the Creative Commons Attribution 4.0 License.
the Creative Commons Attribution 4.0 License.
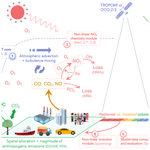
A simplified non-linear chemistry transport model for analyzing NO2 column observations: STILT–NOx
Joshua L. Laughner
Junjie Liu
Paul I. Palmer
John C. Lin
Paul O. Wennberg
Satellites monitoring air pollutants (e.g., nitrogen oxides; NOx = NO + NO2) or greenhouse gases (GHGs) are widely utilized to understand the spatiotemporal variability in and evolution of emission characteristics, chemical transformations, and atmospheric transport over anthropogenic hotspots. Recently, the joint use of space-based long-lived GHGs (e.g., carbon dioxide; CO2) and short-lived pollutants has made it possible to improve our understanding of emission characteristics. Some previous studies, however, lack consideration of the non-linear NOx chemistry or complex atmospheric transport. Considering the increase in satellite data volume and the demand for emission monitoring at higher spatiotemporal scales, it is crucial to construct a local-scale emission optimization system that can handle both long-lived GHGs and short-lived pollutants in a coupled and effective manner. This need motivates us to develop a Lagrangian chemical transport model that accounts for NOx chemistry and fine-scale atmospheric transport (STILT–NOx) and to investigate how physical and chemical processes, anthropogenic emissions, and background may affect the interpretation of tropospheric NO2 columns (tNO2).
Interpreting emission signals from tNO2 commonly involves either an efficient statistical model or a sophisticated chemical transport model. To balance computational expenses and chemical complexity, we describe a simplified representation of the NOx chemistry that bypasses an explicit solution of individual chemical reactions while preserving the essential non-linearity that links NOx emissions to its concentrations. This NOx chemical parameterization is then incorporated into an existing Lagrangian modeling framework that is widely applied in the GHG community. We further quantify uncertainties associated with the wind field and chemical parameterization and evaluate modeled columns against retrieved columns from the TROPOspheric Monitoring Instrument (TROPOMI v2.1). Specifically, simulations with alternative model configurations of emissions, meteorology, chemistry, and inter-parcel mixing are carried out over three United States (US) power plants and two urban areas across seasons. Using the U.S. Environmental Protection Agency (EPA)-reported emissions for power plants with non-linear NOx chemistry improves the model–data alignment in tNO2 (a high bias of ≤ 10 % on an annual basis), compared to simulations using either the Emissions Database for Global Atmospheric Research (EDGAR) model or without chemistry (bias approaching 100 %). The largest model–data mismatches are associated with substantial biases in wind directions or conditions of slower atmospheric mixing and photochemistry. More importantly, our model development illustrates (1) how NOx chemistry affects the relationship between NOx and CO2 in terms of the spatial and seasonal variability and (2) how assimilating tNO2 can quantify systematic biases in modeled wind directions and emission distribution in prior inventories of NOx and CO2, which laid a foundation for a local-scale multi-tracer emission optimization system.
- Article
(9432 KB) - Full-text XML
-
Supplement
(51997 KB) - BibTeX
- EndNote
Emissions of air pollutants (APs) and greenhouse gases (GHGs) adversely impact urban ecosystems and environments, human health, and the climate via the moderation of energy budgets (Myhre et al., 2013; Watts et al., 2021). APs and GHGs are directly inter-connected, considering that they are co-emitted from many combustion sources, suggesting that reductions in GHGs may bring co-benefits in mitigating APs (Cifuentes et al., 2001; West et al., 2013; Lin et al., 2018). Although quantifying emissions in GHGs and APs and understanding their underlying drivers at all scales are equally important, emission estimates beyond a county or city become more relevant in addressing policy-relevant topics such as emission mitigation.
Space-based remote sensors offer an objective perspective to monitoring global air quality and GHGs. These new data enable us to uncover the spatial variability along with the temporal trend and perturbation of anthropogenic emissions. Air-quality-related observations have been among the first to demonstrate the capability of satellite remote sensing to globally diagnose air quality (Duncan et al., 2016; Laughner and Cohen, 2019; Jin et al., 2020); constrain emissions across time, space, and sectors (Jiang et al., 2018; Goldberg et al., 2019; Tang et al., 2019; Qu et al., 2022); and evaluate real-world decisions (Lamsal et al., 2011; Demetillo et al., 2020). Leveraging satellite observations in understanding the spatiotemporal distribution of emissions within cities is still limited compared to those city total estimates. Data and analysis uncertainty further present the main challenge in extracting robust combustion signals from remotely sensed measurements, and these uncertainties are amplified in attempt to resolve dynamic flows and heterogeneous combustion activities within cities (Valin et al., 2013; Goldberg et al., 2022; Souri et al., 2022).
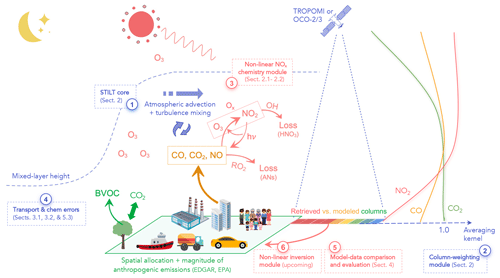
Figure 1A conceptual diagram of our proposed local-scale multi-tracer modeling framework in interpreting column observations. It contains a road map for this study (points 1 through 5). The diagram highlights the key biogenic, physical, and/or chemical processes for quantifying NOx, CO, and CO2 around cities, based on space-based measurements (pixels from red to blue). These include details on the atmospheric conditions (wind speed and planetary boundary layer height for vertical mixing, horizontal mixing, and diffusion lengths); chemical conditions (photolysis rate and NOx regimes and regional versus local oxidant conditions); the spatial distribution of emissions (urban vs. power plant); and sensitivities of the column abundance to individual vertical levels (averaging kernel).
Making full use of the existing and upcoming satellites that retrieve concentrations of APs and GHGs offers an informative way to target urban emissions from different sources at a policy-relevant scale of a few kilometers. Combining satellite observations of species with different atmospheric lifetimes has enabled studies to diagnose chemical conditions and meteorological processes (Jin et al., 2017; Lama et al., 2022), identify urban plumes, constrain emissions for the tracer of interest (Wunch et al., 2009; Yang et al., 2023), and obtain observation-based ratios between tracers (Silva and Arellano, 2017; Wu et al., 2022; MacDonald et al., 2022) to infer structural changes in combustion activities (Reuter et al., 2014; Miyazaki and Bowman, 2023). In light of the rapid rise in satellite data volume, it is beneficial to have an analysis system that adequately accounts for the important local-scale processes in interpreting the abundance of GHGs and APs in a coupled manner (Fig. 1). Analogies to such local-scale systems in a global context include the AP-focused Tropospheric Chemistry Reanalysis version 2 (TCR-2; Miyazaki et al., 2020) and the GHG-focused Carbon Monitoring System Flux (CMS-Flux; Hurtt et al., 2022). Only a few recent multi-tracer modeling systems aim to bridge CO2 and NO2 column measurements (Reuter et al., 2014; Kaminski et al., 2022; Hakkarainen et al., 2023), despite limits in their modeling tools (elaborated upon in the next paragraph). In addition, as emphasized in Reuter et al. (2014), most multi-tracer studies rely on emission ratio or conversion ratio from inventories, which can be problematic.
In efforts to interpret CO2 or NOx emission signatures from satellite observations, most prior studies used either statistical or inversion approaches. The former approach involves the use of a Gaussian plume or exponentially modified Gaussian distribution (EMG) models with input from simple wind information to derive emissions of CO2 and NOx (or a lifetime if for NOx) purely from observations in a computationally efficient manner without relying much on prior assumptions of emissions (Nassar et al., 2022; Beirle et al., 2011). These statistical approaches only provide a plume-integrated emission estimate that can be sensitive to the input wind speed and chemical lifetime. Multiple satellite overpasses need to be aggregated with wind direction aligned for a robust fit in the EMG model to obtain the emission and lifetimes. It is challenging to infer and evaluate sub-grid-cell variations in emissions. The more sophisticated inverse approach involves the use of a chemical transport model (CTM) that comprehensively accounts for atmospheric transport and chemical transformation and a coupled inversion or data assimilation system (e.g., Liu et al., 2022; Qu et al., 2022). CTMs are, however, computationally expensive and often involve hundreds of species and their coupling reactions. Most CTMs used in AP-related studies are Eulerian models, which may suffer from complications caused by rigid model grids (Wohltmann and Rex, 2009; Valin et al., 2011). Motivated by these approaches that rely on a constant lifetime or solve for individual chemical reactions, we have built a modeling framework to balance the advantages and imperfections – i.e., to simplify the chemical transformation process that preserves the non-linear relationship between NOx emissions and the observed concentration field, together with a high-resolution atmospheric transport using a Lagrangian particle dispersion model (LPDM).
LPDMs have been increasingly utilized for emission estimates over the past few decades. For instance, the Stochastic Time-Inverted Lagrangian Transport model (STILT; Lin et al., 2003) building upon HYSPLIT (Stein et al., 2015) has been well adapted to analyze emission signals from all sorts of measurement platforms. STILT was designed to better describe the movement of air parcels only relevant to an observation site and explicitly provide the source–receptor relationship (i.e., the Jacobian matrix) to facilitate efficient atmospheric inversions for optimizing emissions. Besides, LPDMs themselves possess inherent numerical and computational advantages, such as avoiding artificial smoothing of concentration fields by spurious numerical diffusion in confined model boxes (Wohltmann and Rex, 2009; Lin et al., 2013). More importantly, the Lagrangian transport perspective is intuitively coupled with box models that handle chemical reactions. Noticeable examples include STOCHEM (Collins et al., 1997), ATLAS (Wohltmann and Rex, 2009), CLaMS v2.0 (Konopka et al., 2019), and HYSPLIT-based variations, including HYSPLIT CheM (Stein et al., 2000), ELMO-2 for ozone (Strong et al., 2010), and STILT-Chem (Wen et al., 2012). These Lagrangian chemical models describe the chemical reactions of each species or lumped group with similar functional groups to calculate chemical transformation along trajectories but vary in the complexity of implemented chemistry and parameterization for turbulent mixing and numerical diffusion. Despite these prior modeling efforts, Lagrangian chemical models are more often adopted to inform the origins of APs but are less commonly used to constrain emissions. Such under-appreciation is in part a result of the heavy computational expenses in solving chemical changes at a high frequency via ordinary differential equations (similar to most Eulerian CTMs) and the reliance on external meteorological fields.
To reduce computational costs in dealing with complex chemistry, studies have proposed machine learning techniques or defaulted to a constant-lifetime assumption as a shortcut. Machine learning techniques have been applied to approximate the chemical mechanisms (Keller and Evans, 2019; Huang and Seinfeld, 2022), predict the OH field with observational constraint (Zhu et al., 2022), and calculate emissions (He et al., 2022). Other studies have assumed a constant first-order lifetime to estimate NOx emissions and emission ratios between NOx and CO2 (Lee et al., 2014; Hakkarainen et al., 2023). However, unlike chemically passive species such as CO2, the chemical tendency of NOx is not independent of atmospheric advection and turbulent mixing because of the chemically driven non-linearity between the NOx lifetime and the NO and NO2 concentrations (Laughner and Cohen, 2019). More specifically, during the day, NOx is lost through two more permanent pathways of (1) NO2 + OH to nitric acid and (2) NO + peroxy radicals (RO2), with a minor branch in producing alkyl nitrates or ANs (point 3 in Fig. 1). The two pathways compete with one another and either may dominate, depending on the chemical conditions. Such non-linear dependence of the NOx lifetime or chemical tendency with the NOx concentration must be accounted for to estimate the NOx emissions from atmospheric NO2 concentrations. Such non-linearity will affect the interpretation of tracer-to-tracer emission ratios from observed enhancement ratios.
In this study, we present a non-linear modeling framework, STILT–NOx, to simulate tropospheric column-averaged NO2 mixing ratio (tNO2) as retrieved from the TROPOspheric Monitoring Instrument (TROPOMI). Note that initial NO2 vertical column density (VCD; ) is converted to tNO2 (ppb) by dividing by a dry air VCD. The dry air VCD is calculated by integrating a profile of the ideal gas number density of air minus a modeled water vapor profile. As illustrated in Fig. 1, the overarching goal of this framework is to facilitate emission optimizations over global anthropogenic hotspots by simulations of the concentrations of key trace gases of CO2, CO, and NOx at the local scale. To do so, the current work aims to equip the STILT model with simplified chemistry that avoids explicit calculations of chemical reactions, while preserving the non-linearity that ties the NOx concentrations to its emission (point 3 in Fig. 1). The proposed STILT–NOx framework is comprised of four components, outlined below, which correspond, respectively, to points 1 to 4 in Fig. 1 and will be coupled to an upcoming non-linear flux inversion module (point 6).
-
The HYSPLIT–STILT core resolves fine-scale atmospheric advection and turbulence and calculates the sensitivity of concentration anomalies to upwind fluxes (footprint) (Lin et al., 2003; Fasoli et al., 2018; Loughner et al., 2021), with an additional simplified inter-parcel mixing scheme (Sect. 2.3).
-
A column-weighting module to simulate atmospheric columns (and uncertainties) that incorporates pressure weighting functions and retrieval-specific averaging kernel profiles (X-STILT; Wu et al., 2018).
-
A simplified chemistry module that describes the NOx chemical tendency (Sect. 2.1) and how much NOx is presented as NO2 (i.e., NO2 : NOx ratio; Sect. 2.2).
-
An error analysis module that quantifies errors and biases in wind fields and chemical parameters (Sect. 3), following the methods initially proposed in Lin and Gerbig (2005) and Wu et al. (2018), which can be used for future flux inversions.
We illustrate the skill of this framework using comparisons of modeled tNO2 and those diagnosed from TROPOMI over three United States (US) power plants and two cities across seasons (Sect. 4). Last, we discuss possible future advances in Sect. 5.3 and demonstrate the benefits of applying this framework, especially on the quantification of CO2 emissions, emission ratios between NOx and CO2, and near-field wind biases in Sects. 5.1 and 5.2.
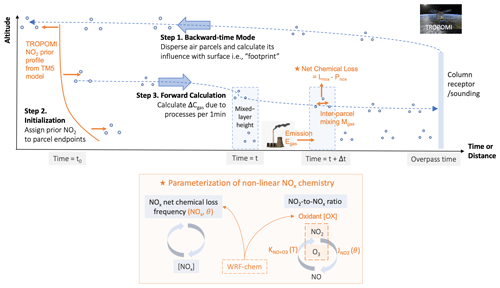
Figure 2A schematic of STILT–NOx for simulating concentrations in three steps. Step 1 is a routine backward-time calculation that records the locations of air parcels at each timestamp (Δt) of 1 min or shorter and their influence from potential fluxes (footprint). Step 2 is to calculate the initial condition for which the trajectory endpoint at time t0 is given a concentration from 4D fields (e.g., TM5 in the case of NOx). Step 3 is a forward-time concentration calculation that updates change in concentrations due to emissions, net chemical losses, and inter-parcel mixing along each trajectory at a timescale of ≤ 1 min. To clarify, Step 3 makes use of trajectories originating from a column receptor stretching from the surface to 2 km, as generated from Step 1.
Building upon the HYSPLIT–STILT atmospheric transport core, the STILT–NOx framework traces the origin of the atmospheric column observed by the satellite and calculates changes in the NOx concentrations due to emissions, inter-parcel mixing, and chemical transformations at the (sub-)minute scale. The STILT–NOx simulations are conducted in three steps (Fig. 2).
First, the backward-trajectory mode records the latitude, longitude, and pressure coordinates of air parcels originating from the same atmospheric column sampled by satellites and being driven by the Eulerian meteorological fields (Step 1 in Fig. 2). In this work, we test two meteorological fields when they are available for each examined region, namely from the Global Forecast System (GFS0p25) and the High-Resolution Rapid Refresh (HRRR), with a respective horizontal grid spacing of 0.25∘ and 3 km (Rolph et al., 2017). As most anthropogenic and all soil sources of NOx are from the surface, air parcels are evenly distributed and released from the surface to 2 km, which is slightly above the typical planetary boundary layer (PBL) height (Wu et al., 2018). To evaluate how representative enhancements between 0 and 2 km are compared to the total tropospheric column enhancements (which can include sources from lightning and aviation), we analyzed vertical distributions of NOx mixing ratios from TCR-2 (Miyazaki et al., 2020). TCR-2 is a global chemical reanalysis that includes full physical and chemical processes for various species and assimilates multiple satellite products of NO2, ozone, CO, and SO2. As a result, the monthly mean NOx concentrations over the 2∘ × 2∘ area around the top 1000 cities is quite insignificant for pressure ≤ 700 hPa when compared to huge signals within the PBL (Fig. S1 in the Supplement). Although 0 to 2 km columns include most anthropogenic enhancements over urban areas, we subtracted a local NO2 background from the total tropospheric columns to minimize the non-anthropogenic influences with a plume detection algorithm, following Kuhlmann et al. (2019). The model–data comparisons with background subtracted are discussed in Sect. 4.1.
After being released from a given TROPOMI sounding at the overpass time (∼ 13:00 local time for nadir soundings), air parcels are dispersed backward in time for 12 h (time at t0 in Fig. 2). Step 1 also provides the STILT footprint () per air parcel per timestamp (Lin et al., 2003). The STILT footprint of a given air parcel is proportional to the time this parcel spends in a small area (of ∼ 100 m) and describes how the downwind concentration may be altered if this air parcel is influenced by emissions. A much more complete description of STILT can be found in Lin et al. (2003); Fasoli et al. (2018). The footprint concept, by definition, relies on atmospheric transport and only accounts for concentration changes due to emissions but not chemical transformations.
Next, NOx concentrations at the endpoints of the model trajectory are extracted from the Tracer Model version 5, massively parallel version (TM5-MP) to serve as the initial conditions (Step 2 in Fig. 2). TM5-MP is an auxiliary dataset in which the NO2 vertical profiles serve as the prior knowledge facilitating the stratosphere–troposphere separation in Level 2 (L2) NO2 retrieval (Van Geffen et al., 2022). Here, we simply assume that most NOx is presented as NO2 at nighttime, despite the apparent caveat for neglecting NO3 chemistry and heterogenous reactions involving N2O5.
Once NOx is initialized at the time t0 for the endpoint of every trajectory, we proceed with Step 3 (Fig. 2) to estimate the changes in concentrations due to emissions, chemical transformation, and inter-particle mixing. Mathematically, the concentration per air parcel per timestamp (Cp,t) relies on that from the last timestamp, following Eq. (1):
where the time interval for updating concentrations, Δt, is defaulted to 1 min or reduced to a sub-minute value when Ct becomes nonphysically negative to ensure numerical stability. Concentration gains from emissions, ΔCemis, result from multiplying STILT-parcel-specific footprints (Fp,t) with prior emissions (E) from EDGARv6.1 (Monforti Ferrario et al., 2022) and the U.S. Environmental Protection Agency (EPA; United States Environmental Protection Agency, 2022) for power plant cases in this study. We neglect soil NOx emissions, given the relatively small contributions in cities. Unlike sophisticated CTMs which resolve the chemical reactions of an individual or lumped groups of species, concentration anomalies due to chemical reactions, ΔCchem, are solved in an explicit first-order fashion involving a net chemical tendency, with a unit of parts per billion per hour (ppb h−1). Such a chemical tendency ( in Eq. 2b) is parameterized offline as functions of NOx concentrations and solar zenith angles, θ, which is explained in Sect. 2.1. The final term, ΔCmix,p,t, denotes the concentration exchange between a given air parcel and its volumetric neighborhood (pngb), which is explained in Sect. 2.3.
Following these steps, we obtain the modeled NOx mixing ratio for every trajectory released between the surface and 2 km, based on NOx curves described in Sect. 2.1. To compare against TROPOMI tropospheric NO2 columns, we account for the fraction of NOx that is present as NO2 (Sect. 2.2) and properly weight the modeled NO2 from different altitudes, according to the pressure weighting function and averaging kernel profiles, following Wu et al. (2018). Such an approach in applying averaging kernel (Fig. 1) to the modeled profiles is equivalent to a more commonly used approach, which re-calculated the retrieved tNO2, as seen from the CTM, by re-calculating the air mass fraction based on modeled NOx profiles, as investigated in Goldberg et al. (2022). In addition, we evaluate the modeled meteorology and chemistry using a separate set of STILT–NOx simulations with true NOx emissions from the EPA for three US power plants (Sect. 4.1).
2.1 NOx net chemical tendency, , and uncertainty
Inspired by the theoretical non-linear curves of NOx lifetimes as functions of NO2 vertical column density and volatile organic compound reactivity (VOCR) and based on a box model in Laughner and Cohen (2019), we extract similar non-linear parameterizations using the Weather Research and Forecasting model coupled with Chemistry (WRF-Chem v4.0.2; Grell et al., 2005). Focusing primarily on polluted environments, we carried out WRF-Chem simulations for three mid-latitude cities and extracted model outputs from a 2∘ × 2∘ region centered around each city. Three cities, namely Los Angeles in the USA, Shanghai in China, and Madrid in Spain, represent typical megacities in North America, Asia, and Europe. Their varied climatic conditions and sectoral emissions of NOx, VOC, and GHGs provide a holistic view of the variability in the NOx chemical tendency. While our analyses extended to power plants and cities beyond these three training sites when compared to TROPOMI data (Sect. 4), it helps assess the broader applicability of our chemical parameterizations.
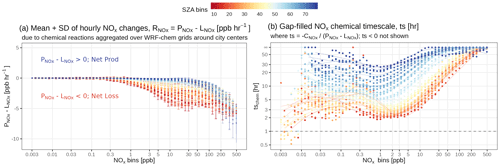
Figure 3A diagram of NOx net chemical loss tendency (; ppb h−1) as functions of the NOx concentration () and solar zenith angle (θ). The net loss timescale was first calculated for each 12 km grid cell of all WRF-Chem simulations for three cities (with specific model setups summarized in Appendix A) and then aggregated into multiple bins of NOx concentration (ppb). The NOx bins are equally divided in the logarithmic space. The solid dots and error bars denote the average and standard deviation of within each combined θ and bin. For the net loss timescale, only positive values are displayed, given the logarithmic scale of the y axis in panel (b), and data points with values > 72 h are simply treated as infinite.
Appendix A describes our specific WRF-Chem settings used to generate lookup tables of NOx chemical loss tendencies, which we will refer to as NOx curves (Fig. 3). Of the WRF-Chem settings, the chosen chemical mechanism (Regional Acid Deposition Model or RADM2; Stockwell et al., 1990) is the most relevant to the accuracy of these NOx curves. Despite uncertainties in these WRF-Chem simulations, what matters the most for reproducing the NOx tendency is how NOx varies with, for example, the solar zenith angle (SZA) and ozone, rather than the exact accuracy of NOx concentrations themselves (from WRF-Chem). Thus, non-chemical components (prior emissions, boundary conditions, and physical processes) in this specific WRF-Chem configuration do not necessarily need to be perfect or optimized against observations. We clarify that WRF-Chem simulations had been performed to facilitate the parameterization of the NOx tendency within STILT–NOx but are not required when running STILT–NOx.
By leveraging WRF-Chem's chemical diagnostic capability, we derive the net chemical tendency of NOx within each hour (, ppb h−1) for every model grid within the lower 12 vertical levels (). is calculated specifically from the cumulative changes in NO and NO2 concentrations, solely due to chemical reactions (i.e., chem_no2 and chem_no in WRF-Chem registry), following Eq. (2):
where the model hour h denotes the start time of each hour interval in the WRF-Chem outputs, and z denotes the index of model vertical levels (i.e., from 1 to 12). describes the cumulative net changes to the NOx concentration, given the chemical reactions from the initial model hour h0.
WRF-Chem pixel-specific hourly NOx rate changes, , are then grouped by both SZA (θ) bins, with a spacing of 2∘, and bins, with equal spacing, in log10 scale (Fig. 3a). θ is chosen, given the close relation to solar radiation under clear-sky conditions, and controls the photolysis frequency of ozone and OH production (Rohrer and Berresheim, 2006) when the ozone and water vapor abundance remain unchanged. Because the intention in using STILT–NOx is to inform the relationship between emission sources and satellite NO2 columns, which are almost always filtered to remove cloudy scenes (i.e., quality assurance of ≥ 0.7), the choice of θ without considering cloud coverage is reasonable. Specifically, these net chemical changes explicitly contain all NOx-relevant reactions within the WRF-Chem and RADM2 scheme, such as the recycling of NOx from oxidized odd-nitrogen species like peroxyacetyl nitrate.
The above grouping procedure of is based on a finite number of bins of , and θ unavoidably reduces the variability in the that were directly derived from WRF-Chem. To assess the extent to which the variability can be explained by the selected binning feature variables, we performed a sensitivity test to quantify the deviation of bin-averaged from the initial . Generally, the variability is better preserved over polluted regimes with a higher-NOx level > 1 ppb than over low-NOx regimes (Fig. S2a and b in the Supplement). Choosing alone better explains the variability than choosing SZA or air temperature alone. Including additional variables (e.g., air temperature, NO2 : NOx ratio, and VOCR) on top of our default choice of SZA and marginally improves the prediction of , except for the inclusion of ozone. However, estimating ozone remains a challenging problem; thereby, ozone is not included as a feature variable in this study.
As a net result, is mostly negative during the day, meaning that NOx is removed from the system. is large, with small spread at low θ of ≤ 20∘ and gradually decreases during the day. becomes positive as it approaches the nighttime hours (Fig. S2c), and its variability peaks during sunset when , with a fractional uncertainty of over 100 % (blue error bars in Fig. 3a) when considering the transition to nighttime chemistry. When focusing on the daytime portion with θ < 70∘ and ≥ 1 ppb, the spread in among the WRF-Chem urban pixels ranges from 12.2 % to 67.9 %, according to varied θ and (red to yellow error bars in Fig. 3a), with an average uncertainty of 41.2 %. When focusing on the nighttime portion with θ ≥ 70∘ and ≥ 1 ppb, the spread in spans from 27.9 % to over 100 %, with an average uncertainty of 96.3 % that is largely skewed by the high uncertainty around the dusk hours. Last, the average daytime uncertainty in the NOx tendency at medium to high NOx concentrations (i.e., 41.2 %) will be propagated into chemical uncertainties in tNO2 for cases of power plants and urban areas, which is further described in Sect. 3.
Given the further fluctuation in with , we define a net loss timescale (h) as and distinguish it from the conventional chemical lifetime that only accounts for chemical losses. For reference, a positive (or negative) timescale corresponds to a net loss (or production) of NOx (Fig. 3b). The contribution from NOx production is minor during noon hours. The non-linear dependence of with is largely driven by several NOx loss pathways (predominately by the loss processes of NO2 + OH and the formation of alkyl nitrates during the daytime and by the NO3 chemistry and heterogeneous chemistry at nighttime; Fig. S2). Here, we do not differentiate between the NOx curves by VOCR, despite its critical role in determining the turning point when NOx is mainly lost to either nitric acid or alkyl nitrates (Laughner and Cohen, 2019). We instead perform a sensitivity study of the impact on NOx curves for three VOCR intervals in Sect. 5.3. Note that these NOx curves should be considered to be a first-order approximation and can certainly be improved upon to evaluate more complex parameterization (Sect. 5.3). When it comes to calculating chemical changes within STILT–NOx per air parcel per timestamp (i.e., ΔCchem,p,t in Eq. 1), such a loss timescale is looked up according to parcel-specific θ and to enable the non-linearity core (Fig. 2; bottom panel).
2.2 NO2 : NOx ratio
As only the vertical column density of NO2 is retrieved, the fraction of NOx present as NO2 as TROPOMI passed over is an important component of our analysis. Prior studies estimated such ratios using a constant value (e.g., 0.75) at noon hours across seasons, with a 10 % uncertainty (Beirle et al., 2011, 2019; Goldberg et al., 2022), monthly mean climatology of ozone from reanalysis (Beirle et al., 2021), and CTMs. NOx is primarily emitted as NO but converted to NO2 via the reaction with ozone. During the daytime, NO2 is photolyzed back to NO with a photolysis frequency, . Thus, the NO2 : NOx ratio scales with the ratio of ozone and (Eq. 3a).
Considering the close coupling between NO2 and O3, their sum Ox in Eq. (3b) is a key indicator of the atmospheric oxidant capability for understanding the urban air chemistry (Clapp and Jenkin, 2001; Fujita et al., 2016) and informing chemical dynamics (e.g., during the COVID-19 pandemic; Parker et al., 2020; Lee et al., 2020). Ox levels within the PBL can be regarded as a NOx-independent component related to regional ozone inflow plus a NOx-dependent component that varies non-linearly with local NOx and VOCR conditions (Clapp and Jenkin, 2001; Jenkin, 2004). The complexity in the local Ox–NOx non-linearity is caused by key reactions behind NOx curves, which are discussed in Sect. 5.3.1.
For simplification, we prescribed a typical Ox level of 50 ppb in the first version of STILT–NOx and calculated the NO2 : NOx ratio via Eq. (3), assuming a steady state.
where relies on θ for daytime, and the reaction rate coefficient of NO with O3 () is a function of the air temperature TA and pressure P (Fig. 2; bottom panel). The inclusion of Ox in calculating the NO2 : NOx ratio is to avoid a non-physical infinite conversion of NO to NO2 at high-emitting sources, following the titration of the ambient ozone. Sensitivity tests were performed to reveal how biases in the prescribed Ox level may modify the modeled tNO2 (Sect. 3). Typical NO2 : NOx ratios over the examined mid-latitude targets across seasons are summarized in Sect. 5.2. In the future, satellite observations of tropospheric ozone could be used to add the additional complexity of variable Ox.
2.3 Inter-parcel mixing
Eulerian chemical models often suffer from mixing or numerical diffusion that is too strong within their model grid, while Lagrangian models (equivalent to those possessing extremely high spatial resolution) may lack any mixing between air parcels that are normally assumed to be independent of one another (Lin et al., 2013; Brunner, 2012). Such a lack of mixing has a negligible impact on the passive tracers, as mixing alters only the spatial distribution of concentration among air parcels but not the resultant concentration averaged across parcels at the receptor. However, non-linear processes alter both the spatial distribution of parcel-specific concentrations and the average resultant concentration. As a result, the calculation of the total NOx tendency will be sensitive to how inter-parcel mixing is parameterized. Common ways to realize turbulence mixing are through (1) stochastic processes followed by the exchanging or averaging properties of air parcels found within a certain mixing length (e.g., STILT-Chem; Wen et al., 2012), (2) implemented deformation-driven and instability-driven schemes that rely on atmospheric stability and wind shear or stress characteristics (e.g., CLaMS; McKenna et al., 2002; Konopka et al., 2019), and (3) diffusion approaches that require the vertical gradient of concentrations (e.g., CiTTyCAT and ELMO-2; Pugh et al., 2012; Strong et al., 2010).
Here we follow the STILT-Chem approach to enable a process of exchanging concentrations per timestamp among the air parcels in close proximity to each other (ΔCmix term back in Eq. 1), which smooth the horizontal gradient of concentrations among those air parcels. Specifically, at the timestamp of t, the concentration for a given air parcel p is updated, based on the concentration gradient between p and its neighborhood according to a mixing timescale (τmix) within a grid volume, with a mixing length scale of a horizontal area and the mixed layer height for the height as follows:
where implies the degree of horizontal mixing, and represents the average concentration among air parcels within the mixing volume. The update of from Cp,t responds to ΔCmix in Eq. (1). A relatively fast mixing timescale of 3 h and a horizontal mixing length of 1 km is used for testing the mixing impact on modeling tNO2. Although we neglect the mixing in the free troposphere and the mixing between the mixed layer and the free troposphere in this first model version, we tested a spectrum of the horizontal mixing scales and include possible future improvements (Sect. 5.3.2).
As atmospheric transport and chemical transformation are the two main components in any CTMs, we assess how uncertainties tied to the modeled wind field, NOx loss timescale, and NO2 : NOx ratio may contribute to uncertainties in tNO2 (in ppb).
Here we briefly describe how various tNO2 uncertainties were approximated, based on our understanding of errors in respective model parameters or inputs (i.e., wind error, NOx chemical tendency, or Ox levels). To approximate the tNO2 uncertainties due to transport errors, we followed previous approaches to first assess the GFS- and HRRR-modeled wind profiles against radiosonde; calculate respective error statistics including wind error, correlation time, and length scales; and, last, propagate wind error statistics into errors in column concentrations. Mathematically, in Eq. (5) is derived from the difference in the variance of STILT–NOx air-parcel-specific NO2 concentrations between the original simulation and a second simulation with wind error (Lin and Gerbig, 2005; Wu et al., 2018). The derivations of modeled wind errors and contributions to tNO2 errors are elaborated in Appendix B. To evaluate the impact due to errors associated with chemical parameters, we perturbed the NOx curves or the Ox level according to 20 perturbing factors. Perturbed curves or parameters are used to generate 20 new sets of tNO2 fields, of which their respective standard deviation among perturbations serves as the chemical uncertainty (ppb) due to NOx net loss timescale and NO2 : NOx ratio (σts and σnn in Eq. 5). These 20 perturbing factors were randomly selected from a normal distribution N (). Here we tested out σparam of 40 % for NOx loss timescales, according to uncertainties in the chemical tendency (Fig. 3) and a σparam of 40 % for the Ox level (Eqs. 3).
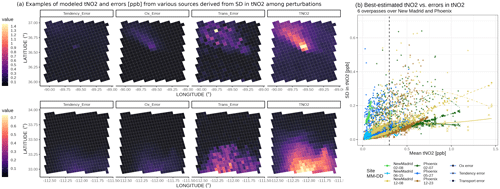
Figure 4(a) Demonstrations of best-estimated tNO2 (TNO2) and their uncertainties (ppb) due to random u or v wind errors (Trans_Error), NOx chemical tendency (Tendency_Error), and Ox levels (Ox_Error) on 8 December 2020, over the New Madrid power plant (USA), and 23 December 2020, over Phoenix (USA). (b) Scaling between uncertainties and mean tNO2 signals over six overpasses for the two targets with smooth splines fitted (crosses with solid lines for tendency errors, stars with dashed lines for Ox errors, and circles with dotted-dashed lines for transport errors). Colors differentiate the sites and TROPOMI overpass times.
Due to heavy computational expenses in conducting such wind and chemical perturbation analyses for all overpasses and locations, we only ran error analyses for a total of six overpasses over a power plant and a city. To cover the seasonal changes in the NO2 signals and their uncertainties, overpasses in varied seasons are examined for the New Madrid (USA) power plant on 8 February, 15 June, and 8 December 2020 and Phoenix (USA) on 7 February, 27 May, and 23 December 2020. Two winter cases with relatively large signals are shown in Fig. 4a. Considering the non-linearity between the chemical tendency and NOx concentration, sounding-specific uncertainties for all six cases are presented against modeled tNO2 in Fig. 4b. When conducting those perturbations, other model parameters like the meteorological field and emissions remain unchanged.
As a result, the average percent error in u or v wind speed in the PBL is roughly 22 % for the New Madrid case (Fig. S3a in the Supplement), which contributes to 50 % uncertainty in tNO2 at the sounding level (third column in Fig. 4a). Higher transport errors may occur more frequently if an intensive point source is in the area or over pixels on the border of the NO2 plumes, with moderate signals of about 0.2 to 0.5 ppb (e.g., dots in Fig. 4b). This is because a small deviation in the modeled wind vectors causes air parcels to either hit or miss the intensive source. The transport uncertainty appears to first correlate positively with the signals and then decreases when signals are sufficiently high, e.g., > 0.7 ppb. Such a decline may be associated with hyper-near-field soundings, where the deviation in wind fields may not alter modeled signals, as modeled air parcels will always experience a large influence from the emission source (dotted–dashed lines in Fig. 4b). Compared to power plants, cities may be associated with a more homogeneous transport uncertainty if emissions are more homogeneous and better mixed in the PBL.
Given a roughly 40 % uncertainty in the Ox levels or NOx chemical tendency, chemical uncertainties (in ppb) remain small when modeled signals are compared (Fig. 4a). Uncertainties from chemical tendency first increase with tNO2 signals and gradually plateau for tNO2 beyond 0.7 ppb, likely because NOx is lost slowly when the NOx concentration stays high, and further perturbations in the chemical tendency are less impactful. In contrast, the uncertainty from Ox levels appears to consistently scale against the signals, i.e., more apparent for soundings adjacent to the power plant, with reasons explained as follows. When the certain perturbed Ox level approaches zero, the amount of NO that can be oxidized as NO2 becomes minimal (second column in Fig. 4a). This case mimics the scenario where O3 can be titrated in proximity to an intense release of NO before the ozone-depleted plume air is mixed with the ambient ozone-rich air. Nevertheless, considering the entire sample, the percent of errors due to chemical parameters remains relatively low (13 % to 18 % for six cases in Fig. 4b).
Whether chemical or meteorological errors dominate the total model errors fundamentally depends on tropospheric NO2 signals which further rely on factors like atmospheric stability with wind errors, chemical tendency, and emission distribution. Such a dependence leads to a spatial gradient and seasonal variations in estimated errors, as seen from the above examples. In brief, our limited perturbation experiments suggest that transport uncertainties dominate the total modeled uncertainties, except for a few hyper-near-field soundings, where chemical uncertainties become more substantial.
For future emission optimizations, uncertainties in the emissions, retrieval, and background should also be included. Despite the significant advance in the TROPOMI NO2 retrieval version of v2 compared to v1 (Van Geffen et al., 2022), v2 retrieval is associated with a fractional uncertainty (normalized over retrieved tNO2) of ∼ 30 % to 50 % for most soundings within the plume. Uncertainties in the NOx emissions between inventories can serve as the prior uncertainty, which is substantial at the pixel level (Figs. S4 and S5 in the Supplement). Besides the regional wind assessment, a novel plume rotation algorithm based on model–data NO2 plumes is proposed in Sect. 5.2 to quantify near-field wind biases.
The tropospheric NO2 mixing ratio at a given sounding location is influenced by the regional inflow, atmospheric advection and turbulence mixing, underlying emission characteristics, and chemical changes en route to the sounding (Fig. 2). Modeled tropospheric NO2 mixing ratios using a variety of model configurations are compared against retrieved values from TROPOMI. Such model–data comparisons help evaluate the overall model performance and the roles of individual physical and chemical processes with a naming convention of 〈MET〉_〈EMISS〉_〈GAS〉_〈PROC〉, which is explained as follows:
-
〈MET〉 represent the meteorological fields of either 0.25∘ GFS or 3 km HRRR that are used to drive STILT air parcels.
-
〈EMISS〉 represent two prior NOx emission inventories. EDGARv6.1 is presented with monthly mean emissions, and the latest year available (2018) is the primary one for simulating all cases. Hourly mean emissions from EPA reports are only used to evaluate modeled chemistry and meteorology for several US power plants (Sect. 4.1).
-
〈GAS〉 represent the simulated species, with a default string of TNO2 without subtracting a localized tNO2 background. A separate comparison with the background subtracted is shown in multi-track comparisons (Fig. 6; Sect. 4.1).
-
〈PROC〉 denotes the physical and chemical processes considered per run. Two main configurations include (1) DEF runs, with both inter-parcel mixing and chemical parameterization included, and (2) NOCHEM runs, with mixing but without considering the NOx chemical tendency. The NOCHEM runs do account for the NO2 : NOx conversion but as a constant ratio of 0.74, according to EMG-based studies.
Only model–data comparisons using TROPOMI v2 are shown. As the satellite averaging kernel (AK) and observed tNO2 differ substantially between v1 and v2, modeled concentrations are weighted by the version-specific AKs to yield apples-to-apples comparisons. Changes in AKs and the retrieved and modeled values between versions are summarized in Fig. S6 in the Supplement.
4.1 Model validation: US power plants
The New Madrid power plant along the Mississippi River is a 1300 MW coal-fired power station (GEM, 2021), which ranks first in 2020 among the US power plants regarding NOx emissions provided by EPA. The Thomas Hill and Martin Lake power plants ranked second and third in 2020, respectively. We also report results for an overpass over the Intermountain power plant in Utah, where the surrounding complex terrain is difficult to model properly. Let us start with two examples to illustrate plumes modeled by different model configurations (Sect. 4.1.1) and then present model–data comparisons over dozens of overpasses of the three power plants (Sect. 4.1.2).
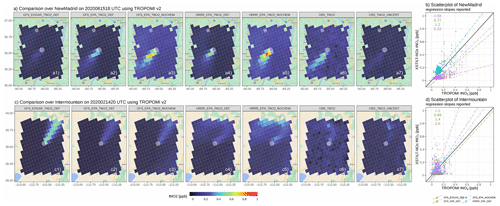
Figure 5Maps (a) and scatterplots (b) of the modeled plumes, based on several model configurations (first five columns) versus retrieved plumes and uncertainties from TROPOMI v2.3 (last two columns) for the New Madrid power plant on 15 June 2020 and Intermountain, a Utah power plant, on 14 February 2020. Varied model configurations are labeled at the top of each panel, following the naming convention of 〈MET〉_〈EMISS〉_〈GAS〉_〈PROC〉, as explained in the list of Sect. 4. In particular, _DEF and _NOCHEM denote the modeled columns using the default (with mixing and chemistry) and non-chemistry configurations. Grid cells with intensive NO emissions from EDGARv6 are labeled as white circles, with the sizes denoting the relative emission magnitude. The type-II linear regression slope is fitted for each configuration (dotted–dashed line), and modeled and retrieval uncertainties are added (dashed error bars). The underlying road maps were created using the ggmap library in R (map data © 2023).
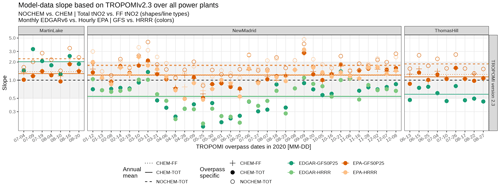
Figure 6A summary figure of the linear slope between the observed tNO2 and simulated tNO2, using a variety of model configurations over all three US power plants. Model configurations include simulations (1) with or without NOx chemistry parameterization (empty vs. solid dots); (2) using default EDGAR or scaled emissions from EPA (green vs. orange dots); (3) using 0.25∘ GFS or 3 km HRRR (dark green or orange vs. light green or orange dots); and (4) using total tNO2 or background-removed local enhancements (e.g., CHEM-FF as crosses). Annual mean slopes are displayed as horizontal solid or dashed lines. The model evaluation here uses TROPOMI v2.3 and emissions from EDGARv6.1. Evaluations that are based on TROPOMI v1.3 and annual mean EDGARv5 emissions are shown in Fig. S13a in the Supplement.
4.1.1 Single-track demonstration
The correction in NOx emissions greatly improves the model–data alignment. For example, EDGAR-based simulations substantially underestimate or overestimate the tropospheric columns (Fig. 5a1 and c1), as EDGAR emissions are almost one-third or twice of the reported hourly EPA emissions for the New Madrid or Intermountain power plants, respectively (Fig. S7 in the Supplement). EPA-based simulations align better with retrieved values from TROPOMI v2, despite deviations over the far-field region (Fig. 5a2 vs. Fig. 5a6). Such improvements in the model–data alignment are also inferred from the linear regression slopes reported in Fig. 5b and d. Not accounting for NOx chemistry or lifetime elevates NO2 concentrations both within the plume and over the background, even if EPA emissions are assumed to be correct (Fig. 5a3 and a5). The inter-parcel mixing with a 3 h mixing timescale redistributes the NOx concentrations among adjacent air parcels but leads to a minimal impact of ≤ 5 % of the modeled tNO2 at individual column receptors (thereby not shown).
The choice of meteorological fields with different spatial resolutions insignificantly affects the modeled signals, except for cases surrounded by complex topography and flows. For example, the HRRR-based plumes resemble the GFS-based plumes for the New Madrid power plant (Fig. 5a2 vs. Fig. 5a4), which is also revealed by their similar wind error statistics (Fig. S3a). However, the complex terrain and stable PBL during wintertime complicate and usually worsen the model performance as a result of increased meteorological errors. On 14 February 2020, EPA-based plumes over the Intermountain power plant in Utah using two meteorological fields differ substantially from each other, and they both deviate from the observed plume regarding the plume shape. GFS delineates the mean wind direction within its coarser 0.25∘ grid box, while 3 km HRRR offers more spatial variability in wind directions (Fig. 5c2 vs. Fig. 5c4). Yet, precisely capturing the curvature in the wind vector is extremely challenging, even when using 3 km meteorological fields (Fig. 5c6) and is more difficult when using Gaussian plume approaches that rely on only one effective wind vector. Such a model–data mismatch in plume shapes can further help to quantify the wind biases, which are discussed in Sect. 5.2.1.
Besides modeling challenges, the retrieval uncertainty cannot be neglected, as it ranges from 22 % to 31 % of the retrieved signal for the New Madrid case (Fig. 5a7) and up to 100 % for the Intermountain case (Fig. 5c7) at the sounding level. When using retrieved data and the averaging kernel from TROPOMI v1, the regression slope becomes 1.18 and 1.25 (Fig. S8 in the Supplement), indicating that modeled plumes using both meteorological fields are larger than observed plumes. While using TROPOMI v2, the respective slopes are 0.88 and 1.2 (Fig. 5b and d). This again emphasizes the substantial uncertainty in the retrieved signals, which is large enough to even alter the conclusion of whether emissions are underestimated or overestimated for a single overpass, and the need for analyzing multiple overpasses for evaluations (Sect. 4.1.2).
4.1.2 Multi-track evaluation
To provide a broad impression of the model performance, we expand the model–data comparisons to a total of 50 TROPOMI overpasses across all seasons in 2020, including 34 overpasses for the New Madrid power plant and 9 and 7 summertime overpasses for the Thomas Hill and Martin Lake power plants, respectively. These overpasses are selected based on their relatively intense signals compared to the surroundings. Model–data comparisons for all overpasses are shown in the maps in Figs. S9–S12 in the Supplement, with the linear regression slopes reported and summarized in Fig. 6 and Table S1 in the Supplement.
Cases with slopes deviating significantly from 1 are usually associated with substantial near-field wind directional biases. For instance, modeled wind vectors on 11 March, 28 April, and 9 September 2020 have directional biases of > 30∘ (Figs. S9b and S10b), which explain the respective abnormal linear regression slopes of −1.75, 0.49, and 3.2 (Fig. 6). EDGAR-based simulations are biased too high or too low by a factor of 2 or more when compared to observed values from TROPOMI v2.3 (green dots in Fig. 6) that are driven by the biases in the EDGAR emissions (Fig. S7). The NOCHEM simulations without accounting for NOx losses overestimate tNO2 by a factor of 2 across all seasons and three power plants, regardless of the meteorological or emission fields adopted (empty circles in Fig. 6). Upgrading meteorological fields to a higher resolution seems to contribute less to the improvement of model–data agreements than correcting the emissions or chemistry. In the end, modeled values with NOx chemistry and correct EPA emissions using either GFS or HRRR yield the best agreement with retrieved values from TROPOMI v2 (orange dots and lines in Fig. 6). When aggregating the results of all overpasses, simulations using the best knowledge of the emissions, the simplified chemistry, two different meteorological fields, and inter-parcel mixing are biased slightly high (regression slope up to 1.2; Table S1). RMSE values between the observed and modeled tNO2 with NOx chemistry-enabled range from 0.11 to 0.15 ppb (Table S1), which is comparable to the random uncertainty in the NO2 retrieval of 0.09 ppb.
The statistics discussed above compared the total tropospheric NO2 columns from the model and TROPOMI for soundings around each power plant. It is noticeable that modeled tNO2 uncontaminated by emissions (i.e., background tNO2) are sometimes slightly lower than observed background tNO2 (Figs. S9a and S10a), possibly because higher chemical uncertainties are related to low-NOx regimes, and non-anthropogenic NOx sources from soil and lightning are excluded from current simulations but can play a significant role on tNO2 over rural regions (Goldberg et al., 2022; Shah et al., 2023). In particular, column contributions from lightning NOx emissions aloft may be amplified, since the TROPOMI NO2 retrieval has a higher sensitivity towards the free troposphere than PBL. Since our current model setup only accounts for anthropogenic NOx sources below 2 km, we conducted an additional test by subtracting the background tNO2 from total tNO2 to arrive at observed anthropogenic enhancements (the second paragraph in Sect. 2), assuming that the soundings within or outside the plumes have equal contributions from the nearby non-anthropogenic NOx sources. After subtracting the tNO2 background, the model–data comparison based on observed tNO2 enhancements does not change dramatically (e.g., orange crosses vs. orange solid dots in Fig. 6).
In summary, using more accurate NOx emissions with chemistry considerably improves the model–data comparison. Increasing the spatial resolution of meteorological fields has less impact on cases with relatively flat terrain. Larger model–data mismatches generally are associated with larger wind directional biases. Modeled values in tNO2 may be biased to be slightly low in summer months from April to June and high in winter months from November to February, with minimal annual biases, assuming that the EPA emissions and observed tNO2 are unbiased.
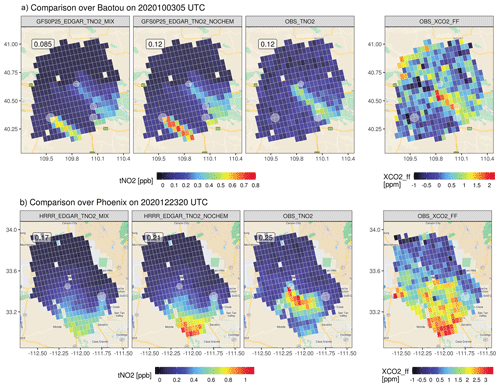
Figure 7An example of GFS-based tNO2 plumes over Baotou (China) on 3 October 2020 (a) and the HRRR-based tNO2 plumes over Phoenix (USA) on 23 December 2020 (b). Modeled plumes are generated using annual mean ENOx from EDGAR, with the top emitters highlighted in light-gray circles. Both observed tNO2 and the anthropogenic XCO2 enhancements from OCO-3 are plotted. XCO2 enhancements calculated from a local background have been averaged based on the TROPOMI sounding size. Overpass time differences between TROPOMI and OCO-3 for the two cases are < 1 h. TROPOMI observations are cropped to match the boundary of the available OCO-3 soundings. The underlying road maps over Baotou and Phoenix were created using the ggmap library in R (map data © 2023).
4.2 Model application: two cities
We now move to city cases that include an industrial Chinese city, Baotou, and one of the fastest growing megacities in the US, Phoenix. As CO2 and NOx are commonly co-emitted into the atmosphere, observed XCO2 enhancements derived from the Orbiting Carbon Observatory 3 (OCO-3) Snapshot Area Mapping (SAM) mode are displayed with observed tNO2 (Fig. 7). Background XCO2 is defined as the mean values over the background region that is determined by NO2 plumes (modified from the background approach in Wu et al., 2018). Both cities possess relatively richer OCO-3 SAM observations co-located with TROPOMI data. Since no true NOx emissions are available for cities, EDGAR is utilized as the prior emission inventory for simulating tNO2 and optimizing NOx emissions.
We simulated 18 and 12 TROPOMI overpasses, respectively, for Baotou and Phoenix (Figs. S14 and S15 in the Supplement) and first presented one example per city in Fig. 7. Baotou is surrounded by four point sources, as suggested by EDGARv6, but one large source in the city center, as informed by both the observed tNO2 and XCO2 enhancements on 3 October 2020 (Fig. 7a). Such a mismatch is confirmed by the comparison of normalized tNO2 across all 18 TROPOMI overpasses with various wind speeds and directions (Fig. S14b), suggesting that EDGAR very likely misallocated anthropogenic NOx sources. Similarly, the largest emission source to the east of the city center of Phoenix, according to EDGAR, seems suspicious and may again be misplaced when more overpasses are simulated (Fig. S15b). The observed plume is concentrated near the city center, compared to the HRRR-derived plume that dispersed farther away from the city center on 23 December 2020 (Fig. 7b). Such a spatial offset of the tNO2 plumes is likely due to an overestimation in the modeled wind speed, which pushes the plumes to the southern edge while diluting tNO2 values over the urban core.
When more overpasses are examined, the model captures the seasonal variation in tNO2 well (i.e., higher or lower values in winter or summer months; Figs. S14a and S15a). Other than emission biases that affect all cases, a few overpasses stand out for Baotou, with poorer agreements with TROPOMI likely owing to (1) clear biases in wind direction on 31 May, 9 August, 15 December 2020, and 19 February 2021; (2) a likely overestimation in STILT footprint that may trigger several effects on 29 September, 29 March 2020, and 16 October 2021 (Fig. S14a). Although STILT can characterize the sub-grid-cell turbulent mixing by its stochastic nature, the 0.25∘ GFS may be insufficient to resolve the complex terrain and air flows, contributing to biases in wind directions and planetary boundary layer heights (PBLHs) over mountainous locations (Lin et al., 2017) such as over Baotou. Deviations in the PBLH may cause a cascade of effects, such as deciding up to what height the emissions are diluted, whether such height is above or below the emission or plume height, and chemical changes along the way. Such effects may be magnified under low-mixing and low-wind conditions where the model particularly struggles with the accuracy of PBLH. Without much mixing between the plume and background air, the prescribed available Ox level may be overestimated adjacent to intensive NOx sources. Overestimation in the NO2 concentration may further be amplified when considering the dependence of NOx rate changes on its concentration. Hence, concentrations of chemically reactive species under low-mixing scenarios are extremely challenging to model properly with an extreme during the nighttime.
Our ultimate goal is to explore what can be learned about the emission characteristics from anthropogenic hotspots with the joint use of space-based NO2, CO, and CO2 plumes. As an intermediate step, this study is informed by previous efforts to extract and constrain urban CO2 emissions from satellites using a Lagrangian framework (Wu et al., 2018; Roten et al., 2022) and extends it to the interpretation of tropospheric NO2 satellite data. To diagnose NOx emissions from NO2 column signals, we need to effectively account for how NOx evolves in air parcels from its initial source to locations sampled by TROPOMI, making the Lagrangian perspective an ideal candidate. Now we discuss when and how such a framework can be of most use and also the possible future improvements.
5.1 Model advantages and flexibility
Our framework accounts for atmospheric transport and chemical transformation in a more rigorous way than typical statistical approaches such as the EMG method and in a more efficient way than full-chemistry models that explicitly resolve individual chemical reactions.
Another advantage of the STILT–NOx design is that each of the three main components (trajectory calculations representing air transport, chemical production or loss of the target species, and optimization of emission) are independent and each can reuse the previously saved output from the others. For example, if one wanted to test how sensitive model concentrations were to the chosen chemical scheme, then the simulations of atmospheric transport can be reused via the storage of trajectory-based modeling, thereby reducing the computational cost. Our prototype demonstrates a global solution of the NOx chemical tendency parameterized by the one set of NOx curves in Fig. 3. Although this simplification may be thought of as a limitation, one can easily replace those default curves with alternatives that are tailored toward a specific region or regime of interest. Such flexibility can inform us of the influence on modeled columns from NOx curves derived from different chemical mechanisms. Similarly, one can investigate the sole meteorological influence by diversifying the meteorological and mixing parameters. Moreover, because air parcels in LPDMs are not tied to a certain atmospheric tracer, we can estimate the concentrations of various species along model trajectories. It allows us to constrain emissions for multiple atmospheric constituents in a consistent framework, which may shed light on tracer–tracer analyses (Sect. 5.2).
The Lagrangian modeling approach has its inherent benefits. First, the generation and recording of trajectories can easily reveal the source regions that are only relevant to a specific satellite sounding and the sub-city-scale variations in emission characteristics (Wu et al., 2022). In addition to storing latitudinal and longitudinal coordinates and extrapolated meteorological quantities along every trajectory at each timestamp, STILT–NOx outputs and records NOx concentration changes due to every process, including emission, net chemical changes, and inter-parcel mixing at minute scales. Those trajectory-level concentration changes are further driven by several model configurations listed in Sect. 4, which facilitates model debugging and comprehends modeled results. See Sect. 5.2 for one of the applications. Second, the spatial resolution of concentration calculations is not bounded by the rigid boundary of model grid cells, which is particularly important for dealing with non-linear processes for chemically active species. As demonstrated in several studies (e.g., Valin et al., 2011), the grid-averaged concentration may undergo excessive mixing in Eulerian models, and the concentration-driven chemical tendency varies with the adopted spatial resolution. While the Lagrangian perspective solves for concentration changes at extremely high spatiotemporal resolutions, inter-parcel mixing schemes can be implemented to smooth the concentration gradients, whereas it may be challenging to recover the sub-grid-cell concentration gradients in the Eulerian framework unless also increasing the spatial resolution.
More broadly, the proposed simplified parameterization of the non-linear NOx tendency or NOx curves is not limited to the STILT framework and can potentially be incorporated into other Lagrangian modeling frameworks or even Eulerian frameworks with a fine spatial resolution to resolve the local variability in chemistry.
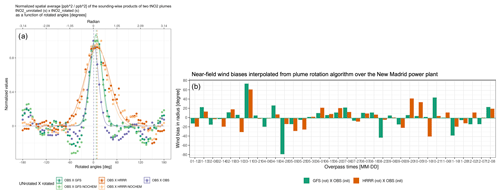
Figure 8(a) An example of the normalized spatial mean of the sounding-wise product (ppb2 ppb−2) between an unrotated observed tNO2 and a rotated plume for the New Madrid power plant on 15 June 2020. Gaussian-like curves are fitted to each set, with the mean and standard deviation indicating modeled wind biases. Five sets of rotated plumes include observed tNO2 (purple) and simulated tNO2 driven by GFS (dark green) or HRRR (dark orange), with or without the account of the NOx lifetime (light green or light orange). The horizontal dashed lines denote the μ parameter that can translate into wind bias in degrees or radians. (b) Near-field wind directional bias quantified by the modeled tNO2 plumes, using 3 km HRRR (orange bars) or 0.25∘ GFS (green bars), and retrieved tNO2 plumes for every examined TROPOMI overpass (y axis; in degrees), following the rotation algorithm in panel (a).
5.2 Implications for constraining urban CO2 emission and emission ratios
The knowledge learned from analyzing NO2 plumes can be transferrable to constraining bottom-up CO2 emissions. Two main sources of biases influencing the urban CO2 emission constraint include biases in wind direction and emission locations. Model–data mismatches in NO2 columns have shown great value in easily identifying the biases with emission locations, even without deploying atmospheric inverse analyses (Sect. 4.2), especially for point sources in urban areas, when plumes from multiple sources overlap less with one another. Additionally, diagnosing the NOx emissions can facilitate CO2 emission estimates in two ways. It can reveal systematic biases in near-field wind directions (Sect. 5.2.1) and quantify the NOx : CO2 emission ratios for point and/or area sources to assist in sector-based attribution (Sect. 5.2.2).
5.2.1 Quantifying wind bias
In addition to leveraging limited radiosonde measurements, the obtained modeled and retrieved NO2 plumes can be used to quantify wind biases to improve the accuracy of top-down CO2 emission constraints, whether or not conventional atmospheric inversions are employed. To do so, we conducted a second wind assessment involving a plume rotation algorithm. In brief, a NO2 plume from either model or retrieval is rotated clockwise (α from −180 to −5∘, with a spacing of 5∘) or counter-clockwise (from 5 to 180∘) around the emission source and then resampled onto the original TROPOMI pixels (Fig. S16 in the Supplement). tNO2 from an original and a rotated plume are multiplied to arrive at a cross-product of tNO2 (in ppb2), which is analogous to the concept of cross-correlation. The original or rotated plume can be chosen from either the model or observations, and their normalized cross-product can be expressed as a function of rotating angles (colors in Fig. 8a). More details on intermediate steps to calculate such a function are described in Appendix C.
As a result, the width of the Gaussian-shaped curve of the cross-product (as measured by the σ parameter of a Gaussian fit) reflects the bias in the plume shape resulting from horizontal dispersion. A larger area under the Gaussian curve indicates a greater overlap between the initial plume and the rotated plume. More importantly, deviations in the central line of the Gaussian fit away from zero (as measured by the μ parameter) imply possible biases in the near-field wind direction for each TROPOMI overpass (Fig. 8b). Specifically, wind directional biases of both GFS and HRRR appear to be smaller from May to early September than in the remaining months (Fig. 8b). A few outliers stand out due to large wind biases on 11 March, 28 April, 20 September, 5 October, 16 October, and 16 November 2020.
By identifying those outliers with strong wind directional biases, one can consider either removing those cases or assigning a larger observational uncertainty when attempting to constrain emissions of NOx, CO, and CO2, assuming that their emissions are mostly co-located. Alternatively, we can use this rotating algorithm to create a model plume with minimized wind directional bias before being fed into atmospheric inversions or data assimilation systems (which usually deal with random uncertainties). A more sophisticated approach would be to optimize the emission and wind field simultaneously (Liu et al., 2017). More investigations may be needed to examine the degree of freedom of such a wind-emission optimization framework.
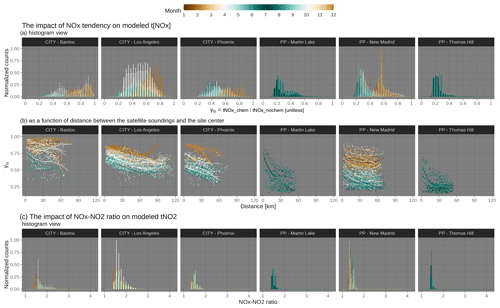
Figure 9A quantitative metric of the impact from NOx chemistry on tropospheric NOx and NO2 columns. Ratios in tNOx between simulations with and without chemistry are calculated as , which is displayed as a histogram (a) and as a function of the distance of the satellite sounding from the site center (b). Soundings in summertime overpasses are colored in dark green, whereas brown is used for soundings in the dormant months. Soundings of all overpasses for all city and power plant cases are included in histograms. Only downwind soundings in the NO2 plumes are included in the distance panel (b), with a smooth spline fitted per overpass to reveal the anti-correlation. The ratio between the modeled tropospheric NOx column versus the tropospheric NO2 column is derived from each sounding to reveal the NO2 : NOx ratio influence (c). As a reference, most previous studies adopted a constant NOx : NO2 ratio (reciprocal of NO2 : NOx ratio) of 1.32 and can reach 2 in a hyper-near-field area of a major NOx source.
5.2.2 Quantifying emission ratios between NOx and GHGs
Our modeling development offers additional insights into the discrepancy between emission ratios at the sources and directly observed enhancement ratios between two species with different chemical lifetimes. The joint use of NO2 and CO2 has enabled the calculation of emission ratios by adopting a spatial constant NOx lifetime (MacDonald et al., 2022; Hakkarainen et al., 2023) and the constraint of CO2 emissions using NO2 plumes by adopting inventory-based emission ratios (Zheng et al., 2020; Zhang et al., 2023). However, inventory-based emission ratios might not be well constrained, and the impact of how NOx decays over time and space on observed ENOx : ECO2 emission ratios has not been comprehensively assessed, which may impair the ability to accurately quantify such observed emission ratios (Kuhlmann et al., 2021).
Thanks to the ability of our model to track NOx and NO2 concentrations along trajectories with different model configurations, we can provide an assessment of the influence of atmospheric chemistry on estimating emission ratios. Specifically, the impact from NOx net losses from each satellite sounding(s) is specified as the ratio of modeled tropospheric NOx with chemistry over that without chemistry in the following: . Because NOx is simply treated as a passive tracer like CO2 in the NOCHEM simulations, γts are naturally smaller than 1. Lower γts corresponds to faster NOx chemical frequency and more chemical losses en route to the sounding location, suggesting that NO2 : CO2 enhancement ratios derived directly from satellites need to be scaled up to render the ENOx : ECO2 emission ratios at source locations.
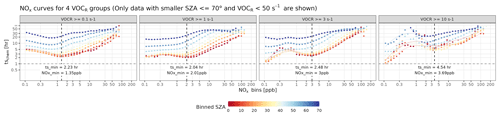
Figure 10(a) Similar to Fig. 3b but differentiated by four intervals of VOCR and SZA bins smaller than 70∘, with a spacing of 10∘. All panels here utilized model results from the same WRF-Chem simulations described in Sect. 2 and Appendix A.
We calculated γts for every sounding and present their distribution as histograms in Fig. 9a or as a function of the distance from the emission source (Fig. 9b). γts ranges from 0.24 to 0.61 for three power plants and from 0.42 to 0.84 for three cities, where lower values correspond to summer months (green bars in Fig. 9a). That is to say, the directly observed NO2 : CO2 enhancement ratios may have to be scaled up by 1.2 to even 4 times across seasons to properly recover the NOx being lost en route from emission sources to the sounding locations. Not properly accounting for such an effect leads to an underestimation of the derived emission ratios from satellites. More importantly, discrepancies between enhancement ratios and emission ratios, reflected by γts, are not spatially uniform. γts gradually decline as soundings move away from the emission sources (Fig. 9b). Soundings located farther downwind of emission sources tend to undergo more chemical transformations, likely because NOx losses become more rapid as NOx concentrations become lower by atmospheric dispersion (triggering positive feedback). We clarify that only downwind soundings affected by major NOx emissions are included in Fig. 9b, and simulations with or without chemistry have included the effect of atmospheric dispersion as distance increases. Furthermore, how quickly γts declines with distance depends on the wind speed and heterogeneity in emissions. For example, the faster the wind may be, or the more isolated emissions there are, the steeper γts decline with distance. γts at the distance of 0 are much lower than 1 in summer, which suggests that the chemical transformation can affect the NOx inflow. We further observe slight differences in the distribution of γts for cities versus power plants. Histograms of both tropospheric NOx (Fig. S17 in the Supplement) and γts over cities are associated with a wider spread than power plants because cities contain a wider spectrum of emission types and intensities.
Last, enhancement ratios need to be adjusted considering the NO2 : NOx ratio and differences in averaging kernels among two retrievals. The medians of our estimated NOx : NO2 ratio over power plants and cities range from 1.33 to 1.66, which generally aligns with previous studies of around 1.32 (Beirle et al., 2011; Goldberg et al., 2022). Our estimates are lower in winter than in summer and can be as large as 2 or 3 for a few soundings experiencing intense NOx sources (Fig. 9c).
5.3 Limitations and room for improvement
The diversity of VOC emissions, the vertical profiles of emissions, and the extent of inter-parcel mixing may impact the modeled results. Perhaps one of the biggest limitations of the current NOx chemical representation lies in not directly accounting for VOCs, which may affect (a) the sweet spot on NOx curves, where two NOx loss pathways reach their maximum, and (b) the Ox-based NO2 : NOx ratios (Sect. 5.3.1). Moreover, the influence of representations of the emission profile on modeled tNO2 can be magnified when further when considering the TROPOMI NO2 averaging kernel (Sect. 5.3.2). Simulations of point sources like power plants may be more sensitive to these factors compared to simulations of areal sources.
5.3.1 The impact from VOCR
To investigate the impact of VOCs on NOx curves, we calculated the VOC reactivity against OH from existing WRF-Chem results, based on the following formula: VOCR = and generated separate sets of NOx curves for four respective VOCR intervals of [0.1, 1), [1, 3), [3, 10), and [10, 50) s−1, with a coarse SZA bin spacing of 10∘. Curves become much noisier at night and in pristine environments with extremely low NOx (≤ 0.1 ppb), where WRF-Chem and RADM2 may be less suitable (thereby not shown in Fig. 10).
When considering lower SZAs (consistent with the TROPOMI overpass time of 13:00 local time), the general non-linear characteristic of these NOx curves holds as VOCR increases (Fig. 10). Higher VOCR relative to lower NOx concentration favors the oxidation of VOCs by OH and the associated minor loss pathway of NO + RO2 to form alkyl nitrates with a minor branching ratio over the competing major NOx loss pathway of NO2 + OH (Fig. 1). With rising VOCR, the NOx chemical tendency becomes more positive (P – L; Fig. S18a in the Supplement), and the net loss timescale elongates (e.g., ts_min from 2 to 4 h in Fig. 10). Moreover, NOx is required to reach a higher level to compete with the reactions involving VOCs, evident by the shift in the trough of the NOx curves (e.g., NOx_min from 1.4 to 3.7 ppb in Fig. 10). To put it in context, the NOx curves shown in Fig. 3 represent typical patterns, as long as VOCR remains below 10 s−1.
VOCR may also affect the Ox level and the NO2 : NOx ratio. The prescribed Ox level of 50 ppb (Sect. 2.2) overlooks the non-linear Ox variability related to VOCR (Murphy et al., 2007; Li et al., 2022). In NOx-limited scenarios, OH favors the oxidation of VOCs, and local-scale Ox is predominately produced by NO + RO2 or HO2, suggesting higher Ox levels with increased NOx concentrations. The omission of NO + RO2 or HO2 in Eqs. (3) could lead to an underestimation of the NO2 : NOx ratio, which likely explains the modeled tNO2 being consistently lower than observations over background regions. Conversely, under NOx-saturated conditions, the consumption of OH by NOx may limit the VOC oxidation and Ox production, leading to a decline in Ox level as the NOx concentration rises. Consequently, the NO2 : NOx ratio might be overestimated when the true Ox levels fall below 50 ppb (particularly under stagnant atmospheric mixing) or underestimated due to the absence of NO + RO2 reactions. Nevertheless, our predetermined Ox level of 50 ppb acts as a first-order limit to prevent unrealistic conversion from NO to NO2 at extremely high NOx levels when O3 is being titrated.
To address these limitations, one potential approach is to leverage formaldehyde concentrations retrieved from TROPOMI. Recent studies revisited the use of the formaldehyde : NO2 ratios (i.e., FNR) from satellites as a means of inferring O3 production rates (Goldberg et al., 2022; Souri et al., 2022). Our WRF-Chem simulations, which were used to parameterize the NOx chemical tendency, show that modeled formaldehyde generally increases with VOCR with varying slopes influenced by SZA and NOx concentrations (Fig. S18c), and O3 concentrations scale non-linearly with FNRs with O3 concentration, approaching a background value at a high FNR > 10 (Fig. S18d). Even though satellite-based FNRs may theoretically help probe the O3 or Ox concentration to better parameterize NO2 : NOx ratios, Souri et al. (2022) stressed that retrieval errors, especially from formaldehyde (40 % to 90 % with ≤ 50 % over cities) and inherent chemical errors in the predictive power of FNRs, may hinder the broad application of space-based FNRs at the current stage. Nonetheless, sensitivity analyses in Sect. 3 indicate an overall chemical uncertainty in tNO2 of about 10 % to 20 % with respect to NO2 signals, even if the perturbed Ox level is much lower than 50 ppb (Fig. 4).
5.3.2 Uncertainties in non-chemical processes
Besides the simplification of chemical reactions, modeled tNO2 values can be subject to a few physical processes and parameters, including emission profiles, inter-parcel mixing scales, and dry deposition.
The underlying STILT v2 (Fasoli et al., 2018) accounted for a gradual growth of the mixed layer height over the hyper-near-field area around emissions. Yet, by convolving the STILT footprint with NOx emissions, we assumed that emissions originate from the surface and are uniformly mixed over the mixed layer without considering the possible uneven distribution of emissions from different vertical levels. In reality, under stable atmospheric conditions, the stack heights or plume heights of emission sources can sometimes extend above the shallow PBL. Our current assumption may thus lead to an overestimation in modeled concentrations, and such biases can in turn affect the estimate of the NOx tendency. More importantly, changes in the vertical profile of emissions can lead to changes in concentration per model level, which affect the tropospheric columns, as the typical averaging kernel profile is far from uniform within the PBL. Recall that TROPOMI NO2 AKs decrease rapidly towards the surface (Fig. 1). Hence, placing an emission plume at the surface or an elevated altitude (e.g., 400 m) can cause a discrepancy in modeled column concentrations. In addition, if the wind shear is strong over an intensive point source (likely the Intermountain example in Fig. 5c), assumptions about the injection height and vertical profile of emission plumes may affect the modeled plume shape and possibly deviate the estimated near-field wind bias, following Sect. 5.2.1. Noticeably, Maier et al. (2022) investigated the influence of the inaccurate representation of emission profiles on the flask-like modeled concentrations by implementing a time-varying, sector-specific emission profile into STILT. Such an impact on column concentrations may be minimized but requires future in-depth investigations, particularly over point sources.
Accounting for inter-parcel mixing was an important aspect when developing Lagrangian chemical models. Omitting inter-parcel mixing makes solving for non-linear processes (such as chemical NOx loss) problematic. On the contrary, Eulerian models suffer from excessive numerical diffusion. Mixing that is too strong smooths the spatial gradient of concentration and can lower the concentration within the fixed model grids, which may cause slight shifts in the NOx regimes. Valin et al. (2011) suggested that a spatial resolution of 4 to 12 km is sufficient to capture the non-linearity in the NOx loss rate. As for Lagrangian models, efforts can be made to enable the flux exchange between air parcels via deformations (Konopka et al., 2019; McKenna et al., 2002). In addition to the inter-parcel mixing within the mixed layer (ML), several other turbulent mixing processes require future investigation, including (1) horizontal mixing in the free troposphere (FT), (2) vertical mixing between the ML and FT, and (3) mixing between tracked air parcels with the untracked surrounding background. For example, Real et al. (2008) utilized a linear relaxation, with an exponential decay of the plume concentrations towards the background based on a timescale of 2 d to address the third mixing process. The second mixing process requires future modifications involving the determination of entrainment zones and mixing hyperparameters for such ML–FT exchange.
The original STILT model realized vertical mixing by diluting the surface emissions across the ML height (Lin et al., 2003), and we further enabled an exchange in pollutants' concentrations with prescribed mixing length scales and timescales representing typical horizontal mixing rates (Sect. 2.3). As part of the final sensitivity tests, we simulated tNO2 based on a spectrum of mixing hyperparameters for the New Madrid power plant. Uncertainties in the mixing parameters result in minimal uncertainties in the sounding-specific modeled tNO2 values (Fig. S19 in the Supplement). For example, differences in modeled tNO2 between the mixing and non-mixing simulations become larger as mixing becomes faster and for receptors or soundings located on the edge of the plume (i.e., only a small fraction of the trajectories encountered power plant emission in Fig. S20 in the Supplement). Uncertainties in the prescribed mixing hyperparameters contribute even less to the modeled values over urban areas (i.e., < 10 % for Phoenix cases), where emissions are generally better mixed than at power plants. In addition, such a mixing influence can vary with the spatial resolution of the emission inventory used in the simulations.
The dry deposition of NO2 was not factored into this study, which could lead to an overestimation of modeled NO2. For future model implementations, it is possible to track the loss of NO2 concentrations due to dry deposition by calculating the dry deposition velocities (e.g., Wesely, 1988) when air parcels descend close to the surface, e.g., 50 m above the surface (Wen et al., 2012).
In developing STILT–NOx, we aim to quantify anthropogenic NOx emission signals for power plants and cities using remote sensors with a novel Lagrangian chemical system that preserves the non-linear relationship between NOx concentrations and emissions. This development is motivated by the desire to reduce computational costs by replacing the conventional kinetics-based approach to solve for concentration changes with a simplified parameterization relying on as few variables as possible. Such a simplified parameterization can be improved and adopted by other Lagrangian models. This work expands the capability of (X-)STILT in tracing the origins of chemically reactive species to simulate their concentrations at satellite soundings (Fig. 1). Although uncertainties exist in modeling atmospheric transport, mixing, and chemical processes, this study covers the key NOx chemical mechanisms, various error sources, model validation, and the benefit of using NOx to constrain CO2 emissions and tracer–tracer emission ratios.
To evaluate our modeling system, which consists of the HYSPLIT–STILT core and modules of column weighting, simplified chemistry, and error analyses (Fig. 1), we compared modeled tropospheric NO2 columns using EPA-reported emissions against observed columns from TROPOMI over three power plants in the US (Fig. 6). The largest model–data discrepancies are found for overpasses with substantial wind directional biases. Across three power plants and seasons, the systematic bias informed by the model–data regression slope appears to be small when using EPA emissions. Switching NOx emissions from prior emissions to EPA (usually with a scaling factor of 2 to 3) greatly improves the model–data agreement, followed by the impact of whether to turn on the NOx chemistry. Upgrading to a higher-resolution meteorological field minimally alleviated the model–data mismatches but should be considered for regions with complex terrain. Subtracting the background NO2 is necessary, especially over stagnant days and regions with strong non-anthropogenic emission influences. Based on our limited case studies, NO2 simulations of power plants are usually more challenging compared to urban areas with more of the areal source for several reasons (from atmospheric mixing, spatial heterogeneity, and vertical profiles of emissions to the exposure of ambient ozone-rich air when estimating the NO2 : NOx ratios).
Our comprehensive analyses on modeling tNO2 further shed light on the estimation of CO2 emissions at the local scale. Modeling two species in a consistent modeling framework makes the quantification of two key bias terms easier, namely from wind directions and emission locations. For example, we demonstrate the use of model–data NO2 plumes to obtain a quantitative value of the directional biases associated with the modeled wind (Sect. 5.2.1) and biases with the emission distribution in prior inventories like EDGARv6 (Sect. 4.2). As growing interest arose from the joint use of GHG and air pollutants, we also investigated the differences between NO2 : CO2 enhancement ratios and the ENOx : ECO2 emission ratios (Fig. 9). Such differences between the two tracer–tracer ratios vary across seasons and space and are again driven by the non-linearity between the emissions and concentrations. For instance, to be consistent with emission ratios at the sources, observation-based enhancement ratios need to be scaled up by 2 to 3 times in the summer months due to faster photochemistry. Soundings with a separation of 60 km from the site center need to be scaled up further by roughly 1.3 times more than near-field soundings when concerning changes in chemical tendency.
STILT–NOx, in conjunction with the forthcoming local-scale multi-tracer non-linear modeling and inversion system (Fig. 1), can be employed to simultaneously constrain emissions from multiple species of both GHGs and key air pollutants, along with their respective emission ratios, allowing for improvements in sectoral attributions. Such a framework has the potential to be scaled up to a large number of cities for estimating emissions of NOx, CO2, and possibly other tracers from space-based sensors.
We used meteorological fields from the Global Forecast System (0.25∘ × 0.25∘ GFS-FNL; NCEP, 2015) to generate hourly outputs at a grid spacing of 12 km for 5 consecutive days in each month of 2020. The first day is regarded as the spin-up time to stabilize the model whose concentration fields are excluded from the following analyses. Anthropogenic emissions of air pollutants and VOCs are adopted, respectively, from EDGARv6.1 (Monforti Ferrario et al., 2022) and EDGARv4.3.2 (Huang et al., 2017), with biogenic VOC emissions derived from the Model of Emissions of Gases and Aerosols from Nature (MEGANv2; Guenther et al., 2012). No lightning or soil NOx source is included in WRF-Chem simulations. The boundary condition of chemicals relies on the CAM-CHEM model (Buchholz et al., 2019). The most important part is the gas-phase photochemistry scheme, which is driven by the second generation of the Regional Acid Deposition Model (RADM2; Stockwell et al., 1990), with dry and wet depositions included. RADM2 is well suited under polluted environments but may miss several key aromatic components for pristine environments dominated by biogenic volatile organic compounds (BVOCs; Stockwell et al., 1997).
We assess the wind uncertainty associated with two meteorological fields that drive (X-)STILT, namely the 3 km HRRR and 0.25∘ GFS. The first approach targets regional wind error statistics by comparing modeled wind fields (both HRRR and GFS) against true wind observations at radiosonde stations. The wind error statistic is further used to translate wind errors to uncertainties in tNO2. Radiosonde balloons are normally launched at 00:00 or 12:00 UTC. Only u- and v-component wind observations for levels below 2 km over the 24 h ahead of the TROPOMI overpass time are selected. We then estimate the random uncertainties in the u-/v-component wind speed (i.e., RMSE in m s−1) and normalize the RMSEs over mean wind speed to yield fractional uncertainties (%) for every overpass (Fig. S3). For example, fractional wind uncertainties over Missouri (around 20 % to 40 %) are generally smaller than uncertainties over mountainous lands in Utah (> 40 %), which relates to the model's capabilities in resolving topography and topographic flows. In addition, HRRR-based winds at radiosonde stations appear to be more erroneous compared to GFS-based winds. High-resolution models provide better descriptions of the surface land cover type, terrain height, and surface roughness, which may improve the spatial variability in the PBLH (Lin et al., 2017) and wind vectors. Without true wind measurements, it remains unclear whether higher-resolution models can capture more accurate fine-scale meteorology. Nevertheless, the radiosonde analysis provides an overall picture of the regional wind uncertainty.
To propagate wind error statistics to transport uncertainty in concentrations, a wind error component is added to the mean wind component and the turbulence component when generating backward trajectories. Transport uncertainties in tNO2 are defined as the differences in variations in the parcel-specific NO2 mixing ratio with the proper vertical weighting of AK and pressure-weighting function (PWF) between the perturbed and the initial set of the trajectories.
As introduced in Sect. 5.2.1, a NO2 plume from either model or retrieval is rotated clockwise (α from −180 to −5∘, with a spacing of 5∘) or counter-clockwise (from 5 to 180∘) around the emission source and then resampled onto the original TROPOMI pixels (Fig. S16). We then multiply gridded tNO2 from the initial plume with gridded tNO2 from each rotated plume under each rotating angle, α. The cross-product of two tNO2 plumes (XP; ppb2) measures how two tNO2 plumes are similar in terms of their spatial structures, as one is rotated around its source location for 360∘ (Eq. C1):
We next calculate the square-root mean of these sounding-specific cross-products per rotating angle (Fig. 8a). The peak of the Gaussian shape suggests when two plume signals reach the maximum correlation, while the wing suggests when one plume signal starts to decouple with another plume signal. The plume that undergoes rotation () can either be a modeled plume with different model configurations (e.g., GFS or HRRR; with or without chemistry) or an observed plume. For example, root mean products based on the simulations without chemistry display a high bias compared to root mean products using observed tNO2, which implies that the entire modeled scene including the background signal is biased high when NOx lifetime is not included. When an observed plume was rotated to match its original self, then the tNO2 product can serve as a baseline. Normalizing the cross-products offers a diagnostic (Fig. 8), analogous to the concept of the cross-correlation coefficient.
TROPOMI is available at https://doi.org/10.5270/S5P-9bnp8q8 (ESA, 2021). OCO-3 Level 2 B10p4r XCO2 data are available at https://doi.org/10.5067/970BCC4DHH24 (OCO-2/OCO-3 Science Team et al., 2022). EDGARv6.1 emissions can be accessed from https://data.jrc.ec.europa.eu/dataset/df521e05-6a3b-461c-965a-b703fb62313e (Monforti Ferrario et al., 2022) and have been preprocessed. The STILT–NOx v1 model is built on previous efforts of the X-STILT model in modeling NO2. The exact version used in the paper is archived on Zenodo at https://doi.org/10.5281/zenodo.8057850 (Wu, 2023). The GFS and HRRR meteorological files are available from the READY website (https://www.ready.noaa.gov/archives.php, Rolph et al., 2017).
The supplement related to this article is available online at: https://doi.org/10.5194/gmd-16-6161-2023-supplement.
DW, POW, and JL designed the modeling experiments and contributed to the interpretation of results. DW realized the STILT–NOx model code and conducted WRF-Chem simulations. Specific insights from individuals were provided by POW, JLL, and PIP for NOx chemical parameterization; JLL for the WRF-Chem model setup; and JCL for the Lagrangian inter-parcel mixing. All authors contributed to writing and editing the paper.
The contact author has declared that none of the authors has any competing interests.
Publisher's note: Copernicus Publications remains neutral with regard to jurisdictional claims in published maps and institutional affiliations.
The computations presented here were conducted in the Resnick High-Performance Computing Center, a facility supported by the Resnick Sustainability Institute at the California Institute of Technology. We acknowledge the use of the WRF-Chem preprocessor tool of mozbc, which was provided by the Atmospheric Chemistry Observations and Modeling Lab (ACOM) of the National Center for Atmospheric Research (NCAR). The authors acknowledge the National Oceanic and Atmospheric Administration (NOAA) Air Re45 sources Laboratory (ARL) for the provision of the GFS and HRRR meteorological files used in this publication, which were downloaded from the READY website (http://www.ready.noaa.gov, last access: 1 May 2018). The first author extends their appreciation to Kazuyuki Miyazaki (JPL) for discussions on NOx modeling and Rob Nelson and Annmarie Eldering (JPL) for the OCO-3 data. The authors thank the two anonymous referees for their careful reading of our paper and for their constructive suggestions that have helped improve our study.
The analysis has been supported by the National Aeronautics and Space Administration (NASA; grant no. 80NSSC21K1064). A portion of the research was carried out at the Jet Propulsion Laboratory, California Institute of Technology, under a contract with NASA (grant no. 80NM0018D0004).
This paper was edited by Jason Williams and reviewed by two anonymous referees.
Beirle, S., Boersma, K. F., Platt, U., Lawrence, M. G., and Wagner, T.: Megacity emissions and lifetimes of nitrogen oxides probed from space, Science, 333, 1737–1739, https://doi.org/10.1126/science.1207824, 2011. a, b, c
Beirle, S., Borger, C., Dörner, S., Li, A., Hu, Z., Liu, F., Wang, Y., and Wagner, T.: Pinpointing nitrogen oxide emissions from space, Science Advances, 5, eaax9800, https://doi.org/10.1126/sciadv.aax9800, 2019. a
Beirle, S., Borger, C., Dörner, S., Eskes, H., Kumar, V., de Laat, A., and Wagner, T.: Catalog of NOx emissions from point sources as derived from the divergence of the NO2 flux for TROPOMI, Earth Syst. Sci. Data, 13, 2995–3012, https://doi.org/10.5194/essd-13-2995-2021, 2021. a
Brunner, D.: Atmospheric chemistry in lagrangian models – overview, in: Lagrangian Modeling of the Atmosphere, edited by: Lin, J. C., Brunner, D., Gerbig, C., Stohl, A., Luchar, A., and Webley, P., Geophysical Monograph Series, 200, https://doi.org/10.1029/2012GM001431, 2012. a
Buchholz, R., Emmons, L., and Tilmes, S.: The CESM2 Development Team: CESM2.1/CAM-chem Instantaneous Output for Boundary Conditions, UCAR/NCAR–Atmospheric Chemistry Observations and Modeling Laboratory, Subset used January 2020–December 2020, 2019. a
Cifuentes, L., Borja-Aburto, V. H., Gouveia, N., Thurston, G., and Davis, D. L.: Hidden health benefits of greenhouse gas mitigation, Science, 293, 1257–1259, https://doi.org/10.1126/science.1063357, 2001. a
Clapp, L. J. and Jenkin, M. E.: Analysis of the relationship between ambient levels of O3, NO2 and NO as a function of NOx in the UK, Atmos. Environ., 35, 6391–6405, 2001. a, b
Collins, W., Stevenson, D. S., Johnson, C., and Derwent, R.: Tropospheric ozone in a global-scale three-dimensional Lagrangian model and its response to NOx emission controls, J. Atmos. Chem., 26, 223–274, 1997. a
Demetillo, M. A. G., Navarro, A., Knowles, K. K., Fields, K. P., Geddes, J. A., Nowlan, C. R., Janz, S. J., Judd, L. M., Al-Saadi, J., Sun, K., McDonald, C. B., Diskin, S. G., and Pusede, E. S.: Observing nitrogen dioxide air pollution inequality using high-spatial-resolution remote sensing measurements in houston, Texas, Environ. Sci. Technol., 54, 9882–9895, 2020. a
Duncan, B. N., Lamsal, L. N., Thompson, A. M., Yoshida, Y., Lu, Z., Streets, D. G., Hurwitz, M. M., and Pickering, K. E.: A space-based, high-resolution view of notable changes in urban NOx pollution around the world (2005–2014), J. Geophys. Res.-Atmos., 121, 976–996, 2016. a
ESA: Copernicus Sentinel-5P, TROPOMI Level 2 Nitrogen Dioxide total column products, Version 02, European Space Agency [data set], https://doi.org/10.5270/S5P-9bnp8q8, 2021. a
Fasoli, B., Lin, J. C., Bowling, D. R., Mitchell, L., and Mendoza, D.: Simulating atmospheric tracer concentrations for spatially distributed receptors: updates to the Stochastic Time-Inverted Lagrangian Transport model's R interface (STILT-R version 2), Geosci. Model Dev., 11, 2813–2824, https://doi.org/10.5194/gmd-11-2813-2018, 2018. a, b, c
Fujita, E. M., Campbell, D. E., Stockwell, W. R., Saunders, E., Fitzgerald, R., and Perea, R.: Projected ozone trends and changes in the ozone-precursor relationship in the South Coast Air Basin in response to varying reductions of precursor emissions, J. Air Waste Manage., 66, 201–214, 2016. a
Goldberg, D. L., Lu, Z., Streets, D. G., de Foy, B., Griffin, D., McLinden, C. A., Lamsal, L. N., Krotkov, N. A., and Eskes, H.: Enhanced capabilities of TROPOMI NO2: estimating NOx from North American cities and power plants, Environ. Sci. Technol., 53, 12594–12601, 2019. a
Goldberg, D. L., Harkey, M., de Foy, B., Judd, L., Johnson, J., Yarwood, G., and Holloway, T.: Evaluating NOx emissions and their effect on O3 production in Texas using TROPOMI NO2 and HCHO, Atmos. Chem. Phys., 22, 10875–10900, https://doi.org/10.5194/acp-22-10875-2022, 2022. a, b, c, d, e, f
Grell, G. A., Peckham, S. E., Schmitz, R., McKeen, S. A., Frost, G., Skamarock, W. C., and Eder, B.: Fully coupled “online” chemistry within the WRF model, Atmos. Environ., 39, 6957–6975, 2005. a
Guenther, A. B., Jiang, X., Heald, C. L., Sakulyanontvittaya, T., Duhl, T., Emmons, L. K., and Wang, X.: The Model of Emissions of Gases and Aerosols from Nature version 2.1 (MEGAN2.1): an extended and updated framework for modeling biogenic emissions, Geosci. Model Dev., 5, 1471–1492, https://doi.org/10.5194/gmd-5-1471-2012, 2012. a
Hakkarainen, J., Ialongo, I., Oda, T., Szelag, M. E., O'Dell, C. W., Eldering, A., and Crisp, D.: Building a bridge: Characterizing major anthropogenic point sources in the South African Highveld region using OCO-3 carbon dioxide Snapshot Area Maps and Sentinel-5P/TROPOMI nitrogen dioxide columns, Environ. Res. Lett., 18, 035003, https://doi.org/10.1088/1748-9326/acb837, 2023. a, b, c
He, T.-L., Jones, D. B. A., Miyazaki, K., Bowman, K. W., Jiang, Z., Chen, X., Li, R., Zhang, Y., and Li, K.: Inverse modelling of Chinese NOx emissions using deep learning: integrating in situ observations with a satellite-based chemical reanalysis, Atmos. Chem. Phys., 22, 14059–14074, https://doi.org/10.5194/acp-22-14059-2022, 2022. a
Huang, G., Brook, R., Crippa, M., Janssens-Maenhout, G., Schieberle, C., Dore, C., Guizzardi, D., Muntean, M., Schaaf, E., and Friedrich, R.: Speciation of anthropogenic emissions of non-methane volatile organic compounds: a global gridded data set for 1970–2012, Atmos. Chem. Phys., 17, 7683–7701, https://doi.org/10.5194/acp-17-7683-2017, 2017. a
Huang, Y. and Seinfeld, J. H.: A Neural Network-Assisted Euler Integrator for Stiff Kinetics in Atmospheric Chemistry, Environ. Sci. Technol., 56, 4676–4685, 2022. a
Hurtt, G. C., Andrews, A., Bowman, K., Brown, M. E., Chatterjee, A., Escobar, V., Fatoyinbo, L., Griffith, P., Guy, M., Healey, S. P., Jacob, J. D., Kennedy, R., Lohrenz, S., McGroddy, E. M., Morales, V., Nehrhorn, T., Ott, L., Saatchi, S., Carlo, S. E., Serbin, P. S., and Tian H.: The NASA Carbon Monitoring System Phase 2 synthesis: scope, findings, gaps and recommended next steps, Environ. Res. Lett., 17, 063010, 2022. a
Jenkin, M. E.: Analysis of sources and partitioning of oxidant in the UK–Part 2: contributions of nitrogen dioxide emissions and background ozone at a kerbside location in London, Atmos. Environ., 38, 5131–5138, 2004. a
Jiang, Z., McDonald, B. C., Worden, H., Worden, J. R., Miyazaki, K., Qu, Z., Henze, D. K., Jones, D. B., Arellano, A. F., Fischer, E. V., Zhu, L., and Boersma, K. F.: Unexpected slowdown of US pollutant emission reduction in the past decade, P. Natl. Acad. Sci. USA, 115, 5099–5104, 2018. a
Jin, X., Fiore, A. M., Murray, L. T., Valin, L. C., Lamsal, L. N., Duncan, B., Folkert Boersma, K., De Smedt, I., Abad, G. G., Chance, K., and Tonnesen, G. G.: Evaluating a space-based indicator of surface ozone-NOx-VOC sensitivity over midlatitude source regions and application to decadal trends, J. Geophys. Res.-Atmos., 122, 10–439, 2017. a
Jin, X., Fiore, A., Boersma, K. F., Smedt, I. D., and Valin, L.: Inferring changes in summertime surface Ozone–NOx–VOC chemistry over US urban areas from two decades of satellite and ground-based observations, Environ. Sci. Technol., 54, 6518–6529, 2020. a
Kaminski, T., Scholze, M., Rayner, P., Houweling, S., Voßbeck, M., Silver, J., Lama, S., Buchwitz, M., Reuter, M., Knorr, W., Chen, W. H., Kuhlmann, G., Brunner, D., Dellaert, S., van der Gon, D. H., Super, I., Loscher, A., and Meijer, Y.: Assessing the Impact of Atmospheric CO2 and NO2 Measurements From Space on Estimating City-Scale Fossil Fuel CO2 Emissions in a Data Assimilation System, Frontiers in Remote Sensing, 3, 887456, https://doi.org/10.3389/frsen.2022.887456, 2022. a
Keller, C. A. and Evans, M. J.: Application of random forest regression to the calculation of gas-phase chemistry within the GEOS-Chem chemistry model v10, Geosci. Model Dev., 12, 1209–1225, https://doi.org/10.5194/gmd-12-1209-2019, 2019. a
Konopka, P., Tao, M., Ploeger, F., Diallo, M., and Riese, M.: Tropospheric mixing and parametrization of unresolved convective updrafts as implemented in the Chemical Lagrangian Model of the Stratosphere (CLaMS v2.0), Geosci. Model Dev., 12, 2441–2462, https://doi.org/10.5194/gmd-12-2441-2019, 2019. a, b, c
Kuhlmann, G., Broquet, G., Marshall, J., Clément, V., Löscher, A., Meijer, Y., and Brunner, D.: Detectability of CO2 emission plumes of cities and power plants with the Copernicus Anthropogenic CO2 Monitoring (CO2M) mission, Atmos. Meas. Tech., 12, 6695–6719, https://doi.org/10.5194/amt-12-6695-2019, 2019. a
Kuhlmann, G., Henne, S., Meijer, Y., and Brunner, D.: Quantifying CO2 emissions of power plants with CO2 and NO2 imaging satellites, Frontiers in Remote Sensing, 2, 14, https://doi.org/10.3389/frsen.2021.689838, 2021. a
Lama, S., Houweling, S., Boersma, K. F., Aben, I., Denier van der Gon, H. A. C., and Krol, M. C.: Estimation of OH in urban plumes using TROPOMI-inferred , Atmos. Chem. Phys., 22, 16053–16071, https://doi.org/10.5194/acp-22-16053-2022, 2022. a
Lamsal, L., Martin, R., Padmanabhan, A., Van Donkelaar, A., Zhang, Q., Sioris, C., Chance, K., Kurosu, T., and Newchurch, M.: Application of satellite observations for timely updates to global anthropogenic NOx emission inventories, Geophys. Res. Lett., 38, L05810, https://doi.org/10.1029/2010GL046476, 2011. a
Laughner, J. L. and Cohen, R. C.: Direct observation of changing NOx lifetime in North American cities, Science, 366, 723–727, 2019. a, b, c, d
Lee, H.-J., Kim, S.-W., Brioude, J., Cooper, O., Frost, G., Kim, C.-H., Park, R., Trainer, M., and Woo, J.-H.: Transport of NOx in East Asia identified by satellite and in situ measurements and Lagrangian particle dispersion model simulations, J. Geophys. Res.-Atmos., 119, 2574–2596, 2014. a
Lee, J. D., Drysdale, W. S., Finch, D. P., Wilde, S. E., and Palmer, P. I.: UK surface NO2 levels dropped by 42 % during the COVID-19 lockdown: impact on surface O3, Atmos. Chem. Phys., 20, 15743–15759, https://doi.org/10.5194/acp-20-15743-2020, 2020. a
Li, C., Zhu, Q., Jin, X., and Cohen, R. C.: Elucidating Contributions of Anthropogenic Volatile Organic Compounds and Particulate Matter to Ozone Trends over China, Environ. Sci. Technol., 56, 12906–12916, 2022. a
Lin, J. C. and Gerbig, C.: Accounting for the effect of transport errors on tracer inversions, Geophys. Res. Lett., 32, L01802, https://doi.org/10.1029/2004GL021127, 2005. a, b
Lin, J. C., Gerbig, C., Wofsy, S., Andrews, A., Daube, B., Davis, K., and Grainger, C.: A near-field tool for simulating the upstream influence of atmospheric observations: The Stochastic Time-Inverted Lagrangian Transport (STILT) model, J. Geophys. Res.-Atmos., 108, 4493, https://doi.org/10.1029/2002JD003161, 2003. a, b, c, d, e
Lin, J. C., Brunner, D., Gerbig, C., Stohl, A., Luhar, A., and Webley, P.: Lagrangian modeling of the atmosphere, John Wiley & Sons, AGU Geophysical Monograph, ISBN 978-0-875-90490-0, 2013. a, b
Lin, J. C., Mallia, D. V., Wu, D., and Stephens, B. B.: How can mountaintop CO2 observations be used to constrain regional carbon fluxes?, Atmos. Chem. Phys., 17, 5561–5581, https://doi.org/10.5194/acp-17-5561-2017, 2017. a, b
Lin, J. C., Mitchell, L., Crosman, E., Mendoza, D. L., Buchert, M., Bares, R., Fasoli, B., Bowling, D. R., Pataki, D., Catharine, D., Strong, C., Gurney, R. K., Patarasuk, R., Baasandorj, M., Jacques, A., Hoch, S., Horel, J., and Ehleringer, J.: CO2 and carbon emissions from cities: Linkages to air quality, socioeconomic activity, and stakeholders in the Salt Lake City urban area, B. Am. Meteorol. Soc., 99, 2325–2339, 2018. a
Liu, F., Tao, Z., Beirle, S., Joiner, J., Yoshida, Y., Smith, S. J., Knowland, K. E., and Wagner, T.: A new method for inferring city emissions and lifetimes of nitrogen oxides from high-resolution nitrogen dioxide observations: a model study, Atmos. Chem. Phys., 22, 1333–1349, https://doi.org/10.5194/acp-22-1333-2022, 2022. a
Liu, X., Mizzi, A. P., Anderson, J. L., Fung, I. Y., and Cohen, R. C.: Assimilation of satellite NO2 observations at high spatial resolution using OSSEs, Atmos. Chem. Phys., 17, 7067–7081, https://doi.org/10.5194/acp-17-7067-2017, 2017. a
Loughner, C. P., Fasoli, B., Stein, A. F., and Lin, J. C.: Incorporating features from the stochastic time-inverted lagrangian transport (STILT) model into the Hybrid Single-Particle Lagrangian Integrated Trajectory (HYSPLIT) model: a unified dispersion model for time-forward and time-reversed applications, J. Appl. Meteorol. Clim., 60, 799–810, 2021. a
MacDonald, C. G., Mastrogiacomo, J.-P., Laughner, J. L., Hedelius, J. K., Nassar, R., and Wunch, D.: Estimating enhancement ratios of nitrogen dioxide, carbon monoxide and carbon dioxide using satellite observations, Atmos. Chem. Phys., 23, 3493–3516, https://doi.org/10.5194/acp-23-3493-2023, 2023. a, b
Maier, F., Gerbig, C., Levin, I., Super, I., Marshall, J., and Hammer, S.: Effects of point source emission heights in WRF–STILT: a step towards exploiting nocturnal observations in models, Geosci. Model Dev., 15, 5391–5406, https://doi.org/10.5194/gmd-15-5391-2022, 2022. a
McKenna, D. S., Konopka, P., Grooß, J.-U., Günther, G., Müller, R., Spang, R., Offermann, D., and Orsolini, Y.: A new Chemical Lagrangian Model of the Stratosphere (CLaMS) 1. Formulation of advection and mixing, J. Geophys. Res.-Atmos., 107, ACH 15-1–ACH 15-15, https://doi.org/10.1029/2000JD000114, 2002. a, b
Miyazaki, K. and Bowman, K.: Predictability of fossil fuel CO2 from air quality emissions, Nat. Commun., 14, 1604, https://doi.org/10.1038/s41467-023-37264-8, 2023. a
Miyazaki, K., Bowman, K., Sekiya, T., Eskes, H., Boersma, F., Worden, H., Livesey, N., Payne, V. H., Sudo, K., Kanaya, Y., Takigawa, M., and Ogochi, K.: Updated tropospheric chemistry reanalysis and emission estimates, TCR-2, for 2005–2018, Earth Syst. Sci. Data, 12, 2223–2259, https://doi.org/10.5194/essd-12-2223-2020, 2020. a, b
Monforti Ferrario, F., Crippa, M., Guizzardi, D.; Muntean, M., Schaaf, E., Banja, M., Pagani, F., Solazzo, E.: EDGAR v6.1 global air pollutant emissions, Publisher: European Commission, Joint Research Centre (JRC) [data set], https://data.jrc.ec.europa.eu/dataset/df521e05-6a3b-461c-965a-b703fb62313e (last access: 19 August 2023), 2022. a, b, c
Murphy, J. G., Day, D. A., Cleary, P. A., Wooldridge, P. J., Millet, D. B., Goldstein, A. H., and Cohen, R. C.: The weekend effect within and downwind of Sacramento – Part 1: Observations of ozone, nitrogen oxides, and VOC reactivity, Atmos. Chem. Phys., 7, 5327–5339, https://doi.org/10.5194/acp-7-5327-2007, 2007. a
Myhre, G., Shindell, D., Bréon, F.-M., Collins, W., Fuglestvedt, J., Huang, J., Koch, D., Lamarque, J.-F., Lee, D., Mendoza, B., Nakajima, T., Robock, A., Stephens, G., Takemura, T., and Zhang, H.: Anthropogenic and Natural Radiative Forcing, in: Climate Change 2013: The Physical Science Basis. Contribution of Working Group I to the Fifth Assessment Report of the Intergovernmental Panel on Climate Change, edited by: Stocker, T. F., Qin, D., Plattner, G.-K., Tignor, M., Allen, S. K., Boschung, J., Nauels, A., Xia, Y., Bex, V., and Midgley, P. M., Cambridge University Press, Cambridge, United Kingdom and New York, NY, USA., 2013. a
Nassar, R., Moeini, O., Mastrogiacomo, J.-P., O'Dell, C. W., Nelson, R. R., Kiel, M., Chatterjee, A., Eldering, A., and Crisp, D.: Tracking CO2 emission reductions from space: A case study at Europe's largest fossil fuel power plant, Frontiers in Remote Sensing, 3, 98, https://doi.org/10.3389/frsen.2022.1028240, 2022. a
NCEP: NCEP GDAS/FNL 0.25 Degree Global Tropospheric Analyses and Forecast Grids, NCEP [data set], https://doi.org/10.5065/D65Q4T4Z, 2015. a
OCO-2/OCO-3 Science Team, Chatterjee, A., and Payne, V.: OCO-3 Level 2 bias-corrected XCO2 and other select fields from the full-physics retrieval aggregated as daily files, Retrospective processing v10.4r, Greenbelt, MD, USA, Goddard Earth Sciences Data and Information Services Center (GES DISC) [data set], https://doi.org/10.5067/970BCC4DHH24, 2022. a
Parker, H., Hasheminassab, S., Crounse, J., Roehl, C., and Wennberg, P.: Impacts of traffic reductions associated with COVID-19 on southern California air quality, Geophy. Res. Lett., 47, e2020GL090164, https://doi.org/10.1029/2020GL090164, 2020. a
Pugh, T. A. M., Cain, M., Methven, J., Wild, O., Arnold, S. R., Real, E., Law, K. S., Emmerson, K. M., Owen, S. M., Pyle, J. A., Hewitt, C. N., and MacKenzie, A. R.: A Lagrangian model of air-mass photochemistry and mixing using a trajectory ensemble: the Cambridge Tropospheric Trajectory model of Chemistry And Transport (CiTTyCAT) version 4.2, Geosci. Model Dev., 5, 193–221, https://doi.org/10.5194/gmd-5-193-2012, 2012. a
Qu, Z., Henze, D. K., Worden, H. M., Jiang, Z., Gaubert, B., Theys, N., and Wang, W.: Sector-based top-down estimates of NOx, SO2, and CO emissions in East Asia, Geophys. Res. Lett., 49, e2021GL096009, https://doi.org/10.1029/2021GL096009, 2022. a, b
Real, E., Law, K. S., Schlager, H., Roiger, A., Huntrieser, H., Methven, J., Cain, M., Holloway, J., Neuman, J. A., Ryerson, T., Flocke, F., de Gouw, J., Atlas, E., Donnelly, S., and Parrish, D.: Lagrangian analysis of low altitude anthropogenic plume processing across the North Atlantic, Atmos. Chem. Phys., 8, 7737–7754, https://doi.org/10.5194/acp-8-7737-2008, 2008. a
Reuter, M., Buchwitz, M., Hilboll, A., Richter, A., Schneising, O., Hilker, M., Heymann, J., Bovensmann, H., and Burrows, J.: Decreasing emissions of NOx relative to CO2 in East Asia inferred from satellite observations, Nat. Geosci., 7, 792–795, 2014. a, b, c
Rohrer, F. and Berresheim, H.: Strong correlation between levels of tropospheric hydroxyl radicals and solar ultraviolet radiation, Nature, 442, 184–187, 2006. a
Rolph, G., Stein, A., and Stunder, B.: Real-time environmental applications and display system: READY, Environ. Model. Softw., 95, 210–228, https://doi.org/10.1016/j.envsoft.2017.06.025, 2017 (data available at: https://www.ready.noaa.gov/archives.php, last access: 19 August 2023). a, b
Roten, D., Lin, J. C., Kunik, L., Mallia, D., Wu, D., Oda, T., and Kort, E. A.: The Information Content of Dense Carbon Dioxide Measurements from Space: A High-Resolution Inversion Approach with Synthetic Data from the OCO-3 Instrument, Atmos. Chem. Phys. Discuss. [preprint], https://doi.org/10.5194/acp-2022-315, in review, 2022. a
Shah, V., Jacob, D. J., Dang, R., Lamsal, L. N., Strode, S. A., Steenrod, S. D., Boersma, K. F., Eastham, S. D., Fritz, T. M., Thompson, C., Peischl, J., Bourgeois, I., Pollack, I. B., Nault, B. A., Cohen, R. C., Campuzano-Jost, P., Jimenez, J. L., Andersen, S. T., Carpenter, L. J., Sherwen, T., and Evans, M. J.: Nitrogen oxides in the free troposphere: implications for tropospheric oxidants and the interpretation of satellite NO2 measurements, Atmos. Chem. Phys., 23, 1227–1257, https://doi.org/10.5194/acp-23-1227-2023, 2023. a
Silva, S. J. and Arellano, A.: Characterizing regional-scale combustion using satellite retrievals of CO, NO2 and CO2, Remote Sens.-Basel, 9, 744, https://doi.org/10.3390/rs9070744, 2017. a
Souri, A. H., Johnson, M. S., Wolfe, G. M., Crawford, J. H., Fried, A., Wisthaler, A., Brune, W. H., Blake, D. R., Weinheimer, A. J., Verhoelst, T., Compernolle, S., Pinardi, G., Vigouroux, C., Langerock, B., Choi, S., Lamsal, L., Zhu, L., Sun, S., Cohen, R. C., Min, K.-E., Cho, C., Philip, S., Liu, X., and Chance, K.: Characterization of errors in satellite-based tropospheric column ratios with respect to chemistry, column-to-PBL translation, spatial representation, and retrieval uncertainties, Atmos. Chem. Phys., 23, 1963–1986, https://doi.org/10.5194/acp-23-1963-2023, 2023. a, b, c
Stein, A. F., Lamb, D., and Draxler, R. R.: Incorporation of detailed chemistry into a three-dimensional Lagrangian–Eulerian hybrid model: Application to regional tropospheric ozone, Atmos. Environ., 34, 4361–4372, 2000. a
Stein, A. F., Draxler, R. R., Rolph, G. D., Stunder, B. J., Cohen, M., and Ngan, F.: NOAA's HYSPLIT atmospheric transport and dispersion modeling system, B. Am. Meteorol. Soc., 96, 2059–2077, 2015. a
Stockwell, W. R., Middleton, P., Chang, J. S., and Tang, X.: The second generation regional acid deposition model chemical mechanism for regional air quality modeling, J. Geophys. Res.-Atmos., 95, 16343–16367, 1990. a, b
Stockwell, W. R., Kirchner, F., Kuhn, M., and Seefeld, S.: A new mechanism for regional atmospheric chemistry modeling, J. Geophys. Res.-Atmos., 102, 25847–25879, 1997. a
Strong, J., Whyatt, J., Hewitt, C., and Derwent, R.: Development and application of a Lagrangian model to determine the origins of ozone episodes in the UK, Atmos. Environ., 44, 631–641, 2010. a, b
Tang, W., Arellano, A. F., Gaubert, B., Miyazaki, K., and Worden, H. M.: Satellite data reveal a common combustion emission pathway for major cities in China, Atmos. Chem. Phys., 19, 4269–4288, https://doi.org/10.5194/acp-19-4269-2019, 2019. a
United States Environmental Protection Agency: Power Sector Emissions Data, Office of Atmospheric Protection, Clean Air Markets Division, Washington, DC, available from EPA's Air Markets Program Data web site: https://campd.epa.gov (last access: 19 August 2023), 2022. a
Valin, L. C., Russell, A. R., Hudman, R. C., and Cohen, R. C.: Effects of model resolution on the interpretation of satellite NO2 observations, Atmos. Chem. Phys., 11, 11647–11655, https://doi.org/10.5194/acp-11-11647-2011, 2011. a, b, c
Valin, L., Russell, A., and Cohen, R.: Variations of OH radical in an urban plume inferred from NO2 column measurements, Geophys. Res. Lett., 40, 1856–1860, 2013. a
van Geffen, J., Eskes, H., Compernolle, S., Pinardi, G., Verhoelst, T., Lambert, J.-C., Sneep, M., ter Linden, M., Ludewig, A., Boersma, K. F., and Veefkind, J. P.: Sentinel-5P TROPOMI NO2 retrieval: impact of version v2.2 improvements and comparisons with OMI and ground-based data, Atmos. Meas. Tech., 15, 2037–2060, https://doi.org/10.5194/amt-15-2037-2022, 2022. a, b
Watts, N., Amann, M., Arnell, N., Ayeb-Karlsson, S., Beagley, J., Belesova, K., Boykoff, M., Byass, P., Cai, W., Campbell- Lendrum, D., Capstick, S., Chambers, J., Coleman, S., Dalin, C., Daly, M., Dasandi, N., Dasgupta, S., Davies, M., Di Napoli, C., Dominguez-Salas, P., Drummond, P., Dubrow, R., Ebi, L. K., Eckelman, M., Ekins, P., Escobar, E. L., Georgeson, L., Golder, S., Grace, D., Graham, H., Haggar, P., Hamilton, I., Hartinger, S., Hess, J., Hsu, S., Hughes, N., Jankin Mikhaylov, S., Jimenez, P. M., Kelman, I., Kennard, H., Kiesewetter, G., Kinney, L. P., Kjellstrom, T., Kniveton, D., Lampard, P., Lemke, B., Liu, Y., Liu, Z., Lott, M., Lowe, R., Martinez-Urtaza, J., Maslin, M., McAllister, L., McGushin, A., McMichael, C., Milner, J., Moradi-Lakeh, M., Morrissey, K., Munzert, S., Murray, A. K., Neville, T., Nilsson, M., Odhiambo Sewe, M., Oreszczyn, T., Otto, M., Owfi, F., Pearman, O., Pencheon, D., Quinn, R., Rabbaniha, M., Robinson, E., Rocklov, J., Romanello, M., Semenza, C, J., Sherman, J., Shi, L., Springmann, M., Tabatabaei, M., Taylor, J., Trinanes, J., Shumake-Guillemot, J., Vu, B., Wilkinson, P., Winning, M., Gong P., Montgomery, H., and Costello, A.: The 2020 report of the Lancet Countdown on health and climate change: responding to converging crises, Lancet, 397, 129–170, 2021. a
Wen, D., Lin, J. C., Millet, D. B., Stein, A. F., and Draxler, R. R.: A backward-time stochastic Lagrangian air quality model, Atmos. Environ., 54, 373–386, 2012. a, b, c
Wesely, M. L.: Parameterization of surface resistances to gaseous dry deposition in regional-scale numerical models, Atmos Environ., 23, 1293–1304, https://doi.org/10.1016/0004-6981(89)90153-4, 1988. a
West, J. J., Smith, S. J., Silva, R. A., Naik, V., Zhang, Y., Adelman, Z., Fry, M. M., Anenberg, S., Horowitz, L. W., and Lamarque, J.-F.: Co-benefits of mitigating global greenhouse gas emissions for future air quality and human health, Nat. Clim. Change, 3, 885–889, 2013. a
Wohltmann, I. and Rex, M.: The Lagrangian chemistry and transport model ATLAS: validation of advective transport and mixing, Geosci. Model Dev., 2, 153–173, https://doi.org/10.5194/gmd-2-153-2009, 2009. a, b, c
Wu, D.: STILT-NOx v1 (for GMD submission) (v1.6), Zenodo [code], https://doi.org/10.5281/zenodo.8057850, 2023. a
Wu, D., Lin, J. C., Fasoli, B., Oda, T., Ye, X., Lauvaux, T., Yang, E. G., and Kort, E. A.: A Lagrangian approach towards extracting signals of urban CO2 emissions from satellite observations of atmospheric column CO2 (XCO2): X-Stochastic Time-Inverted Lagrangian Transport model (“X-STILT v1”), Geosci. Model Dev., 11, 4843–4871, https://doi.org/10.5194/gmd-11-4843-2018, 2018. a, b, c, d, e, f, g
Wu, D., Liu, J., Wennberg, P. O., Palmer, P. I., Nelson, R. R., Kiel, M., and Eldering, A.: Towards sector-based attribution using intra-city variations in satellite-based emission ratios between CO2 and CO, Atmos. Chem. Phys., 22, 14547–14570, https://doi.org/10.5194/acp-22-14547-2022, 2022. a, b
Wunch, D., Wennberg, P., Toon, G., Keppel-Aleks, G., and Yavin, Y.: Emissions of greenhouse gases from a North American megacity, Geophy. Res. Lett., 36, L15810, https://doi.org/10.1029/2009GL039825, 2009. a
Yang, E. G., Kort, E. A., Ott, L. E., Oda, T., and Lin, J. C.: Using Space-Based CO2 and NO2 Observations to Estimate Urban CO2 Emissions, J. Geophys. Res.-Atmos., 128, e2022JD037736, https://doi.org/10.1029/2022JD037736, 2023. a
Zhang, Q., Boersma, K. F., Zhao, B., Eskes, H., Chen, C., Zheng, H., and Zhang, X.: Quantifying daily NOx and CO2 emissions from Wuhan using satellite observations from TROPOMI and OCO-2, Atmos. Chem. Phys., 23, 551–563, https://doi.org/10.5194/acp-23-551-2023, 2023. a
Zheng, B., Geng, G., Ciais, P., Davis, S. J., Martin, R. V., Meng, J., Wu, N., Chevallier, F., Broquet, G., Boersma, F., van der A, R., Lin, J., Guan, G., Lei, Y., He, K., and Zhang Q.: Satellite-based estimates of decline and rebound in China's CO2 emissions during COVID-19 pandemic, Science Advances, 6, eabd4998, https://doi.org/10.1126/sciadv.abd4998, 2020. a
Zhu, Q., Laughner, J. L., and Cohen, R. C.: Combining Machine Learning and Satellite Observations to Predict Spatial and Temporal Variation of near Surface OH in North American Cities, Environ. Sci. Technol., 56, 7362–7371, https://doi.org/10.1021/acs.est.1c05636, 2022. a
- Abstract
- Introduction
- STILT–NOx model descriptions
- Model uncertainty in tNO2 due to wind and chemistry
- Model–data evaluations and comparisons
- Discussion
- Summary
- Appendix A: WRF-Chem setups
- Appendix B: Technical notes on regional wind assessment
- Appendix C: Technical notes on near-field wind bias quantification
- Code and data availability
- Author contributions
- Competing interests
- Disclaimer
- Acknowledgements
- Financial support
- Review statement
- References
- Supplement
- Abstract
- Introduction
- STILT–NOx model descriptions
- Model uncertainty in tNO2 due to wind and chemistry
- Model–data evaluations and comparisons
- Discussion
- Summary
- Appendix A: WRF-Chem setups
- Appendix B: Technical notes on regional wind assessment
- Appendix C: Technical notes on near-field wind bias quantification
- Code and data availability
- Author contributions
- Competing interests
- Disclaimer
- Acknowledgements
- Financial support
- Review statement
- References
- Supplement