the Creative Commons Attribution 4.0 License.
the Creative Commons Attribution 4.0 License.
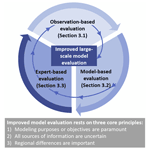
GMD perspective: The quest to improve the evaluation of groundwater representation in continental- to global-scale models
Thorsten Wagener
Petra Döll
Samuel C. Zipper
Charles West
Yoshihide Wada
Richard Taylor
Bridget Scanlon
Rafael Rosolem
Shams Rahman
Nurudeen Oshinlaja
Reed Maxwell
Min-Hui Lo
Hyungjun Kim
Mary Hill
Andreas Hartmann
Graham Fogg
James S. Famiglietti
Agnès Ducharne
Inge de Graaf
Mark Cuthbert
Laura Condon
Etienne Bresciani
Marc F. P. Bierkens
Continental- to global-scale hydrologic and land surface models increasingly include representations of the groundwater system. Such large-scale models are essential for examining, communicating, and understanding the dynamic interactions between the Earth system above and below the land surface as well as the opportunities and limits of groundwater resources. We argue that both large-scale and regional-scale groundwater models have utility, strengths, and limitations, so continued modeling at both scales is essential and mutually beneficial. A crucial quest is how to evaluate the realism, capabilities, and performance of large-scale groundwater models given their modeling purpose of addressing large-scale science or sustainability questions as well as limitations in data availability and commensurability. Evaluation should identify if, when, or where large-scale models achieve their purpose or where opportunities for improvements exist so that such models better achieve their purpose. We suggest that reproducing the spatiotemporal details of regional-scale models and matching local data are not relevant goals. Instead, it is important to decide on reasonable model expectations regarding when a large-scale model is performing “well enough” in the context of its specific purpose. The decision of reasonable expectations is necessarily subjective even if the evaluation criteria are quantitative. Our objective is to provide recommendations for improving the evaluation of groundwater representation in continental- to global-scale models. We describe current modeling strategies and evaluation practices, and we subsequently discuss the value of three evaluation strategies: (1) comparing model outputs with available observations of groundwater levels or other state or flux variables (observation-based evaluation), (2) comparing several models with each other with or without reference to actual observations (model-based evaluation), and (3) comparing model behavior with expert expectations of hydrologic behaviors in particular regions or at particular times (expert-based evaluation). Based on evolving practices in model evaluation as well as innovations in observations, machine learning, and expert elicitation, we argue that combining observation-, model-, and expert-based model evaluation approaches, while accounting for commensurability issues, may significantly improve the realism of groundwater representation in large-scale models, thus advancing our ability for quantification, understanding, and prediction of crucial Earth science and sustainability problems. We encourage greater community-level communication and cooperation on this quest, including among global hydrology and land surface modelers, local to regional hydrogeologists, and hydrologists focused on model development and evaluation.
- Article
(295 KB) - Full-text XML
- BibTeX
- EndNote
Groundwater is the largest human- and ecosystem-accessible freshwater storage component of the hydrologic cycle (UNESCO, 1978; Margat and Van der Gun, 2013; Gleeson et al., 2016). Therefore, better understanding of groundwater dynamics is critical at a time when the “great acceleration” (Steffen et al., 2015) of many human-induced processes is increasing stress on water resources (Wagener et al., 2010; Montanari et al., 2013; Sivapalan et al., 2014; van Loon et al., 2016), especially in regions with limited data availability and analytical capacity. Groundwater is often considered to be an inherently regional rather than global resource or system. This is partially reasonable because local to regional peculiarities of hydrology, politics, and culture are paramount to groundwater resource management (Foster et al., 2013), and groundwater dynamics in different continents are less directly connected and coupled than atmospheric dynamics. Regional-scale analysis and models are essential for addressing local to regional groundwater issues. Generally, regional-scale modeling is a mature, well-established field (Hill and Tiedeman, 2007; Kresic, 2009; Zhou and Li, 2011; Hiscock and Bense, 2014; M. P. Anderson et al., 2015) with clear and robust model evaluation guidelines (e.g., ASTM, 2016; Barnett et al., 2012). Regional models have been developed around the world; for example, Rossman and Zlotnik (2013) and Vergnes et al. (2020) synthesize regional-scale groundwater models across the western United States and Europe, respectively.
Yet, important global aspects of groundwater both as a resource and as part of the Earth system are emerging (Gleeson et al., 2020). First, our increasingly globalized world trades virtual groundwater and other groundwater-dependent resources in the food–energy–water nexus, and groundwater often crosses borders in transboundary aquifers. A solely regional approach can be insufficient for analyzing and managing these complex global interlinkages. Second, from an Earth system perspective, groundwater is part of the hydrological cycle connected to the atmosphere, oceans, and the deeper lithosphere. A solely regional approach is insufficient to uncover and understand the complex interactions of groundwater within the Earth system and teleconnections, which are groundwater levels or flows in one region linked to geographically separated regions via physical or socioeconomic processes. Regional approaches generally focus on important aquifers, which underlie only a portion of the world's land mass or population and do not include many other parts of the land surface that may be important for processes like surface water–groundwater exchange flows and evapotranspiration. A global approach is also essential to assess the impact of groundwater depletion on sea level rise, since the groundwater storage loss rate on all continents of the Earth must be aggregated. Thus, we argue that groundwater is simultaneously a local, regional, and increasingly global resource and system and that examining groundwater problems, solutions, and interactions at all scales is crucial. As a consequence, we urgently require predictive understanding about how groundwater, as used by humans and connected with other components of the Earth system, operates at a variety of scales.
Based on the arguments above for considering global perspectives on groundwater, we see four specific purposes of representing groundwater in continental- to global-scale hydrological or land surface models and their climate modeling frameworks.
-
The first is to understand and quantify interactions between groundwater and past, present, and future climate. Groundwater systems can have far-reaching effects on climate, affecting modulation of surface energy and water partitioning with a long-term memory (Anyah et al., 2008; Kollet and Maxwell, 2008; Koirala et al., 2014; Krakauer et al., 2014; Maxwell et al., 2016; Taylor, et al., 2013a; Meixner et al., 2016; Wang et al., 2018; Keune et al., 2018). While there have been significant advances in understanding the role of lateral groundwater flow in evapotranspiration (Maxwell and Condon, 2016; Bresciani et al., 2016), the interactions between climate and groundwater over longer timescales (Cuthbert et al., 2019a) as well as between irrigation, groundwater, and climate (Condon and Maxwell, 2019; Condon et al., 2020) remain largely unresolved. Additionally, it is well-established that old groundwater with slow turnover times is common at depth (Befus et al., 2017; Jasechko et al., 2017). Groundwater connections to the atmosphere are well-documented in modeling studies (e.g., Forrester and Maxwell, 2020). Previous studies have demonstrated connections between the atmospheric boundary layer and water table depth (e.g., Maxwell et al., 2007; Rahman et al., 2015), under land cover disturbance (e.g., Forrester et al., 2018), under extremes (e.g., Kuene et al., 2016), and due to groundwater pumping (Gilbert et al., 2017). While a number of open-source platforms have been developed to study these connections, these platforms are regional to continental in extent. Recent work has shown global impacts of groundwater on atmospheric circulation (Wang et al., 2018), but groundwater is still quite simplified in this study.
-
The second is to understand and quantify two-way interactions between groundwater, the rest of the hydrologic cycle, and the broader Earth system. As the main storage component of the freshwater hydrologic cycle, groundwater systems support baseflow levels in streams and rivers and thereby ecosystems and agricultural productivity as well as other ecosystem services in both irrigated and rainfed systems (Scanlon et al., 2012; Qiu et al., 2019; Visser, 1959; Zipper et al., 2015, 2017). When pumped groundwater is transferred to oceans (Konikow, 2011; Döll et al., 2014a; Wada, 2016; Caceres et al., 2020; Luijendijk et al., 2020), resulting sea level rise can impact salinity levels in coastal aquifers and freshwater and solute inputs to the ocean (Moore, 2010; Sawyer et al., 2016). Difficulties are complicated by international trade of virtual groundwater, which causes aquifer stress in disparate regions (Dalin et al., 2017).
-
The third is to inform water decisions and policy for large, often transboundary groundwater systems in an increasingly globalized world (Wada and Heinrich, 2013; Herbert and Döll, 2019). For instance, groundwater recharge from large-scale models has been used to quantify groundwater resources in Africa, even though large-scale models do not yet include all recharge processes that are important in this region (Taylor et al., 2013b; Jasechko et al., 2014; Cuthbert et al., 2019b; Hartmann et al., 2017).
-
The fourth is to create visualizations and interactive opportunities that inform citizens and consumers, whose decisions have global-scale impacts, about the state of groundwater all around the world such as the World Resources Institute Aqueduct website (https://www.wri.org/aqueduct, last access: 16 November 2021), a decision-support tool to identify and evaluate global water risks.
The first two purposes are science-focused, while the latter two are sustainability-focused. In sum, continental- to global-scale hydrologic models incorporating groundwater offer a coherent scientific framework to examine the dynamic interactions between the Earth system above and below the land surface, and they are compelling tools for conveying the opportunities and limits of groundwater resources to people so that they can better manage the regions they live in and better understand the world around them. We consider both large-scale and regional-scale models to be useful in practice such that both should continue to be utilized rather than one replacing another. Ideally large-scale and regional-scale models should benefit from the other since each has strengths and weaknesses, and together the two types enrich our understanding and support the management of groundwater across scales (Sect. 2).
The challenge of incorporating groundwater processes into continental- or global-scale models is formidable and sometimes controversial. Some of the controversy stems from unanswered questions about how to best represent groundwater in the models, whereas some comes from skepticism about the feasibility of modeling groundwater at nontraditional scales. We advocate for the representation of groundwater stores and fluxes in continental to global models for the four reasons described above. We do not claim to have all the answers on how to best meet this challenge. We contend, however, that the hydrologic community needs to work deliberately and constructively towards effective representations of groundwater in global models.
Driven by the increasing recognition of the purpose of representing groundwater in continental- to global-scale models, many global hydrological models and land surface models have incorporated groundwater to varying levels of complexity depending on the model provenance and purpose. Different from regional-scale groundwater models that generally focus on subsurface dynamics, the focus of these models is on estimating either runoff and streamflow (hydrological models) or land–atmosphere water and energy exchange (land surface models). Simulation of groundwater storage and hydraulic heads mainly serves to quantify baseflow that affects streamflow during low-flow periods or capillary rise that increases evapotranspiration. Some land surface models use approaches based on the topographic index to simulate fast surface and slow subsurface runoff based on the fraction of saturated area in the grid cell (Clark et al., 2015; Fan et al., 2019); groundwater in these models does not explicitly have water storage or hydraulic heads (Famiglietti and Wood, 1994; Koster et al., 2000; Niu et al., 2005; Takata et al., 2003). In many hydrological models, groundwater is represented as a linear reservoir that is fed by groundwater recharge and drains to a river in the same grid cell (Müller Schmied et al., 2014; Gascoin et al., 2009; Ngo-Duc et al., 2007). Time series of groundwater storage but not hydraulic heads are computed. This prevents simulation of lateral groundwater flow between grid cells, capillary rise, and two-way exchange flows between surface water bodies and groundwater (Döll et al., 2016). However, representing groundwater as a water storage compartment that is connected to soil and surface water bodies by groundwater recharge and baseflow that is affected by groundwater abstractions and returns enables global-scale assessment of groundwater resources and stress (Herbert and Döll, 2019) as well as groundwater depletion (Döll et al., 2014a; Wada et al., 2014; de Graaf et al., 2014). In some land surface models, the location of the groundwater table with respect to the land surface is simulated within each grid cell to enable simulation of capillary rise (Niu et al., 2007), but, as in the case of simulating groundwater as a linear reservoir, lateral groundwater transport or two-way surface water–groundwater exchange cannot be simulated with this approach.
Models for simulating groundwater flows between all model grid cells in entire countries or globally have increasingly been developed either as stand-alone models or as part of hydrological models (Vergnes and Decharme, 2012; Fan et al., 2013; Lemieux et al., 2008; de Graaf et al., 2017; Kollet et al., 2017; Maxwell et al., 2015; Reinecke et al., 2019a, de Graaf et al., 2019). The simulation of groundwater in large-scale models is a nascent and rapidly developing field with significant computational and parameterization challenges, which have led to significant and important efforts to develop and evaluate individual models. It is important to note that “large-scale models” herein refer to models that are laterally extensive across multiple regions (hundreds to thousands of kilometers) and generally include the upper tens to hundreds of meters of subsurface and have resolutions sometimes as small as ∼1 km. In contrast, “regional-scale” models (tens to hundreds of kilometers) have long been developed for a specific region or aquifer and can include greater depths and resolutions as well as more complex hydrostratigraphy, and they are often developed from conceptual models with significant regional knowledge (Enemark et al., 2015). Regional-scale models include a diverse range of approaches from stand-alone groundwater models (i.e., representing surface water and vadose zone processes using boundary conditions such as recharge) to fully integrated groundwater–surface water models. In the future, large-scale models could be developed in a number of different directions, which we only briefly introduce here to maintain our primary focus on model evaluation. One important direction is clearer representation of three-dimensional geology and heterogeneity including karst (Condon et al., 2021), which should be considered part of conceptual model development prior to numerical model implementation.
Now that a number of models that represent groundwater at continental to global scales have been developed and will continue evolving, it is equally important that we advance how we evaluate these models. To date, large-scale model evaluation has largely focused on individual models, with inconsistent practices between models and little community-level discussion or cooperation, that lack the rigor of regional-scale model evaluation. Overall, we have only a partial and piecemeal understanding of the capabilities and limitations of different approaches to representing groundwater in large-scale models. Our objective is to provide clear recommendations for evaluating groundwater representation in continental and global models. We focus on model evaluation because this is the heart of model trust and reproducibility (Hutton et al., 2016), and improved model evaluation will guide how and where it is most important to focus future model development. We describe current model evaluation practices (Sect. 2) and consider diverse and uncertain sources of information, including observations, models, and experts, to holistically evaluate the simulation of groundwater-related fluxes, stores, and hydraulic heads (Sect. 3). We stress the need for an iterative and open-ended process of model improvement through continuous model evaluation against the different sources of information. We explicitly contrast the terminology used herein of “evaluation” and “comparison” against terminology such as “calibration”, “validation”, or “benchmarking”, which suggests a modeling process that is at some point complete. We extend previous commentaries advocating improved hydrologic process representation and evaluation in large-scale hydrologic models (Clark et al., 2015; Melsen et al., 2016) by adding expert elicitation and machine learning for more holistic evaluation. We also consider model objective and model evaluation across the diverse hydrologic landscapes, which can both uncover blind spots in model development. It is important to note that we do not consider water quality or contamination, even though water quality and contamination are important for water resources, management, and sustainability, since large-scale water quality models are in their infancy (van Vliet et al., 2019)
We bring together somewhat disparate scientific communities as a step towards greater community-level cooperation on these challenges, including global hydrology and land surface modelers, local to regional hydrogeologists, and hydrologists focused on model development and evaluation. We see three audiences beyond those currently directly involved in large-scale groundwater modeling that we seek to engage to accelerate model evaluation: (1) regional hydrogeologists who could be reticent about global models and yet have crucial knowledge and data that would improve evaluation; (2) data scientists with expertise in machine learning and artificial intelligence, among other areas, whose methods could be useful in a myriad of ways; and (3) the multiple Earth science communities that are currently working towards integrating groundwater into a diverse range of models so that improved evaluation approaches are built directly into model development.
Here we provide a brief overview of current large-scale groundwater models, the synergies and differences between regional-scale and large-scale model evaluation and development, and the imitations of current evaluation practices for large-scale models.
2.1 Brief overview of current large-scale groundwater models
Various large-scale models exist along a spectrum of model complexity, which can make it difficult to determine the most appropriate model for a specific application. We developed a simple but systematic classification of current large-scale groundwater models (Table 1) to summarize the main characteristics of existing models for the interdisciplinary audience of GMD. This classification builds on other reviews (Bierkens 2015; Condon et al., 2021) and is not exhaustive, nor is it the only way to classify large-scale groundwater models. It is meant to be a first classification attempt that should evolve with time. We suggest that groundwater in current large-scale models can be classified functionally by two aspects that are crucial to how groundwater impacts water, energy, and nutrient budgets. The first is whether lateral subsurface flow to a river is simulated within each cell independently of other cells, as 2D lateral groundwater flow between all cells, or as 3D groundwater flow. Second, we distinguish two types of coupling between groundwater and related compartments (variably saturated soil zone, surface water, atmospheric processes): “one-way” coupling (for example, recharge is imposed from the surface with no feedback from capillary rise or vegetation uptake, or groundwater flow to the surface does not depend on surface head) and “two-way” coupling that involves feedback loops. We also note atmospheric coupling, which involves coupling a groundwater–surface model with an atmospheric model to propagate the influence of groundwater from the surface to the atmosphere and the resulting feedback onto the surface and groundwater. This classification scheme (which could also be called a model typology) is based on a number of model characteristics such as the fluxes, stores, and other features (Table 1).
Table 1Model classification for large-scale models representing groundwater (1).
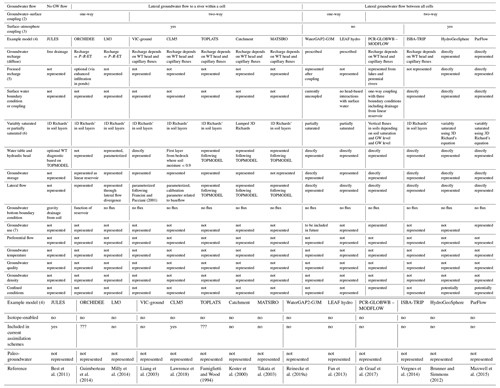
Notes: (1) only the most recent versions of models with published results at continental to global scales are included. Analytical solutions (including the water table ratio or groundwater response times) are not described here.
(2) One-way coupling means soil moisture ⇒ recharge ⇒ groundwater system ⇒ streamflow, but there is no reverse influence; in this case, the groundwater model is dependent on surface simulations to provide recharge. Two-way coupling means there is a full coupling of surface and subsurface heads.
(3) Surface–atmosphere coupling means that the groundwater component can be coupled with atmospheric or weather models.
(4) Other models exist with similar features.
(5) Focused recharge refers to any recharge that occurs beneath water bodies such as streams or lakes, whereas preferential flow means recharge that bypasses the soil matrix during diffuse recharge through fractures or other macropores.
(6) Variably saturated means that the saturation and related constitutive relations can vary continuously, while partially saturated means that saturation can only discretely vary between fully saturated and unsaturated.
(7) Groundwater use means groundwater pumping rather than via evapotranspiration.
2.2 Synergies between regional scale and large scales
Regional-scale and large-scale groundwater models are both governed by the same physical equations and share many of the same challenges. Like large-scale models, some regional-scale models have challenges with representing important regional hydrologic processes such as mountain block recharge (Markovich et al., 2019), and data availability challenges (such as the lack of reliable subsurface parameterization and hydrologic monitoring data) are common. We propose that there are largely untapped potential synergies between regional-scale and large-scale models based on these commonalities and the inherent strengths and limitations of each scale (Sect. 1).
Much can be learned from regional-scale models to inform the development and evaluation of large-scale groundwater models. Regional-scale models are evaluated using a variety of data types, some of which are available and already used at the global scale and some of which are not. In general, the most common data types used for regional-scale groundwater model evaluation match global-scale groundwater models: hydraulic head and either total streamflow or baseflow estimated using hydrograph separation approaches (e.g., RRCA, 2003; Woolfenden and Nishikawa, 2014; Tolley et al., 2019). However, numerous data sources unavailable or not currently used at the global scale have also been applied in regional-scale models, such as elevation of surface water features (Hay et al., 2018), existing maps of the potentiometric surface (Meriano and Eyles, 2003), dendrochronology (Schilling et al., 2014), and stable and radiogenic isotopes for determining water sources and residence times (Sanford, 2011). These and other “nonclassical” observations (Schilling et al., 2019) could be the inspiration for model evaluation of large-scale models in the future but are beyond our scope to discuss. Further, given the smaller domain size of regional-scale models, expert knowledge and local ancillary data sources can be more directly integrated, and automated parameter estimation approaches such as PEST are tractable (Leaf et al., 2015; Hunt et al., 2013). We directly build upon this practice of integration of expert knowledge below in Sect. 3.3.
We propose that there may also be potential benefits of large-scale models for the development of regional-scale models. For instance, the boundary conditions of some regional-scale models could be improved with large-scale model results. The boundary conditions of regional-scale models are often assumed, calibrated, or derived from other models or data. In a regional-scale model, increasing the model domain (moving the boundary conditions away from region of interests) or incorporating more hydrologic processes (for example, moving the boundary condition from recharge to the land surface incorporating evapotranspiration and infiltration) can both reduce the impact of boundary conditions on the region and problem of interest. Another potential benefit of large-scale models for regional-scale models is fuller inclusion of large-scale hydrologic and human processes that could further enhance the ability of regional-scale models to address both the science-focused and sustainability-focused purposes described in Sect. 1. For example, the stronger representation of large-scale atmospheric processes means that the downwind impact of groundwater irrigation on evapotranspiration, precipitation, and streamflow can be assessed (DeAngelis et al., 2010; Kustu et al., 2011). Or, the effects of climate change and increased water use that affect the inflow of rivers into the regional modeling domain can be taken from global-scale analyses (Wada and Bierkens, 2014). Also, regional groundwater depletion might be largely driven by virtual water trade, which can be better represented in global analysis and models than regional-scale models (Dalin et al., 2017). Therefore, the processes and results of large-scale models could be used to make regional-scale models even more robust and better address key science and sustainability questions.
Given the strengths of regional models, a potential alternative to development of large-scale groundwater models would be combining or aggregating multiple regional models in a patchwork approach (as in Zell and Sanford, 2020) to provide global coverage. This would have the advantage of better respecting regional differences but potentially create additional challenges because the regional models would have different conceptual models, governing equations, and boundary conditions, for example, in different regions. Some challenges of this patchwork approach include (1) the required collaboration of a large number of experts from all over the world over a long period of time, (2) the fact that regional groundwater flow models alone are not sufficient (they need to be integrated into a hydrological model so that groundwater–soil water and the surface water–groundwater interactions can be simulated), (3) the fact that the extent of regional aquifers does not necessarily coincide with the extent of river basins, and (4) the bias of regional groundwater models towards important aquifers, which, as described above, underlie only a portion of the world's land mass or population and may bias estimates of fluxes such as surface water–groundwater exchange or evapotranspiration. Given these challenges, we argue that a patchwork approach of integrating multiple regional models is a compelling idea but likely insufficient to achieve the purposes of large-scale groundwater modeling described in Sect. 1. Although this nascent idea of aggregating regional models is beyond the scope of this paper, we consider this an important future research avenue and encourage further exploration and improvement of regional-scale model integration from the groundwater modeling community.
2.3 Differences between regional scale and large scales
Although there are important similarities and potential synergies across scales, it is important to consider how or if large-scale models are fundamentally different to regional-scale models, especially in ways that could impact evaluation. The primary differences between large-scale and regional-scale models are that large-scale models (by definition) cover larger areas and, as a result, typically include more data-poor areas and are generally built at coarser resolution. These differences impact evaluations in at least five relevant ways.
-
Commensurability errors (also called “representativeness” errors) occur either when modeled grid values are interpolated and compared to an observation “point” or when aggregation of observed point values are compared to a modeled grid value (Beven, 2005; Tustison et al., 2001; Beven, 2016; Pappenberger et al., 2009; Rajabi et al., 2018). For groundwater models in particular, commensurability error will depend on the number and locations of observation points, the variability structure of the variables being compared such as hydraulic head, and the interpolation or aggregation scheme applied (Tustison et al., 2001; Pappenberger et al., 2009; Reinecke et al., 2020). Commensurability is a problem for most scales of modeling but is likely more significant the coarser the model. Regional-scale groundwater models typically have fewer (though not insignificant) commensurability issues due to smaller grid cell sizes compared to large-scale models.
-
Specificity to region, objective, and model evaluation criteria occurs because regional-scale models are developed specifically for a certain region and modeling or management objective, whereas large-scale models are often more general and include different regions. As a result, large-scale models often have greater heterogeneity of processes and parameters, may not adopt the same calibration targets and variables, and are not subject to the policy or litigation that sometimes drives model evaluation of regional-scale models.
-
Computational requirements can be immense for large-scale models, which leads to challenges with uncertainty and sensitivity analysis. While some regional-scale models also have large computational demands, large-scale models cover larger domains and are therefore more vulnerable to this potential constraint.
-
Data availability for large-scale models can be limited because they typically include data-poor areas, which leads to challenges when only using observations for model evaluation. While data availability also affects regional-scale models, they are often developed for regions with known hydrological challenges based on existing data, and/or modeling efforts are preceded by significant regional data collection from detailed sources (such as local geological reports) that are not often included in continental to global datasets used for large-scale model parameterization.
-
Subsurface detail in regional-scale models routinely includes heterogeneous and anisotropic parameterizations, which could be improved in future large-scale models. For example, intense vertical anisotropy routinely induces vertical flow dynamics from vertical head gradients that are tens to thousands of times greater than horizontal gradients, which profoundly alter the meaning of the deep and shallow groundwater levels, with only the latter remotely resembling the actual water table. In contrast, most large-scale models currently use a single vertically homogeneous value for each grid cell or at best have two layers (de Graaf et al., 2017).
2.4 Limitations of current evaluation practices for large-scale models
Evaluation of large-scale models has often focused on streamflow or evapotranspiration observations, but joint evaluation together with groundwater-specific variables is appropriate and necessary (e.g., Maxwell et al., 2015; Maxwell and Condon, 2016). Groundwater-specific variables useful for evaluating the groundwater component of large-scale models include the following: (a) hydraulic head or water table depth; (b) groundwater storage and groundwater storage changes, which refer to long-term, negative, or positive trends in groundwater storage, with long-term negative trends referred to as groundwater depletion; (c) groundwater recharge; (d) flows between groundwater and surface water bodies; and (e) human groundwater abstractions and return flows to groundwater. It is important to note that groundwater and surface water hydrology communities often have slightly different definitions of terms like recharge and baseflow (Barthel, 2014); we therefore suggest trying to precisely define the meanings of such words using the actual hydrologic fluxes, which we do below. Table 2 shows the availability of observational data for these variables but does not evaluate the quality and robustness of observations. Overall there are significant inherent challenges of commensurability and measurability of groundwater observations in the evaluation of large-scale models. We describe the current model evaluation practices for each of these variables here.
- a.
Simulated hydraulic heads or water table depths in large-scale models are frequently compared to well observations, which are often considered the crucial data for groundwater model evaluation. Hydraulic head observations from a large number groundwater wells (>1 million) have been used to evaluate the spatial distribution of steady-state heads (Fan et al., 2013, de Graaf et al., 2015; Maxwell et al., 2015; Reinecke et al., 2019a, 2020). Transient hydraulic heads with seasonal amplitudes (de Graaf et al., 2017), declining heads in aquifers with groundwater depletion (de Graaf et al., 2019), and daily transient heads (Tran et al., 2020) have also been compared to well observations. All evaluation with well observations is severely hampered by the incommensurability of point values of observed heads with simulated heads that represent averages over cells of a size of tens to hundreds of square kilometers; within such a large cell, land surface elevation, which strongly governs hydraulic head, may vary a few hundred meters, and average observed head strongly depends on the number and location of wells within the cell (Reinecke et al., 2020). Additional concerns with head observations are the (1) strong sampling bias of wells towards accessible locations, low elevations, shallow water tables, and more transmissive aquifers in wealthy, generally temperate countries (Fan et al., 2019); (2) the impacts of pumping, which may or may not be well-known; (3) observational errors and uncertainty (Post and von Asmuth, 2013; Fan et al., 2019); and (4) the fact that heads can reflect the poro-elastic effects of mass loading and unloading rather than necessarily aquifer recharge and drainage (Burgess et al., 2017). To date, simulated hydraulic heads have more often been compared to observed heads (rather than water table depth), which results in lower relative errors (Reinecke et al., 2020) because the range of heads (tens to thousands of meters) is much larger than the range of water table depths (<1 m to hundreds of meters).
- b.
Simulated groundwater storage trends or anomalies in large-scale hydrological models have been evaluated using observations of groundwater well levels combined with estimates of storage parameters, such as specific yield, local-scale groundwater modeling, and translation of regional total water storage trends and anomalies from satellite gravimetry (GRACE: Gravity Recovery And Climate Experiment) to groundwater storage changes by estimating changes in other hydrological storage (Döll et al., 2012, 2014a). Groundwater storage change volumes and rates have been calculated for numerous aquifers, primarily in the United States, using calibrated groundwater models, analytical approaches, or volumetric budget analyses (Konikow, 2011). Regional-scale models have also been used to simulate groundwater storage trends by untangling the impacts of water management during drought (Thatch et al., 2020). Satellite gravimetry (GRACE) is important but has limitations (Alley and Konikow, 2015). First, monthly time series of very coarse-resolution groundwater storage are indirectly estimated from observations of total water storage anomalies by satellite gravimetry (GRACE) but only after model- or observation-based subtraction of water storage changes in glaciers, snow, soil, and surface water bodies (Lo et al., 2016; Rodell et al., 2009; Wada, 2016). As soil moisture, river, or snow dynamics often dominate total water storage dynamics, the derived groundwater storage dynamics can be so uncertain that severe groundwater drought cannot be detected in this way (Van Loon et al., 2017). Second, GRACE cannot detect the impact of groundwater abstractions on groundwater storage unless groundwater depletion occurs (Döll et al., 2014a, b). Third, the very coarse resolution can lead to incommensurability but in the opposite direction of well observations. It is important to note that the focus is on storage trends or anomalies since total groundwater storage to a specific depth (Gleeson et al., 2016) or in an aquifer (Konikow, 2011) can be estimated, but the total groundwater storage in a specific region or cell cannot be simulated or observed unless the depth of interest is specified (Condon et al., 2020).
- c.
Simulated large-scale groundwater recharge (vertical flux across the water table) has been evaluated using compilations of point estimates of groundwater recharge, results of regional-scale models, baseflow indices, and expert opinion (Döll and Fiedler, 2008; Hartmann et al., 2015) or compared between models (e.g., Wada et al., 2010). In general, groundwater recharge is not directly measurable except by meter-scale lysimeters (Scanlon et al., 2002), and many groundwater recharge methods such as water table fluctuations and chloride mass balance also suffer from similar commensurability issues as water table depth data. Although sometimes an input or boundary condition to regional-scale models, recharge in many large-scale groundwater models is simulated and can thus be evaluated.
- d.
The flows between groundwater and surface water bodies (rivers, lakes, wetlands) are simulated by many models but are generally not evaluated directly against observations of such flows since they are very rare and challenging. Baseflow (the slowly varying portion of streamflow originating from groundwater or other delayed sources) or streamflow “low flows” (when groundwater or other delayed sources predominate) generally cannot be used to directly quantify the flows between groundwater and surface water bodies at large scales. Groundwater discharge to rivers can be estimated from streamflow observations only in a very dense gauge network and/or if streamflow during low-flow periods is mainly caused by groundwater discharge and not by water storage in upstream lakes, reservoirs, or wetlands. These conditions are rarely met in the case of streamflow gauges with large upstream areas that can be used for comparison to large-scale model output. De Graaf et al. (2019) compared the simulated timing of changes in groundwater discharge to observations and regional-scale models but only compared the fluxes directly between the global- and regional-scale models. Due to the challenges of directly observing the flows between groundwater and surface water bodies at large scales, this is not included in the available data in Table 2; instead, in Sect. 3 we highlight the potential for using baseflow or the spatial distribution of perennial, intermittent, and ephemeral streams in the future.
- e.
Groundwater abstractions have been evaluated by comparison to national-, state-, and county-scale statistics in the US (Wada et al., 2010; Döll et al., 2012, 2014a; de Graaf et al., 2014). Irrigation is the dominant groundwater use sector in many regions; however, irrigation pumpage is generally estimated from crop water demand and rarely metered. GRACE and other remote sensing data have been used to estimate irrigation water abstractions (R. G. Anderson et al., 2015). The lack of records or observations of abstraction introduces significant uncertainties into large-scale models, is simulated, and can thus be evaluated. Human groundwater abstractions and return flows as well as groundwater recharge and the flows between groundwater and surface water bodies are necessary to simulate storage trends (described above). But each of these are considered separate observations since they each have different data sources and assumptions. Groundwater abstraction data at the well scale are severely hampered by incommensurability like hydraulic head and recharge described above.
Based on Sect. 2, we argue that current model evaluation practices are insufficient to robustly evaluate large-scale models. We therefore propose evaluating large-scale models using at least three strategies (pie-shapes in Fig. 1): observation-, model-, and expert-driven evaluation, which are potentially mutually beneficial because each strategy has its strengths and weaknesses. We are not proposing a brand new evaluation method here but rather separating strategies to consider the problem of large-scale model evaluation from different but highly interconnected perspectives. All three strategies work together for the common goal of improved large-scale model evaluation, which is the center of Fig. 1.
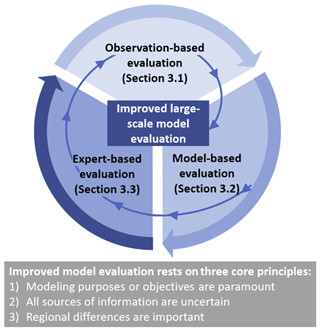
Figure 1Improved large-scale model evaluation rests on observation-, model-, and expert-based model evaluation. We argue that each strategy is essential so that all three should simultaneously be pursued by the scientific community. The three strategies of model evaluation all rest on three core principles related to (1) model objectives, (2) uncertainty, and (3) regional differences.
When evaluating large-scale models, it is necessary to first consider reasonable expectations or how to know a model is performing “well enough”. Reasonable expectations should be based on the modeling purpose, hydrologic process understanding, and the plausibly achievable degree of model realism. First, model evaluation should be clearly linked to the four science- or sustainability-focused purposes of representing groundwater in large-scale models (Sect. 1). Second, it should be linked to our understanding of relevant hydrologic processes. The objective of large-scale models cannot be to reproduce the spatiotemporal details that regional-scale models can reproduce. Determining the reasonable expectations is necessarily subjective but can be approached using observation-, model-, and expert-driven evaluation. As a simple first step in setting realistic expectations, we propose that three physical variables can be used to form more convincing arguments that a large-scale model is performing well enough: change in groundwater storage, water table depth, and regional fluxes between groundwater and surface water. Below we explore in more detail additional variables and approaches that can support this simple approach.
Across all three model evaluation strategies of observation-, model-, and expert-driven evaluation, we advocate three principles underpinning model evaluation (base of Fig. 1), none of which we are the first to suggest but we highlight here as a reminder: (1) model objectives, such as the groundwater science or groundwater sustainability objective summarized in Sect. 1, are important to model evaluation because they provide the context through which relevance of the evaluation outcome is set; (2) all sources of information (observations, models, and experts) are uncertain, and this uncertainty needs to be quantified for robust evaluation; and (3) regional differences are likely important for large-scale model evaluation – understanding these differences is crucial for the transferability of evaluation outcomes to other places or times.
We stress the fact that we see the consideration and quantification of uncertainty as essential needs across all three types of model evaluation we describe below, so we discuss it here rather than with model-driven model evaluation (Sect. 3.2) for which uncertainty analysis more narrowly defined would often be discussed. We further note that large-scale models have only been assessed to a very limited degree with respect to understanding, quantifying, and attributing relevant uncertainties. Expanding computing power, developing computationally frugal methods for sensitivity and uncertainty analysis, and potentially employing surrogate models can enable more robust sensitivity and uncertainty analysis such as that used in regional-scale models (Habets et al., 2013; Hill, 2006; Hill and Tiedeman, 2007; Reinecke et al., 2019b). For now, we suggest applying computationally frugal methods such as the elementary effect test or local sensitivity analysis (Hill, 2006; Morris, 1991; Saltelli et al., 2000). Such sensitivity and uncertainty analyses should be applied not only to model parameters and forcings but also to model structural properties (e.g., boundary conditions, grid resolution, process simplification) (Wagener and Pianosi, 2019). This implies that the (independent) quantification of uncertainty in all model elements (observations, parameters, states, etc.) needs to be improved and better captured in available metadata.
We advocate for considering regional differences more explicitly in model evaluation since no single model will likely perform consistently across the diverse hydrologic landscapes of the world (Van Werkhoven et al., 2008). Considering regional differences in large-scale model evaluation is motivated by recent model evaluation results and is already starting to be practiced. Two recent sensitivity analyses of large-scale models reveal how sensitivities to input parameters vary in different regions for both hydraulic heads and flows between groundwater and surface water (de Graaf et al., 2019; Reinecke et al., 2020). In mountain regions, large-scale models tend to underestimate steady-state hydraulic head, possibly due to overestimated hydraulic conductivity in these regions, which highlights the fact that model performance varies in different hydrologic landscapes. (de Graaf et al., 2015; Reinecke et al., 2019b). Additionally, there are significant regional differences in performance with low flows for a number of large-scale models (Zaherpour et al., 2018), likely because of diverse implementations of groundwater and baseflow schemes. Large-scale model evaluation practice is starting to shift towards highlighting regional differences as exemplified by two different studies that explicitly mapped hydrologic landscapes to enable clearer understanding of regional differences. Reinecke et al. (2019b) identified global hydrological response units, which highlighted the spatially distributed parameter sensitivities in a computationally expensive model, whereas Hartmann et al. (2017) developed and evaluated models for karst aquifers in different hydrologic landscapes based on different a priori system conceptualizations. Considering regional differences in model evaluation suggests that global models could in the future consider a patchwork approach of different conceptual models, governing equations, and boundary conditions, for example, in different regions. Although beyond the scope of this paper, we consider this an important future research avenue.
3.1 Observation-based model evaluation
Observation-based model evaluation is the focus of most current efforts and is important because we want models to be consistent with real-world observations. Section 2 and Table 2 highlight both the strengths and limitations of current practices using observations. Despite existing challenges, we foresee significant opportunities for observation-based model evaluation and do not see data scarcity as a reason to exclude groundwater in large-scale models or to avoid evaluating these models. It is important to note that most so-called “observations” are modeled or derived quantities that are often at the wrong scale for evaluating large-scale models (Table 2; Beven, 2019). Given the inherent challenges of direct measurement of groundwater fluxes and stores, especially at large scales, herein we consider “observations” to loosely be any measurements of physical stores or fluxes that are combined with or filtered through models for an output. For example, GRACE gravity measurements are combined with model-based estimates of water storage changes in glaciers, snow, soil, and surface water for “groundwater storage change observations”, or streamflow measurements are filtered through baseflow separation algorithms for “baseflow observations”. The strengths and limitations as well as the data availability and spatial and temporal attributes of different observations are summarized in Table 2, which we hope will spur more systematic and comprehensive use of observations.
Here we highlight nine important future priorities for improving evaluation using available observations. The first five priorities focus on current observations (Table 2), whereas the latter four focus on new methods or approaches.
-
Focus on transient observations of the water table depth rather than hydraulic head observations that are long-term averages or individual times (often following well drilling). Water table depth is likely a more robust evaluation metric than hydraulic head because water table depth reveals great discrepancies and is a complex function of the relationship between hydraulic head and topography that is crucial to predicting system fluxes (including evapotranspiration and baseflow). Comparing transient observations and simulations instead of long-term averages or individual times incorporates more system dynamics of storage and boundary conditions as temporal patterns are more important than absolute values (Heudorfer et al., 2019). For regions with significant groundwater depletion, comparing to declining water tables is a useful strategy (de Graaf et al., 2019), whereas in aquifers without groundwater depletion, seasonally varying water table depths are likely more useful observations (de Graaf et al., 2017).
-
Use baseflow, the slowly varying portion of streamflow originating from groundwater, or other delayed sources. Döll and Fiedler (2008) included the baseflow index in evaluating recharge, and baseflow has been used to calibrate the groundwater component of a land surface model (Lo et al., 2008, 2010). But the baseflow index (BFI), linear and nonlinear baseflow recession behavior, or baseflow fraction (Gnann et al., 2019) have not been used to evaluate any large-scale model that simulates groundwater flows between all model grid cells. There are limitations of using BFI and baseflow recession characteristics to evaluate large-scale models (Table 2). Using baseflow only makes sense when the baseflow separation algorithm is better than the large-scale model itself, which may not be the case for some large-scale models and only in time periods that can be assumed to be dominated by groundwater discharge. Similarly, using recession characteristics is dependent on an appropriate choice of recession extraction methods. But this approach remains available, and data derived from streamflow or spring flow observations have been underused to date.
-
Use the spatial distribution of perennial, intermittent, and ephemeral streams as an observation, which to our best knowledge has not been done by any large-scale model evaluation. The transition between perennial and ephemeral streams is an important system characteristic in groundwater–surface water interactions (Winter et al., 1998), so we suggest that this might be a revealing evaluation criterion, although there are similar limitations to using baseflow. The results of both quantifying baseflow and mapping perennial streams depend on the methods applied; they are not useful for quantifying groundwater–surface water interactions when there is upstream surface water storage, and they do not directly provide information about fluxes between groundwater and surface water.
-
Use data on land subsidence to infer head declines or aquifer properties for regions where groundwater depletion is the main cause of compaction (Bierkens and Wada, 2019). Lately, remote sensing methods such as GPS as well as airborne and spaceborne radar and lidar are frequently used to infer land subsidence rates (Erban et al., 2014). Also, a number of studies combine geomechanical modeling (Ortega-Guerrero et al., 1999; Minderhoud et al., 2017) and geodetic data to explain the main drivers of land subsidence. A few papers (e.g., Zhang and Burbey, 2016) use a geomechanical model together with withdrawal data and geodetic observations to estimate hydraulic and geomechanical subsoil properties.
-
Consider using socioeconomic data for improving model input. For example, reported crop yields in areas with predominant groundwater irrigation could be used to evaluate groundwater abstraction rates, or well depth data (Perrone and Jasechko, 2019) can be used to assess minimum aquifer depths and, in coastal regions and deltas, the presence of deeper fresh groundwater under semi-confining layers.
-
Derive additional new datasets using meta-analysis and/or geospatial analysis such as gaining or losing stream reaches (e.g., from interpolated head measurements close to the streams), springs and groundwater-dependent surface water bodies, or tracers. Each of these new data sources could in principle be developed from available data using methods already applied at regional scales but that do not currently have an “off-the-shelf” global dataset. For example, some large-scale models have been explicitly compared with residence time and tracer data (Maxwell et al., 2016), which have also been recently compiled globally (Gleeson et al., 2016; Jasechko et al., 2017). This could be an important evaluation tool for large-scale models that are capable of simulating flow paths or can be modified to do so, though a challenge of this approach is the conservativity of tracers. Future meta-analyses and data compilations should report on the quality of the data and include possible uncertainty ranges as well as the mean estimates.
-
Use machine learning to identify process representations (e.g., Beven, 2020) or spatiotemporal patterns, for example of perennial streams, water table depths, or baseflow fluxes, which might not be obvious in multidimensional datasets but could be useful in evaluation. For example, Yang et al. (2019) predicted the state of losing and gaining streams in New Zealand using random forest algorithms. A staggering variety of machine-learning tools are available and their use is nascent yet rapidly expanding in geoscience and hydrology (Reichstein et al., 2019; Shen, 2018; Shen et al., 2018; Wagener et al., 2021). While large-scale groundwater models are often considered “data-poor”, it may seem strange to propose using data-intensive machine-learning methods to improve model evaluation. But some of the data sources are large (e.g., over 2 million water level measurements in Fan et al., 2013, although biased in distribution), whereas other observations such as evapotranspiration (Jung et al., 2011) and baseflow (Beck et al., 2013) are already interpolated and extrapolated using machine learning. Moving forwards, it is important to consider commensurability while applying machine learning in this context.
-
Consider comparing models against hydrologic signatures – indices that provide insight into the functional behavior of the system under study (Wagener et al., 2007; McMilan, 2020). The direct comparison of simulated and observed variables through statistical error metrics has at least two downsides. One is the abovementioned unresolved problem of commensurability, and the other is the issue that such error metrics are rather uninformative in a diagnostic sense – simply knowing the size of an error does not tell the modeler how the model needs to be improved, only that it does (Yilmaz et al., 2009). One way to overcome these issues is to derive hydrologically meaningful signatures from the original data, such as the signatures derived from transient groundwater levels by Heudorfer et al. (2019). For example, recharge ratio (defined as the ratio of groundwater recharge to precipitation) might be hydrologically more informative than recharge alone (Jasechko et al., 2014) or the water table ratio and groundwater response time (Cuthbert et al., 2019a; Opie et al., 2020), which are spatially distributed signatures of groundwater system dynamics. Such signatures might be used to assess model consistency (Wagener and Gupta, 2005; Hrachowitz et al., 2014) by looking at the similarity of patterns or spatial trends rather than the size of the aggregated error, thus reducing the commensurability problem.
-
Understand and quantify commensurability error issues better so that a fairer comparison can be made across scales using existing data. As described above, commensurability errors will depend on the number and locations of observation points, the variability structure of the variables being compared such as hydraulic head, and the interpolation or aggregation scheme applied. While to some extent we may appreciate how each of these factors affects commensurability error in theory, in practice their combined effects are poorly understood and methods to quantify and reduce commensurability errors for groundwater model purposes remain largely undeveloped. As such, quantification of commensurability error in (large-scale) groundwater studies is regularly overlooked as a source of uncertainty because it cannot be satisfactorily evaluated (Tregoning et al., 2012). Currently, evaluation of simulated groundwater heads is plagued by, as yet, poorly quantified uncertainties stemming from commensurability errors, and we therefore recommend that future studies focus on developing solutions to this problem. An additional subtle but important and unresolved commensurability issue can stem from conceptual models. Different hydrogeologists examining different scales and/or data or interpreting geology differently can produce quite different conceptual models of the same region (Troldborg et al., 2007).
We recommend evaluating models with a broader range of currently available data sources (with explicit consideration of data uncertainty and regional differences) while also simultaneously working to derive new datasets. Using data (such as baseflow, land subsidence, or the spatial distribution of perennial, intermittent, and ephemeral streams) that are more consistent with the scale-modeled grid resolution will hopefully reduce the commensurability challenges. However, data distribution and commensurability issues will likely still be present, which underscores the importance of the two following strategies.
3.2 Model-based model evaluation
Model-based model evaluation, which includes model intercomparison projects (MIPs) and model sensitivity and uncertainty analysis, can be done with or without explicitly using observations. We describe both inter-model and inter-scale comparisons, which could be leveraged to maximize the strengths of each of these approaches.
The original MIP concept offers a framework to consistently evaluate and compare models, as well as associated model input, structural, and parameter uncertainty under different objectives (e.g., climate change, model performance, human impacts and developments). Early model intercomparisons of groundwater models focused on nuclear waste disposal (SKI, 1984). Since the Project for the Intercomparison of Land-Surface Parameterization Schemes (PILPS; Henderson-Sellers et al., 1993), the first large-scale MIP, the land surface modeling community has used MIPs to deepen understanding of land physical processes and to improve their numerical implementations at various scales from regional (e.g., Rhône aggregation project; Boone et al., 2004) to global (e.g., Global Soil Wetness Project; Dirmeyer, 2011). Two examples of recent model intercomparison efforts illustrate the general MIP objectives and practice. First, ISIMIP (Schewe et al., 2014; Warszawski et al., 2014) assessed water scarcity at different levels of global warming. Second, IH-MIP2 (Kollet et al., 2017) used both synthetic domains and an actual watershed to assess fully integrated hydrologic models because these cannot be validated easily by comparison with analytical solutions, and uncertainty remains in the attribution of hydrologic responses to model structural errors. Model comparisons have revealed differences, but it is often unclear whether these stem from differences in the model structures, differences in how the parameters were estimated, or from other modeling choices (Duan et al., 2006). Attempts at modular modeling frameworks to enable comparisons (Wagener et al., 2001; Leavesley et al., 2002; Clark et al., 2008; Fenicia et al., 2011; Clark et al., 2015) or at least shared explicit modeling protocols and boundary conditions (Refsgaard et al., 2007; Ceola et al., 2015; Warszawski et al., 2014) have been proposed to reduce these problems.
Inter-scale model comparison – for example, comparing a global model to a regional-scale model – is a potentially useful approach which is emerging for surface hydrology models (Hattermann et al., 2017; Huang et al., 2017) and could be applied to large-scale models with groundwater representation. For example, declining heads and decreasing groundwater discharge have been compared between a calibrated regional-scale model (RRCA, 2003) and a global model (de Graaf et al., 2019). A challenge to inter-scale comparisons is that regional-scale models often have more spatially complex subsurface parameterizations because they have access to local data, which can complicate model intercomparison. Another approach which may be useful is running large-scale models over smaller (regional) domains at a higher spatial resolution (same as a regional-scale model) so that model structure influences the comparison less. In the future, various variables that are hard to directly observe at large scales but routinely simulated in regional-scale models such as baseflow or recharge could be used to evaluate large-scale models, although these flux estimates can contain large uncertainty. In this way, the output fluxes and intermediate spatial scale of regional models provide a bridge across the “river of incommensurability” between highly location-specific data such as well observations and the coarse resolution of large-scale models. In such an evaluation, the uncertainty of flux estimates and scale of aggregation are both important to consider. It is important to consider the fact that regional-scale models are not necessarily or inherently more accurate than large-scale models since problems may arise from conceptualization, groundwater–surface water interactions, scaling issues, and parameterization, among others.
In order for a regional-scale model to provide a useful evaluation of a large-scale model, there are several important documentation and quality characteristics it should meet. At a bare minimum, the regional-scale model must be accessible and therefore meet basic replicability requirements including open and transparent input and output data as well as model code to allow large-scale modelers to run the model and interpret its output. Documentation through peer review, either through a scientific journal or agency such as the US Geological Survey, would be ideal. It is particularly important that the documentation discusses limitations, assumptions, and uncertainties in the regional-scale model so that a large-scale modeler can be aware of potential weaknesses and guide their comparison accordingly. Second, the boundary conditions and/or parameters being evaluated need to be reasonably comparable between the regional- and large-scale models. For example, if the regional-scale model includes human impacts through groundwater pumping while the large-scale model does not, a comparison of baseflow between the two models may not be appropriate. Similarly, there needs to be consistency in the time period simulated between the two models. Finally, as with data-driven model evaluation, the purpose of the large-scale model needs to be consistent with the model-based evaluation; matching the hydraulic head of a regional-scale model, for instance, does not indicate that estimates of stream–aquifer exchange are valid. Ideally, we recommend developing a community database of regional-scale models that meet these criteria. It is important to note that Rossman and Zlotnik (2013) review 88 regional-scale models, while a good example of such a repository is the California Groundwater Model Archive (https://ca.water.usgs.gov/sustainable-groundwater-management/california-groundwater-modeling.html, last access: 15 November 2021).
In addition to evaluating whether models are similar in terms of their outputs, e.g., whether they simulate similar groundwater head dynamics, it is also relevant to understand whether the influence of controlling parameters is similar across models. This type of analysis provides insights into process controls and dominant uncertainties. Sensitivity analysis provides the mathematical tools to perform this type of model evaluation (Saltelli et al., 2004; Pianosi et al., 2016; Borgonovo et al., 2017). Recent applications of sensitivity analysis to understand modeled controls on groundwater-related processes include the study by Reinecke et al. (2019b) trying to understand parametric controls on groundwater heads and flows within a global groundwater model. Maples et al. (2020) demonstrated that parametric controls on groundwater recharge can be assessed for complex models, though over a smaller domain. As highlighted by both of these studies, more work is needed to understand how to best use sensitivity analysis methods to assess computationally expensive, spatially distributed, and complex groundwater models across large domains (Hill et al., 2016). In the future, it would be useful to go beyond parameter uncertainty analysis (e.g., Reinecke et al., 2019b) to begin to look at all of the modeling decisions holistically such as the forcing data (Weiland et al., 2015) and digital elevation models (Hawker et al., 2018). Addressing this problem requires advancements in statistics (more efficient sensitivity analysis methods) and computing (more effective model execution), as well as access to large-scale model codes (Hutton et al., 2016), but also better utilization of process understanding, for example, to create process-based groups of parameters which reduce the complexity of the sensitivity analysis study (e.g., Hartmann et al., 2015; Reinecke et al., 2019b).
3.3 Expert-based model evaluation
A path much less traveled is expert-based model evaluation, which would develop hypotheses of phenomena (and related behaviors, patterns, or signatures) we expect to emerge from large-scale groundwater systems based on expert knowledge, intuition, or experience. In essence, this model evaluation approach flips the traditional scientific method around by using hypotheses to test the simulation of emergent processes from large-scale models rather than using large-scale models to test our hypotheses about environmental phenomena. This might be an important path forward for regions where available data are very sparse or unreliable. The recent discussion by Fan et al. (2019) shows how hypotheses about large-scale behavior might be derived from expert knowledge gained through the study of smaller-scale systems such as critical zone observatories (Fan, 2015). While there has been much effort to improve our ability to make hydrologic predictions in ungauged locations through the regionalization of hydrologic variables or model parameters (Bloeschl et al., 2013), there has been much less effort to directly derive expectations of hydrologic behavior based on our perception of the systems under study.
Large-scale models could then be evaluated against such hypotheses, thus providing a general opportunity to advance how we connect hydrologic understanding with large-scale modeling – a strategy that could also potentially reduce epistemic uncertainty (Beven et al., 2018) and which may be especially useful for groundwater systems given the data limitations described above. Developing appropriate and effective hypotheses is crucial and should likely focus on large-scale controlling factors or relationships between controlling factors and output in different parts of the model domain; hypotheses that are too specific may only be able to be tested by certain model complexities or in certain regions. To illustrate the type of hypotheses we are suggesting, we list some examples of hypotheses drawn from current literature.
-
Water table depth and lateral flow strongly affect transpiration partitioning (Famiglietti and Wood, 1994; Salvucci and Entekhabi, 1995; Maxwell and Condon, 2016).
-
The percentage of inter-basinal regional groundwater flow increases with aridity or decreases with the frequency of perennial streams (Gleeson and Manning, 2008; Goderniaux et al., 2013; Schaller and Fan, 2009).
-
Human water use systematically redistributes water resources at the continental scale via nonlocal atmospheric feedbacks (Al-Yaari et al., 2019; Keune et al., 2018).
Alternatively, it might be helpful to also include hypotheses that have been shown to be incorrect since models should not show relationships that have been shown not to exist in nature. For example, a hypotheses that has recently been shown to be incorrect is that the baseflow fraction (baseflow volume to precipitation volume) follows the Budyko curve (Gnann et al., 2019). As yet another alternative, hydrologic intuition could form the basis of model experiments, potentially including extreme model experiments (far from the natural conditions). For example, an experiment that artificially lowers the water table by decreasing precipitation (or recharge directly) could hypothesize the spatial variability across a domain regarding how the drainage flux will increase and evaporation flux will decrease as the water table is lowered. These hypotheses are meant only for illustrative purposes, and we hope future community debate will clarify the most appropriate and effective hypotheses. We believe that the debate around these hypotheses alone will lead to advances in our understanding or at least highlight differences in opinion.
Formal approaches are available to gather the opinions of experts and to integrate them into a joint result, often called expert elicitation (Aspinall, 2010; Cooke, 1991; O'Hagan, 2019). Expert elicitation strategies have been used widely to describe the expected behavior of environmental or man-made systems for which we have insufficient data or knowledge to build models directly. Examples include aspects of future sea level rise (Bamber and Aspinall, 2013), tipping points in the Earth system (Lenton et al., 2008), and the vulnerability of bridges to scour due to flooding (Lamb et al., 2017). In the groundwater community, expert opinion is already widely used to develop system conceptualizations and related model structures (Krueger et al., 2012; Rajabi et al., 2018; Refsgaard et al., 2007) or to define parameter priors (Ross et al., 2009; Doherty and Christensen, 2011; Brunner et al., 2012; Knowling and Werner, 2016; Rajabi and Ataie-Ashtiani, 2016). The term expert opinion may be preferable to the term expert knowledge because it emphasizes a preliminary state of knowledge (Krueger et al., 2012).
A critical benefit of expert elicitation is the opportunity to bring together researchers who have experienced very different groundwater systems around the world. It is infeasible to expect that a single person could have gained in-depth experience in modeling groundwater in semi-arid regions, in cold regions, and in tropical regions. Being able to bring together different experts who have studied one or a few of these systems to form a group would certainly create a whole that is bigger than the sum of its parts. If captured, it would be a tremendous source of knowledge for the evaluation of large-scale groundwater models. Expert elicitation also has a number of challenges including (1) formalizing this knowledge in such a way that it is still usable by third parties that did not attend the expert workshop itself and (2) perceived or real differences in perspectives, priorities, and backgrounds between regional-scale and large-scale modelers.
So, while expert opinion and judgment play a role in any scientific investigation (O'Hagan, 2019), including that of groundwater systems, we rarely use formal strategies to elicit this opinion. It is also less common to use expert opinion to develop hypotheses about the dynamic behavior of groundwater systems rather than just priors on its physical characteristics. Yet, it is intuitive that information about system behavior can help in evaluating the plausibility of model outputs (and thus of the model itself). This is what we call expert-based evaluation herein. Expert elicitation is typically done in workshops with groups of a dozen or so experts (e.g., Lamb et al., 2018). Upscaling such expert elicitation in support of global modeling would require some web-based strategy and a formalized protocol to engage a sufficiently large number of people. Contributors could potentially be incentivized to contribute to the web platform by publishing a data paper with all contributors as co-authors and a secondary analysis paper with just the core team as co-authors. We recommend that the community develop expert elicitation strategies to identify effective hypotheses that directly link to the relevant large-scale hydrologic processes of interest.
Ideally, all three strategies (observation-based, model-based, expert-based) should be pursued simultaneously because the strengths of one strategy might further improve others. For example, expert- or model-based evaluation may highlight and motivate the need for new observations in certain regions or at new resolutions. Or observation-based model evaluation could highlight and motivate further model development or lead to refined or additional hypotheses. We thus recommend that the community significantly strengthen efforts to evaluate large-scale models using all three strategies. Implementing these three model evaluation strategies may require a significant effort from the scientific community, so we therefore conclude with two tangible community-level initiatives that would be excellent first steps that can be pursued simultaneously with efforts by individual research groups or collaborations of multiple research groups.
First, we need to develop a groundwater modeling data portal that would both facilitate and accelerate the evaluation of groundwater representation in continental- to global-scale models (Bierkens, 2015). Existing initiatives such as IGRAC's Global Groundwater Monitoring Network (https://www.un-igrac.org/special-project/ggmn-global-groundwater-monitoring-network, last access: 15 November 2021) and HydroFrame (https://hydroframe.org/, last access: 15 November 2021) are an important first step but were not designed to improve the evaluation of large-scale models, and the synthesized data remain very heterogeneous – unfortunately, even groundwater level time series data often remain either hidden or inaccessible for various reasons. This open and well-documented data portal should include the following:
- a.
observations for evaluation (Table 2) and derived signatures (Sect. 3.1);
- b.
regional-scale models that meet the standards described above and could facilitate inter-scale comparison (Sect. 3.2) as well as being a first step towards linking regional models (Sect. 2.2);
- c.
schematizations and/or conceptual or perceptual models of large-scale models since these are the basis of computational models; and
- d.
hypotheses and other results derived from expert elicitation (Sect. 3.3).
Metadata documentation, data tagging, aggregation, and services as well as consistent data structures using well-known formats (NetCDF, .csv, .txt) will be critical to developing a useful, dynamic, and evolving community resource. The data portal should be directly linked to harmonized input data such as forcings (climate, land and water use, etc.) and parameters (topography, subsurface parameters, etc.), model codes, and harmonized output data. Where possible, the portal should follow established protocols, such as the Dublin Core Standards for metadata (https://dublincore.org/, last access: 15 November 2021) and ISIMIP protocols for harmonizing data and modeling approaches, and would ideally be linked to or contained within an existing disciplinary repository such as HydroShare (https://www.hydroshare.org/, last access: 15 November 2021) to facilitate discovery, maintenance, and long-term support. Additionally, an emphasis on model objective, uncertainty, and regional differences as highlighted (Sect. 3) will be important in developing the data portal. Like expert elicitation, contribution to the data portal could be incentivized through co-authorship in data papers and by providing digital object identifiers (DOIs) to submitted data and models so that they are citable. By synthesizing and sharing groundwater observations, models, and hypotheses, this portal would be broadly useful to the hydrogeological community beyond just improving global model evaluation.
Second, we suggest that ISIMIP, or a similar model intercomparison project, could be harnessed as a platform to improve the evaluation of groundwater representation in continental- to global-scale models. For example, in ISIMIP (Warszawski et al., 2014), modeling protocols have been developed with an international network of climate-impact modelers across different sectors (e.g., water, agriculture, energy, forestry, marine ecosystems) and spatial scales. Originally, ISIMIP started with multi-model comparison (model-based model evaluation), with a focus on understanding how model projections vary across different sectors and different climate change scenarios (ISIMIP Fast Track). However, more rigorous model evaluation drew attention more recently with ISIMIP2a, and various observation data, such as river discharge (Global Runoff Data Center), terrestrial water storage (GRACE), and water use (national statistics), have been used to evaluate historical model simulations (observation-based model evaluation). To better understand model differences and to quantify the associated uncertainty sources, ISIMIP2b includes evaluating scenarios (land use, groundwater use, human impacts, etc.) and key assumptions (no explicit groundwater representation, groundwater availability for the future, water allocation between surface water and groundwater), highlighting the fact that different types of hypotheses derived as part of the expert-based model evaluation could possibly be simulated as part of the ISIMIP process in the future. While there has been a significant amount of research and many publications on MIPs including surface water availability, limited multi-model assessments for large-scale groundwater studies exist. Important aspects of MIPs in general could facilitate all three model evaluation strategies: community building and cooperation with various scientific communities and research groups, as well as making the model input and output publicly available in a standardized format.
Large-scale hydrologic and land surface models increasingly represent groundwater, which we envision will lead to a better understanding of large-scale water systems and to more sustainable water resource use. We call on various scientific communities to join us in this effort to improve the evaluation of groundwater in continental to global models. As described by examples above, we have already started this journey and we hope this will lead to better outcomes, especially for the goals of including groundwater in large-scale models that we started with above: improving our understanding of Earth system processes and informing water decisions and policy. Along with the community currently directly involved in large-scale groundwater modeling, above we have made pointers to other communities who we hope will engage to accelerate model evaluation: (1) regional hydrogeologists, who would be especially useful in expert-based model evaluation (Sect. 3.3); (2) data scientists with expertise in machine learning and artificial intelligence, among other areas, whose methods could be especially useful for observation- and model-based model evaluation (Sect. 3.1 and 3.2); and (3) the multiple Earth science communities that are currently working towards integrating groundwater into a diverse range of models so that improved evaluation approaches are built directly into model development. Together we can better understand what has always been beneath our feet but often forgotten or neglected.
This perspective paper does not present any computational results. There are therefore no codes or data associated with this paper.
TG, TW, and PD were responsible for conceptualization and writing the original draft. All co-authors contributed to writing, review, and editing. Authors are ordered by contribution for the first three co-authors (TG, TW, and PD) and then ordered in reverse alphabetical order for all remaining co-authors (using the CRediT taxonomy, which offers standardized descriptions of author contributions).
The authors declare that they have no conflict of interest.
Publisher's note: Copernicus Publications remains neutral with regard to jurisdictional claims in published maps and institutional affiliations.
The commentary is based on a workshop at the University of Bristol as well as significant debate and discussion before and after. This community project was directly supported by a Benjamin Meaker Visiting Professorship at the University of Bristol to Tom Gleeson and by funding from the Alexander von Humboldt Foundation to Thorsten Wagener in the framework of the Alexander von Humboldt Professorship endowed by the German Federal Ministry of Education and Research. We thank many members of the community who contributed to the discussions, especially at the IGEM (Impact of Groundwater in Earth System Models) workshop in Taiwan.
This paper was edited by Jatin Kala and reviewed by two anonymous referees.
Al-Yaari, A., Ducharne, A., Cheruy, F., Crow, W. T., and Wigneron, J. P.: Satellite-based soil moisture provides missing link between summertime precipitation and surface temperature biases in CMIP5 simulations over conterminous United States, Scientific Reports, 9, 1657, https://doi.org/10.1038/s41598-018-38309-5, 2019.
Anderson, M. P., Woessner, W. W., and Hunt, R. J.: Applied groundwater modeling, 2nd edn., Academic Press, San Diego, 2015.
Anderson, R. G., Lo, M.-H., Swenson, S., Famiglietti, J. S., Tang, Q., Skaggs, T. H., Lin, Y.-H., and Wu, R.-J.: Using satellite-based estimates of evapotranspiration and groundwater changes to determine anthropogenic water fluxes in land surface models, Geosci. Model Dev., 8, 3021–3031, https://doi.org/10.5194/gmd-8-3021-2015, 2015.
Alley, W. M. and Konikow, L. F. Bringing GRACE down to earth, Groundwater, 53, 826–829, 2015.
Anyah, R. O., Weaver, C. P., Miguez-Macho, G., Fan, Y., and Robock, A. Incorporating water table dynamics in climate modeling: 3. Simulated groundwater influence on coupled land-atmosphere variability, J. Geophys. Res., 113, D07103, https://doi.org/10.1029/2007JD009087, 2008.
Aspinall, W.: A route to more tractable expert advice, Nature, 463, 294–295, https://doi.org/10.1038/463294a, 2010.
ASTM Standard Guide for Conducting a Sensitivity Analysis for a Groundwater Flow Model Application, ASTM International D5611-94, West Conshohocken, PA, available at: https://www.astm.org/ (last access: 15 November 2021), 2016.
Bamber, J. L. and Aspinall, W. P.: An expert judgement assessment of future sea level rise from the ice sheets, Nat. Clim. Change, 3, 424–427, 2013.
Barnett, B., Townley, L. R., Post, V. E. A., Evans, R. E., Hunt, R. J., Peeters, L., Richardson, S., Werner, A. D., Knapton, A., Boronkay, A.: Australian groundwater modelling guidelines, National Water Commission, Canberra, 203 pp., 2012.
Barthel, R.: HESS Opinions “Integration of groundwater and surface water research: an interdisciplinary problem?”, Hydrol. Earth Syst. Sci., 18, 2615–2628, https://doi.org/10.5194/hess-18-2615-2014, 2014.
Beck, H. E., van Dijk, A. I. J. M., Miralles, D. G., de Jeu, R. A. M., Bruijnzeel, L. A., McVicar, T. R., and Schellekens, J.: Global patterns in base flow index and recession based on streamflow observations from 3394 catchments, Water Resour. Res., 49, 7843–7863, 2013.
Befus, K., Jasechko, S., Luijendijk, E., Gleeson, T., and Cardenas, M. B.: The rapid yet uneven turnover of Earth's groundwater, Geophys. Res. Lett., 11, 5511–5520, https://doi.org/10.1002/2017GL073322, 2017.
Best, M. J., Pryor, M., Clark, D. B., Rooney, G. G., Essery, R. L. H., Ménard, C. B., Edwards, J. M., Hendry, M. A., Porson, A., Gedney, N., Mercado, L. M., Sitch, S., Blyth, E., Boucher, O., Cox, P. M., Grimmond, C. S. B., and Harding, R. J.: The Joint UK Land Environment Simulator (JULES), model description – Part 1: Energy and water fluxes, Geosci. Model Dev., 4, 677–699, https://doi.org/10.5194/gmd-4-677-2011, 2011.
Beven, K.: On the concept of model structural error, Water Sci. Technol., 52, 167–175, 2005.
Beven, K.: Facets of uncertainty: epistemic uncertainty, nonstationarity, likelihood, hypothesis testing, and communication, Hydrolog. Sci. J., 61, 1652–1665, https://doi.org/10.1080/02626667.2015.1031761, 2016.
Beven, K.: How to make advances in hydrological modelling, Hydrol. Res., 50, 1481–1494, 2019.
Beven, K.: Deep learning, hydrological processes and the uniqueness of place, Hydrol. Process., 34, 3608–3613, https://doi.org/10.1002/hyp.13805, 2020.
Beven, K. J., Aspinall, W. P., Bates, P. D., Borgomeo, E., Goda, K., Hall, J. W., Page, T., Phillips, J. C., Simpson, M., Smith, P. J., Wagener, T., and Watson, M.: Epistemic uncertainties and natural hazard risk assessment – Part 2: What should constitute good practice?, Nat. Hazards Earth Syst. Sci., 18, 2769–2783, https://doi.org/10.5194/nhess-18-2769-2018, 2018.
Bierkens, M. F. P.: Global hydrology 2015: State, trends, and directions, Water Resour. Res., 51, 4923–4947, https://doi.org/10.1002/2015WR017173, 2015.
Bierkens, M. F. P. and Wada, Y.: Non-renewable groundwater use and groundwater depletion: A review, Environ. Res. Lett., 14, 063002, https://doi.org/10.1088/1748-9326/ab1a5f, 2019.
Blöschl, G., Sivapalan, M., Wagener, T., Viglione, A., and Savenije, H. (Eds.): Runoff Prediction in Ungauged Basins: Synthesis Across Processes, Places and Scales, Cambridge University Press, 465 pp., ISBN: 978-1107028180, 2013.
Boone, A. A., Habets, F., Noilhan, J., Clark, D., Dirmeyer, P., Fox, S., Gusev, Y., Haddeland, I., Koster, R., Lohmann, D., Mahanama, S., Mitchell, K., Nasonova, O., Niu, G. Y., Pitman, A., Polcher, J., Shmakin, A. B., Tanaka, K., Van Den Hurk, B., Vérant, S., Verseghy, D., Viterbo, P., and Yang, Z. L.: The Rhône-aggregation land surface scheme intercomparison project: An overview, J. Climate, 17, 187–208, https://doi.org/10.1175/1520-0442(2004)017<0187:TRLSSI>2.0.CO;2, 2004.
Borgonovo, E., Lu, X., Plischke, E., Rakovec, O., and Hill, M. C.: Making the most out of a hydrological model data set: Sensitivity analyses to open the model black-box, Water Resour. Res., 53, 7933–7950, https://doi.org/10.1002/2017WR020767, 2017.
Bresciani, E., Goderniaux, P., and Batelaan, O.: Hydrogeological controls of water table-land surface interactions, Geophys. Res. Lett., 43, 9653–9661, 2016.
Bresciani, E., Cranswick, R. H., Banks, E. W., Batlle-Aguilar, J., Cook, P. G., and Batelaan, O.: Using hydraulic head, chloride and electrical conductivity data to distinguish between mountain-front and mountain-block recharge to basin aquifers, Hydrol. Earth Syst. Sci., 22, 1629–1648, https://doi.org/10.5194/hess-22-1629-2018, 2018.
Brunner, P. and Simmons, C. T.: HydroGeoSphere: A Fully Integrated, Physically Based Hydrological Model, Groundwater, 50, 170–176, https://doi.org/10.1111/j.1745-6584.2011.00882.x, 2012.
Brunner, P., Doherty, J., and Simmons, C. T.: Uncertainty assessment and implications for data acquisition in support of integrated hydrologic models, Water Resour. Res., 48, W07513, https://doi.org/10.1029/2011WR011342, 2012.
Burgess, W. G., Shamsudduha, M., Taylor, R. G., Zahid, A., Ahmed, K. M., Mukherjee, A., Lapworth, D. J., and Bense, V. F.: Terrestrial water load and groundwater fluctuation in the Bengal Basin, Scientific Reports, 7, 3872, 2017.
Cáceres, D., Marzeion, B., Malles, J. H., Gutknecht, B. D., Müller Schmied, H., and Döll, P.: Assessing global water mass transfers from continents to oceans over the period 1948–2016, Hydrol. Earth Syst. Sci., 24, 4831–4851, https://doi.org/10.5194/hess-24-4831-2020, 2020.
Ceola, S., Arheimer, B., Baratti, E., Blöschl, G., Capell, R., Castellarin, A., Freer, J., Han, D., Hrachowitz, M., Hundecha, Y., Hutton, C., Lindström, G., Montanari, A., Nijzink, R., Parajka, J., Toth, E., Viglione, A., and Wagener, T.: Virtual laboratories: new opportunities for collaborative water science, Hydrol. Earth Syst. Sci., 19, 2101–2117, https://doi.org/10.5194/hess-19-2101-2015, 2015.
Clark, M. P., Slater, A. G., Rupp, D. E., Woods, R. A., Vrugt, J. A., Gupta, H. V., Wagener, T., and Hay, L. E.: Framework for Understanding Structural Errors (FUSE): A modular framework to diagnose differences between hydrological models, Water Resour. Res., 44, W00B02, https://doi.org/10.1029/2007WR006735, 2008.
Clark, M. P., Nijssen, B., Lundquist, J. D., Kavetski, D., Rupp, D. E., Woods, R. A., Freer, J. E., Gutmann, E. D., Wood, A. W., Brekke, L. D., Arnold, J. R., Gochis, D. J., and Rasmussen R. M.: A unified approach for process-based hydrologic modeling: 1. Modeling concept, Water Resour. Res., 51, 2498–2514, https://doi.org/10.1002/2015WR017198, 2015.
Condon, L. E. and Maxwell, R. M.: Simulating the sensitivity of evapotranspiration and streamflow to large-scale groundwater depletion, Science Advances, 5, eaav4574, https://doi.org/10.1126/sciadv.aav4574, 2019.
Condon, L. E., Markovich, K. H., Kelleher, C. A., McDonnell, J. J., Ferguson, G., and McIntosh, J. C.: Where Is the Bottom of a Watershed?, Water Resour. Res., 56, e2019WR026010, https://doi.org/10.1029/2019wr026010, 2020.
Condon, L. E., Kollet, S., Bierkens, M. F. P., Maxwell, R. M., Hill, M. C., Verhoef, A., Van Loon, A. F., Fogg, G. E., Sulis, M., Fransen, H.-J. H., and Corinna Abesser, C.: Global groundwater modeling and monitoring?: Opportunities and challenges, Water Resour. Res., in review, 2021.
Cooke, R.: Experts in uncertainty: opinion and subjective probability in science, Oxford University Press, UK, ISBN-10: 0195064658, 1991.
Cuthbert, M. O., Gleeson, T., Moosdorf, N., Befus, K. M., Schneider, A., Hartmann, J., and Lehner, B.: Global patterns and dynamics of climate–groundwater interactions, Nat. Clim. Change, 9, 137–141, https://doi.org/10.1038/s41558-018-0386-4, 2019a.
Cuthbert, M. O., Taylor, R. G., Favreau, G., Todd, M. C., Shamsudduha, M., Villholth, K. G., MacDonald, A. M., Scanlon, B. R., Kotchoni, D. O. V., Vouillamoz, J. M., Lawson, F. M. A., Adjomayi, P. A., Kashaigili, J., Seddon, D., Sorensen, J. P. R., Ebrahim, G. Y., Owor, M., Nyenje, P. M., Nazoumou, Y., Goni, I., Ousmane, B. I., Sibanda, T., Ascott, M. J., Macdonald, D. M. J., Agyekum, W., Koussoube, Y., Wanke, H., Kim, H., Wada, Y., Lo, M. H., Oki, T., and Kukuric, N.: Observed controls on resilience of groundwater to climate variability in sub-Saharan Africa, Nature, 572, 230–234, 2019b.
Dalin, C., Wada, Y., Kastner, T., and Puma, M. J.: Groundwater depletion embedded in international food trade, Nature, 543, 700–704, https://doi.org/10.1038/nature21403, 2017.
DeAngelis, A., Dominguez, F., Fan, Y., Robock, A., Kustu, M. D., and Robinson, D.: Evidence of enhanced precipitation due to irrigation over the Great Plains of the United States, J. Geophys. Res., 115, D15115, https://doi.org/10.1029/2010JD013892, 2010.
Dirmeyer, P. A.: A History and Review of the Global Soil Wetness Project (GSWP), J. Hydrometeorol., 12, 729–749, https://doi.org/10.1175/jhm-d-10-05010, 2011.
Doherty, J., and Christensen, S.: Use of paired simple and complex models to reduce predictive bias and quantify uncertainty, Water Resour. Res., 47, W12534, https://doi.org/10.1029/2011WR010763, 2011.
Döll, P. and Fiedler, K.: Global-scale modeling of groundwater recharge, Hydrol. Earth Syst. Sci., 12, 863–885, https://doi.org/10.5194/hess-12-863-2008, 2008.
Döll, P., Douville, H., Güntner, A., Müller Schmied, H., and Wada, Y.: Modelling freshwater resources at the global scale: Challenges and prospects, Surv. Geophys., 37, 195–221, https://doi.org/10.1007/s10712-015-9343-1, 2016.
Döll, P., Müller Schmied, H., Schuh, C., Portmann, F. T., and Eicker, A.: Global-scale assessment of groundwater depletion and related groundwater abstractions: Combining hydrological modeling with information from well observations and GRACE satellites, Water Resour. Res., 50, 5698–5720, https://doi.org/10.1002/2014WR015595, 2014a.
Döll, P., Fritsche, M., Eicker, A., and Müller Schmied, H.: Seasonal water storage variations as impacted by water abstractions: Comparing the output of a global hydrological model with GRACE and GPS observations, Surv. Geophys., 35, 1311—1331, https://doi.org/10.1007/s10712-014-9282-2, 2014b.
Döll, P., Hoffmann-Dobrev, H., Portmann, F. T., Siebert, S., Eicker, A., Rodell, M., Strassberg, G., and Scanlon, B.: Impact of water withdrawals from groundwater and surface water on continental water storage variations, J. Geodyn., 59–60, 143–156, https://doi.org/10.1016/j.jog.2011.05.001, 2012.
Duan Q., Schaake, J., Andreassian, V., Franks, S., Gupta, H. V., Gusev, Y. M., Habets, F., Hall, A., Hay, L., Hogue, T. S., Huang, M., Leavesley, G., Liang, X., Nasonova, O. N., Noilhan, J., Oudin, L., Sorooshian, S., Wagener, T., and Wood, E. F.: Model Parameter Estimation Experiment (MOPEX): Overview and Summary of the Second and Third Workshop Results, J. Hydrol., 320, 3–17, 2006.
Enemark, T., Peeters, L. J. M., Mallants, D., and Batelaan, O.: Hydrogeological conceptual model building and testing: A review, J. Hydrol., 569, 310–329, https://doi.org/10.1016/j.jhydrol.2018.12.007, 2019.
Erban, L. E., Gorelick, S. M., and Zebker, H. A.: Groundwater extraction, land subsidence, and sea-level rise in the Mekong Delta, Vietnam, Environ. Res. Lett., 9, 084010, https://doi.org/10.1088/1748-9326/9/8/084010, 2014.
Famiglietti, J. S. and Wood, E. F.: Multiscale modeling of spatially variable water and energy balance processes, Water Resour. Res., 30, 3061–3078, https://doi.org/10.1029/94WR01498, 1994.
Fan, Y., Clark, M., Lawrence, D. M., Swenson, S., Band, L. E., Brantley, S. L., Brooks, P. D., Bitrich, W. E., Flores, A., Grant, G., Kirchner, J. W., Mackay, D.S., McDonnel J. J., Milly, P. C. D., Sulivan, P. L., Tague, C., Ajmai, H., Chaney, N., Harmann, A., Hazenberg, P., McNamara, J., Ppelletier, J., Perket, J., Rouholahnejad-Freund, E., Wagner, T., Zeng, X., Beighley, E., Buzan, J., Huang, M., Livneh, B., Mohanty, B. P., Nijssen, B., Safeeq, M., Shen, C., Van Verseveld, W., Volk, J., and Yamazaki, D.: Hillslope hydrology in global change research and Earth System modeling, Water Resour. Res., 55, 1737–1772, https://doi.org/10.1029/2018WR023903, 2019.
Fan, Y.: Groundwater in the Earth's critical zone: Relevance to large-scale patterns and processes, Water Resour. Res., 51, 3052–3069, https://doi.org/10.1002/2015WR017037, 2015.
Fan, Y., Li, H., and Miguez-Macho, G.: Global patterns of groundwater table depth, Science, 339, 940–943, 2013.
Fenicia, F., Kavetski, D., and Savenije, H. H. G.: Elements of a flexible approach for conceptual hydrological modeling: 1. Motivation and theoretical development, Water Resour. Res., 47, W11510, https://doi.org/10.1029/2010wr010174, 2011.
Forrester, M. M. and Maxwell, R. M.: Impact of lateral groundwater flow and subsurface lower boundary conditions on atmospheric boundary layer development over complex terrain, J. Hydrometeorol., 21, 1133–1160, https://doi.org/10.1175/JHM-D-19-0029.1, 2020.
Forrester, M. M., Maxwell, R. M., Bearup, L. A., and Gochis, D. J.: Forest Disturbance Feedbacks from Bedrock to Atmosphere Using Coupled Hydro-Meteorological Simulations Over the Rocky Mountain Headwaters, J. Geophys. Res.-Atmos., 123, 9026–9046, https://doi.org/10.1029/2018JD028380, 2018.
Foster, S., Chilton, J., Nijsten, G.-J., and Richts, A.: Groundwater – a global focus on the 'local resource', Curr. Opin. Env. Sust., 5, 685–695, https://doi.org/10.1016/j.cosust.2013.10.010, 2013.
Gascoin, S., Ducharne, A., Ribstein, P., Carli, M., and Habets, F.: Adaptation of a catchment-based land surface model to the hydrogeological setting of the Somme River basin (France), J. Hydrol., 368, 105–116, https://doi.org/10.1016/j.jhydrol.2009.01.039, 2009.
Gilbert, J. M., Maxwell, R. M., and Gochis, D. J.: Effects of water table configuration on the planetary boundary layer over the San Joaquin River watershed, California, J. Hydrometeorol., 18, 1471–1488, https://doi.org/10.1175/JHM-D-16-0134.1, 2017.
Gleeson, T., Wagener, T., Döll, P., Zipper, S. C., West, C., Wada, Y., Taylor, R., Scanlon, B., Rosolem, R., Rahman, S., Oshinlaja, N., Maxwell, R., Lo, M.-H., Kim, H., Hill, M., Hartmann, A., Fogg, G., Famiglietti, J. S., Ducharne, A., de Graaf, I., Cuthbert, M., Condon, L., Bresciani, E., and Bierkens, M. F. P.: HESS Opinions: Improving the evaluation of groundwater representation in continental to global scale models, Hydrol. Earth Syst. Sci. Discuss. [preprint], https://doi.org/10.5194/hess-2020-378, 2020.
Gleeson, T. and Manning, A. H.: Regional groundwater flow in mountainous terrain: Three-dimensional simulations of topographic and hydrogeologic controls, Water Resour. Res., 44, W10403, https://doi.org/10.1029/2008WR006848, 2008.
Gleeson, T., Befus, K. M., Jasechko, S., Luijendijk, E., and Cardenas, M. B.: The global volume and distribution of modern groundwater, Nat. Geosci., 9, 161–167, 2016.
de Graaf, I. E. M., van Beek, L. P. H., Wada, Y., and Bierkens, M. F. P.: Dynamic attribution of global water demand to surface water and groundwater resources: Effects of abstractions and return flows on river discharges, Adv. Water Resour., 64, 21–33 https://doi.org/10.1016/j.advwatres.2013.12.002, 2014.
de Graaf, I. E. M., Sutanudjaja, E. H., van Beek, L. P. H., and Bierkens, M. F. P.: A high-resolution global-scale groundwater model, Hydrol. Earth Syst. Sci., 19, 823–837, https://doi.org/10.5194/hess-19-823-2015, 2015.
de Graaf, I. E. M., van Beek, L. P. H., Gleeson, T., Moosdorf, N., Schmitz, O., Sutanudjaja, E. H., and Bierkens, M. F. P.: A global-scale two-layer transient groundwater model: Development and application to groundwater depletion, Adv. Water Resour., 102, 53–67, https://doi.org/10.1016/j.advwatres.2017.01.011, 2017.
de Graaf, I. E. M., Gleeson, T., Beek, L. P. H. (Rens) van, Sutanudjaja, E. H., and Bierkens, M. F. P.: Environmental flow limits to global groundwater pumping, Nature, 574, 90–94, https://doi.org/10.1038/s41586-019-1594-4, 2019.
Gnann, S. J., Woods, R. A., and Howden, N. J.: Is there a baseflow Budyko curve?, Water Resour. Res., 55, 2838–2855, 2019.
Goderniaux, P., Davy, P., Bresciani, E., de Dreuzy, J.-R., and Le Borgne, T.: Partitioning a regional groundwater flow system into shallow local and deep regional flow compartments, Water Resour. Res., 49, 2274—2286, 2013.
Guimberteau, M., Ducharne, A., Ciais, P., Boisier, J. P., Peng, S., De Weirdt, M., and Verbeeck, H.: Testing conceptual and physically based soil hydrology schemes against observations for the Amazon Basin, Geosci. Model Dev., 7, 1115–1136, https://doi.org/10.5194/gmd-7-1115-2014, 2014.
Habets, F., Boé, J., Déqué, M., Ducharne, A., Gascoin, S., Hachour, A., Martin, E., Pagé, C., Sauquet, E., Terray, L., Thiéry, D., Oudin, L., and Viennot, P.: Impact of climate change on surface water and ground water of two basins in Northern France: analysis of the uncertainties associated with climate and hydrological models, emission scenarios and downscaling methods, Climatic Change, 121, 771–785, https://doi.org/10.1007/s10584-013-0934-x, 2013.
Hartmann, A., Gleeson, T., Rosolem, R., Pianosi, F., Wada, Y., and Wagener, T.: A large-scale simulation model to assess karstic groundwater recharge over Europe and the Mediterranean, Geosci. Model Dev., 8, 1729–1746, https://doi.org/10.5194/gmd-8-1729-2015, 2015.
Hartmann, Andreas, Gleeson, T., Wada, Y., and Wagener, T.: Enhanced groundwater recharge rates and altered recharge sensitivity to climate variability through subsurface heterogeneity, P. Natl. Acad. Sci. USA, 114, 2842–2847, https://doi.org/10.1073/pnas.1614941114, 2017.
Hattermann, F. F., Krysanova, V., Gosling, S. N., Dankers, R., Daggupati, P., Donnelly, C., Florke, M., Huang, S., Motovilov, Y., Buda, S., Yang, T., Muller, C., Leng, G., Tang, Q., Portman, F. T., Hanemann, S,. Gerten, D., Wada, Y., Masaki, Y., Alemayehu, T., Satoh, Y., and Samaniego, L.: Cross-scale intercomparison of climate change impacts simulated by regional and global hydrological models in eleven large river basins, Climatic Change, 141, 561–576, https://doi.org/10.1007/s10584-016-1829-4, 2017.
Hawker, L. P., Rougier, J., Neal, J. C., Bates, P. D., Archer, L., and Yamazaki, D.: Implications of simulating global digital elevation models for flood inundation studies, Water Resour. Res., 54, 7910–7928, 2018.
Hay, L., Norton, P., Viger, R., Markstrom, S., Regan, R. S., and Vanderhoof, M.: Modelling surface-water depression storage in a Prairie Pothole Region, Hydrol. Process., 32, 462–479, https://doi.org/10.1002/hyp.11416, 2018.
Henderson-Sellers, A., Yang, Z. L., and Dickinson, R. E.: The Project for Intercomparison of Land-Surface Schemes (PILPS), B. Am. Meteorol. Soc., 74, 1335–1349, 1993.
Herbert, C. and Döll, P.: Global assessment of current and future groundwater stress with a focus on transboundary aquifers, Water Resour. Res., 55, 4760–4784, https://doi.org/10.1029/2018WR023321, 2019.
Heudorfer, B., Haaf, E., Stahl, K., and Barthel, R.: Index-based characterization and quantification of groundwater dynamics, Water Resour. Res., 55, 5575–5592, https://doi.org/10.1029/2018WR024418, 2019.
Hill, M. C.: The practical use of simplicity in developing ground water models, Ground Water, 44, 775–781, https://doi.org/10.1111/j.1745-6584.2006.00227.x, 2006.
Hill, M. C. and Tiedeman, C. R.: Effective groundwater model calibration, Wiley, USA, ISBN: 978-0-471-77636-9, 2007.
Hill, M.C., Kavetski, D., Clark, M., Ye, M., Arabi, M., Lu, D., Foglia, L. and Mehl, S.: Practical use of computationally frugal model analysis methods, Groundwater, 54, 159–170, https://doi.org/10.1111/gwat.12330, 2016.
Hiscock, K. M. and Bense, V. F.: Hydrogeology – principles and practice, 2nd edn., Wiley-Blackwell, USA, ISBN: 978-0-470-65662-4, 2014.
Huang, S., Kumar, R., Flörke, M., Yang, T., Hundecha , Y., Kraft, P., Gao, C., Gelfan, A., Liersch, S., Lobanova, A., Strauch, M., Van Ogtrop, F., Reinhardt, J., Haberlandt, U., Krysanova, V.: Evaluation of an ensemble of regional hydrological models in 12 large-scale river basins worldwide, Climatic Change, 141, 381–397, https://doi.org/10.1007/s10584-016-1841-8, 2017.
Hrachowitz, M., Fovet, O., Ruiz, L., Euser, T., Gharari, S., Nijzink, R., Freer, J., Savenije, H. H. G., and Gascuel-Odoux, C.: Process Consistency in Models: the Importance of System Signatures, Expert Knowledge and Process Complexity, Water Resour. Res., 50, 7445–7469, 2014.
Hunt, R. J., Walker, J. F., Selbig, W. R., Westenbroek, S. M., and Regan, R. S.: Simulation of climate-change effects on streamflow, lake water budgets, and stream temperature using GSFLOW and SNTEMP, Trout Lake Watershed, Wisconsin, Geological Survey, Reston, VA, U.S., USGS Scientific Investigations Report No. 2013–5159, 2013.
Hutton, C., Wagener, T., Freer, J., Han, D., Duffy, C., and Arheimer, B.: Most computational hydrology is not reproducible, so is it really science?, Water Resour. Res., 52, 7548–7555, https://doi.org/10.1002/2016WR019285, 2016.
Jasechko, S., Birks, S. J., Gleeson, T., Wada, Y., Sharp, Z. D., Fawcett, P. J., McDonnell, J. J., and Welker, J. M.: Pronounced seasonality in the global groundwater recharge, Water Resour. Res., 50, 8845–8867, https://doi.org/10.1002/2014WR015809, 2014.
Jasechko, S., Perrone, D., Befus, K. M., Bayani Cardenas, M., Ferguson, G., Gleeson, T., Luijendijk, E., McDonnell, J. J., Taylor, R. G., Wada, Y., and Kirchner, J. W.: Global aquifers dominated by fossil groundwaters but wells vulnerable to modern contamination, Nat. Geosci., 10, 425–429, https://doi.org/10.1038/ngeo2943, 2017.
Jung, M., Reichstein, M., Margolis, H. A., Cescatti, A., Richardson, A. D., Arain, M. A., Arneth, A., Bernhofer, C., Bonal, D., Chen, J., Gainelle, D., Gobron, N., Kiely, G., Kutsch, W., Lasslop, G., Law, B. E., Lindroth, A., Merbold, L., Montagnani, L., Moors, E. J., Papale, D., Sottocornola, M., Vaccari, F., and Williams, C.: Global patterns of land-atmosphere fluxes of carbon dioxide, latent heat, and sensible heatderived from eddy covariance, satellite, and meteorological observations, J. Geophys. Res., 116, G00J07, https://doi.org/10.1029/2010JG001566, 2011.
Keune, J., Sulis, M., Kollet, S., Siebert, S., and Wada, Y.: Human Water Use Impacts on the Strength of the Continental Sink for Atmospheric Water, Geophys. Res. Lett., 45, 4068–4076, https://doi.org/10.1029/2018GL077621, 2018.
Knowling, M. J. and Werner, A. D.: Estimability of recharge through groundwater model calibration: Insights from a field-scale steady-state example, J. Hydrol., 540, 973–987, 2016.
Koirala, S., Yeh, P. J. F., Hirabayashi, Y., Kanae, S., and Oki, T.: Global-scale land surface hydrologic modeling with the representation of water table dynamics, dynamics, J. Geophys. Res.-Atmos., 119, 75–89, https://doi.org/10.1002/2013JD020398, 2014.
Kollet, S. J. and Maxwell, R. M.: Capturing the influence of groundwater dynamics on land surface processes using an integrated, distributed watershed model, Water Resour. Res., 44, W02402, https://doi.org/10.1029/2007WR006004, 2008.
Kollet, S., Sulis, M., Maxwell, R. M., Paniconi, C., Putti, M., Bertoldi, G., Coon, E. T., Cordano, E., Endrizzi, S., Kikinzon, E., Mouche, E., Mugler, C., Park, Y., Refsgaard, J. C., Stisen, S., and Sudicky, E.: The integrated hydrologic model intercomparison project, IH-MIP2: A second set of benchmark results to diagnose integrated hydrology and feedbacks, Water Resour. Res., 53, 867–890, 2017.
Konikow, L. F.: Contribution of global groundwater depletion since 1900 to sea-level rise, Geophys. Res. Lett., 38, L17401, https://doi.org/10.1029/2011GL048604, 2011.
Koster, R. D., Suarez, M. J., Ducharne, A., Praveen, K., and Stieglitz, M.: A catchment-based approach to modeling land surface processes in a GCM – Part 1: Model structure, J. Geophys. Res., 105, 24809–24822, 2000.
Krakauer, N. Y., Li, H., and Fan, Y.: Groundwater flow across spatial scales: importance for climate modeling, Environ. Res. Lett., 9, 034003, https://doi.org/10.1088/1748-9326/9/3/034003, 2014.
Kresic, N.: Groundwater resources: sustainability, management and restoration, McGraw-Hill, ISBN: 9780071492737, 2009.
Krueger, T., Page, T., Hubacek, K., Smith, L., and Hiscock, K.: The role of expert opinion in environmental modelling, Environ. Modell. Softw., 36, 4–18, 2012.
Kustu, M. D., Fan, Y., and Rodell, M.: Possible link between irrigation in the US High Plains and increased summer streamflow in the Midwest, Water Resour. Res., 47, W03522, https://doi.org/10.1029/2010WR010046, 2011.
Lamb, R., Aspinall, W., Odbert, H., and Wagener, T.: Vulnerability of bridges to scour: insights from an international expert elicitation workshop, Nat. Hazards Earth Syst. Sci., 17, 1393–1409, https://doi.org/10.5194/nhess-17-1393-2017, 2017.
Lawrence, D., Fisher, R., Koven, C., Oleson, K., Swenson, S., Vertenstein, M., Andre, B., Bonan, G., Ghimire, B., van Kampenhout, L., Kennedy, D., Kluzek, E., Knox, R., Lawrence, P., Li, F., Li, H., Lombardozzi, D., Lu, Y., Perket, J., Riley, W., Sacks, W., Shi, M., Wieder, W., Xu, C., Ali, A., Badger, A., Bisht, G., Broxton, P., Brunke, M., Buzan, J., Clark, M., Craig, T., Dahlin, K., Drewniak, B., Emmons, L., Fisher, J., Flanner, M., Gentine, P., Lenaerts, J., Levis, S., Leung, L. R., Lipscomb, W., Pelletier, J., Ricciuto, D. M., Sanderson, B., Shuman, J., Slater, A., Subin, Z., Tang, J., Tawfik, A., Thomas, Q., Tilmes, S., Vitt, F., and Zeng, X.: Technical Description of version 5.0 of the Community Land Model (CLM), 329 pp., available at: http://www.cesm.ucar.edu/models/cesm2/land/CLM50_Tech_Note.pdf (last access: 5 October 2021), 2018.
Leaf, A. T., Fienen, M. N., Hunt, R. J., and Buchwald, C. A.: Groundwater/surface-water interactions in the Bad River Watershed, Wisconsin, USGS Numbered Series No. 2015–5162, Geological Survey, Reston, VA, U.S., 2015.
Leavesley, G. H., Markstrom, S. L., Restrepo, P. J., and Viger, R. J.: A modular approach for addressing model design, scale, and parameter estimation issues in distributed hydrological modeling, Hydrol. Processes, 16, 173–187, https://doi.org/10.1002/hyp.344, 2002.
Lemieux, J. M., Sudicky, E. A., Peltier, W. R., and Tarasov, L.: Dynamics of groundwater recharge and seepage over the Canadian landscape during the Wisconsinian glaciation, J. Geophys. Res., 113, F01011, https://doi.org/10.1029/2007JF000838, 2008.
Lenton, T. M., Held, H., Kriegler, E., Hall, J. W., Lucht, W., Rahmstorf, S., and Schellnhuber, H. J.: Tipping elements in the Earth's climate system, P. Natl. Acad. Sci. USA, 105, 1786–1793, 2008.
Liang, X., Xie, Z., and Huang, M.: A new parameterization for surface and groundwater interactions and its impact on water budgets with the variable infiltration capacity (VIC) land surface model, J. Geophys. Res., 108, 8613, https://doi.org/10.1029/2002JD003090, 2003.
Lo, M.-H., Famiglietti, J. S., Reager, J. T., Rodell, M., Swenson, S., and Wu, W.-Y.: GRACE-Based Estimates of Global Groundwater Depletion, in: Terrestrial Water Cycle and Climate Change, edited bY: Tang, Q. and Oki, T., John Wiley and Sons, Inc., 135–146, https://doi.org/10.1002/9781118971772.ch7, 2016.
Lo, M.-H., Yeh, P. J.-F., and Famiglietti, J. S.: Constraining water table depth simulations in a land surface model using estimated baseflow, Adv. Water Resour., 31, 1552–1564, 2008.
Lo, M.-H., J. S. Famiglietti, P. J.-F. Yeh, and T. H. Syed.: Improving Parameter Estimation and Water Table Depth Simulation in a Land Surface Model Using GRACE Water Storage and Estimated Baseflow Data, Water Resour. Res., 46, W05517, https://doi.org/10.1029/2009WR007855, 2010.
Luijendijk, E., Gleeson, T., and Moosdorf, N.: Fresh groundwater discharge insignificant for the world's oceans but important for coastal ecosystems. Nat. Commun., 11, 1260, https://doi.org/10.1038/s41467-020-15064-8, 2020.
Maples, S. R., Foglia, L., Fogg, G. E., and Maxwell, R. M.: Sensitivity of hydrologic and geologic parameters on recharge processes in a highly heterogeneous, semi-confined aquifer system, Hydrol. Earth Syst. Sci., 24, 2437–2456, https://doi.org/10.5194/hess-24-2437-2020, 2020.
Margat, J. and Van der Gun, J.: Groundwater around the world: a geographic synopsis, CRC Press, London, ISBN 9780367576509, 2013.
Markovich, K. H., Manning, A. H., Condon, L. E., and McIntosh, J. C.: Mountain-block Recharge: A Review of Current Understanding, Water Resour. Res., 55, 8278–8304, https://doi.org/10.1029/2019WR025676, 2019.
Maxwell, R. M. and Condon, L. E.: Connections between groundwater flow and transpiration partitioning, Science, 353, 377–380, 2016.
Maxwell, R. M., Chow, F. K., and Kollet, S. J.: The groundwater-land-surface-atmosphere connection: soil moisture effects on the atmospheric boundary layer in fully-coupled simulations, Adv. Water Resour., 30, 2447–2466, https://doi.org/10.1016/j.advwatres.2007.05.018, 2007.
Maxwell, R. M., Condon, L. E., and Kollet, S. J.: A high-resolution simulation of groundwater and surface water over most of the continental US with the integrated hydrologic model ParFlow v3, Geosci. Model Dev., 8, 923–937, https://doi.org/10.5194/gmd-8-923-2015, 2015.
Maxwell, R. M., Condon, L. E., Kollet, S. J., Maher, K., Haggerty, R., and Forrester, M. M.: The imprint of climate and geology on the residence times of groundwater, Geophys. Res. Lett., 43, 701–708, https://doi.org/10.1002/2015GL066916, 2016.
McMilan, H.: Linking hydrologic signatures to hydrologic processes: A review, Hydrol. Process., 34, 1393–1409, 2020.
Meixner, T., Manning, A. H., Stonestrom, D. A., Allen, D. M., Ajami, H., Blasch, K. W., Brookfield, A. E., Castro, C. L., Clark, J. F., Gochis, D. J., Flint, A. L., Neff, K. L., Niraula, R., Rodell, M., Scanlon, B. R., Singha, K., and Walvoord, M. A.: Implications of projected climate change for groundwater recharge in the western United States, J. Hydrol., 534, 124–138, 2016.
Melsen, L. A., Teuling, A. J., Torfs, P. J. J. F., Uijlenhoet, R., Mizukami, N., and Clark, M. P.: HESS Opinions: The need for process-based evaluation of large-domain hyper-resolution models, Hydrol. Earth Syst. Sci., 20, 1069–1079, https://doi.org/10.5194/hess-20-1069-2016, 2016.
Meriano, M. and Eyles, N.: Groundwater flow through Pleistocene glacial deposits in the rapidly urbanizing Rouge River-Highland Creek watershed, City of Scarborough, southern Ontario, Canada, Hydrogeol. J., 11, 288–303, https://doi.org/10.1007/s10040-002-0226-4, 2003.
Milly, P. C. D., Malyshev, S. L., Shevliakova, E., Dunne, K. A., Findell, K. L., Gleeson, T., Liang, Z., Phillipps, P., Stouffer, R. J., and Swenson, S.: An Enhanced Model of Land Water and Energy for Global Hydrologic and Earth-System Studies, J. Hydrometeorol., 15, 1739–1761, https://doi.org/10.1175/JHM-D-13-0162.1, 2014.
Minderhoud, P. S. J., Erkens, G., Pham, V. H., Bui, T. V., Erban, L. E., Kooi, H., and Stouthamer, E.: Impacts of 25 years of groundwater extraction on subsidence in the Mekong delta, Vietnam, Environ. Res. Lett., 12, 064006, https://doi.org/10.1088/1748-9326/aa7146, 2017.
Minderhoud, P. S. J., Coumou, L., Erkens, G., Middelkoop, H., and Stouthamer, E.: Mekong delta much lower than previously assumed in sea-level rise impact assessments, Nat. Commun., 10, 3847, https://doi.org/10.1038/s41467-019-11602-1, 2019.
Minderhoud, P. S. J., Middelkoop, H., Erkens, G., and Stouthamer, E.: Groundwater extraction may drown mega-delta: projections of extraction-induced subsidence and elevation of the Mekong delta for the 21st century, Environ. Res. Commun., 2, 011005, https://doi.org/10.1088/2515-7620/ab5e21, 2020.
Miralles, D. G., Jiménez, C., Jung, M., Michel, D., Ershadi, A., McCabe, M. F., Hirschi, M., Martens, B., Dolman, A. J., Fisher, J. B., Mu, Q., Seneviratne, S. I., Wood, E. F., and Fernández-Prieto, D.: The WACMOS-ET project – Part 2: Evaluation of global terrestrial evaporation data sets, Hydrol. Earth Syst. Sci., 20, 823–842, https://doi.org/10.5194/hess-20-823-2016, 2016.
Moeck, C., Grech-Cumbo, N., Podgorski, J., Bretzler, A., Gurdak, J. J., Berg, M., and Schirmer, M.: A global-scale dataset of direct natural groundwater recharge rates: A review of variables, processes and relationships, Sci. Total Environ., 717, 137042, https://doi.org/10.1016/j.scitotenv.2020.137042, 2020.
Mohan, C., Western, A. W., Wei, Y., and Saft, M.: Predicting groundwater recharge for varying land cover and climate conditions – a global meta-study, Hydrol. Earth Syst. Sci., 22, 2689–2703, https://doi.org/10.5194/hess-22-2689-2018, 2018.
Montanari, A., Young, G., Savenije, H. H. G., Hughes, D., Wagener, T., Ren, L. L., Koutsoyiannis, D., Cudennec, C., Toth, E., Grimaldi, S., Bloschl, G., Sivapalam, M., Beven, K., Gupta, H., Hipsey, M., Schaefli, B., Arheimer, B., Boegh, E., Schymanski, S. J., Di Baldassarre, G., Yu, B., Hubert, P., Huang, Y., Schumann, A., Post, D. A., Srinivasan, V., Harman, C., Thompson, S., Rogger, M., Viglione, A., McMillan, H., Characklis, G., Pang, Z., and Belyaev, V.: “Panta Rhei—Everything Flows”: Change in hydrology and society–The IAHS Scientific Decade 2013–2022, Hydrolog. Sci. J., 58, 1256–1275, 2013.
Moore, W. S.: The effect of submarine groundwater discharge on the ocean, Annu. Rev. Mar. Sci., 2, 59–88, 2010.
Morris, M. D.: Factorial sampling plans for preliminary computational experiments, Technometrics, 33, 161–174, 1991.
Müller Schmied, H., Eisner, S., Franz, D., Wattenbach, M., Portmann, F. T., Flörke, M., and Döll, P.: Sensitivity of simulated global-scale freshwater fluxes and storages to input data, hydrological model structure, human water use and calibration, Hydrol. Earth Syst. Sci., 18, 3511–3538, https://doi.org/10.5194/hess-18-3511-2014, 2014.
Niu, G.-Y., Yang, Z.-L., Dickinson, R. E., and Gulden, L. E.: A simple TOPMODEL-based runoff parameterization (SIMTOP) for use in global climate models, J. Geophys. Res., 110, D21106, https://doi.org/10.1029/2005JD006111, 2005.
Niu, G.-Y., Yang, Z.-L., Dickinson, R. E., Gulden, L. E., and Su, H.: Development of a simple groundwater model for use in climate models and evaluation with Gravity Recovery and Climate Experiment data, J. Geophys. Res., 112, D07103, https://doi.org/10.1029/2006JD007522, 2007.
Ngo-Duc, T., Laval, K., Ramillien, G., Polcher, J., and Cazenave, A.: Validation of the land water storage simulated by Organising Carbon and Hydrology in Dynamic Ecosystems (ORCHIDEE) with Gravity Recovery and Climate Experiment (GRACE) data, Water Resour. Res., 43, W04427, https://doi.org/10.1029/2006WR004941, 2007.
O'Hagan, A.: Expert Knowledge Elicitation: Subjective but Scientific, Am. Stat., 73, 69–81, https://doi.org/10.1080/00031305.2018.1518265, 2019.
Olarinoye, T., Gleeson, T., Marx, V., Seeger, S., Adinehvand, R., Allocca, V., Andreo, B., Apaestegui, J., Apolit, C., Arfib, B., Auler, A., Bailly-Comte, V., Barbera, J. A., Batiot-Guilhe, C., Bechtel, T., Binet, S., Bittner, D., Blatnik, M., Bolger, T., Brunet, P., Charlier, J., Chen, Z., Chiogna, G., Coxon, G., De Vita, P., Doummar, J., Epting, J., Fleury, P., Fournier, M., Goldscheider, N., Gunn, J., Guo, F., Guyot, J. L., Howden, N., Huggenberger, P., Hunt, B., Jeannin, P., Jiang, G., Jones, G., Jourde H., Karmann, I., Koit, O., Kordilla, J., Labat, D., Ladouch, B., Liso, I. S., Liu, Z., Marechal, J., Massei, N., Mazzilli, N., Mudarra, M., Parise, M., Pu, J., Ravbar, N., Sanchez, L. H., Santo, A., Sauter, M., Seidel, J., Sivelle, V., Skoglund, R. O., Stevanovic, Z., Wood, Cameron., Worthington, S., and Hartmann, A.: Global karst springs hydrograph dataset for research and management of the world's fastest-flowing groundwater, Scientific Data, 7, 59, https://doi.org/10.1038/s41597-019-0346-5, 2020.
Opie, S., Taylor, R. G., Brierley, C. M., Shamsudduha, M., and Cuthbert, M. O.: Climate–groundwater dynamics inferred from GRACE and the role of hydraulic memory, Earth Syst. Dynam., 11, 775–791, https://doi.org/10.5194/esd-11-775-2020, 2020.
Ortega-Guerrero, A., Rudolph, D. L., and Cherry, J. A.: Analysis of long-term land subsidence near Mexico City: field investigations and predictive modeling, Water Resour. Res., 35, 3327–3341, https://doi.org/10.1029/1999WR900148, 1999.
Pappenberger, F., Ghelli, A., Buizza, R., and Bódis, K.: The Skill of Probabilistic Precipitation Forecasts under Observational Uncertainties within the Generalized Likelihood Uncertainty Estimation Framework for Hydrological Applications, J. Hydrometeorol., 10, 807–819, https://doi.org/10.1175/2008JHM956.1, 2009.
Perrone, D. and Jasechko, S.: Deeper well drilling an unsustainable stopgap to groundwater depletion, Nature Sustainability, 2, 773–782, 2019.
Pianosi, F., Beven, K., Freer, J., Hall, J. W., Rougier, J., Stephenson, D. B., and Wagener, T.: Sensitivity analysis of environmental models: A systematic review with practical workflow, Environ. Modell. Softw., 79, 214–232, 2016.
Post, V. E. and von Asmuth, J. R.: Hydraulic head measurements–new technologies, classic pitfalls, Hydrogeol. J., 21, 737–750, 2013.
Qiu, J. Q., Zipper, S. C., Motew, M., Booth, E. G., Kucharik, C. J., and Loheide, S. P.: Nonlinear groundwater influence on biophysical indicators of ecosystem services, Nature Sustainability, 2, 475–483, https://doi.org/10.1038/s41893-019-0278-2, 2019.
Rahman, A. S. M. M., Sulis, M., and Kollet, S. J.: The subsurface–land surface–atmosphere connection under convective conditions, Adv. Water Resour., 83, 240–249, https://doi.org/10.1016/j.advwatres.2015.06.003, 2015.
Rajabi, M. M. and Ataie-Ashtiani, B.: Efficient fuzzy Bayesian inference algorithms for incorporating expert knowledge in parameter estimation, J. Hydrol., 536, 255–272, 2016.
Rajabi, M. M., Ataie-Ashtiani, B., and Simmons, C. T.: Model-data interaction in groundwater studies: Review of methods, applications and future directions, J. Hydrol., 567, 457–477, 2018.
Refsgaard, J. C., van der Sluijs, J. P., Højberg, A. L., and Vanrolleghem, P. A.: Uncertainty in the environmental modelling process–a framework and guidance, Environ. Modell. Softw., 22, 1543–1556, 2007.
Reichstein, M., Camps-Valls, G., Stevens, B., Jung, M., Denzler, J., Carvalhais, N., and Prabhat: Deep learning and process understanding for data-driven Earth system science, Nature, 566, 195–204, 2019.
Reinecke, R., Foglia, L., Mehl, S., Trautmann, T., Cáceres, D., and Döll, P.: Challenges in developing a global gradient-based groundwater model (G3M v1.0) for the integration into a global hydrological model, Geosci. Model Dev., 12, 2401–2418, https://doi.org/10.5194/gmd-12-2401-2019, 2019a.
Reinecke, R., Foglia, L., Mehl, S., Herman, J. D., Wachholz, A., Trautmann, T., and Döll, P.: Spatially distributed sensitivity of simulated global groundwater heads and flows to hydraulic conductivity, groundwater recharge, and surface water body parameterization, Hydrol. Earth Syst. Sci., 23, 4561–4582, https://doi.org/10.5194/hess-23-4561-2019, 2019b.
Reinecke, R., Wachholz, A., Mehl, S., Foglia, L., Niemann, C., and Döll, P.: Importance of spatial resolution in global groundwater modeling, Groundwater, 58, 363–376, https://doi.org/10.1111/gwat.12996, 2020.
Rodell, M., Velicogna, I., and Famiglietti, J. S.: Satellite-based estimates of groundwater depletion in India, Nature, 460, 999–1002, 2009.
Ross, J. L., Ozbek, M. M., and Pinder, G. F.: Aleatoric and epistemic uncertainty in groundwater flow and transport simulation, Water Resour. Res., 45, W00B15, https://doi.org/10.1029/2007WR006799, 2009.
Rossman, N. and Zlotnik, V.: Review: Regional groundwater flow modeling in heavily irrigated basins of selected states in the western United States, Hydrogeol. J., 21, 1173–1192, https://doi.org/10.1007/s10040-013-1010-3, 2013.
RRCA (Republican River Compact Administration Ground Water Model): http://www.republicanrivercompact.org/ (last access: 15 November 2021), 2003.
Saltelli, A., Tarantola, S., Campolongo, F., and Ratto, M.: Sensitivity Analysis in Practice: A Guide to Assessing Scientific Models, Wiley, USA, ISBN: 978-0-470-87093-8, 2004.
Salvucci, G. D. and Entekhabi, D.: Hillslope and climatic controls on hydrologic fluxes, Water Resour. Res., 31, 1725–1739, 1995.
Sanford, W., Calibration of models using groundwater age, Hydrogeol. J., 19, 13–16, https://doi.org/10.1007/s10040-010-0637-6, 2011.
Sawyer, A. H., David, C. H., and Famiglietti, J. S.: Continental patterns of submarine groundwater discharge reveal coastal vulnerabilities, Science, 353, 705–707, 2016.
Scanlon, B., Healy, R., and Cook, P.: Choosing appropriate techniques for quantifying groundwater recharge, Hydrogeol. J., 10, 18–39, 2002.
Scanlon, B. R., Faunt, C. C., Longuevergne, L., Reedy, R. C., Alley, W. M., McGuire, V. L., and McMahon, P. B.: Groundwater depletion and sustainability of irrigation in the US High Plains and Central Valley, P. Natl. Acad. Sci. USA, 109, 9320–9325, https://doi.org/10.1073/pnas.1200311109, 2012.
Scanlon, B. R., Zhang, Z., Save, H., Wiese, D. N., Landerer, F. W., Long, D., Longuevergne, L., and Chen, J.: Global evaluation of new GRACE mascon products for hydrologic applications, Water Resour. Res., 52, 9412–9429, 2016.
Schaller, M. and Fan, Y.: River basins as groundwater exporters and importers: Implications for water cycle and climate modeling, J. Geophys. Res., 114, D04103, https://doi.org/10.1029/2008JD010636, 2009.
Schewe, J., Heinke, J., Gerten, D., Haddeland, I., Arnell, N. W., Clark, D. B., Dankers, R., Eisner, S., Fekete, B. M., Colon-Gonzalez, F. J., Gosling, S. N., Kim, H., Liu, X., Masaki, Y., Portmann, F. T., Satoh, Y., Stacke, T., Tang, Q., Wada, Y., Wisser, D., Albrecht, T., Frieler, K., Piontek, F., Warszawski, L., and Kabat, P.: Multimodel assessment of water scarcity under climate change, P. Natl. Acad. Sci. USA, 111, 3245–3250, https://doi.org/10.1073/pnas.1222460110, 2014.
Schilling, O. S., Doherty, J., Kinzelbach, W., Wang, H., Yang, P. N., and Brunner, P.: Using tree ring data as a proxy for transpiration to reduce predictive uncertainty of a model simulating groundwater–surface water–vegetation interactions, J. Hydrol., 519, 2258–2271, https://doi.org/10.1016/j.jhydrol.2014.08.063, 2014.
Schilling, O. S., Cook, P. G., and Brunner, P.: Beyond classical observations in hydrogeology: The advantages of including exchange flux, temperature, tracer concentration, residence time, and soil moisture observations in groundwater model calibration, Rev. Geophys., 57, 146–182, 2019.
Schneider, A. S., Jost, A., Coulon, C., Silvestre, M., Théry, S., and Ducharne, A.: Global scale river network extraction based on high-resolution topography, constrained by lithology, climate, slope, and observed drainage density, Geophys. Res. Lett., 44, 2773–2781, https://doi.org/10.1002/2016GL071844, 2017.
Shen, C.: A transdisciplinary review of deep learning research and its relevance for water resources scientists, Water Resour. Res., 54, 8558–8593, 2018.
Shen, C., Laloy, E., Elshorbagy, A., Albert, A., Bales, J., Chang, F.-J., Ganguly, S., Hsu, K.-L., Kifer, D., Fang, Z., Fang, K., Li, D., Li, X., and Tsai, W.-P.: HESS Opinions: Incubating deep-learning-powered hydrologic science advances as a community, Hydrol. Earth Syst. Sci., 22, 5639–5656, https://doi.org/10.5194/hess-22-5639-2018, 2018.
Sivapalan, M., Konar, M., Srinivasan, V., Chhatre, A., Wutich, A., Scott, C. A., Wescoat, J. L., and Rodríguez-Iturbe, I.: Socio-hydrology: Use-inspired water sustainability science for the Anthropocene, Earth’s Future, 2, 225–230, https://doi.org/10.1002/2013EF000164, 2014.
SKI. Intracoin – International Nuclide Transport Code Intercomparison Study (No. SKI–84-3), Swedish Nuclear Power Inspectorate, available at: https://inis.iaea.org/search/search.aspx?orig_q=RN:16046803 (last access: 16 November 2021), 1984.
Springer, A. and Stevens, L.: Spheres of discharge of springs, Hydrogeol. J., 17, 83–93, https://doi.org/10.1007/s10040-008-0341-y, 2009.
Steffen, W., Broadgate, W., Deutsch, L., Gaffney, O., and Ludwig, C.: The trajectory of the Anthropocene: the great acceleration, The Anthropocene Review, 2, 81–98, 2015.
Takata, K., Emori, S., and Watanabe, T.: Development of the minimal advanced treatments of surface interaction and runoff, Global Planet. Change, 38, 209–222, https://doi.org/10.1016/S0921-8181(03)00030-4, 2003.
Taylor, R. G., Scanlon, B., Doll, P., Rodell, M., van Beek, R., Wada, Y., Longuevergne, L., Leblanc, M., Famiglietti, J. S., Edmunds, M., Konikow, L., Green, T. R., Chen, J., Taniguchi, M., Bierkens, M. F. P., MacDonald, A., Fan, Y., Maxwell, R. M., Yechieli, Y., Gurdak, J. J., Allen, D. M., Shamsudduha, M., Hiscock, K., Yeh, P. J. -F., Holman, I., and Treidel, H.: Groundwater and climate change, Nat. Clim. Change, 3, 322–329, https://doi.org/10.1038/nclimate1744, 2013a.
Taylor, R. G., Todd, M. C., Kongola, L., Maurice, L., Nahozya, E., Sanga, H., and MacDonald, A. M.: Evidence of the dependence of groundwater resources on extreme rainfall in East Africa, Nat. Clim. Change, 3, 374–378, https://doi.org/10.1038/nclimate1731, 2013b.
Thatch, L. M., Gilbert, J. M., and Maxwell, R. M.: Integrated hydrologic modeling to untangle the impacts of water management during drought, Groundwater, 58, 377–391, 2020.
Tolley, D., Foglia, L., and Harter, T.: Sensitivity Analysis and Calibration of an Integrated Hydrologic Model in an Irrigated Agricultural Basin with a Groundwater-Dependent Ecosystem, Water Resour. Res., 55, 7876–7901, https://doi.org/10.1029/2018WR024209, 2019.
Tran, H., Zhang, J., Cohard, J.-M., Condon, L. E., and Maxwell, R. M.: Simulating g roundwater-Streamflow Connections in the Upper Colorado River Basin, Groundwater, 58, 392–405, https://doi.org/10.1111/gwat.13000, 2020.
Tregoning, P., McClusky, S., van Dijk, A. I. J. M., and Crosbie, R. S.: Assessment of GRACE satellites for groundwater estimation in Australia, National Water Commission, Canberra, Waterlines Report Series No 71, 2012.
Troldborg, L., Refsgaard, J. C., Jensen, K. H., and Engesgaard, P.: The importance of alternative conceptual models for simulation of concentrations in a multi-aquifer system, Hydrogeol. J., 15, 843–860, 2007.
Tustison, B., Harris, D., and Foufoula-Georgiou, E.: Scale issues in verification of precipitation forecasts, J. Geophys. Res., 106, 11775–11784, 2001.
UNESCO: World water balance and water resources of the earth, Vol. USSR committee for the international hydrologic decade, UNESCO, Paris, 1978.
Van Vliet, M. T., Flörke, M., Harrison, J. A., Hofstra, N., Keller, V., Ludwig, F., Spanier, J. E., Strokal, M., Wada, Y., Wem, Y., and Williams, R. J.: Model inter-comparison design for large-scale water quality models, Curr. Opin. Env. Sust., 36, 59–67, https://doi.org/10.1016/j.cosust.2018.10.013, 2019.
Van Werkhoven, K., Wagener, T., Tang, Y., and Reed, P.: Understanding watershed model behavior across hydro-climatic gradients using global sensitivity analysis, Water Resour. Res., 44, W01429, https://doi.org/10.1029/2007WR006271, 2008.
Van Loon, A. F., Gleeson, T., Clark, J., Van Dijk, A. I. J. M., Stahl, K., Hannaford, J., Di Baldassarre, G., Teuling, A. J., Tallaksen, L. M., Uijlenhoet, R., Hannah, D. M., Sheffield, J., Svoboda, M., Verbeiren, B., Wagener, T., Rangecroft, S., Wanders, N., and Van Lanen, H. A. J.: Drought in the Anthropocene, Nat. Geosci., 9, 89–91, https://doi.org/10.1038/ngeo2646, 2016.
Van Loon, A. F., Kumar, R., and Mishra, V.: Testing the use of standardised indices and GRACE satellite data to estimate the European 2015 groundwater drought in near-real time, Hydrol. Earth Syst. Sci., 21, 1947–1971, https://doi.org/10.5194/hess-21-1947-2017, 2017.
Vergnes, J.-P. and Decharme, B.: A simple groundwater scheme in the TRIP river routing model: global off-line evaluation against GRACE terrestrial water storage estimates and observed river discharges, Hydrol. Earth Syst. Sci., 16, 3889–3908, https://doi.org/10.5194/hess-16-3889-2012, 2012.
Vergnes, J.-P., Decharme, B., and Habets, F.: Introduction of groundwater capillary rises using subgrid spatial variability of topography into the ISBA land surface model, J. Geophys. Res.-Atmos., 119, 11065–11086, https://doi.org/10.1002/2014JD021573, 2014.
Vergnes, J.-P., Roux, N., Habets, F., Ackerer, P., Amraoui, N., Besson, F., Caballero, Y., Courtois, Q., de Dreuzy, J.-R., Etchevers, P., Gallois, N., Leroux, D. J., Longuevergne, L., Le Moigne, P., Morel, T., Munier, S., Regimbeau, F., Thiéry, D., and Viennot, P.: The AquiFR hydrometeorological modelling platform as a tool for improving groundwater resource monitoring over France: evaluation over a 60-year period, Hydrol. Earth Syst. Sci., 24, 633–654, https://doi.org/10.5194/hess-24-633-2020, 2020.
Visser, W. C.: Crop growth and availability of moisture, J. Sci. Food Agr., 10, 1–11, 1959.
Wada, Y., van Beek, L. P. H., van Kempen, C. M., Reckman, J. W. T. M., Vasak, S., and Bierkens, M. F. P.: Global depletion of groundwater resources. Geophys. Res. Lett. 37, L20402, https://doi.org/10.1029/2010GL044571, 2010.
Wada, Y., Wisser, D., and Bierkens, M. F. P.: Global modeling of withdrawal, allocation and consumptive use of surface water and groundwater resources, Earth Syst. Dynam., 5, 15–40, https://doi.org/10.5194/esd-5-15-2014, 2014.
Wada, Y.: Modeling Groundwater Depletion at Regional and Global Scales: Present State and Future Prospects, Surv. Geophys., 37, 419–451, https://doi.org/10.1007/s10712-015-9347-x, 2016.
Wada, Y. and Bierkens, M. F. P.: Sustainability of global water use: past reconstruction and future projections, Environ. Res. Lett., 9, 104003, https://doi.org/10.1088/1748-9326/9/10/104003, 2014.
Wada, Y. and Heinrich, L.: Assessment of transboundary aquifers of the world–vulnerability arising from human water use, Environ. Res. Lett., 8, 024003, https://doi.org/10.1088/1748-9326/8/2/024003, 2013.
Wagener, T. and Gupta, H. V.: Model identification for hydrological forecasting under uncertainty, Stoch. Env. Res. Risk A., 19, 378–387, 2005.
Wagener, T., Sivapalan, M., Troch, P., and Woods, R.: Catchment classification and hydrologic similarity, Geography Compass, 1, 901–931, https://doi.org/10.1111/j.1749-8198.2007.00039.x, 2007.
Wagener, T. and Pianosi, F.: What has Global Sensitivity Analysis ever done for us? A systematic review to support scientific advancement and to inform policy-making in earth system modelling, Earth-Sci. Rev., 194, 1–18, https://doi.org/10.1016/j.earscirev.2019.04.006, 2019.
Wagener, T., Boyle, D. P., Lees, M. J., Wheater, H. S., Gupta, H. V., and Sorooshian, S.: A framework for development and application of hydrological models, Hydrol. Earth Syst. Sci., 5, 13–26, https://doi.org/10.5194/hess-5-13-2001, 2001.
Wagener, T., Sivapalan, M., Troch, P. A., McGlynn, B. L., Harman, C. J., Gupta, H. V., Kumar, P., Rao, C. S., Basu, N. B., and Wilson, J. S.: The future of hydrology: An evolving science for a changing world, Water Resour. Res., 46, W05301, https://doi.org/10.1029/2009WR008906, 2010.
Wagener, T., Gleeson, T., Coxon, G., Hartmann, A., Howden, N., Pianosi, F., Rahman, M., Rosolem, R., Stein, L., and Woods, R.: On doing hydrology with dragons: Realizing the value of perceptual models and knowledge accumulation, Wiley Interdisciplinary Reviews: Water, 8, e1550, https://doi.org/10.1002/wat2.1550, 2021.
Wang, F., Ducharne, A., Cheruy, F., Lo, M. H., and Grandpeix, J. L.: Impact of a shallow groundwater table on the global water cycle in the IPSL land-atmosphere coupled model, Clim. Dynam., 50, 3505–3522, https://doi.org/10.1007/s00382-017-3820-9, 2018.
Warszawski, L., Frieler, K., Huber, V., Piontek, F., Serdeczny, O., and Schewe, J.: The Inter-Sectoral Impact Model Intercomparison Project (ISI–MIP): Project framework, P. Natl. Acad. Sci. USA, 111, 3228–3232, https://doi.org/10.1073/pnas.1312330110, 2014.
Weiland, F. C. S., Vrugt, J. A., van Beek, R. (L.) P. H., Weerts, A. H., and Bierkens, M. F. P.: Significant uncertainty in global scale hydrological modeling from precipitation data errors, J. Hydrol., 529, 1095–1115, https://doi.org/10.1016/j.jhydrol.2015.08.061, 2015.
Winter, T. C., Harvey, J. W., Franke, O. L., and Alley, W. M.: Ground water and surface water: a single resource, U.S. Geological Survey, Circular, 1139, 79 pp., 1998.
Woolfenden, L. R. and Nishikawa, T.: Simulation of groundwater and surface-water resources of the Santa Rosa Plain watershed, Sonoma County, California, USGS Scientific Investigations Report 2014–5052, U.S. Geological Survey, Reston, VA, 2014.
Yang, J., Griffiths, J., and Zammit, C.: National classification of surface–groundwater interaction using random forest machine learning technique, River Res. Appl., 35, 932–943, https://doi.org/10.1002/rra.3449, 2019.
Yilmaz, K., Gupta, H. V., and Wagener, T.: Towards improved distributed modeling of watersheds: A process based diagnostic approach to model evaluation, Water Resour. Res., 44, W09417, https://doi.org/10.1029/2007WR006716, 2009.
Zaherpour, J., Gosling, S. N., Mount, N., Schmied, H. M., Veldkamp, T. I. E., Dankers, R., Eisner, S., Gerten, D., Gudmundsson, L., Haddeland, I., Hanasaki, N., Kim, H., Leng, G., Liu, J., Masaki, Y., Oki, T., Pokhrel, Y., Satoh, Y., Schewe, J., and Wada, Y.: Worldwide evaluation of mean and extreme runoff from six global-scale hydrological models that account for human impacts, Environ. Res. Lett., 13, 065015, https://doi.org/10.1088/1748-9326/aac547, 2018.
Zell, W. O. and Sanford, W. E.: Calibrated Simulation of the Long-Term Average Surficial Groundwater System and Derived Spatial Distributions of its Characteristics for the Contiguous United States, Water Resour. Res., 56, e2019WR026724, https://doi.org/10.1029/2019WR026724, 2020.
Zipper, S. C., Soylu, M. E., Booth, E. G., and Loheide, S. P.: Untangling the effects of shallow groundwater and soil texture as drivers of subfield-scale yield variability, Water Resour. Res., 51, 6338–6358, 2015.
Zipper, S. C., Soylu, M. E., Kucharik, C. J., and Loheide, S. P.: Quantifying indirect groundwater-mediated effects of urbanization on agroecosystem productivity using MODFLOW-AgroIBIS (MAGI), a complete critical zone model, Ecol. Model., 359, 201–219, 2017.
Zhang, M. and Burbey, T. J.: Inverse modelling using PS-InSAR data for improved land subsidence simulation in Las Vegas Valley, Nevada, Hydrol. Process., 30, 4494–516, 2016.
Zhou, Y. and Li, W.: A review of regional groundwater flow modeling, Geosci. Front., 2, 205–214, 2011.
- Abstract
- Introduction: why and how is groundwater modeled at continental to global scales?
- Current model evaluation practices
- How to improve the evaluation of large-scale groundwater models
- Conclusions: towards a holistic evaluation of groundwater representation in large-scale models
- Code and data availability
- Author contributions
- Competing interests
- Disclaimer
- Acknowledgements
- Review statement
- References
hiddenunderground and thus hard to measure. We suggest using multiple complementary strategies to assess the performance of a model (
model evaluation).
- Abstract
- Introduction: why and how is groundwater modeled at continental to global scales?
- Current model evaluation practices
- How to improve the evaluation of large-scale groundwater models
- Conclusions: towards a holistic evaluation of groundwater representation in large-scale models
- Code and data availability
- Author contributions
- Competing interests
- Disclaimer
- Acknowledgements
- Review statement
- References