the Creative Commons Attribution 4.0 License.
the Creative Commons Attribution 4.0 License.
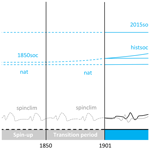
Scenario setup and forcing data for impact model evaluation and impact attribution within the third round of the Inter-Sectoral Impact Model Intercomparison Project (ISIMIP3a)
Katja Frieler
Jan Volkholz
Stefan Lange
Jacob Schewe
Matthias Mengel
María del Rocío Rivas López
Christian Otto
Christopher P. O. Reyer
Dirk Nikolaus Karger
Johanna T. Malle
Simon Treu
Christoph Menz
Julia L. Blanchard
Cheryl S. Harrison
Colleen M. Petrik
Tyler D. Eddy
Kelly Ortega-Cisneros
Camilla Novaglio
Yannick Rousseau
Reg A. Watson
Charles Stock
Xiao Liu
Ryan Heneghan
Derek Tittensor
Olivier Maury
Matthias Büchner
Thomas Vogt
Tingting Wang
Fubao Sun
Inga J. Sauer
Johannes Koch
Inne Vanderkelen
Jonas Jägermeyr
Christoph Müller
Sam Rabin
Jochen Klar
Iliusi D. Vega del Valle
Gitta Lasslop
Sarah Chadburn
Eleanor Burke
Angela Gallego-Sala
Noah Smith
Jinfeng Chang
Stijn Hantson
Chantelle Burton
Anne Gädeke
Simon N. Gosling
Hannes Müller Schmied
Fred Hattermann
Jida Wang
Fangfang Yao
Thomas Hickler
Rafael Marcé
Don Pierson
Wim Thiery
Daniel Mercado-Bettín
Robert Ladwig
Ana Isabel Ayala-Zamora
Matthew Forrest
Michel Bechtold
This paper describes the rationale and the protocol of the first component of the third simulation round of the Inter-Sectoral Impact Model Intercomparison Project (ISIMIP3a, http://www.isimip.org, last access: 2 November 2023) and the associated set of climate-related and direct human forcing data (CRF and DHF, respectively). The observation-based climate-related forcings for the first time include high-resolution observational climate forcings derived by orographic downscaling, monthly to hourly coastal water levels, and wind fields associated with historical tropical cyclones. The DHFs include land use patterns, population densities, information about water and agricultural management, and fishing intensities. The ISIMIP3a impact model simulations driven by these observation-based climate-related and direct human forcings are designed to test to what degree the impact models can explain observed changes in natural and human systems. In a second set of ISIMIP3a experiments the participating impact models are forced by the same DHFs but a counterfactual set of atmospheric forcings and coastal water levels where observed trends have been removed. These experiments are designed to allow for the attribution of observed changes in natural, human, and managed systems to climate change, rising CH4 and CO2 concentrations, and sea level rise according to the definition of the Working Group II contribution to the IPCC AR6.
- Article
(4542 KB) - Full-text XML
- BibTeX
- EndNote
The Inter-Sectoral Impact Model Intercomparison Project ISIMIP (http://www.isimip.org, last access: 2 November 2023) provides a common scenario framework for cross-sectorally consistent climate impact simulations currently covering the following sectors: agriculture (global, in cooperation with AgMIP's Global Gridded Crop Model Intercomparison Project – GGCMI, water – global and regional, lakes – global and regional, biomes – global, forest – regional, fisheries and marine ecosystems – global and regional, terrestrial biodiversity – global, fire – global, permafrost – global, peat – global, coastal systems – global, energy – global, health: temperature-related mortality, waterborne diseases, vector-borne diseases, and food security and nutrition – global and local, labor productivity – global and local). The impact model simulations are made freely available, allowing for all types of follow-up analysis. The consistent design of the simulations does allow for the comparison of climate impact simulations within each sector. However, it also enables the bottom-up integration of impacts across sectors. Thus, it provides a unique basis for the estimation of the effects of climate change on, e.g., the economy, displacement and migration, health, or water quality, resolving the mechanisms along different impact channels and fully exploiting the process understanding represented in the biophysical impact models.
Initialized in 2012, ISIMIP is organized in individual modeling rounds. The decision about their design and the development of the associated simulation protocols has been developed into an iterative process between stakeholders and users of ISIMIP data, the sectoral coordinators representing participating modeling teams, the Scientific Advisory Board, and the Cross-Sectoral and Coordination Team at PIK (ISIMIP Coordination Team, Sectoral Coordinators, Scientific Advisory Board, 2018). Since its second round the ISIMIP protocols has comprised an “a” part describing impact model simulations that cover the historical period forced by observational climate-related and direct human forcings (evaluation setup) and a “b” part dedicated to impact simulations based on simulated climate-related forcings including future projections. This paper describes the ISIMIP3a simulation framework only where the DHFs described here are also used for the historical simulations within ISIMIP3b. Compared to ISIMIP2a the evaluation setup based on observational forcing data has been extended to now include additional years up to 2021 and sensitivity experiments using high-resolution historical climate forcing data to quantify associated improvements of impact simulations (see Sect. 3.1). Besides, the set of historical observation-based direct human forcings has been updated compared to previous ISIMIP simulation rounds (see Table 1). For the first time, and closely connected to the evaluation setup, ISIMIP3a now also includes an “impact attribution” scenario setup designed to address the following question: to what degree have observed changes in the climate-related systems contributed to observed changes in natural, human, or managed systems compared to direct human influences? Here, changes in climate-related systems mean climate change itself, changes in atmospheric CO2 and CH4 concentration, and sea level changes. The attribution question can refer to both the impacts of individual events (e.g., to what extent has long-term climate change contributed to the observed extent of a specific river flood?) and to long-term changes (e.g., to what extent have long-term climate change and increasing CO2 fertilization contributed to an observed change in crop yields?). The IPCC AR5 (Cramer et al., 2014) and AR6 (O'Neill et al., 2022; Hope et al., 2022) have established a framework for impact attribution according to which an “observed impact of climate change or change in any other climate-related system” is defined as the difference between the observed state of the human, natural, or managed system and a counterfactual baseline that characterizes the system's behavior in the absence of changes in the climate-related systems. This counterfactual baseline may be stationary or vary in response to direct human influences such as changes in land use patterns, agricultural or water management, or population distribution and economic development affecting exposure and vulnerability to weather-related hazards. While the definition has been established for about a decade, the number of studies addressing impact attribution based on this basic definition is still relatively small compared to the number of studies addressing climate attribution, i.e., the question of to what degree anthropogenic emissions of climate forcers, in particular greenhouse gases, have induced changes in climate-related systems. While climate attribution is mainly confronted by the challenge of separating anthropogenically forced changes from the internal variability of climate-related systems, the focus of climate impact attribution is on separating the impacts of observed changes in these climate-related systems from the effects of other direct (human) drivers of changes in the considered natural, human, or managed systems. Use of the phrase “observed changes in the climate-related systems” does not necessarily imply changes induced by anthropogenic climate forcing but only means any long-term trend, in line with the IPCC definition of climate change (see Glossary of the AR5 – IPCC, 2014; AR6 – Matthews et al., 2021).
Impact attribution studies usually face the problem that the counterfactual baseline assuming no long-term changes in the climate-related systems cannot be observed (see Hansen et al., 2016, for examples). However, impact models such as the ones participating in ISIMIP are well suited to simulate this baseline. Impact models usually account not only for changes in climate or climate-related systems but also for direct human forcings such as land use and irrigation changes, changes in water and agricultural management, and population distributions (see Table 1 for a comprehensive list of direct human forcings provided within ISIMIP3a); they are ideal tools to address the attribution question: in line with the IPCC definition it requires the comparison of a factual simulation based on the observed variations in the climate-related and direct human drivers to a counterfactual simulation where only the climate-related forcings are replaced by counterfactual versions from which long-term trends have been removed. While the factual simulations correspond to the evaluation runs within ISIMIP3a (see Sect. 2.1), the protocol now also includes counterfactual simulations based on the newly generated counterfactual datasets derived from observational data on climate and coastal water levels (see Sect. 2.2 for the associated concept and scenario design and Table 3 for a comprehensive list of the counterfactual climate and sea level forcing data that are described in more detail in Sect. 3.1 and 3.3, respectively). To allow for an attribution of observed changes in natural, human, and managed systems in contrast to an attribution of simulated changes it has to be demonstrated that the processes represented in the impact model can explain the observed changes in the affected system; i.e., it has to be shown that the model forced by observed changes in the climate-related systems and accounting for the historical development of direct (human) forcings is able to reproduce the observed changes in the affected system. In this way the attribution exercise is closely linked to the ISIMIP3a evaluation exercise. Thereby, models can either explicitly represent known changes in non-climate drivers such as known adjustments of fertilizer input or growing seasons (explicit accounting for non-climate drivers) or implicitly account for their potential contributions by, e.g., allowing for non-climate-related temporal trends in empirical models as often done in empirical approaches (implicitly accounting for non-climate drivers).
While the default attribution experiment in ISIMIP3a is designed for the attribution of observed changes in human, natural, and managed systems to observed change in climate-related systems in combination (in the current ISIMIP3a setting this means changes in atmospheric climate forcing in combination with changes in atmospheric CO2 and CH4 concentrations, see Table 3), the protocol also includes sensitivity experiments that allow for the quantification of the influence of increasing CO2 concentrations separately and for an attribution of observed changes in natural, human, and managed systems to historical changes in atmospheric CO2 concentrations only (see Sect. 2.1). Here, we consistently define an observed impact of a change in any component of the historical forcing as the difference between the observed state of the system to a counterfactual world where only this specific component of the forcing has not changed. So the observed impact of increasing CO2 concentrations is approximated by the difference between a full forcing run and a run where CO2 concentrations are held constant. This is different from the CO2-only experiment considered within TRENDY (Trends in the land carbon cycle – TRENDY; Sitch et al., 2015 2023) where the pure effect of increasing CO2 concentrations on the terrestrial carbon cycle (e.g., net biome production) is estimated by simulations where dynamic global vegetation models (as participating in the biomes sector of ISIMIP) are forced by the observed increases in CO2 concentrations but a time-invariant “pre-industrial” climate and land use mask. In the above sense, other ISIMIP3a experiments can also be considered counterfactual baseline experiments that allow for the attribution of observed changes in human, natural, or managed systems to changes in the direct human forcings as a whole (DHF set to zero or fixed at 1901 and 2015 levels) or to changes in individual components such as changes in water management, irrigation patterns, and riverine influx of nutrients into the ocean (see Sect. 2.1 and Table 2). The attribution to changes in direct human forcings is, e.g., similar to the comparison of the full forcing run within TRENDY to the CO2- and climate-only run where climate change and atmospheric CO2 concentrations are prescribed according to observations but land use changes are held constant to quantify the contribution of this direct human forcing to observed changes in the carbon cycle for the annual report of the Global Carbon Project (e.g., Friedlingstein et al., 2022). However, in this paper the term impact attribution is used as a short form for attribution of observed changes in natural, human, and managed systems to observed changes in the climate-related systems, which is the focus of the ISIMIP3a experiments. In other cases the driver to which the changes are attributed is explicitly named. In addition to ISIMIP3a, there are other model intercomparison projects that address different kinds of attribution questions such as the Land Use Model Intercomparison Project (LUMIP; Lawrence et al., 2016) and the Detection and Attribution Model Intercomparison Project (DAMIP, Gillett et al., 2016) embedded into the sixth phase of the Coupled Model Intercomparison Project (CMIP6). While the phase 2 LUMIP experiments include historical climate model simulations to quantify the contribution of historical land use changes to observed climate change, the AMIP protocol includes a counterfactual “no anthropogenic climate forcing” baseline to attribute observed changes in climate to anthropogenic climate forcings.
The development of the protocol was coordinated by the ISIMIP Cross-Sectoral Science Team (CSST) at the Potsdam Institute for Climate Impact Research (PIK) and involved the sectoral coordinators, participating modeling teams, and the Scientific Advisory Board. The process was initiated by a proposal for the main research questions to be addressed and an associated scenario setup accounting for suggestions collected in a stakeholder engagement process (Lejeune et al., 2018). Following ISIMIP's mission and implementation document (ISIMIP Coordination Team, Sectoral Coordinators, Scientific Advisory Board, 2018), the basic proposal was approved by the ISIMIP strategy group at the cross-sectoral ISIMIP workshop in Potsdam in September 2018 (Outcomes of the ISIMIP Strategy Group Meeting, 2023). Thereby the CSST and the sectoral coordinators were tasked with translating the decisions into a cross-sectorally consistent simulation protocol and with generating, pre-processing, or collecting the required climate-related and direct human forcing data. The provided forcing datasets (e.g., the climate variables or components of atmospheric composition or types of land use) are very much demand-driven. The data we describe here represent a core set that is sufficient for the range of models participating so far (see ISIMIP the output data table that also provides information about the input data used by the individual models; ISIMIP Output Data Table, 2023) but may be extended if there are further demands. This paper presents the results of this process and the motivation and reasoning behind the individual steps for ISIMIP3a, while a follow-up paper will provide the same information for ISIMIP3b dedicated to impact projections based on climate model simulations (Frieler, 2024). It provides the point of reference for modeling teams interested in participating in ISIMIP3a but also for users of the impact simulation data, which will become freely accessible according to the ISIMIP terms of use (ISIMIP terms of use, 2023). The paper is accompanied by a simulation protocol (ISIMIP3 simulation protocol, 2023) providing all technical details such as file and variable naming conventions and sector-specific lists of output variables to be reported by the participating modeling teams. The ISIMIP3 simulation round was officially started on 21 February 20201 with the release of the associated protocol. Since then, the protocol has already received some updates through the addition of output variables, correction of errors, and inclusion of new sectors. This paper refers to the protocol version of 14 January 2023. However, the protocol may still receive updates similar to the ones mentioned above. Impact modelers interested in contributing to ISIMIP should therefore refer to the ISIMIP3 simulation protocol (2023) for the most up-to-date version for planned impact model simulations. The protocol landing page (http://protocol.isimip.org, last access: 2 November 2023) includes a unique version identifier (the commit hash) that links to the latest protocol version on GitHub for traceability.
In the second round of ISIMIP the observation-based model evaluation part (ISIMIP2a) was temporally separate from the climate-model-based second part (ISIMIP2b, (Frieler et al., 2017). This has led to inconsistencies in the models and model versions contributing to ISIMIP2a and ISIMIP2b. Also, not all models providing future projections within ISIMIP2b also provided model evaluation runs for ISIMIP2a. To avoid this problem and ensure that each model's set of future projections is accompanied by associated historical simulations allowing for model evaluation, in the third simulation round (ISIMIP3), the ISMIP3a and ISIMIP3b protocols were released together and participating in ISIMIP3 means contributing to ISIMIP3a and ISIMIP3b using the same impact model versions.
In the following Sect. 2 of this paper, we provide a comprehensive list of all ISIMIP3a model evaluation and sensitivity experiments (see Table 2 within Sect. 2.1); the counterfactual no-climate-change experiments (see Table 4 within Sect. 2.2) describe the rationale behind the scenario setups. Detailed descriptions of the climate-related forcing datasets (see CRF section of Table 1 in Sect. 2.1 and Table 3 in Sect. 2.2) are provided in the third section: atmospheric climate data (see Sect. 3.1), tropical cyclone data (see Sect. 3.2), coastal water levels (see Sect. 3.3), and ocean data (see Sect. 3.4). Section 4 presents the ISIMIP3a direct human forcing datasets (see DHF section of Table 1), comprising population data (see Sect. 4.1), gross domestic product (see Sect. 4.2), land use and irrigation patterns (see Sect. 4.3), fertilizer inputs (see Sect. 4.4), land transformations (see Sect. 4.5), nitrogen deposition (see Sect. 4.6), crop calendar (see Sect. 4.7), dams and reservoirs (see Sect. 4.8), fishing intensities (see Sect. 4.9), regional forest management (see Sect. 4.10), and desalination (see Sect. 4.11).
ISIMIP3a includes a core (“default”) set of experiments that are specified by a specific set of underlying climate-related forcings and direct human forcings that have to be indicated in the file names when submitting simulation data to the ISIMIP repository. In the following we first introduce these default experiments by defining the combination of the two types of forcing datasets. In the subheadings naming the experiments the associated CRF and DHF specifiers to be used in the file names are indicated in brackets where the third sensitivity specifier is set to default (CRF specifier + DHF specifier; default). The different combinations of the default sets of ISIMIP3a CRFs (obsclim, counterclim) and DHFs (histsoc, 2015soc, 1901soc, 1850soc, nat) are sketched in Fig. 1 and defined in more detail below (see Table 1 for the default obsclim CRF and the default DHFs and Table 3 for the counterclim CRF). Some of the forcing datasets are mandatory: i.e., if impact models account for the forcing, the specified dataset must be used; if an alternative input dataset is used instead, the run cannot be considered an ISIMIP simulation. We also provide “optional” forcing data that could be used but are not “mandatory” in the above sense (see second column of Tables 1 and 3). In addition, the protocol includes a set of sensitivity experiments that are described as deviations from the default runs and labeled by the baseline CRF and DHF settings, with the third specifier then indicating the deviation from this default setting instead of being set to default. The ISIMIP3a sensitivity runs include experiments with high-resolution climate forcing (30arcsec, 90arcsec, 300arcsec, or 1800arcsec), fixed levels of atmospheric CO2 concentrations (1901co2), a scenario assuming no water management (nowatermgt), simulations excluding the occurrence of wildfires (nofire), keeping irrigation patterns at 1901 levels (1901irr), and assuming fixed 1955 riverine inputs of freshwater and nutrients into the ocean (1955-riverine-input) (see Table 2). Tables 2 and 4 provide a comprehensive list of all obsclim- and counterclim-based experiments, respectively, and also indicate the priority of the experiments where “first priority” means that modelers should focus on this set of experiments if their capacities are limited and they want to limit the set of experiments. However, this is just an indication trying to ensure the generation of a small set of experiments that is covered by as many impact models as possible. If an impact modeler can only do part of the first priority setup or has to start from second-priority simulations these fragmented datasets can also be submitted to the ISIMIP3a repository.
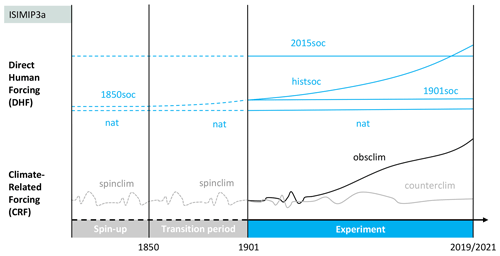
Figure 1ISIMIP3a scenario design: illustration of the default ISIMIP3a forcing datasets. Each experiment is defined by a combination of a CRF dataset with a DHF dataset. The considered combinations are listed in Tables 2 and 4, and the underlying rationale is described in Sect. 2.1 (evaluation runs based on “obsclim” defined in Table 1) and Sect. 2.2 (attribution runs based on “counterclim” defined in Table 3). Table 1 also lists all datasets defining the “histsoc” DHF. Solid lines indicate the part of the experiments that should be reported, while the dashed lines illustrate the different spin-up procedures for the models that require a spin-up. Note that the oceanic climate-related forcing for the marine ecosystems and fisheries sector is only available for obsclim and the period 1961–2010; i.e., the actual experiments only start from the year 1961. The associated spin-up procedure and the simulation setup for a transition period are not illustrated in the figure but are described below for the “obsclim + histsoc; default”, “obsclim + nat; default”, “obsclim + histsoc; 60arcmin”, and “obsclim + nat; 60arcmin” experiments considered in this sector.
2.1 Model evaluation and sensitivity experiments based on observed CRFs (obsclim)
The experiments described in this section are all based on observational (factual) climate data, coastal water levels, and atmospheric CO2 as well as CH4 concentrations including observed trends. The only exception are the sensitivity experiments where CO2 concentrations are fixed at 1901 levels (1901co2). However, as these experiments only deviate in this one aspect from the factual CRF they are also described by the obsclim CRF specifier but the 1901co2 sensitivity specifier to indicate the deviation. So all experiments described in this section share the common obsclim CRF specifier in the file names. In contrast, all experiments described in Sect. 2.2 can be identified by the counterclim specifier in the names of the output files containing the impact model simulations.
2.1.1 Default evaluation experiments based on observed CRFs (obsclim)
In this first part of Sect. 2.1 we describe the default ISIMIP3a experiments (sensitivity specifier in the file names set to default) that are based on the standard observed climate-related forcings (obsclim, see CRF part of Table 1) in combination with different assumptions regarding direct human forcings (histsoc, 2015soc, 1901soc, and nat) illustrated in Fig. 1.
Standard evaluation experiment (obsclim + histsoc; default). The first set of observation-based simulations is dedicated to impact model evaluation, i.e., to test our ability to reproduce and explain observed long-term changes or variations in impact indicators such as crop yields, river discharge, changes in natural vegetation carbon, vegetation types, and peatland moisture conditions. To this end, we provide the climate-related (obsclim), direct human (histsoc), and static geographical forcings listed in Table 1. They are described in more detail in Sects. 3 and 4.
For impact model simulations that require a spin-up to, e.g., balance carbon stocks, 100 years of climate data (spinclim) are provided that represent stable 1900 climate conditions. The spinclim data are equivalent to the first 100 years of the counterfactual climate data that are described in Sect. 3.1. If more than 100 years of spin-up are needed, the spinclim data can be repeated as often as needed. For the spin-up, CO2 concentrations and direct human forcing should be kept constant at 1850 levels. To get to the historical reporting period starting in 1901, modelers should simulate a transition period from 1850 to 1900 using spinclim climate data and the observed increase in CO2 concentrations and historical changes in socioeconomic forcings (from 1850–1900).
The temporal coverage of the evaluation experiment is limited to 1961–2010 in the marine ecosystems and fisheries sector due to the availability of reanalysis-based oceanic forcing data (Liu et al., 2021). As a spin-up + transition period for the “obsclim + histsoc; default” experiments starting in 1961 the models should be run through six cycles of 1961–1980 1955-riverine-input CRFs (120 years, see Table 1) assuming reconstructed fishing efforts from 1861–1960 and constant 1861 levels before during 1841–1860 (see Table 1 and Fig. 3 in Sect. 4.9). If more years of spin-up are required, additional cycles of the 1961–1980 1955-riverine-input CRFs should be added, assuming constant 1861 fishing efforts.
Table 1Climate-related, direct human, and static geographic forcing data provided for the model evaluation and sensitivity experiments within ISIMIP3a. The CRFs are grouped according to the definition of the default obsclim CRF (30 arcmin for the atmospheric data and 15 arcmin for the oceanic data); the higher-resolution 30arcsec, 90arcsec, 300arcsec, and 1800arcsec atmospheric CRF; the lower-resolution 60arcmin oceanic CRF; and the 1955-riverine-input oceanic CRF for the sensitivity experiments. The listed set of DHFs defines the histsoc setup.
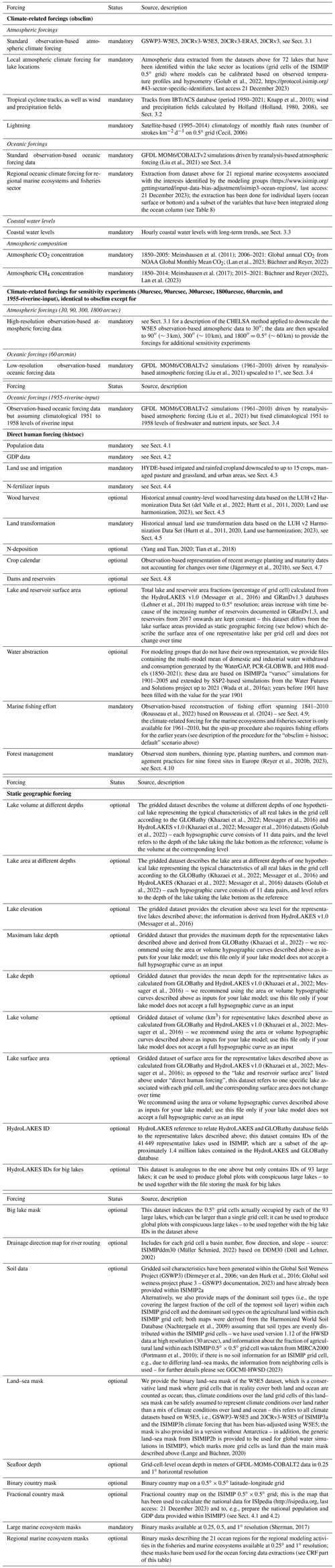
Fixed 2015 direct human forcing (obsclim + 2015soc; default). To allow for the quantification of the effect of historical changes in direct human forcings, ISIMIP3a also contains an experiment where all direct human forcings are held constant at 2015 levels. The difference between the evaluation run described above and this baseline simulation can be considered the impact of changes in direct human forcings. In this sense the experiment allows for the attribution of observed changes in the natural, human, and managed systems to changes in DHF after 2015. In addition, the simulated changes in models' output variables can be considered the “pure effects of climate-related forcings”, conditional on present-day socioeconomic conditions. The experiment is also introduced because not all impact models can account for varying direct human forcings but rather assume fixed “present-day” conditions. All modeling teams are asked to do this experiment even if they are able to account for varying direct human forcings to generate one set of impact simulations that can be integrated across all participating models from different sectors or where all simulations from one sector can be compared. If a spin-up is required, it should be based on the spinclim data as described above but fixed 2015 direct human forcings.
Fixed 1901 direct human forcing baseline (obsclim + 1901soc; default). Fixing direct human forcings at 1901 levels is an alternative approach to quantify (i) the effects of direct human forcings when comparing these baseline simulations to the evaluation run and (ii) the “pure effect of observed change in climate-related systems”, conditional on socioeconomic conditions observed before the onset of this change. As such the experiment is the counterfactual baseline when aiming for the attribution of observed changes in natural, human, and managed systems to observed changes in direct human forcings instead of the attribution to observed changes in climate-related systems based on the analogous “counterfactual + histsoc; default” experiment described in Sect. 2.2. Both experiments consider changes in direct human forcings or climate-related systems from 1901 levels, respectively. Because of the low levels of direct human forcings in 1901, this experiment is similar to the sector-specific “nat” experiment that includes no direct human forcings whatsoever (see below). However, while the fully naturalized nat run is suitable for dynamic vegetation models from the biomes sector that simulate land cover by vegetation on their own, models in other sectors need land cover as an input. As this information is not available for pristine conditions, we introduce the 1901soc scenario such that models in the water sector can use land cover data approximately representative of 1901 conditions to describe a situation with minor human influences. If a spin-up is required, it should be based on the spinclim data as described above but fixed 1901 direct human forcings.
No direct human forcing baseline (obsclim + nat; default). To estimate the full effect of 2015 levels of DHF we also introduce a baseline nat experiment that does not consider any DHFs but a natural state of the world. Then the difference to the “obsclim + 2015soc; default” experiment can be considered the effect of 2015 levels of DHF. The comparison to the “obsclim + histsoc; default” experiment allows for the attribution of observed changes in the natural, human, and managed systems to historical changes in the DHF. Trends in the “obsclim + nat; default” run only represent the impacts historical changes in the climate-related forcings would have had on an otherwise natural state of the world. While the 1901soc conditions may be similar to nat conditions, trends in the “obsclim + 1901soc; default” run may not only be induced by historical changes in the CRFs but could also represent lagged responses to changes in DHFs during the transition period. The nat experiment can also be used to quantify the natural carbon sequestration potential of natural vegetation without any management or land use as an important counterfactual baseline to assess the addition of carbon sequestration measures. The nat experiment is sector-specific for the biomes–peat and marine ecosystems–fisheries sectors. If a spin-up is required in the biomes and peat sector, it should be based on the spinclim data as described above but assuming no direct human forcings. In the marine ecosystems and fisheries sector the spin-up should be based on the 1955-riverine-input CRF as described for the “obsclim + histsoc; default” section but assuming no DHF, i.e., no fishing efforts.
2.1.2 Sensitivity experiments based on observed CRFs (obsclim)
This second part of Sect. 2.1 is dedicated to the different sensitivity experiments described as deviations from the default cases described in Sect. 2.1.1. Instead of the default specifier, all experiments described here are labeled by sensitivity specifiers indicating their deviation from the default cases. The experiments listed here are not explicitly depicted in Fig. 1.
High- and low-resolution sensitivity experiments (obsclim + histsoc; 30arcsec, 90arcsec, 300arcsec, 1800arcsec, and 60arcmin). To test whether high-resolution atmospheric climate data improve the climate impact model simulations, we also provide observational atmospheric forcing data at 30′′ (30arcsec), 90′′ (90arcsec), and 300′′ (300arcsec) resolution as well as atmospheric forcings at the original 1800′′ resolution but derived from the 30′′ (∼1 km) data (1800arcsec). In addition, the oceanic data (original resolution of 0.25∘) are upscaled to 1∘ to also test the sensitivity of the impact simulations to this modification (60arcmin).
The 30′′ atmospheric data (1979–2016) are derived from a topographic downscaling of the observational W5E5 data (resolution of 0.5∘) that particularly corrects for systematic effects induced by orographic details not represented in global reanalyses (CHELSA-W5E5, see Sect. 3.1). The dataset comprises daily mean precipitation, daily mean surface downwelling shortwave radiation, daily mean near-surface air temperature, daily maximum near-surface air temperature, and daily minimum near-surface air temperature (see Table 5). We additionally provide simple approaches to downscale surface downwelling longwave radiation, near-surface relative humidity, air pressure, and near-surface wind speed (see Sect. 3.1). Given the considerable storage capacities required by daily 1 km × 1 km data and constraints on data handling and download, we also aggregate the CHELSA-W5E5 data to 90′′ (∼3 km), 300′′ (∼10 km), and 1800∘ (∼60 km) to determine which resolution is required to improve the impact model simulations compared to observed impact indicators. The evaluation of these historical sensitivity experiments will inform future downscaling activities for GCM climate forcing data including future projections. The 1800arcsec experiment is included as a reference, as the aggregated CHELSA-W5E5 data differ from the standard W5E5 data at the same resolution (see Sect. 3.1). So far the experiments have been added to the agriculture, lakes, global and regional water, regional forests, terrestrial biodiversity, and labor protocol. However, they may be added to other sectors, too. The inclusion of the experiment is only constrained by the restricted set of variables included in CHELSA-W5E5. We do not provide spin-up data for the experiments. This means that models requiring a spin-up currently cannot perform the experiments. We will work on a solution on demand.
In contrast to the experiment testing the sensitivity of the impact simulations to a higher resolution of the atmospheric CRFs, the associated sensitivity experiment for the marine ecosystems and fisheries sector is not based on higher- but on lower-resolution oceanic data. While the default obsclim oceanic forcing data are derived by interpolating the observation-based historical ocean simulations from a tripolar 0.25∘ grid to a regular 0.25∘ grid (see Sect. 3.4), the CRFs for the sensitivity experiment are derived by aggregating the default obsclim data to a regular 1.0∘ grid (60arcmin). Evaluating the 1.0∘ resolution is of interest because this is the resolution of the oceanic forcing data in ISIMIP3b. The low-resolution simulations could either start from the end of the simulations of the transition period of the associated higher-resolution runs (“obsclim + histsoc; default”) or starting conditions could be newly generated by following the spin-up + transition procedure of the “obsclim + histsoc; default” experiment but using the low-resolution 1955-riverine-input CRF from the years 1961–1980.
The low-resolution sensitivity experiment (obsclim + nat; 60arcmin) for the marine ecosystems and fisheries sector is analogous to the “obsclim + nat; default” experiment described further above but using the lower-resolution oceanic CRF (60arcmin). The difference between this experiment and the “obsclim + histsoc; 60arcmin” sensitivity experiment can be considered the effect of the historical changes in DHF as estimated using lower-resolution CRF, and comparison with the same difference in the default experiments then indicates how the estimate of this effect depends on the resolution of the oceanic forcing. The simulations could either start from the end of the simulations of the transition period of the associated higher-resolution runs (“obsclim + nat; default”) or starting conditions could be newly generated by following the spin-up + transition procedure of the “obsclim + nat; default” experiment but using the low-resolution 1955-riverine-input CRF from the years 1961–1980.
CO2 sensitivity experiments (obsclim + histsoc, obsclim + 2015soc, or obsclim + 1901soc; 1901co2). To quantify the pure effect of the historical increase in atmospheric CO2 concentrations on vegetation leaf gas exchange and follow-on effects such as on carbon stocks, water use efficiency, and vegetation distribution, we introduced three sensitivity experiments where atmospheric CO2 concentrations are held constant at 1901 levels (296.13 ppm) in contrast to the default obsclim + histsoc, obsclim + 2015soc, or obsclim + 1901soc experiments, respectively, where atmospheric CO2 concentrations are assumed to increase according to observations. The effect is known as CO2 fertilization through an increase in the photosynthesis rate of plants and limited leaf transpiration (increase in water use efficiency), enabling a more efficient uptake of carbon by the plants. Comparing the “obsclim + histsoc; default” experiment to the “obsclim + histsoc, 1901soc” experiment can be considered attributing historical changes in natural, human, and managed systems to historical changes in CO2 concentrations as a single component of the changes in climate-related systems. The experiment is included in the protocols of the sector for agriculture, terrestrial biodiversity, biomes, fire, lakes (global and local), permafrost, and peat and water (global and regional). A potentially required spin-up should be identical to the spin-up for the associated default experiments using the transition period 1850–1900 to reach the 1901 CO2 level.
Water management sensitivity experiment (obsclim + histsoc, obsclim + 2015soc; nowatermgt). In this “no water management” experiment, models are run assuming no irrigation, no human water abstraction, no dams or reservoirs, and no seawater desalination, while other direct human forcings such as land use changes are considered according to histsoc or 2015soc. By comparison to the default experiments, the simulations allow for a quantification of the pure effects of dedicated water management measures on, e.g., discharge. When comparing “obsclim + histsoc, nowatermgt” to “obsclim + histsoc; default” this can be considered attributing observed changes in natural, human, or managed systems to (changes in) water management. The sensitivity experiment has been introduced into the global and regional water sector protocols. If a spin-up is required, it should be done similar to the spin-up for the associated default experiments but assuming no water management.
Irrigation sensitivity experiment (obsclim + histsoc, 1901irr). In this “no irrigation expansion” experiment, models are run assuming irrigation extent and irrigation water use efficiencies fixed at the year 1901, while other direct human forcings such as land use changes and water management categories are considered according to histsoc or 2015soc. By comparison to the default experiments, the simulations allow for a quantification of the pure effects of historical irrigation expansion (i.e., the attribution of historical changes in natural, human, or managed systems to changes in irrigation compared to 1901). The sensitivity experiment has been introduced into the global water and biome sector protocols. If a spin-up is required, it should be done similar to the spin-up for the associated default experiments but assuming no irrigation expansion. This experiment is designed such that its outcomes are comparable to those of the Irrigation Impacts Model Intercomparison Project (IRRMIP; https://hydr.vub.be/projects/irrmip, last access: 21 December 2023), in which Earth system models simulate irrigation influences on the Earth system.
No-fire sensitivity experiment (obsclim + histsoc; nofire). In this “nofire” experiment, fire is switched off in the model simulations. In comparison to the default obsclim + histsoc simulations, the historical effects of fires on, e.g., carbon fluxes and vegetation distributions can be determined. The sensitivity experiment has been introduced into the fire, biomes, permafrost, and peat protocols. The required spin-up should be done similar to the spin-up for the associated default experiments but assuming no fire activities.
Fixed 1955 riverine input into the ocean sensitivity experiment (obsclim + histsoc, obsclim + nat; 1955-riverine-input). In this 1955-riverine-input experiment, riverine input into the ocean (amount of freshwater and nutrients) is held constant at 1955 levels. In comparison to the default obsclim + histsoc simulation, the experiment allows for the quantification of the impacts of historical climate-induced variations in freshwater influx in combination with the climate- and directly human-induced changes in nutrient inputs (attribution of observed changes in marine ecosystems and fisheries to long-term changes in riverine freshwater and nutrient inputs). The riverine inputs in the “obsclim + nat; 1955-riverine-input” experiment are identical to the ones in the “obsclim + histsoc; 1955-riverine-input”; i.e., the riverine inputs also account for the human contribution to the nutrient influx due to land use changes and fertilizer inputs and are not “naturalized”. Instead the nat specifier in the marine ecosystems and fisheries sector only means no fishing efforts. Thus, the comparison to the naturalized default experiment (obsclim + nat; default) not accounting for any fishing efforts to the “obsclim + nat; 1955-riverine-input” experiment allows for a quantification of the contribution of climate-induced changes in freshwater influx to the overall impacts of climate change in combination with the contribution of the effect of the human contribution to nutrient inputs at 1955 levels. The sensitivity experiment has been introduced into the marine ecosystems and fisheries protocol. A potentially required spin-up should be done similar to the spin-up for the associated default experiments but assuming riverine inputs fixed at 1955 levels.
2.2 Counterfactual baseline simulations for impact attribution (counterclim)
The second set of impact model simulations within ISIMIP3a is dedicated to the attribution of historical changes in natural, managed, and human systems to long-term changes in climate-related systems, i.e., the atmosphere, ocean, and cryosphere as physical or chemical systems (see Sect. 1). In ISIMIP3a, we address attribution to changes in the climate system itself, e.g., trends in atmospheric temperature and precipitation, as well as changes in coastal water levels and atmospheric CO2 concentrations. The provided counterfactual forcing data comprise daily atmospheric climate derived from the ISIMIP observational climate datasets (see Sect. 3.1), daily counterfactual coastal water levels derived from the ISIMIP historical coastal water-level dataset (see Sect. 3.3), and constant 1901 atmospheric CO2 and CH4 concentrations (see Table 3). So far, we have not addressed attribution to long-term changes in (i) the ocean (e.g., temperature or ocean acidification changes), (ii) the cryosphere (e.g., glacier mass loss), and (iii) tropical cyclone characteristics (e.g., trends in associated heavy precipitation or wind speeds) other than the effects mediated through sea level rise. Table 3 lists the climate-related forcings defining the counterclim experiments. The counterclim climate-related forcings are combined with the observed direct human forcing to facilitate the attribution experiments listed in Table 4 and explained below.
Standard attribution experiment using counterfactual climate-related forcings and observed variations of direct human forcings (counterclim + histsoc; default). This is the twin experiment to the default obsclim + histsoc evaluation experiment. It uses the counterclim climate-related forcings as described in Table 3, while all direct human forcings are the same as the ones used in the evaluation experiment (histsoc). As the corresponding evaluation experiment aims to ensure that impact models can fully capture historical variations including long-term trends, this experiment is best suited for impact attribution. It is therefore the standard impact attribution experiment that each sector should strive to follow.
Fixed 2015 direct human forcing attribution experiment (counterclim + 2015soc; default). This is the twin experiment to the obsclim + 2015soc experiment. It uses the counterclim climate-related forcings as described in Table 3 and constant direct human forcings at 2015 levels (2015soc). Impact attribution using this experiment has caveats because the twin obsclim + 2015soc experiment is not built to fully explain the historical observations including its trends. Impact attribution building on this experiment therefore needs to find other means to ensure that the impact model correctly captures the response to changes in climate-related systems. It may, e.g., build on the assumption that fixed direct human forcings do not change the models' sensitivity to historical climate change. The impact models that cannot account for varying historical direct human forcings can take up the attribution task through this experiment.
Fixed 1901 direct human forcing attribution experiment (counterclim + 1901soc; default). This is the twin experiment to the obsclim + 1901soc experiment. It allows for a quantification of the combined effect of changes in all forcings (climate-related and direct human) during the historical period when compared to the default evaluation experiment (obsclim + histsoc). It also allows for a quantification of the effect of varying direct human drivers when compared to the counterclim + histsoc experiment and the effect of the 2015 to 1901 difference in direct human forcing if compared to the counterclim + 2015soc experiment, conditional on counterclim climate-related forcings.
No direct human forcing attribution experiment (counterclim + nat; default). This is the twin experiment to the default obsclim + nat experiment. It allows for a quantification of the effect of climate change under conditions of absent direct human forcings but a natural state of the world. The nat experiment is included in the biomes sector protocol.
3.1 Observational atmospheric climate forcing data (factual + counterfactual)
The datasets described in this section all contain the variables listed in Table 5 at the resolution indicated there. While Sect. 3.1.1 described the standard atmospheric climate forcing as one component of the default obsclim CRF used within the evaluation experiments (see Sect. 2.1.1), Sect. 3.1.2 describes the derivation of the high-resolution data used within the obsclim-based sensitivity experiments (see Sect. 2.1.2), and Sect. 3.1.3 provides a description of the basic approach and the references for the derivation of the counterfactual atmospheric climate forcings used for the counterclim experiments described in Sect. 2.2.
3.1.1 Default factual data
As one component of the default obsclim CRFs, we provide four observational datasets specifically generated for the evaluation experiments of ISIMIP3a: GSWP3-W5E5, 20CRv3-W5E5, 20CRv3-ERA5, and 20CRv3. All four datasets have daily temporal and 0.5∘ spatial resolution and cover the variables listed in Table 5. Their temporal coverage varies, with GSWP3-W5E5 and 20CRv3-W5E5 covering 1901–2019, while 20CRv3-ERA5 covers 1901–2021 and 20CRv3 covers 1901–2015. Instead of excluding datasets that do not cover the most recent years, we focused on including datasets that start in 1901 to allow for a common spin-up procedure (described in Sect. 2.1 for the “obsclim + histsoc; default” experiment) in order to support models that need to spin up, e.g., their carbon pools under stable climate-related and direct human forcings before they can do the actual experiments.
The GSWP3-W5E5 dataset is based on W5E5 v2.0 (Lange et al., 2021), which is also used as the observational reference dataset for the bias adjustment of climate input data for ISIMIP3b that will be described in an ISIMIP3b protocol paper (Frieler, 2024). W5E5 v2.0 combines WFDE5 v2.0 (Cucchi et al., 2020) with data from the latest version of the European Reanalysis (ERA5; Hersbach et al., 2020) over the ocean. WFDE5 v2.0 is generated with the WATCH forcing data methodology that includes bias adjustment of all variables (Cucchi et al., 2020). Since W5E5 v2.0 only covers the years 1979 to 2019, it was extended backward in time to the year 1901. For this extension, we used version 1.09 of the Global Soil Wetness Project phase 3 (GSWP3) dataset (Kim, 2017), bias-adjusted to W5E5 v2.0 in order to reduce discontinuities at the 1978–1979 transition. The method used for this bias adjustment was ISIMIP3BASD v2.5 (Lange, 2019, 2021). The GSWP3 dataset is a dynamically downscaled and bias-adjusted version of the Twentieth Century Reanalysis version 2 (20CRv2; Compo et al., 2011). For a detailed description of the GSWP3-W5E5 dataset and its constituents, see Mengel et al. (2021).
Unfortunately, for some variables, GSWP3 shows discontinuities at every turn of the month. The month-by-month bias adjustment applied in its creation is responsible for this artifact (Rust et al., 2015). In order to overcome this issue, which also affects GSWP3-W5E5, we additionally provide 20CRv3-W5E5, a dataset where W5E5 v2.0 is backward-extended using ensemble member 1 of the Twentieth Century Reanalysis version 3 (20CRv3; Slivinski et al., 2019, 2021), interpolated to 0.5∘ and then bias-adjusted to W5E5 v2.0 using ISIMIP3BASD v2.5. The 20CRv3-W5E5 data are continuous at every turn of the month thanks to the application of ISIMIP3BASD v2.5 in running-window mode (see Sect. 3.1). Since GSWP3 is based on 20CRv2, the 20CRv3-W5E5 dataset can be considered an update of GSWP3-W5E5.
Two more climate input datasets are provided in ISIMIP3a in order to facilitate climate-input-data-related quantifications of uncertainty in the associated impact assessments. Those datasets are not based on W5E5 to account for trend and variability artifacts in W5E5 that are related to the climatological infilling procedures used to deal with gaps in the station observations employed for the bias adjustment of ERA5 for the production of WFDE5 (for a detailed description of this caveat see https://data.isimip.org/caveats/20/, last access: 21 December 2023). The first of the additional ISIMIP3a climate input datasets is 20CRv3-ERA5, which was created in the same way as 20CRv3-W5E5, but using ERA5 instead of W5E5 for the time period 1979–2021, and also as the bias adjustment target for the time period 1901–1978. Finally, we also provide the “raw” 20CRv3 data, i.e., ensemble member 1 of 20CRv3, interpolated to 0.5∘ but not bias-adjusted to any other dataset. This dataset is included since it was generated with only one method and did not need to be combined with another dataset to fully cover the 20th century.
3.1.2 High-resolution atmospheric factual data (CHELSA-W5E5)
This dataset is provided to facilitate the high-resolution sensitivity experiment described in Sect. 2.1.2. It covers the global land area at 30′′ (∼1 km) horizontal and daily temporal resolution from 1979 to 2016 for the variables precipitation (pr), surface downwelling shortwave radiation (rsds), and daily mean, minimum, and maximum near-surface air temperature (tas, tasmin, tasmax). CHELSA-W5E5 v1.0 (Karger et al., 2022) is a downscaled version of the W5E5 v1.0 dataset, where the downscaling is done with the Climatologies at High resolution for the Earth's Land Surface Areas (CHELSA) v2.0 algorithm (Karger et al., 2017, 2021, 2023).
This algorithm applies topographic adjustments based on surface altitude (orog) information from the Global Multi-resolution Terrain Elevation Data 2010 (GMTED2010; Danielson and Gesch, 2011). The algorithm is applied day by day. CHELSA-W5E5 tas is obtained by applying a lapse-rate adjustment to W5E5 tas using differences between CHELSA-W5E5 orog and W5E5 orog in combination with temperature lapse rates from ERA5. Those lapse rates are calculated based on atmospheric temperature, T, at 950 and 850 hPa and the geopotential height, z, of those pressure levels. The lapse rate used for the adjustment is calculated as the daily mean of hourly values of . The variables tasmax and tasmin are downscaled in the same way using the same lapse-rate value.
Precipitation downscaling uses daily mean zonal and meridional wind components from ERA5 to approximate the orographic wind effect on small-scale precipitation patterns (differences between windward and leeward precipitation rates) and combines that with the height of the planetary boundary layer to estimate the total orographic effect on precipitation intensity. Using that, precipitation from W5E5 is downscaled such that precipitation fluxes are preserved at the original 0.5∘ resolution of W5E5. More details are given in Karger et al. (2021).
Surface downwelling shortwave radiation, rsds, at 30 arcsec resolution is strongly influenced by topographic features such as aspect or terrain shadows, which are less pronounced at 0.5∘ resolution. The downscaling algorithm combines such geometric effects with orographic effects on cloud cover for an orographic adjustment of rsds. Geometric effects are considered by computing 30′′ clear-sky radiation estimates using the method described in Karger et al. (2023) and a simplified, uniform atmospheric transmittance of 80 %. These effects include shadowing from surrounding terrain, diffuse radiation, and terrain aspect. To include how orographic effects on cloud cover influence rsds, the clear-sky radiation estimates are adjusted using downscaled ERA5 total cloud cover. The cloud cover downscaling uses ERA5 cloud cover at all pressure levels and the orographic wind field following the methods described in Brun et al. (2022b). Finally, the clear-sky radiation estimates adjusted for cloud cover are rescaled such that they match W5E5 rsds, B-spline-interpolated to 30′′.
We provide the original CHELSA-W5E5 data with a horizontal resolution of 30 (∼1 km) as well as spatially aggregated versions with resolutions of 1.5′ (∼3 km, aggregation factor 3), 5.0′ (∼10 km, aggregation factor 10), and 30.0∘ (∼60 km, aggregation factor 60). The aggregation to 0.5∘ is necessary since the aggregated CHELSA-W5E5 data differ from the default GSWP3-W5E5 and 20CRv3-W5E5 data provided in the obsclim setup for 1979–2016. There are two reasons for this. First, the downscaled data are based on W5E5 v1.0, whereas GSWP3-W5E5 and 20CRv3-W5E5 are based on W5E5 v2.0. Secondly, for all variables except pr, the CHELSA downscaling algorithm produces data that differ from the original data when upscaled (spatially aggregated) back to the original resolution.
We do not provide a counterfactual version of the high-resolution climate forcing.
The CHELSA method is not yet available for all variables included in the standard forcing data. Relative humidity, surface wind, air pressure, and longwave radiation cannot yet be downscaled by the approach. To allow modelers to already start the sensitivity experiments, we provide an alternative downscaling approach as described below. We use observational data with the required higher spatial resolution but lower temporal resolution to generate the high-resolution daily relative humidity and surface wind speeds. Air pressure is derived by on orographic correction of the linearly interpolated sea level pressure, and surface downwelling longwave radiation is derived from high-resolution temperatures derived by CHELSA and relative humidity. The code required to generate the data is freely available (Malle, 2023).
For daily mean near-surface relative humidity (hurs) the provided downscaling algorithm combines monthly 30′′ CHELSA-BIOCLIM+ data (Brun et al., 2022a, b) with daily W5E5 data. In a first step we regrid daily 0.5∘ W5E5 hurs to the target grid (30′′) by bilinear interpolation. We assume relative humidity to follow a beta distribution and logit-transform both regridded monthly averaged W5E5 ( and monthly CHELSA-BIOCLIM+ ( relative humidity data. The difference (Δhursmon) is then added to daily regridded and logit-transformed W5E5 hurs of the respective month, and the final raster is obtained by back-transforming the sum:
where
To include orographic effects in daily mean near-surface wind speed (sfcwind) we follow the approach of Brun et al. (2022b) and use an aggregation of the Global Wind Atlas 3.0 data (Davis et al., 2023) from the Technical University of Denmark (Davis et al., 2023) in combination with daily 0.5∘ sfcwind from W5E5. We first regrid both the Global Wind Atlas data and the W5E5 sfcwind data to the target grid of 30′′ using bilinear interpolation. The Global Wind Atlas data product ( represents average wind speeds for 2008 to 2017. We therefore average daily regridded W5E5 data over this time period (. We assume surface wind speeds follows a Weibull distribution and log-transform both datasets before computing the difference ΔsfcWindcli, whereby a small positive constant (c) was added to all data points before applying the transformation to avoid the problem that log(0) is undefined. We add this difference layer (ΔsfcWindcli) to each log-transformed daily W5E5 raster and back-transform the sum to obtain the final daily mean near-surface wind speed raster:
where
Daily mean surface air pressure (ps) is calculated using the barometric formula:
with being the regridded 0.5∘ W5E5 daily mean sea level pressure (bilinear interpolation to 30′′), g the gravitational acceleration constant (9.80665 m s−2), “orog” the altitude at which air pressure is calculated (CHELSA-W5E5 orog, m), M the molar mass of dry air (0.02896968 kg mol−1), R the universal gas constant (8.314462618 J (mol K)−1), and T0 the sea level standard temperature (288.16 K).
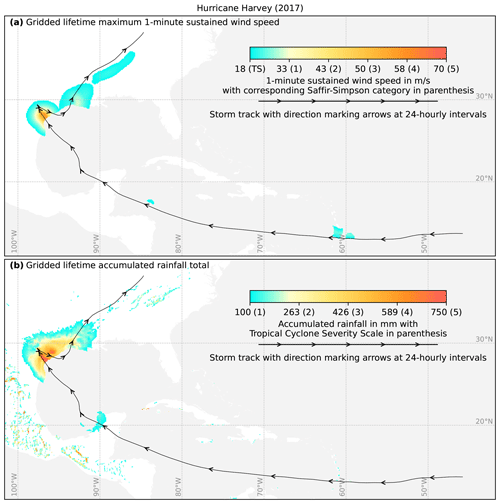
Figure 2Tropical cyclone storm track (a, b, line with arrows), derived maximum wind speeds (a, colored shades), and accumulated rainfall totals (b, colored shades) of Hurricane Harvey that made landfall in Texas (USA) in August 2017. The wind speeds are according to the Holland wind profile (Holland, 1980, 2008), and the rainfall is according to the TCR model (Zhu et al., 2013). The coloring in (b) follows the tropical cyclone severity scale (Bloemendaal et al., 2021).
For surface downwelling longwave radiation (rlds) we follow Fiddes and Gruber (2014) as well as Konzelmann et al. (1994) and account for orographic effects by reducing the clear-sky component of all-sky emissivity with elevation. We assume cloud emissivity remains unchanged when moving from coarse to fine resolution. First, we compute clear-sky emissivity components for both the 0.5∘ W5E5 grid and the target 30′′ grid (, , respectively):
where x1=0.43, x2=5.7, and is water vapor pressure as a function of relative humidity at the respective resolution (see Fiddes and Gruber, 2014). By using 0.5∘ W5E5 rlds and tas data and inverting the Stefan–Boltzmann equation we obtain all-sky emissivity:
with σ being the Stefan–Boltzmann constant ( J s−1 m−2 K−4). In a next step, the cloud-based component of emissivity () can be estimated as the difference between all-sky and clear-sky emissivity, which is then regridded to the target grid via bilinear interpolation.
In a last step we obtain elevation-corrected longwave radiation (rldsdly) by adding to the high-resolution clear-sky emissivity () and applying the Stefan–Boltzmann law again:
As soon as the CHELSA approach is extended to also cover the missing variable we plan to provide these data and test the sensitivity of the impact simulations to these two alternative downscaling methods.
3.1.3 Default counterfactual data
To simulate the baseline no-climate-change state of a human or natural system that is required for impact attribution, we provide a detrended version of the observational factual forcing data using the ATTRICI approach (ATTRIbuting Climate Impacts, Mengel et al., 2021). The method identifies the long-term shifts in the factual daily climate variables that are correlated with global mean temperature change assuming a smooth annual cycle of the associated scaling coefficients for each day of the year. The observed trends since 1901 are then removed from the observational data by projecting the observed data onto the estimated distributions assuming a fixed 1901 level of global warming. The projection is done through quantile mapping, a method borrowed from the bias adjustment literature. In this way we preserve the internal variability of the observed data in the sense that factual and counterfactual data for a given day have the same rank in their respective statistical distributions. The impact model simulations forced by the counterfactual climate inputs therefore allow for quantifying the contribution of the observed climate change (no matter from where the trends originate) to observed long-term changes in impact indicators but also for quantifying the contribution of the observed trend in climate to the magnitude of individual impact events.
3.2 Tropical cyclone (TC) data (factual)
As additional CRF, we provide historical TC tracks (information about the observed location of minimal pressure), with associated gridded wind and rain fields (see variable names and units in Table 6 and the maps of maximum wind speed and accumulated rainfall totals for the example of Hurricane Harvey in Fig. 2). In addition to this purely climate-related forcing, we also provide wind exposure in terms of (i) shares of national territory affected by extreme winds speeds, (ii) national shares of people exposed to extreme winds speeds, and (iii) national shares of economic assets affected by extreme winds speeds as derived from the estimated wind fields and historical population and GDP distributions (see below). Table 6 provides a comprehensive list of all variables, with their meaning and resolution as well as their source.
TC tracks include the position of storm center, central pressure, environmental pressure, radius of maximum wind speed, and the outermost closed isobar. We provide processed track information of historical TCs from 1950 to 2021. The information is derived from IBTrACS, the most comprehensive global dataset of historical TC activity (Knapp et al., 2010) that provides information about the location of the storm center, the pressure at the center and at the outermost closed isobar, and the maximum 1 min sustained wind speed as reported by the WMO Regional Specialized Meteorological Centers (RSMCs) and by agencies in Shanghai and Hong Kong. For recent events and most reporting agencies, IBTrACS also contains observational information about the radius from the center where maximum wind speed is attained and the radius of the outermost closed isobar. Information is provided in at least 6-hourly time steps. Usually temporal resolution reaches 3 h or even less. The latest version (v04r00) of IBTrACS is continuously updated with near-real-time data taken from regional meteorological agencies. The data are marked as provisional before they are replaced by the so-called best track data up to 2 years after the events. IBTrACS contains data from 1842 to present, but coverage by the WMO RSMCs starts much later for some of the basins (around 1850 for the North Atlantic and southern Indian Ocean, in 1905 for the South Pacific, in 1950 for the North Pacific, and in 1990 for the northern Indian basin). Data quality is globally consistent starting from the mid-1970s when satellite observations became available.
The dataset we provide uses best track data from 1950 to 2021. For each TC in IBTrACS, we merge the data of different reporting agencies into a single track dataset with information about the following variables: time, location of the storm center, ocean basin, central pressure, maximum 1 min sustained wind speed, environmental pressure, radius of maximum wind speeds, and radius of the outermost closed isobar (see Table 8). Several processing steps are applied to ensure consistency and completeness of the data. For each storm, the variables that are not reported by the officially responsible WMO RSMC for this storm are taken from the next agency in the following list that did report this variable for this storm: the US agencies (NHC, JTWC, CPHC), Japanese Meteorological Agency, Indian Meteorological Department, MeteoFrance (La Réunion), Bureau of Meteorology (Australia), Fiji Meteorological Service, New Zealand MetService, Chinese Meteorological Administration, and Hong Kong Observatory. Thus, for different storms, the same variable might be taken from different agencies. As sustained wind speeds are reported at different averaging intervals by different agencies, we use multiplicative factors to rescale all wind speeds to 1 min sustained winds (Knapp and Kruk, 2010). All variables are extracted at the highest temporal resolution where time and location information is available in IBTrACS. Temporal reporting gaps within a variable are linearly interpolated so that the temporal resolution is at least 3-hourly. After interpolation, time steps where neither central pressure nor maximum wind speeds are available are discarded. Tracks with fewer than two valid time steps are discarded. If at least central pressure or maximum wind speed is available, one variable is estimated from the other using statistical wind–pressure relationships. Missing RMW and ROCI values are estimated from the central pressure using statistical relationships. Finally, missing environmental pressure values are filled with basin-specific defaults (1010 hPa for the Atlantic and eastern Pacific, 1005 hPa for the Indian Ocean and western Pacific, and 1004 hPa for the South Pacific).
We provide two additional along-track variables that are taken from the European Reanalysis (ERA5; Hersbach et al., 2020) and that are needed for the computation of precipitation (see below): the temperature at the storm center on the 600 hPa pressure level and the wind speed on the 850 hPa pressure level averaged over the 200–500 km annulus around the storm center.
For gridded maps of (maximum) wind speeds, we derive two different gridded wind field products from an extrapolation of the observed TC track information to gridded estimates of surface wind speeds (1 min sustained winds at 10 m above ground) at a spatial resolution of 300 arcsec (approximately 10 km). The two products are based on circular wind fields from different radial wind profiles. The first is a semiempirical model that estimates the full wind profile from the central pressure variable based on the gradient wind balance assumption (Holland, 1980, 2008). The second more physics-based model uses the less reliable maximum wind speed variable to derive the wind profile from the boundary layer angular momentum balance (Emanuel and Rotunno, 2011). This wind profile represents the storm's inner core very well but tails off too sharply in the outer region (Chavas and Lin, 2016). However, for high-impact events, the core is the most relevant storm region, and outer wind profiles are not analytically solvable, incurring considerable computational expense when applied to a large track set.
In both cases, the circular wind fields are combined with translational wind vectors that arise from the TC movement, assuming that the influence of translational wind decreases with distance from the TC center (Cyclone Database Manager, 2023). We use the highest available temporal resolution (up to 3-hourly) provided in IBTrACS and interpolate it to 1-hourly resolution before applying the parametric wind field models. In a post-processing step, we also calculate the maximum value of wind speeds over the duration of the TC event (max_wind).
The approach by Holland has been successfully applied in socioeconomic risk and impact analyses (Peduzzi et al., 2012; Geiger et al., 2018; Eberenz et al., 2021). The Emanuel–Rotunno approach has been used for storm surge simulations (Krien et al., 2017; Marsooli et al., 2019; Gori et al., 2020; Yang et al., 2021) and as the basis for the rain field model that we describe below (Feldmann et al., 2019).
In terms of wind exposure, as an extension of the tropical cyclone exposure dataset TCE-DAT (Geiger et al., 2018), we provide national shares of people and economic assets exposed to 1 min sustained winds above 34, 48, 64, and 96 kt for each storm. In addition to that, shares of national territory affected by 1 min sustained winds above 34, 48, 64, and 96 kt are provided. To estimate the exposed population and assets we use the histsoc population and GDP distributions described in Sect. 4.1 and 4.2, respectively. The GDP values are converted to assets by applying the decadal (2010–2019) mean of national capital stock to GDP ratios from the Penn World Table version 10.0 (Feenstra et al., 2015). We also provide exposed population and assets assuming fixed 2015 population and asset distributions.
For precipitation, we are also planning to provide rainfall fields, following a physics-based model that simulates convective TC rainfall by relating the precipitation rate to the total upward velocity within the TC vortex (Zhu et al., 2013). The approach has been successfully applied in rainfall risk assessments in the US (Feldmann et al., 2019; Gori et al., 2022). The rain rate will be simulated for all events in the IBTrACS database at 0.5-hourly temporal and 300 arcsec (approximately 10 km) spatial resolution within a 1500 km radius around the storm center. We provide the derived rainfall totals at hourly resolution as well as the maximum 24-hourly rainfall total during the entire storm duration since this variable is frequently used for rainfall risk assessment studies (Fagnant et al., 2020).
Different TC wind profiles can be used as an input for the rain field model (Lu et al., 2018; Xi et al., 2020). We will provide the rainfall fields for the two wind profile models by Holland and Emanuel–Rotunno that we also use for the wind fields described above.
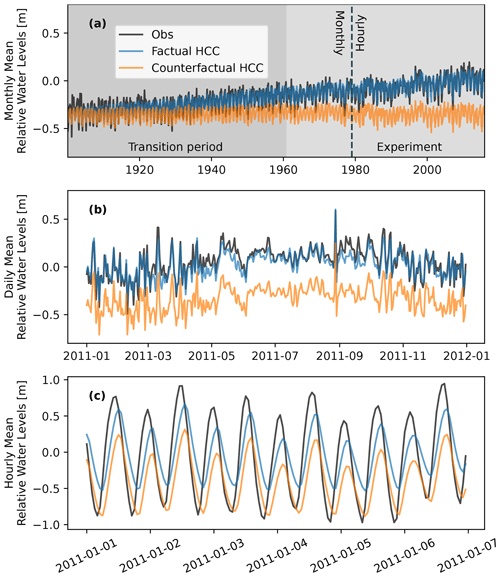
Figure 3Observed and reconstructed coastal relative water levels at New York, USA. The counterfactual baseline represents water levels without long-term trend since 1900. Water levels are aggregated to monthly means in panel (a) and daily means in the year 2011 in panel (b), while panel (c) shows some of the data at hourly resolution. The reconstructed water levels are available as monthly mean values from 1901 to 1978 and as hourly mean values from 1979 to 2015.
3.3 Coastal water levels (factual + counterfactual)
To enable the quantification of impacts of historical relative sea level rise on coastal systems we provide observation-based coastal water levels building on the HCC dataset (hourly coastal water levels with counterfactual; Treu et al., 2023). In contrast to absolute sea levels, relative sea levels are measured against a land-based reference frame (tide gauge measurements). This means that they are determined not only by thermal expansion, loss of land ice, or dynamical processes influenced by climate change, but also by vertical land movements (Wöppelmann and Marcos, 2016) induced by, e.g., glacial isostatic adjustments (Caron et al., 2018; Whitehouse, 2018) or human interventions such as groundwater abstraction (Wada et al., 2016b). HCC encompasses factual and counterfactual coastal water levels along global coastlines from 1901 to 1978 at monthly resolution and from 1979 to 2015 at hourly resolution (see Fig. 3). The counterfactual coastal water levels are derived from the factual dataset by removing the trend in relative sea level since 1900. The detrending preserves the timing of historical extreme sea level events similar to the counterfactual atmospheric climate forcing described in Sect. 3.1 (see Fig. 3b). Hence, the data can be used for an event-based attribution of, e.g., observed flooding to observed relative sea level rise with pairs of impact simulations driven with the factual and counterfactual datasets. It is important to highlight that “attribution to observed changes in relative water levels” does not imply attribution to anthropogenic climate forcing because such observed changes may include trends that are not driven by human greenhouse gas emissions. Important sources for such trends are the ongoing adjustments of ice sheets, glaciers, and the Earth's crust to climate conditions before industrialization (Slangen et al., 2016) and the land subsidence due to water, gas, and oil extraction (Nicholls et al., 2021). In the following the derivation of the data is described in more detail.
Default factual data. To capture the impacts of extreme water levels we provide hourly observation-based coastal water levels as forcing data. To this end we combine the Coastal Dataset for the Evaluation of Climate Impact (CoDEC) dataset (Muis et al., 2020) that describes high-frequency variation of sea level along global coastlines with a recent reconstruction of observed long-term sea level rise (Dangendorf et al., 2019). The CoDEC hourly data build on a shallow-water model with fixed ocean density driven by ERA5 wind and atmospheric pressure fields. The CoDEC data thus start only in the year 1979 and do not include variations due to ocean density changes and multiyear trends from observed sea level rise or vertical land movement. In contrast, the hybrid reconstruction (HR) dataset from Dangendorf et al. (2019) represents sea level change since 1900 on a monthly timescale, including density variations and multiyear trends. Long-term sea level change in HR is based on fitting theoretically known and modeled spatial–temporal fields of individual contributing factors of sea level change to a set of observations of sea level change from tide gauges. The individual contributing factors are theoretically known cryospheric fingerprints from two ice sheets, 18 major glacier regions, glacial isostatic adjustment from 161 Earth rheological models, and dynamic changes in sea surface height modeled by six global climate models. Short-term sea level variations are represented in HR by extending the spatiotemporal patterns from satellite altimetry back to the year 1900 using tide gauge records. We create the HCC dataset by low-pass-filtering the HR dataset and high-pass-filtering the CoDEC dataset before summing them. Vertical land motion is subsequently added to yield relative changes in water levels along global coastlines. HCC shows improved agreement with tide gauge records on hourly to monthly timescales when compared to CoDEC due to the inclusion of density variations. This is most apparent for lower latitudes. The performance on inter-annual timescales is equal to Dangendorf et al. (2019).
Default counterfactual data. To estimate the effects of historical sea level rise on coastal systems, we provide a counterfactual sea level dataset as forcing for coastal impact models (Treu et al., 2023). To this end the long-term trend in the HCC data (1900–2015) was identified by a simple quadratic model in time and subtracted from the factual HCC data. The quadratic model assumes a constant acceleration of sea level rise over time. Analysis of sea level rise acceleration shows variation throughout the last century with an acceleration phase in the early century followed by a deceleration and then again acceleration until today (Dangendorf et al., 2019). By design, this variation is not included in our quadratic trend estimate. In general, we expect our trend estimation to largely exclude natural variability from the trend due to the low dimensionality of the trend model and the long data period. This is a desired outcome and preserves the natural variability in the counterfactual. Extreme sea level events have the same timing in the counterfactual and the factual dataset, facilitating event-based impact attribution.
3.4 Ocean data (factual)
Default factual data. For the fisheries and marine ecosystem models, we provide a number of physical and biogeochemical variables for the period 1961 to 2010 at different depth levels in the ocean (see Table 8). Since direct measurements of these variables are very scarce (Sarmiento and Gruber, 2006; WOCE Atlas, 2023), the only way to obtain a globally (or even regionally) complete and consistent forcing dataset is to use numerical models. Global ocean models, which also serve as oceanic components of Earth system models, often simulate many or all of the required variables. To let observations at least indirectly enter the oceanic forcing data for ISIMIP3a, we provide outputs from an ocean model run that is forced by an observation-based reanalysis product of atmospheric forcing (Liu et al., 2021). Compared to the oceanic forcing (Stock et al., 2014) provided to generate the ISIMIP2a simulations for the marine ecosystems and fisheries sector (Tittensor et al., 2018), this new dataset is based on the latest GFDL-MOM6 and COBALTv2 physical and biogeochemical ocean models running on a tripolar 0.25∘ grid and using the JRA-55 reanalysis (Tsujino et al., 2018) as the surface forcing, in contrast to the inter-annual forcing dataset of Large and Yeager (2009), which was previously used to drive GFDL-MOM4. The simulations also account for dynamic, time-varying river freshwater and nitrogen inputs that were simulated based on GFDL's land–watershed model LM3-TAN (Land Model version 3 with Terrestrial and Aquatic Nitrogen; Lee et al., 2019), adjusted using observations from the Global Nutrient Export from WaterSheds (NEWS) database (Seitzinger et al., 2006). To create the default obsclim climate-related forcings for the fisheries and marine ecosystem models these ocean model simulation data have been interpolated to a regular 0.25∘ grid while vertical resolution is preserved. In contrast to the atmospheric data, oceanic CRFs are provided at monthly temporal resolution.
Low-resolution factual data. To test to what degree a lower spatial resolution of the climate-related forcings affects the impact model simulations, the oceanic climate-related forcings have also been aggregated to 1∘ resolution as input for the “obsclim + histsoc, 60arcmin” sensitivity experiment.
CRF for the 1955-riverine-input sensitivity experiment. The 1955-riverine-input sensitivity experiment builds on the 0.25∘ GFDL-COBALT2 simulation forced by the JRA-55 reanalysis but without time-varying riverine inputs. Instead the influxes of freshwater and nutrients are fixed at mean 1951 to 1958 levels as described in the “control run” introduced by Liu et al. (2021). The data are interpolated to a regular 0.25∘ grid in the same way as the default obsclim CRFs.
We currently do not provide counterfactual versions of the ocean data forcing, though options are being explored.
Table 8ISIMIP3a oceanic climate-related forcing. Variables with suffixes -bot, -surf, and -vint were obtained from the seafloor, the top layer of the ocean, and vertical integration, respectively.

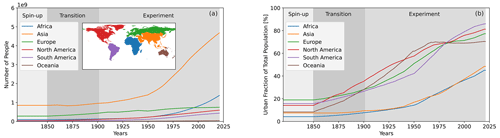
Figure 4Historical evaluation of population for different continents. Total number of people living in the region (a) and the urban population as a fraction of the total population per region (b).
4.1 Population data
For ISIMIP3a we provide consistent gridded and national population data (see Table 9) by rescaling the gridded data to match the national aggregates. Figure 4 shows the temporal evolution of total and urban population for different continents.
National data. Annual national population data are taken from the 2019 UN World Population Prospects (WPP) database for the period from 1950–2021 (United Nations, 2019). The 2019 revision of the WPP provides census-based population numbers from 1950 through 2020. For the year 2021, we use the “medium variant” of the probabilistic forecast also provided by the WPP. The forecast accounts for the past experience of each country, while reflecting uncertainty about future changes based on the past experience of other countries under similar conditions (see United Nations, 2019, for details). For countries not covered in the database, estimates are taken from the MissingIslands dataset (Arujo et al., 2021) to finally provide population data for 249 countries.
Gridded data. We provide gridded population data that are based on HYDE v3.3 (Kees Klein Goldewijk, personal communication, 2022). Just like the original dataset we provide total, rural, and urban population per grid cell. The original HYDE 3.3 data were on a ∘ × ∘ grid and have been interpolated to ISIMIP's 0.5∘ × 0.5∘ grid. Furthermore, the land–sea distinction was modified to comply with the ISIMIP country mask (see Table 1). Before the year 1950 HYDE provides data every 10 years, and the intermediate years have been filled by linear interpolation. Also, the original HYDE data end in 2020. So to cover the whole ISIMIP3a time frame the final year 2020 has been duplicated as 2021. In this way annual coverage for 1850 to 2021 has been achieved.
Data for all grid cells of a country, as defined by the ISIMIP 0.5∘ × 0.5∘ fractional country map (see Table 1), have been rescaled such that the country's total population matches the numbers provided in the national population data. Since the national data only start in 1950, all years prior to 1950 have been rescaled by the national scaling factors of 1950. The urban and rural populations have been rescaled by the same national scaling factors as the total population.
4.2 Gross domestic product (GDP)
Similar to the population data we also provide gridded and national GDP data (see Table 10). The downscaling of the national numbers is based on population and nightlight data (see below). In contrast to ISIMIP2a the gridded GDP and population data are now consistent such that previous artifacts in the derived GDP per capita could be eliminated (see below). Figure 5 shows the historical increase in GDP for different continents.
National GDP data. Time series of per capita GDP for the time period 1960–2021 are taken from the World Bank's World Development Indicator database (WDI) (Anon, 2008) and converted into constant 2005 Int$PPP using deflators and PPP conversion factors from WDI. For countries not covered in the WDI database, data from the MissingIslands dataset (Arujo et al., 2021) are used to allow covering 249 countries. Following a method developed by Koch and Leimbach (2023), the values for the year 2021 are derived from the IMF's World Economic Outlook short-term estimates of GDP per capita growth (International Monetary Fund, 2021) that comprise estimates of the growth impacts of the Covid-19 shock.
Gridded GDP data. Gridded GDP data at 0.5∘ resolution are derived from the national GDP time series by applying the LitPop method (Zhao et al., 2017; Eberenz et al., 2019), which uses the ISIMIP3a gridded population based on HYDE v.3.3 and nighttime light (NTL) data to downscale national GDP data for the period 1960–2021 to the ISIMIP 0.5∘ × 0.5∘ grid.
As the disaggregation of GDP is not only based on population but also uses the NTL GDP per capita, it is not constant within different countries. Deriving the gridded GDP data from the gridded population data provided within ISIMIP3a ensures that the both datasets can be combined such that the associated GDP per capita no longer shows the artifacts that have been found in the ISIMIP2a GDP per capita (ISIMIP2a, 2023).
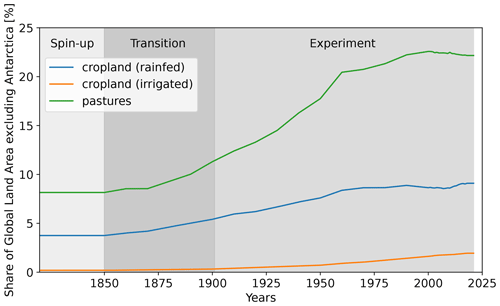
Figure 6Share of global land area excluding Antarctica covered by rainfed cropland (green), irrigated cropland (blue), and pasture (orange) [%]. The information is from the LUH v2 dataset provided as direct human forcing for ISIMIP3a (see details below).
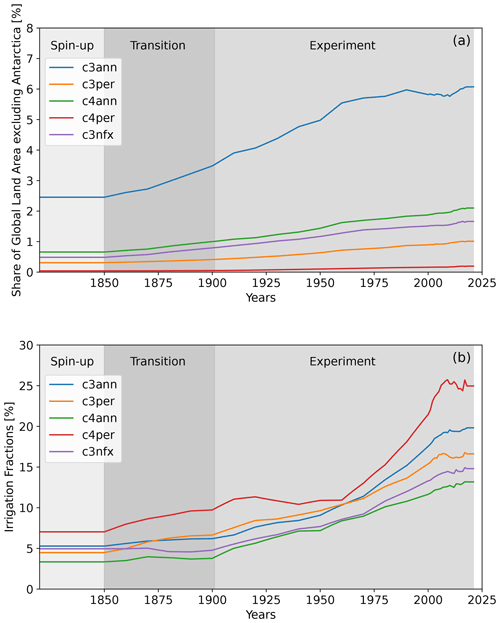
Figure 7(a) Share of global land area excluding Antarctica covered by different groups of crops (C3 annual – blue, C3 perennial – orange, C4 annual – green, C4 perennial – red, C3 nitrogen-fixing – purple). (b) Fraction of irrigated land for the different groups of crops. The information is from the LUH v2 dataset (see details on further disaggregation of the LUH v2 groups below).
4.3 Land use and irrigation patterns
Historical land use and irrigation patterns for ISIMIP3a simulations are taken from LUH v2 (Hurtt et al., 2020; Land use harmonization, 2023). The dataset is, up to 2018, identical to the data provided with ISIMIP2b. The data are based on the HYDE 3.2 land use dataset (Klein Goldewijk et al., 2017) and have been constantly extended up to 2021, i.e., by copying the 2018 patterns into 2019, 2020, and 2021.
The original HYDE 3.2 data distinguish four categories of land use: rainfed and irrigated cropland, managed pastures, and more extensively managed rangelands (see Table 11). The latter two categories are combined into grazing lands (ISIMIP variable pastures, see Fig. 6). In LUH v2 the crop land information is further downscaled to five crop types: C3 annual plants, C3 perennial plants, C3 nitrogen-fixing plants, C4 annual plants, and C4 perennial plants (see global aggregates in Fig. 7). In the same vein as the HYDE case, the LUH v2 dataset distinguishes between rainfed and irrigated croplands. For the purpose of driving the ISIMIP impact models, the LUH v2 data were interpolated from the original 0.25∘ × 0.25∘ to the standard ISIMIP 0.5∘ × 0.5∘ global grid. In a further downscaling step the land use data for five crops have been downscaled even further to 15 crop types (see global aggregates in Fig. 7). For this purpose the Monfreda land use dataset (Monfreda et al., 2008) has been used. It describes the crop land areas of 175 crops in the year 2000, and we use this to downscale the five crop categories into land use areas of 15 more specific crop types (maize, groundnut, rapeseed, soybeans, sunflower, rice, sugarcane, pulses, temperate cereals including wheat, temperate roots, tropical cereals, tropical roots, other annual, other perennial, and other N-fixing). The ratios determined from the year 2000 numbers have then been applied to all years. For further details please refer to Frieler et al. (2017).
The area outside of the specified agricultural and urban land is considered “natural vegetation” and not prescribed further to not constrain the dynamical vegetation models.
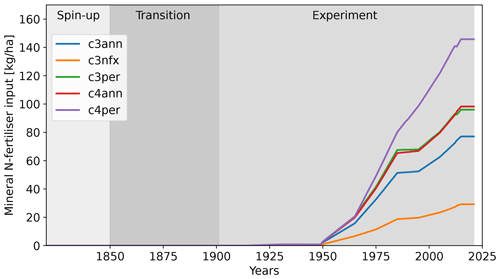
Figure 8Mean mineral N-fertilizer input averaged across the land areas where the considered crop groups are grown.
4.4 Fertilizer input
The LUH v2 dataset also includes national application rates of industrial nitrogen fertilizer (Hurtt et al., 2020). This does not include manure. The fertilizer data are not based on HYDE but were derived from other sources. The data for the years 1915–1960 are based on Smil (2001); those for 1961–2011 are based on a compilation by Zhang et al. (2015), which in turn is based on FAOSTAT (FAO, 2016), and data for 2012–2015 are based on a projection by the International Fertilizer Association (IFASTAT, 2015). For the pure crop runs within ISIMIP, where the considered crops are assumed to be grown everywhere without a land use specification, the LUH v2 national fertilizer inputs are assumed to be applied everywhere within the country. To calculate crop production, the LUH2 v2 land use patterns are applied in post-processing, i.e., by multiplying the crop yields from the pure crop run with the land use patterns (fraction of the grid cell where the crop has been grown).
4.5 Land transformation
These datasets are based on the LUH v2 Harmonization Data Set covering 850 to 2015 (Hurtt et al., 2020; Land use harmonization, 2023). The wood harvest data were obtained by aggregating from the original LUH v2 grid to the ISIMIP 0.5∘ × 0.5∘ grid (first-order conservative remapping) and then aggregating to the national sums. Wood harvesting data are used in the vegetation models to mimic wood removal as part of forest management and clearing and have a strong influence on the carbon balance. National data are provided so that models can use their internal routines to distribute the harvesting within a country's forest area. The gridded land transformation data were obtained by aggregating from the original LUH v2 grid to the ISIMIP 0.5∘ × 0.5∘ grid; these data always end a year earlier than all other land use data because a year in these datasets actually describes the changes from the current to the next year. The data have been extended up to 2021 by copying the 2015 data into the following years (files end in 2020).
4.6 Nitrogen deposition
Reduced nitrogen deposition and oxidized nitrogen deposition (NHx, NOy) are based on simulations by the NCAR Chemistry–Climate Model Initiative during 1850–2014 (Tian et al., 2018). Nitrogen deposition data were interpolated to 0.5∘ by 0.5∘ using the nearest grid point method. Data in 2015–2021 are assumed to be the same as those in 2014.
4.7 Crop calendar
Unfortunately, there is no global dataset describing changes in growing seasons across the historical period. Instead we provide a static crop calendar that has been developed within the AgMIP Global Gridded Crop Model Intercomparison GGCMI and merges information from various observational data sources (Jägermeyr et al., 2021a). It provides planting and maturity days for 18 different crops on the ISIMIP standard 0.5∘ grid. Grid cells outside of currently cultivated areas are spatially extrapolated (details below). For wheat and rice two growing seasons are provided, while for all other crops the calendar only specifies one main growing season. The reported growing seasons should not be considered the growing seasons for one specific year but as “representative growing season” across recent years. Within the crop models different crop varieties are represented by different heat units required to reach physiological maturity. The crop calendar should be implemented by adjusting the required heat units to the average of the annual sums of heat units between the specified planting and maturity date over all growing seasons between 1979 and 2010.
If modelers use a temporal adjustment of cultivars by varying required heat units in response to socioeconomic development or historical climate change this is certainly allowed within the histsoc setup. If cultivars are fixed according to the method described above this simulation will be considered a 2015soc simulation as long as other direct human drivers are also held constant at 2015 levels. However, if, e.g., fertilizer inputs are varied over time according to provided forcing data (see Sect. 4.4), the run will be considered a histsoc run.
GGCMI is currently working on a temporally resolved global crop calendar at the same spatial resolution based on various new data sources including agricultural ministries, census reports, phenological data bases, and experimental sites. This dataset will be published separately and could then be used to inform histsoc simulations.
4.8 Dams and reservoirs
In order to offer a consistent and common source of information about reservoirs and associated dams for climate impact modelers (see Table 16), we joined the Global Reservoir and Dam Database of the Global Water System Project (GRanD v1.3; (Lehner et al., 2011a, b) with a subset of the Georeferenced global Dams And Reservoirs (GeoDAR v1.2) database (Wang et al., 2022), developed at Kansas State University (KSU) and provided by Jida Wang ahead of publication, so that it could be provided when launching ISIMIP3 in 2020. These additional dams have construction or projected finalization dates between 2016 and 2025, while GRanD v1.3 includes dams constructed up until 2017. In total, the combined database now includes 7331 dams whose construction will be finished by 2025. It includes dams that were constructed before the simulation period but still exist (the first reported dam was finished in the year 286). For the simulations described here, dams with (projected) construction dates after 2020 are not considered; these will become relevant in the ISIMIP3b simulations, with the exception of the Grand Ethiopian Renaissance Dam, which we decided to include since its reservoir reached the first stage of filling of 4.9 km3 in July 2020 (BBC news: Nile dam row, 2020; Tractebel, 2020).
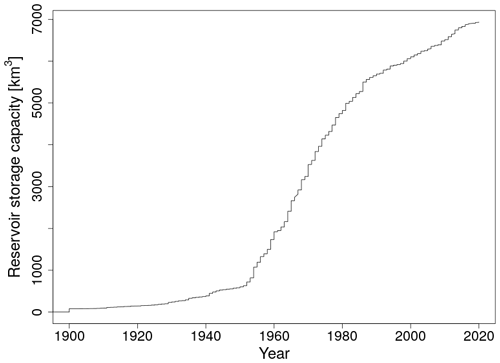
Figure 9Cumulative reservoir storage capacity between 1900 and 2020. Reservoirs that were active before the year 1901 have been assigned to the year 1900. The horizontal axis shows the year of construction, completion, or commissioning, reflecting ambiguity in available data.
The original GRanDv1.3 dam locations were mapped to the global 30 min drainage direction map (ISIMIPddm30, (Müller Schmied, 2022) based on DDM30 (Döll and Lehner, 2002) by applying the following algorithm.
Firstly, the locations have been rounded to the closest 0.5∘ grid cell center. Then, the area of the upstream catchment draining into the GRanD reservoirs (previous version of GRanDv1.3) in the ISIMIPddm30 map has been calculated and compared against the ones reported in GRanD. All dams with an upstream area bigger than 10 000 km2 in GRanD and more than 50 % deviation from the GRanD upstream area have been shifted to the eight possible neighboring cells. If any of these shifts resulted in a smaller deviation from the GRanD upstream areas, the dam was moved to the grid cell resulting in the smallest deviation in the upstream area.
Additionally, a visual validation and, where appropriate, manual relocation were applied with the aim of finding the best-fitting grid cell from a hydrological perspective. Due to the low resolution of the model grid, reservoirs might get wrongly assigned to, e.g., the main stream (either before or after the confluence of two rivers), even though the dam is located in a particular tributary according to the database.
In those cases, and based on visual GIS inspection, the best location was searched, e.g., by moving the dam location one cell upstream to preserve the routing order and to avoid a different or highly deviating river basin in the ISIMIPddm30 stream network. In the case that a dam is not assigned to any river basin in the ISIMIPddm30 (which can happen due to the difference in spatial resolution), the most suitable location according to the observed upstream area was selected. Because of limited capacity, this visual validation procedure was applied only for dams present in the earlier GranDv1.1 version that have a maximum storage capacity greater than 0.5 km3 (1108 dams), as well as for all 458 additional dams in GRanDv1.3 and the 11 dams (excluding post-2020 dams) added from GeoDAR v1.2 but not for several thousand smaller dams present in GranDv1.1. In total the reported dams have a global cumulative storage capacity of approximately 6932 km3 (Fig. 9).
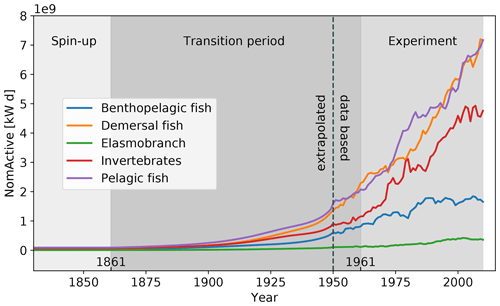
Figure 10Evolution of historical nominal active fishing effort (NomActive) as provided for the spin-up, transition period, and “obsclim + histoc; default” ISIMIP3a experiment, separated by target functional group. The groups represent an aggregation of 29 even finer categories covered by the dataset (see Table 17).
Table 17Information about historical fishing intensities provided as DHF within ISIMIP3a. For the spin-up + transition period required by models within the marine ecosystems and fisheries sector the forcing is provided for 1841–2010, although the “obsclim + histoc; default” experiment only starts in 1961.
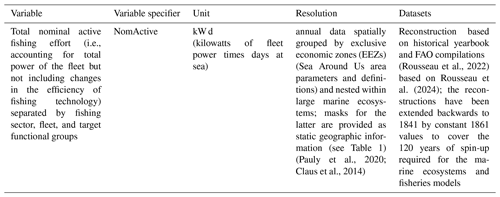
4.9 Fishing intensities
The dataset of reconstructed historical fishing efforts (Rousseau et al., 2022) serves as the DHF for the marine ecosystems and fisheries sector. The efforts are quantified separately for the artisanal and industrial fishing (sector), 66 large marine ecosystems (LMEs), 187 national exclusive economic zones (EEZs) and “high seas”, 244 country identifiers from the Sea Around Us Project (SAUP), 16 different categories of applied gear (e.g., bottom trawls, long lines, and purse seines), and 29 target functional groups (see nominal active fishing effort for five aggregated categories in Fig. 10).
The original annual time series spanning 1950–2015 were further extrapolated into the past to 1861 using generalized additive models (Rousseau et al., 2024; see Fig. 10). To cover the spin-up + transition period from 1841–1960 the dataset has been extended backwards by 1861 values. Forcing with this dataset allows for a comparison of simulated catches against the congruent (Watson, 2019) reconstruction of historical fishery catches (spanning the period 1869–2015; (Watson and Tidd, 2018). To permit integration into marine ecosystem models that capture different fishing sectors, fleets, and functional groups these data include nominal active fishing effort disaggregated by location (exclusive economic zone, high seas, and large marine ecosystem), fishing country, fishing gear, targeted functional group, and fishing sector (coastal artisanal and industrial). Impact modelers are allowed to distribute this effort across space and time, as well as to target organisms in any method compatible with their models' structure. The fishing effort data do not include any information about changes in the efficiency of fishing technology over time (technological creep). Assumptions about these efficiencies are left to the individual modelers and usually determined in model calibration.
4.10 Forest management for the regional forest sector
For the regional forest sector, forest management is defined for nine forest sites in Europe, four of them in Germany (Peitz, KROOF, Solling beech, Solling spruce) and one each in the Czech Republic (Bily Kriz), Denmark (Sorø), France (Le Bray), Italy (Collelongo), and Finland (Hyytiälä) (Reyer et al., 2020b).
Additionally, a set of forest-site-specific management rules and planting numbers based on historical standard management practices of the area where the forest sites are located is defined and spelled out in concrete management schedules to enable modelers to simulate 2015soc conditions (Reyer et al., 2023). The regional forest management data have not been harmonized to the global gridded wood harvest data provided for the biomes sector because the data are very site-specific and the variation not resolved in the global dataset.
The first part of the third simulation round of the Inter-Sectoral Impact Model Intercomparison Project ISIMIP (ISIMIP3a) is intended to facilitate impact model evaluation and impact attribution experiments to significantly move forward our understanding of observed changes in natural and human systems and their respective drivers. Impact models participating in ISIMIP encode our process knowledge on how several drivers (climate-related ones as well as direct human influences) come together to generate observed changes. As such, they are ideal tools for this task. The new ISIMIP3a simulation framework, including the provision of the relevant forcing data, is intended to unleash the power of a wide range of models from different sectors to quantify the contribution of observed changes in climate-related systems to observed environmental or societal changes.
As a first step towards impact attribution, the ISIMIP3a evaluation experiments will help to clarify how well the current generation of impact models can explain observed changes in impacted systems based on provided information about the different forcings. The performance of the models in reproducing observed variations and long-term changes in the impacted systems certainly does not depend only on the models themselves but also on the availability and uncertainties associated with the climate-related and direct human forcings (see Table 1). We capture some of this uncertainty by providing four different observational atmospheric climate forcing data and associated counterfactual forcings (see Sect. 2.1) as well as TC wind fields derived from two different modeling approaches (see Sect. 3.2). Uncertainties in the direct human forcings are represented to the degree that the forcing datasets considered to be “optional” vary from model to model. In addition, the multi-model framework of ISIMIP allows for testing to what degree different process-representations may be better suited to explain the observations than others.
High explanatory power is then a prerequisite for impact attribution through the ISIMIP3a attribution experiments based on counterfactual climate-related forcings following the IPCC definition (O'Neill et al., 2022).
The setup is the first that allows easily and broadly addressing impact attribution across many impact categories. This will fill an important gap as only few process-based impact models have been used in this field despite their general suitability. The presented work can thus lay the foundation for urgently necessary works to inform climate litigation (Burger et al., 2020; Burger and Tigre, 2023), the loss and damage debate (Mechler et al., 2018; Wyns, 2023), and last but not least also decisions about short-term adaptation measures. It will ultimately help to carve out the sensitivity of our ecosystems and human societies to historical climate change, which is a precondition for robustly projecting future climate impacts.
This paper aims to give an overview of the ISIMIP3a experiments and the provided climate-related and direct human forcing datasets. It is intended to work as a catalogue where modelers can find all relevant information about the datasets they need for the impact model simulations within ISIMIP3a. As a community-driven initiative across multiple disciplines the selection of the best available forcing data for ISIMIP builds on the expertise within the different sectoral communities.
We would like to improve or complement these datasets in a continuous process wherever possible. So this paper can also be read as a call for contributing additional data that could (i) be provided within the current round (ISIMIP3) as optional data (see explanation in the Introduction) that are not harmonized within or across sectors or (ii) as mandatory forcing for an upcoming simulation round. In particular, we aim for temporally resolved historical growing seasons that have been shown to be critical to reproduce observed crop yields (Jägermeyr and Frieler, 2018), counterfactual oceanic climate-related forcings, counterfactual TC-related precipitation (Risser and Wehner, 2017; van Oldenborgh et al., 2017; Wang et al., 2018; Patricola and Wehner, 2018), temporally resolved lightning data for the full set of considered climate model simulations, and temporally resolved human drainage and restoration activities in peatlands as one of the key controls over global peatland greenhouse gas emissions (Loisel et al., 2020).
All input data described are available for participating modelers with a respective account from the DKRZ server. Data will be made publicly available, and most data are already publicly available at https://data.isimip.org/ (ISIMIP data repository, 2023). Availability is documented at https://www.isimip.org/gettingstarted/input-data-bias-adjustment/ (ISIMIP Input data table, 2023) where the way to access the data is described as well. Model output is already partly available at https://data.isimip.org/ (ISIMIP data repository, 2023).
The ISIMIP repository fulfills the archive standards as stated in the “GMD code and data policy”. The repository is hosted and maintained by the Potsdam Institute for Climate Impact Research (PIK). Data can only be published or removed from the repository by the ISIMIP data team, which is monitored by the ISIMIP steering committee according to the organizational structure of ISIMIP (ISIMIP organigram, 2020). DOIs are used to refer to datasets in a persistent way. Whenever a dataset is replaced for any reason a copy is kept on tape, and a new DOI is issued, while the old DOI is kept online with information on how to retrieve the archived data. Detailed information can be found in the ISIMIP terms of use (ISIMIP terms of use, 2023).
KF led the project and developed the concept with contributions from JS, MM, CO, CPOR, JLB, CSH, CMP, TDE, KOC, CN, RH, DT, OM, JJ, GL, SC, EB, AGS, NS, JC, SH, CB, AG, FL, SNG, HMS, FH, TH, RM, DP, WT, DMB, and MB. JV supported the data generation and harmonization of the protocol across all sectors. SL provided atmospheric climate forcing data. MM provided coastal water-level data and atmospheric forcing data. MdRRL, JW, and FY provided dam data. CO and IJS provided GDP data. CPOR provided forest management data. DNK and JTM provided high-resolution climate forcing data. ST provided coastal water levels and counterfactual climate forcing data. YR provided data on fishing efforts. CS and XL provided ocean forcing data. TV provided TC data. TW and FS provided gridded GDP data. IV provided lake data. JJ provided growing seasons. CM provided soil data. KF prepared the paper with contributions from all co-authors.
At least one of the (co-)authors is a member of the editorial board of Geoscientific Model Development. The peer-review process was guided by an independent editor, and the authors also have no other competing interests to declare.
Publisher's note: Copernicus Publications remains neutral with regard to jurisdictional claims made in the text, published maps, institutional affiliations, or any other geographical representation in this paper. While Copernicus Publications makes every effort to include appropriate place names, the final responsibility lies with the authors.
This article is based upon work from COST Action CA19139 PROCLIAS (PROcess-based models for CLimate Impact Attribution across Sectors), supported by COST (European Cooperation in Science and Technology; https://www.cost.eu, last access: 21 December 2023). Funding from the EU Horizon 2020 research and innovation program under grant agreement 821010 (CASCADES) supported the work of Christopher P. O. Reyer, Jan Volkholz, and Iliusi D. Vega del Valle, the provisioning of the high-resolution climate data, and the work of Simon Treu under grant agreement no. 820712 (RECEIPT). Stefan Lange received funding from the German Research Foundation (DFG, project number 427397136). The German Federal Ministry of Education and Research (BMBF) supported the work under the research projects ISIAccess (16QK05), SLICE (01LA1829A), QUIDIC (01LP1907A), CHIPS (01LS1904A), and ISIpedia (01LS1711A). Fang Li received funding from the National Key Research and Development Program of China (project number 2022YFE0106500). Jinfeng Chang received funding from the National Key Research and Development Program of China (project number 2022YFF0801904). MB acknowledges funding from the Research Foundation – Flanders (FWO, G095720N). Sarah Chadburn and Noah Smith were supported by the National Environmental Research Council (NERC) under grant NE/R015791/1. Sarah Chadburn, Angela Gallego-Sala, Michel Bechtold, and Noah Smith acknowledge funding through NERC NE/V01854X/1 (MOTHERSHIP). Chantelle Burton was supported by the Newton Fund through the Met Office. The research by Dirk Nikolaus Karger was funded through the 2019–2020 BiodivERsA joint call for research proposals under the BiodivClim ERA-Net COFUND program and through the funding organization of the Swiss National Science Foundation (SNF; project FeedBaCks, 193907; project Adohris, 205530). Rafael Marcé was supported by the Alter-C project (PID2020-114024GB-C32/AEI /10.13039/501100011033). Cheryl S. Harrison was supported by Open Philanthropy, NSF award 2218777, and NOAA CPO. We also thank Jason Evans for his positive review and an anonymous reviewer for an extremely careful and comprehensive review that significantly contributed to the improvement of the paper.
The underlying work for putting together this paper was a community effort that could be achieved by the support of manifold and diverse projects as outlined in the acknowledgements.
For preparing and publishing the paper we received support from the COST Action CA19139 PROCLIAS (PROcess-based models for CLimate Impact Attribution across Sectors), supported by COST (European Cooperation in Science and Technology; https://www.cost.eu, last access: 21 December 2023).
The publication of this article was funded by the Open Access Fund of the Leibniz Association.
This paper was edited by Jinkyu Hong and reviewed by Jason Evans and one anonymous referee.
Anon: World development indicators, World Bank Publications, Washington, D.C., 2008.
Arujo, E., Bodirsky, B. L., Crawford, M. S., Leip, D., and Dietrich, J.: MissingIslands dataset for filling in data gaps from the WDI datasets, Zenodo [data set], https://doi.org/10.5281/zenodo.4421504, 2021.
Bloemendaal, N., de Moel, H., Mol, J. M., Bosma, P. R. M., Polen, A. N., and Collins, J. M.: Adequately reflecting the severity of tropical cyclones using the new Tropical Cyclone Severity Scale, Environ. Res. Lett., 16, 014048, https://doi.org/10.1088/1748-9326/abd131, 2021.
Brun, P., Zimmermann, N. E., Hari, C., Pellissier, L., and Karger, D. N.: CHELSA-BIOCLIM+ A novel set of global climate-related predictors at kilometre-resolution, ENVIDAT [data set], https://doi.org/10.16904/envidat.332, 2022a.
Brun, P., Zimmermann, N. E., Hari, C., Pellissier, L., and Karger, D. N.: Global climate-related predictors at kilometer resolution for the past and future, Earth Syst. Sci. Data, 14, 5573–5603, https://doi.org/10.5194/essd-14-5573-2022, 2022b.
Büchner, M. and Reyer, C.: ISIMIP3a atmospheric composition input data, https://doi.org/10.48364/ISIMIP.664235.2, 2022.
Burger, M. and Tigre, M. A.: Global Climate Litigation Report: 2023 Status Review, 2023.
Burger, M., Wentz, J., and Horton, R.: The Law and Science of Climate Change Attribution, Columbia Journal of Environmental Law, 1, 45, https://doi.org/10.7916/cjel.v45i1.4730, 2020.
Caron, L., Ivins, E. R., Larour, E., Adhikari, S., Nilsson, J., and Blewitt, G.: GIA model statistics for GRACE hydrology, cryosphere, and ocean science, Geophys. Res. Lett., 45, 2203–2212, 2018.
Cecil, D.: LIS/OTD 0.5 Degree High Resolution Monthly Climatology (HRMC), NASA Global Hydrometeorology Resource Center DAAC, Huntsville, Alabama, USA [data set], https://doi.org/10.5067/LIS/LIS-OTD/DATA303, 2006.
Chavas, D. R. and Lin, N.: A Model for the Complete Radial Structure of the Tropical Cyclone Wind Field. Part II: Wind Field Variability, J. Atmos. Sci., 73, 3093–3113, 2016.
Claus, S., De Hauwere, N., Vanhoorne, B., Deckers, P., Souza Dias, F., Hernandez, F., and Mees, J.: Marine Regions: Towards a global standard for georeferenced marine names and boundaries, Marine Geodesy, 37, 99–125, https://doi.org/10.1080/01490419.2014.902881, 2014.
Compo, G. P., Whitaker, J. S., Sardeshmukh, P. D., Matsui, N., Allan, R. J., Yin, X., Gleason, B. E., Vose, R. S., Rutledge, G., Bessemoulin, P., Brönnimann, S., Brunet, M., Crouthamel, R. I., Grant, A. N., Groisman, P. Y., Jones, P. D., Kruk, M. C., Kruger, A. C., Marshall, G. J., Maugeri, M., Mok, H. Y., Nordli, Ø., Ross, T. F., Trigo, R. M., Wang, X. L., Woodruff, S. D., and Worley, S. J.: The twentieth century reanalysis project, Q. J. Roy. Meteor. Soc., 137, 1–28, 2011.
Cramer, W., Yohe, G. W., Auffhammer, M., Huggel, C., Molau, U., da Silva Dias, M. A. F., Solow, A., Stone, D. A., and Tibig, L.: Detection and attribution of observed impacts, in: Climate Change 2014: Impacts, Adaptation, and Vulnerability. Part A: Global and Sectoral Aspects, Contribution of Working Group II to the Fifth Assessment Report of the Intergovernmental Panel on Climate Change, edited by: Field, C. B., Barros, V. R., Dokken, D. J., Mach, K. J., Mastrandrea, M. D., Bilir, T. E., Chatterjee, M., Ebi, K. L., Estrada, Y. O., Genova, R. C., Girma, B., Kissel, E. S., Levy, A. N., MacCracken, S., Mastrandrea, P. R., and White, L. L., Cambridge University Press, Cambridge, United Kingdom and New York, NY, USA, 979–1037, ISBN 978-1-107-05807-1, 2014.
Cucchi, M., Weedon, G. P., Amici, A., Bellouin, N., Lange, S., Müller Schmied, H., Hersbach, H., and Buontempo, C.: WFDE5: bias-adjusted ERA5 reanalysis data for impact studies, Earth Syst. Sci. Data, 12, 2097–2120, https://doi.org/10.5194/essd-12-2097-2020, 2020.
Cyclone Database Manager: https://citeseerx.ist.psu.edu/document?repid=rep1&type=pdf&doi=b80049f3add16c6c4b8937c8f6b804071dd110b3, last access: 18 October 2023.
Dangendorf, S., Hay, C., Calafat, F. M., Marcos, M., Piecuch, C. G., Berk, K., and Jensen, J.: Persistent acceleration in global sea-level rise since the 1960s, Nat. Clim. Chang., 9, 705–710, 2019.
Danielson, J. J. and Gesch, D. B.: Global multi-resolution terrain elevation data 2010 (GMTED2010), U.S. Geological Survey, https://doi.org/10.3133/ofr20111073, 2011.
Davis, N. N., Badger, J., Hahmann, A. N., Hansen, B. O., Mortensen, N. G., Kelly, M., Larsén, X. G., Olsen, B. T., Floors, R., Lizcano, G., Casso, P., Lacave, O., Bosch, A., Bauwens, I., Knight, O. J., Potter van Loon, A., Fox, R., Parvanyan, T., Krohn Hansen, S. B., Heathfield, D., Onninen, M., and Drummond, R.: The Global Wind Atlas: A high-resolution dataset of climatologies and associated web-based application, B. Am. Meteorol. Soc., 104, E1507–E1525, https://doi.org/10.1175/BAMS-D-21-0075.1, 2023.
del Valle, I. V., Reyer, C., and Perrette, M.: ISIMIP3a wood harvesting input data, https://doi.org/10.48364/ISIMIP.482888.1, 2022.
Dirmeyer, P. A., Gao, X., Zhao, M., Guo, Z., Oki, T., and Hanasaki, N.: GSWP-2: Multimodel Analysis and Implications for Our Perception of the Land Surface, B. Am. Meteorol. Soc., 87, 1381–1398, 2006.
Döll, P. and Lehner, B.: Validation of a new global 30-min drainage direction map, J. Hydrol., 258, 214–231, 2002.
Eberenz, S., Stocker, D., Röösli, T., and Bresch, D. N.: LitPop: Global Exposure Data for Disaster Risk Assessment, ETH Zürich Research Collection [data set], https://doi.org/10.3929/ethz-b-000331316, 2019.
Eberenz, S., Lüthi, S., and Bresch, D. N.: Regional tropical cyclone impact functions for globally consistent risk assessments, Nat. Hazards Earth Syst. Sci., 21, 393–415, https://doi.org/10.5194/nhess-21-393-2021, 2021.
Emanuel, K. and Rotunno, R.: Self-Stratification of Tropical Cyclone Outflow. Part I: Implications for Storm Structure, J. Atmos. Sci., 68, 2236–2249, 2011.
Fagnant, C., Gori, A., Sebastian, A., Bedient, P. B., and Ensor, K. B.: Characterizing spatiotemporal trends in extreme precipitation in Southeast Texas, Nat. Hazards, 104, 1597–1621, 2020.
FAO: Fertilizer data, FAOSTAT Database, Food and Agriculture Organization of the United Nations, Rome, Italy, http://www.fao.org/faostat/en/#data/RFN (last access: 22 December 2023), 2016.
Feenstra, R. C., Inklaar, R., Timmer, M., and Woltjer, P.: Penn World Table 10.0, https://doi.org/10.15141/S5Q94M, 2015.
Feldmann, M., Emanuel, K., Zhu, L., and Lohmann, U.: Estimation of Atlantic Tropical Cyclone Rainfall Frequency in the United States, J. Appl. Meteorol. Climatol., 58, 1853–1866, 2019.
Fiddes, J. and Gruber, S.: TopoSCALE v.1.0: downscaling gridded climate data in complex terrain, Geosci. Model Dev., 7, 387–405, https://doi.org/10.5194/gmd-7-387-2014, 2014.
Friedlingstein, P., O'Sullivan, M., Jones, M. W., Andrew, R. M., Gregor, L., Hauck, J., Le Quéré, C., Luijkx, I. T., Olsen, A., Peters, G. P., Peters, W., Pongratz, J., Schwingshackl, C., Sitch, S., Canadell, J. G., Ciais, P., Jackson, R. B., Alin, S. R., Alkama, R., Arneth, A., Arora, V. K., Bates, N. R., Becker, M., Bellouin, N., Bittig, H. C., Bopp, L., Chevallier, F., Chini, L. P., Cronin, M., Evans, W., Falk, S., Feely, R. A., Gasser, T., Gehlen, M., Gkritzalis, T., Gloege, L., Grassi, G., Gruber, N., Gürses, Ö., Harris, I., Hefner, M., Houghton, R. A., Hurtt, G. C., Iida, Y., Ilyina, T., Jain, A. K., Jersild, A., Kadono, K., Kato, E., Kennedy, D., Klein Goldewijk, K., Knauer, J., Korsbakken, J. I., Landschützer, P., Lefèvre, N., Lindsay, K., Liu, J., Liu, Z., Marland, G., Mayot, N., McGrath, M. J., Metzl, N., Monacci, N. M., Munro, D. R., Nakaoka, S.-I., Niwa, Y., O'Brien, K., Ono, T., Palmer, P. I., Pan, N., Pierrot, D., Pocock, K., Poulter, B., Resplandy, L., Robertson, E., Rödenbeck, C., Rodriguez, C., Rosan, T. M., Schwinger, J., Séférian, R., Shutler, J. D., Skjelvan, I., Steinhoff, T., Sun, Q., Sutton, A. J., Sweeney, C., Takao, S., Tanhua, T., Tans, P. P., Tian, X., Tian, H., Tilbrook, B., Tsujino, H., Tubiello, F., van der Werf, G. R., Walker, A. P., Wanninkhof, R., Whitehead, C., Willstrand Wranne, A., Wright, R., Yuan, W., Yue, C., Yue, X., Zaehle, S., Zeng, J., and Zheng, B.: Global Carbon Budget 2022, Earth Syst. Sci. Data, 14, 4811–4900, https://doi.org/10.5194/essd-14-4811-2022, 2022.
Frieler, K.: Scenario Set-up and the new CMIP6-based climate-related forcings provided within the third round of the Inter-Sectoral Model Intercomparison Project (ISIMIP3b, group I and II), Geosci. Model Dev., submitted, 2024.
Frieler, K., Lange, S., Piontek, F., Reyer, C. P. O., Schewe, J., Warszawski, L., Zhao, F., Chini, L., Denvil, S., Emanuel, K., Geiger, T., Halladay, K., Hurtt, G., Mengel, M., Murakami, D., Ostberg, S., Popp, A., Riva, R., Stevanovic, M., Suzuki, T., Volkholz, J., Burke, E., Ciais, P., Ebi, K., Eddy, T. D., Elliott, J., Galbraith, E., Gosling, S. N., Hattermann, F., Hickler, T., Hinkel, J., Hof, C., Huber, V., Jägermeyr, J., Krysanova, V., Marcé, R., Müller Schmied, H., Mouratiadou, I., Pierson, D., Tittensor, D. P., Vautard, R., van Vliet, M., Biber, M. F., Betts, R. A., Bodirsky, B. L., Deryng, D., Frolking, S., Jones, C. D., Lotze, H. K., Lotze-Campen, H., Sahajpal, R., Thonicke, K., Tian, H., and Yamagata, Y.: Assessing the impacts of 1.5 ∘C global warming – simulation protocol of the Inter-Sectoral Impact Model Intercomparison Project (ISIMIP2b), Geosci. Model Dev., 10, 4321–4345, https://doi.org/10.5194/gmd-10-4321-2017, 2017.
Geiger, T., Frieler, K., and Bresch, D. N.: A global historical data set of tropical cyclone exposure (TCE-DAT), Earth Syst. Sci. Data, 10, 185–194, https://doi.org/10.5194/essd-10-185-2018, 2018.
GGCMI-HWSD: https://github.com/AgMIP-GGCMI/processing_hwsd_for_GGCMI, last access: 9 February 2023.
Gillett, N. P., Shiogama, H., Funke, B., Hegerl, G., Knutti, R., Matthes, K., Santer, B. D., Stone, D., and Tebaldi, C.: The Detection and Attribution Model Intercomparison Project (DAMIP v1.0) contribution to CMIP6, Geosci. Model Dev., 9, 3685–3697, https://doi.org/10.5194/gmd-9-3685-2016, 2016.
Global soil wetness project phase 3 – GSWP3 documentation: http://hydro.iis.u-tokyo.ac.jp/GSWP3/, last access: 9 January 2023.
Golub, M., Thiery, W., Marcé, R., Pierson, D., Vanderkelen, I., Mercado-Bettin, D., Woolway, R. I., Grant, L., Jennings, E., Kraemer, B. M., Schewe, J., Zhao, F., Frieler, K., Mengel, M., Bogomolov, V. Y., Bouffard, D., Côté, M., Couture, R.-M., Debolskiy, A. V., Droppers, B., Gal, G., Guo, M., Janssen, A. B. G., Kirillin, G., Ladwig, R., Magee, M., Moore, T., Perroud, M., Piccolroaz, S., Raaman Vinnaa, L., Schmid, M., Shatwell, T., Stepanenko, V. M., Tan, Z., Woodward, B., Yao, H., Adrian, R., Allan, M., Anneville, O., Arvola, L., Atkins, K., Boegman, L., Carey, C., Christianson, K., de Eyto, E., DeGasperi, C., Grechushnikova, M., Hejzlar, J., Joehnk, K., Jones, I. D., Laas, A., Mackay, E. B., Mammarella, I., Markensten, H., McBride, C., Özkundakci, D., Potes, M., Rinke, K., Robertson, D., Rusak, J. A., Salgado, R., van der Linden, L., Verburg, P., Wain, D., Ward, N. K., Wollrab, S., and Zdorovennova, G.: A framework for ensemble modelling of climate change impacts on lakes worldwide: the ISIMIP Lake Sector, Geosci. Model Dev., 15, 4597–4623, https://doi.org/10.5194/gmd-15-4597-2022, 2022.
Gori, A., Lin, N., and Smith, J.: Assessing compound flooding from landfalling tropical cyclones on the North Carolina coast, Water Resour. Res., 56, e2019WR026788, https://doi.org/10.1029/2019wr026788, 2020.
Gori, A., Lin, N., Xi, D., and Emanuel, K.: Tropical cyclone climatology change greatly exacerbates US extreme rainfall–surge hazard, Nat. Clim. Chang., 12, 171–178, 2022.
Hansen, G., Stone, D., Auffhammer, M., Huggel, C., and Cramer, W.: Linking local impacts to changes in climate: a guide to attribution, Regional Environ. Change, 16, 527–541, 2016.
Hersbach, H., Bell, B., Berrisford, P., Hirahara, S., Horányi, A., Muñoz-Sabater, J., Nicolas, J., Peubey, C., Radu, R., Schepers, D., Simmons, A., Soci, C., Abdalla, S., Abellan, X., Balsamo, G., Bechtold, P., Biavati, G., Bidlot, J., Bonavita, M., Chiara, G., Dahlgren, P., Dee, D., Diamantakis, M., Dragani, R., Flemming, J., Forbes, R., Fuentes, M., Geer, A., Haimberger, L., Healy, S., Hogan, R. J., Hólm, E., Janisková, M., Keeley, S., Laloyaux, P., Lopez, P., Lupu, C., Radnoti, G., Rosnay, P., Rozum, I., Vamborg, F., Villaume, S., and Thépaut, J.-N.: The ERA5 global reanalysis, Q. J. Roy. Meteor. Soc., 146, 1999–2049, 2020.
Holland, G.: A Revised Hurricane Pressure–Wind Model, Mon. Weather Rev., 136, 3432–3445, 2008.
Holland, G. J.: An Analytic Model of the Wind and Pressure Profiles in Hurricanes, Mon. Weather Rev., 108, 1212–1218, 1980.
Hope, P., Cramer, W., van Aalst, M., Flato, G., Frieler, K., Gillett, N., Huggel, C., Minx, J., Otto, F., Parmesan, C., Rogelj, J., Rojas, M., Seneviratne, S. I., Slangen, A., Stone, D., Terray, L., Vautard, R., and Zhang, X.: Cross-Working Group Box ATTRIBUTION | Attribution in the IPCC Sixth Assessment Report, in: Climate Change 2022 – Impacts, Adaptation and Vulnerability: Working Group II Contribution to the Sixth Assessment Report of the Intergovernmental Panel on Climate Change, edited by: Pörtner, H.-O., Roberts, D. C., Tignor, M., Poloczanska, E. S., Mintenbeck, K., Alegría, A., Craig, M., Langsdorf, S., Löschke, S., Möller, V., Okem, A., and Rama, B., Cambridge University Press, 121–196, https://doi.org/10.1017/9781009325844, 2022.
Hurtt, G. C., Chini, L. P., Frolking, S., Betts, R. A., Feddema, J., Fischer, G., Fisk, J. P., Hibbard, K., Houghton, R. A., Janetos, A., Jones, C. D., Kindermann, G., Kinoshita, T., Klein Goldewijk, K., Riahi, K., Shevliakova, E., Smith, S., Stehfest, E., Thomson, A., Thornton, P., van Vuuren, D. P., and Wang, Y. P.: Harmonization of land-use scenarios for the period 1500–2100: 600 years of global gridded annual land-use transitions, wood harvest, and resulting secondary lands, Clim. Change, 109, 117, https://doi.org/10.1007/s10584-011-0153-2, 2011.
Hurtt, G. C., Chini, L., Sahajpal, R., Frolking, S., Bodirsky, B. L., Calvin, K., Doelman, J. C., Fisk, J., Fujimori, S., Klein Goldewijk, K., Hasegawa, T., Havlik, P., Heinimann, A., Humpenöder, F., Jungclaus, J., Kaplan, J. O., Kennedy, J., Krisztin, T., Lawrence, D., Lawrence, P., Ma, L., Mertz, O., Pongratz, J., Popp, A., Poulter, B., Riahi, K., Shevliakova, E., Stehfest, E., Thornton, P., Tubiello, F. N., van Vuuren, D. P., and Zhang, X.: Harmonization of global land use change and management for the period 850–2100 (LUH2) for CMIP6, Geosci. Model Dev., 13, 5425–5464, https://doi.org/10.5194/gmd-13-5425-2020, 2020.
Nachtergaele, F., van Velthuizen, H., and Verelst, L.: Harmonized World Soil Database (HWSD). Version 1.1, http://www.fao.org/fileadmin/templates/nr/documents/HWSD/HWSD_Documentation.pdf (last access: 21 December 2023), 2009.
IFASTAT: Statistics, International Fertilizer Association, IFASTAT Database [data set], https://www.ifastat.org, last access: 21 January 2015.
International Monetary Fund: Recovery during a Pandemic: Health Concerns, Supply Disruptions, and Price Pressures, World Economic Outlook/International Monetary Fund 2021, October, International Monetary Fund, Washington, DC, ISBN 978-1-5135-7752-4, 2021.
IPCC: Annex II: Glossary, in: Climate Change 2014: Synthesis Report. Contribution of Working Groups I, II and III to the Fifth Assessment Report of the Intergovernmental Panel on Climate Change, edited by: Mach, K. J., Planton, S., von Stechow, C., Pachauri, R. K., and Meyer, L. A., Geneva, Switzerland, 117–130, 2014.
ISIMIP Coordination Team, Sectoral Coordinators, Scientific Advisory Board: The Inter-Sectoral Impact Model Intercomparison Project (ISIMIP), Mission & Implementation Document, https://www.isimip.org/documents/646/MissionAndImplementation_12Sep2018_5Hlvj2N.pdf (last access: 21 December 2023), 2018.
ISIMIP data repository: ISIMIP [data set], https://data.isimip.org/, last access: 21 December 2023.
ISIMIP Input data table: ISIMIP [data set], https://www.isimip.org/gettingstarted/input-data-bias-adjustment/, last access: 21 December 2023.
ISIMIP organigram: https://www.isimip.org/about/#organisational-structure, last access: 7 March 2023.
ISIMIP Output Data Table: https://www.isimip.org/outputdata/, last access: 19 October 2023.
ISIMIP terms of use: https://www.isimip.org/gettingstarted/terms-of-use/, last access: 14 January 2023.
ISIMIP2a: suspicious gridded GDP per capita data; new functions in the isimip data repository; Forum on Scenarios for Climate and Societal Futures: https://www.isimip.org/newsletter/simip2a-suspicious-gridded-gdp-capita-data-new-functions-isimip-data-repository-forum-scenarios-climate-and-societal-futures/, last access: 10 February 2023.
ISIMIP3 simulation protocol: https://protocol.isimip.org/, last access: 14 January 2023.
Jägermeyr, J. and Frieler, K.: Spatial variations in crop growing seasons pivotal to reproduce global fluctuations in maize and wheat yields, Sci. Adv., 4, eaat4517, https://doi.org/10.1126/sciadv.aat4517, 2018.
Jägermeyr, J., Müller, C., Minoli, S., Ray, D., and Siebert, S.: GGCMI Phase 3 crop calendar, Zenodo [data set], https://doi.org/10.5281/ZENODO.5062513, 2021a.
Jägermeyr, J., Müller, C., Ruane, A. C., Elliott, J., Balkovic, J., Castillo, O., Faye, B., Foster, I., Folberth, C., Franke, J. A., Fuchs, K., Guarin, J. R., Heinke, J., Hoogenboom, G., Iizumi, T., Jain, A. K., Kelly, D., Khabarov, N., Lange, S., Lin, T.-S., Liu, W., Mialyk, O., Minoli, S., Moyer, E. J., Okada, M., Phillips, M., Porter, C., Rabin, S. S., Scheer, C., Schneider, J. M., Schyns, J. F., Skalsky, R., Smerald, A., Stella, T., Stephens, H., Webber, H., Zabel, F., and Rosenzweig, C.: Climate impacts on global agriculture emerge earlier in new generation of climate and crop models, Nature Food, 2, 873–885, 2021b.
Karger, D. N., Conrad, O., Böhner, J., Kawohl, T., Kreft, H., Soria-Auza, R. W., Zimmermann, N. E., Linder, H. P., and Kessler, M.: Climatologies at high resolution for the earth's land surface areas, Sci. Data, 4, 170122, https://doi.org/10.1038/sdata.2017.122, 2017.
Karger, D. N., Wilson, A. M., Mahony, C., Zimmermann, N. E., and Jetz, W.: Global daily 1 km land surface precipitation based on cloud cover-informed downscaling, Sci. Data, 8, 307, https://doi.org/10.1038/s41597-021-01084-6, 2021.
Karger, D. N., Lange, S., Hari, C., Reyer, C. P. O., and Zimmermann, N. E.: CHELSA-W5E5 v1.0: W5E5 v1.0 downscaled with CHELSA v2.0, https://doi.org/10.48364/ISIMIP.836809.3, 2022.
Karger, D. N., Lange, S., Hari, C., Reyer, C. P. O., Conrad, O., Zimmermann, N. E., and Frieler, K.: CHELSA-W5E5: daily 1 km meteorological forcing data for climate impact studies, Earth Syst. Sci. Data, 15, 2445–2464, https://doi.org/10.5194/essd-15-2445-2023, 2023.
Khazaei, B., Read, L. K., Casali, M., Sampson, K. M., and Yates, D. N.: GLOBathy, the global lakes bathymetry dataset, Sci. Data, 9, 36, https://doi.org/10.1038/s41597-022-01132-9, 2022.
Kim, H.: Global Soil Wetness Project Phase 3 Atmospheric Boundary Conditions (Experiment 1), https://doi.org/10.20783/DIAS.501, 2017.
Klein Goldewijk, K., Beusen, A., Doelman, J., and Stehfest, E.: Anthropogenic land use estimates for the Holocene – HYDE 3.2, Earth Syst. Sci. Data, 9, 927–953, https://doi.org/10.5194/essd-9-927-2017, 2017.
Knapp, K. R. and Kruk, M. C.: Quantifying Interagency Differences in Tropical Cyclone Best-Track Wind Speed Estimates, Mon. Weather Rev., 138, 1459–1473, 2010.
Knapp, K. R., Kruk, M. C., Levinson, D. H., Diamond, H. J., and Neumann, C. J.: The International Best Track Archive for Climate Stewardship (IBTrACS): Unifying Tropical Cyclone Data, B. Am. Meteorol. Soc., 91, 363–376, 2010.
Koch, J. and Leimbach, M.: SSP economic growth projections: Major changes of key drivers in integrated assessment modelling, Ecol. Econ., 206, 107751, https://doi.org/10.1016/j.ecolecon.2023.107751, 2023.
Konzelmann, T., van de Wal, R. S. W., Greuell, W., Bintanja, R., Henneken, E. A. C., and Abe-Ouchi, A.: Parameterization of global and longwave incoming radiation for the Greenland Ice Sheet, Glob. Planet. Change, 9, 143–164, 1994.
Krien, Y., Dudon, B., Roger, J., Arnaud, G., and Zahibo, N.: Assessing storm surge hazard and impact of sea level rise in the Lesser Antilles case study of Martinique, Nat. Hazards Earth Syst. Sci., 17, 1559–1571, https://doi.org/10.5194/nhess-17-1559-2017, 2017.
Lan, X., Tans, P., and Thoning, K. W.: Trends in globally-averaged CO2 determined from NOAA Global Monitoring Laboratory measurements. Version 2023-01 NOAA/GML, 2023.
Land use harmonization: https://luh.umd.edu, last access: 9 January 2023.
Lange, S.: Trend-preserving bias adjustment and statistical downscaling with ISIMIP3BASD (v1.0), Geosci. Model Dev., 12, 3055–3070, https://doi.org/10.5194/gmd-12-3055-2019, 2019.
Lange, S.: ISIMIP3BASD, Zenodo [data set], https://doi.org/10.5281/zenodo.4686991, 2021.
Lange, S. and Büchner, M.: ISIMIP3 land-sea masks, https://doi.org/10.48364/ISIMIP.822294, 2020.
Lange, S., Menz, C., Gleixner, S., Cucchi, M., Weedon, G. P., Amici, A., Bellouin, N., Schmied, H. M., Hersbach, H., Buontempo, C., and Cagnazzo, C.: WFDE5 over land merged with ERA5 over the ocean (W5E5 v2.0), https://doi.org/10.48364/ISIMIP.342217, 2021.
Large, W. G. and Yeager, S. G.: The global climatology of an interannually varying air–sea flux data set, Clim. Dynam., 33, 341–364, 2009.
Lawrence, D. M., Hurtt, G. C., Arneth, A., Brovkin, V., Calvin, K. V., Jones, A. D., Jones, C. D., Lawrence, P. J., de Noblet-Ducoudré, N., Pongratz, J., Seneviratne, S. I., and Shevliakova, E.: The Land Use Model Intercomparison Project (LUMIP) contribution to CMIP6: rationale and experimental design, Geosci. Model Dev., 9, 2973–2998, https://doi.org/10.5194/gmd-9-2973-2016, 2016.
Lee, M., Shevliakova, E., Stock, C. A., Malyshev, S., and Milly, P. C. D.: Prominence of the tropics in the recent rise of global nitrogen pollution, Nat. Commun., 10, 1437, https://doi.org/10.1038/s41467-019-09468-4, 2019.
Lehner, B., Reidy Liermann, C., Revenga, C., Vorosmarty, C., Fekete, B., Crouzet, P., Doll, P., Endejan, M., Frenken, K., Magome, J., Nilsson, C., Robertson, J. C., Rodel, R., and Sindorf, N.: Global Reservoir and Dam Database, Version 1 (GRanDv1): Dams, Revision 01, https://doi.org/10.7927/H4N877QK, 2011a.
Lehner, B., Liermann, C. R., Revenga, C., Vörösmarty, C., Fekete, B., Crouzet, P., Döll, P., Endejan, M., Frenken, K., Magome, J., Nilsson, C., Robertson, J. C., Rödel, R., Sindorf, N., and Wisser, D.: High-resolution mapping of the world's reservoirs and dams for sustainable river-flow management, Front. Ecol. Environ., 9, 494–502, 2011b.
Lejeune, Q., Maskell, G., Menke, I., and Pleeck, S.: Stakeholder Survey Report, https://www.isimip.org/documents/376/ISIpedia_survey_result_report_w47NU6L.pdf (last access: 21 December 2023), 2018.
Liu, X., Stock, C. A., Dunne, J. P., Lee, M., Shevliakova, E., Malyshev, S., and Milly, P. C. D.: Simulated global coastal ecosystem responses to a half-century increase in river nitrogen loads, Geophys. Res. Lett., 48, e2021GL094367, https://doi.org/10.1029/2021gl094367, 2021.
Loisel, J., Gallego-Sala, A. V., Amesbury, M. J., Magnan, G., Anshari, G., Beilman, D. W., Benavides, J. C., Blewett, J., Camill, P., Charman, D. J., Chawchai, S., Hedgpeth, A., Kleinen, T., Korhola, A., Large, D., Mansilla, C. A., Müller, J., van Bellen, S., West, J. B., Yu, Z., Bubier, J. L., Garneau, M., Moore, T., Sannel, A. B. K., Page, S., Väliranta, M., Bechtold, M., Brovkin, V., Cole, L. E. S., Chanton, J. P., Christensen, T. R., Davies, M. A., De Vleeschouwer, F., Finkelstein, S. A., Frolking, S., Gaka, M., Gandois, L., Girkin, N., Harris, L. I., Heinemeyer, A., Hoyt, A. M., Jones, M. C., Joos, F., Juutinen, S., Kaiser, K., Lacourse, T., Lamentowicz, M., Larmola, T., Leifeld, J., Lohila, A., Milner, A. M., Minkkinen, K., Moss, P., Naafs, B. D. A., Nichols, J., O'Donnell, J., Payne, R., Philben, M., Piilo, S., Quillet, A., Ratnayake, A. S., Roland, T. P., Sjögersten, S., Sonnentag, O., Swindles, G. T., Swinnen, W., Talbot, J., Treat, C., Valach, A. C., and Wu, J.: Expert assessment of future vulnerability of the global peatland carbon sink, Nat. Clim. Chang., 11, 70–77, 2020.
Lu, P., Lin, N., Emanuel, K., Chavas, D., and Smith, J.: Assessing Hurricane Rainfall Mechanisms Using a Physics-Based Model: Hurricanes Isabel (2003) and Irene (2011), J. Atmos. Sci., 75, 2337–2358, 2018.
Malle, J.: w5e5_downscale, Github [code], https://github.com/johanna-malle/w5e5_downscale (last access: 21 December 2023), 2023.
Marsooli, R., Lin, N., Emanuel, K., and Feng, K.: Climate change exacerbates hurricane flood hazards along US Atlantic and Gulf Coasts in spatially varying patterns, Nat. Commun., 10, 3785, https://doi.org/10.1038/s41467-019-11755-z, 2019.
Matthews, J. B. R., Möller, V., van Diemen, R., Fuglestvedt, J. S., Masson-Delmotte, V., Méndez, C., Semenov, S., and Reisinger, A.: Annex VII: Glossary, in: Climate Change 2021: The Physical Science Basis. Contribution of Working Group I to the Sixth Assessment Report of the Intergovernmental Panel on Climate Change, edited by: Masson-Delmotte, V., Zhai, P., Pirani, A., Connors, S. L., Péan, C., Berger, S., Caud, N., Chen, Y., Goldfarb, L., Gomis, M. I., Huang, M., Leitzell, K., Lonnoy, E., Matthews, J. B. R., Maycock, T. K., Waterfield, T., Yelekçi, O., Yu, R., and Zhou, B., Cambridge, United Kingdom and New York, NY, USA, 2215–2256, 2021.
Mechler, R., Bouwer, L. M., Schinko, T., Surminski, S., and Linnerooth-Bayer, J.: Loss and damage from climate change: Concepts, methods and policy options, 1st edn., edited by: Mechler, R., Bouwer, L. M., Schinko, T., Surminski, S., and Linnerooth-Bayer, J., Springer International Publishing, Cham, Switzerland, 557 pp., 2018.
Meinshausen, M., Smith, S. J., Calvin, K., Daniel, J. S., Kainuma, M. L. T., Lamarque, J.-F., Matsumoto, K., Montzka, S. A., Raper, S. C. B., Riahi, K., Thomson, A., Velders, G. J. M., and van Vuuren, D. P. P.: The RCP greenhouse gas concentrations and their extensions from 1765 to 2300, Clim. Change, 109, 213, https://doi.org/10.1007/s10584-011-0156-z, 2011.
Meinshausen, M., Vogel, E., Nauels, A., Lorbacher, K., Meinshausen, N., Etheridge, D. M., Fraser, P. J., Montzka, S. A., Rayner, P. J., Trudinger, C. M., Krummel, P. B., Beyerle, U., Canadell, J. G., Daniel, J. S., Enting, I. G., Law, R. M., Lunder, C. R., O'Doherty, S., Prinn, R. G., Reimann, S., Rubino, M., Velders, G. J. M., Vollmer, M. K., Wang, R. H. J., and Weiss, R.: Historical greenhouse gas concentrations for climate modelling (CMIP6), Geosci. Model Dev., 10, 2057–2116, https://doi.org/10.5194/gmd-10-2057-2017, 2017.
Mengel, M., Treu, S., Lange, S., and Frieler, K.: ATTRICI v1.1 – counterfactual climate for impact attribution, Geosci. Model Dev., 14, 5269–5284, https://doi.org/10.5194/gmd-14-5269-2021, 2021.
Messager, M. L., Lehner, B., Grill, G., Nedeva, I., and Schmitt, O.: Estimating the volume and age of water stored in global lakes using a geo-statistical approach, Nat. Commun., 7, 13603, https://doi.org/10.1038/ncomms13603, 2016.
Monfreda, C., Ramankutty, N., and Foley, J. A.: Farming the planet: 2. Geographic distribution of crop areas, yields, physiological types, and net primary production in the year 2000, Global Biogeochem. Cycles, 22, GB1022, https://doi.org/10.1029/2007gb002947, 2008.
Muis, S., Apecechea, M. I., Dullaart, J., de Lima Rego, J., Madsen, K. S., Su, J., Yan, K., and Verlaan, M.: A high-resolution global dataset of extreme sea levels, tides, and storm surges, including future projections, Front. Mar. Sci., 7, 263, https://doi.org/10.3389/fmars.2020.00263, 2020.
Müller Schmied, H.: DDM30 river routing network for ISIMIP3, https://doi.org/10.48364/ISIMIP.865475, 2022.
Nicholls, R. J., Lincke, D., Hinkel, J., Brown, S., Vafeidis, A. T., Meyssignac, B., Hanson, S. E., Merkens, J.-L., and Fang, J.: A Global Analysis of Subsidence, Relative Sea-Level Change and Coastal Flood Exposure, Nat. Clim. Change, 11, 338–3342, https://doi.org/10.1038/s41558-021-00993-z, 2021.
O'Neill, B., van Aalst, M., Z., Z. I., Berrang Ford, L., Bhadwal, S., Buhaug, H., Diaz, D., Frieler, K., Garschagen, M., Magnan, A., Midgley, G., Mirzabaev, A., Thomas, A., and Warren, R.: Climate Change 2022: Impacts, Adaptation and Vulnerability. Key Risks Across Sectors and Regions, in: Contribution of Working Group II to the Sixth Assessment Report of the Intergovernmental Panel on Climate Change, edited by: Pörtner, H.-O., Roberts, D. C., Tignor, M., Poloczanska, E. S., Mintenbeck, K., Alegría, A., Craig, M., Langsdorf, S., Löschke, S., Möller, V., Okem, A., and Rama, B., Cambridge University Press, Cambridge, UK and New York, NY, USA, 2411–2538, 2022.
Outcomes of the ISIMIP Strategy Group Meeting: https://www.isimip.org/news/outcome-isimip-strategy-group-meeting-2018/, last access: 14 January 2023.
Patricola, C. M. and Wehner, M. F.: Anthropogenic influences on major tropical cyclone events, Nature, 563, 339–346, 2018.
Pauly, D., Zeller, D., and Palomares, M. L. D. (Eds.): Sea Around Us Concepts, Design and Data, http://www.seaaroundus.org/, last access: 22 December 2023), 2020.
Peduzzi, P., Chatenoux, B., Dao, H., De Bono, A., Herold, C., Kossin, J., Mouton, F., and Nordbeck, O.: Global trends in tropical cyclone risk, Nat. Clim. Chang., 2, 289–294, 2012.
Portmann, F. T., Siebert, S., and Döll, P.: MIRCA2000-Global monthly irrigated and rainfed crop areas around the year 2000: A new high-resolution data set for agricultural and hydrological modeling, Global Biogeochem. Cycles, 24, GB1011, https://doi.org/10.1029/2008gb003435, 2010.
Protocol – TRENDY: https://blogs.exeter.ac.uk/trendy/protocol/, last access: 18 October 2023.
Reyer, C., Silveyra Gonzalez, R., Dolos, K., Hartig, F., Hauf, Y., Noack, M., Lasch-Born, P., Rötzer, T., Pretzsch, H., Meesenburg, H., Fleck, S., Wagner, M., Bolte, A., Sanders, T., Kolari, P., Mäkelä, A., Vesala, T., Mammarella, I., Pumpanen, J., Matteucci, G., Collalti, A., D'Andrea, E., Foltýnová, L., Krejza, J., Ibrom, A., Pilegaard, K., Loustau, D., Bonnefond, J.-M., Berbigier, P., Picart, D., Lafont, S., Dietze, M., Cameron, D., Vieno, M., Tian, H., Palacios-Orueta, A., Cicuendez, V., Recuero, L., Wiese, K., Büchner, M., Lange, S., Volkholz, J., Kim, H., Weedon, G., Sheffield, J., Vega del Valle, I., Suckow, F., Horemans, J., Martel, S., Bohn, F., Steinkamp, J., Chikalanov, A., Mahnken, M., Gutsch, M., Trotta, C., Babst, F., and Frieler, K.: The PROFOUND database for evaluating vegetation models and simulating climate impacts on European forests. V. 0.3. GFZ Data Services, https://doi.org/10.5880/PIK.2020.006, 2020a.
Reyer, C. P. O., Silveyra Gonzalez, R., Dolos, K., Hartig, F., Hauf, Y., Noack, M., Lasch-Born, P., Rötzer, T., Pretzsch, H., Meesenburg, H., Fleck, S., Wagner, M., Bolte, A., Sanders, T. G. M., Kolari, P., Mäkelä, A., Vesala, T., Mammarella, I., Pumpanen, J., Collalti, A., Trotta, C., Matteucci, G., D'Andrea, E., Foltýnová, L., Krejza, J., Ibrom, A., Pilegaard, K., Loustau, D., Bonnefond, J.-M., Berbigier, P., Picart, D., Lafont, S., Dietze, M., Cameron, D., Vieno, M., Tian, H., Palacios-Orueta, A., Cicuendez, V., Recuero, L., Wiese, K., Büchner, M., Lange, S., Volkholz, J., Kim, H., Horemans, J. A., Bohn, F., Steinkamp, J., Chikalanov, A., Weedon, G. P., Sheffield, J., Babst, F., Vega del Valle, I., Suckow, F., Martel, S., Mahnken, M., Gutsch, M., and Frieler, K.: The PROFOUND Database for evaluating vegetation models and simulating climate impacts on European forests, Earth Syst. Sci. Data, 12, 1295–1320, https://doi.org/10.5194/essd-12-1295-2020, 2020b.
Reyer, C. P. O., Schelhaas, M.-J., Mäkelä, A., Peltoniemi, M., Gutsch, M., Mahnken, M., Loustau, D., Martel, S., Merganiè, J., Merganièová, K., Meesenburg, H., Rötzer, T., Heym, M., Collalti, A., D'Andrea, E., Matteucci, G., Ibrom, A., and Kvist Johannsen, V.: Current Site-specific management guidelines and schedules for the 9 PROFOUND forest sites of the regional forest sector in ISIMIP, Zenodo [data set], https://doi.org/10.5281/zenodo.7622027, 2023.
Risser, M. D. and Wehner, M. F.: Attributable human-induced changes in the likelihood and magnitude of the observed extreme precipitation during hurricane Harvey, Geophys. Res. Lett., 44, 12457–12464, 2017.
Rousseau, Y., Blanchard, J., Novaglio, C., Kirsty, P., Tittensor, D., Watson, R., and Ye, Y.: Global Fishing Effort, Institute for Marine and Antarctic Studies (IMAS), University of Tasmania (UTAS) [data set], https://doi.org/10.25959/MNGY-0Q43, 2022.
Rousseau, Y., Blanchard, J., Novaglio, C., Pinnell, K., Tittensor, D., Watson, R., and Ye, Y.: A data base of mapped global fishing activity, 1950–2017, Sci. Data, in press, 2024.
Rust, H. W., Kruschke, T., Dobler, A., Fischer, M., and Ulbrich, U.: Discontinuous Daily Temperatures in the WATCH Forcing Datasets, J. Hydrometeorol., 16, 465–472, 2015.
Sarmiento, J. L. and Gruber, N.: Ocean Biogeochemical Dynamics, Geol. Mag., 144, 1034–1034, 2006.
Seitzinger, S., Harrison, J. A., Böhlke, J. K., Bouwman, A. F., Lowrance, R., Peterson, B., Tobias, C., and Van Drecht, G.: Denitrification across landscapes and waterscapes: a synthesis, Ecol. Appl., 16, 2064–2090, 2006.
Sherman, K.: Large Marine Ecosystems, in: Encyclopedia of Ocean Sciences, Elsevier, 709–723, 2017.
Sitch, S., Friedlingstein, P., Gruber, N., Jones, S. D., Murray-Tortarolo, G., Ahlström, A., Doney, S. C., Graven, H., Heinze, C., Huntingford, C., Levis, S., Levy, P. E., Lomas, M., Poulter, B., Viovy, N., Zaehle, S., Zeng, N., Arneth, A., Bonan, G., Bopp, L., Canadell, J. G., Chevallier, F., Ciais, P., Ellis, R., Gloor, M., Peylin, P., Piao, S. L., Le Quéré, C., Smith, B., Zhu, Z., and Myneni, R.: Recent trends and drivers of regional sources and sinks of carbon dioxide, Biogeosciences, 12, 653–679, https://doi.org/10.5194/bg-12-653-2015, 2015.
Slangen, A. B. A., Church, J. A., Agosta, C., Fettweis, X., Marzeion, B., and Richter, K.: Anthropogenic Forcing Dominates Global Mean Sea-Level Rise since 1970, Nat. Clim. Change, 6, 701–705, https://doi.org/10.1038/nclimate2991, 2016.
Slivinski, L. C., Compo, G. P., Whitaker, J. S., Sardeshmukh, P. D., Giese, B. S., McColl, C., Allan, R., Yin, X., Vose, R., Titchner, H., Kennedy, J., Spencer, L. J., Ashcroft, L., Brönnimann, S., Brunet, M., Camuffo, D., Cornes, R., Cram, T. A., Crouthamel, R., Domínguez-Castro, F., Freeman, J. E., Gergis, J., Hawkins, E., Jones, P. D., Jourdain, S., Kaplan, A., Kubota, H., Blancq, F. L., Lee, T.-C., Lorrey, A., Luterbacher, J., Maugeri, M., Mock, C. J., Moore, G. W. K., Przybylak, R., Pudmenzky, C., Reason, C., Slonosky, V. C., Smith, C. A., Tinz, B., Trewin, B., Valente, M. A., Wang, X. L., Wilkinson, C., Wood, K., and Wyszyñski, P.: Towards a more reliable historical reanalysis: Improvements for version 3 of the Twentieth Century Reanalysis system, Q. J. Roy. Meteor. Soc., 145, 2876–2908, 2019.
Slivinski, L. C., Compo, G. P., Sardeshmukh, P. D., Whitaker, J. S., McColl, C., Allan, R. J., Brohan, P., Yin, X., Smith, C. A., Spencer, L. J., Vose, R. S., Rohrer, M., Conroy, R. P., Schuster, D. C., Kennedy, J. J., Ashcroft, L., Brönnimann, S., Brunet, M., Camuffo, D., Cornes, R., Cram, T. A., Domínguez-Castro, F., Freeman, J. E., Gergis, J., Hawkins, E., Jones, P. D., Kubota, H., Lee, T. C., Lorrey, A. M., Luterbacher, J., Mock, C. J., Przybylak, R. K., Pudmenzky, C., Slonosky, V. C., Tinz, B., Trewin, B., Wang, X. L., Wilkinson, C., Wood, K., and Wyszyñski, P.: An Evaluation of the Performance of the Twentieth Century Reanalysis Version 3, J. Climate, 34, 1417–1438, 2021.
Smil, V.: Enriching the Earth: Fritz Haber, Carl Bosch and the Transformation of World Food Production, MIT Press, https://doi.org/10.7551/mitpress/2767.001.0001, 2001.
Stock, C. A., Dunne, J. P., and John, J. G.: Global-scale carbon and energy flows through the marine planktonic food web: An analysis with a coupled physical–biological model, Prog. Oceanogr., 120, 1–28, 2014.
Tian, H., Yang, J., Lu, C., Xu, R., Canadell, J. G., Jackson, R. B., Arneth, A., Chang, J., Chen, G., Ciais, P., Gerber, S., Ito, A., Huang, Y., Joos, F., Lienert, S., Messina, P., Olin, S., Pan, S., Peng, C., Saikawa, E., Thompson, R. L., Vuichard, N., Winiwarter, W., Zaehle, S., Zhang, B., Zhang, K., and Zhu, Q.: The Global N2O Model Intercomparison Project, B. Am. Meteorol. Soc., 99, 1231–1251, 2018.
Tittensor, D. P., Eddy, T. D., Lotze, H. K., Galbraith, E. D., Cheung, W., Barange, M., Blanchard, J. L., Bopp, L., Bryndum-Buchholz, A., Büchner, M., Bulman, C., Carozza, D. A., Christensen, V., Coll, M., Dunne, J. P., Fernandes, J. A., Fulton, E. A., Hobday, A. J., Huber, V., Jennings, S., Jones, M., Lehodey, P., Link, J. S., Mackinson, S., Maury, O., Niiranen, S., Oliveros-Ramos, R., Roy, T., Schewe, J., Shin, Y.-J., Silva, T., Stock, C. A., Steenbeek, J., Underwood, P. J., Volkholz, J., Watson, J. R., and Walker, N. D.: A protocol for the intercomparison of marine fishery and ecosystem models: Fish-MIP v1.0, Geosci. Model Dev., 11, 1421–1442, https://doi.org/10.5194/gmd-11-1421-2018, 2018.
Tractebel: Filling of the reservoir of the Grand Renaissance Dam, https://tractebel-engie.com/en/news/2020/ethiopia-first-stage-of-the-filling-of-the-reservoir-of-the-grand-renaissance-dam (last access: 10 February 2023), September 2020.
Treu, S., Muis, S., Dangendorf, S., Wahl, T., Oelsmann, J., Heinicke, S., Frieler, K., and Mengel, M.: Reconstruction of hourly coastal water levels and counterfactuals without sea level rise for impact attribution, Earth Syst. Sci. Data Discuss. [preprint], https://doi.org/10.5194/essd-2023-112, in review, 2023.
Tsujino, H., Urakawa, S., Nakano, H., Small, R. J., Kim, W. M., Yeager, S. G., Danabasoglu, G., Suzuki, T., Bamber, J. L., Bentsen, M., Böning, C. W., Bozec, A., Chassignet, E. P., Curchitser, E., Boeira Dias, F., Durack, P. J., Griffies, S. M., Harada, Y., Ilicak, M., Josey, S. A., Kobayashi, C., Kobayashi, S., Komuro, Y., Large, W. G., Le Sommer, J., Marsland, S. J., Masina, S., Scheinert, M., Tomita, H., Valdivieso, M., and Yamazaki, D.: JRA-55 based surface dataset for driving ocean–sea-ice models (JRA55-do), Ocean Model., 130, 79–139, 2018.
UN 2019 World Population Prospects (WPP) Database 2019: https://population.un.org/wpp/, last access: 15 February 2023.
United Nations, Department of Economic and Social Affairs: Population Division (2019), World Population Prospects 2019, archive [data set], https://population.un.org/wpp/Download/Archive/Standard/ (last access: 22 December 2023), 2019.
van den Hurk, B., Kim, H., Krinner, G., Seneviratne, S. I., Derksen, C., Oki, T., Douville, H., Colin, J., Ducharne, A., Cheruy, F., Viovy, N., Puma, M. J., Wada, Y., Li, W., Jia, B., Alessandri, A., Lawrence, D. M., Weedon, G. P., Ellis, R., Hagemann, S., Mao, J., Flanner, M. G., Zampieri, M., Materia, S., Law, R. M., and Sheffield, J.: LS3MIP (v1.0) contribution to CMIP6: the Land Surface, Snow and Soil moisture Model Intercomparison Project – aims, setup and expected outcome, Geosci. Model Dev., 9, 2809–2832, https://doi.org/10.5194/gmd-9-2809-2016, 2016.
van Oldenborgh, G. J., van der Wiel, K., Sebastian, A., Singh, R., Arrighi, J., Otto, F., Haustein, K., Li, S., Vecchi, G., and Cullen, H.: Attribution of extreme rainfall from Hurricane Harvey, August 2017, Environ. Res. Lett., 12, 124009, https://doi.org/10.1088/1748-9326/aa9ef2, 2017.
Wada, Y., Flörke, M., Hanasaki, N., Eisner, S., Fischer, G., Tramberend, S., Satoh, Y., van Vliet, M. T. H., Yillia, P., Ringler, C., Burek, P., and Wiberg, D.: Modeling global water use for the 21st century: the Water Futures and Solutions (WFaS) initiative and its approaches, Geosci. Model Dev., 9, 175–222, https://doi.org/10.5194/gmd-9-175-2016, 2016a.
Wada, Y., Lo, M.-H., Yeh, P. J.-F., Reager, J. T., Famiglietti, J. S., Wu, R.-J., and Tseng, Y.-H.: Fate of water pumped from underground and contributions to sea-level rise, Nat. Clim. Chang., 6, 777–780, 2016b.
Wang, J., Walter, B. A., Yao, F., Song, C., Ding, M., Maroof, A. S., Zhu, J., Fan, C., McAlister, J. M., Sikder, S., Sheng, Y., Allen, G. H., Crétaux, J.-F., and Wada, Y.: GeoDAR: georeferenced global dams and reservoirs dataset for bridging attributes and geolocations, Earth Syst. Sci. Data, 14, 1869–1899, https://doi.org/10.5194/essd-14-1869-2022, 2022.
Wang, S.-Y. S., Zhao, L., Yoon, J.-H., Klotzbach, P., and Gillies, R. R.: Quantitative attribution of climate effects on Hurricane Harvey's extreme rainfall in Texas, Environ. Res. Lett., 13, 054014, https://doi.org/10.1088/1748-9326/aabb85, 2018.
Wang, T. and Sun, F.: Global gridded GDP data set consistent with the shared socioeconomic pathways, Sci. Data, 9, 221, https://doi.org/10.1038/s41597-022-01300-x, 2022.
Watson, R.: Global Fisheries Landings V4.0, https://doi.org/10.25959/5C522CADBEA37, 2019.
Watson, R. A. and Tidd, A.: Mapping nearly a century and a half of global marine fishing: 1869–2015, Mar. Policy, 93, 171–177, 2018.
Whitehouse, P. L.: Glacial isostatic adjustment modelling: historical perspectives, recent advances, and future directions, Earth Surf. Dynam., 6, 401–429, https://doi.org/10.5194/esurf-6-401-2018, 2018.
WOCE Atlas: http://woceatlas.ucsd.edu, last access: 11 January 2023.
Wöppelmann, G. and Marcos, M.: Vertical land motion as a key to understanding sea level change and variability, Rev. Geophys., 54, 64–92, 2016.
Wyns, A.: COP27 establishes loss and damage fund to respond to human cost of climate change, Lancet Planet Health, 7, e21–e22, 2023.
Xi, D., Lin, N., and Smith, J.: Evaluation of a Physics-Based Tropical Cyclone Rainfall Model for Risk Assessment, J. Hydrometeorol., 21, 2197–2218, 2020.
Yang, J. and Tian, H.: ISIMIP3a N-deposition input data, https://doi.org/10.48364/ISIMIP.759077.1, 2020.
Yang, J., Yan, F., and Chen, M.: Effects of sea level rise on storm surges in the south Yellow Sea: A case study of Typhoon Muifa (2011), Cont. Shelf Res., 215, 104346, https://doi.org/10.1016/j.csr.2021.104346, 2021.
Zhang, X., Davidson, E. A., Mauzerall, D. L., Searchinger, T. D., Dumas, P., and Shen, Y.: Managing nitrogen for sustainable development, Nature, 528, 51–59, 2015.
Zhao, N., Liu, Y., Cao, G., Samson, E. L., and Zhang, J.: Forecasting China's GDP at the pixel level using nighttime lights time series and population images, GISci. Remote Sens., 54, 407–425, 2017.
Zhu, L., Quiring, S. M., and Emanuel, K. A.: Estimating tropical cyclone precipitation risk in Texas, Geophys. Res. Lett., 40, 6225–6230, 2013.
This was announced by email via the ISIMIP mailing list on 21 February 2020.