the Creative Commons Attribution 4.0 License.
the Creative Commons Attribution 4.0 License.
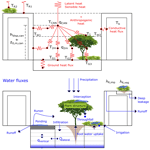
An urban ecohydrological model to quantify the effect of vegetation on urban climate and hydrology (UT&C v1.0)
Gabriele Manoli
Paolo Burlando
Elie Bou-Zeid
Winston T. L. Chow
Andrew M. Coutts
Edoardo Daly
Kerry A. Nice
Matthias Roth
Nigel J. Tapper
Erik Velasco
Enrique R. Vivoni
Simone Fatichi
Increasing urbanization is likely to intensify the urban heat island effect, decrease outdoor thermal comfort, and enhance runoff generation in cities. Urban green spaces are often proposed as a mitigation strategy to counteract these adverse effects, and many recent developments of urban climate models focus on the inclusion of green and blue infrastructure to inform urban planning. However, many models still lack the ability to account for different plant types and oversimplify the interactions between the built environment, vegetation, and hydrology. In this study, we present an urban ecohydrological model, Urban Tethys-Chloris (UT&C), that combines principles of ecosystem modelling with an urban canopy scheme accounting for the biophysical and ecophysiological characteristics of roof vegetation, ground vegetation, and urban trees. UT&C is a fully coupled energy and water balance model that calculates 2 m air temperature, 2 m humidity, and surface temperatures based on the infinite urban canyon approach. It further calculates the urban hydrological fluxes in the absence of snow, including transpiration as a function of plant photosynthesis. Hence, UT&C accounts for the effects of different plant types on the urban climate and hydrology, as well as the effects of the urban environment on plant well-being and performance. UT&C performs well when compared against energy flux measurements of eddy-covariance towers located in three cities in different climates (Singapore, Melbourne, and Phoenix). A sensitivity analysis, performed as a proof of concept for the city of Singapore, shows a mean decrease in 2 m air temperature of 1.1 ∘C for fully grass-covered ground, 0.2 ∘C for high values of leaf area index (LAI), and 0.3 ∘C for high values of Vc,max (an expression of photosynthetic capacity). These reductions in temperature were combined with a simultaneous increase in relative humidity by 6.5 %, 2.1 %, and 1.6 %, for fully grass-covered ground, high values of LAI, and high values of Vc,max, respectively. Furthermore, the increase of pervious vegetated ground is able to significantly reduce surface runoff.
- Article
(2281 KB) -
Supplement
(2364 KB) - BibTeX
- EndNote
More than 50 % of the world's population currently lives in cities, with a predicted increase in all regions of the world (United Nations, 2014). This growing urban population, together with the projected rise in global temperature and associated higher frequency of heat waves (IPCC, 2014), is likely to exacerbate the urban heat island (UHI) effect (Li and Bou-Zeid, 2013), which can have adverse effects on outdoor thermal comfort (Mitchell et al., 2016; Mora et al., 2017), energy demand of cooling systems (Hadley et al., 2006), and urban ecology (Zhang et al., 2004; Jochner et al., 2013). At the same time, urban expansion increases impervious surface area and can enhance heavy rainfall events (Holst et al., 2016). These modifications intensify surface runoff that needs to be counteracted with greater investments in storm water sewer systems to avoid urban flooding and damage to infrastructure and valuable properties. Hence, the negative externalities of urbanization need to be addressed and proper mitigation strategies analysed.
Nature-based solutions, such as the increase of urban vegetation, are often encouraged to mitigate UHI and decrease surface runoff as part of a sustainable urban development (Lim and Lu, 2016; Roth, 2007; Bowler et al., 2010; Pataki et al., 2011; Li et al., 2014; Gillner et al., 2015). For instance, urban trees provide shade for pedestrians and evaporative cooling (Bowler et al., 2010; Konarska et al., 2016), while an increase in ground vegetation can further provide storm water retention (Berland et al., 2017). In addition to urban climate and water regulation, urban vegetation also provides other ecosystem services, for example, carbon storage (Nowak and Crane, 2002), enhanced biodiversity (Grimm et al., 2008), and aesthetic, cultural, and health benefits (Salmond et al., 2016; Ng et al., 2018). Therefore, many policy-makers promote an increase of urban vegetation (Lim and Lu, 2016).
In this context, innovative numerical approaches are needed, given the complexity of the problem, to quantify the influence of green infrastructure on climate and water fluxes in cities and to provide guidelines for urban planners. A suitable modelling tool should resolve air temperature and humidity at the pedestrian level, surface temperatures (including mean radiant temperature), and wind speed to predict outdoor thermal comfort (OTC) (e.g. Höppe, 1999; Golasi et al., 2018). Furthermore, canopy interception and subsurface hydrology need to be included to assess surface runoff and account for potential water stress of urban vegetation. Plant biophysical and ecophysiological characteristics are also important to accurately predict the effects of plant evapotranspiration and shading on the urban climate and hydrological cycle, as well as to evaluate climatic feedback on the well-being of plants and their ability to continue performing the aforementioned ecosystem services.
In recent years, a number of urban climate models started to consider the influence of vegetation on urban micrometeorology and hydrology. On the one hand, some models focus on the detailed representation of a particular process, for example, solar irradiation (e.g. SOLWEIG: Lindberg et al.; 2008; RayMan: Matzarakis et al., 2007, 2010). Methods typical of computational fluid dynamics (CFD) have been used to predict wind patterns and profiles in the urban environment (e.g. OpenFoam: Allegrini and Carmeliet, 2017, Manickathan et al., 2018; ENVI-met: Bruse and Fleer, 1998), but they usually neglect or simplify other components of the urban energy and water balance. On the other hand, mesoscale meteorological models, for example, the Weather Research and Forecasting model (WRF) (Skamarock et al., 2008), provide a description of the large-scale meteorological conditions and, when coupled with urban canopy models, can give feedback effects between mitigation strategies and urban climate, as well as quantify the impact at different scales of the implementation. Urban canopy models solve energy and water balances and have been improved in recent years to include short ground vegetation (TEB-Veg: Lemonsu et al., 2012; PUCM: Wang et al., 2013), trees (VUCM: Park and Lee, 2008; TEB-Veg: Redon et al., 2017; PUCM: Ryu et al., 2016; BEP-Tree: Krayenhoff et al., 2014, 2015), and more detailed representations of subsurface hydrology (TEB-Hydro: Stavropulos-Laffaille et al., 2018). Further advancements allow distinguishing between deciduous and evergreen shrubs and trees (SUEWS: Ward et al., 2016), irrigated and non-irrigated vegetation (TARGET: Broadbent et al., 2018a), and plant types (VTUF-3D: Nice et al., 2018). While these studies represent significant advancements in urban geoscience, some of them still present limitations, for example, neglecting the effects of precipitation (e.g. Broadbent et al., 2018a) or the inability to model canopy level humidity (e.g. Nice et al., 2018). Hence, while a number of urban canopy models accounting for vegetation exist, the majority of them still have a simplistic or empirical representation of plant physiological processes, and thus transpiration, or entirely neglect components of the hydrological cycle.
In this study, we combine components of the ecohydrological model Tethys-Chloris (T&C) (Fatichi et al., 2012a, b) with components of urban canopy modelling, such as the tree-shading scheme of the Princeton Urban Canopy Model (Wang et al., 2013; Ryu et al., 2016), to develop the urban ecohydrological model Urban Tethys-Chloris (UT&C). UT&C accounts for detailed plant biophysical and ecophysiological characteristics and models transpiration as a function of environmental conditions (e.g. soil moisture, photosynthetic active radiation, vapour pressure deficit) and plant physiological traits. Interception on plant canopy and ponding on impervious and soil surfaces, as well as urban subsurface hydrology, are accounted for. UT&C is able to simulate the influence of different configurations of green spaces (green roofs, street trees, ground vegetation), vegetation types, and plant species on the urban climate and hydrology. It is a fully coupled energy and water balance model that calculates 2 m air temperature, 2 m humidity, and skin temperatures of urban surfaces.
In this article and its technical reference material (TRM), we (1) introduce UT&C and provide a detailed technical description; (2) show an evaluation of the model performance in three cities with distinctive climates – Singapore, Melbourne (Australia), and Phoenix (USA); and (3) provide proofs of concept of the model capability with a sensitivity analysis to urban vegetation cover, and plant biophysical (leaf area index, LAI) and ecophysiological (maximum RuBisCO capacity, Vc,max) parameters.
UT&C is based on the infinite urban canyon approximation (Masson, 2000; Kusaka et al., 2001). The urban geometry is specified with a canyon height (HCanyon), canyon width (WCanyon), and roof width (WRoof) (Fig. 1). Street directions are explicitly accounted for, resulting in one (partially) sunlit and one shaded wall (Wang et al., 2013). The ground is partitioned into impervious (λG,imp), bare soil (λG,bare), and vegetated (λG,veg) ground fractions, whereas the roof is partitioned into impervious (λR,imp) and vegetated (λR,veg) roof fractions (Wang et al., 2013). If trees are present in the urban environment, they are represented by two infinite rows of street trees described by their height (HT), canopy radius (RT), and distance to the nearest wall (dT), as developed by Ryu et al. (2016).
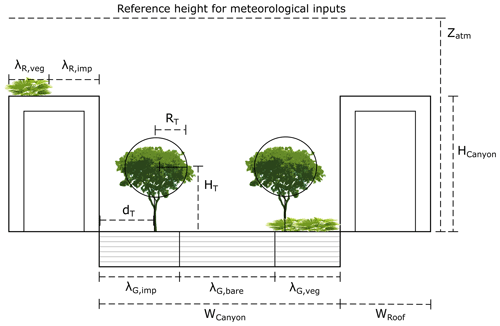
Figure 1Geometric set-up of UT&C. Zatm is the reference height for meteorological input data, HCanyon the mean building height, WCanyon the mean width of the urban canyon, and WRoof the mean roof width. The ground is partitioned into impervious (λG,imp), bare (λG,bare), and vegetated (λG,veg) fractions. The roof is partitioned into impervious (λR,imp) and vegetated (λR,veg) fractions. The location and size of urban trees is specified by the tree height (HT), tree radius (RT), and tree distance to wall (dT).
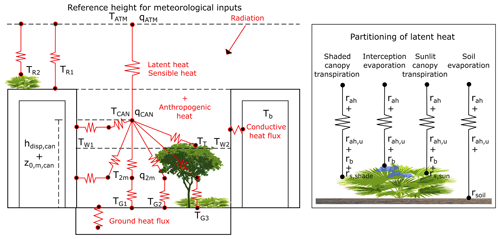
Figure 2Modelled energy fluxes in UT&C. TR,i, TW,i, TG,i, and TT are the roof, wall, ground, and tree temperatures, which are calculated solving the individual surface energy balances. The canyon air is subdivided into two layers, and air temperature and humidity are calculated at 2 m height (T2 m, q2 m) and at the canyon reference height (Tcan, qcan), which is equal to the sum of zero-plane displacement height (hdisp,can) and momentum roughness length (z0,m,can) of the canyon. Tatm and qatm are the air temperature and humidity at the reference height for meteorological inputs, and Tb is the prescribed interior building temperature. The graph on the right shows the resistances applied to calculate shaded and sunlit canopy transpiration, evaporation from interception, and soil evaporation within the urban canyon. rs,shade is the stomatal resistance of shaded vegetation canopy, rs,sun the stomatal resistance of sunlit vegetation canopy, rb the leaf boundary resistance, rsoil the soil resistance, rah,u the vertical aerodynamic resistance within the canyon, and rah the aerodynamic resistance above the urban canyon.
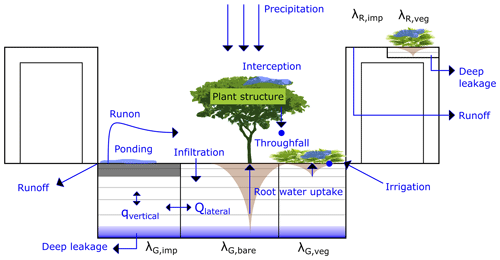
Figure 3Modelled water fluxes in UT&C. The urban soil is subdivided into three different soil columns according to the impervious (λG,imp), bare (λG,bare), and vegetated (λG,veg) ground fractions. Vertical (qvertical) and lateral (Qlateral) soil water fluxes are calculated. Runoff occurs when the maximum ponding storage capacity is exceeded. An user-specified fraction of runoff can be kept in the system as runon.
UT&C solves the energy and water budget (Figs. 2 and 3) to calculate surface temperatures of sunlit and shaded wall, tree, ground, and roof fractions. The canyon air space is subdivided into two layers. The canyon air temperature and humidity are calculated at 2 m canyon height and at canyon reference height, which is the sum of the zero-plane displacement height of the canyon and canyon roughness length (hdisp,can+z0,m,can; Fig. 2). The urban energy budget for the whole atmospheric layer and the water budget are
where Rn is the net all-wave radiation, Qf the anthropogenic heat input, H the sensible heat flux, λE the evapotranspiration E (kg m−2 s−1) multiplied by the latent heat of vaporization λ (J kg−1), G the conductive heat flux which includes the heat storage effect of the urban fabric, P the precipitation, Ir the anthropogenic water input (irrigation), R the surface runoff, Lk the deep leakage at the bottom of the soil column that can be regarded as a recharge term to groundwater, and ΔS the change in water storage both on the surface and in the soil. The heat storage within the canyon air is not included in the current version of the model. The evaporation from wall surfaces is assumed negligible. Input data used by UT&C are observed meteorological time series of air temperature, humidity, air pressure, incoming shortwave and longwave radiation, precipitation, and wind speed at a user-specified reference height above the urban canyon, and it is therefore run offline but could potentially be coupled to mesoscale meteorological models in the future. The model runs at hourly or sub-hourly time steps and the computational speed is approximately 500 ms per time step resulting in a simulation time of one grid cell model set-up of roughly 1 h for 1 year of data (hourly time step) on a commercial laptop (Intel Core i7-6820HQ 2.7 GHz, 16 GB RAM).
2.1 Energy budget
2.1.1 Radiative transfer
The net all-wave radiation Rn, typically referred to simply as net radiation, is the sum of net shortwave and net longwave radiation:
where S↓ is the incoming and S↑ the reflected shortwave radiation, L↓ the incoming longwave radiation, and L↑ the emitted and reflected longwave radiation. The incoming shortwave radiation is partitioned into direct beam and diffuse radiation using a weather generator (Fatichi et al., 2011), and the absorbed shortwave radiation of surface i, Sn,i, is a function of its albedo:
where αi is the albedo of surface i, and and are the direct and diffuse incoming shortwave radiation to surface i. The amount of direct shortwave radiation received by each urban surface is calculated considering shade according to established methodologies (Masson, 2000; Kusaka et al., 2001; Wang et al., 2013) if trees are absent or according to Ryu et al. (2016) if trees are present. The diffuse shortwave radiation received from the sky on each surface is calculated with the respective sky-view factor. It is assumed that all surfaces are Lambertian with diffuse and isotropic scattering and that the different ground cover fractions are homogeneously distributed over the ground area. Following these assumptions, infinite reflections of shortwave radiation are calculated within the urban canyon with the use of view factors (Sparrow and Cess, 1970; Harman et al., 2003; Wang, 2010, 2014). The air within the canyon does not interact in the radiative exchange; for example, the effect of airborne aerosols is neglected (Wang, 2014).
The absorbed longwave radiation of each surface i is calculated as
where ϵi is the emissivity and (1−ϵi) the reflectivity of a surface for longwave radiation, L↓i the incoming longwave radiation, (W m−2 K−4) is the Stefan–Boltzmann constant, and Ti (K) the surface temperature. The incoming longwave radiation L↓i is calculated as a function of the emitted longwave radiation by the atmosphere and the surrounding surfaces. As with shortwave radiation, infinite reflections of longwave radiation within the urban canyon are calculated with the use of reciprocal view factors (Harman et al., 2003). The view factors are calculated with analytically derived equations for an urban canyon without trees (Sparrow and Cess, 1970; Masson, 2000; Harman et al., 2003; Park and Lee, 2008; Wang et al., 2013). If trees are present, the view factors are calculated with a simplified two-dimensional Monte Carlo ray-tracing algorithm developed and included in the UT&C code similar to the algorithms described by Wang (2014) and Frank et al. (2016). The Monte Carlo ray-tracing view factors are corrected for reciprocity to guarantee energy conservation.
The detailed description of shortwave and longwave radiation, view factor, and Monte Carlo ray-tracing calculations are described in Sect. 1 of the TRM.
2.1.2 Turbulent energy fluxes
The total sensible and latent heat fluxes are calculated as the area-weighted average flux of roof and canyon area. The turbulent transport of sensible and latent heat is calculated according to a resistance parameterization (Shuttleworth, 2012) as
where ρa (kg m−3) is the air density, Cp (J kg−1 K−1) the specific heat capacity of air at constant pressure, Ti (K) the temperature of surface i, Ta (K) the air temperature, (–) the saturated specific humidity of surface i, qa (–) the specific humidity of the air, and ∑rj (s m−1) the sum of resistances j to the turbulent transport of sensible and latent heat. UT&C accounts for vertical aerodynamic resistance above and within the urban canyon, horizontal aerodynamic resistance within the urban canyon, leaf boundary layer resistance, stomatal resistance of sunlit and shaded leaves, and soil resistance (Fig. 2). The vertical wind speed profile is assumed logarithmic above the urban canopy, exponential within the canyon, and logarithmic again close to the canyon ground (Masson, 2000; Mahat et al., 2013). Zero-plane displacement height, hdisp,can, and momentum roughness length, z0,m,can, of the urban canopy are calculated according to the formulations developed by Macdonald et al. (1998), which were modified by Kent et al. (2017) to include the effects of urban trees. The roughness length for heat and water vapour is assumed to be 1∕10 of the momentum roughness length. The aerodynamic resistance above the urban canopy, rah, is calculated according to Mascart et al. (1995) with a simplified parameterization of the Monin–Obukhov similarity theory. The vertical aerodynamic resistance within the canyon is calculated with an undercanopy resistance parameterization, rah,u (Mahat et al., 2013). The air volume within the canyon is subdivided into two layers with a height equal to the minimum between 4 m and HCanyon for the first layer and HCanyon−4 m for the second layer, which is not present if HCanyon is less than 4 m. The total wall sensible heat flux is calculated as the area-weighted average of the two layers, with only the first layer contributing to the wall sensible heat flux at 2 m canyon height (Sect. 2.1.4 of the TRM). UT&C allows for an average canyon height HCanyon lower than 4 m, and, in such cases, the sensible heat flux from the wall is entirely contributing to the 2 m air temperature. The horizontal aerodynamic resistance from the wall to the canyon air, rah,w, is calculated with the respective wind speeds at mid-height of each canyon air layer with the formulations of Rowley et al. (1930) and Rowley and Eckley (1932). The leaf boundary layer resistance, rb, describing the resistance imposed by a thin viscous sublayer of air around the leaf surfaces, is calculated as a function of wind speed and leaf dimension (Fatichi et al., 2012a, b; Leuning et al., 1995; Monteith, 1973; Choudhury and Monteith, 1988; Shuttleworth and Gurney, 1990). The soil resistance, rsoil, describes the transport of water vapour from the soil pores to the air above the soil surface boundary layer and is a function of the atmospheric conditions and wetness of the surface layer (Haghighi et al., 2013; Fatichi and Pappas, 2017). The total soil resistance is the sum of the soil boundary layer resistance and internal capillary-viscous resistance (Haghighi et al., 2013; Fatichi and Pappas, 2017). The stomatal resistance, rs, describes the transport of water vapour from the leaf interior to the air. UT&C calculates the stomatal resistance with a biochemical model as a function of photosynthetic activity, as described in Sect. 2.3.1. Transpiration fluxes only occur from the vegetation canopy fraction, which is not covered by intercepted water. Evaporative fluxes occur from ground, impervious surfaces (except walls) and the canopy fraction covered by intercepted water. The fraction of vegetation canopy covered by water is calculated according to Deardorff (1978).
The detailed description of all the sensible and latent heat fluxes, resistance parameterizations, wind profile, displacement height, and roughness length calculations can be found in Sects. 2 and 3 of the TRM.
2.1.3 Conductive heat fluxes
The conductive heat fluxes of wall and roof are calculated with a numerical solution of the heat diffusion equation (Hu and Islam, 1995; Hillel, 1998; Núnez et al., 2010; Masson, 2000). UT&C considers two physical layers for vegetated roof and one physical layer for impervious roof, and sunlit and shaded wall. The numerical solution is based on three nodes (two layers) with the inner boundary condition equal to the interior building temperature, Tb, which is set equal to the atmospheric forcing temperature within the range of a specified minimum Tb,min and maximum temperature Tb,max. Below and above Tb,min and Tb,max, the interior building temperature is fixed to Tb,min and Tb,max assuming air-conditioning or heating of the building interior (de Munck et al., 2018). Furthermore, UT&C is able to account for a fixed prescribed interior building temperature Tb. The outer boundary condition is given by the prognostic surface temperature and in between an internal wall and roof temperature is calculated to account for heat storage effects. The ground conductive heat flux is calculated with the force restore method (Hu and Islam, 1995; Noilhan and Planton, 1989; Fatichi et al., 2012a, b). Soil volumetric heat capacity and soil thermal conductivity are calculated as a function of soil type and soil water content according to de Vries (1963), Farouki (1981), and Oleson et al. (2013), as described in Fatichi et al. (2012a, b).
Further information on the calculation of the conductive heat fluxes can be found in Sect. 4 of the TRM.
2.1.4 Anthropogenic heat fluxes
UT&C accounts for a prescribed time series of anthropogenic heat flux, which is added to the canyon air, assuming that heat emissions mostly occur within the urban canyon. Hence, anthropogenic heat emissions caused by air conditioning, car exhaust, industry, human metabolism, or any other anthropogenic heat source need to be estimated prior to simulation, e.g. using existing approaches (Sailor and Lu, 2004; Sailor et al., 2015). Anthropogenic heat effects caused by domestic heating or cooling of building interiors are already accounted for through the conductive heat flux from building interior to canyon air that is influenced by the fixed interior building temperature, as described in Sect. 2.1.3 and in Sect. 5 of the TRM. The anthropogenic heat inputs used to assess the model performance are based on site-specific values (Roth et al., 2016; Chow et al., 2014) and summarized in the TRM (Sect. 9).
2.2 Water budget
2.2.1 Interception and ponding
UT&C calculates interception of water by vegetation canopies and ponding on impervious surfaces, bare, and vegetated soils. The interception and ponding dynamics are calculated with a mass budget approach that can be written as (Rutter et al., 1971, 1975; Ivanov et al., 2008; Fatichi et al., 2012a, b)
where In (mm) is the intercepted or ponding water, P* (mm h−1) the incoming water flux from precipitation and runon, D (mm h−1) the canopy drainage or infiltration flux from ponding water, and EIn (mm h−1) the evaporation from intercepted and ponding water. The maximum water ponding or storage capacity of impervious surfaces is an uncertain but important parameter to accurately model the latent heat flux after rain events (Wouters et al., 2015; Ramamurthy and Bou-Zeid, 2014). UT&C accounts for a maximum impervious ponding capacity as well as runon, a fraction of runoff that is kept in the system (Sect. 2.2.3).
The detailed description of interception and ponding dynamics can be found in Sect. 6.1 of the TRM and Sect. 2.3.3 for vegetation canopy. The maximum impervious ponding capacity and the fraction of runoff assigned to runon used in the model performance assessment are summarized in the TRM (Sect. 9).
2.2.2 Vadose soil moisture dynamics
The canyon ground is discretized into n vertical soil layers and three soil columns corresponding to the impervious, bare, and vegetated ground fractions (Fig. 3). The vegetated roof fraction is discretized into one column with m vertical soil layers. The first two layers of the impervious ground fraction are assumed impermeable with negligible porosity and do not participate in the vadose zone dynamics. Soil underneath buildings is not considered in the current parameterization. The 1-D Richards equation (Richards, 1931) is first solved in the vertical direction for each soil column using a finite volume approach with the methods of lines (Lee et al., 2004; Fatichi et al., 2012a, b) as
where θj (–) is the soil water content, dz,j (mm) is the soil layer thickness, and qj−1 and qj (mm h−1) are the vertical inflow and outflow of soil layer j. The transpiration sinks of ground vegetation and trees, Tveg and Ttree (mm h−1), are weighted by their root biomass fraction in each soil layer, and (–). The soil evaporation, Eg (mm h−1), is only present in the first (j=1) soil layer of the bare and vegetated soil column. In a second step, the 1-D Richards equation (Richards, 1931) is solved laterally as
where Ql,in,j and Ql,out,j (mm h−1) are the lateral inflow and outflow of soil layer j with respect to the adjacent soil columns. Exchange of soil moisture between all three soil columns is included in the model, resulting in three lateral fluxes. The vertical qj and lateral Ql,j fluxes of water in the soil are calculated according to the gradients of soil water potentials (see Sect. 6.2.1 of the TRM). The infiltration into the first soil layer is either the maximum infiltration capacity or the water available at the surface, depending on which is limiting. The maximum infiltration capacity for bare and vegetated surfaces is calculated based on the hydraulic gradient between ponding water (if any) and water potential in the first soil layer. The maximum infiltration through the impervious ground surface is a model parameter, and the infiltrated water is directly added to the third soil layer as the first two layers are not interacting with the vadose zone dynamics. The water percolating from the last soil layer n or m is called deep leakage. The formation of a shallow groundwater table is possible if soil hydraulic conditions allow or if an impermeable boundary condition is prescribed at the bottom of the soil column (Fatichi et al., 2012a, b). The soil hydraulic properties are calculated based on the soil textural composition using pedotransfer functions, and soil hydraulic conductivity and soil water retention curve can either be described with the van Genuchten (1980) or Saxton and Rawls (2006) parameterizations.
The detailed description of the vadose zone dynamics can be found in Sect. 6.2 of the TRM.
2.2.3 Runoff and runon
Runoff is generated when the maximum infiltration capacity and then interception capacity of a surface are exceeded. The total roof and ground runoff is calculated as the area averaged runoff of each surface fraction. UT&C allows users to specify a percentage of runoff that stays in the system for one time step (1 h) and it is re-added as runon evenly to either roof or ground areas. Allowing for a runon component is important to model urban areas where excess water from one surface does not exit immediately the system but remains in place (e.g. flat roof) or is redirected to another surface, for example, bioswales. Further information on the calculation of runoff and runon can be found in Sect. 6.3 of the TRM.
2.2.4 Anthropogenic water
UT&C accounts for anthropogenic water in the form of a prescribed urban irrigation time series for vegetated roof, bare ground, and vegetated ground. The irrigation can be added to the soil surface underneath vegetation to represent drip irrigation or above the vegetation surface to represent sprinkler or hose irrigation. The irrigation schemes used during the model performance assessment are described in Sect. 9 of the TRM. Urban vegetation in Phoenix is heavily dependent on irrigation year round and the irrigation time series is modelled as described by Volo et al. (2014).
2.3 Vegetation processes
2.3.1 Photosynthesis and stomata behaviour
Plants open their stomata to allow CO2 exchange between the atmosphere and the chloroplasts inside their leaves and perform photosynthesis. This leads to an inevitable loss of water vapour from the water-saturated tissue within the leaves (Sellers et al., 1997). UT&C applies a biochemical model to describe the coupling between stomatal resistance and photosynthesis (Fatichi et al., 2012a, b). The stomatal behaviour is dependent on the net CO2 assimilation rate (i.e. photosynthesis), atmospheric vapour pressure deficit, and intercellular CO2 concentration (Leuning, 1995). The net assimilation rate is a function of three limiting rates of enzyme kinetics: the RuBisCO enzyme limited carboxylation rate, the rate of photosynthetic active radiation (PAR) captured by the leaf chlorophyll, and the limiting rate of product export and usage (Farquhar et al., 1980; Collatz et al., 1991, 1992; Fatichi et al., 2012a, b). The rates of enzyme kinetics are influenced by the leaf temperature. The net photosynthetic assimilation rate is further influenced by water stress that is inducing stomatal closure (e.g. Zhou et al., 2013).
The detailed mathematical formulations of the biochemical model to calculate net CO2 assimilation rate and stomatal resistance are described in Sect. 3.6.2 and 3.6.3 of the TRM.
2.3.2 Upscaling from leaf to canopy
UT&C applies a “two big leaves” approach that divides vegetation canopy into sunlit and shaded fractions (Wang and Leuning, 1998; Fatichi et al., 2012a). The photosynthetic activity is calculated individually for the two fractions to account for the light limitation occurring in the shaded leaves, which only receive diffuse radiation. UT&C uses an exponential decay of direct beam radiation and leaf nitrogen content with leaf area throughout the vegetation canopy to scale photosynthetic capacity from leaf to canopy level (Dai et al., 2004; Ivanov et al., 2008; Fatichi et al., 2012a). The current version of UT&C does not include a seasonally changing LAI, but time series of LAI can be supplied as model input if needed.
The detailed description of the leaf to canopy upscaling can be found in Sect. 3.6.1 of the TRM.
2.3.3 Canopy interception
Vegetation canopy interception is modelled using a mass budget approach and the Rutter model, as described in Sect. 2.2.1. The fraction of precipitation arriving onto the canopy foliage and its throughfall is modelled as a function of the projected leaf area fraction onto the ground. The projected leaf area fraction is a function of leaf area index (LAI) and stem area index (SAI) (Mahfouf and Jacquemin, 1989). Interception excess drainage occurs if the precipitation on the canopy foliage exceeds the maximum interception capacity of the vegetation canopy. The maximum canopy interception capacity is calculated as a function of LAI and SAI according to Dickinson et al. (1993). Dripping from intercepted water on the canopy is calculated according to the Rutter model (Rutter et al., 1971; Mahfouf and Jacquemin, 1989).
Further description of the canopy interception calculations can be found in Sect. 6.1.1 of the TRM.
2.3.4 Root water uptake and root biomass distribution
The root water uptake from different soil layers is calculated according to the vertical and horizontal plant root biomass distribution.
UT&C allows to distinguish between four different vertical root biomass profiles (Fatichi et al., 2012a, b): (1) an exponential vertical root profile (Arora and Boer, 2005), (2) a linear dose response root profile (Schenk and Jackson, 2002; Collins and Bras, 2007), (3) a constant vertical root profile, and (4) a linear dose response profile with tap roots. The root biomass profile of short stature roof and ground vegetation is horizontally contained within the roof and ground vegetated areas, while two different horizontal root profiles are distinguished for tree roots: (1) the tree roots are evenly distributed over the total canyon width, and (2) the tree roots are horizontally restricted to the tree canopy extent, which is assumed to be mainly located over the vegetated and bare ground fractions. The choice of horizontal tree root distribution is influenced by the patch size distribution as well as the heterogeneity of the pervious ground cover fraction and this affects soil moisture access by trees. The root water uptake can be limited by the water availability in the soil or the hydraulic resistance from the soil to the root (Fatichi et al., 2012a, b). Currently, UT&C does not include a plant hydraulic module and it is assumed that the leaf and xylem water potential are equal to the soil water potential experienced within the root zone (Fatichi et al., 2012a, b). Hence, root water uptake is equal to transpiration, and water storage in plant tissue is neglected even though in certain conditions it could be significant (e.g. Mirfenderesgi et al., 2016; Huang et al., 2017).
The detailed description of vertical and horizontal root profiles, soil-to-root resistance, and root water uptake calculations can be found in Sect. 7 of the TRM.
Table 1Mean values calculated for the entire time period of the meteorological forcing data time series in Telok Kurau in Singapore, Preston in Melbourne, and Maryvale in Phoenix.

1 Velasco et al. (2013); Roth et al. (2016). 2 Coutts et al. (2007a, b). 3 Chow et al. (2014).
3.1 Model performance assessment sites: Singapore, Melbourne, and Phoenix
UT&C is tested to reproduce tower-based eddy-covariance measurements from Telok Kurau in Singapore (Velasco et al., 2013; Roth et al., 2016), Preston in Melbourne, Australia (Coutts et al., 2007a, b), and Maryvale in Phoenix, AZ (Chow et al., 2014). The measurements at all three sites have been performed according to known guidelines to ensure that the measurements are representative of the underlying surface at the neighbourhood scale, have followed accepted measurement protocols, and passed quality-control checks as described in detail in Velasco et al. (2013), Roth et al. (2016), Coutts et al. (2007a, b), and Chow et al. (2014). The measurement sites will afterwards be referred to as Singapore, Melbourne, and Phoenix, respectively.
Singapore experiences a tropical rainforest climate (Köppen classification: Af) with uniformly high air temperature throughout the year (data mean: 27.5 ∘C), high relative humidity (data mean: 71 %), and abundant rainfall (data mean: ∼ 1840 mm yr−1, which is lower than the long-term mean of ∼ 2340 mm yr−1) (Table 1) (Velasco et al., 2013; Roth et al., 2016). Two monsoonal wind regimes are observed: the southwest monsoon (June to September) and the northeast monsoon (December to mid-March) (Velasco et al., 2013; Roth et al., 2016). The meteorological time series used in this study is characterized by an unusual dry period from mid-January 2014 to mid-March 2014, with an almost complete absence of rainfall (Harshan et al., 2017; Demuzere et al., 2017). The Singapore measurement site is located in the Telok Kurau district (1∘18′51′′ N, 103∘54′40′′ E; ∼ 10 m a.s.l.), which corresponds to a “compact low-rise” local climate zone (LCZ3) (Stewart and Oke, 2012). It is a residential area with a mean building and tree height of 9.86 and 7.26 m, respectively, and an area averaged height-to-width ratio (H ∕ W) of 0.61 (Velasco et al., 2013; Roth et al., 2016; Demuzere et al., 2017). The surface cover consists of 39 % buildings, 34 % paved and gravel, 12 % roads, 11 % trees, 4 % grass, and 1 % water (Velasco et al., 2013; Roth et al., 2016). The Telok Kurau eddy-covariance measurement site and set-up are described in detail in Velasco et al. (2013) and Roth et al. (2016).
Melbourne experiences a seasonal temperature cycle with warm summers and mild winters (data mean: 13.5 ∘C). The mean observed relative humidity is relatively high (data mean: 67 %), while the precipitation amount is moderate (data mean: ∼ 741 mm yr−1) and is evenly distributed throughout the year (Table 1). The flux tower was located in the suburb of Preston (37∘49′ S, 144∘53′ E; ∼ 93 m a.s.l.) (Coutts et al., 2007a, b) in a low-density, moderately developed residential area classified as an “open low-rise” local climate zone (LCZ6) (Stewart and Oke, 2012; Best and Grimmond, 2015) with mean building height of 6.4 m (Coutts et al., 2007a, b). The land surface is covered by 44.5 % buildings, 4.5 % concrete, 13 % road, 22.5 % vegetation, 15 % grass, and 0.5 % bare ground or pools (Coutts et al., 2007a, b; Grimmond et al., 2011; Best and Grimmond, 2015). Further information on the Preston measurement campaign can be found in Coutts et al. (2007a, b).
Phoenix has a hot, arid subtropical desert climate (Köppen classification: BWh) (Chow et al., 2014). Its temperature is characterized by a yearly cycle with very high summer and cooler winter temperatures (data mean: 24.1 ∘C), and very low relative humidity (data mean: 28 %) (Table 1). The yearly precipitation amount is small and occurs during winter (December–February) and in summer during the North American monsoon season (July–September) (Templeton et al., 2018). The measured time period exhibits lower-than-average rainfall with 99 mm yr−1 (Table 1). The eddy-covariance measurement tower was set up in the suburb of Maryvale (33∘29′2′′ N, 112∘8′35′′ W; 337 m a.s.l.), which corresponds to an “open low-rise” local climate zone (LCZ6) (Stewart and Oke, 2012). It is a suburban residential area with low-rise, single-family, one-story houses with a mean building and tree height of 4.5 and 4 m, respectively, and a H ∕ W of 0.4 (Chow et al., 2014). The land cover consists of 26 % buildings, 22 % roads and asphalt, 5 % trees, 10 % grass, 37 % bare soil, and < 1 % water and pools (Chow et al., 2014). The landscape is mostly xeric (dry), and hose irrigation is used to water gardens. Detailed information on the Maryvale eddy-covariance study site can be found in Chow et al. (2014).
The exact model parameters used in the UT&C validation in Singapore, Melbourne, and Phoenix can be found in Sect. 9 of the TRM.
3.2 Model performance metrics
The UT&C assessment is based on the comparison between measured and simulated outgoing shortwave radiation S↑, outgoing longwave radiation L↑, net absorbed all-wave radiation Rn, sensible heat flux H, and latent heat flux λE. The comparison is based on time series of hourly day- and nighttime fluxes, and daily cycles of flux mean and standard deviation. Model performance is assessed considering the coefficient of determination (R2), root mean square error (RMSE), mean absolute error (MAE), and mean bias error (MBE). Furthermore, the systematic (RMSEs) and non-systematic (RMSEu) components of the RMSE error (Willmott, 1982) are calculated and reported in Sect. 10 of the TRM. All model performance indices are calculated with the available data of the full time period specified for each location (Tables 1, 2, and 3) including all weather conditions, except for hours with instantaneously occurring rainfall (Chow et al., 2014; Roth et al., 2016). Shortwave radiation performance is assessed only considering daytime values. Separate model performance is also calculated for day- and nighttime and reported in Sect. 10 of the TRM as well as for an exceptional dry period from 15 February to 16 March 2014 in Singapore (Table 3). Daytime is defined as 08:00–18:00 LT for Singapore and as times with positive incoming shortwave radiation for Melbourne and Phoenix. Nighttime is defined as 20:00–06:00 LT for Singapore and as times with no incoming shortwave radiation for Melbourne and Phoenix. The overall model performance results are compared to literature that validates other urban canyon models using flux tower measurements from Telok Kurau in Singapore, Preston in Melbourne, and Maryvale in Phoenix (Table 2).
The total assessment period in Telok Kurau, Singapore, is 1 year (1 May 2013–30 April 2014; Table 1). The UT&C model performance results are compared to the previous studies of Demuzere et al. (2017), Harshan et al. (2017), and Liu et al. (2017), who used the same eddy-covariance measurements from Telok Kurau. Demuzere et al. (2017) analysed the model performance of four urban canopy models (SURFEX: Masson et al., 2013; CLM v4.0: Bonan et al., 2011; Lawrence et al., 2011; TERRA_URB: Wouters et al., 2015, 2016; SUEWS: Ward et al., 2016). Harshan et al. (2017) analysed the performance of one model (TEB: Masson, 2000), and Liu et al. (2017) used flux tower data to validate a coupled Noah/SLUCM model after the implementation of tree evapotranspiration. Additionally, the simulation of 2 m air temperature in Singapore is compared to the measurements (11 November 2013–19 April 2014) presented by Harshan et al. (2017), which were digitized for this purpose.
The total observational period in Preston, Melbourne, is approximately 15.5 months (13 August 2003–28 November 2004) (Table 1). The UT&C model performance results are compared to results from the international urban energy model comparison, phase 2, by Grimmond et al. (2011), who analysed the performance of 32 urban land surface models with eddy-covariance measurements from Preston. The reported RMSE and MBE is the median performance of all the models with radiation budget closure, while R2 values are determined from the reported Taylor diagrams. Furthermore, the UT&C model performance results for Melbourne are compared to the performance of VTUF-3D v1.0 (Nice et al., 2018), which also includes an ecohydrological component and was assessed against Preston eddy-covariance measurements (Nice et al., 2018).
The total assessment period in Maryvale, Phoenix, is approximately 1 year (17 December 2011–31 December 2012) (Table 1) (Chow et al., 2014). The UT&C model performance results are compared to the results of Song and Wang (2015), who assessed a single-layer urban canopy model (Wang et al., 2011, 2013) in Maryvale, Phoenix. Song and Wang (2015) only use a 5 d period for model performance assessment though, while the UT&C model statistics are calculated for the full reported time period. Additionally, the simulation of bare ground temperature at 2 cm soil depth in Phoenix is compared with soil temperature measurements at the same depth conducted by Chow et al. (2014). Since the soil thermal profile is not a direct output of the model, the simulated bare ground surface temperature at 2 cm soil depth was calculated using the bare ground surface temperature and a numerical solution of the heat diffusion equation with mixed boundary conditions assigning surface temperature at the top of the soil column and zero ground heat flux at 2 m depth.
Table 2Coefficient of determination (R2), mean bias error (MBE), root mean square error (RMSE), and mean absolute error (MAE) of the UT&C model performance assessment in Singapore, Melbourne, and Phoenix, and comparison with literature values assessing urban canopy models in the same locations. The validation period specifies the total UT&C simulation period in hours (h) and the percentage of time with available eddy-covariance measurements for model performance assessment. Values marked in bold specify an improved and italic a decreased model performance of UT&C compared to values reported in literature.
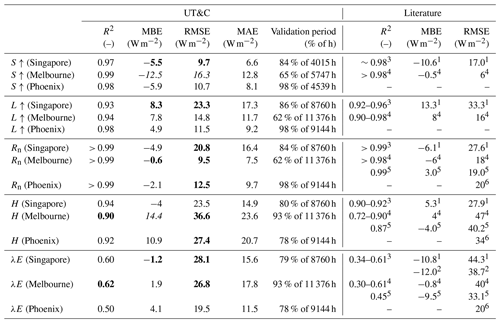
Reference (validation time series): 1 Harshan et al. (2017) (18 May 2013–19 April 2014). 2 Liu et al. (2017) (18 May 2013–19 April 2014). 3 Demuzere et al. (2017) (1 June 2013–17 April 2014): Taylor diagrams. 4 Grimmond et al. (2011) (August 2003–November 2004): coefficients of determination R2 are determined from the Taylor diagrams and specify the performance range of the majority of models. The reported RMSE, MBE, and MAE specify the median model performance in the subset of models with radiation budget closure. 5 Nice et al. (2018) (10 February–10 March 2004). 6 Song and Wang (2015) (12–17 June 2012).
Table 3Same as Table 2 for the dry period (15 February–16 March 2014) in Telok Kurau, Singapore. Values marked in bold specify an improved model performance of UT&C compared to values reported in literature.
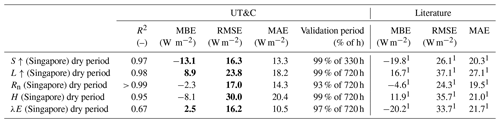
1 Harshan et al. (2017) (15 February–16 March 2014).
3.3 Model capability and sensitivity analysis
The capability of UT&C to describe urban climate, hydrology, and vegetation is further shown through the modelled time series of soil moisture, the resulting plant water stress, and decrease in latent heat during the dry period of February 2014 in Singapore. Furthermore, the effect of changes in vegetated ground cover within the urban canyon (λG,veg), LAI, and maximum RuBisCO capacity (Vc,max) on the long-term 2 m air temperature, 2 m relative humidity, and the energy and water budget is shown through a sensitivity analysis using the background climate, urban fabric, and geometries of Telok Kurau in Singapore (see Sect. 9 of the TRM for parameter set-up of Telok Kurau). Relative humidity is dependent on the saturation vapour pressure which is directly connected to the air temperature, and therefore relative humidity changes are also linked to temperature changes and not only the water content in the air. In this study, the analysis of relative humidity is chosen, as it plays a key role in the outdoor thermal comfort of humans. The simulation time series length is 1 year, and the results are analysed as mean changes over the whole time period, mean changes during an unirrigated dry period (15 February–16 March 2014), and mean daily cycles averaged over the whole year, respectively. Mean changes are computed in comparison to a non-vegetated condition for the increase of λG,veg, in comparison to the flux tower baseline condition (λG,veg=25 % and λtree=18 % within the urban canyon) with a LAI of 0.5 for the LAI increase, and in comparison to the flux tower baseline condition with Vc,max of 20 µmol CO2 s−1 m−2 for the Vc,max increase. λG,veg is varied between 0 and 100 % (0 and 1), LAI between 0.5 and 5, and Vc,max between 20 and 120 µmol CO2 s−1 m−2 (the figure of the schematic set-up is presented in Sect. 10 of the TRM). These ranges correspond to realistic values of biophysical and physiological parameters observed in nature (Wullschleger, 1993; Kattge et al., 2009; Iio et al., 2014; Paschalis et al., 2018; Manoli et al., 2018). Low values of λG,veg specify a low amount of ground vegetation within the urban canyon, low values of LAI specify a thin vegetation canopy, and low values of Vc,max specify plants with small photosynthetic and transpiration capacity. The sensitivity analysis for vegetated ground cover is performed without trees, as a fully sealed ground surface with trees is not a realistic scenario. The increase of LAI and Vc,max includes vegetated ground cover and trees, and the parameters are simultaneously increased for both vegetation types.
4.1 Model performance
4.1.1 Outgoing shortwave and longwave radiation, and net all-wave radiation
Modelled and observed S↑ show good agreement with a high R2 of 0.97, 0.99, and 0.98 for Singapore, Melbourne, and Phoenix, respectively (Table 2). S↑ is generally well predicted in urban climate models with high R2 of 0.98 or above, as shown by Grimmond et al. (2011) and Demuzere et al. (2017) in their model intercomparison studies. UT&C is able to accurately simulate the mean diurnal cycle and variability of S↑ (Sect. 10 of the TRM) but slightly underpredicts S↑ in all three locations with MBEs of −5.5, −12.5, and −5.9 W m−2 for Singapore, Melbourne, and Phoenix, respectively (Table 2). UT&C shows improved modelling of S↑ for the Singapore site, with MBE = −5.5 and RMSE = 9.7 W m−2, compared to TEB with MBE = −10.6 and RMSE = 17.0 W m−2 (Harshan et al., 2017). The MBE = −12.5 and RMSE = 16.3 W m−2 of the UT&C simulation in Melbourne lie within the range reported by Grimmond et al. (2011) but are worse than the median model (conserving radiation budget), with MBE = -0.5 and RMSE = 6 W m−2. Phoenix overall shows good results, with MBE = −5.9 and RMSE = 10.7 W m−2.
Modelled and measured L↑ show a high R2 of 0.93, 0.94, and 0.98 for Singapore, Melbourne, and Phoenix, respectively (Table 2). These values are within the range reported by Demuzere et al. (2017) in Singapore (R2 = 0.92–0.96) and the range reported by Grimmond et al. (2011) in Melbourne (R2= 0.90–0.98). The UT&C simulation in Singapore shows an overestimation of L↑ during the day and an underestimation of L↑ during the night (Sect. 10 of the TRM). These trends are consistent throughout the year and similar trends are also observed by Harshan et al. (2017). UT&C shows an improved modelling of L↑ with MBE = 8.3 and RMSE = 23.3 W m−2, compared to TEB in Singapore with MBE = 13.3 and RMSE = 33.3 W m−2 (Harshan et al., 2017) (Table 2). The MBE = 7.8 and RMSE = 14.8 W m−2 of the UT&C simulation in Melbourne are similar to the median model (MBE = 8 and RMSE = 16 W m−2) reported by Grimmond et al. (2011). The mean daily cycle and variability of L↑ is well represented by the UT&C simulation in Phoenix, with a small positive MBE = 4.9 W m−2 and RMSE = 11.5 W m−2 (Table 2 and Sect. 10 of the TRM).
The net all-wave radiation Rn shows very good agreement in all three sites with R2 of > 0.99, > 0.99, and > 0.99 for Singapore, Melbourne, and Phoenix, respectively (Table 2). These results agree with the high R2 values of > 0.98 reported in the literature for Singapore (Demuzere et al., 2017) and Melbourne (Grimmond et al., 2011). Similarly, the diurnal cycle, time series, and correlation plots show good agreement between model prediction and measurement (Fig. 4). The MBE = −4.9 and RMSE = 20.8 W m−2 of the UT&C simulation in Singapore shows a slight improvement compared to the values of MBE = −6.1 and RMSE = 27.6 W m−2 reported by Harshan et al. (2017) (Table 2). The MBE = −0.6 and RMSE = 9.5 W m−2 of the UT&C simulation in Melbourne shows an improvement compared to the median of the models with MBE = −6 and RMSE = 18 W m−2 reported by Grimmond et al. (2011) and MBE = 3 and RMSE = 19 W m−2 reported by Nice et al. (2018) for VTUF-3D (Table 2). The RMSE = 12.5 W m−2 of the simulation in Phoenix shows a slight improvement compared to the RMSE = 20 W m−2 reported by Song and Wang (2015) (Table 2).
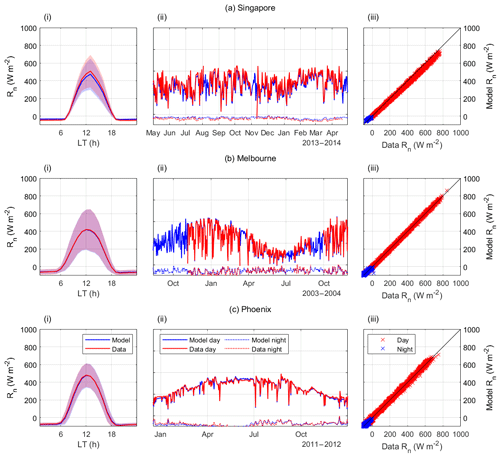
Figure 4Comparison of modelled and measured net absorbed all-wave radiation Rn for the sites in (a) Singapore, (b) Melbourne, and (c) Phoenix. (i) Average diurnal cycle (lines) ±1 standard deviation (shaded area). (ii) Time series of mean daytime (solid lines) and nighttime (dashed lines) fluxes. (iii) Scatter plot of measurements and simulations of hourly daytime and nighttime fluxes.
4.1.2 Sensible heat flux
A relatively high R2 between measured and simulated sensible heat flux, H, is observed, with R2= 0.94, R2= 0.90, and R2= 0.92 for Singapore, Melbourne, and Phoenix, respectively (Table 2). These values lie within the range reported in the literature with R2= 0.90–0.92 for Singapore (Demuzere et al., 2017), and R2 = 0.72–0.90 for Melbourne (Grimmond et al., 2011; Nice et al., 2018). UT&C overestimates sensible heat flux in Melbourne during daytime, while the daytime sensible heat flux in Singapore and Phoenix is well predicted (Fig. 5). The overall model performance statistics with MBE = −4.0 W m−2 and RMSE = 23.5 W m−2 for Singapore are similar to the results of MBE = 5.3 W m−2 and RMSE = 27.9 W m−2 reported by Harshan et al. (2017) (Table 2). The simulation in Melbourne shows an improvement in RMSE, with RMSE = 36.6 W m−2 compared to the literature values, i.e. RMSE = 47 W m−2 (Grimmond et al., 2011) and RMSE = 40.2 W m−2 (Nice et al., 2018); however, the UT&C simulation shows a larger bias with MBE = 14.4 W m−2 compared to MBE = 4 W m−2 (Grimmond et al., 2011) and MBE = −4 W m−2 (Nice et al., 2018) (Table 2). Even though the mean daytime cycle is well represented, the simulation in Phoenix shows a relatively large MBE = 10.9 W m−2 due to a overprediction at night. The simulated RMSE = 27.4 W m−2 shows a slight improvement compared to the literature value of RMSE = 34 W m−2 (Song and Wang, 2015) (Table 2).
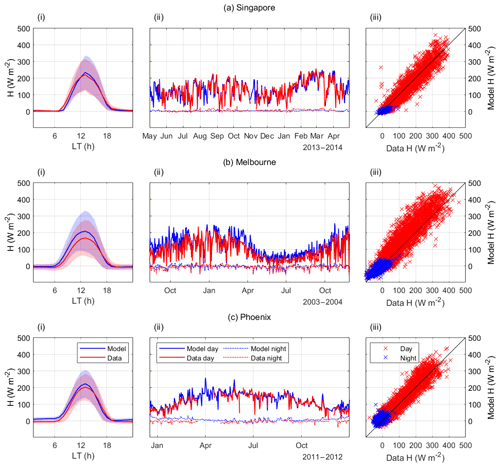
Figure 5Comparison of modelled and measured sensible heat flux H for the sites in (a) Singapore, (b) Melbourne, and (c) Phoenix. (i) Average diurnal cycle (lines) ±1 standard deviation (shaded area). (ii) Time series of mean daytime (solid lines) and nighttime (dashed lines) fluxes. (iii) Scatter plot of measurements and simulations of hourly daytime and nighttime fluxes.
4.1.3 Latent heat flux
The latent heat flux λE is commonly the most difficult energy flux to predict in urban canopy modelling (Grimmond et al., 2011; Ramamurthy et al., 2014), because it is typically of lower magnitude and more variable than the other fluxes, with assumptions about frequency and amount of irrigation adding further uncertainty. The R2 values of the UT&C simulation with R2= 0.60, R2= 0.62, and R2= 0.50 for Singapore, Melbourne, and Phoenix, respectively, lie within the reported literature range of R2= 0.34–0.61 (Demuzere et al., 2017) for Singapore, and R2= 0.30–0.61 (Grimmond et al., 2011), R2= 0.45 (Nice et al., 2018) for Melbourne (Table 2). The UT&C simulation is able to capture the mean daily cycle of latent heat in Singapore, Melbourne, and Phoenix (Fig. 6). The variability of λE shown as standard deviation in the mean daily cycle plots is well predicted in Melbourne, whereas it is underestimated in Singapore and Phoenix (Fig. 6). During model development, it was observed that the variability of λE is heavily influenced by the maximum ponding storage capacity of impervious surfaces, which is difficult to estimate in a heterogeneous urban environment. UT&C shows an improvement of latent heat simulation in Singapore with MBE = −1.2 and RMSE = 28.1 W m−2, compared to the MBE = −10.8 and RMSE = 44.3 W m−2 reported by Harshan et al. (2017), and the MBE = −12.0 and RMSE = 38.7 W m−2 reported by Liu et al. (2017). Likewise, the simulation in Melbourne shows a slight improvement in RMSE, with RMSE = 26.8 W m−2 compared to RMSE = 40 W m−2 (Grimmond et al., 2011) and RMSE = 33.1 W m−2 (Nice et al., 2018), while MBE = 1.9 W m−2 of the simulation in Melbourne shows a decrease and increase in model performance compared to MBE = −0.8 W m−2 (Grimmond et al., 2011) and MBE = −9.5 W m−2 (Nice et al., 2018). Simulated RMSE = 19.5 W m−2 with UT&C and literature RMSE = 20 W m−2 (Song and Wang, 2015) are relatively similar for Phoenix.
Overall, UT&C shows an equal or improved ability to model the latent heat flux in comparison to other models applied to Singapore, Melbourne, and Phoenix. Additionally, UT&C shows an improved modelling of latent heat during the dry period in Singapore with an R2 value of 0.67, MBE of 2.5 W m−2, RMSE of 16.2 W m−2, and MAE of 10.5 W m−2 compared to the results of Harshan et al. (2017) that show MBE of −20.2 W m−2, RMSE of 33.7 W m−2, and MAE of 21.7 W m−2. The reason for UT&C's more accurate prediction of the latent heat flux during prolonged dry periods is its explicit representation of soil moisture access by plant roots at different soil depths and modelling of plant response to water stress (see Sect. 4.2). The improved prediction can also be seen from mid-January to mid-March 2014 when UT&C predicts a latent heat flux comparable in magnitude to the measured latent heat flux (Fig. 6), whereas other models significantly underpredict λE during this period (Demuzere et al., 2017; Harshan et al., 2017).
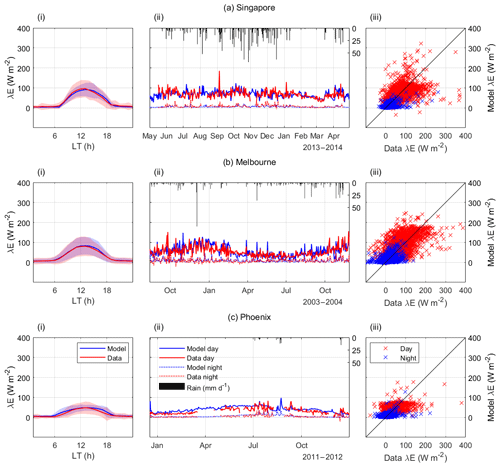
Figure 6Comparison of modelled and measured latent heat flux λE for the sites in (a) Singapore, (b) Melbourne, and (c) Phoenix. (i) Average diurnal cycle (lines) ±1 standard deviation (shaded area). (ii) Time series of mean daytime (solid lines) and nighttime (dashed lines) fluxes. (iii) Scatter plot of measurements and simulations of hourly daytime and nighttime fluxes.
4.1.4 Bare ground surface temperature (Phoenix) and 2 m air temperature (Singapore)
We compare simulated bare ground temperature at 2 cm depth with measured 2 cm soil temperature in Phoenix. Modelled and measured bare ground temperatures show a high agreement with R2 of 0.98, MBE of −0.1 ∘C, RMSE of 2.2 ∘C, and MAE of 1.7 ∘C. UT&C slightly underpredicts (overpredicts) ground temperature during the day (night) and shows a slight phase shift but is overall able to accurately predict bare ground temperature (Fig. 7).
UT&C overpredicts (underpredicts) 2 m air temperature in Singapore during the day (night) compared to the measurement conducted by Harshan et al. (2017). The overall mean difference (MBE) is −0.05 ∘C. The mean overprediction during daytime is 0.9 ∘C, with the maximum value of 2.3 ∘C occurring at 13:00 LT. The overall mean underprediction during nighttime is −1.2 ∘C, with the largest negative value of −1.4 ∘C occurring at 06:00 LT (Fig. 8). This result is not surprising and is coherent with the biases observed in Singapore for longwave radiation. Furthermore, the 2 m air temperature measured at the flux tower area, an open grass field, might not be representative of the average urban land cover based on a 500 m radius in Telok Kurau.
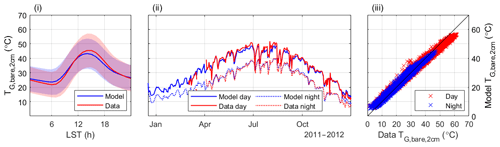
Figure 7Comparison of modelled and measured ground temperature at 2 cm depth (Tg) for the site in Phoenix. (i) Average diurnal cycle (lines) ±1 standard deviation (shaded area). (ii) Time series of mean daytime (solid lines) and nighttime (dashed lines) ground temperature. (iii) Scatter plot of measurements and simulations of hourly daytime and nighttime temperature.
4.2 Ecohydrological dynamics during a dry period
UT&C is able to quantify the contribution of energy and water fluxes from different urban surfaces (impervious, bare and vegetated ground, sunlit and shaded wall, and impervious and vegetated roof) and source mechanisms (e.g. flux of water vapour from transpiration and canopy interception). The contribution of latent heat from impervious surfaces (roof and ground), vegetated ground, and trees to the overall latent heat flux for the simulation time period in Telok Kurau, Singapore, is analysed and shown in Fig. 9. Latent heat from impervious surfaces is highly variable and depends on the amount of rain fallen in the previous hours. On the other hand, latent heat from vegetated ground and trees varies less and forms the baseline of the total latent heat flux. Of special interest in this study is the exceptionally dry period observed between mid-January and mid-March 2014 (Ziegler et al., 2014). During this period, rain was absent and no latent heat from impervious surfaces was observed besides a spike on 8 February 2014 related to a small rainfall event of 2.2 mm on this day. The latent heat from vegetated ground is initially high but starts to decrease as the dry period persists, while the latent heat from trees remains constant and high (Fig. 9). This different behaviour of ground vegetation (grass) and trees can be explained by the water stress experienced by the different vegetation types. Plant water stress is modelled as a function of the overall soil water potential experienced by grass and tree roots (Fig. 9). In the current parameterization for Singapore, stomata closure due to plant water stress starts at a soil water potential of −0.5 and −0.9 MPa for grass and trees, respectively, and stomata closure reaches 50 % at a soil water potential of −1.6 and −1.7 MPa. During the dry period from mid-January to mid-March 2014, the grass experiences water stress (Fig. 9), which leads to stomata closure and a decrease in latent heat, while trees experience only moderate water stress and their transpiration continues at high rates. This difference in water stress is caused by the grass and tree root profiles, which allows them to access water at different soil depths. During the dry period, the upper soil layers of the vegetated soil column dry out, while the deep soil layers are barely affected by the weather conditions, as shown in Fig. 10. The grass has only access to the drier top soil layers (Fig. 10) as 95 % of its roots are shallower than 30 cm, while trees are able to access the wet deeper soil layers (e.g. from 70 to 175 cm depth; Fig. 10) as their roots are assumed to reach a depth of 1.5 m (Harshan et al., 2017) (ZR95; Sect. 9 of the TRM). This explicit representation of soil moisture in different soil layers and the vertical and horizontal root profile are important to represent the effects of climate and environment on plant performance. Furthermore, such a modelling solution improves model performance during the dry period from mid-January to mid-March 2014 in Singapore, as shown in Sect. 4.1.3 and Fig. 6.
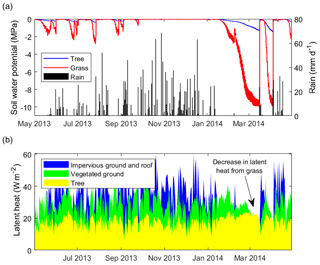
Figure 9(a) Rain in mm d−1 (measurement) and soil water potential averaged over the root zone (MPa) (simulation) showing the water stress experienced by the ground vegetation (grass) and trees during the model validation in Telok Kurau, Singapore. In the current parameterization, plant stomatal closure starts at a soil water potential of −0.5 and −0.9 MPa for grass and trees, respectively. Stomatal closure reaches 50 % at −1.6 and −1.7 MPa for grass and trees, respectively. (b) Simulated time series of latent heat from impervious surfaces, vegetated ground, and trees during the model validation period in Telok Kurau, Singapore. The shown fluxes correspond to the additive flux contribution from each surface to the total canyon latent heat flux.
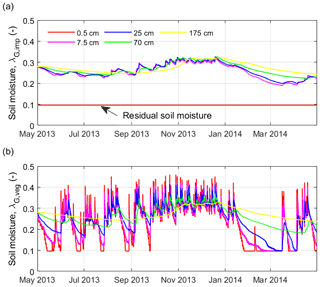
Figure 10Simulated soil moisture in soil columns underneath impervious ground cover (a) and vegetation (b) at 0.5, 7.5, 25, 70, and 175 cm depth at Telok Kurau, Singapore. Residual water content is 0.096 (–) and saturated water content is 0.460 (–). As the top soil layer of the impervious ground cover is fully sealed, it is displayed here with the residual soil moisture. The time series includes one unusually dry period from mid-January to mid-March 2014.
4.3 Singapore sensitivity analysis
4.3.1 Air temperature, relative humidity, and evapotranspiration
The increase of vegetated ground cover (λG,veg) in Singapore from 0 to 100 % leads to an overall reduction of 2 m air temperature (T2 m) of 1.1 ∘C, while relative humidity at 2 m (RH2 m) and canyon evapotranspiration (ETcanyon) are increased by 6.5 % and 1.8 mm d−1, respectively (Figs. 11, 12 and Sect. 10 of the TRM). The daily cycle analysis shows a larger average decrease of T2 m and increase of RH2 m and ETcanyon around solar noon with maximum values of 2.2 ∘C (14:00 LT), 12.9 % (13:00 LT), and 0.33 mm h−1 (13:00 LT), respectively (Table 4, Figs. 11, 12, and Sect. 10 of the TRM).
The increase of LAI from 0.5 to 5 for vegetated ground and trees leads to a reduction of T2 m by 0.2 ∘C. The mean maximum decrease of T2 m is observed at a LAI of 2.5, while no further decrease occurs at higher values of LAI (Fig. 11). The overall increase of LAI leads to an increase of RH2 m and ETcanyon by 2.1 % and 0.7 mm d−1, respectively (Fig. 12 and Sect. 10 of the TRM). The daily cycle analysis shows small differences in the decrease of T2 m and increase of RH2 m throughout the day, with maximum values occurring during morning and evening hours of 0.3 ∘C (17:00 LT) and 2.7 % (08:00 LT), respectively (Figs. 11 and 12). On the other hand, the maximum increase of ETcanyon is observed at solar noon, with a magnitude of 0.07 mm h−1 (13:00 LT) (Sect. 10 of the TRM).
The sensitivity to maximum RuBisCO capacity (Vc,max), as indicative of plant photosynthetic capacity, leads to an average reduction of T2 m by 0.3 ∘C, an increase of RH2 m by 1.6 %, and ETcanyon by 0.7 mm d−1, respectively (Figs. 11, 12, and Sect. 10 of the TRM). The daily cycle shows a larger decrease of T2 m and increase of RH2 m and ETcanyon around solar noon and in the late morning hours with maximum values of 0.7 ∘C (13:00 LT), 4.2 % (11:00 LT), and 0.09 mm h−1 (13:00 LT), respectively (Table 4, Figs. 11, 12, and Sect. 10 of the TRM).
During the dry period (15 February–16 March 2014), the mean decrease in T2 m and increase in RH2 m is lower than the decrease observed considering all weather conditions (Figs. 11 and 12). This is expected as no irrigation is applied and the vegetation is water stressed, as described in Sect. 4.2. A stronger reduction in cooling potential is obtained when modifying LAI and Vc,max as the cooling effect of these parameters relies on an increase in transpiration per unit of ground area, which is not possible if soil moisture is not available. At high values of Vc,max, the cooling effect even further decreases as high transpiration rates during a dry period lead to a quick depletion of soil moisture and a longer period with decreased transpiration afterwards. Increasing the vegetated ground cover (λG,veg) is only slightly less effective during the dry period than over the whole year. This is explained by the fact that an increase in vegetated ground cover also increases the total soil moisture available for transpiration within the canyon even though soil moisture available per unit vegetated ground area does not change much.
As expected, the largest changes in T2 m, RH2 m, and ETcanyon are observed when modifying λG,veg, while the increase of LAI and Vc,max leads to alterations of smaller magnitudes. However, the capability of providing a mechanistically constrained quantification of these values is a non-trivial result of the UT&C application and opens the doors to test various scenarios of urban-green arrangements and types in various climates. The increase of λG,veg and Vc,max leads to a steady decrease of T2 m mostly caused by an increase in latent heat. On the other hand, the increase of LAI does not lead to a steady decrease of T2 m. Mechanisms such as obstruction to turbulent heat exchange with higher LAI, accounted for in the parameterization of zero-plane displacement height and roughness length of the urban canopy (Sect. 3.2 of the TRM), increased longwave radiation, and light limitation to photosynthesis start to counteract or limit the beneficial effects of higher LAI, such as shading and evapotranspiration. Additionally, the diurnal timing of maximal change is of interest, as higher T2 m reduction during midday, for example, observed with increasing λG,veg, can be especially beneficial for outdoor thermal comfort.
Table 4Mean change over the whole simulation period and maximum change simulated within the mean daily cycle in local time (LT) of 2 m air temperature (ΔT2 m), 2 m relative humidity (ΔRH2 m), and evapotranspiration fluxes (ΔETcanyon) at λG,veg=100 % compared to λG,veg=0 %, LAI = 5 compared to LAI = 0.5, and Vc,max = 120 µmol CO2 s−1 m−2 compared to Vc,max = 20 µmol CO2 s−1 m−2. The hour of the day experiencing the maximum change is reported.
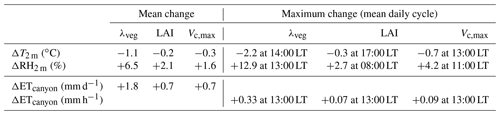
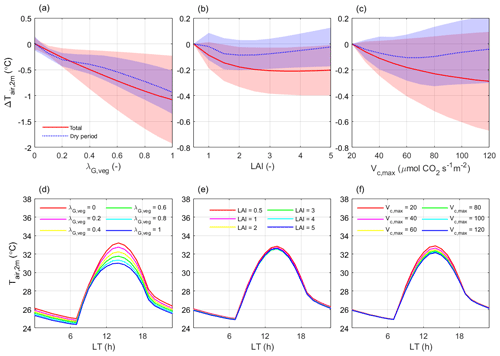
Figure 11Change in 2 m canopy layer air temperature (Tair,2 m) caused by the change in vegetated ground cover fraction (λG,veg), leaf area index (LAI), and maximum RuBisCO capacity (Vc,max) in Telok Kurau, Singapore. (a–c) Mean air temperature change considering all weather conditions (solid line) and mean air temperature change during the dry period (15 February–16 March 2014) (dotted line) with respect to the baseline cases ±1 standard deviation (shaded area). The subplots (d–f) show long-term mean daily cycle of air temperature for different values of (d) λG,veg, (e) LAI, and (f) Vc,max considering all weather conditions.
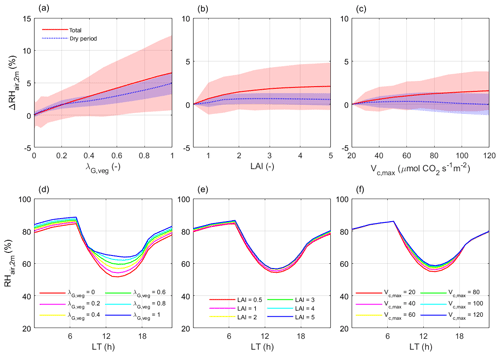
Figure 12Change in 2 m canopy layer relative humidity (RHair,2 m) caused by the change in vegetated ground cover fraction (λG,veg), leaf area index (LAI), and maximum RuBisCO capacity (Vc,max) in Telok Kurau, Singapore. (a–c) Mean relative humidity change considering all weather conditions (solid line) and mean relative humidity change during the dry period (15 February–16 March 2014) (dotted line) with respect to the baseline cases ±1 standard deviation (shaded area). The subplots (d–f) show long-term mean daily cycle of relative humidity for different values of (d) λG,veg, (e) LAI, and (f) Vc,max considering all weather conditions.
Table 5Mean change over the whole simulation period of surface runoff within the canyon (ΔQcanyon), water percolation at the bottom of the soil (ΔLkcanyon), change in water storage on the surface and in the soil (Δ(ΔScanyon)), latent heat flux (ΔλEcanyon), sensible heat flux (ΔHcanyon), conductive heat flux into or out of buildings and ground surface (ΔGcanyon), net absorbed shortwave radiation (ΔSn,canyon), and net absorbed longwave radiation (ΔLn,canyon) at λG,veg=100 % compared to λG,veg=0 %, LAI = 5 compared to LAI = 0.5, and Vc,max = 120 µmol CO2 s−1 m−2 compared to Vc,max = 20 µmol CO2 s−1 m−2.
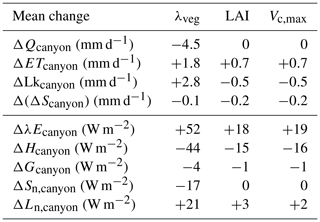
4.3.2 Energy and water balance
The increase of vegetated ground cover (λG,veg) from 0 to 100 % leads to a decrease of runoff (Q) by 4.5 mm d−1, while evapotranspiration (ETcanyon) and deep ground leakage (Lk) increase by 1.8 and 2.8 mm d−1, respectively (Fig. 13, Table 5). These numbers compare with a mean daily rainfall observed during the modelling period of 5.0 mm d−1 (Table 1).
The increase of LAI and maximum RuBisCO capacity (Vc,max) does not alter runoff significantly but slightly increases ETcanyon (0.7 and 0.7 mm d−1) and decreases deep ground leakage (−0.5 and −0.5 mm d−1) (Fig. 13, Table 5). As intuitively expected, these results indicate that plant biophysical and physiological characteristics are much less effective in modifying surface runoff production than the fraction of pervious ground. It has to be noted that these results are dependent on the soil type, in this case a sandy loam with relatively high hydraulic conductivity.
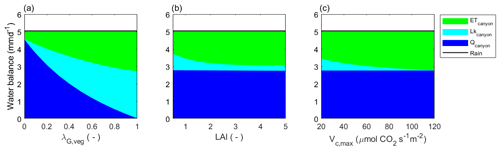
Figure 13Water balance components in the urban canyon (ETcanyon: evapotranspiration; Lkcanyon: deep ground leakage; Qcanyon: surface runoff) as a function of (a) vegetated ground cover fraction (λG,veg), (b) leaf area index (LAI), and (c) maximum RuBisCO capacity (Vc,max) in Telok Kurau, Singapore. The mean daily rainfall is 5 mm d−1.
The increase of ETcanyon and λE caused by the increase of λG,veg, LAI, and Vc,max leads to a decrease in H, while Rn and G show very minor changes (Sect. 10 of the TRM and Table 5). These results are dependent on the albedo of the vegetation for which a value of 0.27 was chosen, as used by Harshan et al. (2017) (Sect. 9 of the TRM), which quite a high value.
The model (UT&C v1.0) presented in this study is among the first attempts to include in a systematic way physiological and biophysical characteristics of vegetation in the solution of the energy and water budget in the urban environment. While many studies have analysed the influence of vegetation on urban climate, UT&C is uniquely capable of answering the question of how different vegetation configurations and species perform in a given climate.
The inclusion of detailed plant physiological and biophysical characteristics is indeed important to quantify said effects. An example of model capability is shown through the sensitivity of simulated 2 m air temperature and 2 m relative humidity in Singapore to the vegetated ground cover fraction, LAI, and maximum RuBisCO capacity. The largest decrease (increase) of air temperature (relative humidity), when compared to the case without vegetation, is observed with a fully grass-covered ground that can generate a change of −2.2 ∘C (+12.9 %) at solar noon and an overall long-term change of mean air temperature (relative humidity) of −1.1 ∘C (+6.5 %). A fully vegetated ground cover might be unrealistic in a normal urban setting but is chosen in this study to demonstrate the maximum expected effect caused by this intervention and therefore its physical limit as a heat mitigation strategy. LAI and maximum RuBisCO capacity show an air temperature and relative humidity modification of much lower magnitude. It is further observed that the increase of maximum RuBisCO capacity leads to a steady decrease (increase) of air temperature (relative humidity) because it does not affect plant structure. Modifying LAI triggers mechanisms, such as changes in radiation exchange, light limitations of photosynthesis within dense canopy, and hindering of turbulent energy exchanges, which do not lead to a further air temperature reduction once a LAI of 2.5 is exceeded in a low-rise setting in the climate of Singapore. These results show that UT&C is sensitive and able to account for multiple effects of vegetation on the local urban climate. It has to be noted that relative humidity is dependent on the water holding capacity of air at a certain temperature, and the relative humidity increase reported here is also dependent on air temperature changes. Nevertheless, the magnitude of relative humidity is important as it influences OTC and might reduce the positive effect of decreasing air temperature.
The results obtained for a low-rise neighbourhood of Singapore, a hot, humid, tropical city, show that maximum urban greening can lead to a non-negligible decrease in air temperature at screen level (2 m) during some hours but will unlikely be able to mitigate the UHI effect on its own. Higher magnitudes of urban cooling due to urban vegetation are reported, for example, by Wang et al. (2018) in the contiguous United States where tree shading reduces near-surface air temperature by 3.06 ∘C and by Middel et al. (2015) in Phoenix where a moderate increase in tree cover can decrease average urban air temperature by 2.0 ∘C. This is consistent with the global analysis performed by Manoli et al. (2019) showing that the cooling potential of urban vegetation is lower in the tropics. Higher air temperature decrease in drier climates is often linked to urban irrigation, as shown by Broadbent et al. (2018b) in Mawson Lakes in Adelaide, where irrigation during a heat wave can reduce average air temperature by up to 2.3 ∘C. In dry climates, however, the trade-off between temperature reduction potential of urban vegetation and water use through irrigation needs to be considered to fully assess the feasibility of such a mitigation strategy (Yang and Wang, 2017; Wang et al., 2019).
The increase in green cover is shown to be more effective in reducing 2 m air temperature and ground surface runoff production than the change in plant types. While changes in urban air temperature and humidity caused by a change in plant physiological and biophysical characteristics are minor in the current analysis in the Singapore climate, their inclusion in urban canopy modelling is very important, as it allows quantification of the order of magnitude of predicted changes and helps to define reasonable expectations of urban planners and landscape designers using vegetation to mitigate the UHI or to improve OTC.
The explicit inclusion of ecohydrology and subsurface hydrology in urban canopy modelling leads to an improved simulation during dry-down periods, as shown in Singapore. This is of particular interest as dry periods may increase in many cities in the future (Bastin et al., 2019) and allow UT&C to analyse the response of urban vegetation under different climate scenarios. Furthermore, UT&C is potentially more accurate in predicting relative humidity at pedestrian level given its more comprehensive inclusion of soil and vegetation processes. This is important to analyse the combined effects of air temperature and relative humidity alterations caused by the urban fabric and urban vegetation on the outdoor thermal comfort of city dwellers, which represent one target application of UT&C.
Future studies could focus on the application of UT&C to analyse different types of urban greening to produce guidelines for urban planners and landscape designers. Possible areas of interest are the study of the effect of urban plant types in different climates, the analysis of various urban densities, a systematic evaluation of urban irrigation practices, as well as the partition of the vegetation role in shade provision versus evapotranspiration cooling in controlling OTC.
The current version of UT&C does not yet include snow hydrology and hence should not be used to investigate the effects of vegetation during winter in cities with snow-dominated climates. Further UT&C developments can also focus on the inclusion of tree shading onto roofs and green walls, and on seasonal vegetation dynamics and vegetation phenology, as in the original T&C model, rather than using a prescribed LAI as currently done.
Future model performance assessment should also focus on a more extensive use of 2 m canyon air temperature, 2 m canyon humidity, and surface temperature data, as the comparison presented here with air temperature in Singapore and ground temperature in Phoenix only gives an indication of model performance, as these variables are highly location specific and potentially not representative of the whole footprint areas below the flux towers modelled here. Additionally, the validation data from low-rise urban climate zones offer only a partial picture of urban conditions, and further validations could focus on high-rise and dense urban settings.
A couple of notable behaviours that were observed during model development and assessment are that the prescribed interior building temperature can influence the urban canyon air temperature, especially in narrow canyons, and hence realistic time series of interior building temperature are fundamental to obtain accurate results (see Sect. 5 of the TRM). Furthermore, it was observed during model development that latent heat variability and peaks are highly dependent on the maximum ponding storage capacity of the impervious surface. The maximum ponding storage capacity of impervious surfaces is difficult to estimate in the highly heterogeneous urban environment, which contains smooth surfaces but also micro-depressions due to its complex geometry and may require innovative ways of observing it to constrain model parameterizations (Wouters et al., 2015).
This study introduces the urban ecohydrological model, Urban Tethys-Chloris (UT&C), and provides a technical description of its components, an assessment of model performance against three different case studies, and a sensitivity analysis to illustrate the model capabilities. UT&C is a fully coupled energy and water balance model that calculates 2 m air temperature, 2 m humidity, urban surface temperatures, and all components of the energy and water balance, including surface runoff. UT&C includes a detailed representation of plant biophysical and ecophysiological characteristics. It is able to account for the effects of different plant types and urban-green typologies on the local microclimate and water fluxes. In turn, it can also provide information on how the urban environment affects plant well-being and performance.
The model was assessed against eddy-covariance measurements in Singapore, Melbourne, and Phoenix, often showing better performance in terms of model validation indices compared to existing models for these three cities. UT&C shows a clear advantage in periods of water stress, as it solves in detail soil hydrological dynamics and can account for different root profiles of urban vegetation and its access to soil moisture as shown for the dry-down period in Singapore.
Explicitly resolving subsurface hydrology and including plant biophysical and ecophysiological characteristics allow the analysis of plant performance under water-limiting conditions. Hence, UT&C is especially suited for arid and semi-arid climates where urban irrigation is or will be applied. Furthermore, UT&C has a low computational demand and allows for analyses spanning multiple years with an hourly or sub-hourly time step, thus facilitating long-term and seasonal studies testing multiple scenarios. Hence, UT&C can assess plant performance under different existing and future climatic conditions, for example, during droughts and responses to increasing temperature, or test the effectiveness of various irrigation practices.
The development of UT&C, model validation, and graphs presented in this paper were conducted in MATLAB R2018b. The exact version of UT&C used to produce the results used in this paper is archived on Zenodo (Meili and Fatichi, 2019). The original source code for the ecohydrological model Tethys-Chloris was obtained from the author (Fatichi et al., 2012a, b), while the building and tree-shading calculations are based on the code of Ryu et al. (2016). The tower-based eddy-covariance measurements used for model validation were obtained from the authors in Telok Kurau, Singapore (Velasco et al., 2013; Roth et al., 2016), and in Preston, Melbourne (Coutts et al., 2007a, b; Nice et al., 2018), and from the Global Institute of Sustainability, Arizona State University (ASU), in Maryvale, Phoenix (Chow et al., 2014; Chow, 2017).
The supplement related to this article is available online at: https://doi.org/10.5194/gmd-13-335-2020-supplement.
NM and SF designed the study, developed the code, conducted the analysis, and wrote the manuscript with inputs from GM. MR, EV, AC, and WC collected and shared their eddy-covariance measurements for the purpose of model validation. EBZ shared the code presented in Ryu et al. (2016). All authors gave comments and contributed to the final version of the manuscript.
The authors declare that they have no conflict of interest.
The research was conducted at the Future Cities Laboratory at the Singapore-ETH Centre, which was established collaboratively between ETH Zurich and Singapore's National Research Foundation (FI370074016) under its Campus for Research Excellence and Technological Enterprise programme. GM was supported by the “The Branco Weiss Fellowship – Society in Science” administered by ETH Zurich. EV acknowledges a research fellowship granted by the Centre for Urban Greenery and Ecology of Singapore’s National Parks Board.
This research has been supported by the Future Cities Laboratory at the Singapore-ETH Centre, which was established collaboratively between ETH Zurich and Singapore's National Research Foundation under its Campus for Research Excellence and Tech (grant no. FI370074016).
This paper was edited by Carlos Sierra and reviewed by two anonymous referees.
Allegrini, J. and Carmeliet, J.: Coupled CFD and building energy simulations for studying the impacts of building height topology and buoyancy on local urban microclimates, Urban Climate, 21, 278–305, https://doi.org/10.1016/j.uclim.2017.07.005, 2017. a, b
Arora, V. K. and Boer, G. J.: A parameterization of leaf phenology for the terrestrial ecosystem component of climate models, Glob. Change Biol., 11, 39–59, 2005. a
Bastin, J.-F., Clark, E., Elliott, T., Hart, S., van den Hoogen, J., Hordijk, I., Ma, H., Majumder, S., Manoli, G., Maschler, J., Mo, L., Routh, D., Yu, K., Zohner, C., and Crowther, T. W.: Understanding climate change from a global analysis of city analogues, PLoS ONE, 14, e0217592, https://doi.org/10.1371/journal.pone.0217592, 2019. a
Berland, A., Shiflett, S. A., Shuster, W. D., Garmestani, A. S., Goddard, H. C., Herrmann, D. L., and Hopton, M. E.: The role of trees in urban stormwater management, Landscape Urban Plan., 162, 167–177, https://doi.org/10.1016/j.landurbplan.2017.02.017, 2017. a
Best, M. J. and Grimmond, C. S. B.: Key conclusions of the first international urban land surface model comparison project, B. Am. Meteorol. Soc., 96, 805–819, https://doi.org/10.1175/BAMS-D-14-00122.1, 2015. a, b
Bonan, G. B., Lawrence, D. M., Swenson, S. C., Oleson, K. W., Jung, M., Lawrence, P. J., Levis, S., and Reichstein, M.: Improving canopy processes in the Community Land Model version 4 (CLM4) using global flux fields empirically inferred from FLUXNET data, J. Geophys. Res., 116, 1–22, https://doi.org/10.1029/2010jg001593, 2011. a
Bowler, D. E., Buyung-Ali, L., Knight, T. M., and Pullin, A. S.: Urban greening to cool towns and cities : A systematic review of the empirical evidence, Landscape Urban Plan., 97, 147–155, https://doi.org/10.1016/j.landurbplan.2010.05.006, 2010. a, b
Broadbent, A. M., Coutts, A. M., Nice, K. A., Demuzere, M., Krayenhoff, E. S., Tapper, N. J., and Wouters, H.: The Air-temperature Response to Green/blue-infrastructure Evaluation Tool (TARGET v1.0): an efficient and user-friendly model of city cooling, Geosci. Model Dev., 12, 785–803, https://doi.org/10.5194/gmd-12-785-2019, 2019a. a, b, c
Broadbent, A. M., Coutts, A. M., Tapper, N. J., and Demuzere, M.: The cooling effect of irrigation on urban microclimate during heatwave conditions, Urban Climate, 23, 309–329, https://doi.org/10.1016/j.uclim.2017.05.002, 2018b. a
Bruse, M. and Fleer, H.: Simulating surface-plant-air interactions inside urban environments with a three dimensional numerical model, Environ. Model. Softw., 13, 373–384, https://doi.org/10.1016/S1364-8152(98)00042-5, 1998. a, b
Choudhury, B. J. and Monteith, J. L.: A four-layer model for the heat budget of homogeneous land surfaces, Q. J. Roy. Meteor. Soc., 114, 378–398, 1988. a
Chow, W.: Eddy covariance data measured at the CAP LTER flux tower located in the west Phoenix, AZ neighborhood of Maryvale from 2011-12-16 through 2012-12-31, Environmental Data Initiative, https://doi.org/10.6073/pasta/fed17d67583eda16c439216ca40b0669, 2017. a
Chow, W. T. L., Volo, T. J., Vivoni, E. R., Darrel, G., and Ruddell, B. L.: Seasonal dynamics of a suburban energy balance in Phoenix , Arizona, Int. J. Climatol., 34, 3863–3880, https://doi.org/10.1002/joc.3947, 2014. a, b, c, d, e, f, g, h, i, j, k, l
Collatz, G. J., Ball, J. T., Grivet, C., and Berry, J. A.: Physiological and environmental regulation of stomatal conductance, photosynthesis and transpiration-A model that includes a laminar boundary-layer, Agr. Forest Meteorol., 54, 107–136, 1991. a
Collatz, G. J., Ribas-Carbo, M., and Berry, J. A.: Coupled photosynthesis-stomatal conductance model for leaves of C4 plants, Aust. J. Plant Physiol., 19, 519–538, 1992. a
Collins, D. B. G. and Bras, R. L.: Plant rooting strategies in water-limited ecosystems, Water Resour. Res., 43, https://doi.org/10.1029/2006WR005541, 2007. a
Coutts, A. M., Beringer, J., and Tapper, N. J.: Characteristics influencing the variability of urban CO2 fluxes in Melbourne, Australia, Atmos. Environ., 41, 51–62, https://doi.org/10.1016/j.atmosenv.2006.08.030, 2007a. a, b, c, d, e, f, g, h
Coutts, A. M., Beringer, J., and Tapper, N. J.: Impact of increasing urban density on local climate: Spatial and temporal variations in the surface energy balance in Melbourne, Australia, J. Appl. Meteorol. Climatol., 46, 477–493, https://doi.org/10.1175/JAM2462.1, 2007b. a, b, c, d, e, f, g, h
Dai, Y., Dickinson, R. E., and Wang, Y.-P.: A two-big-leaf model for canopy temperature, photosynthesis, and stomatal conductance, J. Climate, 17, 2281–2299, 2004. a
Deardorff, J. W.: Efficient prediction of ground surface temperature and moisture with inclusion of a layer of vegetation, J. Geophys. Res., 83, 1889–1903, 1978. a
de Munck, C., Lemonsu, A., Masson, V., Le Bras, J., and Bonhomme, M.: Evaluating the impacts of greening scenarios on thermal comfort and energy and water consumptions for adapting Paris city to climate change, Urban Climate, 23, 260–286, https://doi.org/10.1016/j.uclim.2017.01.003, 2018. a
Demuzere, M., Harshan, S., Jaervi, L., Roth, M., Grimmond, C. S. B., Masson, V., Oleson, K. W., Velasco, E., and Wouters, H.: Impact of urban canopy models and external parameters on the modelled urban energy balance in a tropical city, Q. J. Roy. Meteor. Soc., 143, 1581–1596, https://doi.org/10.1002/qj.3028, 2017. a, b, c, d, e, f, g, h, i, j, k
de Vries, D. A.: Thermal Properties of Soils, in: Physics of the Plant Environment, edited by: van Wijk, W., North-Holland, Amsterdam, 1963. a
Dickinson, R. E., Henderson-Sellers, A., and Kennedy, P. J.: Biosphere-atmosphere transfer scheme (BATS) version 1E as coupled to the NCAR Community Climate Model, Tech. Rep. NCAR/TN-387+STR, Natl. Cent. for Atmos. Res., Boulder, Colorado, 1993. a
Farouki, O. T.: The thermal properties of soils in cold regions, Cold Reg. Sci. Technol., 5, 67–75, 1981. a
Farquhar, G. D., Caemmerer, S. V., and Berry, J. A.: A biochemical model of photosynthetic CO2 assimilation in leaves of C3 species, Planta, 149, 78–90, 1980. a
Fatichi, S. and Pappas, C.: Constrained variability of modeled T:ET ratio across biomes, Geophys. Res. Lett., 44, 6795–6803, https://doi.org/10.1002/2017GL074041, 2017. a, b
Fatichi, S., Ivanov, V. Y., and Caporali, E.: Simulation of future climate scenarios with a weather generator, Adv. Water Resour., 34, 448–467, https://doi.org/10.1016/j.advwatres.2010.12.013, 2011. a
Fatichi, S., Ivanov, V. Y., and Caporali, E.: A mechanistic ecohydrological model to investigate complex interactions in cold and warm water-controlled environments: 1. Theoretical framework and plot-scale analysis, J. Adv. Model. Earth Syst., 4, M5002, https://doi.org/10.1029/2011MS000086, 2012a. a, b, c, d, e, f, g, h, i, j, k, l, m, n, o
Fatichi, S., Ivanov, V. Y., and Caporali, E.: A mechanistic ecohydrological model to investigate complex interactions in cold and warm water-controlled environments: 2. Spatiotemporal analyses, J. Adv. Model. Earth Syst., 4, M5003, https://doi.org/10.1029/2011MS000087, 2012b. a, b, c, d, e, f, g, h, i, j, k, l, m
Frank, A., Heidemann, W., and Spindler, K.: Modeling of the surface-to-surface radiation exchange using a Monte Carlo method, in: J. Phys. Conf. Ser., 745, 032143, https://doi.org/10.1088/1742-6596/745/3/032143, 2016. a
Gillner, S., Vogt, J., Tharang, A., Dettmann, S., and Roloff, A.: Role of street trees in mitigating effects of heat and drought at highly sealed urban sites, Landscape Urban Plan., 143, 33–42, https://doi.org/10.1016/j.landurbplan.2015.06.005, 2015. a
Golasi, I., Salata, F., de Lieto Vollaro, E., and Coppi, M.: Complying with the demand of standardization in outdoor thermal comfort: a first approach to the Global Outdoor Comfort Index (GOCI), Buil. Environ., 130, 104–119, https://doi.org/10.1016/j.buildenv.2017.12.021, 2018. a
Grimm, N. B., Faeth, S. H., Golubiewski, N. E., Redman, C. L., Wu, J., Bai, X., and Briggs, J. M.: Global Change and the Ecology of Cities, Science, 39, 756–760, 2008. a
Grimmond, C. S. B., Blackett, M., Best, M. J., Baik, J., Belcher, S. E., Beringer, J., Bohnenstengel, S. I., Calmet, I., Chen, F., Coutts, A., Dandou, A., Fortuniak, K., Gouvea, M. L., Hamdi, R., Hendry, M., Kanda, M., Kawai, T., Kawamoto, Y., Kondo, H., Krayenhoff, E. S., Lee, S., Loridan, T., Martilli, A., Masson, V., Miao, S., Oleson, K., Ooka, R., Pigeon, G., Porson, A., Ryu, Y., Salamanca, F., Steeneveld, G. J., and Tombrou, M.: Initial results from Phase 2 of the international urban energy balance model comparison, Int. J. Climatol., 272, 244–272, https://doi.org/10.1002/joc.2227, 2011. a, b, c, d, e, f, g, h, i, j, k, l, m, n, o, p
Hadley, S. W., Erickson III, D. J., Hernandez, J. L., Broniak, C. T., and Blasing, T. J.: Responses of energy use to climate change: A climate modeling study, Geophys. Res. Lett., 33, 2–5, https://doi.org/10.1029/2006GL026652, 2006. a
Haghighi, E., Shahraeeni, E., Lehmann, P., and Or, D.: Evaporation rates across a convective air boundary layer are dominated by diffusion, Water Resour. Res., 49, 1602–1610, https://doi.org/10.1002/wrcr.20166, 2013. a, b
Harman, I., Best, M. J., and Belcher, S. E.: Radiative exchange in an urban street canyon, Bound.-Lay. Meteorol., 110, 301–316, 2003. a, b, c
Harshan, S., Roth, M., Velasco, E., and Demuzere, M.: Evaluation of an urban land surface scheme over a tropical suburban neighborhood, Theor. Appl. Climatol., 133, 867–886, https://doi.org/10.1007/s00704-017-2221-7, 2017. a, b, c, d, e, f, g, h, i, j, k, l, m, n, o, p, q
Hillel, D.: Environmental Soil Physics: Fundamentals, Applications, and Environmental Considerations, Academic Press, London, UK, 1998. a
Holst, C. C., Tam, C.-Y., and Chan, J. C. L.: Sensitivity of urban rainfall to anthropogenic heat flux: A numerical experiment, Geophys. Res. Lett., 43, 2240–2248, https://doi.org/10.1002/2015GL067628.Received, 2016. a
Höppe, P.: The physiological equivalent temperature – a universal index for the biometeorological assessment of the thermal environment, Int. J. Biometeorol., 43, 71–75, https://doi.org/10.1007/s004840050118, 1999. a
Hu, Z. and Islam, S.: Prediction of ground surface temperature and soil moisture content by the force restore-method, Water Resour. Res., 31, 2531–2539, 1995. a, b
Huang, C.-W., Domec, J.-C., Ward, E. J., Duman, T., Manoli, G., Parolari, A. J., and Katul, G. G.: The effect of plant water storage on water fluxes within the coupled soil-plant system, New Phytol., 213, 1093–1106, https://doi.org/10.1111/nph.14273, 2017. a
Iio, A., Hikosaka, K., Anten, N. P., Nakagawa, Y., and Ito, A.: Global dependence of field-observed leaf area index in woody species on climate: A systematic review, Global Ecol. Biogeogr., 23, 274–285, https://doi.org/10.1111/geb.12133, 2014. a
IPCC: Climate Change 2014, Synthesis Report, Summary for Policymakers, 2014. a
Ivanov, V. Y., Bras, R. L., and Vivoni, E. R.: Vegetation-hydrology dynamics in complex terrain of semiarid areas: 1. A mechanistic approach to modeling dynamic feedbacks, Water Resour. Res., 44, W03429, https://doi.org/10.1029/2006WR005588, 2008. a, b
Jochner, S., Alves-Eigenheer, M., Menzel, A., and Morellato, L. P. C.: Using phenology to assess urban heat islands in tropical and temperate regions, Int. J. Climatol., 33, 3141–3151, https://doi.org/10.1002/joc.3651, 2013. a
Kattge, J., Knorr, W., Raddatz, T., and Wirth, C.: Quantifying photosynthetic capacity and its relationship to leaf nitrogen content for global-scale terrestrial biosphere models, Glob. Change Biol., 15, 976–991, https://doi.org/10.1111/j.1365-2486.2008.01744.x, 2009. a
Kent, C. W., Grimmond, S., and Gatey, D.: Aerodynamic roughness parameters in cities: Inclusion of vegetation, J. Wind Eng. Ind. Aerod., 169, 168–176, https://doi.org/10.1016/j.jweia.2017.07.016, 2017. a
Konarska, J., Holmer, B., Lindberg, F., and Thorsson, S.: Influence of vegetation and building geometry on the spatial variations of air temperature and cooling rates in a high-latitude city, Int. J. Climatol., 36, 2379–2395, https://doi.org/10.1002/joc.4502, 2016. a
Krayenhoff, E. S., Christen, A., Martilli, A., and Oke, T. R.: A Multi-layer Radiation Model for Urban Neighbourhoods with Trees, Bound.-Lay. Meteorol., 151, 139–178, https://doi.org/10.1007/s10546-013-9883-1, 2014. a, b
Krayenhoff, E. S., Santiago, J.-L., Martilli, A., Christen, A., and Oke, T.: Parametrization of Drag and Turbulence for Urban Neighbourhoods with Trees, Bound.-Lay. Meteorol., 156, 157–189, https://doi.org/10.1007/s10546-015-0028-6, 2015. a
Kusaka, H., Kondo, H., and Kikegawa, Y.: A simple single-layer urban canopy model for amtospheric models: Comparison with multi-layer and slab models, Bound.-Lay. Meteorol., 101, 329–358, 2001. a, b
Lawrence, D. M., Levis, S., Zeng, X., Flanner, M. G., Bonan, G. B., Oleson, K. W., Swenson, S. C., Lawrence, D. M., Sakaguchi, K., Slater, A. G., Yang, Z.-L., Lawrence, P. J., and Thornton, P. E.: Parameterization improvements and functional and structural advances in Version 4 of the Community Land Model, J. Adv. Model. Earth Syst., 3, M03001, https://doi.org/10.1029/2011MS00045, 2011. a
Lee, H. S., Matthews, C. J., Braddock, R. D., Sander, G. C., and Gandola, F.: A MATLAB method of lines template for transport equations, Environ. Model. Softw., 19, 603–614, https://doi.org/10.1016/j.envsoft.2003.08.017, 2004. a
Lemonsu, A., Masson, V., Shashua-Bar, L., Erell, E., and Pearlmutter, D.: Inclusion of vegetation in the Town Energy Balance model for modelling urban green areas, Geosci. Model Dev., 5, 1377–1393, https://doi.org/10.5194/gmd-5-1377-2012, 2012. a, b
Leuning, R.: A critical appraisal of a combined stomatal- photosynthesis model for C3 plants, Plant Cell Environ., 18, 357–364, 1995. a
Leuning, R., Kelliher, F. M., Pury, D. G. G., and Schulze, E.-D.: Leaf nitrogen, photosynthesis, conductance and transpiration: Scaling from leaves to canopies, Plant, Cell Environ., 18, 1183–1200, 1995. a
Li, D. and Bou-Zeid, E.: Synergistic Interactions between Urban Heat Islands and Heat Waves : The Impact in Cities Is Larger than the Sum of Its Parts, J. Appl. Meteorol. Climatol., 52, 2051–2064, https://doi.org/10.1175/JAMC-D-13-02.1, 2013. a
Li, D., Bou-Zeid, E., and Oppenheimer, M.: The effectiveness of cool and green roofs as urban heat island mitigation strategies, Environ. Res. Lett., 9, 055002, https://doi.org/10.1088/1748-9326/9/5/055002, 2014. a
Lim, H. S. and Lu, X. X.: Sustainable urban stormwater management in the tropics : An evaluation of Singapore's ABC Waters Program, J. Hydrol., 538, 842–862, https://doi.org/10.1016/j.jhydrol.2016.04.063, 2016. a, b
Lindberg, F., Holmer, B., and Thorsson, S.: SOLWEIG 1.0 – Modelling spatial variations of 3D radiant fluxes and mean radiant temperature in complex urban settings, Int. J. Biometeorol., 52, 697–713, https://doi.org/10.1007/s00484-008-0162-7, 2008. a, b
Liu, X., Li, X.-x., Harshan, S., Roth, M., and Velasco, E.: Evaluation of an urban canopy model in a tropical city : the role of tree evapotranspiration, Environ. Res. Letters, 12, 094008, https://doi.org/10.1088/1748-9326/aa7ee7, 2017. a, b, c, d
Macdonald, R. W., Griffiths, R. F., and Hall, D. J.: An improved method for the estimation of surface roughness of obstacle arrays, Atmos. Environ., 32, 1857–1864, 1998. a
Mahat, V., Tarboton, D. G., and Molotch, N. P.: Testing above- and below-canopy representations of turbulent fluxes in an energy balance snowmelt model, Water Resour. Res., 49, 1107–1122, https://doi.org/10.1002/wrcr.20073, 2013. a, b
Mahfouf, J.-F. and Jacquemin, B.: A study of rainfall interception using a land surface parameterization for mesoscale meteorological models, J. Appl. Meteorol., 28, 1282–1302, 1989. a, b
Manickathan, L., Defraeye, T., Allegrini, J., Derome, D., and Carmeliet, J.: Parametric study of the influence of environmental factors and tree properties on the transpirative cooling effect of trees, Agr. Forest Meteorol., 248, 259–274, https://doi.org/10.1016/j.agrformet.2017.10.014, 2018. a, b
Manoli, G., Ivanov, V. Y., and Fatichi, S.: Dry-Season Greening and Water Stress in Amazonia: The Role of Modeling Leaf Phenology, J. Geophys. Res.-Biogeo., 123, 1909–1926, https://doi.org/10.1029/2017JG004282, 2018. a
Manoli, G., Fatichi, S., Schläpfer, M., Yu, K., Crowther, T. W., Meili, N., Burlando, P., Katul, G. G., and Bou-Zeid, E.: Magnitude of urban heat islands largely explained by climate and population, Nature, 573, 55–60, https://doi.org/10.1038/s41586-019-1512-9, 2019. a
Mascart, P., Noilhan, J., and Giordani, H.: A Modified Parameterization of Flux-Profile Relationships in the Surface Layer Using Different Roughness Length Values for Heat and Momentum, Bound.-Lay. Meteorol., 72, 331–344, 1995. a
Masson, V.: A physically-based scheme for the urban energy budget in atmospheric models, Bound.-Lay. Meteorol., 94, 357–397, 2000. a, b, c, d, e, f
Masson, V., Le Moigne, P., Martin, E., Faroux, S., Alias, A., Alkama, R., Belamari, S., Barbu, A., Boone, A., Bouyssel, F., Brousseau, P., Brun, E., Calvet, J.-C., Carrer, D., Decharme, B., Delire, C., Donier, S., Essaouini, K., Gibelin, A.-L., Giordani, H., Habets, F., Jidane, M., Kerdraon, G., Kourzeneva, E., Lafaysse, M., Lafont, S., Lebeaupin Brossier, C., Lemonsu, A., Mahfouf, J.-F., Marguinaud, P., Mokhtari, M., Morin, S., Pigeon, G., Salgado, R., Seity, Y., Taillefer, F., Tanguy, G., Tulet, P., Vincendon, B., Vionnet, V., and Voldoire, A.: The SURFEXv7.2 land and ocean surface platform for coupled or offline simulation of earth surface variables and fluxes, Geosci. Model Dev., 6, 929–960, https://doi.org/10.5194/gmd-6-929-2013, 2013. a
Matzarakis, A., Rutz, F., and Mayer, H.: Modelling radiation fluxes in simple and complex environments – application of the RayMan model, Int. J. Biometeorol., 51, 323–334, https://doi.org/10.1007/s00484-009-0261-0, 2007. a, b
Matzarakis, A., Rutz, F., and Mayer, H.: Modelling radiation fluxes in simple and complex environments: basics of the RayMan model, Int. J. Biometeorol., 54, 131–139, https://doi.org/10.1007/s00484-009-0261-0, 2010. a
Meili, N. and Fatichi, S.: Urban Tethys-Chloris (UT&C v1.0) with the possibility of sub-hourly time steps, Zenodo, https://doi.org/10.5281/zenodo.3548147, 2019. a
Middel, A., Chhetri, N., and Quay, R.: Urban forestry and cool roofs: Assessment of heat mitigation strategies in Phoenix residential neighborhoods, Urban For. Urban Gree., 14, 178–186, https://doi.org/10.1016/j.ufug.2014.09.010, 2015. a
Mirfenderesgi, G., Bohrer, G., Matheny, A., Fatichi, S., Frasson, R. P. D. M., and Schafer, K. V. R.: Tree-level hydrodynamic approach for modeling aboveground water storage and stomatal conductance illuminates the effects of tree hydraulic strategy, J. Geophys. Res.-Biogeo., 121, 1792–1813, https://doi.org/10.1002/2016JG003467, 2016. a
Mitchell, D., Heaviside, C., Vardoulakis, S., Huntingford, C., Masato, G., P Guillod, B., Frumhoff, P., Bowery, A., Wallom, D., and Allen, M.: Attributing human mortality during extreme heat waves to anthropogenic climate change, Environ. Res. Lett., 11, 074006, https://doi.org/10.1088/1748-9326/11/7/074006, 2016. a
Monteith, J. L.: Principles of Environmental Physics, Edward Arnold, London, 1973. a
Mora, C., Dousset, B., Caldwell, I. R., Powell, F. E., Geronimo, R. C., Bielecki, C. R., Counsell, C. W. W., Dietrich, B. S., Johnston, E. T., Louis, L. V., Lucas, M. P., Mckenzie, M. M., Shea, A. G., Tseng, H., Giambelluca, T. W., Leon, L. R., Hawkins, E., and Trauernicht, C.: Global risk of deadly heat, Nat. Clim. Change, 7, 501–506, https://doi.org/10.1038/NCLIMATE3322, 2017. a
Ng, K. S. T., Sia, A., Ng, M. K., Tan, C. T., Chan, H. Y., Tan, C. H., Rawtaer, I., Feng, L., Mahendran, R., Larbi, A., Kua, E. H., and Ho, R. C.: Effects of horticultural therapy on asian older adults: A randomized controlled trial, Int. J. Environ. Res. Pub. He., 15, 1–14, https://doi.org/10.3390/ijerph15081705, 2018. a
Nice, K. A., Coutts, A. M., and Tapper, N. J.: Development of the VTUF-3D v1.0 urban micro-climate model to support assessment of urban vegetation influences on human thermal comfort, Urban Climate, 24, 1052–1076, https://doi.org/10.1016/j.uclim.2017.12.008, 2018. a, b, c, d, e, f, g, h, i, j, k, l, m, n
Noilhan, J. and Planton, S.: A simple parameterization of land surface processes for meteorological models, Mon. Weather Rev., 117, 536–549, 1989. a
Nowak, D. J. and Crane, D. E.: Carbon storage and sequestration by urban trees in the USA, Environ. Pollut., 116, 381–389, 2002. a
Núnez, C. M., Varas, E. A., and Meza, F. J.: Modelling soil heat flux, Theor. Appl. Climatol., 100, 251–260, https://doi.org/10.1007/s00704-009-0185-y, 2010. a
Oleson, K. W., Lawrence, D. M., Bonan, G. B., Drewniak, B., Huang, M., Kowen, C. D., Levis, S., Li, F., Riley, W. J., Subin, Z. M., Swenson, S. C., and Thornton, P. E.: Technical Description of version 4.5 of the Community Land Model (CLM), Tech. Rep. NCAR/TN-503+STR, Natl. Cent. for Atmos. Res., Boulder, Colorado, 2013. a
Park, S.-U. and Lee, S.-H.: A Vegetated Urban Canopy Model for Meteorological and Environmental Modelling, Bound.-Lay. Meteorol., 126, 73–102, https://doi.org/10.1007/s10546-007-9221-6, 2008. a, b, c
Paschalis, A., Fatichi, S., Pappas, C., and Or, D.: Covariation of vegetation and climate constrains present and future T/ET variability, Environ. Res. Lett, 13, 104012, https://doi.org/10.1088/1748-9326/aae267, 2018. a
Pataki, D. E., Carreiro, M. M., Cherrier, J., Grulke, N. E., Jennings, V., Pincetl, S., Pouyat, R. V., Whitlow, T. H., and Zipperer, W. C.: Coupling biogeochemical cycles in urban environments: Ecosystem services, green solutions, and misconceptions, Front. Ecol. Environ., 9, 27–36, https://doi.org/10.1890/090220, 2011. a
Ramamurthy, P. and Bou-Zeid, E.: Contribution of impervious surfaces to urban evaporation, Water Resour. Res., 50, 2889–2902, https://doi.org/10.1111/j.1752-1688.1969.tb04897.x, 2014. a
Ramamurthy, P., Bou-Zeid, E., Smith, J. A., Wang, Z., Baeck, M. L., Saliendra, N. Z., Hom, J. L., and Welty, C.: Influence of subfacet heterogeneity and material properties on the urban surface energy budget, J. Appl. Meteorol. Clim., 53, 2114–2129, https://doi.org/10.1175/JAMC-D-13-0286.1, 2014. a
Redon, E. C., Lemonsu, A., Masson, V., Morille, B., and Musy, M.: Implementation of street trees within the solar radiative exchange parameterization of TEB in SURFEX v8.0, Geosci. Model Dev., 10, 385–411, https://doi.org/10.5194/gmd-10-385-2017, 2017. a, b
Richards, L. A.: Capillary conduction of liquids through porous mediums, Physics, 1, 318–333, 1931. a, b
Roth, M.: Review of urban climate research in (sub)tropical regions, Int. J. Climate, 27, 1859–1873, https://doi.org/10.1002/joc, 2007. a
Roth, M., Jansson, C., and Velasco, E.: Multi-year energy balance and carbon dioxide fluxes over a residential neighbourhood in a tropical city, Int. J. Climatol., 37, 2679–2698, https://doi.org/10.1002/joc.4873, 2016. a, b, c, d, e, f, g, h, i, j, k
Rowley, F. B. and Eckley, W. A.: Surface coefficients as affected by wind direction, ASHREA Trans., 39, 33–46, 1932. a
Rowley, F. B., Algren, A. B., and Blackshaw, J.: Surface conductance as affected by air velocity, temperature and character of surface, ASHREA Trans., 36, 429–446, 1930. a
Rutter, A. J., Kershaw, K. A., Robins, P. C., and Morton, A. J.: A predictive model of rainfall interception in forests. 1. Derivation of the model from observation in a plantation of Corsican pine, Agr. Meteorol., 9, 367–384, 1971. a, b
Rutter, A. J., Morton, A. J., and Robins, P. C.: A predictive model of rainfall interception in forests. 2. Generalization of model and comparison with observations in some coniferous and hardwood stands, J. Appl. Ecol., 12, 367–380, 1975. a
Ryu, Y.-H., Bou-Zeid, E., Wang, Z.-H., and Smith, J. A.: Realistic Representation of Trees in an Urban Canopy Model, Bound.-Lay. Meteorol., 159, 193–220, https://doi.org/10.1007/s10546-015-0120-y, 2016. a, b, c, d, e, f, g
Sailor, D. J. and Lu, L.: A top-down methodology for developing diurnal and seasonal anthropogenic heating profiles for urban areas, Atmos. Environ., 38, 2737–2748, https://doi.org/10.1016/j.atmosenv.2004.01.034, 2004. a
Sailor, D. J., Georgescu, M., Milne, J. M., and Hart, M. A.: Development of a national anthropogenic heating database with an extrapolation for international cities, Atmos. Environ., 118, 7–18, https://doi.org/10.1016/j.atmosenv.2015.07.016, 2015. a
Salmond, J. A., Tadaki, M., Vardoulakis, S., Arbuthnott, K., Coutts, A., Demuzere, M., Dirks, K. N., Heaviside, C., Lim, S., Macintyre, H., Mcinnes, R. N., and Wheeler, B. W.: Health and climate related ecosystem services provided by street trees in the urban environment, Environ. Health, 15, S36, https://doi.org/10.1186/s12940-016-0103-6, 2016. a
Saxton, K. E. and Rawls, W. J.: Soil Water Characteristic Estimates by Texture and Organic Matter for Hydrologic Solutions, Soil Sci. Soc. Am. J., 70, 1569–1578, https://doi.org/10.2136/sssaj2005.0117, 2006. a
Schenk, H. J. and Jackson, R. B.: The global biogeography of roots, Ecol. Monogr., 72, 311–328, 2002. a
Sellers, P. J., Dickinson, R. E., Randall, D. A., Betts, A. K., Hall, F. G., Berry, J. A., Collatz, G. J., Denning, A. S., Mooney, H. A., Nobre, C. A., Sato, N., Field, C. B., and Henderson-Sellers, A.: Modeling the Exchanges of Energy, Water and Carbon Between Continents and the Atmosphere, Science, 275, 502–509, 1997. a
Shuttleworth, W. J.: Terrestrial hydrometeorology, John Wiley & Sons, Ltd, 2012. a
Shuttleworth, W. J. and Gurney, R. J.: The theoretical relationship between foliage temperature and canopy resistance in sparse crops, Q. J. Roy. Meteor. Soc., 116, 497–519, 1990. a
Skamarock, W. C., Klemp, J. B., Dudhia, J., Gill, D. O., Barker, D. M., Duda, M. G., Huang, X.-y., Wang, W., and Powers, J. G.: A Description of the Advanced Research WRF Version 3, NCAR Tech Note, 488–494, https://doi.org/10.5065/D6DZ069T, 2008. a
Song, J. and Wang, Z. H.: Interfacing the Urban Land–Atmosphere System Through Coupled Urban Canopy and Atmospheric Models, Bound.-Lay. Meteorol., 154, 427–448, https://doi.org/10.1007/s10546-014-9980-9, 2015. a, b, c, d, e, f
Sparrow, E. and Cess, R. D.: Radiation Heat Transfer, chap. 3–4, Appendices A & B, Thermal Science Series, Brooks/Cole, 1970. a, b
Stavropulos-Laffaille, X., Chancibault, K., Brun, J.-M., Lemonsu, A., Masson, V., Boone, A., and Andrieu, H.: Improvements to the hydrological processes of the Town Energy Balance model (TEB-Veg, SURFEX v7.3) for urban modelling and impact assessment, Geosci. Model Dev., 11, 4175–4194, https://doi.org/10.5194/gmd-11-4175-2018, 2018. a, b
Stewart, I. D. and Oke, T. R.: Local climate zones for urban temperature studies, B. Am. Meteorol. Soc., 93, 1879–1900, https://doi.org/10.1175/BAMS-D-11-00019.1, 2012. a, b, c
Templeton, N. P., Vivoni, E. R., Wang, Z. H., and Schreiner-McGraw, A. P.: Quantifying Water and Energy Fluxes Over Different Urban Land Covers in Phoenix, Arizona, J. Geophys. Res.-Atmos., 123, 2111–2128, https://doi.org/10.1002/2017JD027845, 2018. a
United Nations: World Urbanization Prospects, 2014. a
van Genuchten, M. T.: A closed-form equation for predicting the hydraulic conductivity of unsaturated soils, Soil Sci. Soc. Am. J., 44, 892–898, 1980. a
Velasco, E., Roth, M., Tan, S. H., Quak, M., Nabarro, S. D. A., and Norford, L.: The role of vegetation in the CO2 flux from a tropical urban neighbourhood, Atmos. Chem. Phys., 13, 10185–10202, https://doi.org/10.5194/acp-13-10185-2013, 2013. a, b, c, d, e, f, g, h, i
Volo, T. J., Vivoni, E. R., Martin, C. A., Earl, S., and Ruddell, B. L.: Modelling soil moisture, water partitioning, and plant water stress under irrigated conditions in desert Urban areas, Ecohydrology, 7, 1297–1313, https://doi.org/10.1002/eco.1457, 2014. a
Wang, C., Wang, Z.-H., and Yang, J.: Cooling Effect of Urban Trees on the Built Environment of Contiguous United States, Earth's Future, 1066–1081, https://doi.org/10.1029/2018EF000891, 2018. a
Wang, C., Wang, Z.-H., and Yang, J.: Urban water capacity: Irrigation for heat mitigation, Comput. Environ. Urban, 78, 101397, https://doi.org/10.1016/j.compenvurbsys.2019.101397, 2019. a
Wang, Y.-P. and Leuning, R.: A two-leaf model for canopy conductance, photosynthesis and portioning of available energy I: Model description and comparison with a multi-layered model, Agr. Forest Meteorol., 91, 89–111, 1998. a
Wang, Z.-H.: Geometric effect of radiative heat exchange in concave structure with application to heating of steel I-sections in fire, Int. J. Heat Mass. Tran., 53, 997–1003, https://doi.org/10.1016/j.ijheatmasstransfer.2009.11.013, 2010. a
Wang, Z.-F.: Monte Carlo simulations of radiative heat exchange in a street canyon with trees, Solar Energy, 110, 704–713, https://doi.org/10.1016/j.solener.2014.10.012, 2014. a, b, c
Wang, Z.-H., Bou-Zeid, E., and Smith, J. A.: A Spatially-Analytical Scheme for Surface Temperatures and Conductive Heat Fluxes in Urban Canopy Models, Bound.-Lay. Meteorol., 138, 171–193, https://doi.org/10.1007/s10546-010-9552-6, 2011. a
Wang, Z.-H., Bou-Zeid, E., and Smith, J. A.: A coupled energy transport and hydrological model for urban canopies evaluated using a wireless sensor network, Q. J. Roy.Meteor. Soc., 139, 1643–1657, https://doi.org/10.1002/qj.2032, 2013. a, b, c, d, e, f, g, h
Ward, H. C., Kotthaus, S., Järvi, L., and Grimmond, C. S.: Surface Urban Energy and Water Balance Scheme (SUEWS): Development and evaluation at two UK sites, Urban Climate, 18, 1–32, https://doi.org/10.1016/j.uclim.2016.05.001, 2016. a, b, c
Willmott, C. J.: Some Comments on the Evaluation of Model Performance, B. Am. Meteorol. Soc., 63, 1309–1313, https://doi.org/10.1175/1520-0477(1982)063<1309:SCOTEO>2.0.CO;2, 1982. a
Wouters, H., Demuzere, M., Ridder, K. D., and Van Lipzig, N. P.: The impact of impervious water-storage parametrization on urban climate modelling, Urban Climate, 11, 24–50, https://doi.org/10.1016/j.uclim.2014.11.005, 2015. a, b, c
Wouters, H., Demuzere, M., Blahak, U., Fortuniak, K., Maiheu, B., Camps, J., Tielemans, D., and van Lipzig, N. P. M.: The efficient urban canopy dependency parametrization (SURY) v1.0 for atmospheric modelling: description and application with the COSMO-CLM model for a Belgian summer, Geosci. Model Dev., 9, 3027–3054, https://doi.org/10.5194/gmd-9-3027-2016, 2016. a
Wullschleger, S. D.: Biochemical Limitations to Carbon Assimilation in C3 Plants—A Retrospective Analysis of the A/C i Curves from 109 Species, J. Exp. Bot., 44, 907–920, https://doi.org/10.1093/jxb/44.5.907, 1993. a
Yang, J. and Wang, Z. H.: Planning for a sustainable desert city: The potential water buffering capacity of urban green infrastructure, Landscape Urban Plan., 167, 339–347, https://doi.org/10.1016/j.landurbplan.2017.07.014, 2017. a
Zhang, X., Friedl, M. A., Schaaf, C. B., Strahler, A. H., and Schneider, A.: The footprint of urban climates on vegetation phenology, Geophys. Res. Lett., 31, 10–13, https://doi.org/10.1029/2004GL020137, 2004. a
Zhou, S., Duursma, R. A., Medlyn, B. E., Kelly, J. W., and Prentice, I. C.: How should we model plant responses to drought? An analysis of stomatal and non-stomatal responses to water stress, Agr. Forest Meteorol., 182–183, 204–214, https://doi.org/10.1016/j.agrformet.2013.05.009, 2013. a
Ziegler, A. D., Terry, J. P., Oliver, G. J., Friess, D. A., Chuah, C. J., Chow, W. T., and Wasson, R. J.: Increasing Singapore's resilience to drought, Hydrol. Proc., 28, 4543–4548, https://doi.org/10.1002/hyp.10212, 2014. a