. Conserv., 26, 3051–3071, https://doi.org/10.1007/s10531-015-0987-4, 2017.
Guannel, G., Arkema, K., Ruggiero, P., Verutes, G., and Bianchi, C. N. (Eds.): The Power of Three: Coral Reefs, Seagrasses and Mangroves Protect Coastal Regions and Increase Their Resilience, PLOS ONE, 11, e0158094, https://doi.org/10.1371/journal.pone.0158094, 2016.
Guerra, C. A., Maes, J., Geijzendorffer, I., and Metzger, M. J.: An assessment of soil erosion prevention by vegetation in Mediterranean Europe: Current trends of ecosystem service provision, Ecol. Indic., 60, 213–222, https://doi.org/10.1016/j.ecolind.2015.06.043, 2016.
Guisan, A. and Thuiller, W.: Predicting species distribution: offering more than simple habitat models, Ecol. Lett., 8, 993–1009, https://doi.org/10.1111/j.1461-0248.2005.00792.x, 2005.
Guisan, A. and Zimmermann, N. E.: Predictive habitat distribution models in ecology, Ecol. Model., 135, 147–186, https://doi.org/10.1016/S0304-3800(00)00354-9, 2000.
Harfoot, M., Tittensor, D. P., Newbold, T., McInerny, G., Smith, M. J., and Scharlemann, J. P. W.: Integrated assessment models for ecologists: the present and the future: Integrated assessment models for ecologists, Global Ecol. Biogeogr., 23, 124–143, https://doi.org/10.1111/geb.12100, 2014a.
Harfoot, M. B. J., Newbold, T., Tittensor, D. P., Emmott, S., Hutton, J., Lyutsarev, V., Smith, M. J., Scharlemann, J. P. W., Purves, D. W., and Loreau, M. (Eds.): Emergent Global Patterns of Ecosystem Structure and Function from a Mechanistic General Ecosystem Model, PLoS Biol., 12, e1001841, https://doi.org/10.1371/journal.pbio.1001841, 2014b.
Haverd, V., Smith, B., Nieradzik, L., Briggs, P. R., Woodgate, W., Trudinger, C. M., Canadell, J. G., and Cuntz, M.: A new version of the CABLE land surface model (Subversion revision r4601) incorporating land use and land cover change, woody vegetation demography, and a novel optimisation-based approach to plant coordination of photosynthesis, Geosci. Model Dev., 11, 2995–3026, https://doi.org/10.5194/gmd-11-2995-2018, 2018.
Heinimann, A., Mertz, O., Frolking, S., Egelund Christensen, A., Hurni, K., Sedano, F., Parsons Chini, L., Sahajpal, R., Hansen, M., Hurtt, G., and Poulter, B. (Eds.): A global view of shifting cultivation: Recent, current, and future extent, PLOS ONE, 12, e0184479, https://doi.org/10.1371/journal.pone.0184479, 2017.
Hempel, S., Frieler, K., Warszawski, L., Schewe, J., and Piontek, F.: A trend-preserving bias correction – the ISI-MIP approach, Earth Syst. Dynam., 4, 219–236, https://doi.org/10.5194/esd-4-219-2013, 2013.
Hijmans, R. J., Cameron, S. E., Parra, J. L., Jones, P. G., and Jarvis, A.: WorldClim Global Climate Data Version 1, available at: http://worldclim.org/version1, last access: 20 November 2017.
Hirsch, T. and Secretariat of the Convention on Biological Diversity (Eds.): Global biodiversity outlook 3, Secretariat of the Convention on Biological Diversity, Montreal, Quebec, Canada, 2010.
Hoskins, A. J., Harwood, T. D., Ware, C., Williams, K. J., Perry, J. J., Ota, N., Croft, J. R., Yeates, D. K., Jetz, W., Golebiewski, M., Purvis, A., and Ferrier, S.: Supporting global biodiversity assessment through high-resolution macroecological modelling: Methodological underpinnings of the BILBI framework, BioRxiv http://biorxiv.org/cgi/content/short/309377v1, in preparation, last access: 28 October 2018.
Hudson, L., Newbold, T., Contu, S., Hill, S., Lysenko, I., De Palma, A., Phillips, H., Senior, R., Bennett, D., Booth, H., Choimes, A., Correia, D., Day, J., Echeverría-Londoño, S., Garon, M., Harrison, M., Ingram, D., Jung, M., Kemp, V., Kirkpatrick, L., Martin, C., Pan, Y., White, H., Aben, J., Abrahamczyk, S., Adum, G., Aguilar-Barquero, V., Aizen, M., Ancrenaz, M., Arbeláez-Cortés, E., Armbrecht, I., Azhar, B., Azpiroz, A., Baeten, L., Báldi, A., Banks, J., Barlow, J., Batáry, P., Bates, A., Bayne, E., Beja, P., Berg, Å., Berry, N., Bicknell, J., Bihn, J., Böhning-Gaese, K., Boekhout, T., Boutin, C., Bouyer, J., Brearley, F., Brito, I., Brunet, J., Buczkowski, G., Buscardo, E., Cabra-García, J., Calvño-Cancela, M., Cameron, S., Cancello, E., Carrijo, T., Carvalho, A., Castro, H., Castro-Luna, A., Cerda, R., Cerezo, A., Chauvat, M., Clarke, F., Cleary, D., Connop, S., D'Aniello, B., da Silva, P., Darvill, B., Dauber, J., Dejean, A., Diekötter, T., Dominguez-Haydar, Y., Dormann, C., Dumont, B., Dures, S., Dynesius, M., Edenius, L., Elek, Z., Entling, M., Farwig, N., Fayle, T., Felicioli, A., Felton, A., Ficetola, G., Filgueiras, B., Fonte, S., Fraser, L., Fukuda, D., Furlani, D., Ganzhorn, J., Garden, J., Gheler-Costa, C., Giordani, P., Giordano, S., Gottschalk, M., Goulson, D., Gove, A., Grogan, J., Hanley, M., Hanson, T., Hashim, N., Hawes, J., Hébert, C., Helden, A., Henden, J., Hernández, L., Herzog, F., Higuera-Diaz, D., Hilje, B., Horgan, F., Horváth, R., Hylander, K., Isaacs-Cubides, P., Ishitani, M., Jacobs, C., Jaramillo, V., Jauker, B., Jonsell, M., Jung, T., Kapoor, V., Kati, V., Katovai, E., Kessler, M., Knop, E., Kolb, A., Krösi, Á., Lachat, T., Lantschner, V., Le Féon, V., LeBuhn, G., Légaré, J., Letcher, S., Littlewood, N., López-Quintero, C., Louhaichi, M., Lövei, G., Lucas-Borja, M., Luja, V., Maeto, K., Magura, T., Mallari, N., Marin-Spiotta, E., Marshall, E., Martínez, E., Mayfield, M., Mikusinski, G., Milder, J., Miller, J., Morales, C., Muchane, M., Muchane, M., Naidoo, R., Nakamura, A., Naoe, S., Nates-Parra, G., Navarrete Gutierrez, D., Neuschulz, E., Noreika, N., Norfolk, O., Noriega, J., Nöske, N., O'Dea, N., Oduro, W., Ofori-Boateng, C., Oke, C., Osgathorpe, L., Paritsis, J., Parra-H, A., Pelegrin, N., Peres, C., Persson, A., Petanidou, T., Phalan, B., Philips, T., Poveda, K., Power, E., Presley, S., Proença, V., Quaranta, M., Quintero, C., Redpath-Downing, N., Reid, J., Reis, Y., Ribeiro, D., Richardson, B., Richardson, M., Robles, C., Römbke, J., Romero-Duque, L., Rosselli, L., Rossiter, S., Roulston, T., Rousseau, L., Sadler, J., Sáfián, S., Saldaña-Vázquez, R., Samnegård, U., Schüepp, C., Schweiger, O., Sedlock, J., Shahabuddin, G., Sheil, D., Silva, F., Slade, E., Smith-Pardo, A., Sodhi, N., Somarriba, E., Sosa, R., Stout, J., Struebig, M., Sung, Y., Threlfall, C., Tonietto, R., Tóthmérész, B., Tscharntke, T., Turner, E., Tylianakis, J., Vanbergen, A., Vassilev, K., Verboven, H., Vergara, C., Vergara, P., Verhulst, J., Walker, T., Wang, Y., Watling, J., Wells, K., Williams, C., Willig, M., Woinarski, J., Wolf, J., Woodcock, B., Yu, D., Zaitsev, A., Collen, B., Ewers, R., Mace, G., Purves, D., Scharlemann, J., and Purvis, A.: The PREDICTS database: a global database of how local terrestrial biodiversity responds to human impacts, Ecol. Evol., 4, 4701–4735, https://doi.org/10.1002/ece3.1303, 2014.
Hudson, L. N., Newbold, T., Contu, S., et al.: The 2016 release of the PREDICTS database, https://doi.org/10.5519/0066354, 2016.
Hudson, L. N., Newbold, T., Contu, S., Hill, S. L. L., Lysenko, I., De Palma, A., Phillips, H. R. P., Alhusseini, T. I., Bedford, F. E., Bennett, D. J., Booth, H., Burton, V. J., Chng, C. W. T., Choimes, A., Correia, D. L. P., Day, J., Echeverría-Londoño, S., Emerson, S. R., Gao, D., Garon, M., Harrison, M. L. K., Ingram, D. J., Jung, M., Kemp, V., Kirkpatrick, L., Martin, C. D., Pan, Y., Pask-Hale, G. D., Pynegar, E. L., Robinson, A. N., Sanchez-Ortiz, K., Senior, R. A., Simmons, B. I., White, H. J., Zhang, H., Aben, J., Abrahamczyk, S., Adum, G. B., Aguilar-Barquero, V., Aizen, M. A., Albertos, B., Alcala, E. L., del Mar Alguacil, M., Alignier, A., Ancrenaz, M., Andersen, A. N., Arbeláez-Cortés, E., Armbrecht, I., Arroyo-Rodríguez, V., Aumann, T., Axmacher, J. C., Azhar, B., Azpiroz, A. B., Baeten, L., Bakayoko, A., Báldi, A., Banks, J. E., Baral, S. K., Barlow, J., Barratt, B. I. P., Barrico, L., Bartolommei, P., Barton, D. M., Basset, Y., Batáry, P., Bates, A. J., Baur, B., Bayne, E. M., Beja, P., Benedick, S., Berg, Å., Bernard, H., Berry, N. J., Bhatt, D., Bicknell, J. E., Bihn, J. H., Blake, R. J., Bobo, K. S., Bóçon, R., Boekhout, T., Böhning-Gaese, K., Bonham, K. J., Borges, P. A. V., Borges, S. H., Boutin, C., Bouyer, J., Bragagnolo, C., Brandt, J. S., Brearley, F. Q., Brito, I., Bros, V., Brunet, J., Buczkowski, G., Buddle, C. M., Bugter, R., Buscardo, E., Buse, J., Cabra-García, J., Cáceres, N. C., et al.: The database of the PREDICTS (Projecting Responses of Ecological Diversity In Changing Terrestrial Systems) project, Ecol. Evol., 7, 145–188, https://doi.org/10.1002/ece3.2579, 2017.
Hurtt, G., Chini, L., Sahajpal, R., Frolking, S., Calvin, K., Fujimori, S., Klein Goldewijk, K., Hasegawa, T., Havlik, P., Lawrence, D., Lawrence, P., Popp, A., Stehfest, E., van Vuuren, D., and Zhang, X.: Land-Use Harmonization 2, available at: http://luh.umd.edu/data.shtml, last access: 21 December 2017.
Hurtt, G., Chini, L., Sahajpal, R., Frolking, S., Calvin, K., Fujimori, S., Klein Goldewijk, K., Hasegawa, T., Havlik, P., Lawrence, D., Lawrence, P., Popp, A., Stehfest, E., van Vuuren, D., and Zhang, X.: Harmonization of global land-use change and management for the period 850–2100, in preparation, 2018.
Hurtt, G. C., Chini, L. P., Frolking, S., Betts, R. A., Feddema, J., Fischer, G., Fisk, J. P., Hibbard, K., Houghton, R. A., Janetos, A., Jones, C. D., Kindermann, G., Kinoshita, T., Klein Goldewijk, K., Riahi, K., Shevliakova, E., Smith, S., Stehfest, E., Thomson, A., Thornton, P., van Vuuren, D. P., and Wang, Y. P.: Harmonization of land-use scenarios for the period 1500–2100: 600 years of global gridded annual land-use transitions, wood harvest, and resulting secondary lands, Climatic Change, 109, 117–161, https://doi.org/10.1007/s10584-011-0153-2, 2011.
Inter-sectoral Impact Model Intercomparison Project Output Data: available at: https://www.isimip.org/outputdata/, last access: 20 October 2017.
IPBES: The methodological assessment report on scenarios and models of biodiversity and ecosystem services, edited by: Ferrier, S., Ninan, K. N., Leadley, P., Alkemade, R., Acosta, L. A., Akçakaya, H. R., Brotons, L., Cheung, W. W. L., Christensen, V., Harhash, K. A., Kabubo-Mariara, J., Lundquist, C., Obersteiner, M., Pereira, H. M., Peterson, G., Pichs-Madruga, R., Ravindranath, N., Rondinini, C., and Wintle, B. A., Secretariat of the Intergovernmental Science-Policy Platform on Biodiversity and Ecosystem Services, Bonn, Germany, 348 pp., 2016.
Janse, J. H., Kuiper, J. J., Weijters, M. J., Westerbeek, E. P., Jeuken, M. H. J. L., Bakkenes, M., Alkemade, R., Mooij, W. M. and Verhoeven, J. T. A.: GLOBIO-Aquatic, a global model of human impact on the biodiversity of inland aquatic ecosystems, Environ. Sci. Policy, 48, 99–114, https://doi.org/10.1016/j.envsci.2014.12.007, 2015.
Janse, J. H., Bakkenes, M., and Meijer, J.: Globio-Aquatic, Technical model description v. 1.3, PBL publication 2829, The Hague, PBL Netherlands Environmental Assessment Agency, 2016.
Jantz, S. M., Barker, B., Brooks, T. M., Chini, L. P., Huang, Q., Moore, R. M., Noel, J., and Hurtt, G. C.: Future habitat loss and extinctions driven by land-use change in biodiversity hotspots under four scenarios of climate-change mitigation: Future Habitat Loss and Extinctions, Conserv. Biol., 29, 1122–1131, https://doi.org/10.1111/cobi.12549, 2015.
Jetz, W., Wilcove, D. S., Dobson, A. P., and Mace, G. M. (Eds.): Projected Impacts of Climate and Land-Use Change on the Global Diversity of Birds, PLoS Biol., 5, e157, https://doi.org/10.1371/journal.pbio.0050157, 2007.
Johnson, J. A., Runge, C. F., Senauer, B., Foley, J., and Polasky, S.: Global agriculture and carbon trade-offs, P. Natl. Acad. Sci. USA, 111, 12342–12347, https://doi.org/10.1073/pnas.1412835111, 2014.
Johnson, J. A., Runge, C. F., Senauer, B., and Polasky, S.: Global Food Demand and Carbon-Preserving Cropland Expansion under Varying Levels of Intensification, Land Econ., 92, 579–592, https://doi.org/10.3368/le.92.4.579, 2016.
Jungclaus, J. H., Bard, E., Baroni, M., Braconnot, P., Cao, J., Chini, L. P., Egorova, T., Evans, M., González-Rouco, J. F., Goosse, H., Hurtt, G. C., Joos, F., Kaplan, J. O., Khodri, M., Klein Goldewijk, K., Krivova, N., LeGrande, A. N., Lorenz, S. J., Luterbacher, J., Man, W., Maycock, A. C., Meinshausen, M., Moberg, A., Muscheler, R., Nehrbass-Ahles, C., Otto-Bliesner, B. I., Phipps, S. J., Pongratz, J., Rozanov, E., Schmidt, G. A., Schmidt, H., Schmutz, W., Schurer, A., Shapiro, A. I., Sigl, M., Smerdon, J. E., Solanki, S. K., Timmreck, C., Toohey, M., Usoskin, I. G., Wagner, S., Wu, C.-J., Yeo, K. L., Zanchettin, D., Zhang, Q., and Zorita, E.: The PMIP4 contribution to CMIP6 – Part 3: The last millennium, scientific objective, and experimental design for the PMIP4 past1000 simulations, Geosci. Model Dev., 10, 4005–4033, https://doi.org/10.5194/gmd-10-4005-2017, 2017.
Knorr, W., Arneth, A., and Jiang, L.: Demographic controls of future global fire risk, Nat. Clim. Change, 6, 781–785, https://doi.org/10.1038/nclimate2999, 2016.
Kriegler, E., Bauer, N., Popp, A., Humpenöder, F., Leimbach, M., Strefler, J., Baumstark, L., Bodirsky, B. L., Hilaire, J., Klein, D., Mouratiadou, I., Weindl, I., Bertram, C., Dietrich, J.-P., Luderer, G., Pehl, M., Pietzcker, R., Piontek, F., Lotze-Campen, H., Biewald, A., Bonsch, M., Giannousakis, A., Kreidenweis, U., Müller, C., Rolinski, S., Schultes, A., Schwanitz, J., Stevanovic, M., Calvin, K., Emmerling, J., Fujimori, S., and Edenhofer, O.: Fossil-fueled development (SSP5): An energy and resource intensive scenario for the 21st century, Global Environ. Chang., 42, 297–315, https://doi.org/10.1016/j.gloenvcha.2016.05.015, 2017.
Lawrence, D. M., Hurtt, G. C., Arneth, A., Brovkin, V., Calvin, K. V., Jones, A. D., Jones, C. D., Lawrence, P. J., de Noblet-Ducoudré, N., Pongratz, J., Seneviratne, S. I., and Shevliakova, E.: The Land Use Model Intercomparison Project (LUMIP) contribution to CMIP6: rationale and experimental design, Geosci. Model Dev., 9, 2973–2998, https://doi.org/10.5194/gmd-9-2973-2016, 2016.
Leadley, P. W., Krug, C. B., Alkemade, R., Pereira, H. M., Sumaila U. R., Walpole, M., Marques, A., Newbold, T., Teh, L. S. L., van Kolck, J., Bellard, C., Januchowski-Hartley, S. R., and Mumby, P. J.: Progress towards the Aichi Biodiversity Targets: An Assessment of Biodiversity Trends, Policy Scenarios and Key Actions, Secretariat of the Convention on Biological Diversity, Montreal, Canada, Technical Series 78, 500 pp., 2014.
Lehsten, V., Sykes, M. T., Scott, A. V., Tzanopoulos, J., Kallimanis, A., Mazaris, A., Verburg, P. H., Schulp, C. J. E., Potts, S. G., and Vogiatzakis, I.: Disentangling the effects of land-use change, climate and CO2 on projected future European habitat types: Disentangling the drivers of habitat change, Global Ecol. Biogeogr., 24, 653–663, https://doi.org/10.1111/geb.12291, 2015.
Lindeskog, M., Arneth, A., Bondeau, A., Waha, K., Seaquist, J., Olin, S., and Smith, B.: Implications of accounting for land use in simulations of ecosystem carbon cycling in Africa, Earth Syst. Dynam., 4, 385–407, https://doi.org/10.5194/esd-4-385-2013, 2013.
Martins, I. S. and Pereira, H. M.: Improving extinction projections across scales and habitats using the countryside species-area relationship, Sci. Rep.-UK, 7, 12899, https://doi.org/10.1038/s41598-017-13059-y, 2017.
Maxwell, S. L., Fuller, R. A., Brooks, T. M., and Watson, J. E. M.: Biodiversity: The ravages of guns, nets and bulldozers, Nature, 536, 143–145, https://doi.org/10.1038/536143a, 2016.
McSweeney, C. F. and Jones, R. G.: How representative is the spread of climate projections from the 5 CMIP5 GCMs used in ISI-MIP?, Clim. Serv., 1, 24–29, https://doi.org/10.1016/j.cliser.2016.02.001, 2016.
Meinshausen, M., Wigley, T. M. L., and Raper, S. C. B.: Emulating atmosphere-ocean and carbon cycle models with a simpler model, MAGICC6 – Part 2: Applications, Atmos. Chem. Phys., 11, 1457–1471, https://doi.org/10.5194/acp-11-1457-2011, 2011a.
Meinshausen, M., Raper, S. C. B., and Wigley, T. M. L.: Emulating coupled atmosphere-ocean and carbon cycle models with a simpler model, MAGICC6 – Part 1: Model description and calibration, Atmos. Chem. Phys., 11, 1417–1456, https://doi.org/10.5194/acp-11-1417-2011, 2011b.
Merow, C., Smith, M. J., and Silander, J. A.: A practical guide to MaxEnt for modeling species' distributions: what it does, and why inputs and settings matter, Ecography, 36, 1058–1069, https://doi.org/10.1111/j.1600-0587.2013.07872.x, 2013.
Millennium Ecosystem Assessment (Program) (Ed.): Ecosystems and human well-being: synthesis, Island Press, Washington, DC, 2005.
Monfreda, C., Ramankutty, N., and Foley, J. A.: Farming the planet: 2. Geographic distribution of crop areas, yields, physiological types, and net primary production in the year 2000: Global crop areas and yields in 2000, Global Biogeochem. Cy., 22, GB1022, https://doi.org/10.1029/2007GB002947, 2008.
Moss, R. H., Edmonds, J. A., Hibbard, K. A., Manning, M. R., Rose, S. K., van Vuuren, D. P., Carter, T. R., Emori, S., Kainuma, M., Kram, T., Meehl, G. A., Mitchell, J. F. B., Nakicenovic, N., Riahi, K., Smith, S. J., Stouffer, R. J., Thomson, A. M., Weyant, J. P., and Wilbanks, T. J.: The next generation of scenarios for climate change research and assessment, Nature, 463, 747–756, https://doi.org/10.1038/nature08823, 2010.
Newbold, T., Hudson, L. N., Arnell, A. P., Contu, S., De Palma, A., Ferrier, S., Hill, S. L. L., Hoskins, A. J., Lysenko, I., Phillips, H. R. P., Burton, V. J., Chng, C. W. T., Emerson, S., Gao, D., Pask-Hale, G., Hutton, J., Jung, M., Sanchez-Ortiz, K., Simmons, B. I., Whitmee, S., Zhang, H., Scharlemann, J. P. W., and Purvis, A.: Has land use pushed terrestrial biodiversity beyond the planetary boundary? A global assessment, Science, 353, 288–291, https://doi.org/10.1126/science.aaf2201, 2016.
Ohashi, H., Hasegawa, T., Hirata, A., Fujimori, S., Takahashi, K., Tsuyama, I., Nakao, K., Kominami, Y., Tanaka, N., Hijioka, Y., and Matsui, T.: Biodiversity can benefit from long-term climate mitigation regardless of land-based measures, submitted, 2018.
Olin, S., Schurgers, G., Lindeskog, M., Wårlind, D., Smith, B., Bodin, P., Holmér, J., and Arneth, A.: Modelling the response of yields and tissue C : N to changes in atmospheric CO2 and N management in the main wheat regions of western Europe, Biogeosciences, 12, 2489–2515, https://doi.org/10.5194/bg-12-2489-2015, 2015.
O'Neill, B. C., Kriegler, E., Riahi, K., Ebi, K. L., Hallegatte, S., Carter, T. R., Mathur, R. and van Vuuren, D. P.: A new scenario framework for climate change research: the concept of shared socioeconomic pathways, Climatic Change, 122, 387–400, https://doi.org/10.1007/s10584-013-0905-2, 2014.
O'Neill, B. C., Tebaldi, C., van Vuuren, D. P., Eyring, V., Friedlingstein, P., Hurtt, G., Knutti, R., Kriegler, E., Lamarque, J.-F., Lowe, J., Meehl, G. A., Moss, R., Riahi, K., and Sanderson, B. M.: The Scenario Model Intercomparison Project (ScenarioMIP) for CMIP6, Geosci. Model Dev., 9, 3461–3482, https://doi.org/10.5194/gmd-9-3461-2016, 2016.
O'Neill, B. C., Kriegler, E., Ebi, K. L., Kemp-Benedict, E., Riahi, K., Rothman, D. S., van Ruijven, B. J., van Vuuren, D. P., Birkmann, J., Kok, K., Levy, M., and Solecki, W.: The roads ahead: Narratives for shared socioeconomic pathways describing world futures in the 21st century, Global Environ. Chang., 42, 169–180, https://doi.org/10.1016/j.gloenvcha.2015.01.004, 2017.
Pecl, G. T., Araújo, M. B., Bell, J. D., Blanchard, J., Bonebrake, T. C., Chen, I.-C., Clark, T. D., Colwell, R. K., Danielsen, F., Evengård, B., Falconi, L., Ferrier, S., Frusher, S., Garcia, R. A., Griffis, R. B., Hobday, A. J., Janion-Scheepers, C., Jarzyna, M. A., Jennings, S., Lenoir, J., Linnetved, H. I., Martin, V. Y., McCormack, P. C., McDonald, J., Mitchell, N. J., Mustonen, T., Pandolfi, J. M., Pettorelli, N., Popova, E., Robinson, S. A., Scheffers, B. R., Shaw, J. D., Sorte, C. J. B., Strugnell, J. M., Sunday, J. M., Tuanmu, M.-N., Vergés, A., Villanueva, C., Wernberg, T., Wapstra, E., and Williams, S. E.: Biodiversity redistribution under climate change: Impacts on ecosystems and human well-being, Science, 355, eaai9214, https://doi.org/10.1126/science.aai9214, 2017.
Pereira, H. M., Leadley, P. W., Proenca, V., Alkemade, R., Scharlemann, J. P. W., Fernandez-Manjarres, J. F., Araujo, M. B., Balvanera, P., Biggs, R., Cheung, W. W. L., Chini, L., Cooper, H. D., Gilman, E. L., Guenette, S., Hurtt, G. C., Huntington, H. P., Mace, G. M., Oberdorff, T., Revenga, C., Rodrigues, P., Scholes, R. J., Sumaila, U. R., and Walpole, M.: Scenarios for Global Biodiversity in the 21st Century, Science, 330, 1496–1501, https://doi.org/10.1126/science.1196624, 2010.
Popp, A., Calvin, K., Fujimori, S., Havlik, P., Humpenöder, F., Stehfest, E., Bodirsky, B. L., Dietrich, J. P., Doelmann, J. C., Gusti, M., Hasegawa, T., Kyle, P., Obersteiner, M., Tabeau, A., Takahashi, K., Valin, H., Waldhoff, S., Weindl, I., Wise, M., Kriegler, E., Lotze-Campen, H., Fricko, O., Riahi, K., and Vuuren, D. P. van: Land-use futures in the shared socio-economic pathways, Global Environ. Chang., 42, 331–345, https://doi.org/10.1016/j.gloenvcha.2016.10.002, 2017.
Poulter, B., Frank, D. C., Hodson, E. L., and Zimmermann, N. E.: Impacts of land cover and climate data selection on understanding terrestrial carbon dynamics and the CO2 airborne fraction, Biogeosciences, 8, 2027–2036, https://doi.org/10.5194/bg-8-2027-2011, 2011.
Prentice, I. C., Bondeau, A., Cramer, W., Harrison, S. P., Hickler, T., Lucht, W., Sitch, S., Smith, B., Sykes, M. T., Canadell, J. G., Pataki, D. E., and Pitelka, L. F. (Eds.): Dynamic Global Vegetation Modeling: Quantifying Terrestrial Ecosystem Responses to Large-Scale Environmental Change, in Terrestrial Ecosystems in a Changing World, Springer Berlin Heidelberg, Berlin, Heidelberg, 175–192, 2007.
Purvis, A., Newbold, T., De Palma, A., Contu, S., Hill, S. L. L., Sanchez-Ortiz, K., Phillips, H. R. P., Hudson, L. N., Lysenko, I., Börger, L., and Scharlemann, J. P. W.: Modelling and Projecting the Response of Local Terrestrial Biodiversity Worldwide to Land Use and Related Pressures: The PREDICTS Project, in: Advances in Ecological Research, Elsevier, vol. 58, 201–241, 2018.
Rabin, S. S., Melton, J. R., Lasslop, G., Bachelet, D., Forrest, M., Hantson, S., Kaplan, J. O., Li, F., Mangeon, S., Ward, D. S., Yue, C., Arora, V. K., Hickler, T., Kloster, S., Knorr, W., Nieradzik, L., Spessa, A., Folberth, G. A., Sheehan, T., Voulgarakis, A., Kelley, D. I., Prentice, I. C., Sitch, S., Harrison, S., and Arneth, A.: The Fire Modeling Intercomparison Project (FireMIP), phase 1: experimental and analytical protocols with detailed model descriptions, Geosci. Model Dev., 10, 1175–1197, https://doi.org/10.5194/gmd-10-1175-2017, 2017.
Redhead, J. W., May, L., Oliver, T. H., Hamel, P., Sharp, R., and Bullock, J. M.: National scale evaluation of the InVEST nutrient retention model in the United Kingdom, Sci. Total Environ., 610–611, 666–677, https://doi.org/10.1016/j.scitotenv.2017.08.092, 2018.
Riahi, K., van Vuuren, D. P., Kriegler, E., Edmonds, J., O'Neill, B. C., Fujimori, S., Bauer, N., Calvin, K., Dellink, R., Fricko, O., Lutz, W., Popp, A., Cuaresma, J. C., Kc, S., Leimbach, M., Jiang, L., Kram, T., Rao, S., Emmerling, J., Ebi, K., Hasegawa, T., Havlik, P., Humpenöder, F., Da Silva, L. A., Smith, S., Stehfest, E., Bosetti, V., Eom, J., Gernaat, D., Masui, T., Rogelj, J., Strefler, J., Drouet, L., Krey, V., Luderer, G., Harmsen, M., Takahashi, K., Baumstark, L., Doelman, J. C., Kainuma, M., Klimont, Z., Marangoni, G., Lotze-Campen, H., Obersteiner, M., Tabeau, A., and Tavoni, M.: The Shared Socioeconomic Pathways and their energy, land use, and greenhouse gas emissions implications: An overview, Global Environ. Chang., 42, 153–168, https://doi.org/10.1016/j.gloenvcha.2016.05.009, 2017.
Rondinini, C., Di Marco, M., Chiozza, F., Santulli, G., Baisero, D., Visconti, P., Hoffmann, M., Schipper, J., Stuart, S. N., Tognelli, M. F., Amori, G., Falcucci, A., Maiorano, L., and Boitani, L.: Global habitat suitability models of terrestrial mammals, Philos. T. Roy. Soc. B Biol., 366, 2633–2641, https://doi.org/10.1098/rstb.2011.0113, 2011.
Rosa, I. M. D., Pereira, H. M., Ferrier, S., Alkemade, R., Acosta, L. A., Akcakaya, R., den Belder, E., Fazel, A. M., Fujimori, S., Harfoot, M., Harhash, K. A., Harrison, P. A., Hauck, J., Hendriks, R. J. J., Hernández, G., Jetz, W., Karlsson-Vinkhuyzen, S. I., Kim, H. J., King, N., Kok, M. T. J., Kolomytsev, G. O., Lazarova, T., Leadley, P., Lundquist, C. J., García Márquez, J., Meyer, C., Navarro, L. M., Nesshöver, C., Ngo, H. T., Ninan, K. N., Palomo, M. G., Pereira, L. M., Peterson, G. D., Pichs, R., Popp, A., Purvis, A., Ravera, F., Rondinini, C., Sathyapalan, J., Schipper, A. M., Seppelt, R., Settele, J., Sitas, N., and van Vuuren, D.: Multiscale scenarios for nature futures, Nat. Ecol. Evol., 1, 1416–1419, 2017.
Rosenzweig, C., Arnell, N. W., Ebi, K. L., Lotze-Campen, H., Raes, F., Rapley, C., Smith, M. S., Cramer, W., Frieler, K., Reyer, C. P. O., Schewe, J., van Vuuren, D., and Warszawski, L.: Assessing inter-sectoral climate change risks: the role of ISIMIP, Environ. Res. Lett., 12, 010301, https://doi.org/10.1088/1748-9326/12/1/010301, 2017.
Sala, O. E.: Global Biodiversity Scenarios for the Year 2100, Science, 287, 1770–1774, https://doi.org/10.1126/science.287.5459.1770, 2000.
Schipper, A. M., Bakkenes, M., Meijer, J. R., Alkemade, R., and Huijbregts, M. J.: The GLOBIO model. A technical description of version 3.5. PBL publication 2369, The Hague, PBL Netherlands Environmental Assessment Agency, 2016.
Schulp, C. J. E., Alkemade, R., Klein Goldewijk, K., and Petz, K.: Mapping ecosystem functions and services in Eastern Europe using global-scale data sets, Int. J. Biodivers. Sci. Ecosyst. Serv. Manag., 8, 156–168, https://doi.org/10.1080/21513732.2011.645880, 2012.
Secretariat of the Convention on Biological Diversity and United Nations Environment Programme (Eds.): Global biodiversity outlook 4: a mid-term assessment of progress towards the implementation of the strategic plan for biodiversity 2011–2020, Secretariat for the Convention on Biological Diversity, Montreal, Quebec, Canada, 2014.
Settele, J., Scholes, R., Betts, R. A., Bunn, S., Leadley, P., Nepstad, D., Overpeck, J. T., Taboada, M. A., Fischlin, A., Moreno, J. M., Root, T., Musche, M., and Winter, M.: Terrestrial and Inland water systems, in: Climate Change 2014 Impacts, Adaptation and Vulnerability: Part A: Global and Sectoral Aspects, Cambridge University Press, 271–360, https://doi.org/10.1017/CBO9781107415379.009, 2015.
Sharp, R., Tallis, H. T., Ricketts, T., Guerry, A. D., Wood, S. A., Chaplin-Kramer, R., Nelson, E., Ennaanay, D., Wolny, S., Olwero, N., Vigerstol, K., Pennington, D., Mendoza, G., Aukema, J., Foster, J., Forrest, J., Cameron, D., Arkema, K., Lonsdorf, E., Kennedy, C., Verutes, G., Kim, C. K., Guannel, G., Papenfus, M., Toft, J., Marsik, M., Bernhardt, J., Griffin, R., Glowinski, K., Chaumont, N., Perelman, A., Lacayo, M., Mandle, L., Hamel, P., Vogl, A. L., Rogers, L., Bierbower, W., Denu, D., and Douglass, J.: InVEST +VERSION+ User's Guide, The Natural Capital Project, Stanford University, University of Minnesota, The Nature Conservancy, and World Wildlife Fund, 2016.
Sitch, S., Smith, B., Prentice, I. C., Arneth, A., Bondeau, A., Cramer, W., Kaplan, J. O., Levis, S., Lucht, W., Sykes, M. T., Thonicke, K., and Venevsky, S.: Evaluation of ecosystem dynamics, plant geography and terrestrial carbon cycling in the LPJ dynamic global vegetation model, Glob. Change Biol., 9, 161–185, https://doi.org/10.1046/j.1365-2486.2003.00569.x, 2003.
Smith, B., Wårlind, D., Arneth, A., Hickler, T., Leadley, P., Siltberg, J., and Zaehle, S.: Implications of incorporating N cycling and N limitations on primary production in an individual-based dynamic vegetation model, Biogeosciences, 11, 2027–2054, https://doi.org/10.5194/bg-11-2027-2014, 2014.
Stehfest, E., van Vuuren, D., Kram, T., Bouwman, L., Alkemade, R., Bakkenes, M., Biemans, H., Bouwman, A., den Elzen, M., Janse, J., Lucas, P., van Minnen, J., Müller, M., and Prins, A.: Integrated Assessment of Global Environmental Change with IMAGE 3.0. Model description and policy applications, The Hague, PBL Netherlands Environmental Assessment Agency, 2014.
Thuiller, W.: Patterns and uncertainties of species' range shifts under climate change, Glob. Change Biol., 10, 2020–2027, https://doi.org/10.1111/j.1365-2486.2004.00859.x, 2004.
Thuiller, W., Lafourcade, B., Engler, R., and Araújo, M. B.: BIOMOD – a platform for ensemble forecasting of species distributions, Ecography, 32, 369–373, https://doi.org/10.1111/j.1600-0587.2008.05742.x, 2009.
Thuiller, W., Lavergne, S., Roquet, C., Boulangeat, I., Lafourcade, B., and Araujo, M. B.: Consequences of climate change on the tree of life in Europe, Nature, 470, 531–534, https://doi.org/10.1038/nature09705, 2011.
Thuiller, W., Münkemüller, T., Lavergne, S., Mouillot, D., Mouquet, N., Schiffers, K., Gravel, D., and Holyoak, M. (Eds.): A road map for integrating eco-evolutionary processes into biodiversity models, Ecol. Lett., 16, 94–105, https://doi.org/10.1111/ele.12104, 2013.
Titeux, N., Henle, K., Mihoub, J.-B., Regos, A., Geijzendorffer, I. R., Cramer, W., Verburg, P. H., and Brotons, L.: Biodiversity scenarios neglect future land-use changes, Glob. Change Biol., 22, 2505–2515, https://doi.org/10.1111/gcb.13272, 2016.
Titeux, N., Henle, K., Mihoub, J.-B., Regos, A., Geijzendorffer, I. R., Cramer, W., Verburg, P. H., Brotons, L., and Syphard, A. (Eds.): Global scenarios for biodiversity need to better integrate climate and land use change, Divers. Distrib., 23, 1231–1234, https://doi.org/10.1111/ddi.12624, 2017.
United Nations Environment Programme (UNEP): UNEP-SETAC Life Cycle Initiative: Global Guidance for Life Cycle Impact Assessment Indicators – Volume 1, 2016.
United Nations Environment Programme–World Conservation Monitoring Centre: Dataset combining Exclusive Economic Zones (EEZ, VLIZ 2014) and terrestrial country boundaries (World Vector Shoreline, 3rd Edn., National Geospatial-Intelligence Agency), Cambridge (UK), UNEP World Conservation Monitoring Centre, 2015.
van Beek, L. P. H., Wada, Y., and Bierkens, M. F. P.: Global monthly water stress: 1. Water balance and water availability: Global Monthly Water Stress, 1, Water Resour. Res., 47, W07517, https://doi.org/10.1029/2010WR009791, 2011.
van Vuuren, D. P., Edmonds, J., Kainuma, M., Riahi, K., Thomson, A., Hibbard, K., Hurtt, G. C., Kram, T., Krey, V., Lamarque, J.-F., Masui, T., Meinshausen, M., Nakicenovic, N., Smith, S. J., and Rose, S. K.: The representative concentration pathways: an overview, Climatic Change, 109, 5–31, https://doi.org/10.1007/s10584-011-0148-z, 2011.
van Vuuren, D. P., Kriegler, E., O'Neill, B. C., Ebi, K. L., Riahi, K., Carter, T. R., Edmonds, J., Hallegatte, S., Kram, T., Mathur, R., and Winkler, H.: A new scenario framework for Climate Change Research: scenario matrix architecture, Climatic Change, 122, 373–386, https://doi.org/10.1007/s10584-013-0906-1, 2014.
van Vuuren, D. P., Stehfest, E., Gernaat, D. E. H. J., Doelman, J. C., van den Berg, M., Harmsen, M., de Boer, H. S., Bouwman, L. F., Daioglou, V., Edelenbosch, O. Y., Girod, B., Kram, T., Lassaletta, L., Lucas, P. L., van Meijl, H., Müller, C., van Ruijven, B. J., van der Sluis, S., and Tabeau, A.: Energy, land-use and greenhouse gas emissions trajectories under a green growth paradigm, Global Environ. Chang., 42, 237–250, https://doi.org/10.1016/j.gloenvcha.2016.05.008, 2017.
Visconti, P., Bakkenes, M., Baisero, D., Brooks, T., Butchart, S. H. M., Joppa, L., Alkemade, R., Di Marco, M., Santini, L., Hoffmann, M., Maiorano, L., Pressey, R. L., Arponen, A., Boitani, L., Reside, A. E., van Vuuren, D. P., and Rondinini, C.: Projecting Global Biodiversity Indicators under Future Development Scenarios: Projecting biodiversity indicators, Conserv. Lett., 9, 5–13, https://doi.org/10.1111/conl.12159, 2016.
Warszawski, L., Frieler, K., Huber, V., Piontek, F., Serdeczny, O., and Schewe, J.: The Inter-Sectoral Impact Model Intercomparison Project (ISI–MIP): Project framework, P. Natl. Acad. Sci. USA, 111, 3228–3232, https://doi.org/10.1073/pnas.1312330110, 2014.
Welbergen, J. A., Klose, S. M., Markus, N., and Eby, P.: Climate change and the effects of temperature extremes on Australian flying-foxes, Philos. T. Roy. Soc. B Biol., 275, 419–425, https://doi.org/10.1098/rspb.2007.1385, 2008.