the Creative Commons Attribution 4.0 License.
the Creative Commons Attribution 4.0 License.
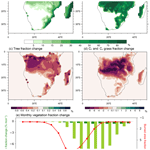
Modeling the short-term fire effects on vegetation dynamics and surface energy in southern Africa using the improved SSiB4/TRIFFID-Fire model
Yongkang Xue
Gregory S. Okin
Fire causes abrupt changes in vegetation properties and modifies flux exchanges between land and atmosphere at subseasonal to seasonal scales. Yet these short-term fire effects on vegetation dynamics and surface energy balance have not been comprehensively investigated in the fire-coupled vegetation model. This study applies the SSiB4/TRIFFID-Fire (the Simplified Simple Biosphere Model coupled with the Top-down Representation of Interactive Foliage and Flora Including Dynamics with fire) model to study the short-term fire impact in southern Africa. Specifically, we aim to quantify how large impacts fire exerts on surface energy through disturbances on vegetation dynamics, how fire effects evolve during the fire season and the subsequent rainy season, and how surface-darkening effects play a role besides the vegetation change effects.
We find fire causes an annual average reduction in grass cover by 4 %–8 % for widespread areas between 5–20∘ S and a tree cover reduction by 1 % at the southern periphery of tropical rainforests. The regional fire effects accumulate during June–October and peak in November, the beginning of the rainy season. After the fire season ends, the grass cover quickly returns to unburned conditions, while the tree fraction hardly recovers in one rainy season. The vegetation removal by fire has reduced the leaf area index (LAI) and gross primary productivity (GPP) by 3 %–5 % and 5 %–7 % annually. The exposure of bare soil enhances surface albedo and therefore decreases the absorption of shortwave radiation. Annual mean sensible heat has dropped by 1.4 W m−2, while the latent heat reduction is small (0.1 W m−2) due to the compensating effects between canopy transpiration and soil evaporation. Surface temperature is increased by as much as 0.33 K due to the decrease of sensible heat fluxes, and the warming would be enhanced when the surface-darkening effect is incorporated. Our results suggest that fire effects in grass-dominant areas diminish within 1 year due to the high resilience of grasses after fire. Yet fire effects in the periphery of tropical forests are irreversible within one growing season and can cause large-scale deforestation if accumulated for hundreds of years.
- Article
(5957 KB) - Full-text XML
-
Supplement
(786 KB) - BibTeX
- EndNote
Fire is an integral component of the Earth's ecosystems (Bowman et al., 2009; Bond et al., 2005). Through prevalent disturbance on surface biophysical properties (i.e., albedo, vegetation characteristics, and soil properties), fire alters radiative forcing on Earth's surface and modifies the energy flux exchanges between land and atmosphere (Chambers and Chapin, 2002; Bond-Lamberty et al., 2009). Changes in surface properties influence the development of the planetary boundary layer and contribute to reduced convective rainfall (Wendt et al., 2007; Saha et al., 2016) and may also negatively influence the monsoonal precipitation (De Sales et al., 2018). Meanwhile, fires also alter atmospheric biogeochemical processes through the release of greenhouse gases, aerosols, and volatile organic compounds (Scholes et al., 1996; F. Li et al., 2019), exerting radiative forcing in the climate system through greenhouse gas effects, aerosol–radiation interactions, and aerosol–cloud interactions (Ward et al., 2012; Jiang et al., 2016; Hamilton et al., 2018; Zou et al., 2020).
Fire models have been developed within dynamic global vegetation models (DGVMs) to explicitly describe the burned area, carbon emission, and fire disturbance on vegetation (Thonicke et al., 2010, 2001; Li et al., 2012; Arora and Boer, 2005; Pfeiffer et al., 2013; Rabin et al., 2018; Burton et al., 2019; Venevsky et al., 2002; Lasslop et al., 2014; Huang et al., 2020a). The fire-coupled DGVMs have been widely used to study the role of fire on vegetation distribution (Bond and Midgley, 2012; Seo and Kim, 2019), terrestrial carbon budget (Li et al., 2014; Yue et al., 2015; Lasslop et al., 2020), surface energy balance (Li et al., 2017; Huang et al., 2020a), and the water cycle (Li and Lawrence, 2017). Previous modeling studies on fire effects mostly focus on the long-term fire effects where the simulations with fire are compared with reference simulations representing “a world without fire” (Lasslop et al., 2020). While the long-term fire effects are comprehensively assessed in multi-model studies (Lasslop et al., 2020), the quantification of short-term fire effects at monthly to annual scales is somewhat lacking. The short-term fire effects include the fire-caused abrupt changes of vegetation status, surface properties (albedo and roughness), and the subsequent effects on surface energy and hydrology cycle before and after a fire season (Lasslop et al., 2020). These short-term effects are significant at local and regional scales, yet to our knowledge, they have not been assessed in any fire–vegetation model studies. The simulated fire impacts can be compared with those from satellite observations to evaluate the description of fire–vegetation interactions in DGVMs, bridging the gap between observational and modeling studies.
The short-term fire effects are highly variable among different ecosystems. Here we focus on fire effects in tropical savannas which have the largest stretch of burned area and the most representative fire–vegetation–climate feedbacks among all the ecosystems (Staver et al., 2011). Satellite observational studies have quantified the short-term fire effects on albedo change and surface radiation in tropical savanna (Gatebe et al., 2014; Dintwe et al., 2017; Saha et al., 2016, 2017, 2019; Beringer et al., 2003; Veraverbeke et al., 2012; Z. H. Liu et al., 2019; López-Saldaña et al., 2015; Staal et al., 2018). An immediate reduction in surface albedo is reported after fire, associated with ash and charcoal deposition (Myhre et al., 2005; Govaerts et al., 2002). Some observations found the surface darkening lasted for 10–60 d, followed by a gradual brightening when charcoals were removed by wind or runoff and bare soil was exposed (Samain et al., 2008; Saha et al., 2019, 2017; Lyons et al., 2008; Gatebe et al., 2014). According to recent estimates in Saha et al. (2019), the average albedo anomaly in the year following fire is for all of sub-Saharan Africa, representing a negative radiative forcing dominated by surface brightening effects. On the other hand, some studies found the darkening maintained for more than 4 months, with the brightening effects only in limited areas (Dintwe et al., 2017; Jin and Roy, 2005). Dintwe et al. (2017) reported a positive radiative forcing by 0.18 W m−2 averaged over sub-Saharan Africa. The variations can be explained from various aspects, including the background climate, soil properties of study regions, burning seasons, and criteria used to define the “control” pixel (Dintwe et al., 2017; Saha et al., 2019).
Fire effects on savanna vegetation have been widely investigated on site-level studies, which show that vegetation can recover to unburned conditions within 8 d to 12 months after fire (Araújo et al., 2017; das Chagas and Pelicice, 2018). However, fire–vegetation interactions on the regional scale remain unclear, both in the observational and fire modeling studies. Observational data analysis proposed that fire-caused vegetation loss was an important component in the negative feedback loop between fire and precipitation in Africa, in which fire suppressed convective precipitation, thereby reducing fuel load and fire in the subsequent season (Saha et al., 2016). The proposed feedbacks are also tested in regional modeling studies, which showed that post-fire land condition deterioration resulted in a decrease in wet season rainfall, associated with atmospheric cooling and subsidence (De Sales et al., 2018), as well as a weakening of West African monsoon progression (De Sales et al., 2016).
This study makes the first attempt to simulate the short-term fire effects using a fire-coupled dynamic vegetation model. Specifically, we focus on quantifying regional fire effects on surface radiative forcing and energy balance through disturbances on vegetation dynamics, describing the temporal evolution of fire effects during the fire season and the subsequent rainy season, and investigating the role of surface-darkening effects due to ash deposition. The process-based fire–vegetation model we use, SSiB4/TRIFFID-Fire (the Simplified Simple Biosphere Model coupled with the Top-down Representation of Interactive Foliage and Flora Including Dynamics with fire), has been comprehensively evaluated with observed burned area and fire emissions on the global scale and is shown to capture the fire–vegetation interactions under the current climate (Huang et al., 2020a). This study further improves the SSiB4/TRIFFID-Fire to better describe the temporal variations of fire regimes and vegetation productivity on monthly scales. The model improvement and experimental design are given in Sect. 2. After comprehensive validation of the fire–vegetation model performance, we apply SSiB4/TRIFFID-Fire to investigate fire effects on vegetation cover, ecosystem productivity, and surface energy on monthly to annual scales in Sect. 3. Discussions and conclusions are given in Sect. 4.
2.1 Study region
We conduct our fire modeling study in southern Africa (SAF; 0–35∘ S, 0–50∘ E), where most areas have a typical savanna climate and high incidence of fires. SAF has the largest continuous stretch of savanna, covering an area of 1.4×103 Mha of the land surface. SAF savanna has an annual burned area of 153.7 Mha yr−1 (Giglio et al., 2018) and carbon emission of 669 Tg C yr−1 (van der Werf et al., 2017), contributing to about 36 % and 31 % of the global total burned area and fire carbon emissions, respectively. Each year in the dry season, fire leaves numerous scars on land surface, and local ecosystems have evolved with fire as an essential contributor to its structure and function. Therefore, we select SAF as the study region to quantify the fire effects at monthly to annual scales.
Over SAF, equatorial Africa (0–5∘ S), east coast of SAF, and east coast of the island of Madagascar are hot and humid throughout the year, with an annual mean temperature of 25 ∘C and rainfall exceeding 1200 mm yr−1 (Fig. 1a–b). From the Equator to the Southern Hemisphere (SH) high latitudes, the annual mean precipitation and temperature decrease while the seasonality is enhanced (Fig. 1c–d). The SAF savanna has a divergent climate during the wet season (November–April) and dry season (May–October). During the rainy season, the daily precipitation can reach 15 mm d−1, resulting in significant floods in Zimbabwe, Zambia, Malawi, and Mozambique (https://reliefweb.int/report/malawi/south-east-africa-deadly-storms-and-floods-malawi-zambia-and-mozambique, last access: 15 October 2020). The dry season is characterized by little precipitation, especially for June–July–August when monthly rainfall is less than 10 mm (Fig. 1d). SAF has diverse ecosystems influenced by climate and fire. From tropical SAF to Southern Hemisphere high latitudes, the climatology land cover ranges from the densely forested area, savanna, grassland, shrubland, and desert, respectively (Fig. 1e). Equatorial Africa is dominated by tropical rainforests, known as the Congolese rainforest. Most areas between 5–20∘ S are dominated by C4 grasses with tree fractions varying between 10 %–20 % with moisture conditions, referred to as the savanna biome. C3 grasses are primarily distributed in the eastern part of SAF along the Great Rift Valley and the eastern portion of the Great Escarpment. Shrubs dominate the southern African plateau.
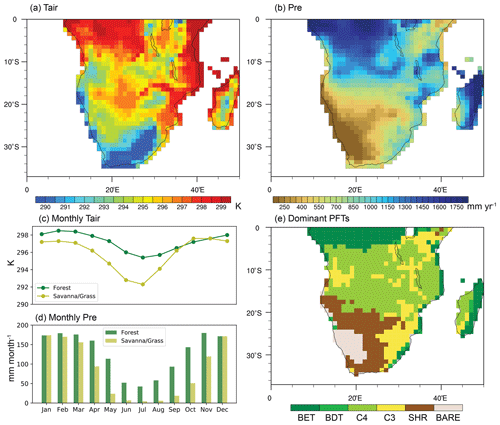
Figure 1(a–d) Climate of SAF from Sheffield et al. (2006) averaged in 2000–2013: (a) annual mean air temperature (Tair), (b) annual total precipitation (Pre), (c) monthly Tair, (d) monthly Pre, and (e) dominant PFTs for each grid simulated in SSiB4/TRIFFID-Fire (BET: broadleaf evergreen tree; BDT: broadleaf deciduous tree; C4: C4 grasses; C3: C3 grasses; SHR: shrub; BARE: bare land).
2.2 The SSiB4/TRIFFID-Fire vegetation–fire model
SSiB4/TRIFFID-Fire consists of three components: a land surface model (Simplified Simple Biosphere Model; SSiB), a dynamic vegetation model (the Top-down Representation of Interactive Foliage and Flora Including Dynamics Model; TRIFFID), and a fire model of intermediate complexity (Huang et al., 2020a). SSiB simulates surface radiation components, momentum fluxes, sensible heat and latent heat fluxes, soil moisture, surface temperature, and vegetation gross/net primary productivity (GPP/NPP) based on energy and water balance (Xue et al., 1991; Zhan et al., 2003). The SSiB was coupled with TRIFFID, which describes the vegetation dynamics based on species competition for common resources and provides an interactive component in the feedback loop of ecosystems and climate (Cox, 2001; Harper et al., 2016). The vegetation competition in TRIFFID is based on the Lotka–Volterra equation, which has been updated in Zhang et al. (2015) to represent the coexistence of grasses and shrubs. Y. Liu et al. (2019) further adjusted the large-scale disturbance parameter, which includes vegetation disturbances due to fires and other processes, to allow for the coexistence between trees, C3 grasses, and C4 grasses. The modeled plant functional types (PFTs) in SSiB4/TRIFFID include broadleaf evergreen trees, needleleaf evergreen trees, broadleaf deciduous trees, C3 grasses, C4 grasses, shrubs, and tundra shrubs. The simulated vegetation distribution in SSiB4/TRIFFID has been evaluated with observations over Northern America (Zhang et al., 2015) and over the globe (Y. Liu et al., 2019).
The SSiB4/TRIFFID is further improved by incorporating a fire scheme (Li et al., 2012) to describe fire disturbance on vegetation dynamics and carbon cycle (hereafter SSiB4/TRIFFID-Fire). SSiB4/TRIFFID-Fire is shown to reproduce the burned area and fire emissions across the spatial and temporal scales (Huang et al., 2020a). Specifically, it produces realistic fire peak months and fire season length in major fire regions, including southern Africa (SAF), South America, Southeast Asia, and equatorial Asia. The fractional coverage of each PFT has been thoroughly validated with observations on the global scale. With an explicit description of the burned area, carbon emission, and fire disturbance on vegetation, SSiB4/TRIFFID-Fire captures fire–vegetation interactions under current climate and can be used to study fire effects on ecosystem characteristics and surface energy.
2.3 Model improvement
This study updates the SSiB4/TRIFFID-Fire to improve the simulation of monthly fire regimes, vegetation productivity, and surface fluxes in SAF. A constant crop fraction from GLC2000 (Bartholome and Belward, 2005) was used to exclude fire in human-managed land use during the entire simulation period in Huang et al. (2020a). Studies show that agriculture expansion has played a role in the spatial and temporal variations of the burned area in the tropical region and should be explicitly described (Andela et al., 2017; Lasslop and Kloster, 2017). We introduce an annually updated crop fraction from Land-Use Harmonization 2 (LUH2) datasets (Hurtt et al., 2006, 2011) to investigate the influence of crop fraction interannual changes on fire. Since the LUH2 has a smaller crop area than GLC2000, we decrease the fire spread and fuel combustibility to keep the burned area in a similar magnitude as in Huang et al. (2020a). Besides, we find that the carbon emission per burned area in SAF is larger than observations from GFED4s in the previous model version. We therefore decrease the leaf combustion completeness to reduce the carbon emission.
Wet season accumulated productivity proves to be one of the determinants for the burned area and carbon emission in the following fire season (Forkel et al., 2019). The vegetation productivity, however, is also influenced by phenology and fire. Our previous study found that SSiB4/TRIFFID-Fire captured its spatial distribution and interannual variations, but the annual mean GPP was overestimated (Huang et al., 2020a). During an annual cycle, the model properly simulates the GPP magnitude in the wet season but overestimates it in the dry season, leading to an underestimation of the seasonality of ecosystem functioning in savanna and grassland (Fig. S1a in the Supplement). A similar conclusion can be drawn in the simulated intra-annual variations of LAI. To reduce GPP magnitude in dry seasons, we optimize root-zone soil moisture potential factor f(θ), a parameter that determines transpiration in SSiB4/TRIFFID-Fire, to constrain photosynthesis activities. The procedure is introduced as follows.
By compiling 32 years of satellite data, W. Li et al. (2019) reported that moisture condition (precipitation) was the first vital driver positively affecting monthly vegetation productivity in non-forest areas. In SSiB4, the vegetation productivity is closely associated with the soil moisture through the root-zone soil moisture potential factor f(θ):
where c1, c2, ph0, and b are PFT-dependent parameters. f(θ) represents the soil moisture (θ) effects on stomatal resistance, which influences CO2 and water exchanges and can also affect leaf turnover. f(θ) does not play a role when it is close to 1 and can largely suppress the transpiration and vegetation productivity when it is close to 0. For C3 and C4 grasses, the original f(θ) decreases sharply when soil moisture (θ) is between 0.3–0.4, yet it has a negligible effect when soil moisture is higher than 0.4 (Fig. 2). In SSiB4/TRIFFID-Fire, the simulated root-zone soil moisture is generally higher than 0.4 in the SAF dry season. Therefore, we adjusted the coefficients c1 and c2 for C4 grasses to reflect the effects of soil water deficit on transpiration in a wider range of soil moisture between 0.3–0.6 (Fig. 2a). In Eq. (1), c1 represents the wilting point at which stomates close completely, and c2 is a slope factor controlling how sensitive the vegetation responds to soil water deficit. We decrease c2 for C3 and C4 grasses to reduce the slope so that the vegetation responds to soil water deficit at relatively wetter conditions. The c1 for C3 grasses is set to be smaller than for C4 grasses so that the stomates close at a drier condition and C3 grasses have more sustainability to drought.
2.4 Experiment design
A series of offline experiments has been conducted using SSiB4/TRIFFID-Fire, including a spin-up simulation to reach quasi-equilibrium vegetation distribution and a transient run with varying climate forcings and CO2 from 1948 to 2014 (Fig. 3). The spin-up simulation is conducted with 1948–1972 climatology forcing from Sheffield et al. (2006) and 1948 atmospheric CO2 concentration at 310.325 ppm with the fire model turned on. The DGVM reaches a quasi-equilibrium status after 200 years of simulation (Fig. 3). Based on the quasi-equilibrium status, a FIREON transient run is carried out with 3-hourly meteorological forcings and yearly updated atmospheric CO2 from 310.325 to 398.99 ppm with the fire model turned on. The fire model requires annual agriculture, population density, and GDP information from 1948 to 2014. The spin-up and transient runs are the same as those in Huang et al. (2020a) except for the yearly updated crop fraction. We focus on the period of 2000–2014 when the satellite observations are available for fire model validation.
To assess the fire effects at monthly and annual scales, we conduct FIREOFF simulations branching from the FIREON simulations on 1 January of each year between 2000 and 2013 (Fig. 3). Each FIREOFF simulation is run for 2 years with the fire model switched off and all remaining parameters and input data the same as those in FIREON. In both FIREON and FIREOFF simulations, the vegetation distribution is allowed to respond to climate variations, while the fire disturbance is only considered in FIREON. Each 2-year simulation in FIREOFF is regarded as an ensemble member, and there are a total of 14 ensemble members. The corresponding periods in FIREON form 14 paired ensemble members. The fire impacts on vegetation properties and surface energy balance are quantified using the differences between FIREON and FIREOFF ensemble means.
The experimental design introduced above examines the biophysical impacts of fire on surface energy balance due to vegetation removal. To assess how surface-darkening effects influence our previous conclusion, we conduct a sensitivity test (FIREONdark) following the methodology in De Sales et al. (2018): In FIREONdark, surface albedo is reduced to 0.1 for 60 d, after which albedo is returned to the unburned condition to mimic the removal of ash and charcoal by wind and precipitation. The value of the darkening period is taken from Saha et al. (2019), which showed that brightening occurs after 60 d on average in SAF.
2.5 Model input and validation data
Table 1 lists the data used for model input and evaluation. The input datasets for the fire model and land surface model are the same as our earlier study (Huang et al., 2020a) except for the transient crop fraction from Hurtt et al. (2006, 2011). All input datasets are interpolated to 1.0∘ × 1.0∘ spatial and 3-hourly temporal resolution to be used as model forcing.
The simulated fire variables, vegetation productivity, and surface fluxes are evaluated against observations. The Global Fire Emission Database (GFED) is a fire dataset derived primarily from the MODIS satellite (Giglio et al., 2013; van der Werf et al., 2010, 2006). The latest version, GFED4s, has been updated to include the contribution from small fires below the MODIS detection limit (van der Werf et al., 2017). The burned area and carbon emission obtained from https://www.globalfiredata.org/data.html (last access: September 2019) are used to evaluate fire simulation in SAF with a focus on monthly variations. FLUXNET Model Tree Ensemble (FLUXNET-MTE) GPP is upscaled from FLUXNET observations to the global scale using the machine learning technique MTE (Jung et al., 2011). The 1982–2011 FLUXNET-MTE GPP downloaded from https://www.bgc-jena.mpg.de/geodb/projects/Data.php (last access: October 2019) has been resampled to 1.0∘ × 1.0∘ to be compared with SSiB4/TRIFFID-Fire.
The FLUXCOM provides monthly gridded LH and SH estimates at 0.5∘ spatial resolution and monthly steps (http://www.fluxcom.org/EF-Products/, last access: November 2020). The data are derived by merging energy flux measurements from the FLUXNET eddy covariance tower with remote sensing and meteorological data using machine learning techniques (Jung et al., 2019). The FLUXCOM database is comprised of two complementary products for surface fluxes: FLUXCOM-RS integrates the FLUXNET measurements and 2001–2015 MODIS data in machine learning techniques, while FLUXCOM-METEO estimates surface fluxes from daily meteorological data and mean seasonal cycles of satellite data. The dataset is specially designed to quantify global land–atmosphere interactions and provide a benchmark for land surface model simulations.
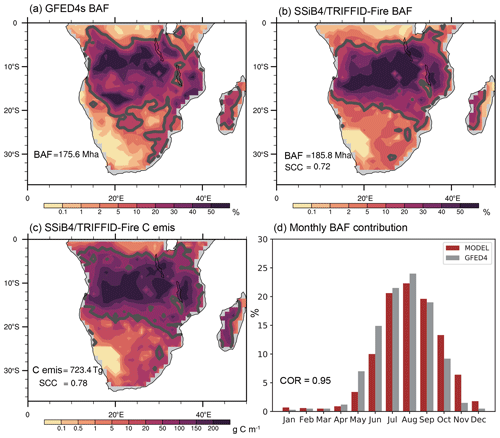
Figure 4(a) Annual burned area fraction (BAF; %) averaged over 2000–2013 in GFED4s; panel (b) is the same as (a) but in SSiB4/TRIFFID-Fire; (c) annual fire carbon emission (C emis; g C m−1) averaged over 2000–2013 in SSiB4/TRIFFID-Fire; and (d) contribution of the monthly burned fraction to the annual burned fraction in model and observation (SCC: spatial correlation coefficient; COR: temporal correlation coefficient).
3.1 Model validation
We first evaluate the model simulation of burned area, carbon emission, vegetation productivity, and surface fluxes in SAF. According to GFED4s, an average of 175.6 Mha land surface is burned each year (Fig. 4a), emitting 678.9 Tg carbon into the atmosphere during 2000–2013. SSiB4/TRIFFID-Fire has captured the magnitude of annual burned area (185.8 Mha) and carbon emission (723.4 Tg C) with spatial correlation coefficients (SCCs) of 0.72 and 0.78, respectively (Fig. 4b, c). Fires are mostly found in central Africa (5 to 20∘ S), extending from the Atlantic coast to Lake Tanganyika. The most extensive burned area is found in savanna with intermediate productivity, where the aboveground biomass and dried soil conditions in the dry season facilitate fire occurrence and spread. Fires in tropical Congolese rainforest and drylands in Namibia and South Africa are constrained by climatic conditions and fuel load, respectively. The observed burned area fraction shows some “hot spots” in Angola, Zambia, and the southern part of Congo; however, the model produces more homogeneously distribution. The heterogeneity in GFED4s may come from landscape fragmentation associated with intensive agriculture, which limits the burned area by reducing fuel connectivity (Bistinas et al., 2014). Although SSiB4/TRIFFID-Fire excludes fire occurrence in agricultural land, it does not consider the effect of landscape fragmentation on fire spread and tends to underestimate the negative impact of cropland on the burned area. Besides, the simulation is conducted at 1.0∘ × 1.0∘ spatial resolution. The relatively coarse model resolution makes it harder to capture the spatial heterogeneity in fire distribution.
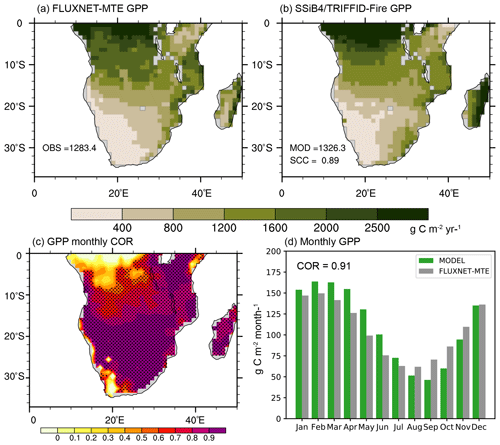
Figure 5Annual GPP (g C m−2 yr−1) averaged over 2000–2011 in (a) FLUXNET-MTE and (b) SSiB4/TRIFFID-Fire; (c) point-by-point climatology monthly correlation between FLUXNET-MTE and SSiB4/TRIFFID-Fire (dots indicate the correlation is significant with p value < 0.05); (d) monthly GPP (g C m−2 month−1) in model and observation.
Fire in SAF is concentrated in the dry season (Fig. 4d). Fire season in SAF is defined as June–October, during which the monthly burned area contributes to more than of the annual burned area (Venevsky et al., 2019). The aboveground dried fuel can be easily ignited and cause extensive fires in the dry season. The monthly burned area drops dramatically at the beginning of the rainy season and remains low until May in the next year. SAF savanna fire has a clear distinction between June–October and November–May, reflecting the contrasting climate during the rainy (non-fire) season and dry (fire) season (Fig. 1d).
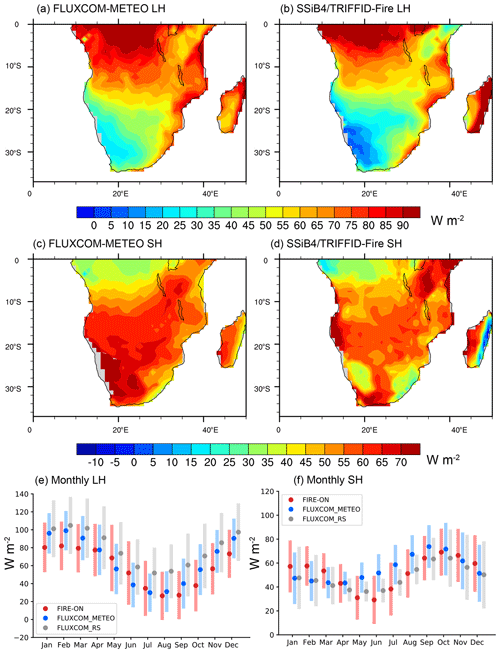
Figure 6Annual LH (W m−2) averaged over 2000–2013 in (a) FLUXCOM-METEO and (b) SSiB4/TRIFFID-Fire; annual SH (W m−2) averaged over 2000–2013 in (c) FLUXCOM-METEO and (d) SSiB4/TRIFFID-Fire; monthly (e) LH and (f) SH for grid within SAF in the model, FLUXCOM-METEO, and FLUXCOM-RS. The dots in panels (e) and (f) denote the regional mean values, while the bars denote the LH/SH values within 1 standard deviation of the mean value of all grid points.
In the following section, we evaluate the model simulation of vegetation productivity and surface fluxes and their seasonality. The model simulation of GPP is evaluated against the FLUXNET-MTE product. The annual average GPP in 2000–2011 is 1283.4 g C m−2 yr−1, ranging from more than 2500 g C m−2 yr−1 in the tropical rainforest to less than 400 g C m−2 yr−1 in the shrubland (Fig. 5a). The GPP magnitude and spatial distribution are simulated in SSiB4/TRIFFID-Fire (1326.3 C m−2 yr−1), with an SCC of 0.89 (Fig. 5b). After optimization of f(θ), the model captures the monthly variations of GPP for most grid cells with a correlation higher than 0.7 (p<0.05; Fig. 5c), showing large improvements compared to the simulation with default parameters (Fig. S2a). The overestimation of GPP, especially from May to October (Fig. S1a), is reduced and the monthly correlation between model and observation increases to 0.91 (Fig. 5d). A similar conclusion can be drawn from LAI before and after parameter optimization, which shows even larger improvements than GPP (Fig. S2c–d). Observational studies have shown that GPP in 3–6 months preceding the fire season is a vital predictor for savanna fire (Forkel et al., 2019). In return, fire can influence the vegetation productivity in the following 2–6 months after fire (Dintwe et al., 2017). SSiB4/TRIFFID-Fire uses aboveground biomass, which is related to vegetation productivity accumulation in preceding wet months, to describe the fuel constraint on fire ignition. The monthly variation of vegetation productivity in the model is in a good consistency to that in observations (Fig. 5d), indicating that SSiB4/TRIFFID-Fire captures some critical processes in fire–vegetation–climate interactions.
The simulated energy fluxes are compared with FLUXCOM datasets to evaluate the surface fluxes partitioning between latent heat (LH) and sensible heat (SH). The spatial distribution of LH shows a predominant latitudinal distribution with a strong N–S gradient in both FLUXCOM-METEO and model, decreasing from 100 W m−2 in tropical Congolese forest to less than 30 W m−2 in the Kalahari Desert (Fig. 6a, b). Regions east of Lake Tanganyika have a much smaller annual precipitation (600 mm yr−1 in Fig. 1b) and therefore have a smaller LH than the west part of SAF. In contrast, SH in SAF peaks in the Kalahari Desert and gradually decreases towards the tropical forest (Fig. 6c). SSiB4/TRIFFID-Fire captures the latitudinal distribution of SH yet underestimates its magnitude in the desert (Fig. 6d).
We further compare the monthly variations of LH and SH in SSiB4/TRIFFID-Fire against the observation-derived datasets, FLUXCOM-METEO and FLUXCOM-RS. There is a high agreement in the regional average and range of LH/SH between the model and observations (Fig. 6e–f). The LH peaks in the rainy season (December–January–February; DJF) and gradually declines and reaches the minimum in June–July–August (JJA). SSiB4/TRIFFID-Fire tends to underestimate LH in the wet season but accurately simulates its magnitude in the dry season. It captures the peak of SH in September–October while slightly overestimating it in January–February–March. Jung et al. (2019) pointed out that FLUXCOM LH estimates in Africa are larger than other observation-based datasets. As such, the simulated LH may compensate for the wet bias in FLUXCOM datasets. Overall, the model is shown to reproduce the annual surface fluxes distribution and their seasonality in SAF.
The comparison with observations shows that SSiB4/TRIFFID-Fire is capable of reproducing the annual mean and intra-annual variations of fire regimes in SAF. The seasonal cycle of vegetation productivity and surface energy fluxes are captured, indicating the fire-coupled vegetation model can describe some key processes in the feedback between fire, vegetation, and surface energy. The fire-coupled DGVM is then used to provide the first model quantification of short-term (monthly to annual scales) fire effects by assessing the differences between FIREON and FIREOFF from June to May in the next year, including a complete fire season (June–October) and post-fire recovery season (November to May in the next year).
3.2 Fire effects on vegetation
The reoccurrence of fire changes the fractional coverage of trees and short PFTs (C3 and C4 grasses) in SAF. Figure 7a and b show the spatial distribution of trees (BET and BDT) and grasses (C3 and C4 grasses) in FIREOFF. Tree PFTs cover more than 80 % in equatorial Africa and the east coast of Africa, while C3 and C4 grasses dominate widespread areas between 5 and 20∘ S and the eastern portion of the Great Escarpment. The reduction in tree cover ranges from 0.2 % to 0.6 % per year in Africa savanna and can exceed 1 % in the transition zone between savanna and the Congolese forest (Fig. 7c; FIREON minus FIREOFF), indicating fire is an important contributor to tropical deforestation (Hansen et al., 2013). A decrease in C3/C4 grasses fraction by 4 %–8 % is found in regions with an annual burned area fraction greater than 10 %, and the magnitude of change is generally proportional to the grid burned area fraction (Fig. 7d). Looking further into the grass cover change per burned area fraction, we find a larger fire impact in drier regions with annual rainfall smaller than 600 mm yr−1 and with GPP generally smaller than 800 g C m−2 yr−1 (not shown). The larger fire effects could be explained by the slower recovery after fire corresponding to the lower vegetation productivity.
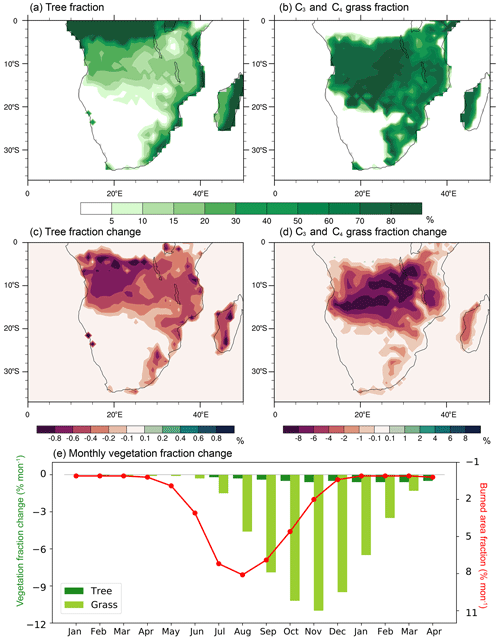
Figure 72000–2013 annual (a) tree fraction (%) and (b) C4 and C3 grass fraction (%) in FIREOFF; 2000–2013 annual fire effects on (c) tree fraction and (d) grass fraction, and (e) monthly fire effects on the fractional coverage of trees and C4 and C3 grasses (% month−1) with burned area fraction (% month−1, red curve) overlaid (the areal-average fire effect is calculated using grids with an annual burned area fraction >10 %). The y axis of burned area fraction in panel (e) is reversed.
Figure 7e shows the monthly vegetation cover change in the fire season and the following rainy season (FIREON minus FIREOFF). The dark green and light green bars denote changes in tree cover and grass cover between FIREON and FIREOFF, which are linked to the monthly burned area fraction denoted by the red line. In the FIREON simulation with a spatial resolution of 1.0∘ × 1.0∘, a grid can have fire-induced vegetation decreasing in parts of the grid while vegetation growing in other parts. The overall vegetation cover change for a grid is the combined result of fire-caused vegetation removal and growth expansion, which depends on meteorological conditions and species. We find little burned area fraction during the precedent wet season from January through May, during which vegetation removal by fire is negligible. While the monthly burned area fraction peaks in August and gradually decreases after that, regional fire disturbances on C3 and C4 grasses accumulate during the entire fire season until November. The vegetation recovery is limited during June–October when the arid conditions produce very small vegetation productivity. In the dry season, the monthly precipitation is generally smaller than 20 mm and GPP is 50 g C m−2 month−1, only one-third of that in the wet months (Fig. 5d). Fire effects reach the maximum in November when a decrease of 11 % is found in the grass coverage. Vegetation recovery is accelerated in the rainy season and grass cover reduction is gradually diminished from December to April in the following year. At the end of the rainy season, little difference in the grass cover is found between FIREON and FIREOFF. Observational studies support our conclusion that the vegetation in SAF savanna is highly adaptive to fire and can mostly recover to unburned conditions within 8 d to 12 months (Dintwe et al., 2017; Araújo et al., 2017; das Chagas and Pelicice, 2018).
Different from grass PFTs, tree cover change caused by fire barely recovers within one growing season (Fig. 7e). This low fire adaptivity makes trees highly vulnerable to fire, especially at the periphery of tropical forests. Our finding corroborates results from observational studies that fire plays a key role in tropical forest loss (Cochrane et al., 1999; Hansen et al., 2013). Meanwhile, fire-induced forest clearance facilitates the growth and spread of grasses, allowing for the coexistence between trees and grasses in the savanna ecosystems which would otherwise be encroached by trees (Higgins et al., 2007; Furley et al., 2008).
The removal of vegetation canopy has caused a reduction in canopy area and vegetation productivity, reflected by the changes of LAI and GPP (Fig. 8a–b). For most parts of SAF savanna, fire has caused a relative change of LAI by −3 % to −5 %, whose magnitude is proportional to the burned area fraction. Over regions with a burned area fraction higher than 10 %, we find a decrease of LAI by 0.10 m2 m−2 on average, accompanied by a decrease of vegetation height by 0.17 m (not shown). The fire impact on LAI accumulates in the dry season, during which the LAI reduction by fire outcompetes the recovery (Fig. 8c). The fire impact on LAI peaks in November when a relative change by −11 % is found. At the end of the recovery season, there is still a small difference of 1 % in the grid-average LAI corresponding to the long-lasting tree cover loss. A greater magnitude of relative change (−5 % to −7 %) is found in vegetation productivity (GPP and NPP) caused by fire. Overall, we find fire causes an annual average reduction of GPP and NPP by 59 and 32 g C yr−1 in regions with a burned area fraction higher than 10 %. The changes in vegetation cover and properties (GPP, NPP, LAI, and vegetation height) influence the radiation absorbed by the surface and the energy partitioning between LH and SH.
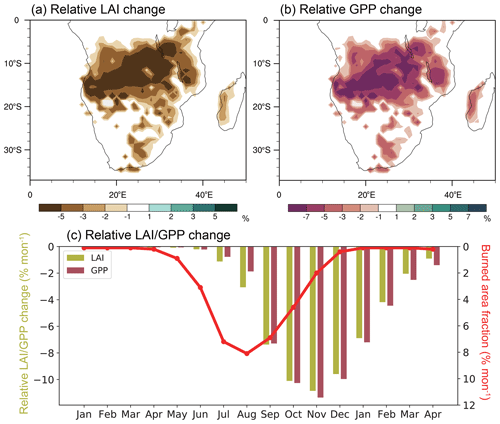
Figure 82000–2013 annual fire effects on (a) LAI (%) and (b) GPP (%), and (c) monthly fire effects on the LAI/GPP (% month−1) with burned area fraction (% month−1) overlaid (the areal-average fire effect is calculated using grids with an annual burned area fraction >10 %). The y axis of burned fraction in panel (c) is reversed.
3.3 Fire effects on surface energy
Over SAF, a decrease of net shortwave radiation (NSW) on surface is found by an average of 0.60 W m−2 (Fig. 9a). The magnitude of change ranges between −2.9 and 0.8 W m−2 (Fig. 9d), generally increasing with the annual burned area fraction. The consumption of vegetation canopy during the fire season has caused exposure of bare soil, which generally has a higher reflectance than vegetation canopy. Therefore, the surface NSW is reduced between June and November (Fig. 10a), especially in October (−1.5 W m−2) when the arid soil has distinct contrast in surface albedo compared to the surrounding vegetation area. The bare soil albedo is largely decreased when the soil is moist by rain, and the corresponding NSW change quickly diminishes. The differences in NSW are mostly invisible after December.
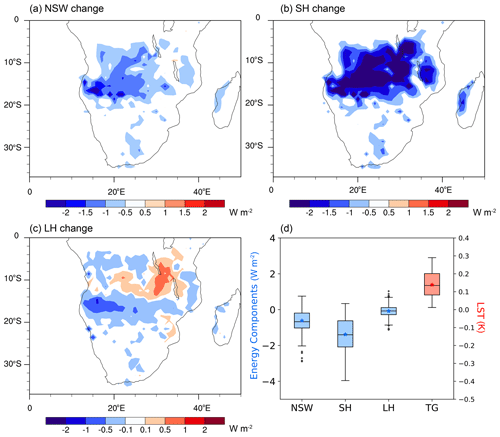
Figure 92000–2013 annual fire effects on (a) NSW (W m−2), (b) SH (W m−2), (c) LH (W m−2). (d) Box plot of annual fire effects on NSW, SH, LH, and TG (K) for grids with an annual burned fraction >10 % within SAF, with medians (middle bars), interquartile ranges (between 25th and 75th percentiles; boxes), maxima/minima (whiskers) within interquartile ranges, and outliers (“+”). The blue and red asterisks (*) denote the areal-weighted mean fire effects in SAF.
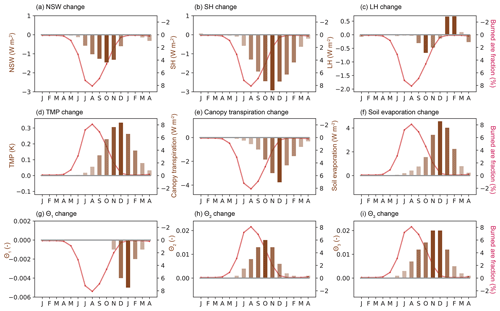
Figure 102000–2013 monthly fire effects on (a) NSW (W m−2), (b) SH (W m−2), (c) LH (W m−2), (d) TMP (K), (e) canopy transpiration (W m−2), (f) soil evaporation (W m−2), (g) θ1, (h) θ2, and (i) θ3 with burned area fraction (%) overlaid. The y axes of burned fraction in panels (a)–(c), (e), and (g) are reversed.
The vegetation removal after fires has reduced the grid-average vegetation height, corresponding to a decrease in surface roughness length and an increase in aerodynamic resistance. The changes of aerodynamic features influence near-surface drag force, affecting the sensible heat flux exchange between land and atmosphere (Liu et al., 2016). In this experiment, we find a widespread reduction of SH by 0.3–4.0 W m−2 with a regional average of 1.4 W m−2 (Fig. 9b). The magnitude of change is generally proportional to the grid's annual burned area fraction (Fig. 4b). The monthly fire effect on SH peaks in November, producing a decrease by 2.9 W m−2 in regions with an annual burned area fraction greater than 10 %. There is a small LH change (−0.1 W m−2) at the annual scale (Fig. 9c) due to the opposite changes in canopy transpiration by −1.9 W m−2 and surface evaporation by 1.8 W m−2 (discussed later). Overall, we find a slight increase in surface temperature by 0.14 K (Fig. 9d), which peaks in December (0.33 K; Fig. 10d). Despite decreasing NSW after fire, surface fluxes changes, especially SH, seem to dominate the temperature changes in tropical savanna. Aside from this, SH and LH are important non-radiative heating sources to the atmosphere. The decrease of SH and LH is expected to cause an atmospheric cooling and subsidence near the surface, negatively influencing convective precipitations (De Sales et al., 2018; Saha et al., 2016). Fire effects on precipitation are not simulated as we use fixed precipitations from the forcings. Yet it can be anticipated that the reduced atmospheric heating can largely suppress convective storms at the beginning of the rainy season and even impede monsoon progression.
In the fire season, a maximum LH reduction by 0.7 W m−2 is found in October, while in the following rainy season, the simulated LH is enhanced by 0.7 W m−2 in February (Fig. 10c). The change of LH is related to the opposing changes of canopy transpiration and soil evaporation. When fire occurs, the removal of vegetation leaf area (Fig. 8a) has caused a decline in canopy transpiration (Fig. 10e), accompanied by increases in root-zone soil moisture (Fig. 10h) and deeper layer soil moisture (Fig. 10i) when less soil water is transported to the atmosphere. Previous studies showed that the removal of dense plant canopy caused a smaller surface resistance for soil evaporation (Schulze et al., 1994), especially when the soil was nearly saturated (Dunin, 1987; Gholz and Clark, 2002). Indeed, we find an increase in soil evaporation throughout our study period. The increase is weak during the dry season as there is not much evaporable water (Fig. 10f), yet it is greatly enhanced after November when the soil is refilled after rain. The elevated soil evaporation has caused a decrease in the surface layer soil moisture (Fig. 10g), which is confined in wet months when soil is moist by rains.
3.4 Darkening effects due to ash deposition
The inclusion of soil-darkening effects does not affect the simulation of the annual burned area, carbon emission, and GPP, as we find the relative differences less than 0.002 % and SCC higher than 0.99 between FIREONdark and FIREON. The fire impacts on LAI and GPP calculated using FIREONdark minus FIREOFF are −0.10 m2 m−2 and −61 g C yr−1, respectively, with the largest effect occurring in November. The annual mean fire effects on vegetation productivity and their monthly variations are highly consistent with those we find in FIREON minus FIREOFF. When soil darkening is considered, we find an annual increase of NSW by 0.07 W m−2 in SAF, which is opposite to our findings in Sect. 3.3 that fire has decreased NSW by 0.60 W m−2. Despite the opposite change in NSW associated with the darkened surface, the responses in SH and LH are consistent with/without darkening effects. We find a decrease in SH and LH by 1.0 and 0.1 W m−2 between FIREONdark and FIREOFF, respectively, and the corresponding change for canopy transpiration and soil evaporation is −1.9 and 1.8 W m−2. An increase in TMP by 0.17 K is simulated when the surface-darkening effect is included (the difference between FIREONdark and FIREOFF), slightly higher than the difference between FIREON and FIREOFF (0.14 K). Although NSW changes are opposite with and without soil-darkening effects, the similar magnitude of change in SH and LH indicates that surface flux changes are dominated by aerodynamic/canopy resistance rather than surface radiation in the tropics, in accordance with our previous finding in a land degradation experiment (Huang et al., 2020b).
We acknowledge that uncertainties may be induced as we assign a 60 d recovery period and an albedo of 0.1 to mimic soil-darkening effects for all pixels regardless of the background climate, vegetation type, soil properties, and the months when fire occurs. All these factors may play a role in the immediate albedo anomalies after ash deposition, the amount of brightening, and the evolution of radiative forcing after fire (Dintwe et al., 2017; Saha et al., 2017). Due to the limited number of observations and the variety in post-fire albedo anomalies, we are currently unable to constrain the uncertainty in the description of the surface-darkening effects. Therefore, the purpose of sensitivity tests is to investigate how surface-darkening effects affect our conclusions in Sect. 3.2 and 3.3, rather than to provide a quantitative estimate on the uncertainties range induced by surface darkening.
4.1 Limitation and uncertainty
This is an offline study without atmospheric feedback. As such, the fire effect is not fully assessed. Previous studies show that atmospheric feedback may influence the SH and LH changes by altering the temperature and moisture gradient between land and atmosphere (Huang et al., 2020b), which cannot be represented in offline simulations as we used fixed atmospheric conditions from the forcing. However, we expect our findings to be valid in coupled-model simulations as surface fluxes changes by fire are shown to be dominated by resistance changes. Besides, De Sales et al. (2018) showed that fire decreased the atmospheric convective instability, which subsequently suppressed precipitation in the following rainy season. The precipitation changes may exert negative feedback on evapotranspiration and vegetation recovery. A full description of the feedback between fire, vegetation, and climate needs to be accomplished next in atmospheric models coupled with fire–vegetation models to further understand the interactions.
There are also some uncertainties in this study due to the model-dependent representation of fire effects on vegetation. The fire impact in SSiB4/TRIFFID-Fire is represented in a relatively simple way using the PFT-specific combustion completeness and mortality factors. This study has made extensive validation of fire regimes and vegetation productivity to make sure that the model captured key processes in fire–vegetation interactions at monthly to annual scales. We notice, however, that some models (for example, MCFIRE and SPITFIRE) have more complex process-based treatments of post-fire effects in which tree damage is determined by fire intensity, residence time, and tree canopy height (Lenihan et al., 1998; Thonicke et al., 2010). These models are supposed to capture the varying fire effects for trees of different heights, as observational studies reported greater damage for younger and smaller trees whose crown could be completely scorched by fire (Higgins et al., 2000; Sankaran et al., 2008). However, it is still unclear if these sophisticated treatments improve the simulation of fire regimes (Hantson et al., 2020) and adequately produce vegetation responses to fire at different timescales. To evaluate the robustness of model results and address the uncertainties in the simulated fire effects, we argue that more simulations should be conducted using different land surface models, DGVMs, and fire models to quantify the short-term fire effects on vegetation dynamics and surface energy budget.
4.2 Conclusions
Fire modifies vegetation dynamics and surface properties. These biogeophysical effects influence the energy fluxes exchanges, hydrology cycle, and regional and global climate. A property quantification of the short-term fire effects is critical to understanding the role of fire in Earth's ecosystems and climate and to providing proper information for societal mitigation activity. This study applied the SSiB4/TRIFFID-Fire to investigate the monthly to annual-scale fire impact in southern Africa, where fire acts as an essential determinant to the structure and functioning of the local ecosystems. The model is shown to reproduce the fire regimes, vegetation productivity, and surface fluxes compared to observation-derived datasets. A sensitivity test is also conducted to assess the possible soil-darkening effects on the model simulated fire impact.
Fire has caused an annual reduction in grass cover by 4 %–8 % for most areas in the SAF savanna. The largest reduction is found at the beginning of the rainy season (November), which quickly diminishes before the next fire season. The reduction of tree cover is concentrated at the transition zone between tropical forests and savannas and is irreversible within one season. The low fire adaptivity of tree PFTs makes it highly vulnerable to fire and can cause large-scale deforestation in extreme years. The canopy removal has caused an annual reduction in LAI and GPP by 3 %–5 % by 5 %–7 %, respectively. The largest productivity change is found in November when both LAI and GPP are reduced by 11 %.
The bare soil exposure after fire has caused an increase in albedo and thus a decrease of net shortwave radiation absorbed by the surface. Sensible heat is decreased by 1.4 W m−2 due to an increase in aerodynamic resistance. Canopy transpiration has dropped as well, which, however, is compensated by the increase in soil evaporation, producing a small annual effect on latent heat (0.1 W m−2). The fire impact on vegetation, surface fluxes, and soil moisture are highly consistent in simulations with/without the descriptions of aerosol deposition. Nevertheless, surface darkening related to aerosol deposition has enhanced the net shortwave absorption, elevating surface warming effects due to fire.
Our results provide quantitative assessments of the regional fire effects over southern Africa and highlight their distinct characteristics on trees and grasses. For grass-dominant areas where fires consume more than 10 % of vegetation cover each year, fire effects on surface energy generally diminish within the rainy season, reflecting the high resilience of grasses to fire disturbance. In contrast, while forest fires have a smaller burned area, their effects are irreversible within one growing season and can cause large-scale deforestation at forests boundaries if accumulated for hundreds of years.
The source code of fire model is archived at https://zenodo.org/record/4670922#.YG4lJ2T0lqs (https://doi.org/10.5281/zenodo.4670922, hhllbao93, 2021).
The fire model output used in this study is archived at https://zenodo.org/record/5778443#.YbjYAfHMLUJ (https://doi.org/10.5281/zenodo.5778443, Huang, 2021).
The supplement related to this article is available online at: https://doi.org/10.5194/gmd-14-7639-2021-supplement.
HH conducted the simulation under the suggestions from YL and drafted the text and made the figures. All authors (HH, YX, YL, FL, and GSO) have contributed to the analysis methods and to the text.
The contact author has declared that neither they nor their co-authors have any competing interests.
Publisher's note: Copernicus Publications remains neutral with regard to jurisdictional claims in published maps and institutional affiliations.
The authors acknowledge Cheyenne (https://doi.org/10.5065/D6RX99HX), provided by NCAR CISL, for providing HPC resources.
This research has been supported by the Division of Atmospheric and Geospace Sciences (grant nos. 1419526 and 1849654). Fang Li received support from the National Key R&D Program of China (grant nos. 2017YFA0604302 and 2017YFA0604804) and National Natural Science Foundation of China (grant no. 41875137).
This paper was edited by Hisashi Sato and reviewed by two anonymous referees.
Andela, N., Morton, D. C., Giglio, L., Chen, Y., van der Werf, G. R., Kasibhatla, P. S., DeFries, R. S., Collatz, G. J., Hantson, S., Kloster, S., Bachelet, D., Forrest, M., Lasslop, G., Li, F., Mangeon, S., Melton, J. R., Yue, C., and Randerson, J. T.: A human-driven decline in global burned area, Science, 356, 1356–1361, https://doi.org/10.1126/science.aal4108, 2017.
Araújo, F. D. C., Tng, D. Y. P., Apgaua, D. M. G., Coelho, P. A., Pereira, D. G. S., and Santos, R. M.: Post-fire plant regeneration across a closed forest-savanna vegetation transition, Forest Ecol. Manag., 400, 77–84, https://doi.org/10.1016/j.foreco.2017.05.058, 2017.
Arora, V. K. and Boer, G. J.: Fire as an interactive component of dynamic vegetation models, J. Geophys. Res.-Biogeo., 110, G02008, https://doi.org/10.1029/2005jg000042, 2005.
Bartholome, E. and Belward, A. S.: GLC2000: a new approach to global land cover mapping from Earth observation data, Int. J. Remote Sens., 26, 1959–1977, 2005.
Beringer, J., Hutley, L. B., Tapper, N. J., Coutts, A., Kerley, A., and O'Grady, A. P.: Fire impacts on surface heat, moisture and carbon fluxes from a tropical savanna in northern Australia, Int. J. Wildland Fire, 12, 333–340, https://doi.org/10.1071/Wf03023, 2003.
Bistinas, I., Harrison, S. P., Prentice, I. C., and Pereira, J. M. C.: Causal relationships versus emergent patterns in the global controls of fire frequency, Biogeosciences, 11, 5087–5101, https://doi.org/10.5194/bg-11-5087-2014, 2014.
Bond, W. J. and Midgley, G. F.: Carbon dioxide and the uneasy interactions of trees and savannah grasses, Philos T. R. Soc. B, 367, 601–612, https://doi.org/10.1098/rstb.2011.0182, 2012.
Bond, W. J., Woodward, F. I., and Midgley, G. F.: The global distribution of ecosystems in a world without fire, New Phytol., 165, 525–537, https://doi.org/10.1111/j.1469-8137.2004.01252.x, 2005.
Bond-Lamberty, B., Peckham, S. D., Gower, S. T., and Ewers, B. E.: Effects of fire on regional evapotranspiration in the central Canadian boreal forest, Global Change Biol., 15, 1242–1254, https://doi.org/10.1111/j.1365-2486.2008.01776.x, 2009.
Bowman, D. M., Balch, J. K., Artaxo, P., Bond, W. J., Carlson, J. M., Cochrane, M. A., D'Antonio, C. M., Defries, R. S., Doyle, J. C., Harrison, S. P., Johnston, F. H., Keeley, J. E., Krawchuk, M. A., Kull, C. A., Marston, J. B., Moritz, M. A., Prentice, I. C., Roos, C. I., Scott, A. C., Swetnam, T. W., van der Werf, G. R., and Pyne, S. J.: Fire in the Earth system, Science, 324, 481–484, https://doi.org/10.1126/science.1163886, 2009.
Burton, C., Betts, R., Cardoso, M., Feldpausch, T. R., Harper, A., Jones, C. D., Kelley, D. I., Robertson, E., and Wiltshire, A.: Representation of fire, land-use change and vegetation dynamics in the Joint UK Land Environment Simulator vn4.9 (JULES), Geosci. Model Dev., 12, 179–193, https://doi.org/10.5194/gmd-12-179-2019, 2019.
Chambers, S. D. and Chapin, F. S.: Fire effects on surface-atmosphere energy exchange in Alaskan black spruce ecosystems: Implications for feedbacks to regional climate, J. Geophys. Res.-Atmos., 107, 8145, https://doi.org/10.1029/2001jd000530, 2002.
CIESIN (Center for International Earth Science Information Network): Gridded population of the world version 3(GPWv3): Population density grids, Technical report, Socioeconomic Data and Applications Center (SEDAC), Columbia University, Palisades, New York, USA, available at: http://sedac.ciesin.columbia.edu/gpw (last access: July 2019), 2005.
Cochrane, M. A., Alencar, A., Schulze, M. D., Souza, C. M., Nepstad, D. C., Lefebvre, P., and Davidson, E. A.: Positive feedbacks in the fire dynamic of closed canopy tropical forests, Science, 284, 1832–1835, 1999.
Cox, P.: Description of the “TRIFFID” Dynamic Global Vegetation Model, Hadley Centre technical note 24, Hadley Centre, Exeter, UK, 1–16, 2001.
das Chagas, D. and Pelicice, F.: Response of vegetation to fire disturbance: short-term dynamics in two savanna physiognomies, Community Ecol., 19, 211–222, 2018.
De Sales, F., Xue, Y. K., and Okin, G. S.: Impact of burned areas on the northern African seasonal climate from the perspective of regional modeling, Clim. Dynam., 47, 3393–3413, https://doi.org/10.1007/s00382-015-2522-4, 2016.
De Sales, F., Okin, G. S., Xue, Y., and Dintwe, K.: On the effects of wildfires on precipitation in Southern Africa, Clim. Dynam., 52, 951–967, https://doi.org/10.1007/s00382-018-4174-7, 2018.
Dintwe, K., Okin, G. S., and Xue, Y. K.: Fire-induced albedo change and surface radiative forcing in sub-Saharan Africa savanna ecosystems: Implications for the energy balance, J. Geophys. Res.-Atmos., 122, 6186–6201, https://doi.org/10.1002/2016jd026318, 2017.
Dunin, F. X.: Run-off and drainage from grassland catchments, in: Managed Grasslands, B. Analytical Studies, edited by: Snaydon, R. W., 205–213, https://doi.org/102.100.100/269549?index=1, 1987.
Forkel, M., Andela, N., Harrison, S. P., Lasslop, G., van Marle, M., Chuvieco, E., Dorigo, W., Forrest, M., Hantson, S., Heil, A., Li, F., Melton, J., Sitch, S., Yue, C., and Arneth, A.: Emergent relationships with respect to burned area in global satellite observations and fire-enabled vegetation models, Biogeosciences, 16, 57–76, https://doi.org/10.5194/bg-16-57-2019, 2019.
Furley, P. A., Rees, R. M., Ryan, C. M., and Saiz, G.: Savanna burning and the assessment of long-term fire experiments with particular reference to Zimbabwe, Prog. Phys. Geog., 32, 611–634, 2008.
Gatebe, C. K., Ichoku, C. M., Poudyal, R., Roman, M. O., and Wilcox, E.: Surface albedo darkening from wildfires in northern sub-Saharan Africa, Environ. Res. Lett., 9, 065003, https://doi.org/10.1088/1748-9326/9/6/065003, 2014.
Gholz, H. L. and Clark, K. L.: Energy exchange across a chronosequence of slash pine forests in Florida, Agr. Forest Meteorol., 112, 87–102, 2002.
Giglio, L., Randerson, J. T., and van der Werf, G. R.: Analysis of daily, monthly, and annual burned area using the fourth-generation global fire emissions database (GFED4), J. Geophys. Res.-Biogeo., 118, 317–328, https://doi.org/10.1002/jgrg.20042, 2013.
Giglio, L., Boschetti, L., Roy, D. P., Humber, M. L., and Justice, C. O.: The Collection 6 MODIS burned area mapping algorithm and product, Remote Sens. Environ., 217, 72–85, https://doi.org/10.1016/j.rse.2018.08.005, 2018.
Goldewijk, K. K., Beusen, A., and Janssen, P.: Long-term dynamic modeling of global population and built-up area in a spatially explicit way: HYDE 3.1, Holocene, 20, 565–573, 2010.
Govaerts, Y. M., Pereira, J. M., Pinty, B., and Mota, B.: Impact of fires on surface albedo dynamics over the African continent, J. Geophys. Res.-Atmos., 107, ACL 8-1–ACL 8-12, https://doi.org/10.1029/2002JD002388, 2002.
Hamilton, D. S., Hantson, S., Scott, C. E., Kaplan, J. O., Pringle, K. J., Nieradzik, L. P., Rap, A., Folberth, G. A., Spracklen, D. V., and Carslaw, K. S.: Reassessment of pre-industrial fire emissions strongly affects anthropogenic aerosol forcing, Nat. Commun., 9, 3182, https://doi.org/10.1038/s41467-018-05592-9, 2018.
Hansen, M. C., Potapov, P. V., Moore, R., Hancher, M., Turubanova, S. A., Tyukavina, A., Thau, D., Stehman, S., Goetz, S. J., and Loveland, T. R.: High-resolution global maps of 21st-century forest cover change, Science, 342, 850–853, 2013.
Hantson, S., Kelley, D. I., Arneth, A., Harrison, S. P., Archibald, S., Bachelet, D., Forrest, M., Hickler, T., Lasslop, G., Li, F., Mangeon, S., Melton, J. R., Nieradzik, L., Rabin, S. S., Prentice, I. C., Sheehan, T., Sitch, S., Teckentrup, L., Voulgarakis, A., and Yue, C.: Quantitative assessment of fire and vegetation properties in simulations with fire-enabled vegetation models from the Fire Model Intercomparison Project, Geosci. Model Dev., 13, 3299–3318, https://doi.org/10.5194/gmd-13-3299-2020, 2020.
Harper, A. B., Cox, P. M., Friedlingstein, P., Wiltshire, A. J., Jones, C. D., Sitch, S., Mercado, L. M., Groenendijk, M., Robertson, E., Kattge, J., Bönisch, G., Atkin, O. K., Bahn, M., Cornelissen, J., Niinemets, Ü., Onipchenko, V., Peñuelas, J., Poorter, L., Reich, P. B., Soudzilovskaia, N. A., and Bodegom, P. V.: Improved representation of plant functional types and physiology in the Joint UK Land Environment Simulator (JULES v4.2) using plant trait information, Geosci. Model Dev., 9, 2415–2440, https://doi.org/10.5194/gmd-9-2415-2016, 2016.
hhllbao93: hhllbao93/SSiB4-TRIFFID-Fire: Release of SSiB4/TRIFFID-Fire v1.1 (v1.1), Zenodo [code], https://doi.org/10.5281/zenodo.4670922, 2021.
Higgins, S. I., Bond, W. J., and Trollope, W. S. W.: Fire, resprouting and variability: a recipe for grass-tree coexistence in savanna, J. Ecol., 88, 213–229, https://doi.org/10.1046/j.1365-2745.2000.00435.x, 2000.
Higgins, S. I., Bond, W. J., February, E. C., Bronn, A., Euston-Brown, D. I. W., Enslin, B., Govender, N., Rademan, L., O'Regan, S., Potgieter, A. L. F., Scheiter, S., Sowry, R., Trollope, L., and Trollope, W. S. W.: Effects of four decades of fire manipulation on woody vegetation structure in savanna, Ecology, 88, 1119–1125, 2007.
Huang, H., Xue, Y., Li, F., and Liu, Y.: Modeling long-term fire impact on ecosystem characteristics and surface energy using a process-based vegetation–fire model SSiB4/TRIFFID-Fire v1.0, Geosci. Model Dev., 13, 6029–6050, https://doi.org/10.5194/gmd-13-6029-2020, 2020a.
Huang, H., Xue, Y., Chilukoti, N., Liu, Y., Chen, G., and Diallo, I.: Assessing Global and Regional Effects of Reconstructed Land-Use and Land-Cover Change on Climate since 1950 Using a Coupled Land–Atmosphere–Ocean Model, J. Climate, 33, 8997–9013, 2020b.
Huang, H.: Data for “Modeling the short-term fire effects on vegetation dynamics and surface energy in southern Africa”, Zenodo [data set], https://doi.org/10.5281/zenodo.5778443, 2021.
Hurtt, G. C., Frolking, S., Fearon, M. G., Moore, B., Shevliakova, E., Malyshev, S., Pacala, S. W., and Houghton, R. A.: The underpinnings of land-use history: three centuries of global gridded land-use transitions, wood-harvest activity, and resulting secondary lands, Global Change Biol., 12, 1208–1229, https://doi.org/10.1111/j.1365-2486.2006.01150.x, 2006.
Hurtt, G. C., Chini, L. P., Frolking, S., Betts, R. A., Feddema, J., Fischer, G., Fisk, J. P., Hibbard, K., Houghton, R. A., Janetos, A., Jones, C. D., Kindermann, G., Kinoshita, T., Goldewijk, K. K., Riahi, K., Shevliakova, E., Smith, S., Stehfest, E., Thomson, A., Thornton, P., van Vuuren, D. P., and Wang, Y. P.: Harmonization of land-use scenarios for the period 1500-2100: 600 years of global gridded annual land-use transitions, wood harvest, and resulting secondary lands, Climatic Change, 109, 117–161, https://doi.org/10.1007/s10584-011-0153-2, 2011.
Jiang, Y., Lu, Z., Liu, X., Qian, Y., Zhang, K., Wang, Y., and Yang, X.-Q.: Impacts of global open-fire aerosols on direct radiative, cloud and surface-albedo effects simulated with CAM5, Atmos. Chem. Phys., 16, 14805–14824, https://doi.org/10.5194/acp-16-14805-2016, 2016.
Jin, Y. and Roy, D. P.: Fire-induced albedo change and its radiative forcing at the surface in northern Australia, Geophys. Res. Lett., 32, L13401, https://doi.org/10.1029/2005GL022822, 2005.
Jung, M., Reichstein, M., Margolis, H. A., Cescatti, A., Richardson, A. D., Arain, M. A., Arneth, A., Bernhofer, C., Bonal, D., Chen, J. Q., Gianelle, D., Gobron, N., Kiely, G., Kutsch, W., Lasslop, G., Law, B. E., Lindroth, A., Merbold, L., Montagnani, L., Moors, E. J., Papale, D., Sottocornola, M., Vaccari, F., and Williams, C.: Global patterns of land-atmosphere fluxes of carbon dioxide, latent heat, and sensible heat derived from eddy covariance, satellite, and meteorological observations, J. Geophys. Res.-Biogeo., 116, G00J07, https://doi.org/10.1029/2010JG001566, 2011.
Jung, M., Koirala, S., Weber, U., Ichii, K., Gans, F., Camps-Valls, G., Papale, D., Schwalm, C., Tramontana, G., and Reichstein, M.: The FLUXCOM ensemble of global land-atmosphere energy fluxes, Scientific Data, 6, 74, https://doi.org/10.1038/s41597-019-0076-8, 2019.
Lasslop, G. and Kloster, S.: Human impact on wildfires varies between regions and with vegetation productivity, Environ. Res. Lett., 12, 115011, https://doi.org/10.1088/1748-9326/aa8c82, 2017.
Lasslop, G., Thonicke, K., and Kloster, S.: SPITFIRE within the MPI Earth system model: Model development and evaluation, J. Adv. Model Earth Sy., 6, 740–755, https://doi.org/10.1002/2013ms000284, 2014.
Lasslop, G., Hantson, S., Harrison, S. P., Bachelet, D., Burton, C., Forkel, M., Forrest, M., Li, F., Melton, J. R., Yue, C., Archibald, S., Scheiter, S., Arneth, A., Hickler, T., and Sitch, S.: Global ecosystems and fire: Multi-model assessment of fire-induced tree-cover and carbon storage reduction, Glob. Change Biol., 26, 5027–5041, https://doi.org/10.1111/gcb.15160, 2020.
Lenihan, J. M., Daly, C., Bachelet, D., and Neilson, R. P.: Simulating broad-scale fire severity in a dynamic global vegetation model, Northwest Sci., 72, 91–101, 1998.
Li, F. and Lawrence, D. M.: Role of Fire in the Global Land Water Budget during the Twentieth Century due to Changing Ecosystems, J. Climate, 30, 1893–1908, https://doi.org/10.1175/Jcli-D-16-0460.1, 2017.
Li, F., Bond-Lamberty, B., and Levis, S.: Quantifying the role of fire in the Earth system – Part 2: Impact on the net carbon balance of global terrestrial ecosystems for the 20th century, Biogeosciences, 11, 1345–1360, https://doi.org/10.5194/bg-11-1345-2014, 2014.
Li, F., Zeng, X. D., and Levis, S.: A process-based fire parameterization of intermediate complexity in a Dynamic Global Vegetation Model, Biogeosciences, 9, 2761–2780, https://doi.org/10.5194/bg-9-2761-2012, 2012.
Li, F., Lawrence, D. M., and Bond-Lamberty, B.: Impact of fire on global land surface air temperature and energy budget for the 20th century due to changes within ecosystems, Environ. Res. Lett., 12, 044014, https://doi.org/10.1088/1748-9326/aa6685, 2017.
Li, F., Val Martin, M., Andreae, M. O., Arneth, A., Hantson, S., Kaiser, J. W., Lasslop, G., Yue, C., Bachelet, D., Forrest, M., Kluzek, E., Liu, X., Mangeon, S., Melton, J. R., Ward, D. S., Darmenov, A., Hickler, T., Ichoku, C., Magi, B. I., Sitch, S., van der Werf, G. R., Wiedinmyer, C., and Rabin, S. S.: Historical (1700–2012) global multi-model estimates of the fire emissions from the Fire Modeling Intercomparison Project (FireMIP), Atmos. Chem. Phys., 19, 12545–12567, https://doi.org/10.5194/acp-19-12545-2019, 2019.
Li, W., Du, J., Li, S., Zhou, X., Duan, Z., Li, R., Wu, S., Wang, S., and Li, M.: The variation of vegetation productivity and its relationship to temperature and precipitation based on the GLASS-LAI of different African ecosystems from 1982 to 2013, Int. J. Biometeorol., 63, 847–860, 2019.
Liu, Y., Guo, W. D., and Song, Y. M.: Estimation of key surface parameters in semi-arid region and their impacts on improvement of surface fluxes simulation, Sci. China Earth Sci., 59, 307–319, https://doi.org/10.1007/s11430-015-5140-4, 2016.
Liu, Y., Xue, Y., MacDonald, G., Cox, P., and Zhang, Z.: Global vegetation variability and its response to elevated CO2, global warming, and climate variability – a study using the offline SSiB4/TRIFFID model and satellite data, Earth Syst. Dynam., 10, 9–29, https://doi.org/10.5194/esd-10-9-2019, 2019.
Liu, Z. H., Ballantyne, A. P., and Cooper, L. A.: Biophysical feedback of global forest fires on surface temperature, Nat. Commun., 10, 214, https://doi.org/10.1038/s41467-018-08237-z, 2019.
López-Saldaña, G., Bistinas, I., and Pereira, J. M. C.: Global analysis of radiative forcing from fire-induced shortwave albedo change, Biogeosciences, 12, 557–565, https://doi.org/10.5194/bg-12-557-2015, 2015.
Lyons, E. A., Jin, Y. F., and Randerson, J. T.: Changes in surface albedo after fire in boreal forest ecosystems of interior Alaska assessed using MODIS satellite observations, J. Geophys. Res.-Biogeo., 113, G02012, https://doi.org/10.1029/2007jg000606, 2008.
Myhre, G., Govaerts, Y., Haywood, J. M., Berntsen, T. K., and Lattanzio, A.: Radiative effect of surface albedo change from biomass burning, Geophys. Res. Lett., 32, L20812, https://doi.org/10.1029/2005gl022897, 2005.
Pfeiffer, M., Spessa, A., and Kaplan, J. O.: A model for global biomass burning in preindustrial time: LPJ-LMfire (v1.0), Geosci. Model Dev., 6, 643–685, https://doi.org/10.5194/gmd-6-643-2013, 2013.
Rabin, S. S., Ward, D. S., Malyshev, S. L., Magi, B. I., Shevliakova, E., and Pacala, S. W.: A fire model with distinct crop, pasture, and non-agricultural burning: use of new data and a model-fitting algorithm for FINAL.1, Geosci. Model Dev., 11, 815–842, https://doi.org/10.5194/gmd-11-815-2018, 2018.
Randerson, J. T., Chen, Y., van der Werf, G. R., Rogers, B. M., and Morton, D. C.: Global burned area and biomass burning emissions from small fires, J. Geophys. Res.-Biogeo., 117, G04012, https://doi.org/10.1029/2012jg002128, 2012.
Saha, M. V., Scanlon, T. M., and D'Odorico, P.: Suppression of rainfall by fires in African drylands, Geophys. Res. Lett., 43, 8527–8533, https://doi.org/10.1002/2016gl069855, 2016.
Saha, M. V., D'Odorico, P., and Scanlon, T. M.: Albedo changes after fire as an explanation of fire-induced rainfall suppression, Geophys. Res. Lett., 44, 3916–3923, https://doi.org/10.1002/2017gl073623, 2017.
Saha, M. V., D'Odorico, P., and Scanlon, T. M.: Kalahari Wildfires Drive Continental Post-Fire Brightening in Sub-Saharan Africa, Remote Sens.-Basel, 11, 1090, https://doi.org/10.3390/rs11091090, 2019.
Samain, O., Kergoat, L., Hiernaux, P., Guichard, F., Mougin, E., Timouk, F., and Lavenu, F.: Analysis of the in situ and MODIS albedo variability at multiple timescales in the Sahel, J. Geophys. Res.-Atmos., 113, D14119, https://doi.org/10.1029/2007JD009174, 2008.
Sankaran, M., Ratnam, J., and Hanan, N.: Woody cover in African savannas: the role of resources, fire and herbivory, Global Ecol. Biogeogr., 17, 236–245, 2008.
Scholes, R. J., Ward, D. E., and Justice, C. O.: Emissions of trace gases and aerosol particles due to vegetation burning in southern hemisphere Africa, J. Geophys. Res.-Atmos., 101, 23677–23682, https://doi.org/10.1029/95jd02049, 1996.
Schulze, E. D., Kelliher, F. M., Korner, C., Lloyd, J., and Leuning, R.: Relationships among Maximum Stomatal Conductance, Ecosystem Surface Conductance, Carbon Assimilation Rate, and Plant Nitrogen Nutrition – a Global Ecology Scaling Exercise, Annu. Rev. Ecol. Syst., 25, 629–662, https://doi.org/10.1146/annurev.es.25.110194.003213, 1994.
Seo, H. and Kim, Y.: Interactive impacts of fire and vegetation dynamics on global carbon and water budget using Community Land Model version 4.5, Geosci. Model Dev., 12, 457–472, https://doi.org/10.5194/gmd-12-457-2019, 2019.
Sheffield, J., Goteti, G., and Wood, E. F.: Development of a 50-year high-resolution global dataset of meteorological forcings for land surface modeling, J. Climate, 19, 3088–3111, https://doi.org/10.1175/Jcli3790.1, 2006.
Staal, A., van Nes, E. H., Hantson, S., Holmgren, M., Dekker, S. C., Pueyo, S., Xu, C., and Scheffer, M.: Resilience of tropical tree cover: The roles of climate, fire, and herbivory, Global Change Biol., 24, 5096–5109, 2018.
Staver, A. C., Archibald, S., and Levin, S. A.: The Global Extent and Determinants of Savanna and Forest as Alternative Biome States, Science, 334, 230–232, https://doi.org/10.1126/science.1210465, 2011.
Thonicke, K., Venevsky, S., Sitch, S., and Cramer, W.: The role of fire disturbance for global vegetation dynamics: coupling fire into a Dynamic Global Vegetation Model, Global Ecol. Biogeogr., 10, 661–677, https://doi.org/10.1046/j.1466-822x.2001.00175.x, 2001.
Thonicke, K., Spessa, A., Prentice, I. C., Harrison, S. P., Dong, L., and Carmona-Moreno, C.: The influence of vegetation, fire spread and fire behaviour on biomass burning and trace gas emissions: results from a process-based model, Biogeosciences, 7, 1991–2011, https://doi.org/10.5194/bg-7-1991-2010, 2010.
van der Werf, G. R., Randerson, J. T., Giglio, L., Collatz, G. J., Kasibhatla, P. S., and Arellano Jr., A. F.: Interannual variability in global biomass burning emissions from 1997 to 2004, Atmos. Chem. Phys., 6, 3423–3441, https://doi.org/10.5194/acp-6-3423-2006, 2006.
van der Werf, G. R., Randerson, J. T., Giglio, L., Collatz, G. J., Mu, M., Kasibhatla, P. S., Morton, D. C., DeFries, R. S., Jin, Y., and van Leeuwen, T. T.: Global fire emissions and the contribution of deforestation, savanna, forest, agricultural, and peat fires (1997–2009), Atmos. Chem. Phys., 10, 11707–11735, https://doi.org/10.5194/acp-10-11707-2010, 2010.
van der Werf, G. R., Randerson, J. T., Giglio, L., van Leeuwen, T. T., Chen, Y., Rogers, B. M., Mu, M., van Marle, M. J. E., Morton, D. C., Collatz, G. J., Yokelson, R. J., and Kasibhatla, P. S.: Global fire emissions estimates during 1997–2016, Earth Syst. Sci. Data, 9, 697–720, https://doi.org/10.5194/essd-9-697-2017, 2017.
van Vuuren, D. P., Lucas, P. L., and Hilderink, H.: Downscaling drivers of global environmental change: Enabling use of global SRES scenarios at the national and grid levels, Global Environ. Chang., 17, 114–130, 2007.
Venevsky, S., Thonicke, K., Sitch, S., and Cramer, W.: Simulating fire regimes in human-dominated ecosystems: Iberian Peninsula case study, Glob. Change Biol., 8, 984–998, https://doi.org/10.1046/j.1365-2486.2002.00528.x, 2002.
Venevsky, S., Le Page, Y., Pereira, J. M. C., and Wu, C.: Analysis fire patterns and drivers with a global SEVER-FIRE v1.0 model incorporated into dynamic global vegetation model and satellite and on-ground observations, Geosci. Model Dev., 12, 89–110, https://doi.org/10.5194/gmd-12-89-2019, 2019.
Veraverbeke, S., Verstraeten, W. W., Lhermitte, S., Van de Kerchove, R., and Goossens, R.: Assessment of post-fire changes in land surface temperature and surface albedo, and their relation with fire-burn severity using multitemporal MODIS imagery, Int. J. Wildland Fire, 21, 243–256, 2012.
Ward, D. S., Kloster, S., Mahowald, N. M., Rogers, B. M., Randerson, J. T., and Hess, P. G.: The changing radiative forcing of fires: global model estimates for past, present and future, Atmos. Chem. Phys., 12, 10857–10886, https://doi.org/10.5194/acp-12-10857-2012, 2012.
Wendt, C. K., Beringer, J., Tapper, N. J., and Hutley, L. B.: Local boundary-layer development over burnt and unburnt tropical savanna: an observational study, Bound.-Lay. Meteorol., 124, 291–304, 2007.
Xue, Y., Sellers, P. J., Kinter, J. L., and Shukla, J.: A Simplified Biosphere Model for Global Climate Studies, J. Climate, 4, 345–364, https://doi.org/10.1175/1520-0442(1991)004<0345:asbmfg>2.0.co;2, 1991.
Yue, C., Ciais, P., Cadule, P., Thonicke, K., and van Leeuwen, T. T.: Modelling the role of fires in the terrestrial carbon balance by incorporating SPITFIRE into the global vegetation model ORCHIDEE – Part 2: Carbon emissions and the role of fires in the global carbon balance, Geosci. Model Dev., 8, 1321–1338, https://doi.org/10.5194/gmd-8-1321-2015, 2015.
Zhan, X. W., Xue, Y. K., and Collatz, G. J.: An analytical approach for estimating CO2 and heat fluxes over the Amazonian region, Ecol. Model., 162, 97–117, 2003.
Zhang, Z., Xue, Y., MacDonald, G., Cox, P. M., and Collatz, G. J.: Investigation of North American vegetation variability under recent climate: A study using the SSiB4/TRIFFID biophysical/dynamic vegetation model, J. Geophys. Res.-Atmos., 120, 1300–1321, 2015.
Zou, Y., Wang, Y., Qian, Y., Tian, H., Yang, J., and Alvarado, E.: Using CESM-RESFire to understand climate–fire–ecosystem interactions and the implications for decadal climate variability, Atmos. Chem. Phys., 20, 995–1020, https://doi.org/10.5194/acp-20-995-2020, 2020.