the Creative Commons Attribution 4.0 License.
the Creative Commons Attribution 4.0 License.
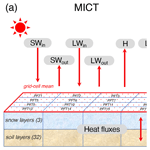
Assessment of a tiling energy budget approach in a land surface model, ORCHIDEE-MICT (r8205)
Chunjing Qiu
Yuan Zhang
Shushi Peng
Gustaf Hugelius
Jinfeng Chang
Elodie Salmon
Philippe Ciais
The surface energy budget plays a critical role in terrestrial hydrological and biogeochemical cycles. Nevertheless, its highly spatial heterogeneity across different vegetation types is still missing in the ORCHIDEE-MICT (ORganizing Carbon and Hydrology in Dynamic EcosystEms–aMeliorated Interactions between Carbon and Temperature) land surface model. In this study, we describe the representation of a tiling energy budget in ORCHIDEE-MICT and assess its short-term and long-term impacts on energy, hydrology, and carbon processes. With the specific values of surface properties for each vegetation type, the new version presents warmer surface and soil temperatures (∼ 0.5 °C, +3 %), wetter soil moisture (∼ 10 kg m−2, +2 %), and increased soil organic carbon storage (∼ 170 Pg C, +9 %) across the Northern Hemisphere. Despite reproducing the absolute values and spatial gradients of surface and soil temperatures from satellite and in situ observations, the considerable uncertainties in simulated soil organic carbon and hydrological processes prevent an obvious improvement in the temperature bias existing in the original ORCHIDEE-MICT model. However, the separation of sub-grid energy budgets in the new version improves permafrost simulation greatly by accounting for the presence of discontinuous permafrost types (∼ 3×106 km2), which will facilitate various permafrost-related studies in the future.
- Article
(19872 KB) - Full-text XML
-
Supplement
(24234 KB) - BibTeX
- EndNote
The surface energy balance is a fundamental component of the Earth system. The incoming solar energy is not only essential for life and plant photosynthesis but also drives the terrestrial hydrological cycle (i.e., evapotranspiration and the freeze–thaw cycle of soils in cold regions) and modulates the speed of biogeochemical reactions (i.e., the decomposition of organic matter). The energy balance depends on the landscape type through distinct vegetation and soil elements that reflect and emit shortwave and longwave electromagnetic radiation in different proportions. Understanding and simulating the complex interactions of energy, hydrology, and biogeochemical processes throughout the Earth system is crucial for tracking the consequences of historical human activities and predicting the future of our Earth's climate system.
Employing land surface models (LSMs) or Earth system models (ESMs) is one of the most common approaches to simulate the surface energy budget and investigate its interactions with hydrological, atmospheric, and biogeochemical processes. The typical spatial resolution of LSMs varies from 0.5° × 0.5° (∼ 50 km × 50 km at the Equator) to 2° × 2° (∼ 200 km × 200 km at the Equator). Significant surface heterogeneity would undoubtedly exist on such large scales. Taking surface temperature (Tsurf) as an example, in reality, two adjacent landscapes could have significantly different Tsurf values due to their distinct surface properties, including surface albedo, leaf area index, rooting depth, and vegetation height, at a scale not resolved by the models. For instance, the larger latent heat loss via evapotranspiration over deep-rooted tropical forests compared with nearby grassland and cropland shows a significant cooling effect, approximately 2.5 °C on a daily basis (Li et al., 2015). The higher albedo across snow-covered areas for short vegetation compared with nearby forest results in a reduction in the absorbed solar energy and a lower Tsurf, with a magnitude depending on the timing and duration of snow cover (Zhang, 2005). To represent the heterogeneous surface energy balance, some LSMs/ESMs have introduced tiling energy budgets, such as the plant-functional-type-specific energy budgets in CLASSIC (Canadian Land Surface Scheme Including Biogeochemical Cycles; Melton and Arora, 2014); the separate energy budgets for snow, soil, and vegetation in ISBA (Soil–Biosphere–Atmosphere LSM; Boone et al., 2017); the partitioning of snow-covered and snow-free land units in CLM 5.0 (Community Land Model; Lawrence et al., 2019); and the sub-grid topographic effects on solar radiation flux in ELM (the Energy Exascale Earth System Model (E3SM) Land Model; Hao et al., 2021). Moreover, three land surface schemes (LSSs) have been adopted to represent the tiling energy budgets: mosaic (the use of specific surface properties for each land cover type), mixed (the grouping of certain land cover types with similar surface properties and the consequent use of a smaller number of distinct surface types), and composite (the use of the average properties of one grid cell) (Melton and Arora, 2014; Rumbold et al., 2023). Through a comparison of the mosaic and the composite LSSs, the CLASSIC model reported a less than 5 % difference in the primary energy fluxes but an up to 46 % difference in carbon fluxes and the carbon pool size at the site level (Li and Arora, 2012); the aforementioned model also found a 19 % higher terrestrial carbon sink for 1959–2005 in the mosaic simulation (Melton and Arora, 2014). Moreover, using the JULES (Joint UK Land Environment Simulator) model, Rumbold et al. (2023) found that the tiling soil scheme does have an impact on the water and energy budgets due to the way vegetation accesses soil moisture. Furthermore, Qin et al. (2023) reported that the tiling CLM model provides more accurate simulations of surface air temperature and precipitation than the single-land-cover version when coupled with the WRF (Weather Research and Forecasting) model, as validated against in situ observations. Despite uncertainties in model-specific structures and configurations, these findings highlight the importance of explicitly representing sub-grid surface heterogeneity in current LSMs.
Besides the necessity to represent surface heterogeneity, the incorporation of new landforms and processes also requires the tiling of energy budgets. For instance, Rooney and Jones (2010) identified the challenges involved in simulating soil temperature under lakes when introducing lakes into the single-soil-tile JULES model, as they have different thermal transfer characteristics due to the higher specific heat capacity of water than adjacent land tiles. When evaluating the impacts of sub-grid-scale disturbances, such as fires and harvest, Curasi et al. (2023) found that the impact of sub-grid heterogeneity is 1.5–4 times the impact of the disturbances themselves on the carbon cycle with the CLASSIC model. Moreover, it is necessary to provide the independent energy budgets, hydrology, and carbon cycles when incorporating a series of new processes for permafrost regions, such as discontinuous permafrost (Smith et al., 2022), melting of ground ice (Rumbold et al., 2023), thermokarst thawing, and lateral drainage (Nitzbon et al., 2020), in LSMs.
To enable the representation of surface heterogeneity and open the door to a series of new landforms and processes, we implement tiling energy budgets at the surface and subsurface for each plant functional type (PFT) in a state-of-the-art LSM, ORCHIDEE-MICT (ORganizing Carbon and Hydrology in Dynamic EcosystEms–aMeliorated Interactions between Carbon and Temperature), which calculates turbulent fluxes for sub-grid PFTs but solves for an average grid-level energy budget, resulting in a single surface temperature (Guimberteau et al., 2018). Some hydrological and biogeochemical processes in the model have been modified correspondingly to include PFT-specific thermal inputs. In Sect. 2, we provide a brief review of the current grid cell mean energy budget at the surface and subsurface, while Sect. 3 describes the modifications made to implement the tiling energy budgets in the model. To evaluate the impacts of separating the energy budget for each PFT on the energy, hydrology, soil thermodynamics, and carbon cycles, we conduct simulations using the original version of ORCHIDEE-MICT (referred to as MICT) and the new tiling energy budget version (referred to as MICT-teb), as described in Sect. 4. The results of these simulations are compared in Sect. 5. Section 6 focuses on the evaluation of energy processes in the new version as well as the improvements for permafrost simulations. Finally, we present conclusions in Sect. 7.
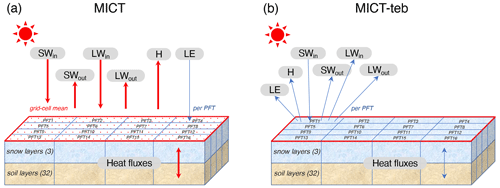
Figure 1Schematic representation of energy budgets at the surface, snow layers, and soil layers in one grid cell of ORCHIDEE-MICT (MICT) (a) and the new tiling energy budget version (MICT-teb) (b). SWin, SWout, LWin, LWout, H, and LE represent incoming shortwave radiation, outward shortwave radiation, incoming longwave radiation, outward longwave radiation, sensible heat flux, and latent heat flux, respectively. PFT indicates the plant functional type. There are 3 layers for snow and 32 layers for soil for each PFT in the model. In MICT, SWin, SWout, LWin, LWout, H, and heat fluxes in the snow and soil layers are calculated as a grid cell mean, but LE is calculated for each PFT; in MICT-teb, all of the heat fluxes are calculated for each PFT. The red and blue arrows denote the grid cell mean and the PFT-specific calculations, respectively.
ORCHIDEE-MICT is a branch of the ORCHIDEE model (Krinner et al., 2005) that has been specifically developed to enhance the representation of hydrological and biogeochemical interactions in high-latitude regions (Guimberteau et al., 2018). In comparison with the trunk version 3976 from which it was developed, ORCHIDEE-MICT includes several key new processes: (1) the feedback effects of the soil organic carbon (SOC) concentration on soil thermal and soil water dynamics (Zhu et al., 2019); (2) soil carbon vertical discretization (Koven et al., 2009; Zhu et al., 2016); (3) vertical mixing of soil carbon due to cryoturbation (in cold soils) and bioturbation (Koven et al., 2009, 2013); (4) reformulation of soil hydric stress above the permafrost table (Zhu et al., 2015); and (5) the inclusion of northern peatlands as a specific PFT with peatland-specific hydrology, carbon decomposition, and accumulation (Qiu et al., 2018, 2019). These new processes have significantly improved the representation of plant productivity, the water cycle, soil carbon stocks, and the simulated permafrost distribution in high-latitude regions, but there is still room for improvement (Guimberteau et al., 2018). One important aspect that calls for attention is the need to include sub-grid representations of surface and subsurface energy budgets, especially at high latitudes where the snow cover and water equivalent differ between PFTs and where soil carbon differences across landscape elements/PFTs within the same grid cell result in a heterogeneous soil temperature and active layer thickness. For the moment, within the ORCHIDEE-MICT model, there are three major modules: carbon, water, and energy. The carbon cycle module operates for each PFT sub-grid, the water cycle operates for each soil tile (divided into bare soil, tree PFTs, grass and crop PFTs, and peatland PFT categories), and the energy module is solved only at the total grid cell level (Best et al., 2004). Figure 1 shows the schematic representation of the energy budgets from the surface to snow and soil layers in the original and new versions of ORCHIDEE-MICT, namely MICT and MICT-teb, respectively. The details of the energy budget in the model are outlined in the following.
2.1 Surface energy budgets
Similar to most LSMs, the surface energy budget equation is used in MICT to describe the balance of net absorbed radiation (Rnet) by the energy transferred out of the ecosystem:
where H is the sensible heat flux (W m−2), LE is the latent heat flux (W m−2), G is the ground heat flux (W m−2), and ΔS is the energy stored in the ecosystem as chemical energy through photosynthesis and as the temperature change in the plant biomass (W m−2). Due to the ΔS only accounting for < 5 % of the total energy budget of the ecosystem in most areas (Georg et al., 2016), it is neglected in the model. Rnet is the balance of the inputs and outputs of shortwave radiation (SWin and SWout) and longwave radiation (LWin and LWout):
where α is the albedo, which determines the proportion of incoming SW absorbed by the ecosystem (unitless); σ is the Stefan–Boltzmann constant (5.6697 × 10−8 ); ε is the emissivity (1, unitless); and Tsurf is the surface temperature (K). In MICT, the grid cell α is calculated as the area-weighted average of the α values across all PFTs. The snow-covered areas and snow-free areas are distinguished in terms of the higher albedo of snow than canopy and bare soil (see Sect. 2.3 for details on the calculation of albedo for snow-covered regions). The albedo of bare soil in the current version is prescribed using static satellite observations (Lurton et al., 2020), with the background albedo extracted using the Joint Research Centre Two-stream Inversion Package (Pinty et al., 2011); thus, it is calculated without interannual variations and decoupled from simulated soil moisture (Fig. S1 in the Supplement). The albedo of vegetated PFTs is prescribed with constant values in the model (Table S1 in the Supplement). As MICT is not capable of calculating leaves' energy budgets separately from those of the soil and stem, there is no vertically layered temperature from the ground surface to the top of the canopy; thus, the Tsurf is used to calculate all surface energy fluxes here. The two heat fluxes out of the surface, i.e., H and LE, are calculated following Eqs. (3) and (4), respectively:
where ρ is air density (kg m−3); v is the horizontal wind speed (m s−1); Cd is the drag coefficient (unitless); cp is the specific heat capacity of dry air (1004.675 ); Tair is the air temperature (K); L is the latent heat of evaporation or sublimation (2.5008 or 2.8345 × 106 J kg−1); β is the limiting factor of potential total evapotranspiration (PET) (unitless); and qsurf and qair are the saturated moisture at the surface and in the air (kg kg−1), respectively. Due to the differences in the canopy height and leaf area index (LAI), Cd should be different among different PFTs. To ensure compatibility with the grid cell calculation of the surface energy budget, the Cd of different PFTs is weighted by their area. The LE (or ET) serves as the link between the energy cycle and the hydrological and carbon cycle. It is PFT-specific via a PFT-specific β (see details in Ducharne, 2018) because the carbon cycle and hydrological processes separate different PFTs or different soil tiles. In MICT, the LE consists of Eflood (flood evaporation, not activated in the simulations of this study), Esubli (snow sublimation), Esoil (evaporation from bare soil), Etrans (transpiration), and Einter (interception). Due to the distinct plant structures between different PFTs, the evaporation components associated with vegetation, i.e., Etrans and Einter are PFT-specific, whereas the Esubli and Esoil are calculated as the grid cell average. For G, the energy exchange between the surface and ground (snow or soil depending on the snow cover fraction) is calculated following the classic Fourier law (Hourdin, 1992). Considering that the calculation of G is identical to heat conduction in soil layers, the detailed derivation is only shown in Sect. 2.2 to avoid redundancy. For the areas covered by snow, G also considers heat fluxes into the snowpack which are used to melt snow.
2.2 Soil energy budgets
Table S2 in the Supplement displays the vertical discretization of soil in the current version of MICT. There are 32 soil layers for soil heat conduction with a total depth of 38 m and 11 soil layers for soil hydrology with a total depth of 2 m. These soil layers are located below the three snow layers in the model when there is snow (Fig. 1a). As mentioned earlier, the heat conduction across soil layers, snow layers, and between the surface and ground is calculated using the classic one-dimensional Fourier law (Hourdin, 1992), with the latent flux of soil freezing taken into consideration (Gouttevin et al., 2012):
where c is the volumetric soil heat capacity (), Tsoil is the soil temperature (K), λ is the soil thermal conductivity (), ρice is the ice density (920 kg m−3), L is the latent heat of fusion (0.3336 × 106 J kg−1), θice is the volumetric ice content (m3 m−3), t is time (s), and z is the soil layer depth (m). The c is calculated as the area-weighted sum of the heat capacity of liquid soil moisture (4.18 × 106 ), frozen soil moisture (2.11 × 106 ), and soil (depending on the soil type and the SOC content). The λ is calculated as follows:
where
Here, Ke is the Kersten number, calculated using a function of the degree of soil moisture saturation; λsat and λdry are the saturated and dry thermal conductivities (), respectively; θsat is saturated soil moisture, depending on the soil type and the SOC content (m3 m−3); θliq is the volumetric liquid water content (m3 m−3); λsolid is the thermal conductivity of soil solid material, calculated as the geometric mean conductivities of mineral soil and SOC; and λliq and λice are the thermal conductivities of water (0.57 ) and ice (2.2 ), respectively. The two thermal parameters are calculated for each soil layer because the heat is transferred vertically across all soil layers. The input liquid or frozen soil moisture (SM) are calculated as the area-weighted sum of all soil tiles to maintain consistency with the grid cell energy budget, despite the fact that soil hydrology and soil carbon processes operate for each sub-grid element (soil tile for hydrology or PFT for carbon).
2.3 Snow energy budgets
An explicit snow model of intermediate complexity has been introduced in MICT, as described in Wang et al. (2013). The snowpack includes three snow layers (Fig. 1), with snow settling, water percolation, and refreezing and thawing of snow taken into account. When evaluated against observation data, the new snow module has improved the heat interaction between snow and the soil or ground surface (Wang et al., 2013). The heat conduction in snow layers uses the same one-dimensional heat diffusion function as that in soil layers (Eq. 5), while the snow's heat capacity (csnow) and thermal conductivity (λsnow) are calculated as follows:
Here, ρsnow is the snow density (kg m−3), which varies with snow settling; cp_ice is the heat capacity of ice (2.11 × 106 ); aλ = 0.02; bλ = 2.50 × 10−6; aλv = −0.06; bλv = −2.54; cλv = −289.99; P0 = 1000 hPa; Tsnow is the temperature of snow layers; and Pa is the atmospheric pressure (hPa). Besides the two thermal parameters, snow albedo (αsnow) is a key variable affecting the surface energy budget (Wang et al., 2013). The value of αsnow is calculated as follows:
where αsnow_min is the minimum snow albedo value after aging, k is the decay rate of snow albedo, agesnow is the snow age, and τ is the time constant of the decay of snow albedo (10 d) (Chalita and Le Treut, 1994). Although αsnow_min and k vary across different vegetation types (Table S3 in the Supplement), αsnow and all other snow-related processes including the heat conduction across the snow layers are still calculated at the grid cell scale by weighted area in MICT.
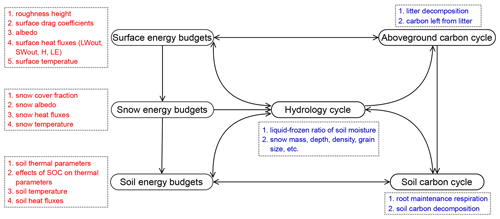
Figure 2Modifications of energy, hydrology, and carbon processes to implement tiling energy budgets in ORCHIDEE-MICT in this study. The black oblongs show the main modules in ORCHIDEE-MICT, and the dashed rectangles containing red or blue text show the processes that are modified to include the multi-tilling energy budget. Here, red and blue differentiate between the respective processes in which the PFT-specific calculations are added actively and those modified passively due to the input variables changing following the multi-tilling surface, snow, and soil thermodynamics.
To represent the sub-grid energy budget in MICT, we calculate PFT-specific surface properties, including the roughness height and the albedo of different PFTs, to start the separation of surface energy budgets for each PFT; we then add the PFT-specific calculation for energy budget at the surface as well as in the snow and soil layers (Figs. 1, 2). As we use distinct input variables from the energy budget module, some processes in the hydrological cycle and carbon cycle are also modified correspondingly. The details of all of the modifications are given in the following.
3.1 Surface energy budgets
Differences in surface properties serve as the foundation for distinct surface energy budgets across sub-grids. For example, variations in vegetation heights among tree, grass, and bare-soil land cover types result in different aerodynamic roughness, drag coefficients, and then turbulent fluxes, including H and LE (Eqs. 3, 4). Different surface types among tree, grass, and bare-soil land cover types also result in different surface albedo (α), influencing the amount of solar radiation reflected by the surface (Eq. 2). In MICT-teb, we employ the specific roughness height (Hrough, the vegetation height minus the zero-plane displacement height) and albedo for each PFT (Tables S1, S3, and S4 and Fig. S1 in the Supplement), instead of the average values of all PFTs within a grid cell used in MICT. Thus, if only considering the changes in surface properties, the more heterogeneous the sub-grid PFT distribution, the larger the possible differences in the energy and the subsequent hydrology and carbon-related processes between MICT-teb and MICT. The distinct surface properties among different PFTs propagate to differences in surface energy fluxes and then differentiate the Tsurf across different PFTs. In MICT-teb, all processes related to the changes in surface properties and Tsurf in the surface energy budget are separated and operate independently for each PFT (Fig. 2).
For LE (or ET), it comprises four components in the model: Esubli, Esoil, Etrans, and Einter. As described in Sect. 2.1, only Etrans and Einter are PFT-specific in the original MICT. However, due to the modifications made to surface energy budgets and surface properties, resulting in varying snow cover fractions across different PFTs, Esubli is now separated for each PFT in MICT-teb. Regarding Esoil, the water limitation in the hydrology module differs among different soil tiles, whereas it is considered at the grid scale only in MICT. The calculation of Esoil has been modified using the specific water limitation for each soil tile in MICT-teb. All PFTs in one soil tile are assigned the same value of Esoil and are then used in PFT-specific calculation in energy modules.
3.2 Snow energy budgets
The modifications to the surface energy budget in MICT-teb, which cause variations in heat fluxes into the snowpack, result in differences in the formation and melting of snow and, in turn, snow mass, snow depth (dzsnow), and snow density (ρsnow) among different PFTs. The snow cover fraction (fsnow), as calculated in MICT, is as follows (Niu and Yang, 2007; Wang et al., 2015):
This value would be different for different PFTs. In the above expression, i is the index of snow layer. The variations in fsnow would subsequently influence the surface albedo due to snow (Eq. 10) and, in turn, the snow feedbacks on the surface energy budget. All of the snow-related processes have been separated for each PFT in MICT-teb (Fig. 2).
3.3 Soil energy budgets
Following Eq. (5), modifications of energy budgets at the surface and in snow layers could result in variations in the starting point of heat conduction in soil for different PFTs. While within the soil, the heat conduction is more regulated by the heat capacity (c) and thermal conductivity (λ). Liquid SM, frozen SM, and SOC are three key factors influencing the two thermal parameters in the model (Eqs. 6, 7). In the original MICT model, the average values of these three factors across all PFTs are used due to the limitation of having a grid-scale mean energy budget. In the new version, we simulate PFT-specific liquid SM and frozen SM in energy modules to represent the heterogeneity of different PFTs, following the separation of soil heat conduction (Fig. 2). Regarding SOC, MICT uses the grid cell SOC obtained from an observation-based SOC map (FAO, 2012; Zhu et al., 2019; Guimberteau et al., 2018; Hugelius et al., 2013); therefore, the effects of SOC on thermal parameters are represented homogeneously within each grid cell. According to Zhu et al. (2019), an increase of 20 kg C m−3 in SOC between two PFTs, which can be found in site-level data (Palmtag et al., 2022) and is reproduced in model simulations (Fig. S2 in the Supplement), could result in a 42 %–52 % decrease in thermal diffusivity () and a subsequent 13 %–18 % increase in the current permafrost extent. The important role of SOC in regulating soil thermal regimes and the heterogeneity of SOC across different PFTs highlight the pressing need to represent the thermal effects of SOC for each sub-grid. However, limited by the availability of observed SOC data that could be prescribed for sub-grids at the regional or global scale, we utilize the simulated SOC for each PFT in MICT-teb and the simulated total SOC of all PFTs in MICT. Using simulated instead of prescribed SOC has the advantage of making the modeled SOC fully consistent with the simulated soil physics, but it has the drawback that SOC formed by processes that cannot be simulated by the model (e.g., Pleistocene ecosystems such as yedoma, thermokarst lakes filling by organic sediments) will be ignored, causing a possible mismatch with observed SOC density. Nevertheless, a comparable spatial pattern of gridded SOC for 0–3 m over the Northern Hemisphere (NH) against observation-based SOC data and a comparable vertical profile against site-level data (Palmtag et al., 2022) confirm the model's ability to simulate the total volume (Fig. S3 in the Supplement) and the PFT-specific vertical profiles (Fig. S2 in the Supplement) of SOC.
Table 1Simulation protocol. MICT and MICT-teb indicate the original ORCHIDEE-MICT (without tiling energy budget) and the new ORCHIDEE-MICT-teb (with tiling energy budget), respectively. OFF and ON indicate turning the flags that control the tiling energy budget in MICT-teb off and on, respectively. If the flags are turned off, all of the PFT-specific variables related to energy budget will use the grid cell mean value in MICT-teb. LUC indicates land use change.
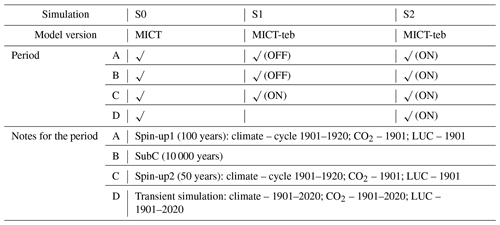
3.4 Associated modifications of hydrological and carbon processes
Soil temperature (Tsoil) is a key factor that influences hydrological and biogeochemical processes in the soil. Therefore, the PFT-specific variations in Tsoil result in a series of associated modifications to the hydrological and carbon-related processes with respect to the original MICT model (Fig. 2). For soil hydrology, there are three main modifications in MICT-teb compared with MICT: (1) the calculation of PFT-specific Tsoil for each PFT to calculate the liquid-to-frozen phase ratio of SM, (2) the use of PFT-specific bare-soil evaporation from the energy module, and (3) the separation of snow-related processes for each PFT. For the soil carbon cycle, there are four main modifications in MICT-teb: (1) the use of PFT-specific Tsoil and SM for litter decomposition, (2) the use of PFT-specific Tsoil and SM to calculate carbon flow from litter to soil, (3) the use of PFT-specific Tsoil and SM for root maintenance respiration, and (4) the use of PFT-specific Tsoil and SM for soil carbon decomposition.
To compare the differences in energy, hydrology, and carbon processes between MICT-teb and MICT, we design three groups of simulations (S0, S1, and S2), as shown in Table 1. All of the three groups are run for the NH (0–90° N) at a 2° × 2° spatial resolution with four simulation periods: (A) Spin-up1 – 100 years of the full ORCHIDEE model with a looped 1901–1920 climate, the CO2 level of 1901 at 296.80 ppm, and the land cover map of 1901; (B) SubC – 10 000 years of the soil carbon sub-model to accumulate SOC; (C) Spin-up2 – 50 years of the full ORCHIDEE model to reach equilibrium with a looped 1901–1920 climate, the CO2 level of 1901, and the land cover map of 1901; and (D) Transient simulation – the full ORCHIDEE model with varying climate and the CO2 level and land cover maps from 1901 to 2020. The climate forcing data are obtained from CRU-JRA v2.3 (the version used in Global Carbon Budget 2022) (Friedlingstein et al., 2022), while the land cover maps are generated by combining the land cover map from TRENDY for 15 PFTs (bare soil, 8 tree PFTs, 4 grass PFTs, and 2 crop PFTs) (Lurton et al., 2020) and the peat map from Xu et al. (2018) for the peat grass PFT (Xu et al., 2018; Qiu et al., 2019). In S0, the original MICT model is used for all four periods. In S1, MICT-teb is used with the flags controlling the tiling energy budget (TEB) turned off for periods A and B (i.e., identical to group S0) but turned on for period C. In this way, the differences in energy, hydrology, and carbon between S0 and S1 solely due to the TEB can be compared based on the same starting point (end of period B). In S2, the flags controlling the TEB are turned on from the beginning of period A. Thus, comparing S0 and S2 could infer differences in the long-term equilibrium of energy and hydrology as well as the near-equilibrium soil carbon storage between MICT-teb and MICT.
Following the description of the simulation protocol in Sect. 4, this section presents the differences in energy, hydrology, and carbon processes between MICT-teb and MICT. Section 5.1 presents the comparison of S1 and S0, i.e., the impacts solely due to TEB, while Sect. 5.2 presents the comparison of all the three simulations across the first three simulation periods, i.e., the long-term impacts of the TEB on energy, hydrology, and carbon processes. Unless otherwise stated, all differences indicate the mean values of the last 10 years in period C from MICT-teb minus those from MICT in Sect. 5.1.
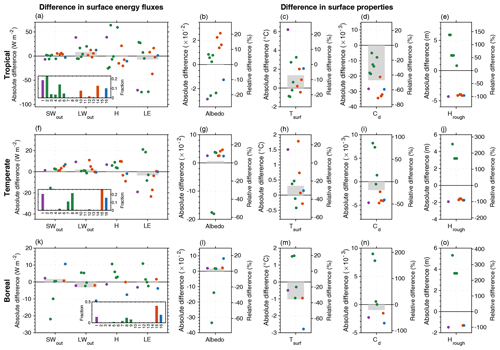
Figure 3Differences in surface energy fluxes (the first column) and surface properties (from the second to the last column) between MICT-teb and MICT for three grid cells located in the tropical (17° N, 155° W), temperate (51° N, 101° W), and boreal (71° N, 147° E) regions, respectively. The surface energy fluxes include outward shortwave radiation (SWout), outward longwave radiation (LWout), sensible heat flux (H), and latent heat flux (LE). The surface properties include albedo (Albedo), surface temperature (Tsurf), surface drag coefficient (Cd), and roughness height (Hrough). The gray bar in the background indicates the grid-scale difference in each variable. The colored points from left to right indicate the differences between 16 PFTs in MICT-teb and the grid-scale values in MICT, and the missing points indicate that the cover fraction of 1 PFT is 0 for the grid cell. The insets in the first column show the cover fraction of 16 PFTs for the grid cell. The 16 PFTs are bare soil (PFT 1, in purple), trees (PFT 2–9, in green), grass (PFT 10–15, in orange), and peat grass (PFT 16, in blue). The reader is referred to Table S3 for the full names of the 16 PFTs.
5.1 Impacts solely due to the tiling energy budget
5.1.1 Surface energy budgets
To explain the differences in energy budgets between MICT-teb and MICT, we begin our analysis by randomly selecting three grid cells at latitudes for tropical (17° N, 155° W), temperate (51° N, 101° W), and boreal (71° N, 147° E) biomes (Fig. 3). For the energy budgets at the surface (Eq. 2), the SWin and LWin are the same between the two versions (not shown), as both variables come from the input climate data, whereas the other four main surface energy fluxes, including SWout, LWout, H, and LE, show significant differences due to the TEB. The differences in energy fluxes can be well explained by the differences in surface properties at all three grid cells for the different latitudes. For instance, the difference in SWout between MICT-teb and MICT can be explained by the difference in albedo, the difference in LWout can be explained by the difference in Tsurf, and the difference in H (or LE) can be explained by the difference in Tsurf and/or Cd (surface drag coefficients). This correlation agrees well with theoretical equations (Eqs. 2–4).
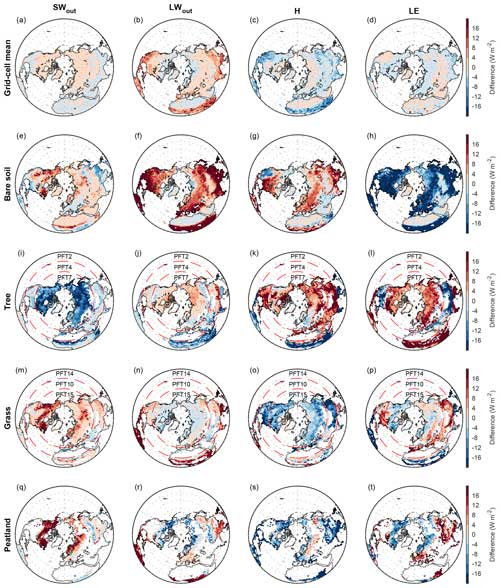
Figure 4Spatial patterns of differences in surface energy fluxes including outward shortwave radiation (SWout), outward longwave radiation (LWout), sensible heat flux (H), and latent heat flux (LE) between MICT-teb and MICT over the Northern Hemisphere. The first to fifth rows show the difference in each flux between the grid cell mean (a–d) or four tiles (e–t) from MICT-teb and the grid cell mean from MICT, respectively. The four tiles include (e–h) bare soil (PFT1), (i–l) tree (a combination of PFT2 (tropical broad-leaved evergreen tree) south of 20° N, PFT4 (temperate needleleaf evergreen) between 20 and 40° N, and PFT7 (boreal needleleaf evergreen tree) north of 40° N), (m–p) grass (a combination of PFT14 (Topical C3 grass) south of 20° N, PFT10 (temperate C3 grass) between 20 and 40° N, and PFT15 (boreal C3 grass) north of 40° N), and (q–t) peatland grass (PFT16). The three PFTs for the tree or grass are combined in order to show as many results as possible, but only one PFT is shown in each grid cell.
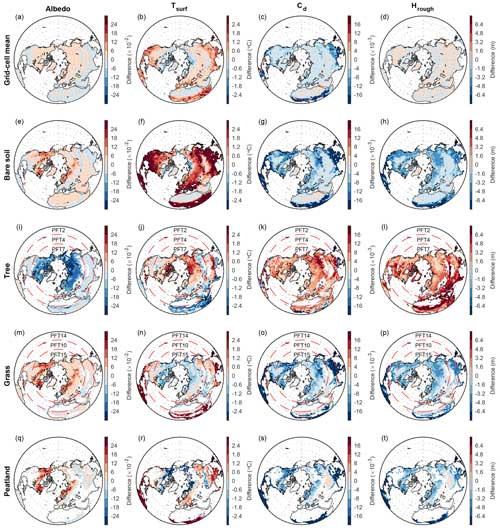
Figure 5Same as Fig. 4 but for spatial patterns in the differences in surface properties, including albedo (Albedo), surface temperature (Tsurf), surface drag coefficients (Cd), and roughness height (Hrough) between MICT-teb and MICT over the Northern Hemisphere.
Table 2Qualitative summary of latitudinal trends in the differences in surface properties and associated differences in surface energy fluxes between MICT-teb and MICT. The red and blue arrows indicate the warming and cooling effects on surface temperature, respectively. Two arrows indicate a stronger effect than one arrow.
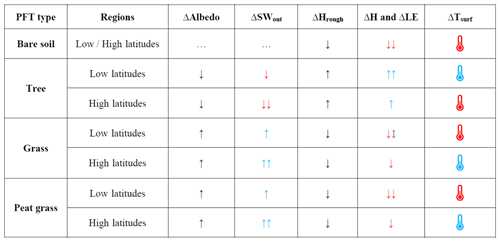
The variations in surface properties are related to the modifications made to represent the PFT-specific information in MICT-teb. Regarding albedo, grass leaves generally have a higher albedo (0.15–0.16) than tree leaves (0.10–0.14) (Table S1 in the Supplement), while the albedo of bare soil varies in the model depending on soil moisture, ranging from ∼ 0.05 in moist areas to ∼ 0.5 in dry areas (Fig. S1 in the Supplement). In the tropical grid cell, all four grass PFTs show a higher albedo than the grid cell mean (0.125); in contrast, some of the six tree PFTs show a lower albedo than the grid cell mean, whereas others show a higher value. The peat PFT has the same leaf albedo value as grass PFTs, but its albedo, as shown in Fig. 3b, is lower than the grid cell mean, which is due to the higher fraction of bare soil (with a small albedo of 0.093) for this PFT (∼ 70 %) relative to the other four grass PFTs (2 %–30 %). We note that the cover fraction of a PFT in the model includes both the leaf-covered area (the canopy) and the non-leaf-covered area (the soil), depending on a function of the leaf area index. The albedo of a PFT is calculated as the area-weighted sum of the albedo of leaves and the albedo of soil within this PFT. In temperate and boreal regions, the albedo of one PFT is greatly influenced by the snow cover fraction, owing to the significantly higher albedo of snow (Table S3). Consequently, the pattern of the difference in albedo between MICT-teb and MICT (Fig. 3g, l) closely resembles the difference in the snow cover fraction (Fig. S4 in the Supplement) for temperate and boreal grid cells. Regarding Cd, the surface drag coefficient, its variation is determined by the variation in the PFT-specific Tsurf and Hrough (roughness height): the smaller the Tsurf and the larger the Hrough, the larger the Cd. The theoretical relationship can be reproduced well from the comparison of simulated results between MICT-teb and MICT in Fig. 3.
When extending this to the whole NH, the correspondence between differences in surface heat fluxes and differences in surface properties at the grid cell scale can still be observed (Figs. 4 and 5 as well as Fig. S5 in the Supplement). Additionally, certain latitudinal trends begin to emerge in the difference between MICT-teb and MICT. Based on our modifications to calculate PFT-specific leaf albedo and Hrough, the most immediate variations in SWout or turbulent fluxes (H and LE) lead to the final direction of differences in Tsurf between MICT-teb and MICT for different PFTs (Table 2). For bare soil, whose Hrough is set to 0 in MICT-teb, the smaller H and LE result in a higher Tsurf (up to 3 °C) compared with MICT across almost all areas with bare soil. For tree PFTs, the higher H and LE due to the larger Hrough contribute to an overall cooler Tsurf (−3 to 0 °C) at low latitudes, while the more important decrease in SWout due to the smaller albedo in MICT-teb results in a warmer Tsurf (0–2 °C) at high latitudes. With the same dominant role of the Hrough variation at low latitudes and of the albedo variation at high latitudes, the grass and peat grass show a warmer Tsurf (0–3 °C) at low latitudes but a cooler Tsurf (−1 to 0 °C for grass and −2 to 0 °C for peat grass) at high latitudes in MICT-teb. As a result, the grid cell Tsurf simulated by MICT-teb is 0–2 °C higher in most regions in the NH, although it is slightly cooler (−1 to 0 °C) north of 60° N and in some arid regions compared with MICT (Fig. 5b).
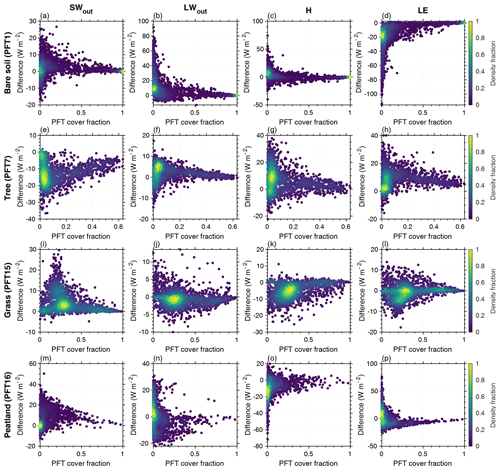
Figure 6Scatterplot of differences in surface energy fluxes between MICT-teb and MICT versus the vegetation cover fraction for bare soil (PFT1), tree (PFT7), grass (PFT15), and peatland grass (PFT16) plant functional types. The surface energy fluxes include outward shortwave radiation (SWout), outward longwave radiation (LWout), sensible heat flux (H), and latent heat flux (LE). The color of each point represents the density fraction of grid cells.
Another interesting result is the relationship between differences in surface heat fluxes or properties between MICT-teb and MICT and the vegetation cover fraction. As mentioned in Sect. 3.1, the difference between the two versions should become smaller in areas where one PFT tends to become more dominant in the grid cell. When the cover fraction of one PFT approaches 100 %, there will be no difference between the grid cell mean and the specific PFT. Taking four PFTs as examples, we found this pattern for both the surface heat fluxes (Fig. 6) and surface property variables (Fig. S6 in the Supplement).
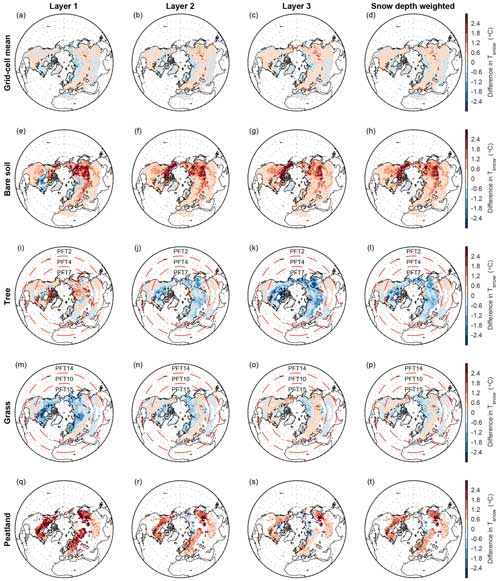
Figure 7Spatial patterns in the difference in Tsnow between MICT-teb and MICT for three snow layers and the snow-depth-weighted results over the Northern Hemisphere. The snow layers from top to bottom are numbered 1, 2, and 3, respectively. The snow-depth-weighted results are shown due to the different snow layer depth across different grid cells.
5.1.2 Snow energy budgets
Figure 7 presents differences in Tsnow (ΔTsnow) for three snow layers between MICT-teb and MICT for the grid cell mean and four PFTs. Overall, the grid cell ΔTsnow follows the ΔTsurf between the two versions, with a snow layer that is up to 1 °C warmer across most areas in MICT-teb. The correlation of ΔTsnow and ΔTsurf weakens from the uppermost snow layer (Tsnow,1) to the bottom one (Tsnow,3), especially for the tree and grass PFTs (Table S5 in the Supplement). For the two aforementioned PFTs, the differences in the two thermal parameters (csnow and λsnow) play a more important role than ΔTsurf in shaping the spatial pattern of ΔTsnow in the bottom two layers (Table S5 and Figs. S7 and S8 in the Supplement). As the snow depth for each layer is not fixed like soil layers (Fig. 1), we calculate the snow-depth-weighted ΔTsnow between the two versions (the last column in Fig. 7). The differences in csnow and λsnow are more important in determining the spatial patterns of the snow-depth-weighted ΔTsnow.
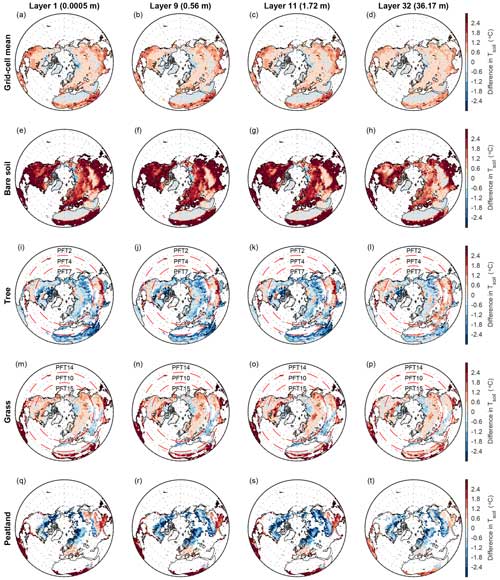
Figure 8Same as Fig. 7 but for spatial patterns in the differences in Tsoil between MICT-teb and MICT for four soil layers over the Northern Hemisphere.
5.1.3 Soil energy budgets
Figure 8 presents the differences in Tsoil (ΔTsoil) of four soil layers between MICT-teb and MICT for the grid cell mean and four PFTs. Similar to ΔTsnow, ΔTsoil shows a larger and significantly positive correlation (R = 0.31–1.00, p < 0.05) with differences in the starting point of heat conduction (Tsurf for 0–30° N and Tsnow,3 for 30–90° N) compared with the two thermal parameters of soil (Table S6 in the Supplement). The grid cell mean Tsoil for the four soil layers simulated by MICT-teb is ∼ 0.6 °C warmer than MICT north of 30° N, whereas it is ∼ 1.2 °C warmer in the tropics across four soil layers. The PFT-specific ΔTsoil values show considerably different magnitudes and directions across the four PFTs: the Tsoil for bare soil is ∼ 3 °C higher in MICT-teb than in MICT, the Tsoil for tree and peat grass PFTs is 0.6–3 °C lower, and the Tsoil for grass is 0.6–3 °C higher. Despite using the same parameter values of leaf albedo and Hrough between peat grass and C3 grass, the Tsoil of peat grass is 0.6–3 °C lower at high latitudes in MICT-teb, which could be related to the considerably different spatial patterns of the two soil thermal parameters between peat grass and C3 grass (Table S6 and Figs. S9 and S10 in the Supplement).
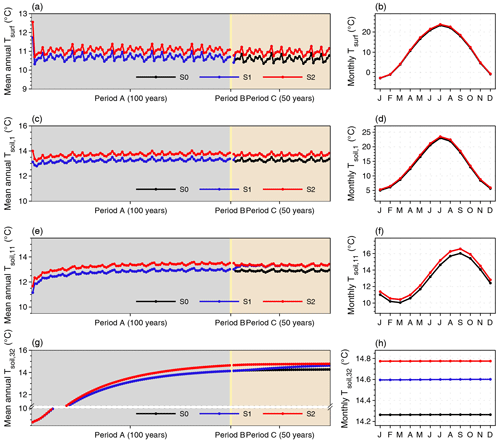
Figure 9Time series and seasonal cycle of surface temperature (Tsurf) and soil temperature for three soil layers (Tsoil,1, Tsoil,11, and Tsoil,32) over the Northern Hemisphere from three simulations. The annual values are calculated using the yearly average, and the monthly values are calculated using the average of the last 10 years in period C. The backgrounds in panels (a), (c), (e), and (g) are shaded to help explain the simulation length of the three periods. Detailed explanations for the three simulations (S0, S1, and S2) and the three periods (A, B, and C) can be found in Table 1 and Sect. 4.
As mentioned in Sect. 3.3, the liquid SM (SMliquid), frozen SM (SMfrozen), and SOC are three key factors influencing the two thermal parameters of soil (Eqs. 6 and 7). Despite the previous soil-tile-based hydrological processes and PFT-based carbon cycle in MICT, the soil thermal parameters (c and λ) are calculated with the grid cell mean values of SMliquid, SMfrozen, and SOC in that version. With far wetter SM (≥ 200 %; Figs. S11 and S12 in the Supplement) and far more SOC storage (≥ 200 %; Fig. S13 in the Supplement), the peat PFT has a ∼ 200 % higher c (Fig. S9 in the Supplement) and a ∼ 100 % higher λ (Fig. S10 in the Supplement) than the grid cell mean. Such a large Δc and Δλ compared with grass (40 % higher/lower c and 20 % higher/lower λ than the grid cell mean) lead to a lower Tsoil for peat grass in MICT-teb compared with MICT (Fig. 8). Besides the peat grass PFT, the important role of the three factors in regulating Tsoil can also be found for other PFTs. For example, we found ∼ 100 % less SOC storage in bare soil than the grid cell mean, which contributes to the higher Tsoil for bare soil due to the absence of the insulating impacts of SOC.
5.2 Long-term impacts on energy, hydrology, and the carbon cycle
5.2.1 Energy
Regarding the long-term impacts of representing the sub-grid energy budgets in the model, we compare the simulated results over the NH among S0, S1, and S2 from three aspects: energy budgets, hydrology, and the carbon cycle (Figs. 9–11). For the energy budgets, we found that the difference in Tsurf over the NH between S1 and S0 or between S2 and S0 appears in the first 2–3 years and then remains stable throughout all three simulation periods (Fig. 9a). By the end of period C, the ΔTsurf over the NH between S2 and S0 remains at 0.37 °C (+3.5 %). A very similar ΔTsurf (0.38 °C, 3.6 %) can be observed between S1 and S0, suggesting that the surface energy budgets can quickly respond to variations in surface properties, including albedo and roughness. As the heat moves down, the determining role of Tsurf with respect to influencing the heat conduction in soil (Table S6) makes the Tsoil at the 1st (0.0005 m), 11st (1.72 m), and 32nd (36.17 m) layers over the NH warmer by 0.44 °C (3.3 %), 0.45 °C (3.5 %), and 0.51 °C (3.6 %), respectively, by the end of period C under S2 compared with under S0. However, it takes longer for Tsoil to reach stability in the bottom soil layers than in the upper ones (Fig. 9c, e, g). The SOC, acting as an insulator, could regulate the soil thermodynamics, especially in summer (Zhu et al., 2019). Nevertheless, the difference in mean annual or monthly Tsoil in all soil layers between S1 and S2 is very small – no more than 0.18 °C (1.2 %). Therefore, the long-term effects of the TEB on the surface or soil energy budgets over the NH are subtle in the simulations in this study.
5.2.2 Hydrology
The hydrological processes over the NH can respond to the representation of sub-grid energy budgets as quickly as temperature, taking up to 5 years to reach the stability for both surface water fluxes, including evapotranspiration (ET) and surface runoff (Q), and subsurface water fluxes, such as drainage (D) (Fig. 10). Overall, MICT-teb shows a smaller ET (−8.7 mm yr−1, −2.3 %) but a larger D (+11.8 mm yr−1, 8.3 %) over the NH compared with MICT. When separating the subcomponents of ET and soil tiles, we found that the decreased ET is mainly contributed by the decreased Etrans (transpiration) from tree PFTs and the decreased Esubli (sublimation) from the grass PFTs, with values of −3.7 and −3.0 mm yr−1 per grid cell over the NH, respectively (Fig. S14 in the Supplement). Spatially, the decreased Etrans values for tree PFTs are mainly distributed in tropical regions and eastern North America, while the decreased grass Esubli is located at high latitudes (Fig. S15 in the Supplement). Both of these decreases are related to the variations in surface properties (the decreased Cd and/or decreased Tsurf) in MICT-teb (Fig. 5). As a result of the balance between the variations in ET and runoff, the SM (0–2 m) in MICT-teb is 9.9 kg m−2 (1.8 %) wetter than in MICT over the NH (Fig. 10).
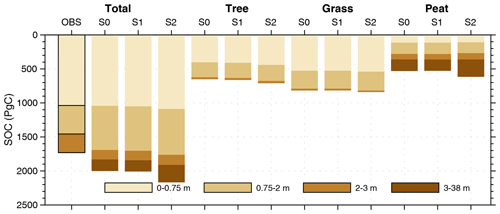
Figure 12Vertical composition of soil organic carbon (SOC) for all PFTs and three vegetated soil tiles (tree, grass, and peat) over the Northern Hemisphere from an observation-based SOC map and three simulations. For observed data, we only show the results for all PFTs due to the lack of biome information. For simulations, all values are calculated using the average of the last 10 years in period C.
5.2.3 Carbon
In response to the warmer and wetter soils (Fig. S16 in the Supplement), vegetation productivity is significantly enhanced over the NH in MICT-teb, with a 1.9 Pg C yr−1 (2.7 %) larger GPP and a 0.8 Pg C yr−1 (2.7 %) larger NPP under S2 than S0 (Fig. 11). The enhanced productivity is primarily driven by tree PFTs across almost all NH regions and grass PFTs at middle and low latitudes, while the productivity of peatland grass is somewhat lower due to the cooler soil (Figs. S16 and S17 in the Supplement). The warmer soil also accelerates the heterotrophic respiration rate (Rh) for tree and grass PFTs, along with the variation in SM. Compared with S0, S2 has a larger SOC (+58.5 Pg C, 9.0 %) for tree PFTs but almost unchanged SOC storage (+22.5 Pg C, 2.8 %) for grass PFTs. Despite being the smallest SOC pool (∼ 27 %, ∼ 600 Pg C) (Hugelius et al., 2014, 2016, 2020; Lindgren et al., 2018) among the three vegetated soil tiles as a result of the small peatland area (∼ 3 % of vegetated land in the NH), the peatland PFT's SOC storage increases by 85.8 Pg C, accounting for more than a half of the total SOC increase from S0 to S2 (Fig. 12). The cooler soil throughout the entire vertical profile of the peat PFT promotes SOC accumulation by significantly slowing the soil respiration (R = 0.38, p < 0.01), showing a more critical role in regulating the SOC decomposition than SM (R = 0.20, p < 0.01). Moreover, unlike the energy and water processes, the difference in SOC (ΔSOC) over the NH between S2 and S0 is obviously larger than that between S1 and S0 (∼ 7 Pg C); from a temporal perspective, it exists after period B and then remains stable until the end of period C (Fig. 11g). This means that the ∼ 170 Pg C ΔSOC between S2 and S0 has accumulates from peat initiation, and the long-term effects of TEB on soil carbon cannot be neglected.
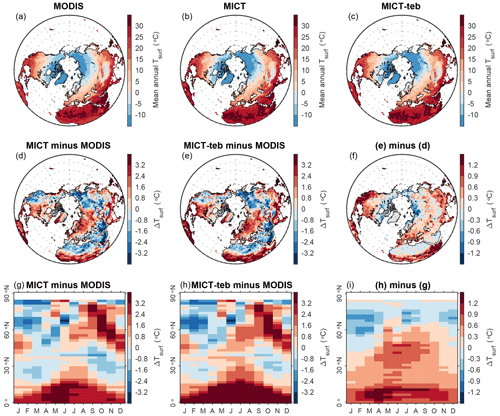
Figure 13Evaluation of simulated surface temperature (Tsurf) with land surface temperature (LST) from MODIS. (a–c) Spatial pattern of mean annual LST for 2001–2020 from MODIS, MICT, and MICT-teb. (d, e) Spatial pattern of the difference in mean annual Tsurf for 2001–2020 between MICT (d) or MICT-teb (e) and MODIS. (f) Spatial pattern of the difference between panels (e) and (d). (g, h) Seasonal cycle of the difference in Tsurf calculated over each latitude band between MICT (g) or MICT-teb (h) and MODIS. (i) Seasonal cycle of the difference between panels (h) and (g).
6.1 Evaluation of the simulated energy budgets
To evaluate the simulated surface energy budget, we compare the Tsurf (surface temperature, mirroring outward longwave radiation and sensible heat flux), albedo (mirroring outward shortwave radiation), and LE (latent flux) from period D (Transient simulation, 1901–2020) with satellite-derived land surface temperature (LST), albedo, and LE from MODIS (the Moderate Resolution Imaging Spectroradiometer), respectively. The MODIS LST product is obtained from MOD11C3 Version 6.1 (https://lpdaac.usgs.gov/products/mod11c3v061/, last access: 3 July 2023), which records the monthly radiative skin temperature of the land surface at a 0.05° × 0.05° spatial resolution, spanning from 2000 to the present (Wan, 2013, 2014). Compared with the MODIS product, MICT and MICT-teb reproduce the spatial pattern of mean annual satellite-derived LST for 2001–2020 (Fig. 13a, b, c) but with an overestimation of up to 3 °C in wet regions, such as tropical regions, Europe, and eastern North America, as well as an underestimation of up to 3 °C in dry areas and northeastern Asia (Fig. 13d, e). Regarding the seasonality of Tsurf, the two model versions show an overestimation of summer and autumn LST (by up to 3 °C) but an underestimation of winter LST (by up to 3 °C) at middle to high latitudes, while an overestimation of up to 3 °C exists throughout the year for the tropics (Fig. 13g, h). Including the representation of PFT-specific energy budgets alleviates the LST bias from MICT in some areas, such as western North America and northern Europe, and in some seasons, such as autumn at high latitudes, but it concurrently aggravates the LST bias in some areas and some seasons, such as all four seasons in tropical regions (Fig. 13f, i).
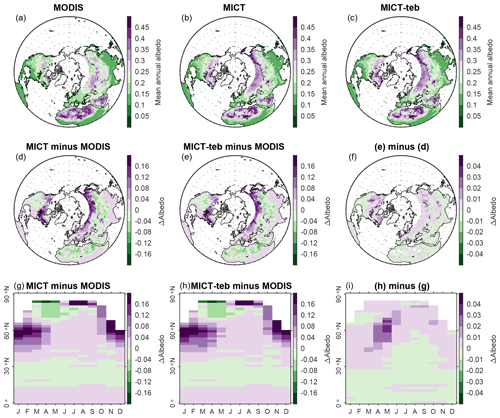
Figure 14Same as Fig. 13 but for the evaluation of simulated albedo with blue-sky albedo from MODIS.
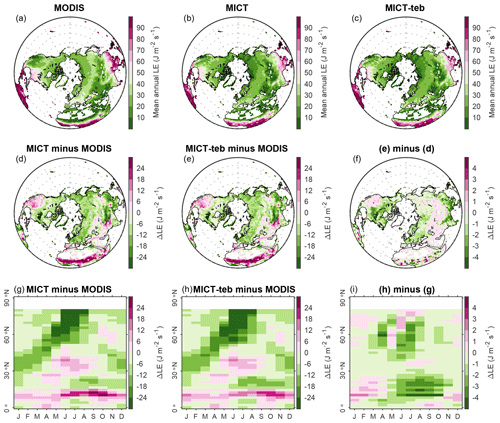
Figure 15Same as Fig. 14 but for the evaluation of simulated latent flux (LE) with that from MODIS.
The MODIS albedo product is obtained from MCD43C3 Version 6.1 (https://lpdaac.usgs.gov/products/mcd43c3v061/, last access: 3 March 2024), including the daily black-sky and white-sky albedo at a 0.05° × 0.05° spatial resolution. For comparison with the albedo from the model, we calculate the blue-sky albedo using the black-sky and white-sky albedo for the shortwave band (0.3–5.0 µm) from MODIS, weighted by the diffuse skylight ratio derived from the direct and total shortwave radiation from the fifth-generation ECMWF reanalysis product (ERA5, https://cds.climate.copernicus.eu/cdsapp#!/dataset/reanalysis-era5-single-levels-monthly-means?tab=form, last access: 3 March 2024) (Hersbach et al., 2023). We found that, except for a ∼ 0.2 overestimation of winter and spring albedo in northern high latitudes, the simulated albedo from MICT and MICT-teb shows a very small bias, no more than 0.04 (Fig. 14). The considerable albedo biases in winter and spring could be related to the bias in the leaf area index and snow cover fraction values simulated by the model (Li et al., 2016). The MODIS LE product is obtained from MOD16A2GF Version 6.1 (https://lpdaac.usgs.gov/products/mod16a2gfv061/, last access: 3 March 2024), providing the 8 d LE at a 500 m × 500 m spatial resolution. Compared with the MODIS LE, the simulated LE tends to be smaller (−6 to −24 ) in most areas except for some arid regions over the NH (Fig. 15). Same as the evaluation for Tsurf, the representation of PFT-specific energy budgets does not reduce the albedo/LE biases significantly (panels g and h in Figs. 14 and 15).
There are several reasons that could explain disagreements in the surface energy budgets between the MODIS products and the models. On the one hand, (1) the under-representation of some important processes in the model, such as the parameterization of ET (LE) and snow insulation, as well as the uncertainties in climate forcing data (Guimberteau et al., 2018; Peng et al., 2016; Domine et al., 2016) and (2) missing data across dry areas and cloudy-weather days in the MODIS products, e.g., a ± 2 °C LST bias compared with ground-truth data found in dry areas (Li et al., 2014; Wan, 2014; Westermann et al., 2012), could partly account for disagreements between the MODIS products and the models. On the other hand, the considerably different land cover maps used by MODIS and the simulations (Fig. S18 in the Supplement) as well as the difference in one specific variable from MODIS and the simulations could contribute to the systematic biases or gaps. For instance, the LST from MODIS more closely reflects the radiative skin temperature, i.e., canopy temperature, while the canopy energy budget is absent in ORCHIDEE-MICT, which could result in a higher Tsurf from the models compared with the MODIS LST in forest ecosystems (Fig. 13) (Gomis-Cebolla et al., 2018).
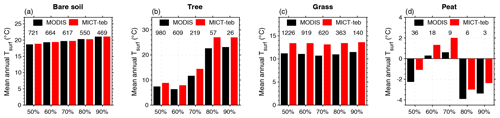
Figure 16Evaluation of simulated surface temperature (Tsurf) with land surface temperature (LST) from MODIS by PFT. (a–d) Mean annual Tsurf for four PFTs (bare soil, tree, grass, and peat) for 2001–2020. The grid cells for each PFT are selected with five threshold fractions (50 %, 60 %, 70 %, 80 %, and 90 %), i.e., the minimum fractional coverage of one land cover type in the grid cell. The numbers of grid cells for each group of bars are shown.
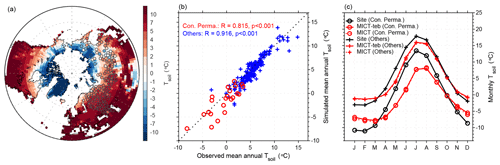
Figure 17Evaluation of simulated soil temperature (Tsoil) at 20 cm with site observations. (a) Spatial patterns of mean annual Tsoil at 20 cm during the 1980–2000 period from the simulation of MICT-teb, with the values of 199 sites shown as color-filled circles. (b) Simulated (from MICT-teb) versus observed mean annual Tsoil at 20 cm across all sites. (c) Mean seasonal cycle of site-averaged Tsoil at 20 cm from site observations, MICT-teb, and MICT. The sites in panels (b) and (c) are divided into those located in continuous permafrost (Con. Perma.) regions (22 sites, circle markers) and others (177 sites, cross markers) according to the permafrost map of Brown et al. (2002).
In light of the new feature of MICT-teb to simulate PFT-specific energy budgets, we compare the simulated and satellite-based Tsurf over grid cells dominated by four PFTs: bare soil, tree, grass, and peatland from simulations (Fig. 16). Despite the significant disagreements regarding the surface energy budgets between the MODIS products and the models, the model can produce a comparable Tsurf to MODIS for the four PFTs. Moreover, the model can capture the variations in the LST from MODIS well when altering the threshold fractions for grid cell selection from 50 % to 90 %. For tree, grass, and peat, due to their extensive coverage in wet areas, the simulated Tsurf is 1–3 °C warmer than the grid cell LST from MODIS for all threshold fractions. For bare soil, the underestimation of Tsurf in desert areas is offset by the overestimation in Greenland (Fig. 13d, e). The notable biases when using a 90 % threshold fraction, a near-complete coverage of one PFT within one grid cell, suggests that the disagreement between the model and satellite products should be attributed to the gap between MODIS and the original version, rather than the separation of PFT-specific energy budgets.
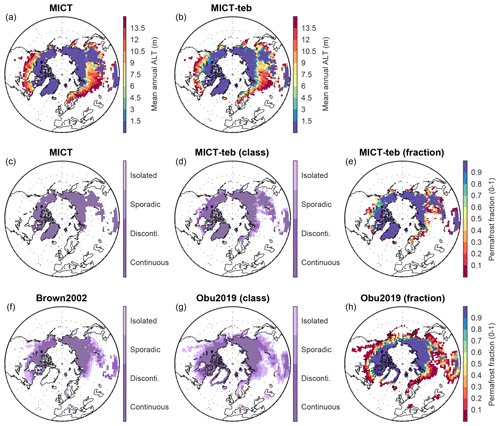
Figure 18Evaluation of simulated permafrost areas with independent permafrost datasets. (a, b) Spatial patterns of the active layer thickness (ALT) simulated by MICT and MICT-teb. (c, e) Spatial patterns of permafrost areas simulated by MICT and MICT-teb according to the definition of ALT. (f–h) Spatial patterns of permafrost areas from Brown2002 and Obu2019. The permafrost areas in panels (c), (d), (f), and (g) are shown following four permafrost classes from Brown2002, while the permafrost areas in panels (e) and (h) are shown as the absolute fraction.
For the simulated soil energy budget, we evaluate the Tsoil at 20 cm with in situ observations from across 268 Russian meteorological station sites (Sherstiukov, 2012). The period of the site data spans from 1980 to 2000. To avoid the bias resulting from missing values, we exclude 69 sites containing missing values for a specific month in over half of years. As shown in Figs. 17 and S19, the simulated mean annual Tsoil at 20 cm from MICT and MICT-teb can well reproduce the spatial gradient of observed values, with a strong and positive correlation (R = 0.94; p < 0.001). However, when it comes to the mean seasonal cycle of Tsoil, a warmer Tsoil during winter and a cooler Tsoil during summer are simulated in comparison with observations, especially for sites located in continuous permafrost regions (Fig. 17c). Apart from climate forcing data uncertainties, as suggested by Guimberteau et al. (2018), SM, SOC, and snow cover are three factors most likely to regulate the seasonal amplitude of Tsoil. Guimberteau et al. (2018) have demonstrated that snow insulation is underestimated by MICT when using either the GSWP3 dataset or the CRUNCEP dataset to force the model. This implies that, from a snow perspective, our simulation should exhibit an amplified seasonal cycle. Conversely, the simulated dampened seasonal amplitude of Tsoil indicates the potentially crucial roles of SM and SOC. Due to the inclusion of the peat PFT in our simulations and the use of simulated SOC rather than prescribed SOC maps to regulate soil thermal properties, the uncertainties in prescribed peatland maps and simulated SOC could propagate the uncertainties in Tsoil. Moreover, we extract LST in the year 2000 across these sites from MODIS data and find a weak correlation between the bias in Tsurf against MODIS and the bias in Tsoil against site data (R = 0.19, p < 0.01 for all sites; R = −0.01, p > 0.05 for continuous permafrost sites; R = 0.03, p > 0.05 for other sites), suggesting potential uncertainties in observed data from different sources.
As for the impacts of tiling energy budgets on Tsurf, our simulations suggest that the variation in albedo plays a dominant role at high latitudes, whereas the variation in Hrough is more significant at low latitudes (Table 2). This result is consistent with numerous studies on the biophysical feedbacks of land cover change, as the use of PFT-specific Hrough and albedo for tree PFTs can be seen as an analogy to reforestation or afforestation, while the use of PFT-specific Hrough and albedo for grass PFTs parallels the case of deforestation or forest degradation. Flux tower measurements, satellites, and climate models have revealed that tropical tree planting mitigates warming through evaporative cooling, while the low albedo of new boreal forests is a positive climate forcing (Betts, 2000; Bonan, 2008; Peng et al., 2014; Su et al., 2023). In contrast, the conversion of forests to grasslands or forest degradation shows a warming effect in tropical regions but a cooling effect in boreal regions (Lawrence and Vandecar, 2015; Alkama and Cescatti, 2016; Li et al., 2022; Zhu et al., 2023). This consistency confirms the modifications that we made in the new MICT version.
6.2 Improvements for permafrost simulations
Given the crucial role of Tsoil in permafrost simulations, we compare the simulated permafrost extent from MICT and MICT-teb with two independent permafrost datasets from Brown et al. (2002) and Obu et al. (2019), hereafter referred to as Brown2002 and Obu2019, respectively (Fig. 18). Brown's map is compiled based on national and regional maps as well as empirical knowledge, categorizing permafrost into four classes: continuous permafrost (permafrost fraction > 0.9), discontinuous permafrost (0.5–0.9), sporadic permafrost (0.1–0.5), and isolated patches (0–0.1) (Brown et al., 2002). Obu's map is generated using a temperature model to simulate soil thermal regimes in three dimensions at a 300 m × 300 m spatial resolution (Obu et al., 2019). The Obu2019 data provide the absolute fraction of the landscape affected by permafrost when aggregated to a coarser grid cell (Obu et al., 2019). The simulated permafrost areas are identified following two definitions given by Guimberteau et al. (2018): (1) an active layer thickness (ALT) less than 3 m or (2) the Tsoil of any soil layer remains below 0 °C for at least 2 years. As the simulated permafrost areas are very similar between the two definitions, we only show the results using the ALT definition in the paper; the results using the Tsoil definition are given in the Supplement (Fig. S20 in the Supplement).
Overall, our models can capture the spatial pattern of continuous permafrost from the two independent datasets. However, the simulated total area of continuous permafrost from MICT after excluding Greenland (18.5 ×106 km2) is 7.6 ×106 and 6.9 ×106 km2 larger than that from Brown2002 and Obu2019, respectively, primarily due to the overestimation of continuous permafrost in the middle to high latitudes of Asia and the Tibetan Plateau. Given that the grid cell mean Tsoil in MICT-teb is ∼ 0.5 °C warmer over the NH compared with MICT (Fig. 8), the total area of continuous permafrost simulated by MICT-teb (15.2 ×106 km2) decreases by 3.3 ×106 km2 compared with that in MICT. Limited by the grid-cell-averaged energy budgets, MICT cannot simulate noncontinuous permafrost (i.e., a grid cell in MICT is either 100 % permafrost or 100 % non-permafrost), whereas the separation of PFT-specific Tsoil in MICT-teb allows the existence of noncontinuous permafrost (Fig. 18e). The sum of all four permafrost areas (including three discontinuous classes) in MICT-teb is 18.3 ×106 km2 (continuous, 15.2 ×106 km2; noncontinuous, 3.1 ×106 km2), which is comparable to 16.9 ×106 km2 in Obu2019 (continuous, 11.6 ×106 km2; noncontinuous, 5.3 ×106 km2).
6.3 Remarks on the tiling land surface scheme
The tiling work in this study was initiated because of the planned introduction of new arctic landforms for permafrost regions into ORCHIDEE-MICT that require independent energy budgets and carbon and water cycles. The decision to tile by PFT, rather than by other units, was determined based on the current model structure. In the new version, additional variables with a new PFT dimension were introduced only for the energy module, rather than all modules, resulting in a 15 %–20 % slower run time compared with the initial version. Recently, several other model groups have also been working on tiling and the evaluation of its impacts on existing and new processes, such as JULES (Rumbold et al., 2023) and CLASSIC (Melton et al., 2017). The implementation of tiling in different land surface models can be compared to inspire other groups planning to represent the sub-grid heterogeneity of energy budgets in their models. Moreover, it is worth reminding potential users of our new version to carefully consider when and where to apply tiling in their studies in order to optimize research objectives and computational costs.
This study describes the new representation of tiling energy budgets in the ORCHIDEE-MICT land surface model and investigates its short-term and long-term impacts on energy, hydrology, and carbon processes. Instead of using grid cell mean surface properties, like roughness height and albedo, PFT-specific values are employed for each vegetation type, and all of the associated energy, hydrology, and carbon processes are modified in the model. Compared with the original version, the separation of PFT-specific energy budgets results in warmer surface and soil temperatures, higher soil moisture, and increased soil organic carbon storage across the Northern Hemisphere. Evaluation with satellite products and site measurements suggests that the new version can reproduce the spatial distributions and seasonal patterns of surface and soil temperature. However, notable positive or negative biases are observed in some regions due to remaining weaknesses in the original ORCHIDEE-MICT model, such as the uncertainties involved in simulating soil moisture and soil organic carbon, as well as uncertainties in the prescribed peatland map. A notable advancement in the new version is the improved simulation of permafrost extent by accounting for the presence of discontinuous permafrost, which will facilitate various permafrost-related studies based on the model in the future.
The ORCHIDEE-MICT-teb model (r8205) code used in this study is open source and distributed under the CeCILL (CEA CNRS INRIA Logiciel Libre) license. It is deposited at https://forge.ipsl.jussieu.fr/orchidee/wiki/GroupActivities/CodeAvalaibilityPublication/ORCHIDEE-MICT-teb (last access: 30 September 2023) and archived at https://doi.org/10.14768/0954a0e9-6a7a-4006-803e-4db36ef2db88 (Xi, 2023a), with guidance to install and run the model at https://forge.ipsl.jussieu.fr/orchidee/wiki/Documentation/UserGuide (last access: 30 September 2023). Codes to process data, generate all results, and produce all figures are archived at https://doi.org/10.5281/zenodo.10014533 (Xi, 2023b).
Due to limitations imposed by their size, the model input files have not been uploaded to the public repository; however, they can be accessed from the corresponding author upon reasonable request. The MODIS LST product can be obtained from https://doi.org/10.5067/MODIS/MOD11C3.061 (Wan et al., 2021). The MODIS albedo product can be obtained from https://doi.org/10.5067/MODIS/MCD43C3.061 (Schaaf and Wang, 2021). The direct and total shortwave radiation from the ERA5 product can be obtained from https://doi.org/10.24381/cds.f17050d7 (Hersbach et al., 2023). The MODIS LE product can be obtained from https://doi.org/10.5067/MODIS/MOD16A2GF.061 (Running et al., 2021). The site-level soil temperature data can be obtained from http://meteo.ru/data/ (Sherstiukov, 2012). The permafrost map by Brown et al. (2002) can be obtained from https://doi.org/10.7265/skbg-kf16. The permafrost map by Obu et al. (2019) can be obtained from https://doi.org/10.1594/PANGAEA.888600 (Obu et al., 2018).
The supplement related to this article is available online at: https://doi.org/10.5194/gmd-17-4727-2024-supplement.
PC conceived of the project. YX implemented tiling energy budgets into ORCHIDEE-MICT. CQ, YZ, DZ, and SP provided general scientific guidance to improve the research and interpret results. YX performed the simulations, did the analysis, and wrote the paper. All authors contributed to commenting on and writing the manuscript.
The contact author has declared that none of the authors has any competing interests.
Publisher's note: Copernicus Publications remains neutral with regard to jurisdictional claims made in the text, published maps, institutional affiliations, or any other geographical representation in this paper. While Copernicus Publications makes every effort to include appropriate place names, the final responsibility lies with the authors.
The authors are grateful to the ORCHIDEE group for their kind help with the model.
This research was supported by the European Union’s Horizon 2020 Research and Innovation program (grant no. 101003687, PROVIDE). Philippe Ciais acknowledges support from the CALIPSO (Carbon Loss in Plant Soils and Oceans) project, funded through the generosity of Eric and Wendy Schmidt by recommendation of the Schmidt Futures program. Dan Zhu acknowledges support from National Natural Science Foundation of China (grant no. 42101090).
This paper was edited by Nathaniel Chaney and reviewed by Joe Melton and Dalei Hao.
Alkama, R. and Cescatti, A.: Biophysical climate impacts of recent changes in global forest cover, Science, 351, 600–604, https://doi.org/10.1126/science.aac8083, 2016.
Best, M. J., Beljaars, A., Polcher, J., and Viterbo, P.: A Proposed Structure for Coupling Tiled Surfaces with the Planetary Boundary Layer, J. Hydrometeorol., 5, 1271–1278, https://doi.org/10.1175/JHM-382.1, 2004.
Betts, R. A.: Offset of the potential carbon sink from boreal forestation by decreases in surface albedo, Nature, 408, 187–190, https://doi.org/10.1038/35041545, 2000.
Bonan, G. B.: Forests and Climate Change: Forcings, Feedbacks, and the Climate Benefits of Forests, Science, 320, 1444–1449, https://doi.org/10.1126/science.1155121, 2008.
Boone, A., Samuelsson, P., Gollvik, S., Napoly, A., Jarlan, L., Brun, E., and Decharme, B.: The interactions between soil–biosphere–atmosphere land surface model with a multi-energy balance (ISBA-MEB) option in SURFEXv8 – Part 1: Model description, Geosci. Model Dev., 10, 843–872, https://doi.org/10.5194/gmd-10-843-2017, 2017.
Brown, J., Ferrians, O., Heginbottom, J. A., and Melnikov, E.: Circum-Arctic Map of Permafrost and Ground-Ice Conditions, Version 2, National Snow and Ice Data Center [data set], Boulder, Colorado, USA, https://doi.org/10.7265/skbg-kf16, 2002.
Chalita, S. and Le Treut, H.: The albedo of temperate and boreal forest and the Northern Hemisphere climate: a sensitivity experiment using the LMD GCM, Clim. Dynam., 10, 231–240, https://doi.org/10.1007/BF00208990, 1994..
Curasi, S. R., Melton, J. R., Humphreys, E. R., Hermosilla, T., and Wulder, M. A.: Implementing a dynamic representation of fire and harvest including subgrid-scale heterogeneity in the tile-based land surface model CLASSIC v1.45, EGUsphere [preprint], https://doi.org/10.5194/egusphere-2023-2003, 2023.
Domine, F., Barrere, M., and Sarrazin, D.: Seasonal evolution of the effective thermal conductivity of the snow and the soil in high Arctic herb tundra at Bylot Island, Canada, The Cryosphere, 10, 2573–2588, https://doi.org/10.5194/tc-10-2573-2016, 2016.
Ducharne, A.: The hydrol module of ORCHIDEE: scientific documentation, http://forge.ipsl.fr/orchidee/attachment/wiki/Documentation/eqs_hydrol_25April2018_Ducharne.pdf (last access: 1 November 2019), 2018.
FAO, IIASA, ISRIC, ISSCAS and JRC: Harmonized WorldSoil Database (version 1.2), Tech. rep., FAO, Rome, Italy and IIASA, Laxenburg, Austria, https://www.fao.org/soils-portal/data-hub/soil-maps-and-databases/harmonized-world-soil-database-v12/en/ (last access: 1 November 2015), 2012.
Friedlingstein, P., O'Sullivan, M., Jones, M. W., Andrew, R. M., Gregor, L., Hauck, J., Le Quéré, C., Luijkx, I. T., Olsen, A., Peters, G. P., Peters, W., Pongratz, J., Schwingshackl, C., Sitch, S., Canadell, J. G., Ciais, P., Jackson, R. B., Alin, S. R., Alkama, R., Arneth, A., Arora, V. K., Bates, N. R., Becker, M., Bellouin, N., Bittig, H. C., Bopp, L., Chevallier, F., Chini, L. P., Cronin, M., Evans, W., Falk, S., Feely, R. A., Gasser, T., Gehlen, M., Gkritzalis, T., Gloege, L., Grassi, G., Gruber, N., Gürses, Ö., Harris, I., Hefner, M., Houghton, R. A., Hurtt, G. C., Iida, Y., Ilyina, T., Jain, A. K., Jersild, A., Kadono, K., Kato, E., Kennedy, D., Klein Goldewijk, K., Knauer, J., Korsbakken, J. I., Landschützer, P., Lefèvre, N., Lindsay, K., Liu, J., Liu, Z., Marland, G., Mayot, N., McGrath, M. J., Metzl, N., Monacci, N. M., Munro, D. R., Nakaoka, S.-I., Niwa, Y., O'Brien, K., Ono, T., Palmer, P. I., Pan, N., Pierrot, D., Pocock, K., Poulter, B., Resplandy, L., Robertson, E., Rödenbeck, C., Rodriguez, C., Rosan, T. M., Schwinger, J., Séférian, R., Shutler, J. D., Skjelvan, I., Steinhoff, T., Sun, Q., Sutton, A. J., Sweeney, C., Takao, S., Tanhua, T., Tans, P. P., Tian, X., Tian, H., Tilbrook, B., Tsujino, H., Tubiello, F., van der Werf, G. R., Walker, A. P., Wanninkhof, R., Whitehead, C., Willstrand Wranne, A., Wright, R., Yuan, W., Yue, C., Yue, X., Zaehle, S., Zeng, J., and Zheng, B.: Global Carbon Budget 2022, Earth Syst. Sci. Data, 14, 4811–4900, https://doi.org/10.5194/essd-14-4811-2022, 2022.
Georg, W., Albin, H., Georg, N., Katharina, S., Enrico, T., and Peng, Z.: On the energy balance closure and net radiation in complex terrain, Agr. Forest Meteorol., 226–227, 37–49, https://doi.org/10.1016/j.agrformet.2016.05.012, 2016.
Gomis-Cebolla, J., Jimenez, J. C., and Sobrino, J. A.: LST retrieval algorithm adapted to the Amazon evergreen forests using MODIS data, Remote Sens. Environ., 204, 401–411, https://doi.org/10.1016/j.rse.2017.10.015, 2018.
Gouttevin, I., Menegoz, M., Domine, F., Krinner, G., Koven, C., Ciais, P., Tarnocai, C., and Boike, J.: How the insulating properties of snow affect soil carbon distribution in the continental pan-Arctic area, J. Geophys. Res.-Biogeo., 117, 407–430, https://doi.org/10.1029/2011JG001916, 2012.
Guimberteau, M., Zhu, D., Maignan, F., Huang, Y., Yue, C., Dantec-Nédélec, S., Ottlé, C., Jornet-Puig, A., Bastos, A., Laurent, P., Goll, D., Bowring, S., Chang, J., Guenet, B., Tifafi, M., Peng, S., Krinner, G., Ducharne, A., Wang, F., Wang, T., Wang, X., Wang, Y., Yin, Z., Lauerwald, R., Joetzjer, E., Qiu, C., Kim, H., and Ciais, P.: ORCHIDEE-MICT (v8.4.1), a land surface model for the high latitudes: model description and validation, Geosci. Model Dev., 11, 121–163, https://doi.org/10.5194/gmd-11-121-2018, 2018.
Hao, D., Bisht, G., Gu, Y., Lee, W.-L., Liou, K.-N., and Leung, L. R.: A parameterization of sub-grid topographical effects on solar radiation in the E3SM Land Model (version 1.0): implementation and evaluation over the Tibetan Plateau, Geosci. Model Dev., 14, 6273–6289, https://doi.org/10.5194/gmd-14-6273-2021, 2021.
Hersbach, H., Bell, B., Berrisford, P., Biavati, G., Horányi, A., Muñoz Sabater, J., Nicolas, J., Peubey, C., Radu, R., Rozum, I., Schepers, D., Simmons, A., Soci, C., Dee, D., Thépaut, J.-N.: ERA5 monthly averaged data on single levels from 1940 to present, Copernicus Climate Change Service (C3S) Climate Data Store (CDS) [data set], https://doi.org/10.24381/cds.f17050d7, 2023.
Hourdin, F.: Study and numerical simulation of the general circulation of planetary atmospheres, PhD thesis, Paris VII University, https://theses.fr/1992PA077321 (last access: 1 October 2022), 1992.
Hugelius, G., Tarnocai, C., Broll, G., Canadell, J. G., Kuhry, P., and Swanson, D. K.: The Northern Circumpolar Soil Carbon Database: spatially distributed datasets of soil coverage and soil carbon storage in the northern permafrost regions, Earth Syst. Sci. Data, 5, 3–13, https://doi.org/10.5194/essd-5-3-2013, 2013.
Hugelius, G., Strauss, J., Zubrzycki, S., Harden, J. W., Schuur, E. A. G., Ping, C.-L., Schirrmeister, L., Grosse, G., Michaelson, G. J., Koven, C. D., O'Donnell, J. A., Elberling, B., Mishra, U., Camill, P., Yu, Z., Palmtag, J., and Kuhry, P.: Estimated stocks of circumpolar permafrost carbon with quantified uncertainty ranges and identified data gaps, Biogeosciences, 11, 6573–6593, https://doi.org/10.5194/bg-11-6573-2014, 2014.
Hugelius, G., Kuhry, P., and Tarnocai, C.: Ideas and perspectives: Holocene thermokarst sediments of the Yedoma permafrost region do not increase the northern peatland carbon pool, Biogeosciences, 13, 2003–2010, https://doi.org/10.5194/bg-13-2003-2016, 2016.
Hugelius, G., Loisel, J., Chadburn, S., Jackson, R. B., Jones, M., MacDonald, G., Marushchak, M., Olefeldt, D., Packalen, M., Siewert, M. B., Treat, C., Turetsky, M., Voigt, C., and Yu, Z.: Large stocks of peatland carbon and nitrogen are vulnerable to permafrost thaw, P. Natl. Acad. Sci. USA, 117, 20438–20446, https://doi.org/10.1073/pnas.1916387117, 2020.
Koven, C., Friedlingstein, P., Ciais, P., Khvorostyanov, D., Krinner, G., and Tarnocai, C.: On the formation of high-latitude soil carbon stocks: Effects of cryoturbation and insulation by organic matter in a land surface model, Geophys. Res. Lett., 36, L21501, https://doi.org/10.1029/2009GL040150, 2009.
Koven, C. D., Riley, W. J., Subin, Z. M., Tang, J. Y., Torn, M. S., Collins, W. D., Bonan, G. B., Lawrence, D. M., and Swenson, S. C.: The effect of vertically resolved soil biogeochemistry and alternate soil C and N models on C dynamics of CLM4, Biogeosciences, 10, 7109–7131, https://doi.org/10.5194/bg-10-7109-2013, 2013.
Krinner, G., Viovy, N., de Noblet-Ducoudré, N., Ogée, J., Polcher, J., Friedlingstein, P., Ciais, P., Sitch, S., and Prentice, I. C.: A dynamic global vegetation model for studies of the coupled atmosphere-biosphere system, Global Biogeochem. Cy., 19, GB1015, https://doi.org/10.1029/2003gb002199, 2005.
Lawrence, D. and Vandecar, K.: Effects of tropical deforestation on climate and agriculture, Nat. Clim. Change, 5, 27–36, https://doi.org/10.1038/nclimate2430, 2015.
Lawrence, D. M., Fisher, R. A., Koven, C. D., Oleson, K. W., Swenson, S. C., Bonan, G., Collier, N., Ghimire, B., van Kampenhout, L., Kennedy, D., Kluzek, E., Lawrence, P. J., Li, F., Li, H., Lombardozzi, D., Riley, W. J., Sacks, W. J., Shi, M., Vertenstein, M., Wieder, W. R., Xu, C., Ali, A. A., Badger, A. M., Bisht, G., van den Broeke, M., Brunke, M. A., Burns, S. P., Buzan, J., Clark, M., Craig, A., Dahlin, K., Drewniak, B., Fisher, J. B., Flanner, M., Fox, A. M., Gentine, P., Hoffman, F., Keppel-Aleks, G., Knox, R., Kumar, S., Lenaerts, J., Leung, L. R., Lipscomb, W. H., Lu, Y., Pandey, A., Pelletier, J. D., Perket, J., Randerson, J. T., Ricciuto, D. M., Sanderson, B. M., Slater, A., Subin, Z. M., Tang, J., Thomas, R. Q., Val Martin, M., and Zeng, X.: The Community Land Model Version 5: Description of New Features, Benchmarking, and Impact of Forcing Uncertainty, J. Adv. Model. Earth Sy., 11, 4245–4287, https://doi.org/10.1029/2018MS001583, 2019.
Li, H., Sun, D., Yu, Y., Wang, H., Liu, Y., Liu, Q., Du, Y., Wang, H., and Cao, B.: Evaluation of the VIIRS and MODIS LST products in an arid area of Northwest China, Remote Sens. Environ., 142, 111–121, https://doi.org/10.1016/j.rse.2013.11.014, 2014.
Li, R. and Arora, V. K.: Effect of mosaic representation of vegetation in land surface schemes on simulated energy and carbon balances, Biogeosciences, 9, 593–605, https://doi.org/10.5194/bg-9-593-2012, 2012.
Li, Y., Zhao, M., Motesharrei, S., Mu, Q., Kalnay, E., and Li, S.: Local cooling and warming effects of forests based on satellite observations, Nat. Commun., 6, 6603, https://doi.org/10.1038/ncomms7603, 2015.
Li, Y., Wang, T., Zeng, Z., Peng, S., Lian, X., and Piao, S.: Evaluating biases in simulated land surface albedo from CMIP5 global climate models, J. Geophys. Res.-Atmos., 121, 6178–6190, https://doi.org/10.1002/2016jd024774, 2016.
Li, Y., Brando, P. M., Morton, D. C., Lawrence, D. M., Yang, H., and Randerson, J. T.: Deforestation-induced climate change reduces carbon storage in remaining tropical forests, Nat. Commun., 13, 1964, https://doi.org/10.1038/s41467-022-29601-0, 2022.
Lindgren, A., Hugelius, G., and Kuhry, P.: Extensive loss of past permafrost carbon but a net accumulation into present-day soils, Nature, 560, 219–222, https://doi.org/10.1038/s41586-018-0371-0, 2018.
Lurton, T., Balkanski, Y., Bastrikov, V., Bekki, S., Bopp, L., Braconnot, P., Brockmann, P., Cadule, P., Contoux, C., Cozic, A., Cugnet, D., Dufresne, J.-L., Éthé, C., Foujols, M.-A., Ghattas, J., Hauglustaine, D., Hu, R.-M., Kageyama, M., Khodri, M., Lebas, N., Levavasseur, G., Marchand, M., Ottlé, C., Peylin, P., Sima, A., Szopa, S., Thiéblemont, R., Vuichard, N., and Boucher, O.: Implementation of the CMIP6 Forcing Data in the IPSL-CM6A-LR Model, J. Adv. Model. Earth Sy., 12, e2019MS001940, https://doi.org/10.1029/2019MS001940, 2020.
Melton, J. R. and Arora, V. K.: Sub-grid scale representation of vegetation in global land surface schemes: implications for estimation of the terrestrial carbon sink, Biogeosciences, 11, 1021–1036, https://doi.org/10.5194/bg-11-1021-2014, 2014.
Melton, J. R., Sospedra-Alfonso, R., and McCusker, K. E.: Tiling soil textures for terrestrial ecosystem modelling via clustering analysis: a case study with CLASS-CTEM (version 2.1), Geosci. Model Dev., 10, 2761–2783, https://doi.org/10.5194/gmd-10-2761-2017, 2017.
Nitzbon, J., Westermann, S., Langer, M., Martin, L. C. P., Strauss, J., Laboor, S., and Boike, J.: Fast response of cold ice-rich permafrost in northeast Siberia to a warming climate, Nat. Commun., 11, 2201, https://doi.org/10.1038/s41467-020-15725-8, 2020.
Niu, G.-Y. and Yang, Z.-L.: An observation-based formulation of snow cover fraction and its evaluation over large North American river basins, J. Geophys. Res.-Atmos., 112, D21101, https://doi.org/10.1029/2007JD008674, 2007.
Obu, J., Westermann, S., Kääb, A., and Bartsch, A.: Ground Temperature Map, 2000–2016, Northern Hemisphere Permafrost, Alfred Wegener Institute, Helmholtz Centre for Polar and Marine Research, Bremerhaven, PANGAEA [data set], https://doi.org/10.1594/PANGAEA.888600, 2018.
Obu, J., Westermann, S., Bartsch, A., Berdnikov, N., Christiansen, H. H., Dashtseren, A., Delaloye, R., Elberling, B., Etzelmüller, B., Kholodov, A., Khomutov, A., Kääb, A., Leibman, M. O., Lewkowicz, A. G., Panda, S. K., Romanovsky, V., Way, R. G., Westergaard-Nielsen, A., Wu, T., Yamkhin, J., and Zou, D.: Northern Hemisphere permafrost map based on TTOP modelling for 2000–2016 at 1 km2 scale, Earth-Sci. Rev., 193, 299–316, https://doi.org/10.1016/j.earscirev.2019.04.023, 2019.
Palmtag, J., Obu, J., Kuhry, P., Richter, A., Siewert, M. B., Weiss, N., Westermann, S., and Hugelius, G.: A high spatial resolution soil carbon and nitrogen dataset for the northern permafrost region based on circumpolar land cover upscaling, Earth Syst. Sci. Data, 14, 4095–4110, https://doi.org/10.5194/essd-14-4095-2022, 2022.
Peng, S., Piao, S., Zeng, Z., Ciais, P., Zhou, L., Li, L. Z. X., Myneni, R. B., Yin, Y., and Zeng, H.: Afforestation in China cools local land surface temperature, P. Natl. Acad. Sci. USA, 111, 2915–2919, https://doi.org/10.1073/pnas.1315126111, 2014.
Peng, S., Ciais, P., Krinner, G., Wang, T., Gouttevin, I., McGuire, A. D., Lawrence, D., Burke, E., Chen, X., Decharme, B., Koven, C., MacDougall, A., Rinke, A., Saito, K., Zhang, W., Alkama, R., Bohn, T. J., Delire, C., Hajima, T., Ji, D., Lettenmaier, D. P., Miller, P. A., Moore, J. C., Smith, B., and Sueyoshi, T.: Simulated high-latitude soil thermal dynamics during the past 4 decades, The Cryosphere, 10, 179–192, https://doi.org/10.5194/tc-10-179-2016, 2016.
Pinty, B., Andredakis, I., Clerici, M., Kaminski, T., Taberner, M., Verstraete, M. M., Gobron, N., Plummer, S., and Widlowski, J.-L.: Exploiting the MODIS albedos with the Two-stream Inversion Package (JRC-TIP): 1. Effective leaf area index, vegetation, and soil properties, J. Geophys. Res.-Atmos., 116, D09105, https://doi.org/10.1029/2010JD015372, 2011.
Qin, Y., Wang, D., Cao, Y., Cai, X., Liang, S., Beck, H. E., and Zeng, Z.: Sub-Grid Representation of Vegetation Cover in Land Surface Schemes Improves the Modeling of How Climate Responds to Deforestation, Geophys. Res. Lett., 50, e2023GL104164, https://doi.org/10.1029/2023GL104164, 2023.
Qiu, C., Zhu, D., Ciais, P., Guenet, B., Krinner, G., Peng, S., Aurela, M., Bernhofer, C., Brümmer, C., Bret-Harte, S., Chu, H., Chen, J., Desai, A. R., Dušek, J., Euskirchen, E. S., Fortuniak, K., Flanagan, L. B., Friborg, T., Grygoruk, M., Gogo, S., Grünwald, T., Hansen, B. U., Holl, D., Humphreys, E., Hurkuck, M., Kiely, G., Klatt, J., Kutzbach, L., Largeron, C., Laggoun-Défarge, F., Lund, M., Lafleur, P. M., Li, X., Mammarella, I., Merbold, L., Nilsson, M. B., Olejnik, J., Ottosson-Löfvenius, M., Oechel, W., Parmentier, F.-J. W., Peichl, M., Pirk, N., Peltola, O., Pawlak, W., Rasse, D., Rinne, J., Shaver, G., Schmid, H. P., Sottocornola, M., Steinbrecher, R., Sachs, T., Urbaniak, M., Zona, D., and Ziemblinska, K.: ORCHIDEE-PEAT (revision 4596), a model for northern peatland CO2, water, and energy fluxes on daily to annual scales, Geosci. Model Dev., 11, 497–519, https://doi.org/10.5194/gmd-11-497-2018, 2018.
Qiu, C., Zhu, D., Ciais, P., Guenet, B., Peng, S., Krinner, G., Tootchi, A., Ducharne, A., and Hastie, A.: Modelling northern peatland area and carbon dynamics since the Holocene with the ORCHIDEE-PEAT land surface model (SVN r5488), Geosci. Model Dev., 12, 2961–2982, https://doi.org/10.5194/gmd-12-2961-2019, 2019.
Rooney, G. G. and Jones, I. D.: Coupling the 1D lake model Flake to the community land surface model JULES, Boreal Environ. Res., 15, 501–512, 2010.
Rumbold, H. S., Gilham, R. J. J., and Best, M. J.: Assessing methods for representing soil heterogeneity through a flexible approach within the Joint UK Land Environment Simulator (JULES) at version 3.4.1, Geosci. Model Dev., 16, 1875–1886, https://doi.org/10.5194/gmd-16-1875-2023, 2023.
Running, S., Mu, Q., Zhao, M., and Moreno, A.: MODIS/Terra Net Evapotranspiration Gap-Filled 8-Day L4 Global 500m SIN Grid V061, NASA EOSDIS Land Processes Distributed Active Archive Center [data set], https://doi.org/10.5067/MODIS/MOD16A2GF.061, 2021.
Schaaf, C. and Wang, Z.: MODIS/Terra+Aqua BRDF/Albedo Albedo Daily L3 Global 0.05Deg CMG V061, NASA EOSDIS Land Processes Distributed Active Archive Center [data set], https://doi.org/10.5067/MODIS/MCD43C3.061, 2021.
Sherstiukov, A.: Statistical quality control of soil temperature dataset, RIHMI-WDC, 176, 224–232, http://meteo.ru/data/ (last access: 1 May 2023), 2012.
Smith, N. D., Burke, E. J., Schanke Aas, K., Althuizen, I. H. J., Boike, J., Christiansen, C. T., Etzelmüller, B., Friborg, T., Lee, H., Rumbold, H., Turton, R. H., Westermann, S., and Chadburn, S. E.: Explicitly modelling microtopography in permafrost landscapes in a land surface model (JULES vn5.4_microtopography), Geosci. Model Dev., 15, 3603–3639, https://doi.org/10.5194/gmd-15-3603-2022, 2022.
Su, Y., Zhang, C., Ciais, P., Zeng, Z., Cescatti, A., Shang, J., Chen, J. M., Liu, J., Wang, Y.-P., Yuan, W., Peng, S., Lee, X., Zhu, Z., Fan, L., Liu, X., Liu, L., Lafortezza, R., Li, Y., Ren, J., Yang, X., and Chen, X.: Asymmetric influence of forest cover gain and loss on land surface temperature, Nat. Clim. Change, 13, 823–831, https://doi.org/10.1038/s41558-023-01757-7, 2023.
Wan, Z.: Collection-6 MODIS Land Surface Temperature ProductsUsers' Guide, Earth Research Institute, University of California, Santa Barbara, https://lpdaac.usgs.gov/documents/118/MOD11_User_Guide_V6.pdf (last access: 3 March 2023), 2013.
Wan, Z.: New refinements and validation of the collection-6 MODIS land-surface temperature/emissivity product, Remote Sens. Environ., 140, 36–45, https://doi.org/10.1016/j.rse.2013.08.027, 2014.
Wan, Z., Hook, S., and Hulley, G.: MODIS/Terra Land Surface Temperature/Emissivity Monthly L3 Global 0.05Deg CMG V061, NASA EOSDIS Land Processes Distributed Active Archive Center [data set], https://doi.org/10.5067/MODIS/MOD11C3.061, 2021.
Wang, T., Ottlé, C., Boone, A., Ciais, P., Brun, E., Morin, S., Krinner, G., Piao, S., and Peng, S.: Evaluation of an improved intermediate complexity snow scheme in the ORCHIDEE land surface model, J. Geophys. Res.-Atmos., 118, 6064–6079, https://doi.org/10.1002/jgrd.50395, 2013.
Wang, T., Peng, S., Krinner, G., Ryder, J., Li, Y., Dantec-Nédélec, S., and Ottlé, C.: Impacts of Satellite-Based Snow Albedo Assimilation on Offline and Coupled Land Surface Model Simulations, PLOS ONE, 10, e0137275, https://doi.org/10.1371/journal.pone.0137275, 2015.
Westermann, S., Langer, M., and Boike, J.: Systematic bias of average winter-time land surface temperatures inferred from MODIS at a site on Svalbard, Norway, Remote Sens. Environ., 118, 162–167, https://doi.org/10.1016/j.rse.2011.10.025, 2012.
Xi, Y: Model code for ORCHIDEE-MICT-teb, IPSL data catelog [code], https://doi.org/10.14768/0954a0e9-6a7a-4006-803e-4db36ef2db88, 2023a.
Xi, Y.: Code for GMD paper: Assessment of a multi-tiling energy budget approach in a land surface model, ORCHIDEE-MICT (r8205), Zenodo [code], https://doi.org/10.5281/zenodo.10014533, 2023b.
Xu, J., Morris, P., Liu, J., and Holden, J.: PEATMAP: Refining estimates of global peatland distribution based on a meta-analysis, Catena, 160, 134–140, https://doi.org/10.1016/j.catena.2017.09.010, 2018.
Zhang, T.: Influence of the seasonal snow cover on the ground thermal regime: An overview, Rev. Geophys., 43, RG4002, https://doi.org/10.1029/2004RG000157, 2005.
Zhu, D., Peng, S. S., Ciais, P., Viovy, N., Druel, A., Kageyama, M., Krinner, G., Peylin, P., Ottlé, C., Piao, S. L., Poulter, B., Schepaschenko, D., and Shvidenko, A.: Improving the dynamics of Northern Hemisphere high-latitude vegetation in the ORCHIDEE ecosystem model, Geosci. Model Dev., 8, 2263–2283, https://doi.org/10.5194/gmd-8-2263-2015, 2015.
Zhu, D., Peng, S., Ciais, P., Zech, R., Krinner, G., Zimov, S., and Grosse, G.: Simulating soil organic carbon in yedoma deposits during the Last Glacial Maximum in a land surface model, Geophys. Res. Lett., 43, 5133–5142, https://doi.org/10.1002/2016GL068874, 2016.
Zhu, D., Ciais, P., Krinner, G., Maignan, F., Jornet Puig, A., and Hugelius, G.: Controls of soil organic matter on soil thermal dynamics in the northern high latitudes, Nat. Commun., 10, 3172, https://doi.org/10.1038/s41467-019-11103-1, 2019.
Zhu, L., Li, W., Ciais, P., He, J., Cescatti, A., Santoro, M., Tanaka, K., Cartus, O., Zhao, Z., Xu, Y., Sun, M., and Wang, J.: Comparable biophysical and biogeochemical feedbacks on warming from tropical moist forest degradation, Nat. Geosci., 16, 244–249, https://doi.org/10.1038/s41561-023-01137-y, 2023.
- Abstract
- Introduction
- Overview of the current energy budget in ORCHIDEE-MICT
- Implementation of tiling energy budgets in ORCHIDEE-MICT-teb
- Simulation protocol and forcing datasets
- Evaluation of the impacts of the tiling energy budget on energy, hydrology, and carbon processes
- Evaluation and potential application
- Conclusion
- Code and data availability
- Author contributions
- Competing interests
- Disclaimer
- Acknowledgements
- Financial support
- Review statement
- References
- Supplement
- Abstract
- Introduction
- Overview of the current energy budget in ORCHIDEE-MICT
- Implementation of tiling energy budgets in ORCHIDEE-MICT-teb
- Simulation protocol and forcing datasets
- Evaluation of the impacts of the tiling energy budget on energy, hydrology, and carbon processes
- Evaluation and potential application
- Conclusion
- Code and data availability
- Author contributions
- Competing interests
- Disclaimer
- Acknowledgements
- Financial support
- Review statement
- References
- Supplement