the Creative Commons Attribution 4.0 License.
the Creative Commons Attribution 4.0 License.
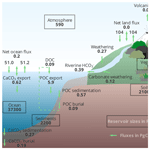
The Earth system model CLIMBER-X v1.0 – Part 2: The global carbon cycle
Tatiana Ilyina
Christoph Heinze
Mahé Perrette
Malte Heinemann
Daniela Dalmonech
Victor Brovkin
Guy Munhoven
Janine Börker
Jens Hartmann
Gibran Romero-Mujalli
Andrey Ganopolski
The carbon cycle component of the newly developed Earth system model of intermediate complexity CLIMBER-X is presented. The model represents the cycling of carbon through the atmosphere, vegetation, soils, seawater and marine sediments. Exchanges of carbon with geological reservoirs occur through sediment burial, rock weathering and volcanic degassing. The state-of-the-art HAMOCC6 model is employed to simulate ocean biogeochemistry and marine sediment processes. The land model PALADYN simulates the processes related to vegetation and soil carbon dynamics, including permafrost and peatlands. The dust cycle in the model allows for an interactive determination of the input of the micro-nutrient iron into the ocean. A rock weathering scheme is implemented in the model, with the weathering rate depending on lithology, runoff and soil temperature. CLIMBER-X includes a simple representation of the methane cycle, with explicitly modelled natural emissions from land and the assumption of a constant residence time of CH4 in the atmosphere. Carbon isotopes 13C and 14C are tracked through all model compartments and provide a useful diagnostic for model–data comparison.
A comprehensive evaluation of the model performance for the present day and the historical period shows that CLIMBER-X is capable of realistically reproducing the historical evolution of atmospheric CO2 and CH4 but also the spatial distribution of carbon on land and the 3D structure of biogeochemical ocean tracers. The analysis of model performance is complemented by an assessment of carbon cycle feedbacks and model sensitivities compared to state-of-the-art Coupled Model Intercomparison Project Phase 6 (CMIP6) models.
Enabling an interactive carbon cycle in CLIMBER-X results in a relatively minor slow-down of model computational performance by ∼ 20 % compared to a throughput of ∼ 10 000 simulation years per day on a single node with 16 CPUs on a high-performance computer in a climate-only model set-up. CLIMBER-X is therefore well suited to investigating the feedbacks between climate and the carbon cycle on temporal scales ranging from decades to >100 000 years.
- Article
(15044 KB) - Full-text XML
- Companion paper
- BibTeX
- EndNote
Atmospheric CO2 exerts a profound control on the state of the Earth system. Although it is present only in tiny concentrations in the present-day atmosphere, by absorbing radiation in the longwave spectral range it has a substantial effect on the energy balance of the Earth. In the present-day atmosphere, CO2 is the second most important greenhouse gas after water vapour. CO2 is also a fundamental molecule for life on Earth, as it serves as “food” in the photosynthesis process. The atmospheric CO2 concentration is hence a main control on the growth rate of plants on land.
From ice core data it is well known that atmospheric CO2 concentrations showed pronounced variations over the last million years (e.g. Petit et al., 1999; Augustin et al., 2004) that played an important role for the climate evolution over the Pleistocene (last ∼ 2.6 million years) by amplifying the variations associated with glacial–interglacial cycles (e.g. Ganopolski and Calov, 2011; Abe-Ouchi et al., 2013). Furthermore, on even longer timescales, a secular decrease in CO2 is thought to have been the main driver of the gradual cooling over the Cenozoic (last 66 million years) (e.g. Raymo and Ruddiman, 1992).
Over the last few centuries, human activities have strongly disrupted the natural CO2 balance by directly emitting CO2 from fossil sources into the atmosphere. The resulting increase in atmospheric CO2 has been the main factor for the observed rapid climate warming since the pre-industrial period (e.g. Gulev et al., 2021).
Modelling the atmospheric CO2 concentration is thus fundamental both for understanding past climate changes and for predicting the future evolution of the Earth system under different anthropogenic emission scenarios. However, it is far from trivial, because atmospheric CO2 is the result of complex biogeochemical processes on land, in the ocean, in marine sediments and in the lithosphere. Additionally, because of the long timescales involved in some of the carbon cycle processes, the interactive simulation of atmospheric CO2 has been, and still is, a challenge for state-of-the-art Earth system models. Fast Earth system models of intermediate complexity have therefore been extensively employed for investigating carbon cycle–climate feedbacks, e.g. Bern3D (Müller et al., 2008; Tschumi et al., 2011; Stocker et al., 2013), cGENIE (Ridgwell et al., 2007; Cao et al., 2009), CLIMBER-2 (Brovkin et al., 2002, 2007, 2012), iLOVECLIM (Bouttes et al., 2015), LOVECLIM (Goosse et al., 2010) and Uvic (Eby et al., 2009; Zickfeld et al., 2011; Mengis et al., 2020). Among these, CLIMBER-2 has successfully reproduced glacial–interglacial variations in CO2 (Ganopolski and Brovkin, 2017; Willeit et al., 2019), but some of the processes involved remain uncertain. CLIMBER-X builds on the past experience in modelling the global carbon cycle with CLIMBER-2 but adds an improved and more detailed representation of carbon cycle processes both on land and in the ocean. Improvements include a generally higher spatial resolution, a 3D ocean model, a state-of-the-art ocean biogeochemistry and marine sediment model, a more comprehensive description of vegetation and soil carbon processes, including permafrost and peatlands, and a new chemical weathering scheme.
In the following, the biogeochemistry components of CLIMBER-X are presented. The climate core of CLIMBER-X is described in detail in Willeit et al. (2022).
CLIMBER-X represents the cycling of carbon through the atmosphere, vegetation, soils, seawater and marine sediments. Through sediment burial, chemical weathering of rocks and volcanic degassing, carbon is also exchanged with geological reservoirs. A schematic illustration of the carbon cycle in the model is shown in Fig. 1. The carbon cycle component of CLIMBER-X consists of the ocean biogeochemistry and marine sediment models from HAMOCC6 (Maier-Reimer and Hasselmann, 1987; Ilyina et al., 2013; Heinze et al., 1999; Mauritsen et al., 2019) and the land model PALADYN (Willeit and Ganopolski, 2016), which includes dynamic vegetation, a soil carbon model and the weathering model of Hartmann (2009) and Börker et al. (2020). The atmospheric CO2 concentration is determined interactively by the exchange of carbon between the atmosphere, seawater, land and lithosphere. The model includes a representation of the dust cycle, with simulated dust deposition determining the input of the micro-nutrient iron into the ocean. CLIMBER-X also includes a simple representation of the methane cycle, with explicitly modelled natural emissions from land and the assumption of a constant residence time of CH4 in the atmosphere. The model is enabled with the carbon isotopes 13C and 14C, which are tracked through all model compartments.
The different model components are described in more detail in the following sections.
2.1 Ocean biogeochemistry and marine sediments: HAMOCC
HAMOCC (Maier-Reimer and Hasselmann, 1987; Maier-Reimer et al., 1993; Ilyina et al., 2013) is a state-of-the-art ocean biogeochemistry model, which is part of the MPI-ESM, the Earth system model of the Max Planck Institute for Meteorology (MPI). The latest version (Mauritsen et al., 2019), which is the version employed by the MPI in the Coupled Model Intercomparison Project Phase 6 (CMIP6), has been the starting point for the implementation of the model in CLIMBER-X. As a first step, the original HAMOCC6 code has been adapted to the CLIMBER-X structure. Notably, for easier parallelization, it has been transformed from a 3D model into a 1D vertical column model in which each water column is independent of the others. This is possible because the biogeochemical processes in the model are restricted to local vertical interactions. The different columns interact only through horizontal advection by ocean currents, which takes place in the ocean model.
HAMOCC represents the biogeochemical processes in the water column, in the sediments and at the air–sea interface. Marine biology dynamics are based on an extended NPZD (nutrients, phytoplankton, zooplankton and detritus) approach (Six and Maier-Reimer, 1996). The carbonate chemistry in the model follows the latest OMIP protocol (Orr et al., 2017), which uses the robust and safe pH calculation routines from SolveSAPHE-r1 (Munhoven, 2013). In the water column, the following biogeochemical tracers are simulated: dissolved inorganic carbon (DIC), total alkalinity (TA), phosphate (PO4), nitrate (NO3), nitrous oxide (N2O), dissolved nitrogen gas (N2), silicate (SiO2), dissolved bioavailable iron (Fe), dissolved oxygen (O2), phytoplankton (Phy), zooplankton (Zoo), dissolved organic matter (DOC), particulate organic matter (POC), opal shells, calcium carbonate shells (CaCO3), terrigenous material (dust) and hydrogen sulfide (H2S). The composition of organic material follows a constant Redfield ratio () after Takahashi et al. (1985) and for the micro-nutrient iron ().
The marine sediment module, which is part of HAMOCC, is based on Heinze et al. (1999). It essentially simulates the same processes between dissolved tracers (DIC, TA, PO4, NO3, O2, Fe, SiO2, H2S and N2) in porewater and solid sediment constituents (POC, opal, CaCO3 and dust) as in the water column. Porewater tracers are exchanged with the overlying water column via diffusion. Sedimentation fluxes of POC, CaCO3, opal and dust are added to the solid components of the sediment. Accumulation of solid sediment material will lead to active sediment layer content being shifted to the burial layer and back if boundary condition changes lead to chemical erosion of previously buried sediment.
Next we describe the changes introduced into HAMOCC as part of its implementation in CLIMBER-X.
N2 fixation is represented by a diagnostic formulation, whereby the nitrate influx into the surface layer is a function of the nitrate deficit relative to phosphate, multiplied by a constant fixation rate (Ilyina et al., 2013). Prognostic N2 fixers have recently been included in HAMOCC (Paulsen et al., 2017), based on the physiological characteristics of the cyanobacterium Trichodesmium. However, for simplicity and because uncertainties in nitrogen fixation remain large (e.g. Zehr and Capone, 2020), in CLIMBER-X cyanobacteria are disabled by default.
Following Heinemann et al. (2019), we have implemented a representation of aggregates in the model. Particulate organic carbon is assumed to form aggregates with the denser calcite and opal built during phytoplankton and zooplankton growth and with dust particles. The sinking speed of these aggregates depends on their excess density (Gehlen et al., 2006; Heinemann et al., 2019). Note that this approach neglects the effects of e.g. aggregate size distribution and porosity on the sinking speed (Maerz et al., 2020), and it does not, like other numerically more expensive schemes (e.g. Kriest and Evans, 2000), explicitly resolve the biological and physical aggregation and disaggregation processes. The introduction of the ballasting scheme required a re-tuning of the dissolution rates of calcite and opal as shown in Table 1.
Following recent evidence that the remineralization of organic carbon depends on temperature (e.g. Laufkötter et al., 2017), we have introduced a Q10 temperature dependence for the remineralization of POC and DOC (Segschneider and Bendtsen, 2013; Crichton et al., 2021), with a default Q10 value of 2. The complete set of remineralization parameters is listed in Table 1.
In the original HAMOCC, iron complexation by organic substances is assumed when the iron concentration exceeds a given threshold, and dissolved iron is then removed from the water column at a fixed rate. In CLIMBER-X, we explicitly model iron complexation, differentiating between free and complexed iron forms following Archer and Johnson (2000) and Parekh et al. (2004). The complexed iron is associated with an organic ligand, and only the free iron is available for scavenging. The ligand concentration is assumed to be constant at 1 nmol kg−1 with a ligand stability constant of 1×1011 kg mol−1. The speciation of iron is then determined by equilibrium kinetics. The scavenging rate of free iron is a combination of a minimum scavenging rate and a scavenging rate that is proportional to the POC, calcite and opal concentrations following Aumont et al. (2015) and Hauck et al. (2013). Compared to HAMOCC, we have also increased the stoichiometric iron ratio in organic compounds from to . The parameters related to the iron cycle are also reported in Table 1.
Table 1Modified HAMOCC parameters used in CLIMBER-X compared to HAMOCC6 (i.e. Table 2 in Ilyina et al., 2013).
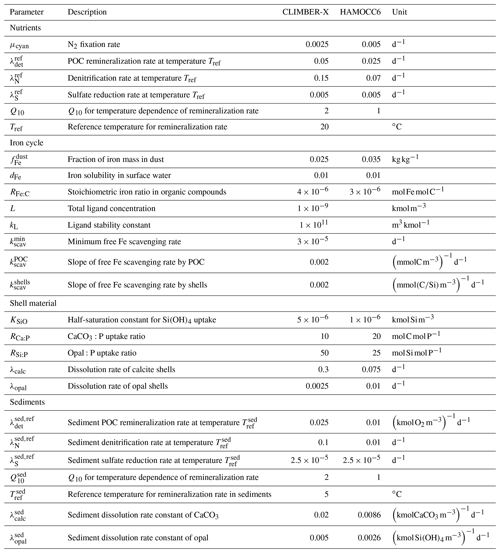
The carbon-13 isotope was recently implemented in HAMOCC by Liu et al. (2021). In CLIMBER-X we extended this approach to also include radiocarbon.
Since the ocean model in CLIMBER-X is a rigid lid model, following the OMIP protocol (Orr et al., 2017), we explicitly take into account the local concentration-dilution effect of the net surface freshwater flux, which changes surface DIC concentration and alkalinity. Two options are available in the model to implement the dilution effect on DIC and alkalinity. The first one ensures that the net global surface tracer flux is zero by applying deviations from the global average freshwater flux to the global average surface tracer concentration. The second (default) option applies the actual local surface freshwater flux to compute a new virtual top ocean layer thickness and then dilutes the tracers accordingly. In this case, the conservation of tracer inventories is ensured by compensating for imbalances over the global ocean. Additionally, during times when ocean volume is changing because of build-up or melt of land ice, concentrations of all tracers are globally adjusted while conserving tracer inventories. This is a reasonable simplification, considering that land ice volume changes occur on multi-millennial timescales, over which the ocean can be considered well mixed.
Based on scale analysis, we have excluded fast sinking tracers (CaCO3, opal, POC and dust) from advection, as these particles have sinking speeds which are large enough so that vertical transfer between different grid cells is more rapid than horizontal transfer by advection would be, considering the relatively coarse resolution of the ocean model. Following a similar line of thought, short-lived tracers like phytoplankton and zooplankton are also excluded from oceanic transport. However, convection and wind-driven surface vertical mixing are applied to all biogeochemical tracers.
In CLIMBER-X, HAMOCC is integrated with a time step of 1 d, which is also the time step of the physical ocean model.
2.2 Land carbon cycle: PALADYN
PALADYN is a comprehensive land surface–vegetation–carbon cycle model designed specifically for use in CLIMBER-X (Willeit and Ganopolski, 2016). It includes a detailed representation of the land carbon cycle. Photosynthesis is computed following the Farquhar model (Farquhar et al., 1980; Collatz et al., 1991) and depends on absorbed shortwave radiation, air temperature, vapour pressure deficit between leaf and ambient air, atmospheric CO2 and soil moisture. Carbon assimilation by vegetation is coupled to the transpiration of water through stomatal conductance. The model includes a dynamic vegetation module with five plant functional types (PFTs) competing for the grid-cell share based on their respective net primary productivity. The model distinguishes between mineral soil carbon, peat carbon, buried carbon and shelf carbon. Each soil carbon “type” has its own soil carbon pools generally represented by a litter and fast and slow carbon pools in each of the five soil layers. Carbon can be redistributed between the layers by vertical diffusion. For the vegetated macro-surface type, decomposition is a function of soil temperature and soil moisture. Carbon in permanently frozen layers is assigned a long turnover time which effectively locks carbon in permafrost. Carbon buried below ice sheets and on ocean shelves is treated separately. The land model also includes a dynamic peat module. PALADYN includes carbon isotopes 13C and 14C, which are tracked through all carbon pools in vegetation and soil. Isotopic discrimination is modelled only during the photosynthetic process. A simple methane module is implemented to represent methane emissions from anaerobic carbon decomposition in wetlands and peatlands. The integration of PALADYN into the coupled CLIMBER-X framework and subsequent sensitivity analyses of the land carbon cycle feedbacks, which were not performed with the offline PALADYN set-up in Willeit and Ganopolski (2016), highlighted the need to improve certain aspects of the model. These improvements are described next.
We have updated the parameterization of the roughness length for heat and moisture. Originally, it was simply taken to be proportional to the roughness length for momentum, but there is ample evidence from observations that the roughness length for scalars can be orders of magnitude lower than that for momentum when the surface roughness is large (e.g. Zilitinkevich, 1995; Chen and Zhang, 2009; Yang et al., 2008; Zheng et al., 2012). We have therefore implemented the parameterization from Zilitinkevich (1995), which includes a dependence of the surface roughness length for heat and moisture on the roughness Reynolds number. With this new parameterization, the exchange coefficient for the turbulent surface fluxes shows a much weaker dependence on the roughness of the surface, which has an impact on the vegetation feedback.
We have introduced a topographic erodibility factor for dust emissions following Ginoux et al. (2001). It assumes that a basin with pronounced topographic variations contains a large amount of sediments which have accumulated in the valleys and depressions and which can easily be mobilized by wind. The following topographic factor is then applied to scale dust emissions:
where z is the grid-cell mean elevation, and zmax and zmin are the maximum and minimum surface elevations computed from the high-resolution topography in the surrounding 15×15∘. The exponent 5 is taken from Zender et al. (2003).
The RuBisCO-limited photosynthesis rate in the version of the PALADYN model described in Willeit and Ganopolski (2016) was based on the “strong optimality” hypothesis of Haxeltine and Prentice (1996), which assumes that RuBisCO activity and the nitrogen content of leaves vary with canopy position and seasonally so as to maximize net assimilation at the leaf level (Schaphoff et al., 2018). However, we found that this formulation led to a relatively small increase in gross primary production over the historical period, which resulted in an overestimation of atmospheric CO2 in coupled historical simulations. We therefore introduced a new formulation for the maximum RuBisCO capacity, with dependencies on PFT-specific, constant foliage nitrogen concentration, specific leaf area and leaf temperature following Thornton and Zimmermann (2007) as implemented in CLM4.5 (Oleson et al., 2010).
In the original PALADYN formulation, the internal leaf CO2 concentration used for photosynthesis was computed based on the Cowan–Farquhar optimality hypothesis (Medlyn et al., 2011). In the new model version, for C3 plants, we have implemented an alternative scheme following the more general least-cost optimality model (Prentice et al., 2014; Lavergne et al., 2019) with the moisture dependence proposed by Lavergne et al. (2020).
In the isotopic discrimination during photosynthesis (Δ), we included an explicit fractionation term for photorespiration as recommended by several recent studies (Ubierna and Farquhar, 2014; Schubert and Jahren, 2018; Lavergne et al., 2019):
where ca and ci are the ambient and leaf-internal CO2 concentrations, pa is the ambient partial pressure of CO2 and Γ∗ is the CO2 compensation point.
For the distinction between evergreen and summergreen trees, in addition to a threshold on the coldest month's temperature, we have introduced a PFT-specific threshold on the growing degree days above 5 ∘C, which is set to 600 for needleleaf trees and 900 for shrubs following Sitch et al. (2003).
In the dynamic vegetation model, a parameter (λ) is used to partition the net primary production (NPP) between local growth of existing vegetation and lateral expansion (“spreading”) of vegetation coverage within the grid cell, with all of the NPP being used for growth for small leaf area index (LAI) values and all of the NPP being used for “spreading” for large LAI values. λ is assumed to be a piecewise linear function of the leaf area index between a minimum and maximum LAI. For small leaf area indices, all of the NPP is used for local growth (λ=0); for LAI above a critical value LAImin, a fraction (λ>0) is used for spreading:
However, since the simulated leaf area index depends strongly on NPP, which in turn has a pronounced dependence on atmospheric CO2, this formulation results in a strong dependence of λ on CO2, with an increasingly larger fraction of NPP being used for spreading as CO2 increases. We have therefore implemented a CO2 dependence in the maximum leaf area index to reduce this effect:
The fraction of decomposed litter respired directly as CO2 to the atmosphere has been reduced from 0.7 to 0.6 and the fraction of decomposed litter transferred to the slow soil carbon pool has been doubled from 0.015 to 0.03. Together these changes result in more carbon accumulating into the soil.
A simple representation of land use change has been introduced into the model following Burton et al. (2019) as described in Willeit et al. (2022). A fraction of each grid cell is prescribed as being used for agriculture and land use is then represented as a limitation to the space available for the woody PFTs to expand into. When forests and shrubs are affected by land use change, an additional disturbance rate of 1 yr−1 is prescribed on top of the standard background disturbance, leading to vegetation dying. The resulting dead vegetation carbon is then added as litter to the soil carbon pools, and a large part will be respired directly to the atmosphere within a few years. Storage of wood from deforestation in products such as paper or wood for construction is not accounted for in the model and soil carbon is assumed to not be directly affected by land use practices. Following deforestation, the model will grow C3 or C4 grasses, depending on climate conditions.
The partitioning of the soil carbon decomposed under anaerobic conditions into CO2 and CH4 used a prescribed constant ratio in Willeit and Ganopolski (2016). We modified this by making the fraction released as CH4 dependent on temperature with a Q10 of 1.8, following Riley et al. (2011) and Kleinen et al. (2020).
We implemented a chemical weathering model to compute the riverine fluxes of bicarbonate ions () (and therefore dissolved inorganic carbon and alkalinity) to the ocean and the consumption of atmospheric CO2. The weathering rate depends on the lithology and on the climate variables temperature and runoff. The lithological map of Hartmann and Moosdorf (2012) distinguishing 16 different lithologies is used to describe the spatial distribution of rocks. The parameters for the chemical weathering equations for all lithologies, except for carbonate sedimentary rocks and loess, are based on a spatially explicit runoff-dependent model of chemical weathering, which was calibrated for 381 catchments in Japan (Hartmann, 2009), with the additional temperature dependence of Hartmann et al. (2014). The effect of soil shielding on the weathering rate suggested by Hartmann et al. (2014) has not been considered since information on soil shielding is not readily available for periods beyond the recent past. For carbonate sedimentary rocks, the weathering rate follows the approach of Amiotte Suchet and Probst (1995) with a dependence on runoff. Alternatively, the temperature-dependent formulation of Romero-Mujalli et al. (2019) is available for use in the model. The weathering rate for loess sediments depends on runoff following Börker et al. (2020). The global distribution of loess cover for the present day and for the Last Glacial Maximum as well as the lithologies of the continental shelves that were exposed at the Last Glacial Maximum are taken from Börker et al. (2020). The weathering fluxes are transferred from the land to the ocean in the same way as water runoff, following the runoff routing scheme.
The carbon isotope fluxes from chemical weathering are computed assuming a δ13C of 1.8 ‰ for carbon originating from carbonate minerals (Derry and France-Lanord, 1996).
Equations describing silicate and phosphorus weathering fluxes are also available as part of the weathering model. However, silicate and phosphorus riverine fluxes are not considered in the default model set-up, as they would result in further complications related to the conservation of nutrients in the ocean. Instead, as discussed in Sect. 2.1, the silicate and phosphorus budgets are closed by assuming that the sediment burial flux is returned as input at the ocean surface.
2.3 Atmospheric CO2
The atmospheric CO2 concentration in CLIMBER-X is a globally uniform value. It can either be prescribed (as constant or time-dependent) or interactively computed by the model from the following prognostic equation for the total carbon content stored as CO2 in the atmosphere (Catm):
The source and sink terms on the right-hand side represent, from left to right, the net sea–air carbon flux, the global net land-to-atmosphere carbon flux, the anthropogenic carbon emissions (excluding land use change), the CO2 consumption by silicate and carbonate weathering, the volcanic degassing flux and the CO2 flux from the oxidation of atmospheric methane originating from non-agricultural sources. The CO2 consumption by weathering is computed assuming that all carbon in the originating from the weathering of silicate rocks () comes from the atmosphere, while only half of the carbon in the originating from the weathering of carbonate rocks and sediments () comes from the atmosphere:
The constant volcanic degassing rate is set to half the silicate weathering rate (e.g. Munhoven and François, 1994) as determined by an equilibrium spin-up simulation:
The flux from the oxidation of methane, , is computed by the CH4 model as described in Sect. 2.4 below. The atmospheric CO2 concentration is then computed from Catm using a conversion factor of 2.12 PgC ppm−1 (Denman et al., 2007).
Equations similar to Eq. (5) are also used for the carbon isotopes 13C and 14C. The prognostic equation for the stable isotope 13C in atmospheric CO2 is
The 13C fluxes from land and ocean are explicitly computed by the land and ocean carbon cycle models as described in detail in Willeit and Ganopolski (2016) and Liu et al. (2021). The δ13C of anthropogenic carbon emissions is prescribed as time-dependent from historical data of Andres et al. (2017), and the 13C flux from CO2 consumption by weathering, assuming no fractionation, is simply computed as
The 13C of volcanic degassing is computed assuming a δ13C of −5 ‰.
The prognostic equation for radiocarbon 14C in atmospheric CO2 reads as
Carbon sources originating from geological reservoirs, i.e. volcanic degassing, are assumed to contain no radiocarbon. Similarly, radiocarbon is assumed to be absent in anthropogenic carbon emissions from fossil fuel burning, because the age of fossils far exceeds the half-life of 14C. The production rate of radiocarbon in the atmosphere () is prescribed in the model and the radiocarbon decay time is years.
2.4 Atmospheric CH4
Similarly to CO2, atmospheric CH4 is also considered to be well mixed in the atmosphere and is therefore represented as a globally uniform value. The atmospheric CH4 concentration can be prescribed, or it can be interactively computed by the model from
Methane sources include natural emissions from wetlands and peatlands (), which are explicitly simulated by the model as originating from anaerobic decomposition processes of carbon in soils (Willeit and Ganopolski, 2016). Other natural sources of methane are generally smaller (e.g. Saunois et al., 2020; Kleinen et al., 2020) and are neglected here for simplicity. Anthropogenic methane emissions () are prescribed in the model. The sink of methane from oxidation in the atmosphere is computed using a constant residence time of CH4, years, which is a reasonable first approximation, at least for climate conditions ranging between the Last Glacial Maximum and the present day (Kleinen et al., 2020; Levine et al., 2011; Hopcroft et al., 2017).
Two different configurations of the carbon cycle model are available and can be chosen according to the specific needs.
The first (and simplest) set-up consists of ocean, land and atmosphere carbon cycle components only. In this set-up marine sediments are disabled and particulate fluxes that reach the ocean floor are completely remineralized/dissolved in the bottom ocean grid cell. Rock weathering from land is also switched off, so that the carbon exchange between ocean, land and atmosphere occurs only through air–sea fluxes and through land–atmosphere exchanges. In this set-up the carbon system is closed in the sense that there are no natural sources and sinks from and to geological reservoirs. As a response to an external climate perturbation, carbon is then simply redistributed between atmosphere, ocean and land, with the total carbon in the system being conserved. This set-up is equivalent to what is used in many state-of-the-art Earth system models for climate change projections on centennial timescales (e.g. Séférian et al., 2020). The model spin-up for this simple set-up is straightforward and requires only that the model is run to steady state with a prescribed atmospheric CO2 concentration for ≈10 000 years. The slowest timescale in this set-up is given by the slow decomposition rate of organic carbon in frozen soils, which is limited to a maximum value set by default to 5000 years. The initial state for the spin-up run is given by observed present-day 3D concentrations of different tracers in the ocean (Lauvset et al., 2016; Olsen et al., 2016; Garcia et al., 2013b), while the land surface is assumed to be covered by bare soil and with no carbon stored on land.
The closed carbon cycle set-up is applicable to simulations of up to 1000 years. On longer timescales, sediment and weathering processes become important and need to be accounted for when performing long-term transient simulations with interactive CO2. Although it is unlikely that in reality the slow carbon cycle processes related to marine sediments, peatlands and permafrost carbon are in equilibrium at any specific point in time, for practical reasons we assume that such an equilibrium is a reasonable first approximation. Assuming that the pre-industrial is an equilibrium state of the climate–carbon cycle system allows us to run perturbation experiments with the interactive carbon cycle without having to deal with possible long-term drifts in atmospheric CO2. However, the long timescale of ∼ 100 000 years involved in ocean sediment processes represents a challenge in running the model into equilibrium, even for a high-throughput model like CLIMBER-X. We therefore implemented a scheme to run the physical ocean and ocean biogeochemistry models in an offline set-up with prescribed climatological daily input fields at the ocean surface. This set-up results in a speed-up of a factor >2 relative to running the fully coupled climate–carbon cycle model, meaning that ocean carbon cycle and marine sediments can be run into equilibrium in about a week of computing time on a high-performance computer. In detail, the spin-up procedure of the full carbon cycle configuration comprises two different stages. Atmospheric CO2 is prescribed to a constant value throughout the process, at 280 ppm for the pre-industrial case. The first stage aims at spinning up the sediment model. For this purpose the full carbon cycle–climate model is run for 5000 years, and every 300 years the sediment model is run offline for 1000 years. During this stage all net fluxes into the sediments are compensated for and returned as inputs at the ocean surface in order to approximately conserve water column tracer inventories while the sediments are filling up. In the second stage we switch to simulated DIC and alkalinity weathering fluxes from land and at the same time also switch to the more efficient offline ocean–biogeochemistry set-up described above and run the model until an approximate equilibrium is reached after ∼ 100 000 years (Fig. 2). A simplification that is made in the open carbon cycle set-up is that organic carbon and opal that are buried in the sediments, and are therefore effectively leaving the system, are returned in remineralized form to the surface ocean, so that phosphorus and silica inventories of the ocean–sediment system are conserved throughout the simulation.
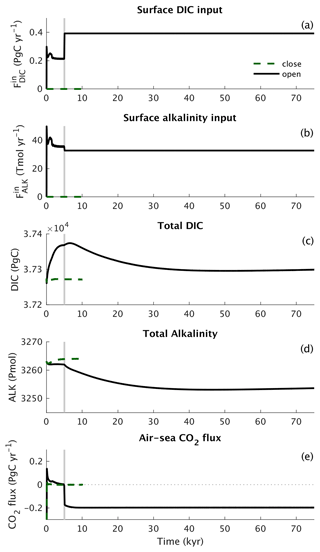
Figure 2Open versus closed carbon cycle spin-up for pre-industrial conditions. The figure shows surface input of (a) DIC and (b) alkalinity, the evolution of (c) DIC and (d) alkalinity inventories in the ocean, and (e) the air–sea CO2 flux. The grey vertical lines indicate the switch between the first and second spin-up phases, as described in the text.
The carbon fluxes among the different model components in the open set-up for equilibrium pre-industrial conditions are schematically illustrated in Fig. 3. The volcanic degassing rate is equal to half the atmospheric CO2 consumption by silicate weathering, in accordance with theory (Munhoven and François, 1994). Note that not only the carbon budget of the different compartments (atmosphere, ocean, lithosphere) is well balanced but that the ocean alkalinity budget also is.
Here we present results from a CLIMBER-X simulation with interactive CO2 and CH4 in the open carbon cycle set-up for the historical period (1850–2015) and provide a comprehensive evaluation of model performance against various observational datasets. The forcings for this simulation include variations in solar radiation (Matthes et al., 2017), radiative forcing of volcanic eruptions (Prather et al., 2013), globally uniform N2O concentrations from Köhler et al. (2017), globally uniform CFC11 and CFC12 concentrations from Meinshausen et al. (2017), 3D O3 concentrations and 2D load from the ensemble mean of CMIP6 models and land use change (pasture and cropland fractions) from Ma et al. (2020). The model is initialized from an 80 000-year equilibrium simulation with the open carbon cycle set-up for pre-industrial boundary conditions and a prescribed atmospheric CO2 of 280 ppm, as described in Sect. 3 and shown in Fig. 2.
4.1 Present day
In the following, different simulated climatological characteristics are compared to observations to assess the model performance for the present day. Unless stated otherwise, the comparison with observations is for the time interval from 1981 to 2010. To give an overview of how CLIMBER-X compares to state-of-the-art Earth system models based on general circulation models, we also include results from model simulations from the recent CMIP6 (Eyring et al., 2016). The following CMIP6 models are included for ocean biogeochemistry: CESM2, IPSL-CM6A-LR, MRI-ESM2-0, MIROC-ES2L, MPI-ESM1-2-LR, UKESM1-0-LL and CanESM5. For the land carbon cycle, the following models are used for comparison: ACCESS-ESM1-5, BCC-CSM2-MR, CanESM5, CNRM-ESM2-1, GFDL-ESM4, IPSL-CM6A-LR, MIROC-ES2L, MPI-ESM1-2-LR, MRI-ESM2-0, NorESM2-LM and UKESM1-0-LL. For ocean biogeochemistry, we highlight how the model compares with results from the MPI-ESM1-2-LR employing the original marine carbon cycle model HAMOCC6.
4.1.1 Ocean biogeochemistry and marine sediments
An overview of simulated global variables characterizing the ocean carbon cycle are presented and compared to observation-based estimates in Table 2, providing a summary of model performance for the present day.
Jacobson et al. (2007)Regnier et al. (2013)Buitenhuis et al. (2018)(Lauvset et al., 2016; Olsen et al., 2016)Garcia et al. (2013b)Garcia et al. (2013b)Garcia et al. (2013b)Johnson and Bif (2021)Carr et al. (2006)Karl et al. (2002)Großkopf et al. (2012)Dunne et al. (2007)Dunne et al. (2007)Tréguer and De La Rocha (2013)Dunne et al. (2007)Battaglia et al. (2016)Milliman and Droxler (1996)Tréguer and De La Rocha (2013)Tréguer et al. (2021)Cartapanis et al. (2018)Cartapanis et al. (2018)Tréguer and De La Rocha (2013)Representing the ocean ventilation timescale reasonably well is a prerequisite for simulating biogeochemical tracers in the ocean. The ocean uptake of CFCs of anthropogenic origin over the historical period is often used to probe the ventilation of the ocean on decadal timescales, while the pre-industrial radiocarbon concentration in the ocean provides information on the age distribution of the water masses in an approximate equilibrium state. We therefore start by comparing how well the model reproduces the CFC11 and radiocarbon distributions in the ocean. The inventory of CFC11 in the ocean starts to increase after ≈1950 as a consequence of its increase in the atmosphere (Fig. 4). Estimates for the CFC11 inventory in the year ≈1994 are available from models from the OCMIP model intercomparison (Dutay et al., 2002) and from direct observations (Willey et al., 2004). CLIMBER-X results are generally consistent with these estimates (Fig. 4), indicating that, at least at the global scale, the decadal ventilation timescale in CLIMBER-X is well in line with observations and other models. In terms of spatial distribution, the CFC11 uptake is overestimated in the North Pacific Ocean, in the North Indian Ocean and around Antarctica, while too small CFC11 concentrations are simulated at mid latitudes in all basins at depths between 500 and 1000 m (Fig. 5).
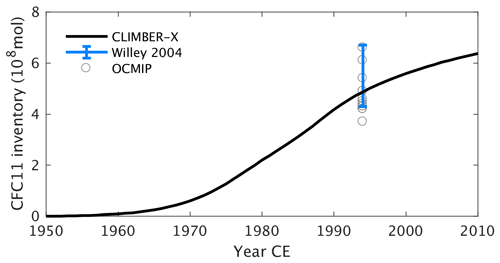
Figure 4Historical global cumulative ocean uptake of CFC11 in CLIMBER-X compared to observations (Willey et al., 2004) and OCMIP models (Dutay et al., 2002).
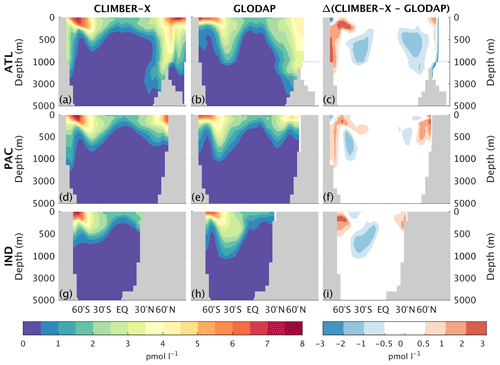
Figure 5Zonally averaged CFC11 concentration for the year 1994 in CLIMBER-X (a, d, g) and GLODAP (Key et al., 2004) (b, e, h) for different basins: Atlantic (a–c), Pacific (d–f), and Indian (g–i) oceans. The model bias is shown in panels (c), (f), and (i).
The radiocarbon ventilation age in the pre-industrial gives additional insights into the ocean ventilation under quasi-equilibrium conditions, information which is complementary to CFC11. The radiocarbon ventilation age of the deep ocean is nicely reproduced by CLIMBER-X, while radiocarbon age is systematically overestimated in the upper kilometre across all ocean basins (Fig. 6). The too old (in terms of radiocarbon age) sub-surface waters could be a result of the model not explicitly resolving synoptic processes in the atmosphere and therefore not representing the non-linear effects of synoptic variability on vertical mixing of tracers. For instance, a one-time mixing down to 200 m depth by a wind storm could have a large effect on some tracers, which cannot be resolved by using climatological mean winds. We would expect this non-linear effect to be much more important for radiocarbon than for nutrients. The analyses of CFC11 and radiocarbon provide important insights into the ocean ventilation in the model and will be useful when discussing model biases in the distribution of other biogeochemical tracers below.
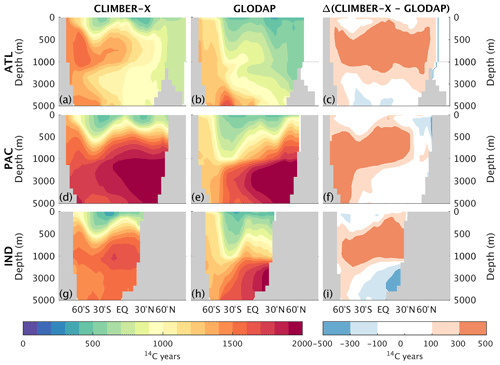
Figure 6Zonally averaged pre-industrial radiocarbon ventilation age in CLIMBER-X (a, d, g) and GLODAP Key et al. (2004) (b, e, h) for different basins: Atlantic (a–c), Pacific (d–f), and Indian (g–i) oceans. The model bias is shown in panels (c), (f), and (i).
The spatial pattern of the air–sea CO2 exchange is well captured by the model (Fig. 7), with outgassing generally taking place in the tropics and CO2 being taken up at mid to high northern latitudes and at mid latitudes of the Southern Hemisphere. The main difference compared to the other models is observed around the Equator, with a less pronounced peak in CO2 release simulated by CLIMBER-X (Fig. 8), which is likely related to deficiencies in the simulated ocean circulation close to the Equator, where the geostrophic approximation employed in CLIMBER-X reaches its limit of applicability. In the Southern Ocean, most CMIP6 models tend to overestimate the CO2 uptake compared to observations (e.g. Gruber et al., 2009) (Fig. 8), while CLIMBER-X is apparently more consistent with recent estimates, although with substantial differences in the spatial distribution of the CO2 flux (Fig. 7). Notably, in the Southern Ocean the CLIMBER-X air–sea CO2 exchange diverges from that simulated by the MPI-ESM1-2-LR model (Fig. 8), which employs the original HAMOCC6 ocean biogeochemistry model. This is possibly related to the lower simulated net primary production in the Southern Ocean in CLIMBER-X compared to MPI-ESM (Fig. 9a). However, the MPI-ESM seems to be an outlier in the simulated primary production in the Southern Ocean, possibly because of biases in climate, which are unrelated to the HAMOCC ocean carbon cycle model.
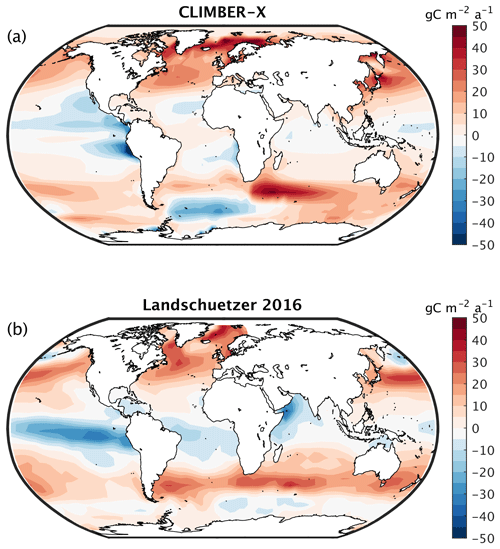
Figure 7The 1985–2010 average air–sea CO2 flux in (a) CLIMBER-X compared to (b) observations from Landschützer et al. (2016).
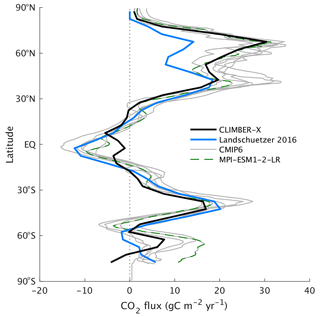
Figure 8Zonal mean air–sea CO2 flux (1985–2010 average) in CLIMBER-X compared to observations from Landschützer et al. (2016) and selected CMIP6 models.
The export of particulate organic carbon from the euphotic layer drives the biological pump and generally follows the primary productivity pattern, with modifications due to varying sinking speeds and remineralization rates of POC in the water column. While the net primary productivity in CLIMBER-X is in line with CMIP6 models (Fig. 9a) and the globally integrated value of 53 PgC yr−1 agrees well with observations (Table 2), the export production in the model is generally at the lower end of the CMIP6 model range (Fig. 9b). CaCO3 and opal export are compared to CMIP6 models in Fig. 9c, d.
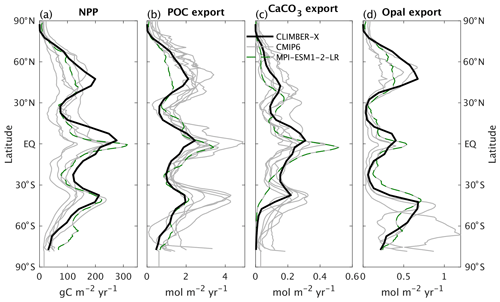
Figure 9The 1981–2010 average global zonal mean (a) net primary production, (b) particulate organic carbon export at 100 m depth, (c) CaCO3, and (d) opal export at 100 m depth. Results from CLIMBER-X are compared to CMIP6 models.
Primary production in the ocean is limited by the availability of nutrients. Over large parts of the surface ocean, nitrogen concentrations constitute the main limiting factor for photosynthesis in CLIMBER-X (Fig. 10). However, over the Southern Ocean, in the equatorial Pacific and in the North Pacific, production is limited by the availability of iron (Fig. 10). This is in accordance with observations showing that iron limitation is usually important where sub-surface nutrient supply is enhanced, such as in oceanic upwelling regions (e.g. Moore et al., 2013). Since one of the main iron sources in the ocean is from mineral dust deposited at the ocean surface (e.g. Tagliabue et al., 2016), iron limitation is confined to regions with low dust deposition. The dust cycle is an integral part of CLIMBER-X, and the dust deposition is therefore explicitly modelled. The simulated dust deposition compares reasonably well with estimates from complex ESMs for the present day (Fig. 11), although they are relatively poorly constrained. A comparison of dust deposition fluxes with observations over land further indicates that the model is able to capture the general pattern of the dust deposition rate (Fig. 12).
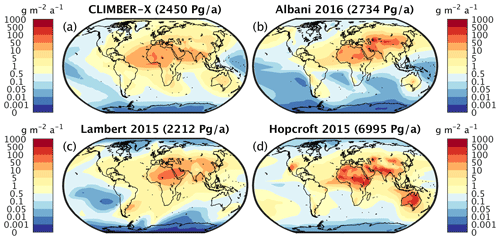
Figure 11(a) CLIMBER-X annual dust deposition flux compared to model-based products of (b) Albani et al. (2016), (c) Lambert et al. (2015), and (d) Hopcroft et al. (2015). The respective globally integrated deposition values are given in brackets in the panel titles.
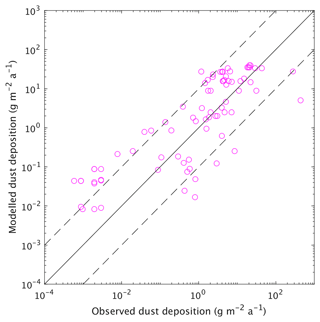
Figure 12Simulated versus observed dust deposition fluxes at different locations available from the AeroCom dataset (Huneeus et al., 2011, and references therein). The dashed lines indicate 1 order of magnitude deviation from the 1:1 line.
The simulated dissolved iron concentration in surface water is closely related to the dust deposition shown in Fig. 11. It is therefore high in the Atlantic and Indian oceans, lower in the Southern Ocean and very small over large parts of the Pacific (Fig. 13). This is broadly consistent with observations (e.g. Tagliabue et al., 2012), but measurements of iron concentration in ocean water are still relatively sparse.
The main features of the surface nitrate concentration are well reproduced by CLIMBER-X, with large concentrations in the Southern Ocean, moderate values in the upwelling region of the eastern equatorial Pacific and in the North Atlantic and North Pacific and low values elsewhere (Figs. 15 and 14a). The most pronounced model biases are found in too high nitrate concentrations in the Arctic and too low values in the North Pacific. The simulated basin-wide vertical distribution of nitrate is in very good agreement with observations (Fig. 16).
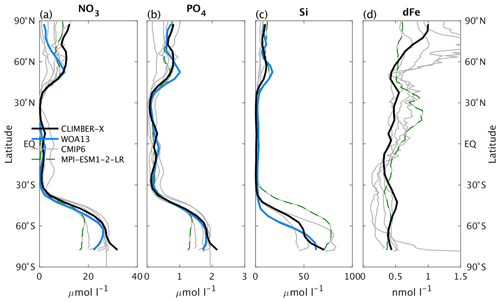
Figure 14The 1981–2010 average global zonal mean surface concentrations of the nutrients (a) nitrate, (b) phosphate, (c) silicate, and (d) dissolved iron. CLIMBER-X is compared to observations (Garcia et al., 2013b) and CMIP6 model results.
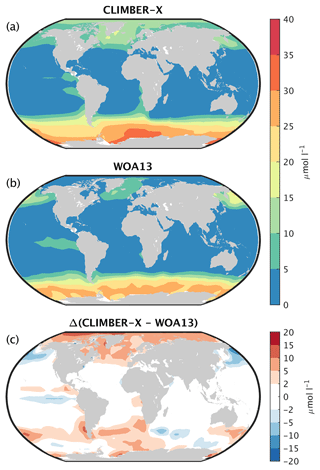
Figure 15Surface NO3 concentration in (a) CLIMBER-X (1981–2010 average) compared to (b) observations from the World Ocean Atlas 2013 (WOA13, Garcia et al., 2013b). The model bias is shown in panel (c).
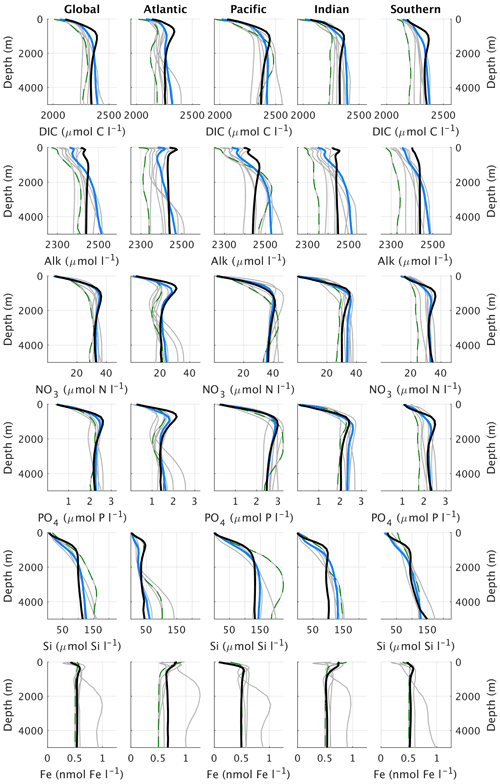
Figure 16Global and basin-wide average profiles of different biogeochemical tracers in the ocean, from top to bottom: DIC, alkalinity, nitrate, phosphate, silicate, and dissolved iron. CLIMBER-X results (black) are compared to observations (blue) (Lauvset et al., 2016; Olsen et al., 2016; Garcia et al., 2013a, b) and CMIP6 model results (grey). Results from the MPI-ESM1-2-LR are shown by the green dashed lines. The boundary of the Southern Ocean is set at 35 ∘ S and the Southern Ocean section is not included in the profiles of the Atlantic, Pacific, and Indian oceans. CLIMBER-X and CMIP6 data are averages over the time period 1981–2010.
The 3D phosphate distribution in the global ocean is nicely captured by the model (Figs. 17, 14b, and 16), except for too low concentrations simulated in the surface ocean of the North Pacific and North Indian oceans. The negative bias in the North Pacific is consistent with the too low simulated surface nitrate concentrations, both originating from a too vigorous ventilation of water masses in the upper kilometre in the physical ocean model.
As a result of reduced primary productivity in the Southern Ocean in CLIMBER-X compared to MPI-ESM1-2-LR, both surface nitrate and phosphate concentrations are consistently higher in CLIMBER-X (Fig. 14a, b), as fewer nutrients are assimilated during photosynthesis.
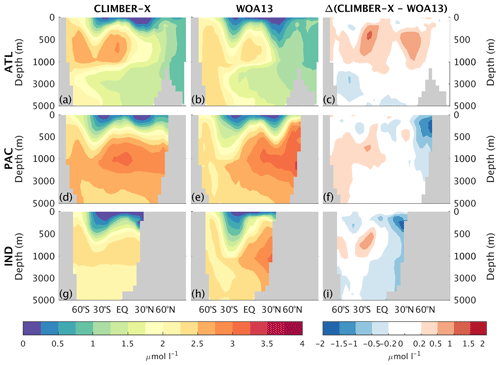
Figure 17Zonally averaged PO4 concentration in CLIMBER-X, 1981–2010 average (a, d, g), and WOA13 (Garcia et al., 2013b) (b, e, h) for different basins: Atlantic (a–c), Pacific (d–f), and Indian (g–i) oceans. The model bias is shown in panels (c), (f), and (i).
Silicate concentration is generally overestimated in the sub-surface ocean and is underestimated in the deep North Pacific and North Indian oceans (Fig. 18), similarly to other nutrients (Fig. 17).
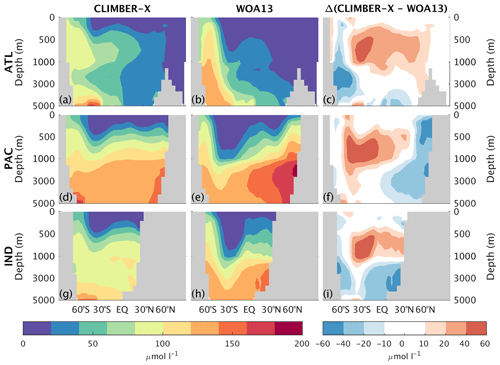
Figure 18Zonally averaged Si concentration in CLIMBER-X, 1981–2010 average (a, d, g), and WOA13 (Garcia et al., 2013b) (b, e, h) for different basins: Atlantic (a–c), Pacific (d–f), and Indian (g–i) oceans. The model bias is shown in panels (c), (f), and (i).
The large-scale patterns of oxygen concentration in ocean waters simulated by CLIMBER-X are largely consistent with observations (Fig. 19), but the extent and depth of the oxygen minimum zones, in particular in the eastern equatorial Pacific, are overestimated. This bias is common to many CMIP5 models (e.g. Cabré et al., 2015). Other biases include a too oxygen-depleted Southern Ocean and too high oxygen concentrations in the upper North Pacific and North Indian oceans, again resulting from the excessive water mass ventilation in those regions as discussed above.
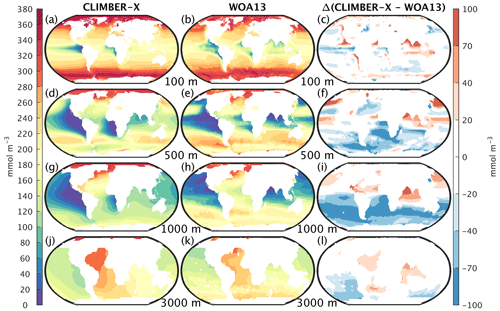
Figure 19Oxygen concentration in CLIMBER-X, 1981–2010 average (left column), and WOA13 (Garcia et al., 2013a) (middle column) at different ocean depths: from top to bottom, 100, 500, 1000, and 3000 m. The model bias is shown in the right column.
Both DIC and alkalinity are generally overestimated in the upper ocean (Fig. 16), particularly in Antarctic intermediate water masses, and underestimated in the deep ocean (Figs. 20 and 21). These biases in the simulated vertical distribution of DIC and alkalinity could be due to a relatively low CaCO3 export from the euphotic layer (Table 2), which leads to a too weak vertical redistribution. Additionally, the simulated DIC concentration is generally too low in the North Pacific and North Indian oceans.
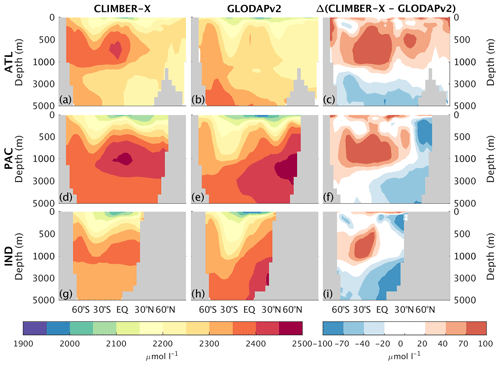
Figure 20Zonally averaged dissolved inorganic carbon in CLIMBER-X, 1981–2010 average (a, d, g), and GLODAPv2 (Lauvset et al., 2016; Olsen et al., 2016) (b, e, h) for different basins: Atlantic (a–c), Pacific (d–f), and Indian (g–i) oceans. The model bias is shown in panels (c), (f), and (i).
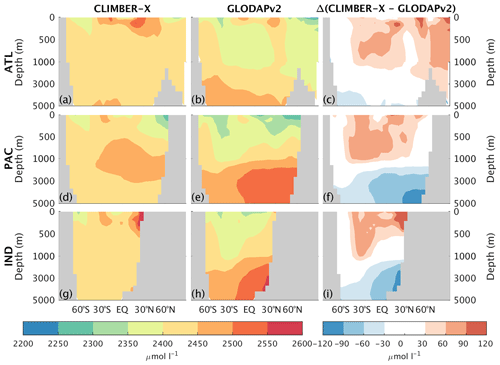
Figure 21Zonally averaged total alkalinity in CLIMBER-X, 1981–2010 average (a, d, g), and GLODAPv2 (Lauvset et al., 2016; Olsen et al., 2016) (b, e, h) for different basins: Atlantic (a–c), Pacific (d–f), and Indian (g–i) oceans. The model bias is shown in panels (c), (f), and (i).
The carbon-13 isotope in the ocean helps to track the distribution of different water masses. The higher δ13C values in the Atlantic Ocean compared to the Pacific Ocean, originating mainly from the pronounced overturning circulation in the Atlantic, which is absent in the Pacific, are generally captured by the model (Fig. 22). The negative biases at 500–1500 m depth are associated with the “nutrient trapping” problem (Aumont et al., 1999; Dietze and Loeptien, 2013) that is often seen in ESMs. This problem is characterized by high concentrations of remineralized nutrients and carbon and, therefore, low δ13C (Liu et al., 2021). The positive biases through the whole water column in the North Atlantic, North Pacific and North Indian oceans are possibly the result of too strong ventilation in these regions in the model.
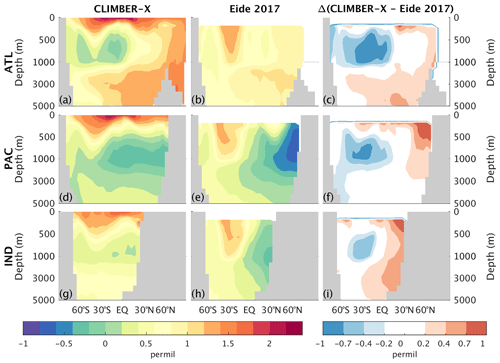
Figure 22Zonally averaged δ13C in CLIMBER-X, 1981–2010 average (a, d, g), and Eide et al. (2017) (b, e, h) for different basins: Atlantic (a–c), Pacific (d–f), and Indian (g–i) oceans. The model bias is shown in panels (c), (f), and (i).
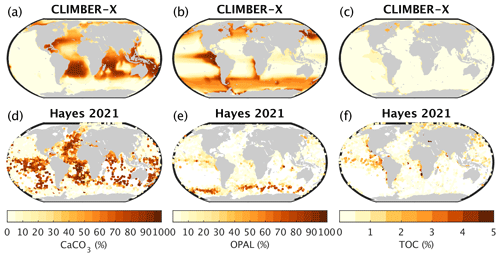
Figure 23Weight fraction of calcite, opal, and organic carbon in marine sediments as simulated by CLIMBER-X (a–c) compared to observations (Hayes et al., 2021) (d–f).
In the Atlantic and Indian oceans, CaCO3 dominates the sediment composition, in accordance with observations (Fig. 23a, d). However, little CaCO3 is simulated in large parts of the sediment in the eastern Pacific Ocean, where observations indicate widespread CaCO3 content in the Southern Hemisphere (Fig. 23a, d). The underestimation of calcite weight fractions in sediments of the eastern South Pacific Ocean is caused by water being undersaturated with respect to calcite in this area. This leads to dissolution of most of the calcite produced at the surface before it can even reach the sediments. The strongly undersaturated water is ultimately a result of deficiencies in the simulated ocean circulation. Some other models show similar deficiencies in the simulated calcite fraction in Pacific sediments (e.g. Kurahashi-Nakamura et al., 2022). Global CaCO3 sediment deposition and burial are in line with observational underestimates (Table 2), with around 25 % of the deposited CaCO3 undergoing dissolution. The opal content in sediments in CLIMBER-X is overestimated (Fig. 23b, e), even though the global opal sedimentation and burial fluxes are fully consistent with observational estimates (Table 2). Opal is particularly abundant in the eastern equatorial Pacific simply as a result of missing CaCO3 in the sediments in that area. Organic carbon is found mainly on the continental margins and in the equatorial eastern Pacific, in agreement with observations (Fig. 23c, f), although CLIMBER-X tends to underestimate the organic carbon content in sediments, possibly because of a too small sediment deposition flux of POC (Table 2).
4.1.2 Land carbon cycle
A detailed evaluation of the land carbon cycle component has already been presented in the original PALADYN description paper (Willeit and Ganopolski, 2016). However, here we partly repeat the analysis to show the model performance in the coupled climate model set-up and with the additional modifications to the model described above.
A selection of simulated global variables characterizing the land carbon cycle is presented and compared to observation-based estimates in Table 3, providing a summary of model performance for the present day.
Beer et al. (2010)Ito (2011)Fan et al. (2020)Fan et al. (2020)Varney et al. (2022)Varney et al. (2022)Brown et al. (1998)Tarnocai et al. (2009)Hugelius et al. (2014)Yu et al. (2010)Yu et al. (2010)Prigent et al. (2007)Papa et al. (2010)Saunois et al. (2020)Saunois et al. (2020)Saunois et al. (2020)Munhoven (2002)Munhoven (2002)Munhoven (2002)Amiotte Suchet et al. (2003)Gaillardet et al. (1999)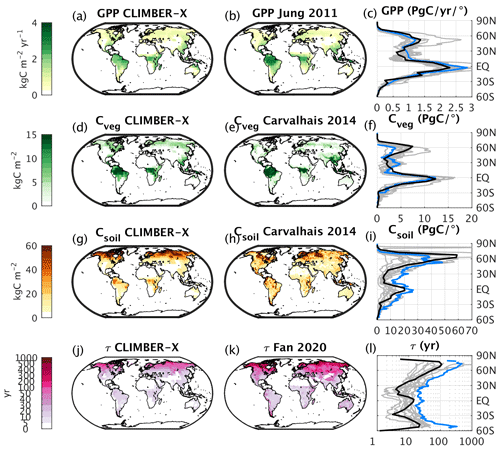
Figure 24(a) Simulated GPP compared to (b) observations (Jung et al., 2011). (c) Comparison of zonally integrated GPP. (d) Simulated vegetation carbon compared to (e) observations (Carvalhais et al., 2014). (f) Comparison of zonally integrated vegetation carbon. (g) Simulated soil carbon compared to (h) observations (Carvalhais et al., 2014). (i) Comparison of zonally integrated soil carbon. (j) Simulated ecosystem carbon turnover time compared to (k) observations (Fan et al., 2020). (l) Comparison of zonal mean ecosystem carbon turnover time. In panels (c), (f), (i), and (l), results from CLIMBER-X are shown in black, observations in blue, and CMIP6 models in grey. CLIMBER-X and CMIP6 data are averages over the time period 1981–2010.
Photosynthesis is the basic process by which carbon enters the land domain. The simulated gross primary production (GPP), which quantifies this process, is in good agreement with observational estimates, both in terms of global integrals (Table 3) and in terms of spatial distribution (Fig. 24a, b, c).
The total carbon stored in the vegetation, both above ground and below ground, is slightly overestimated in the model (Table 3), but the meridional distribution, mainly originating from large-scale differences in precipitation, is well reproduced (Fig. 24d, e, f). Most of the soil carbon in CLIMBER-X is stored in cold soils of the Northern Hemisphere high latitudes, in agreement with observations (Fig. 24g, h, i). However, compared to estimates from Carvalhais et al. (2014), the soil carbon distribution is too skewed toward high northern latitudes, and there is too little carbon in the tropics. Most CMIP6 models underestimate soil carbon in the tropics as well (Fig. 24j).
In CLIMBER-X, ∼ 1500 PgC of carbon is stored in the top soil metre, in good agreement with different estimates (Table 3). However, with ∼ 2150 PgC, the total soil carbon content seems to be underestimated compared to observations, which suggest >3000 PgC. This indicates that too little carbon is simulated in soil below 1 m depth. However, total soil carbon content estimates vary widely between datasets (e.g. Fan et al., 2020), with e.g. 1952±198 PgC in WISE30sec (Batjes, 2016) and 3141±893 PgC in Sanderman et al. (2017) in the top 2 m of soil. Most of the carbon in mineral soil layers below 1 m is recalcitrant, and its response to changes in environmental conditions is uncertain. In Earth system models, total soil carbon storage is usually much lower (1206±445 PgC, Varney et al., 2022), as these models account for active carbon responding on centennial timescales. In CLIMBER-X, one possible explanation for underestimated carbon content in deeper soil layers is that the maximum turnover timescale of soil carbon is set to 5000 years in the model, which limits the amount of carbon that can be accumulated in cold, frozen soil layers. Other possible reasons include (i) a general underestimation of vertical carbon transport by diffusion, particularly into perennially frozen soil layers, and (ii) a possible depth dependence of soil carbon turnover due to processes other than temperature and moisture (e.g. Koven et al., 2013) and that are not included in the model. Consistently, the carbon contained in areas affected by permafrost is ∼ 800 PgC, which is also a bit lower than the ∼ 1100–1500 PgC suggested by observations (Table 3). Let us note that, even when models are initialized with the observed permafrost carbon stock of ≈1300 PgC, remapping at model resolution and accounting for differences in soil temperatures between models and observations generally leads to a reduction in permafrost carbon stocks (e.g. Kleinen and Brovkin, 2018). The CLIMBER-X-simulated peatland extent is lower than estimated (Yu et al., 2010), and the peat carbon is also consistently underestimated accordingly (Table 3).
The turnover time of terrestrial ecosystem carbon is an integrated quantitative measure of the residence time of carbon on land, from the time it is fixed by photosynthesis to the time it is returned to the atmosphere through respiration processes. It is computed as the ratio between land carbon stocks (vegetation + soil) and gross primary production. The ecosystem carbon turnover time simulated by CLIMBER-X is in line with CMIP6 models, while it is underestimated compared to observation-based estimates from Fan et al. (2020) (Fig. 24j, k, l). However, it should be noted that the large uncertainties in soil carbon content result in a rather uncertain estimated ecosystem carbon turnover time (Fan et al., 2020).
The global maximum monthly wetland extent in CLIMBER-X agrees well with observations (Table 3), although with substantial differences in the geographic distribution (Fig. 25). Compared to the multi-satellite product from GIEMS (Global Inundation Extent from Multi-Satellites) (Prigent et al., 2007; Papa et al., 2010), the model simulates a larger wetland extent in tropical forest areas. However, when compared to other wetland products based on data other than from satellites, GIEMS underestimates wetlands below dense forests (e.g. the Amazon forest) (e.g. Melack and Hess, 2010). In South-East Asia, the GIEMS wetland extent also includes extensive rice cultivation areas, which are not represented in the model.
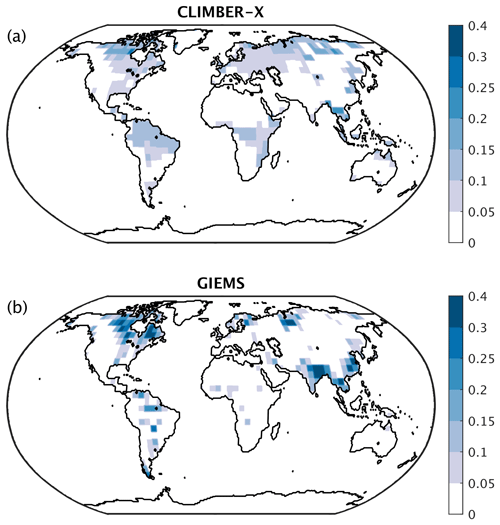
Figure 25Maximum monthly wetland fraction (a) in CLIMBER-X compared to (b) the GIEMS dataset (Papa et al., 2010; Prigent et al., 2007).
In CLIMBER-X, methane is emitted exclusively from wetlands. Because of the dependence of methane emissions on soil carbon decomposition rates and because of the temperature dependence of the fraction of wetland carbon respired as methane, wetland methane emissions are dominated by tropical sources (Table 3, Fig. 26), in agreement with observations (e.g. Saunois et al., 2020). The total CH4 emissions from wetlands are at the high end of recent estimates, which is a result of tuning the emissions in the model to match the observed emissions from all natural sources.
Chemical weathering fluxes are generally high where runoff is high, with the separation between silicate and carbonate weathering being modulated by lithological properties (Fig. 27). The global CO2 consumption rate by weathering and the alkalinity flux to the ocean in the form of bicarbonate produced by rock weathering are in good agreement with observational estimates (Table 3), while the partitioning between carbonate and silicate weathering is skewed toward carbonate weathering (Table 3).
4.2 Historical period
As shown by Willeit et al. (2022), the historical climate evolution is well simulated by CLIMBER-X. Here we extend this analysis by focusing on the carbon cycle response.
The historical atmospheric CO2 concentration is well reproduced by the model, with CO2 at the year 2015 being within ∼ 5ppm of direct measurements (Fig. 28). Biases in simulated CO2 of ∼ 10 ppm are quite common in state-of-the-art ESMs (e.g. Hoffman et al., 2014; Friedlingstein et al., 2014).
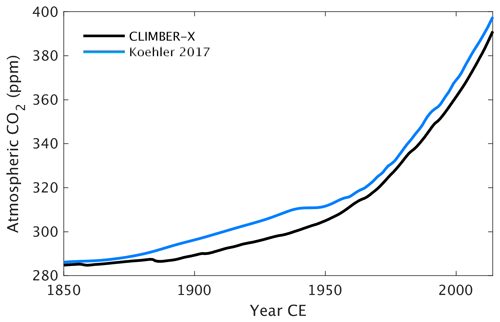
Figure 28Historical atmospheric CO2 concentration from a coupled CLIMBER-X simulation compared to observations (Köhler et al., 2017).
The partitioning of the anthropogenic carbon emitted over the historical period among the different spheres is compared with recent estimates of the Global Carbon Budget (GCB) (Friedlingstein et al., 2022) by the Global Carbon Project in Fig. 29. The amount of fossil carbon emitted from anthropogenic activities is prescribed from empirical data and therefore by definition matches with estimates from Friedlingstein et al. (2022). The carbon emissions resulting from land use change practices are underestimated in CLIMBER-X compared with the GCB, although the actual values remain uncertain (e.g. Gasser et al., 2020). A substantial fraction of this anthropogenic CO2 emission is absorbed by the ocean and the land, while the rest remains in the atmosphere. In CLIMBER-X, the ocean carbon uptake is a bit lower and the land carbon uptake a bit higher than GCB estimates, but the net effect is a realistic airborne fraction of carbon remaining in the atmosphere. The ocean carbon uptake is driven by the chemical disequilibrium between surface air CO2 concentrations and the concentration of dissolved CO2 in the surface ocean water and is relatively well understood, as also indicated by the narrow uncertainty range obtained from different CMIP6 models (Fig. 30a). The CLIMBER-X ocean carbon uptake falls within this narrow range, although it tends to be at the lower end. The land carbon uptake is largely driven by an increase in gross primary productivity as a response to increasing atmospheric CO2. The net primary productivity increase simulated by CLIMBER-X over the historical period is in agreement with what is shown by most CMIP6 models (Fig. 30b). However, the effect of this NPP increase on vegetation carbon varies widely among models (Fig. 30c), also because of the confounding factor of land use change. In CLIMBER-X the net effect is a vegetation carbon stock decrease by ∼ 10 PgC. The historical evolution of soil carbon additionally depends on the response of microbial decomposition to changing environmental conditions, particularly soil temperatures. The increasing NPP and consequently larger input of litter carbon into the soil dominate over the negative effect of increasing temperatures in CLIMBER-X, leading to an increase in soil carbon by ∼ 50 PgC (Fig. 30d).
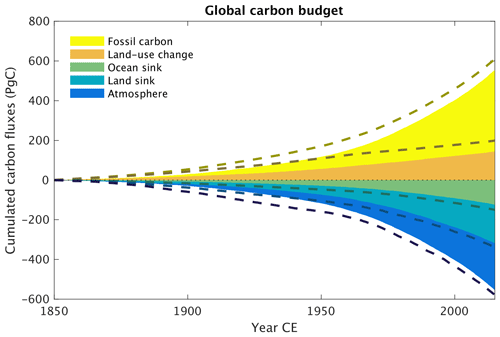
Figure 29Historical global carbon budget in CLIMBER-X. The dashed lines are estimates from Friedlingstein et al. (2022).
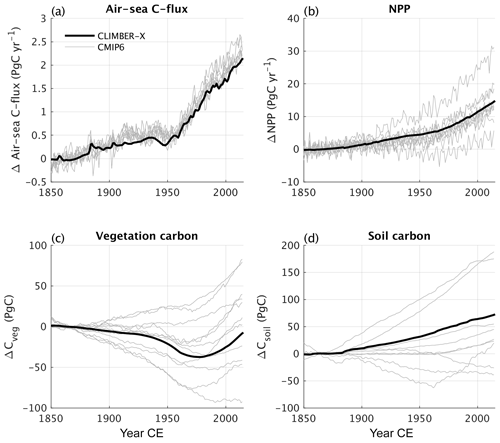
Figure 30Historical anomalies of (a) air–sea CO2 flux, (b) net primary production on land, (c) vegetation carbon, and (d) soil carbon in CLIMBER-X compared to CMIP6 models. The anomalies are computed relative to the time interval 1850–1880 CE.
Since CLIMBER-X is enabled with carbon isotopes, it also allows for a comparison of isotopic signatures to observations, thereby providing additional constraints on processes involved in carbon cycle exchanges. As an example, the historical δ13C of atmospheric CO2 is compared to observations in Fig. 31.
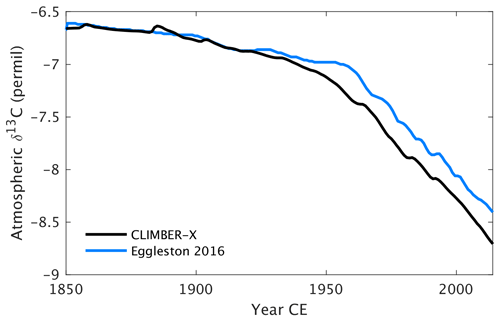
Figure 31Historical δ13C of atmospheric CO2 in CLIMBER-X compared with observations (Eggleston et al., 2016).
The general historical trend in atmospheric CH4 is captured by the model (Fig. 32a). Prescribed anthropogenic methane emissions are the dominant source for the increase in the atmospheric methane burden, but natural emissions from land are also increasing due to the increase in NPP and soil temperature (Fig. 32b).
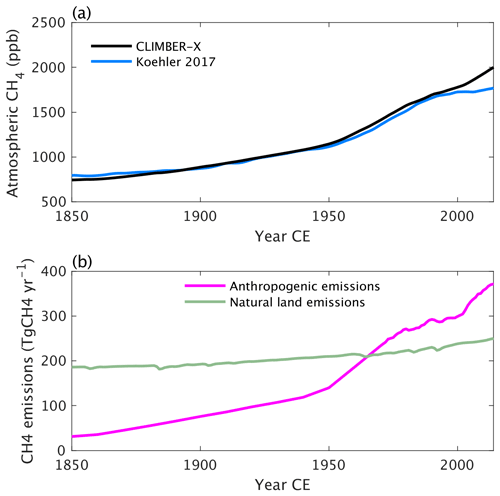
Figure 32Historical (a) atmospheric CH4 concentration in CLIMBER-X compared to observations (Köhler et al., 2017) and (b) prescribed anthropogenic CH4 emissions and natural land emissions as simulated in CLIMBER-X.
Carbon cycle processes both on land and in the ocean are sensitive to changes in climate and atmospheric CO2. This implies that carbon fluxes between ocean and atmosphere and between land and atmosphere will respond to changes in climate and CO2 concentration, which will in turn affect CO2 and consequently climate. Quantifying the strength of these feedbacks is important to understanding how the climate will respond to anthropogenic CO2 emissions. For that, a linear feedback decomposition analysis was proposed by Friedlingstein et al. (2006) to estimate these feedbacks in Earth system models. The analysis relies on a set of model simulations under idealized 1 % yr−1 CO2 increase experiments, whereby in one simulation the CO2 increase is seen only by the radiative code in the atmosphere (radiatively coupled), in another one the CO2 increase is seen only by the carbon cycle (biogeochemically coupled) and in a final one both the radiation and carbon cycle see the increase in atmospheric CO2 (fully coupled). This set of simulations allows us to roughly separate the effect of changes in climate and changes in CO2 on land and ocean carbon fluxes. To a first approximation, the carbon cycle feedback to climate is usually quantified using global temperature changes, while in reality climate obviously influences the carbon cycle in more complex ways than just through (global) temperature.
The carbon cycle feedback parameters have been estimated for the C4MIP, CMIP5 and CMIP6 models (Friedlingstein et al., 2006; Arora et al., 2013, 2020). In Fig. 33 the carbon cycle–climate (γ) and the carbon cycle–concentration (β) feedbacks in CLIMBER-X are compared to CMIP6 model results separately for land and ocean. An increase in CO2 has a positive effect on the uptake of carbon by both land and ocean, resulting in a lowering of CO2 and therefore a negative feedback on climate (implying positive β, Fig. 33a, c). Conversely, climate warming has a generally negative impact on the ability of ocean and land to store carbon, leading to a positive feedback loop (implying negative γ, Fig. 33b, d). The β and γ values obtained here are well within the range of estimates from CMIP6 models (Arora et al., 2020), although in CLIMBER-X the CO2 fertilization effect on land is rather high (Fig. 33a) and the ocean carbon uptake as a response to an increase in atmospheric CO2 is at the lower end of the CMIP6 models (Fig. 33c).
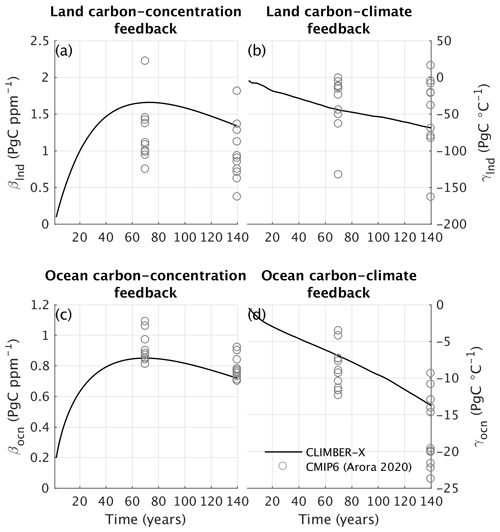
Figure 33Carbon cycle feedbacks in CLIMBER-X compared to CMIP6 models (Arora et al., 2020). The (a, c) β and (b, d) γ parameters are shown at the time of CO2 doubling (year ∼ 70) and at the time of CO2 quadrupling (year ∼ 140) in idealized 1 % yr−1 CO2 increase experiments.
The zero emissions commitment (ZEC) is a measure of the amount of additional future temperature change following a complete cessation of CO2 emissions (e.g. Matthews and Solomon, 2013). A model intercomparison project has been established in an effort to analyse and compare the ZEC in different Earth system models (Jones et al., 2019). Here we use this standardized and idealized experimental set-up to compare the carbon cycle response in CLIMBER-X with results from the Zero Emissions Commitment Model Intercomparison Project (ZECMIP) models for the 1000 PgC emission pulse (MacDougall et al., 2020). The experiment branches off from a 1 % per year CO2 increase run with CO2 emissions set to zero at the point of 1000 PgC of total carbon emissions. We performed this experiment with both the open and closed carbon cycle set-ups.
The results of the CLIMBER-X simulations are generally well within the large range of results from state-of-the-art ESMs and EMICs participating in ZECMIP (Fig. 34). Atmospheric CO2 concentration rapidly decreases after stopping the carbon emissions (Fig. 34b), while global temperature shows a more modest decrease (Fig. 34a). The ocean continues to take up carbon throughout the simulation (Fig. 34c), while the land turns from a sink to a source of carbon roughly at the time of the peak in CO2 (Fig. 34d). CLIMBER-X initially shows a relatively weak ocean carbon uptake compared to ZECMIP models, while the land carbon uptake is at the high end of the ZECMIP model ensemble.
The differences between the experiments with open and closed carbon cycle set-ups are negligible for the first few centuries but continue to grow over time, with CO2 decreasing faster in the open set-up (Fig. 34b).
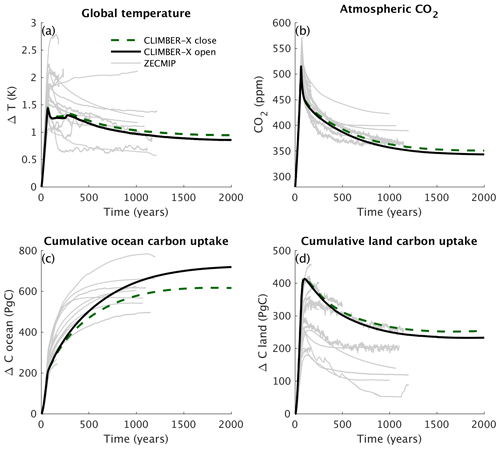
Figure 34Comparison of CLIMBER-X simulations with ZECMIP model results in terms of (a) global temperature, (b) atmospheric CO2 concentration, (c) cumulative ocean carbon uptake, and (d) cumulative land carbon uptake for the standard ZECMIP experiment with 1000 PgC cumulative carbon emissions. For CLIMBER-X, results with both the open (solid lines) and closed (dashed lines) carbon cycle set-ups are shown.
We have described the major features of the carbon cycle components of the newly developed CLIMBER-X Earth system model. The model includes a detailed representation of carbon cycle processes on land, in the ocean and in marine sediments, thus allowing the investigation of the complex interactions between climate and the carbon cycle. Two set-ups of the global carbon cycle, closed and open, are available in CLIMBER-X, allowing both a proper comparison with CMIP6-type models in centennial-scale and multi-millennium simulations. We have evaluated the model performance for the historical period against observations and state-of-the-art CMIP6 models, showing that many characteristics and feedbacks of the carbon cycle are reasonably well captured by the model. Biases in the simulated distribution of ocean biogeochemical tracers exist and can mostly be related to deficiencies in the simulated ocean circulation changes, which can at least partly be attributed to the comparatively coarse resolution of the ocean model and to the frictional–geostrophic approximation that it rests upon. On land, the carbon in soil layers below 1 m is underestimated, particularly in the permafrost zone, with possible implications for the land carbon cycle response to global warming.
Some possible directions for future model developments include the extension of the organic carbon cycle, allowing for burial on land and in sediments and fluxes from land to ocean, the refinement of the carbonate chemistry on shelves, including coral growth, and possibly the addition of the nitrogen cycle on land, which could be important for nitrogen limitation of photosynthesis and would allow for interactive atmospheric N2O, considering that N2O fluxes from the ocean are already available from the ocean biogeochemistry model HAMOCC.
The computational efficiency of CLIMBER-X will enable it to be used for systematic explorations of the coupled climate–carbon cycle evolution over timescales ranging from decades up to ∼ 100 000 years while also allowing a quantification of related uncertainties.
The source code of CLIMBER-X v1.0 is archived on Zenodo (https://doi.org/10.5281/zenodo.7898797, Willeit, 2023), with the exception of the HAMOCC model, which is covered by the Max Planck Institute for Meteorology software licence agreement as part of the MPI-ESM (https://code.mpimet.mpg.de/attachments/download/26986/MPI-ESM_SLA_v3.4.pdf, last access: 5 May 2023). CMIP6 model data are licensed under a Creative Commons Attribution-ShareAlike 4.0 International License (https://creativecommons.org/licenses, last access: 5 May 2023) and can be accessed through the Earth System Grid Federation (ESGF) nodes (for instance https://esgf-data.dkrz.de/search/cmip6-dkrz/, last access: 14 December 2022).
MW and AG designed the model. TI and BL provided the HAMOCC model code and assisted in the implementation of the model in CLIMBER-X. CH helped with the sediment model set-up and configuration. MP re-arranged HAMOCC into a column model for the purpose of integration into CLIMBER-X. MH implemented and tuned the particle-ballasting scheme. DD contributed to the improvements in the land carbon cycle model. VB and GM assisted in the implementation and set-up of the open carbon cycle. JB, JH and GRM developed the weathering model and contributed to its implementation in CLIMBER-X. MW coupled the different model components and tuned and tested the model. MW performed the model simulations, prepared the figures and wrote the paper, with contributions from all the authors.
The contact author has declared that none of the authors has any competing interests.
Publisher’s note: Copernicus Publications remains neutral with regard to jurisdictional claims in published maps and institutional affiliations.
Matteo Willeit, Bo Liu, Malte Heinemann and Janine Börker are funded by the German climate modelling project PalMod supported by the German Federal Ministry of Education and Research (BMBF) as a Research for Sustainability (FONA) initiative (grant nos. 01LP1920B, 01LP1917D, 01LP1919B, 01LP1919C and 01LP1920C). Guy Munhoven is a Research Associate with the Belgian Fund for Scientific Research – F.R.S.-FNRS. We thank Irene Stemmler for technical support in implementing HAMOCC in CLIMBER-X and Thomas Kleinen for discussions on the methane cycle. We thank the World Climate Research Programme, which, through its Working Group on Coupled Modelling, coordinated and promoted CMIP6. We thank the climate modelling groups for producing and making available their model output, the ESGF for archiving the data and providing access, and the multiple funding agencies who support CMIP6 and the ESGF. We thank Nuno Carvalhais for providing the soil and vegetation carbon dataset. The authors are grateful to the European Regional Development Fund (ERDF), the German Federal Ministry of Education and Research and the State of Brandenburg for supporting this project by providing resources on the high-performance computer system at the Potsdam Institute for Climate Impact Research.
This research has been supported by the Bundesministerium für Bildung und Forschung (PalMod project, grant nos. 01LP1920B, 01LP1917D,
01LP1919B, 01LP1919C and 01LP1920C).
The publication of this article was funded by the Open Access Fund of the Leibniz Association.
This paper was edited by Carlos Sierra and reviewed by two anonymous referees.
Abe-Ouchi, A., Saito, F., Kawamura, K., Raymo, M. E., Okuno, J., Takahashi, K., and Blatter, H.: Insolation-driven 100,000-year glacial cycles and hysteresis of ice-sheet volume., Nature, 500, 190–193, https://doi.org/10.1038/nature12374, 2013. a
Albani, S., Mahowald, N. M., Murphy, L. N., Raiswell, R., Moore, J. K., Anderson, R. F., McGee, D., Bradtmiller, L. I., Delmonte, B., Hesse, P. P., and Mayewski, P. A.: Paleodust variability since the Last Glacial Maximum and implications for iron inputs to the ocean, Geophys. Res. Lett., 43, 3944–3954, https://doi.org/10.1002/2016GL067911, 2016. a
Amiotte Suchet, P. and Probst, J. L.: A global model for present-day atmospheric/soil CO2 consumption by chemical erosion of continental rocks (GEM-CO2), Tellus B, 47, 273–280, https://doi.org/10.3402/tellusb.v47i1-2.16047, 1995. a
Amiotte Suchet, P., Probst, J.-L., and Ludwig, W.: Worldwide distribution of continental rock lithology: Implications for the atmospheric/soil CO2 uptake by continental weathering and alkalinity river transport to the oceans, Global Biogeochem. Cy., 17, 1038, https://doi.org/10.1029/2002GB001891, 2003. a
Andres, R. J., Boden, T. A., and Marland, G.: Annual Fossil-Fuel CO2 Emissions: Global Stable Carbon Isotopic Signature. Carbon Dioxide Information Analysis Center, Oak Ridge National Laboratory, U.S. Department of Energy, Oak Ridge, Tenn., U.S.A. [data set], https://doi.org/10.3334/CDIAC/ffe.db1013.2017, 2017. a
Archer, D. E. and Johnson, K.: A model of the iron cycle in the ocean, Global Biogeochem. Cy., 14, 269–279, https://doi.org/10.1029/1999GB900053, 2000. a
Arora, V. K., Boer, G. J., Friedlingstein, P., Eby, M., Jones, C. D., Christian, J. R., Bonan, G., Bopp, L., Brovkin, V., Cadule, P., Hajima, T., Ilyina, T., Lindsay, K., Tjiputra, J. F., and Wu, T.: Carbon-concentration and carbon-climate feedbacks in CMIP5 earth system models, J. Climate, 26, 5289–5314, https://doi.org/10.1175/JCLI-D-12-00494.1, 2013. a
Arora, V. K., Katavouta, A., Williams, R. G., Jones, C. D., Brovkin, V., Friedlingstein, P., Schwinger, J., Bopp, L., Boucher, O., Cadule, P., Chamberlain, M. A., Christian, J. R., Delire, C., Fisher, R. A., Hajima, T., Ilyina, T., Joetzjer, E., Kawamiya, M., Koven, C. D., Krasting, J. P., Law, R. M., Lawrence, D. M., Lenton, A., Lindsay, K., Pongratz, J., Raddatz, T., Séférian, R., Tachiiri, K., Tjiputra, J. F., Wiltshire, A., Wu, T., and Ziehn, T.: Carbon–concentration and carbon–climate feedbacks in CMIP6 models and their comparison to CMIP5 models, Biogeosciences, 17, 4173–4222, https://doi.org/10.5194/bg-17-4173-2020, 2020. a, b, c
Augustin, L., Barbante, C., Barnes, P. R. F., Barnola, J. M., Bigler, M., Castellano, E., Cattani, O., Chappellaz, J., Dahl-Jensen, D., Delmonte, B., Dreyfus, G., Durand, G., Falourd, S., Fischer, H., Flückiger, J., Hansson, M. E., Huybrechts, P., Jugie, G., Johnsen, S. J., Jouzel, J., Kaufmann, P., Kipfstuhl, J., Lambert, F., Lipenkov, V. Y., Littot, G. C., Longinelli, A., Lorrain, R., Maggi, V., Masson-Delmotte, V., Miller, H., Mulvaney, R., Oerlemans, J., Oerter, H., Orombelli, G., Parrenin, F., Peel, D. a., Petit, J.-R., Raynaud, D., Ritz, C., Ruth, U., Schwander, J., Siegenthaler, U., Souchez, R., Stauffer, B., Steffensen, J. P., Stenni, B., Stocker, T. F., Tabacco, I. E., Udisti, R., Van De Wal, R. S. W., Van Den Broeke, M., Weiss, J., Wilhelms, F., Winther, J.-G., Wolff, E. W., and Zucchelli, M.: Eight glacial cycles from an Antarctic ice core., Nature, 429, 623–628, https://doi.org/10.1038/nature02599, 2004. a
Aumont, O., Orr, J. C., Monfray, P., Madec, G., and Maier-Reimer, E.: Nutrient trapping in the equatorial Pacific: The ocean circulation solution, Global Biogeochem. Cy., 13, 351–369, https://doi.org/10.1029/1998GB900012, 1999. a
Aumont, O., Ethé, C., Tagliabue, A., Bopp, L., and Gehlen, M.: PISCES-v2: an ocean biogeochemical model for carbon and ecosystem studies, Geosci. Model Dev., 8, 2465–2513, https://doi.org/10.5194/gmd-8-2465-2015, 2015. a
Batjes, N.: Harmonized soil property values for broad-scale modelling (WISE30sec) with estimates of global soil carbon stocks, Geoderma, 269, 61–68, https://doi.org/10.1016/j.geoderma.2016.01.034, 2016. a
Battaglia, G., Steinacher, M., and Joos, F.: A probabilistic assessment of calcium carbonate export and dissolution in the modern ocean, Biogeosciences, 13, 2823–2848, https://doi.org/10.5194/bg-13-2823-2016, 2016. a
Beer, C., Reichstein, M., Tomelleri, E., Ciais, P., Jung, M., Carvalhais, N., Rodenbeck, C., Arain, M. A., Baldocchi, D., Bonan, G. B., Bondeau, A., Cescatti, A., Lasslop, G., Lindroth, A., Lomas, M., Luyssaert, S., Margolis, H., Oleson, K. W., Roupsard, O., Veenendaal, E., Viovy, N., Williams, C., Woodward, F. I., and Papale, D.: Terrestrial Gross Carbon Dioxide Uptake: Global Distribution and Covariation with Climate, Science, 329, 834–838, https://doi.org/10.1126/science.1184984, 2010. a
Börker, J., Hartmann, J., Amann, T., Romero-Mujalli, G., Moosdorf, N., and Jenkins, C.: Chemical Weathering of Loess and Its Contribution to Global Alkalinity Fluxes to the Coastal Zone During the Last Glacial Maximum, Mid-Holocene, and Present, Geochem. Geophy. Geosy., 21, e2020GC008922, https://doi.org/10.1029/2020GC008922, 2020. a, b, c
Bouttes, N., Roche, D. M., Mariotti, V., and Bopp, L.: Including an ocean carbon cycle model into iLOVECLIM (v1.0), Geosci. Model Dev., 8, 1563–1576, https://doi.org/10.5194/gmd-8-1563-2015, 2015. a
Brovkin, V., Bendtsen, J., Claussen, M., Ganopolski, A., Kubatzki, C., Petoukhov, V., and Andreev, A.: Carbon cycle, vegetation, and climate dynamics in the Holocene: Experiments with the CLIMBER-2 model, Global Biogeochem. Cy., 16, 1139, https://doi.org/10.1029/2001GB001662, 2002. a
Brovkin, V., Ganopolski, A., Archer, D., and Rahmstorf, S.: Lowering of glacial atmospheric CO2 in response to changes in oceanic circulation and marine biogeochemistry, Paleoceanography, 22, PA4202, https://doi.org/10.1029/2006PA001380, 2007. a
Brovkin, V., Ganopolski, A., Archer, D., and Munhoven, G.: Glacial CO2 cycle as a succession of key physical and biogeochemical processes, Clim. Past, 8, 251–264, https://doi.org/10.5194/cp-8-251-2012, 2012. a
Brown, J., Ferrians, O., Heginbottom, J. A., and Melnikov, E.: Circum-Arctic Map of Permafrost and Ground-Ice Conditions, National Snow and Ice Data Center [data set], https://nsidc.org/data/ggd318/versions/2 (last access: 20 January 2022), 1998. a
Buitenhuis, E. T., Suntharalingam, P., and Le Quéré, C.: Constraints on global oceanic emissions of N2O from observations and models, Biogeosciences, 15, 2161–2175, https://doi.org/10.5194/bg-15-2161-2018, 2018. a
Burton, C., Betts, R., Cardoso, M., Feldpausch, T. R., Harper, A., Jones, C. D., Kelley, D. I., Robertson, E., and Wiltshire, A.: Representation of fire, land-use change and vegetation dynamics in the Joint UK Land Environment Simulator vn4.9 (JULES), Geosci. Model Dev., 12, 179–193, https://doi.org/10.5194/gmd-12-179-2019, 2019. a
Cabré, A., Marinov, I., Bernardello, R., and Bianchi, D.: Oxygen minimum zones in the tropical Pacific across CMIP5 models: mean state differences and climate change trends, Biogeosciences, 12, 5429–5454, https://doi.org/10.5194/bg-12-5429-2015, 2015. a
Cao, L., Eby, M., Ridgwell, A., Caldeira, K., Archer, D., Ishida, A., Joos, F., Matsumoto, K., Mikolajewicz, U., Mouchet, A., Orr, J. C., Plattner, G.-K., Schlitzer, R., Tokos, K., Totterdell, I., Tschumi, T., Yamanaka, Y., and Yool, A.: The role of ocean transport in the uptake of anthropogenic CO2, Biogeosciences, 6, 375–390, https://doi.org/10.5194/bg-6-375-2009, 2009. a
Carr, M.-E., Friedrichs, M. A., Schmeltz, M., Noguchi Aita, M., Antoine, D., Arrigo, K. R., Asanuma, I., Aumont, O., Barber, R., Behrenfeld, M., Bidigare, R., Buitenhuis, E. T., Campbell, J., Ciotti, A., Dierssen, H., Dowell, M., Dunne, J., Esaias, W., Gentili, B., Gregg, W., Groom, S., Hoepffner, N., Ishizaka, J., Kameda, T., Le Quéré, C., Lohrenz, S., Marra, J., Mélin, F., Moore, K., Morel, A., Reddy, T. E., Ryan, J., Scardi, M., Smyth, T., Turpie, K., Tilstone, G., Waters, K., and Yamanaka, Y.: A comparison of global estimates of marine primary production from ocean color, Deep Sea Research Part II: Topical Studies in Oceanography, 53, 741–770, https://doi.org/10.1016/j.dsr2.2006.01.028, 2006. a
Cartapanis, O., Galbraith, E. D., Bianchi, D., and Jaccard, S. L.: Carbon burial in deep-sea sediment and implications for oceanic inventories of carbon and alkalinity over the last glacial cycle, Clim. Past, 14, 1819–1850, https://doi.org/10.5194/cp-14-1819-2018, 2018. a, b
Carvalhais, N., Forkel, M., Khomik, M., Bellarby, J., Jung, M., Migliavacca, M., Mu, M., Saatchi, S., Santoro, M., Thurner, M., Weber, U., Ahrens, B., Beer, C., Cescatti, A., Randerson, J. T., and Reichstein, M.: Global covariation of carbon turnover times with climate in terrestrial ecosystems, Nature, 514, 213–217, https://doi.org/10.1038/nature13731, 2014. a, b, c
Chen, F. and Zhang, Y.: On the coupling strength between the land surface and the atmosphere: From viewpoint of surface exchange coefficients, Geophys. Res. Lett., 36, L10404, https://doi.org/10.1029/2009GL037980, 2009. a
Collatz, G., Ball, J., Grivet, C., and Berry, J. A.: Physiological and environmental regulation of stomatal conductance, photosynthesis and transpiration: a model that includes a laminar boundary layer, Agr. Forest Meteorol., 54, 107–136, https://doi.org/10.1016/0168-1923(91)90002-8, 1991. a
Crichton, K. A., Wilson, J. D., Ridgwell, A., and Pearson, P. N.: Calibration of temperature-dependent ocean microbial processes in the cGENIE.muffin (v0.9.13) Earth system model, Geosci. Model Dev., 14, 125–149, https://doi.org/10.5194/gmd-14-125-2021, 2021. a
Denman, K. L., Brasseur, G., Chidthaisong, A., Ciais, P., Cox, P. M., Dickinson, R. E., Hauglustaine, D., Heinze, C., Holland, E., Jacob, D., Lohmann, U., Ramachandran, S., da Silva Dias, P. L., Wofsy, S. C., and Zhang, X.: Couplings Between Changes in the Climate System and Biogeochemistry, in: Climate Change 2007: The Physical Science Basis. Contribution of Working Group I to the Fourth Assessment Report of the Intergovernmental Panel on Climate Change, edited by: Solomon, S., Qin, D., Manning, M., Marquis, M., Averyt, K., Tignor, M. M. B., Jr., H. L. M., and Chen, Z., chap. 7, Cambridge University Press, Cambridge (UK), 499–587, https://www.ipcc.ch/site/assets/uploads/2018/02/ar4-wg1-chapter7-1.pdf (last access: 6 September 2022), 2007. a
Derry, L. A. and France-Lanord, C.: Neogene growth of the sedimentary organic carbon reservoir, Paleoceanography, 11, 267–275, https://doi.org/10.1029/95PA03839, 1996. a
Dietze, H. and Loeptien, U.: Revisiting “nutrient trapping” in global coupled biogeochemical ocean circulation models, Global Biogeochem. Cy., 27, 265–284, https://doi.org/10.1002/gbc.20029, 2013. a
Dunne, J. P., Sarmiento, J. L., and Gnanadesikan, A.: A synthesis of global particle export from the surface ocean and cycling through the ocean interior and on the seafloor, Global Biogeochem. Cy., 21, GB4006, https://doi.org/10.1029/2006GB002907, 2007. a, b, c
Dutay, J.-C., Bullister, J., Doney, S., Orr, J., Najjar, R., Caldeira, K., Campin, J.-M., Drange, H., Follows, M., Gao, Y., Gruber, N., Hecht, M., Ishida, A., Joos, F., Lindsay, K., Madec, G., Maier-Reimer, E., Marschall, J., Matear, R., Monfray, P., Mouchet, A., Plattner, G.-K., Sarmiento, J., Schlitzer, R., Slater, R., Totterdell, I., Weirig, M.-F., Yamanaka, Y., and Yool, A.: Evaluation of ocean model ventilation with CFC-11: comparison of 13 global ocean models, Ocean Model., 4, 89–120, https://doi.org/10.1016/S1463-5003(01)00013-0, 2002. a, b
Eby, M., Zickfeld, K., Montenegro, A., Archer, D., Meissner, K. J., and Weaver, A. J.: Lifetime of anthropogenic climate change: Millennial time scales of potential CO2 and surface temperature perturbations, J. Climate, 22, 2501–2511, https://doi.org/10.1175/2008JCLI2554.1, 2009. a
Eggleston, S., Schmitt, J., Bereiter, B., Schneider, R., and Fischer, H.: Evolution of the stable carbon isotope composition of atmospheric CO2 over the last glacial cycle, Paleoceanography, 31, 434–452, https://doi.org/10.1002/2015PA002874, 2016. a
Eide, M., Olsen, A., Ninnemann, U. S., and Johannessen, T.: A global ocean climatology of preindustrial and modern ocean δ13C, Global Biogeochem. Cy., 31, 515–534, https://doi.org/10.1002/2016GB005473, 2017. a
Eyring, V., Bony, S., Meehl, G. A., Senior, C. A., Stevens, B., Stouffer, R. J., and Taylor, K. E.: Overview of the Coupled Model Intercomparison Project Phase 6 (CMIP6) experimental design and organization, Geosci. Model Dev., 9, 1937–1958, https://doi.org/10.5194/gmd-9-1937-2016, 2016. a
Fan, N., Koirala, S., Reichstein, M., Thurner, M., Avitabile, V., Santoro, M., Ahrens, B., Weber, U., and Carvalhais, N.: Apparent ecosystem carbon turnover time: uncertainties and robust features, Earth Syst. Sci. Data, 12, 2517–2536, https://doi.org/10.5194/essd-12-2517-2020, 2020. a, b, c, d, e, f
Farquhar, G. D., von Caemmerer, S., and Berry, J. A.: A biochemical model of photosynthetic CO2 assimilation in leaves of C3 species, Planta, 149, 78–90, https://doi.org/10.1007/BF00386231, 1980. a
Friedlingstein, P., Cox, P., Betts, R., Bopp, L., von Bloh, W., Brovkin, V., Cadule, P., Doney, S., Eby, M., Fung, I., Bala, G., John, J., Jones, C., Joos, F., Kato, T., Kawamiya, M., Knorr, W., Lindsay, K., Matthews, H. D., Raddatz, T., Rayner, P., Reick, C., Roeckner, E., Schnitzler, K.-G., Schnur, R., Strassmann, K., Weaver, A. J., Yoshikawa, C., and Zeng, N.: Climate–Carbon Cycle Feedback Analysis: Results from the C4MIP Model Intercomparison, J. Climate, 19, 3337–3353, https://doi.org/10.1175/JCLI3800.1, 2006. a, b
Friedlingstein, P., Meinshausen, M., Arora, V. K., Jones, C. D., Anav, A., Liddicoat, S. K., and Knutti, R.: Uncertainties in CMIP5 climate projections due to carbon cycle feedbacks, J. Climate, 27, 511–526, https://doi.org/10.1175/JCLI-D-12-00579.1, 2014. a
Friedlingstein, P., Jones, M. W., O'Sullivan, M., Andrew, R. M., Bakker, D. C. E., Hauck, J., Le Quéré, C., Peters, G. P., Peters, W., Pongratz, J., Sitch, S., Canadell, J. G., Ciais, P., Jackson, R. B., Alin, S. R., Anthoni, P., Bates, N. R., Becker, M., Bellouin, N., Bopp, L., Chau, T. T. T., Chevallier, F., Chini, L. P., Cronin, M., Currie, K. I., Decharme, B., Djeutchouang, L. M., Dou, X., Evans, W., Feely, R. A., Feng, L., Gasser, T., Gilfillan, D., Gkritzalis, T., Grassi, G., Gregor, L., Gruber, N., Gürses, Ö., Harris, I., Houghton, R. A., Hurtt, G. C., Iida, Y., Ilyina, T., Luijkx, I. T., Jain, A., Jones, S. D., Kato, E., Kennedy, D., Klein Goldewijk, K., Knauer, J., Korsbakken, J. I., Körtzinger, A., Landschützer, P., Lauvset, S. K., Lefèvre, N., Lienert, S., Liu, J., Marland, G., McGuire, P. C., Melton, J. R., Munro, D. R., Nabel, J. E. M. S., Nakaoka, S.-I., Niwa, Y., Ono, T., Pierrot, D., Poulter, B., Rehder, G., Resplandy, L., Robertson, E., Rödenbeck, C., Rosan, T. M., Schwinger, J., Schwingshackl, C., Séférian, R., Sutton, A. J., Sweeney, C., Tanhua, T., Tans, P. P., Tian, H., Tilbrook, B., Tubiello, F., van der Werf, G. R., Vuichard, N., Wada, C., Wanninkhof, R., Watson, A. J., Willis, D., Wiltshire, A. J., Yuan, W., Yue, C., Yue, X., Zaehle, S., and Zeng, J.: Global Carbon Budget 2021, Earth Syst. Sci. Data, 14, 1917–2005, https://doi.org/10.5194/essd-14-1917-2022, 2022. a, b, c
Gaillardet, J., Dupré, B., Louvat, P., and Allègre, C.: Global silicate weathering and CO2 consumption rates deduced from the chemistry of large rivers, Chem. Geol., 159, 3–30, https://doi.org/10.1016/S0009-2541(99)00031-5, 1999. a
Ganopolski, A. and Brovkin, V.: Simulation of climate, ice sheets and CO2 evolution during the last four glacial cycles with an Earth system model of intermediate complexity, Clim. Past, 13, 1695–1716, https://doi.org/10.5194/cp-13-1695-2017, 2017. a
Ganopolski, A. and Calov, R.: The role of orbital forcing, carbon dioxide and regolith in 100 kyr glacial cycles, Clim. Past, 7, 1415–1425, https://doi.org/10.5194/cp-7-1415-2011, 2011. a
Garcia, H. E., Locarnini, R. A., Boyer, T. P., Antonov, J. I., Mishonov, A. V., Baranova, O. K., Zweng, O. K., Reagan, J. R., and Johnson, D. R.: World Ocean Atlas 2013. Vol. 3: Dissolved Oxygen, Apparent Oxygen Utilization, and Oxygen Saturation, edited by: Levitus, S., Technical Editor: Mishonov, A., NOAA Atlas NESDIS 75, 27 pp. https://doi.org/10.7289/V5XG9P2W, 2013. a, b
Garcia, H. E., Locarnini, R. A., Boyer, T. P., Antonov, J. I., Baranova, O. K., Zweng, M. M., Reagan, J. R., and Johnson, D. R.: World Ocean Atlas 2013. Vol. 4: Dissolved Inorganic Nutrients (phosphate, nitrate, silicate), edited by: Levitus, S., Technical Editor: Mishonov, A., NOAA Atlas NESDIS 76, 25 pp., https://doi.org/10.7289/V5J67DWD, 2013. a, b, c, d, e, f, g, h, i
Gasser, T., Crepin, L., Quilcaille, Y., Houghton, R. A., Ciais, P., and Obersteiner, M.: Historical CO2 emissions from land use and land cover change and their uncertainty, Biogeosciences, 17, 4075–4101, https://doi.org/10.5194/bg-17-4075-2020, 2020. a
Gehlen, M., Bopp, L., Emprin, N., Aumont, O., Heinze, C., and Ragueneau, O.: Reconciling surface ocean productivity, export fluxes and sediment composition in a global biogeochemical ocean model, Biogeosciences, 3, 521–537, https://doi.org/10.5194/bg-3-521-2006, 2006. a
Ginoux, P., Chin, M., Tegen, I., Prospero, J. M., Holben, B., Dubovik, O., and Lin, S. J.: Sources and distributions of dust aerosols simulated with the GOCART model, J. Geophys. Res.-Atmos., 106, 20255–20273, https://doi.org/10.1029/2000JD000053, 2001. a
Goosse, H., Brovkin, V., Fichefet, T., Haarsma, R., Huybrechts, P., Jongma, J., Mouchet, A., Selten, F., Barriat, P.-Y., Campin, J.-M., Deleersnijder, E., Driesschaert, E., Goelzer, H., Janssens, I., Loutre, M.-F., Morales Maqueda, M. A., Opsteegh, T., Mathieu, P.-P., Munhoven, G., Pettersson, E. J., Renssen, H., Roche, D. M., Schaeffer, M., Tartinville, B., Timmermann, A., and Weber, S. L.: Description of the Earth system model of intermediate complexity LOVECLIM version 1.2, Geosci. Model Dev., 3, 603–633, https://doi.org/10.5194/gmd-3-603-2010, 2010. a
Großkopf, T., Mohr, W., Baustian, T., Schunck, H., Gill, D., Kuypers, M. M. M., Lavik, G., Schmitz, R. A., Wallace, D. W. R., and LaRoche, J.: Doubling of marine dinitrogen-fixation rates based on direct measurements, Nature, 488, 361–364, https://doi.org/10.1038/nature11338, 2012. a
Gruber, N., Gloor, M., Mikaloff Fletcher, S. E., Doney, S. C., Dutkiewicz, S., Follows, M. J., Gerber, M., Jacobson, A. R., Joos, F., Lindsay, K., Menemenlis, D., Mouchet, A., Müller, S. A., Sarmiento, J. L., and Takahashi, T.: Oceanic sources, sinks, and transport of atmospheric CO2, Global Biogeochem. Cy., 23, GB1005, https://doi.org/10.1029/2008GB003349, 2009. a
Gulev, S., Thorne, P., Ahn, J., Dentener, F., Domingues, C., Gerland, S., Gong, D., Kaufman, D., Nnamchi, H., Quaas, J., Rivera, J., Sathyendranath, S., Smith, S., Trewin, B., von Schuckmann, K., and Vose, R.: Chapter 2: Changing state of the climate system, in: Climate Change 2021: The Physical Science Basis. Contribution of Working Group I to the Sixth Assessment Report of the Intergovernmental Panel on Climate Change, edited by: Masson-Delmotte, V., Zhai, P., Pirani, A., Connors, S., Péan, C., Berger, S., Caud, N., Chen, Y., Goldfarb, L., Gomis, M., Huang, M., Leitzell, K., Lonnoy, E., Matthews, J., Maycock, T., Waterfield, T., Yelekçi, O., Yu, R., and Zhou, B., Cambridge University Press, Cambridge, United Kingdom and New York, NY, USA, 287–422, https://doi.org/10.1017/9781009157896.004, 2021. a
Hartmann, J.: Bicarbonate-fluxes and CO2-consumption by chemical weathering on the Japanese Archipelago – Application of a multi-lithological model framework, Chem. Geol., 265, 237–271, https://doi.org/10.1016/j.chemgeo.2009.03.024, 2009. a, b
Hartmann, J. and Moosdorf, N.: The new global lithological map database GLiM: A representation of rock properties at the Earth surface, Geochem. Geophy. Geosy., 13, Q12004, https://doi.org/10.1029/2012GC004370, 2012. a
Hartmann, J., Moosdorf, N., Lauerwald, R., Hinderer, M., and West, A. J.: Global chemical weathering and associated P-release – the role of lithology, temperature and soil properties, Chem. Geol., 363, 145–163, https://doi.org/10.1016/j.chemgeo.2013.10.025, 2014. a, b
Hauck, J., Völker, C., Wang, T., Hoppema, M., Losch, M., and Wolf-Gladrow, D. A.: Seasonally different carbon flux changes in the Southern Ocean in response to the southern annular mode, Global Biogeochem. Cy., 27, 1236–1245, https://doi.org/10.1002/2013GB004600, 2013. a
Haxeltine, A. and Prentice, I. C.: A General Model for the Light-Use Efficiency of Primary Production, Funct. Ecol., 10, 551–561, https://doi.org/10.2307/2390165, 1996. a
Hayes, C. T., Costa, K. M., Anderson, R. F., Calvo, E., Chase, Z., Demina, L. L., Dutay, J. C., German, C. R., Heimbürger-Boavida, L. E., Jaccard, S. L., Jacobel, A., Kohfeld, K. E., Kravchishina, M. D., Lippold, J., Mekik, F., Missiaen, L., Pavia, F. J., Paytan, A., Pedrosa-Pamies, R., Petrova, M. V., Rahman, S., Robinson, L. F., Roy-Barman, M., Sanchez-Vidal, A., Shiller, A., Tagliabue, A., Tessin, A. C., van Hulten, M., and Zhang, J.: Global Ocean Sediment Composition and Burial Flux in the Deep Sea, Global Biogeochem. Cy., 35, e2020GB006769, https://doi.org/10.1029/2020GB006769, 2021. a
Heinemann, M., Segschneider, J., and Schneider, B.: CO2 drawdown due to particle ballasting by glacial aeolian dust: an estimate based on the ocean carbon cycle model MPIOM/HAMOCC version 1.6.2p3, Geosci. Model Dev., 12, 1869–1883, https://doi.org/10.5194/gmd-12-1869-2019, 2019. a, b
Heinze, C., Maier-Reimer, E., Winguth, A. M. E., and Archer, D.: A global oceanic sediment model for long-term climate studies, Global Biogeochem. Cy., 13, 221–250, https://doi.org/10.1029/98GB02812, 1999. a, b
Hoffman, F. M., Randerson, J. T., Arora, V. K., Bao, Q., Cadule, P., Ji, D., Jones, C. D., Kawamiya, M., Khatiwala, S., Lindsay, K., Obata, A., Shevliakova, E., Six, K. D., Tjiputra, J. F., Volodin, E. M., and Wu, T.: Causes and implications of persistent atmospheric carbon dioxide biases in Earth System Models, J. Geophys. Res.-Biogeo., 119, 141–162, https://doi.org/10.1002/2013JG002381, 2014. a
Hopcroft, P. O., Valdes, P. J., Woodward, S., and Joshi, M. M.: Last glacial maximum radiative forcing from mineral dust aerosols in an Earth system model, J. Geophys. Res.-Atmos., 120, 8186–8205, https://doi.org/10.1002/2015JD023742, 2015. a
Hopcroft, P. O., Valdes, P. J., O'Connor, F. M., Kaplan, J. O., and Beerling, D. J.: Understanding the glacial methane cycle, Nat. Commun., 8, 14383, https://doi.org/10.1038/ncomms14383, 2017. a
Hugelius, G., Strauss, J., Zubrzycki, S., Harden, J. W., Schuur, E. A. G., Ping, C.-L., Schirrmeister, L., Grosse, G., Michaelson, G. J., Koven, C. D., O'Donnell, J. A., Elberling, B., Mishra, U., Camill, P., Yu, Z., Palmtag, J., and Kuhry, P.: Estimated stocks of circumpolar permafrost carbon with quantified uncertainty ranges and identified data gaps, Biogeosciences, 11, 6573–6593, https://doi.org/10.5194/bg-11-6573-2014, 2014. a
Huneeus, N., Schulz, M., Balkanski, Y., Griesfeller, J., Prospero, J., Kinne, S., Bauer, S., Boucher, O., Chin, M., Dentener, F., Diehl, T., Easter, R., Fillmore, D., Ghan, S., Ginoux, P., Grini, A., Horowitz, L., Koch, D., Krol, M. C., Landing, W., Liu, X., Mahowald, N., Miller, R., Morcrette, J.-J., Myhre, G., Penner, J., Perlwitz, J., Stier, P., Takemura, T., and Zender, C. S.: Global dust model intercomparison in AeroCom phase I, Atmos. Chem. Phys., 11, 7781–7816, https://doi.org/10.5194/acp-11-7781-2011, 2011. a
Ilyina, T., Six, K. D., Segschneider, J., Maier-Reimer, E., Li, H., and Núñez-Riboni, I.: Global ocean biogeochemistry model HAMOCC: Model architecture and performance as component of the MPI-Earth system model in different CMIP5 experimental realizations, J. Adv. Model. Earth Sy., 5, 287–315, https://doi.org/10.1029/2012MS000178, 2013. a, b, c, d
Ito, A.: A historical meta-analysis of global terrestrial net primary productivity: Are estimates converging?, Glob. Change Biol., 17, 3161–3175, https://doi.org/10.1111/j.1365-2486.2011.02450.x, 2011. a
Jacobson, A. R., Fletcher, S. E., Gruber, N., Sarmiento, J. L., and Gloor, M.: A joint atmosphere-ocean inversion for surface fluxes of carbon dioxide: 1. Methods and global-scale fluxes, Global Biogeochem. Cy., 21, GB1019, https://doi.org/10.1029/2005GB002556, 2007. a
Johnson, K. S. and Bif, M. B.: Constraint on net primary productivity of the global ocean by Argo oxygen measurements, Nat. Geosci., 14, 769–774, https://doi.org/10.1038/s41561-021-00807-z, 2021. a
Jones, C. D., Frölicher, T. L., Koven, C., MacDougall, A. H., Matthews, H. D., Zickfeld, K., Rogelj, J., Tokarska, K. B., Gillett, N. P., Ilyina, T., Meinshausen, M., Mengis, N., Séférian, R., Eby, M., and Burger, F. A.: The Zero Emissions Commitment Model Intercomparison Project (ZECMIP) contribution to C4MIP: quantifying committed climate changes following zero carbon emissions, Geosci. Model Dev., 12, 4375–4385, https://doi.org/10.5194/gmd-12-4375-2019, 2019. a
Jung, M., Reichstein, M., Margolis, H. a., Cescatti, A., Richardson, A. D., Arain, M. A., Arneth, A., Bernhofer, C., Bonal, D., Chen, J., Gianelle, D., Gobron, N., Kiely, G., Kutsch, W., Lasslop, G., Law, B. E., Lindroth, A., Merbold, L., Montagnani, L., Moors, E. J., Papale, D., Sottocornola, M., Vaccari, F., and Williams, C.: Global patterns of land-atmosphere fluxes of carbon dioxide, latent heat, and sensible heat derived from eddy covariance, satellite, and meteorological observations, J. Geophys. Res.-Biogeo., 116, G00J07, https://doi.org/10.1029/2010JG001566, 2011. a
Karl, D., Michaels, A., Bergman, B., Capone, D., Carpenter, E., Letelier, R., Lipschultz, F., Paerl, H., Sigman, D., and Stal, L.: Dinitrogen fixation in the world's oceans, Biogeochemistry, 57, 47–98, https://doi.org/10.1023/A:1015798105851, 2002. a
Key, R. M., Kozyr, A., Sabine, C. L., Lee, K., Wanninkhof, R., Bullister, J. L., Feely, R. A., Millero, F. J., Mordy, C., and Peng, T. H.: A global ocean carbon climatology: Results from Global Data Analysis Project (GLODAP), Global Biogeochem. Cy., 18, GB4031, https://doi.org/10.1029/2004GB002247, 2004. a, b
Kleinen, T. and Brovkin, V.: Pathway-dependent fate of permafrost region carbon, Environ. Res. Lett., 13, 094001, https://doi.org/10.1088/1748-9326/aad824, 2018. a
Kleinen, T., Mikolajewicz, U., and Brovkin, V.: Terrestrial methane emissions from the Last Glacial Maximum to the preindustrial period, Clim. Past, 16, 575–595, https://doi.org/10.5194/cp-16-575-2020, 2020. a, b, c
Köhler, P., Nehrbass-Ahles, C., Schmitt, J., Stocker, T. F., and Fischer, H.: A 156 kyr smoothed history of the atmospheric greenhouse gases CO2, CH4, and N2O and their radiative forcing, Earth Syst. Sci. Data, 9, 363–387, https://doi.org/10.5194/essd-9-363-2017, 2017. a, b, c
Koven, C. D., Riley, W. J., Subin, Z. M., Tang, J. Y., Torn, M. S., Collins, W. D., Bonan, G. B., Lawrence, D. M., and Swenson, S. C.: The effect of vertically resolved soil biogeochemistry and alternate soil C and N models on C dynamics of CLM4, Biogeosciences, 10, 7109–7131, https://doi.org/10.5194/bg-10-7109-2013, 2013. a
Kriest, I. and Evans, G. T.: A vertically resolved model for phytoplankton aggregation, J. Earth Syst. Sci., 109, 453–469, https://doi.org/10.1007/BF02708333, 2000. a
Kurahashi-Nakamura, T., Paul, A., Merkel, U., and Schulz, M.: Glacial state of the global carbon cycle: time-slice simulations for the last glacial maximum with an Earth-system model, Clim. Past, 18, 1997–2019, https://doi.org/10.5194/cp-18-1997-2022, 2022. a
Lambert, F., Tagliabue, A., Shaffer, G., Lamy, F., Winckler, G., Farias, L., Gallardo, L., and De Pol-Holz, R.: Dust fluxes and iron fertilization in Holocene and Last Glacial Maximum climates, Geophys. Res. Lett., 42, 6014–6023, https://doi.org/10.1002/2015GL064250, 2015. a
Landschützer, P., Gruber, N., and Bakker, D. C.: Decadal variations and trends of the global ocean carbon sink, Global Biogeochem. Cy., 30, 1396–1417, https://doi.org/10.1002/2015GB005359, 2016. a, b
Laufkötter, C., John, J. G., Stock, C. A., and Dunne, J. P.: Temperature and oxygen dependence of the remineralization of organic matter, Global Biogeochem. Cy., 31, 1038–1050, https://doi.org/10.1002/2017GB005643, 2017. a
Lauvset, S. K., Key, R. M., Olsen, A., van Heuven, S., Velo, A., Lin, X., Schirnick, C., Kozyr, A., Tanhua, T., Hoppema, M., Jutterström, S., Steinfeldt, R., Jeansson, E., Ishii, M., Perez, F. F., Suzuki, T., and Watelet, S.: A new global interior ocean mapped climatology: the 1∘ × 1∘ GLODAP version 2, Earth Syst. Sci. Data, 8, 325–340, https://doi.org/10.5194/essd-8-325-2016, 2016. a, b, c, d, e
Lavergne, A., Voelker, S., Csank, A., Graven, H., de Boer, H. J., Daux, V., Robertson, I., Dorado‐Liñán, I., Martínez‐Sancho, E., Battipaglia, G., Bloomfield, K. J., Still, C. J., Meinzer, F. C., Dawson, T. E., Camarero, J. J., Clisby, R., Fang, Y., Menzel, A., Keen, R. M., Roden, J. S., and Prentice, I. C.: Historical changes in the stomatal limitation of photosynthesis: empirical support for an optimality principle, New Phytol., 225, 2484–2497, https://doi.org/10.1111/nph.16314, 2019. a, b
Lavergne, A., Sandoval, D., Hare, V. J., Graven, H., and Prentice, I. C.: Impacts of soil water stress on the acclimated stomatal limitation of photosynthesis: Insights from stable carbon isotope data, Glob. Change Biol., 26, 7158–7172, https://doi.org/10.1111/gcb.15364, 2020. a
Levine, J. G., Wolff, E. W., Jones, A. E., Sime, L. C., Valdes, P. J., Archibald, A. T., Carver, G. D., Warwick, N. J., and Pyle, J. A.: Reconciling the changes in atmospheric methane sources and sinks between the Last Glacial Maximum and the pre-industrial era, Geophys. Res. Lett., 38, L23804, https://doi.org/10.1029/2011GL049545, 2011. a
Liu, B., Six, K. D., and Ilyina, T.: Incorporating the stable carbon isotope 13C in the ocean biogeochemical component of the Max Planck Institute Earth System Model, Biogeosciences, 18, 4389–4429, https://doi.org/10.5194/bg-18-4389-2021, 2021. a, b, c
Ma, L., Hurtt, G. C., Chini, L. P., Sahajpal, R., Pongratz, J., Frolking, S., Stehfest, E., Klein Goldewijk, K., O'Leary, D., and Doelman, J. C.: Global rules for translating land-use change (LUH2) to land-cover change for CMIP6 using GLM2, Geosci. Model Dev., 13, 3203–3220, https://doi.org/10.5194/gmd-13-3203-2020, 2020. a
MacDougall, A. H., Frölicher, T. L., Jones, C. D., Rogelj, J., Matthews, H. D., Zickfeld, K., Arora, V. K., Barrett, N. J., Brovkin, V., Burger, F. A., Eby, M., Eliseev, A. V., Hajima, T., Holden, P. B., Jeltsch-Thömmes, A., Koven, C., Mengis, N., Menviel, L., Michou, M., Mokhov, I. I., Oka, A., Schwinger, J., Séférian, R., Shaffer, G., Sokolov, A., Tachiiri, K., Tjiputra, J., Wiltshire, A., and Ziehn, T.: Is there warming in the pipeline? A multi-model analysis of the Zero Emissions Commitment from CO2, Biogeosciences, 17, 2987–3016, https://doi.org/10.5194/bg-17-2987-2020, 2020. a
Maerz, J., Six, K. D., Stemmler, I., Ahmerkamp, S., and Ilyina, T.: Microstructure and composition of marine aggregates as co-determinants for vertical particulate organic carbon transfer in the global ocean, Biogeosciences, 17, 1765–1803, https://doi.org/10.5194/bg-17-1765-2020, 2020. a
Maier-Reimer, E. and Hasselmann, K.: Transport and storage of CO2 in the ocean – an inorganic ocean-circulation carbon cycle model, Clim. Dynam., 2, 63–90, https://doi.org/10.1007/BF01054491, 1987. a, b
Maier-Reimer, E., Mikolajewicz, U., and Hasselmann, K.: Mean Circulation of the Hamburg LSG OGCM and Its Sensitivity to the Thermohaline Surface Forcing, J. Phys. Oceanogr., 23, 731–754, https://doi.org/10.1175/1520-0485(1993)023<0731:MCOTHL>2.0.CO;2, 1993. a
Matthes, K., Funke, B., Andersson, M. E., Barnard, L., Beer, J., Charbonneau, P., Clilverd, M. A., Dudok de Wit, T., Haberreiter, M., Hendry, A., Jackman, C. H., Kretzschmar, M., Kruschke, T., Kunze, M., Langematz, U., Marsh, D. R., Maycock, A. C., Misios, S., Rodger, C. J., Scaife, A. A., Seppälä, A., Shangguan, M., Sinnhuber, M., Tourpali, K., Usoskin, I., van de Kamp, M., Verronen, P. T., and Versick, S.: Solar forcing for CMIP6 (v3.2), Geosci. Model Dev., 10, 2247–2302, https://doi.org/10.5194/gmd-10-2247-2017, 2017. a
Matthews, H. D. and Solomon, S.: Irreversible does not mean unavoidable, Science, 340, 438–439, https://doi.org/10.1126/science.1236372, 2013. a
Mauritsen, T., Bader, J., Becker, T., Behrens, J., Bittner, M., Brokopf, R., Brovkin, V., Claussen, M., Crueger, T., Esch, M., Fast, I., Fiedler, S., Fläschner, D., Gayler, V., Giorgetta, M., Goll, D. S., Haak, H., Hagemann, S., Hedemann, C., Hohenegger, C., Ilyina, T., Jahns, T., Jimenéz-de-la Cuesta, D., Jungclaus, J., Kleinen, T., Kloster, S., Kracher, D., Kinne, S., Kleberg, D., Lasslop, G., Kornblueh, L., Marotzke, J., Matei, D., Meraner, K., Mikolajewicz, U., Modali, K., Möbis, B., Müller, W. A., Nabel, J. E., Nam, C. C., Notz, D., Nyawira, S. S., Paulsen, H., Peters, K., Pincus, R., Pohlmann, H., Pongratz, J., Popp, M., Raddatz, T. J., Rast, S., Redler, R., Reick, C. H., Rohrschneider, T., Schemann, V., Schmidt, H., Schnur, R., Schulzweida, U., Six, K. D., Stein, L., Stemmler, I., Stevens, B., von Storch, J. S., Tian, F., Voigt, A., Vrese, P., Wieners, K. H., Wilkenskjeld, S., Winkler, A., and Roeckner, E.: Developments in the MPI-M Earth System Model version 1.2 (MPI-ESM1.2) and Its Response to Increasing CO2, J. Adv. Model. Earth Sy., 11, 998–1038, https://doi.org/10.1029/2018MS001400, 2019. a, b
Medlyn, B. E., Duursma, R. a., Eamus, D., Ellsworth, D. S., Prentice, I. C., Barton, C. V. M., Crous, K. Y., De Angelis, P., Freeman, M., and Wingate, L.: Reconciling the optimal and empirical approaches to modelling stomatal conductance, Glob. Change Biol., 17, 2134–2144, https://doi.org/10.1111/j.1365-2486.2010.02375.x, 2011. a
Meinshausen, M., Vogel, E., Nauels, A., Lorbacher, K., Meinshausen, N., Etheridge, D. M., Fraser, P. J., Montzka, S. A., Rayner, P. J., Trudinger, C. M., Krummel, P. B., Beyerle, U., Canadell, J. G., Daniel, J. S., Enting, I. G., Law, R. M., Lunder, C. R., O'Doherty, S., Prinn, R. G., Reimann, S., Rubino, M., Velders, G. J. M., Vollmer, M. K., Wang, R. H. J., and Weiss, R.: Historical greenhouse gas concentrations for climate modelling (CMIP6), Geosci. Model Dev., 10, 2057–2116, https://doi.org/10.5194/gmd-10-2057-2017, 2017. a
Melack, J. M. and Hess, L. L.: Remote Sensing of the Distribution and Extent of Wetlands in the Amazon Basin, in: Amazonian floodplain forests: Ecophysiology, ecology, biodiversity and sustainable management, edited by: Junk, W. J., Piedade, M. T., Wittmann, F., Schöngart, J., and Parolin, P., Springer, 43–59, https://doi.org/10.1007/978-90-481-8725-6_3, 2010. a
Mengis, N., Keller, D. P., MacDougall, A. H., Eby, M., Wright, N., Meissner, K. J., Oschlies, A., Schmittner, A., MacIsaac, A. J., Matthews, H. D., and Zickfeld, K.: Evaluation of the University of Victoria Earth System Climate Model version 2.10 (UVic ESCM 2.10), Geosci. Model Dev., 13, 4183–4204, https://doi.org/10.5194/gmd-13-4183-2020, 2020. a
Milliman, J. D. and Droxler, A. W.: Neritic and pelagic carbonate sedimentation in the marine environment: Ignorance is not bliss, Geol. Rundsch., 85, 496–504, https://doi.org/10.1007/BF02369004, 1996. a
Moore, C. M., Mills, M. M., Arrigo, K. R., Berman-Frank, I., Bopp, L., Boyd, P. W., Galbraith, E. D., Geider, R. J., Guieu, C., Jaccard, S. L., Jickells, T. D., La Roche, J., Lenton, T. M., Mahowald, N. M., Marañón, E., Marinov, I., Moore, J. K., Nakatsuka, T., Oschlies, A., Saito, M. A., Thingstad, T. F., Tsuda, A., and Ulloa, O.: Processes and patterns of oceanic nutrient limitation, Nat. Geosci., 6, 701–710, https://doi.org/10.1038/ngeo1765, 2013. a
Müller, S. A., Joos, F., Plattner, G. K., Edwards, N. R., and Stocker, T. F.: Modeled natural and excess radiocarbon: Sensitivities to the gas exchange formulation and ocean transport strength, Global Biogeochem. Cy., 22, GB3011, https://doi.org/10.1029/2007GB003065, 2008. a
Munhoven, G.: Glacial – Interglacial changes of continental weathering: Estimates of the related CO2 and HCO3 – flux variations and their uncertainties, Global Planet. Change, 33, 155–176, https://doi.org/10.1016/S0921-8181(02)00068-1, 2002. a, b, c
Munhoven, G.: Mathematics of the total alkalinity–pH equation – pathway to robust and universal solution algorithms: the SolveSAPHE package v1.0.1, Geosci. Model Dev., 6, 1367–1388, https://doi.org/10.5194/gmd-6-1367-2013, 2013. a
Munhoven, G. and François, L. M.: Glacial-interglacial changes in continental weathering: Possible implications for atmospheric CO2, in: Carbon Cycling in the Glacial Ocean: Constraints on the Ocean's Role in Global Change, edited by: Zahn, R., Pedersen, T. F., Kaminski, M. A., and Labeyrie, L., vol. 17 of NATO ASI Series I: Global Environmental Change, Springer-Verlag, Berlin, 39–58, https://doi.org/10.1007/978-3-642-78737-9_3, 1994. a, b
Oleson, K. W., Lawrence, D. M., Gordon, B., Flanner, M. G., Kluzek, E., Peter, J., Levis, S., Swenson, S. C., Thornton, E., and Feddema, J.: Technical description of version 4.5 of the Community Land Model (CLM), NCAR/TN-478+STR NCAR Technical Note, 266 pp., https://doi.org/10.5065/D6RR1W7M, 2010. a
Olsen, A., Key, R. M., van Heuven, S., Lauvset, S. K., Velo, A., Lin, X., Schirnick, C., Kozyr, A., Tanhua, T., Hoppema, M., Jutterström, S., Steinfeldt, R., Jeansson, E., Ishii, M., Pérez, F. F., and Suzuki, T.: The Global Ocean Data Analysis Project version 2 (GLODAPv2) – an internally consistent data product for the world ocean, Earth Syst. Sci. Data, 8, 297–323, https://doi.org/10.5194/essd-8-297-2016, 2016. a, b, c, d, e
Orr, J. C., Najjar, R. G., Aumont, O., Bopp, L., Bullister, J. L., Danabasoglu, G., Doney, S. C., Dunne, J. P., Dutay, J.-C., Graven, H., Griffies, S. M., John, J. G., Joos, F., Levin, I., Lindsay, K., Matear, R. J., McKinley, G. A., Mouchet, A., Oschlies, A., Romanou, A., Schlitzer, R., Tagliabue, A., Tanhua, T., and Yool, A.: Biogeochemical protocols and diagnostics for the CMIP6 Ocean Model Intercomparison Project (OMIP), Geosci. Model Dev., 10, 2169–2199, https://doi.org/10.5194/gmd-10-2169-2017, 2017. a, b
Papa, F., Prigent, C., Aires, F., Jimenez, C., Rossow, W. B., and Matthews, E.: Interannual variability of surface water extent at the global scale, 1993–2004, J. Geophys. Res., 115, D12111, https://doi.org/10.1029/2009JD012674, 2010. a, b, c
Parekh, P., Follows, M. J., and Boyle, E.: Modeling the global ocean iron cycle, Global Biogeochem. Cy., 18, GB1002, https://doi.org/10.1029/2003GB002061, 2004. a
Paulsen, H., Ilyina, T., Six, K. D., and Stemmler, I.: Incorporating a prognostic representation of marine nitrogen fixers into the global ocean biogeochemical model HAMOCC, J. Adv. Model. Earth Sy., 9, 438–464, https://doi.org/10.1002/2016MS000737, 2017. a
Petit, J. R., Jouzel, J., Raynaud, D., Barkov, N. I., Barnola, J.-M., Basile, I., Bender, M., Chappellaz, J., Davis, M., Delaygue, G., Delmotte, M., Kotlyakov, V. M., Legrand, M., Lipenkov, V. Y., Lorius, C., PÉpin, L., Ritz, C., Saltzman, E., and Stievenard, M.: Climate and atmospheric history of the past 420,000 years from the Vostok ice core, Antarctica, Nature, 399, 429–436, https://doi.org/10.1038/20859, 1999. a
Prather, M., Flato, G., Friedlingstein, P., Jones, C., Lamarque, J., Liao, H., and Rasch, P.: IPCC, 2013: Annex II: Climate System Scenario Table, in: Climate Change 2013: The Physical Science Basis. Contribution of Working Group I to the Fifth Assessment Report of the Intergovernmental Panel on Climate Change, edited by: Stocker, T., Qin, D., Plattner, G.-K., Tignor, M., Allen, S., Boschung, J., Nauels, A., Xia, Y., Bex, V., and Midgle, P., Cambridge University Press, Cambridge, United Kingdom and New York, NY, USA, https://www.ipcc.ch/site/assets/uploads/2017/09/WG1AR5_AnnexII_FINAL.pdf (last access: 10 July 2022), 2013. a
Prentice, I. C., Dong, N., Gleason, S. M., Maire, V., and Wright, I. J.: Balancing the costs of carbon gain and water transport: testing a new theoretical framework for plant functional ecology., Ecol. Lett., 17, 82–91, https://doi.org/10.1111/ele.12211, 2014. a
Prigent, C., Papa, F., Aires, F., Rossow, W. B., and Matthews, E.: Global inundation dynamics inferred from multiple satellite observations, 1993–2000, J. Geophys. Res., 112, D12107, https://doi.org/10.1029/2006JD007847, 2007. a, b, c
Raymo, M. and Ruddiman, W.: Tectonic Forcing of Late Cenozoic Climate, Nature, 359, 117–122, 1992. a
Regnier, P., Friedlingstein, P., Ciais, P., Mackenzie, F. T., Gruber, N., Janssens, I. A., Laruelle, G. G., Lauerwald, R., Luyssaert, S., Andersson, A. J., Arndt, S., Arnosti, C., Borges, A. V., Dale, A. W., Gallego-Sala, A., Goddéris, Y., Goossens, N., Hartmann, J., Heinze, C., Ilyina, T., Joos, F., Larowe, D. E., Leifeld, J., Meysman, F. J., Munhoven, G., Raymond, P. A., Spahni, R., Suntharalingam, P., and Thullner, M.: Anthropogenic perturbation of the carbon fluxes from land to ocean, Nat. Geosci., 6, 597–607, https://doi.org/10.1038/ngeo1830, 2013. a
Ridgwell, A., Hargreaves, J. C., Edwards, N. R., Annan, J. D., Lenton, T. M., Marsh, R., Yool, A., and Watson, A.: Marine geochemical data assimilation in an efficient Earth System Model of global biogeochemical cycling, Biogeosciences, 4, 87–104, https://doi.org/10.5194/bg-4-87-2007, 2007. a
Riley, W. J., Subin, Z. M., Lawrence, D. M., Swenson, S. C., Torn, M. S., Meng, L., Mahowald, N. M., and Hess, P.: Barriers to predicting changes in global terrestrial methane fluxes: analyses using CLM4Me, a methane biogeochemistry model integrated in CESM, Biogeosciences, 8, 1925–1953, https://doi.org/10.5194/bg-8-1925-2011, 2011. a
Romero-Mujalli, G., Hartmann, J., and Börker, J.: Temperature and CO2 dependency of global carbonate weathering fluxes – Implications for future carbonate weathering research, Chem. Geol., 527, 118874, https://doi.org/10.1016/j.chemgeo.2018.08.010, 2019. a
Sanderman, J., Hengl, T., and Fiske, G. J.: Soil carbon debt of 12,000 years of human land use, P. Natl. Acad. Sci. USA, 114, 9575–9580, https://doi.org/10.1073/pnas.1706103114, 2017. a
Saunois, M., Stavert, A. R., Poulter, B., Bousquet, P., Canadell, J. G., Jackson, R. B., Raymond, P. A., Dlugokencky, E. J., Houweling, S., Patra, P. K., Ciais, P., Arora, V. K., Bastviken, D., Bergamaschi, P., Blake, D. R., Brailsford, G., Bruhwiler, L., Carlson, K. M., Carrol, M., Castaldi, S., Chandra, N., Crevoisier, C., Crill, P. M., Covey, K., Curry, C. L., Etiope, G., Frankenberg, C., Gedney, N., Hegglin, M. I., Höglund-Isaksson, L., Hugelius, G., Ishizawa, M., Ito, A., Janssens-Maenhout, G., Jensen, K. M., Joos, F., Kleinen, T., Krummel, P. B., Langenfelds, R. L., Laruelle, G. G., Liu, L., Machida, T., Maksyutov, S., McDonald, K. C., McNorton, J., Miller, P. A., Melton, J. R., Morino, I., Müller, J., Murguia-Flores, F., Naik, V., Niwa, Y., Noce, S., O'Doherty, S., Parker, R. J., Peng, C., Peng, S., Peters, G. P., Prigent, C., Prinn, R., Ramonet, M., Regnier, P., Riley, W. J., Rosentreter, J. A., Segers, A., Simpson, I. J., Shi, H., Smith, S. J., Steele, L. P., Thornton, B. F., Tian, H., Tohjima, Y., Tubiello, F. N., Tsuruta, A., Viovy, N., Voulgarakis, A., Weber, T. S., van Weele, M., van der Werf, G. R., Weiss, R. F., Worthy, D., Wunch, D., Yin, Y., Yoshida, Y., Zhang, W., Zhang, Z., Zhao, Y., Zheng, B., Zhu, Q., Zhu, Q., and Zhuang, Q.: The Global Methane Budget 2000–2017, Earth Syst. Sci. Data, 12, 1561–1623, https://doi.org/10.5194/essd-12-1561-2020, 2020. a, b, c, d, e
Schaphoff, S., von Bloh, W., Rammig, A., Thonicke, K., Biemans, H., Forkel, M., Gerten, D., Heinke, J., Jägermeyr, J., Knauer, J., Langerwisch, F., Lucht, W., Müller, C., Rolinski, S., and Waha, K.: LPJmL4 – a dynamic global vegetation model with managed land – Part 1: Model description, Geosci. Model Dev., 11, 1343–1375, https://doi.org/10.5194/gmd-11-1343-2018, 2018. a
Schubert, B. A. and Jahren, A. H.: Incorporating the effects of photorespiration into terrestrial paleoclimate reconstruction, Earth-Sci. Rev., 177, 637–642, https://doi.org/10.1016/j.earscirev.2017.12.008, 2018. a
Séférian, R., Berthet, S., Yool, A., Palmiéri, J., Bopp, L., Tagliabue, A., Kwiatkowski, L., Aumont, O., Christian, J., Dunne, J., Gehlen, M., Ilyina, T., John, J. G., Li, H., Long, M. C., Luo, J. Y., Nakano, H., Romanou, A., Schwinger, J., Stock, C., Santana-Falcón, Y., Takano, Y., Tjiputra, J., Tsujino, H., Watanabe, M., Wu, T., Wu, F., and Yamamoto, A.: Tracking Improvement in Simulated Marine Biogeochemistry Between CMIP5 and CMIP6, Current Climate Change Reports, 6, 95–119, https://doi.org/10.1007/s40641-020-00160-0, 2020. a
Segschneider, J. and Bendtsen, J.: Temperature-dependent remineralization in a warming ocean increases surface pCO2 through changes in marine ecosystem composition, Global Biogeochem. Cy., 27, 1214–1225, https://doi.org/10.1002/2013GB004684, 2013. a
Sitch, S., Smith, B., Prentice, I. C., Arneth, A., Bondeau, A., Cramer, W., Kaplan, J. O., Levis, S., Lucht, W., Sykes, M. T., Thonicke, K., and Venevsky, S.: Evaluation of ecosystem dynamics, plant geography and terrestrial carbon cycling in the LPJ dynamic global vegetation model, Glob. Change Biol., 9, 161–185, https://doi.org/10.1046/j.1365-2486.2003.00569.x, 2003. a
Six, K. D. and Maier-Reimer, E.: Effects of plankton dynamics on seasonal carbon fluxes in an ocean general circulation model, Global Biogeochem. Cy., 10, 559–583, https://doi.org/10.1029/96GB02561, 1996. a
Stocker, B. D., Roth, R., Joos, F., Spahni, R., Steinacher, M., Zaehle, S., Bouwman, L., and Prentice, I. C.: Multiple greenhouse-gas feedbacks from the land biosphere under future climate change scenarios, Nat. Clim. Change, 3, 666–672, https://doi.org/10.1038/nclimate1864, 2013. a
Tagliabue, A., Mtshali, T., Aumont, O., Bowie, A. R., Klunder, M. B., Roychoudhury, A. N., and Swart, S.: A global compilation of dissolved iron measurements: focus on distributions and processes in the Southern Ocean, Biogeosciences, 9, 2333–2349, https://doi.org/10.5194/bg-9-2333-2012, 2012. a
Tagliabue, A., Aumont, O., DeAth, R., Dunne, J., Dutkiewicz, S., Galbraith, E., Misumi, K., Moore, J., Ridgewell, A., Sherman, E., Stock, C., Vichi, M., Volker, C., and Yool, A.: How well do global ocean biogeochemistry models simulate dissolved iron distributions?, Global Biogeochem. Cy., 30, 149–174, https://doi.org/10.1002/2015GB005289, 2016. a
Takahashi, T., Broecker, W. S., and Langer, S.: Redfield ratio based on chemical data from isopycnal surfaces., J. Geophys. Res., 90, 6907–6924, https://doi.org/10.1029/JC090iC04p06907, 1985. a
Tarnocai, C., Canadell, J. G., Schuur, E. a. G., Kuhry, P., Mazhitova, G., and Zimov, S.: Soil organic carbon pools in the northern circumpolar permafrost region, Global Biogeochem. Cy., 23, GB2023, https://doi.org/10.1029/2008GB003327, 2009. a
Thornton, P. E. and Zimmermann, N. E.: An improved canopy integration scheme for a Land Surface Model with prognostic canopy structure, J. Climate, 20, 3902–3923, https://doi.org/10.1175/JCLI4222.1, 2007. a
Tréguer, P. J. and De La Rocha, C. L.: The World Ocean Silica Cycle, Annu. Rev. Mar. Sci., 5, 477–501, https://doi.org/10.1146/annurev-marine-121211-172346, 2013. a, b, c
Tréguer, P. J., Sutton, J. N., Brzezinski, M., Charette, M. A., Devries, T., Dutkiewicz, S., Ehlert, C., Hawkings, J., Leynaert, A., Liu, S. M., Llopis Monferrer, N., López-Acosta, M., Maldonado, M., Rahman, S., Ran, L., and Rouxel, O.: Reviews and syntheses: The biogeochemical cycle of silicon in the modern ocean, Biogeosciences, 18, 1269–1289, https://doi.org/10.5194/bg-18-1269-2021, 2021. a
Tschumi, T., Joos, F., Gehlen, M., and Heinze, C.: Deep ocean ventilation, carbon isotopes, marine sedimentation and the deglacial CO2 rise, Clim. Past, 7, 771–800, https://doi.org/10.5194/cp-7-771-2011, 2011. a
Ubierna, N. and Farquhar, G. D.: Advances in measurements and models of photosynthetic carbon isotope discrimination in C3 plants, Plant Cell Environ., 37, 1494–1498, https://doi.org/10.1111/pce.12346, 2014. a
Varney, R. M., Chadburn, S. E., Burke, E. J., and Cox, P. M.: Evaluation of soil carbon simulation in CMIP6 Earth system models, Biogeosciences, 19, 4671–4704, https://doi.org/10.5194/bg-19-4671-2022, 2022. a, b, c
Willeit, M.: CLIMBER-X v1.0, Zenodo [code and data set], https://doi.org/10.5281/zenodo.7898797, 2023. a
Willeit, M. and Ganopolski, A.: PALADYN v1.0, a comprehensive land surface–vegetation–carbon cycle model of intermediate complexity, Geosci. Model Dev., 9, 3817–3857, https://doi.org/10.5194/gmd-9-3817-2016, 2016. a, b, c, d, e, f, g, h
Willeit, M., Ganopolski, A., Calov, R., and Brovkin, V.: Mid-Pleistocene transition in glacial cycles explained by declining CO2 and regolith removal, Science Advances, 5, eaav7337, https://doi.org/10.1126/sciadv.aav7337, 2019. a
Willeit, M., Ganopolski, A., Robinson, A., and Edwards, N. R.: The Earth system model CLIMBER-X v1.0 – Part 1: Climate model description and validation, Geosci. Model Dev., 15, 5905–5948, https://doi.org/10.5194/gmd-15-5905-2022, 2022. a, b, c
Willey, D. A., Fine, R. A., Sonnerup, R. E., Bullister, J. L., Smethie, W. M., and Warner, M. J.: Global oceanic chlorofluorocarbon inventory, Geophys. Res. Lett., 31, L01303, https://doi.org/10.1029/2003GL018816, 2004. a, b
Yang, K., Koike, T., Ishikawa, H., Kim, J., Li, X., Liu, H., Liu, S., Ma, Y., and Wang, J.: Turbulent flux transfer over bare-soil surfaces: Characteristics and parameterization, J. Appl. Meteorol. Clim., 47, 276–290, https://doi.org/10.1175/2007JAMC1547.1, 2008. a
Yu, Z., Loisel, J., Brosseau, D. P., Beilman, D. W., and Hunt, S. J.: Global peatland dynamics since the Last Glacial Maximum, Geophys. Res. Lett., 37, L13402, https://doi.org/10.1029/2010GL043584, 2010. a, b, c
Zehr, J. P. and Capone, D. G.: Changing perspectives in marine nitrogen fixation, Science, 368, eaay9514, https://doi.org/10.1126/science.aay9514, 2020. a
Zender, C. S., Newman, D., and Torres, O.: Spatial heterogeneity in aeolian erodibility: Uniform, topographic, geomorphic, and hydrologic hypotheses, J. Geophys. Res.-Atmos., 108, 4543, https://doi.org/10.1029/2002jd003039, 2003. a
Zheng, W., Wei, H., Wang, Z., Zeng, X., Meng, J., Ek, M., Mitchell, K., and Derber, J.: Improvement of daytime land surface skin temperature over arid regions in the NCEP GFS model and its impact on satellite data assimilation, J. Geophys. Res.-Atmos., 117, D06117, https://doi.org/10.1029/2011JD015901, 2012. a
Zickfeld, K., Eby, M., Damon Matthews, H., Schmittner, A., and Weaver, A. J.: Nonlinearity of carbon cycle feedbacks, J. Climate, 24, 4255–4275, https://doi.org/10.1175/2011JCLI3898.1, 2011. a
Zilitinkevich, S.: Non-local turbulent transport: pollution dispersion aspects of coherent structure of convective flows, International Conference on Air Pollution – Proceedings, 1, 53–60, https://doi.org/10.2495/air950071, 1995. a, b
- Abstract
- Introduction
- Model description
- Closed and open carbon cycle model configurations and model spin-up
- Model evaluation for the historical period and present day
- Carbon cycle feedbacks
- The zero emissions commitment
- Conclusions
- Code and data availability
- Author contributions
- Competing interests
- Disclaimer
- Acknowledgements
- Financial support
- Review statement
- References
- Abstract
- Introduction
- Model description
- Closed and open carbon cycle model configurations and model spin-up
- Model evaluation for the historical period and present day
- Carbon cycle feedbacks
- The zero emissions commitment
- Conclusions
- Code and data availability
- Author contributions
- Competing interests
- Disclaimer
- Acknowledgements
- Financial support
- Review statement
- References