the Creative Commons Attribution 4.0 License.
the Creative Commons Attribution 4.0 License.
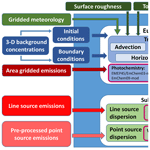
The Eulerian urban dispersion model EPISODE – Part 2: Extensions to the source dispersion and photochemistry for EPISODE–CityChem v1.2 and its application to the city of Hamburg
Sam-Erik Walker
Sverre Solberg
Martin O. P. Ramacher
This paper describes the CityChem extension of the Eulerian urban dispersion model EPISODE. The development of the CityChem extension was driven by the need to apply the model in largely populated urban areas with highly complex pollution sources of particulate matter and various gaseous pollutants. The CityChem extension offers a more advanced treatment of the photochemistry in urban areas and entails specific developments within the sub-grid components for a more accurate representation of dispersion in proximity to urban emission sources. Photochemistry on the Eulerian grid is computed using a numerical chemistry solver. Photochemistry in the sub-grid components is solved with a compact reaction scheme, replacing the photo-stationary-state assumption. The simplified street canyon model (SSCM) is used in the line source sub-grid model to calculate pollutant dispersion in street canyons. The WMPP (WORM Meteorological Pre-Processor) is used in the point source sub-grid model to calculate the wind speed at plume height. The EPISODE–CityChem model integrates the CityChem extension in EPISODE, with the capability of simulating the photochemistry and dispersion of multiple reactive pollutants within urban areas. The main focus of the model is the simulation of the complex atmospheric chemistry involved in the photochemical production of ozone in urban areas. The ability of EPISODE–CityChem to reproduce the temporal variation of major regulated pollutants at air quality monitoring stations in Hamburg, Germany, was compared to that of the standard EPISODE model and the TAPM (The Air Pollution Model) air quality model using identical meteorological fields and emissions. EPISODE–CityChem performs better than EPISODE and TAPM for the prediction of hourly NO2 concentrations at the traffic stations, which is attributable to the street canyon model. Observed levels of annual mean ozone at the five urban background stations in Hamburg are captured by the model within ±15 %. A performance analysis with the FAIRMODE DELTA tool for air quality in Hamburg showed that EPISODE–CityChem fulfils the model performance objectives for NO2 (hourly), O3 (daily max. of the 8 h running mean) and PM10 (daily mean) set forth in the Air Quality Directive, qualifying the model for use in policy applications. Envisaged applications of the EPISODE–CityChem model are urban air quality studies, emission control scenarios in relation to traffic restrictions and the source attribution of sector-specific emissions to observed levels of air pollutants at urban monitoring stations.
- Article
(13349 KB) - Companion paper
-
Supplement
(919 KB) - BibTeX
- EndNote
Air quality (AQ) modelling plays an important role by assessing the air pollution situation in urban areas and by supporting the development of guidelines for efficient air quality planning, as highlighted in the current Air Quality Directive (AQD) of the European Commission (EC, 2008). The main air pollution issues in European cities are the human health impacts of exposure to particulate matter (PM), nitrogen dioxide (NO2) and ozone (O3), while the effects of air pollution due to sulfur dioxide (SO2), carbon monoxide (CO), lead (Pb) and benzene have been reduced during the last 2 decades due to emission abatement measures. Tropospheric (ground-level) ozone is a secondary pollutant generated in in photochemical reaction cycles involving two classes of precursor compounds, i.e. nitrogen oxides and volatile organic compounds (VOCs), initiated by the reaction of the hydroxyl (OH) radical with organic molecules. For health protection, a maximum daily 8 h mean threshold for ozone (120 µg m−3) is specified as a target value in the European Union, which should not be exceeded at any AQ monitoring station on more than 25 d yr−1. However, about 15 % of the population living in urban areas is exposed to ozone concentrations above the European Union (EU) target value (EEA, 2015). Traffic is a major source of nitrogen oxides () and highly contributes to the population exposure to ambient NO2 concentrations in urban areas because these emissions occur close to the ground and are distributed across densely populated areas. Urban emissions of ozone precursors are transported by local and/or regional air mass flows towards suburban and rural areas, which can be impacted by O3 pollution episodes (Querol et al., 2016).
Eulerian chemistry-transport model (CTM) systems using numerical methods for solving photochemistry (including chemical reaction schemes with varying degrees of detail) have mainly been used for regional-scale air quality studies. Recent nested model approaches using regional CTM systems have been applied to capture pollution processes from the continental scale to the local scale using between 1 and 5 km resolution and a temporal resolution of 1 h for the innermost domain (e.g. Borge et al., 2014; Karl et al., 2015; Petetin et al., 2015; Valverde et al., 2016). Regional AQ models can give a reliable representation of O3 concentrations in the urban background, but due to their limitation in resolving the near-field dispersion of emission sources and photochemistry at the sub-kilometre scale, i.e. in street canyons, around industrial stacks and on the neighbourhood level, they cannot provide the information needed by urban policymakers for population exposure mapping, city planning and the assessment of abatement measures.
Urban-scale AQ models overcome the limitation inherent in regional-scale models by taking into account details of the urban topography, wind flow field characteristics, land use information and the geometry of local pollution sources. The urban AQ model EPISODE developed at the Norwegian Institute for Air Research (NILU) is a 3-D Eulerian grid model that operates as a CTM, offline coupled with a numerical weather prediction (NWP) model. EPISODE is typically applied with a horizontal resolution of 1 × 1 km2 over an entire city with domains of up to 2500 km2 in size. The Eulerian grid component of EPISODE simulates advection, vertical and/or horizontal diffusion, background transport across the model domain boundaries, and photochemistry. Several sub-grid-scale modules are embedded in EPISODE to represent emissions (line source and point sources), Gaussian dispersion and local photochemistry. In particular, the model allows the user to retrieve concentrations at the sub-grid scale in specified locations of the urban area. Moreover, the EPISODE model is an integral part of the operational Air Quality Information System AirQUIS 2006 (Slørdal et al., 2008).
Part one (Hamer et al., 2019) of this two-part article series provides a detailed description of the EPISODE model system, including the physical processes for atmospheric pollutant transport, the photo-stationary-state (PSS) approximation, the involvement of nitric oxide (NO), NO2 and O3, sub-grid components, and the interaction between the Eulerian grid and the sub-grid processing of pollutant concentrations. Part one examines the application of EPISODE to air quality scenarios in the Nordic winter setting. During wintertime in northern Europe, the PSS assumption is a rather good approximation of the photochemical conversion occurring close to the emission sources. However, when the solar ultraviolet (UV) radiation is stronger, in particular during summer months or at more southerly locations, net ozone formation may take place in urban areas at a certain distance from the main local emission sources (Baklanov et al., 2007). EPISODE in its routine application does not allow for the treatment of photochemistry involving VOCs and other reactive gases leading to the photochemical formation of ozone.
In this part, the features of the CityChem extension for treating the complex atmospheric chemistry in urban areas and specific developments within the sub-grid components for a more accurate representation of near-field dispersion in proximity to urban emission sources are described. Atmospheric chemistry on an urban scale is complex due to the large spatial variations of input from anthropogenic emissions. VOCs related to emissions from traffic are involved in chemical conversion in urban areas. Therefore, it has become necessary to simulate a large number of chemical interactions involving NOx, O3, VOCs, SO2 and secondary pollutants. In order to use comprehensive photochemical schemes in urban AQ models involving VOC interactions, the highest priority for the initial development was to reduce the number of compounds and reactions to a minimum, while maintaining the essential and most important aspects of chemical reactions taking place in the urban atmosphere on the relevant space scales and timescales.
CityChem offers a more advanced treatment for the photochemistry of multiple gaseous pollutants on the Eulerian grid, as well as for dispersion close to point emission sources (e.g. industrial stacks) and line emission sources (open roads and streets).
-
Photochemistry on the Eulerian grid uses a numerical chemistry solver. The available chemistry schemes include (1) EMEP45 (Walker et al., 2003), which resulted from an appropriate reduction of the former EMEP (European Monitoring and Evaluation Programme) chemistry scheme (Simpson, 1995); (2) EmChem03-mod, with updated reaction equations and coefficients compared to EMEP45; (3) and EmChem09-mod, which is similar to the current EMEP chemistry mechanism (EmChem09; Simpson et al., 2012). EmChem09-mod enables the simulation of biogenic VOCs, such as isoprene and monoterpenes, emitted from urban vegetation.
-
Modifications of the photochemistry in the sub-grid components have replaced the PSS assumption with the EP10-Plume scheme, a compact scheme including inorganic reactions and the photochemical degradation of formaldehyde, using a numerical solver.
-
Modifications of the line source emission model have been made to compute receptor point concentrations in street canyons. A simplified street canyon model (SSCM) is implemented to account for pollutant transfer along streets, including a parameterization of mass transfer within a simplified building geometry at street level.
-
Modifications to the plume rise from elevated point sources allow for a more accurate computation of the plume trajectories. The Meteorological Pre-Processor (WMPP) of the Weak-wind Open Road Model (WORM) is utilized in the CityChem extension to calculate the wind speed at plume height.
Although computational fluid dynamics (CFD) models can be used to solve for local-scale phenomena along point and line emission sources, they are limited to localized applications and are not appropriate for the simulation of dispersion across complex urban areas. In addition, the simulation of the chemical conversions of reactive pollutants using CFD models requires a large amount of computational time (Sanchez et al., 2016).
The EPISODE–CityChem model, which is based on the core of the EPISODE model, integrates the CityChem extension into an urban CTM system. This paper gives a model description of EPISODE–CityChem version 1.2. In the typical setup, EPISODE–CityChem uses downscaled meteorological fields generated by the meteorological component of the coupled meteorology–chemistry model TAPM (The Air Pollution Model; Hurley, 2008; Hurley et al., 2005). TAPM is a prognostic model which uses the complete equations governing the behaviour of the atmosphere and the dispersion of air pollutants. EPISODE–CityChem is coupled offline with the regional-scale air quality model CMAQ (Community Multiscale Air Quality; Byun et al., 1999; Byun and Schere, 2006; Appel et al., 2013) using hourly varying pollutant concentrations at the lateral and vertical boundaries from CMAQ as initial and boundary concentrations.
EPISODE–CityChem has the capability to simulate the photochemical transformation of multiple reactive pollutants along with atmospheric diffusion to produce concentration fields for the entire city on a horizontal resolution of 100 m or even finer and a vertical resolution of 24 layers up to 4000 m of height. The possibility to get a complete picture of the urban area with respect to reactive pollutant concentrations, but also information enabling exposure calculations in highly populated areas close to road traffic line sources and industrial point sources with high spatial resolution, turns EPISODE–CityChem into a valuable tool for urban air quality studies, health risk assessment, sensitivity analysis of sector-specific emissions, and the assessment of local and regional emission abatement policy options.
The paper is organized as follows: Sect. 2 gives an overview of EPISODE–CityChem and a detailed description of the photochemical reaction schemes and modifications of near-source dispersion in the sub-grid components. Section 3 presents tests of the various modules in the CityChem extension. Section 4 describes the application of EPISODE–CityChem within a nested model chain for simulating the air quality and atmospheric chemistry in the city of Hamburg. We assess the performance of EPISODE–CityChem in reproducing the temporal and spatial variation of air pollutant concentrations against data from urban monitoring stations. Model results from EPISODE–CityChem are compared (1) to results from the standard EPISODE model to quantify the total effect of the new implementations and (2) to results from TAPM, acting as reference model for air pollution modelling on the urban scale. Section 5 outlines plans for the future development of the EPISODE–CityChem model, addressing the need for more sophisticated photochemistry, treatment of aerosol formation on an urban scale and further improvements of the source dispersion. A list of acronyms and abbreviations used in this work is given in Appendix A.
EPISODE consists of a 3-D Eulerian grid CTM that interacts with a sub-grid Gaussian dispersion model for the dispersion of pollutants emitted from both line and point sources. We refer to part one (Hamer et al., 2019) for a technical description of the model. The standard EPISODE model simulates the emission and transport of NOx, as well as fine particulate matter with PM2.5 (particles with diameter less than 2.5 µm) and PM10 (particles with diameter less than 10 µm) in urban areas, with the specific aim of predicting concentrations of NO2, which is the major pollutant in many cities of northern Europe.
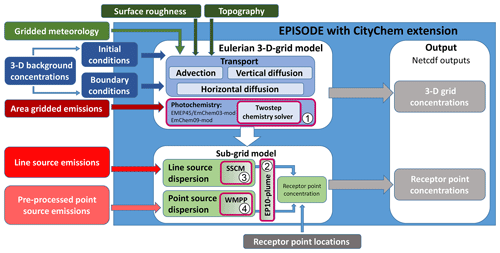
Figure 1Schematic diagram of the EPISODE model with the CityChem extension (EPISODE–CityChem model). The large blue box represents operations carried out during the execution of the EPISODE model. The components of the EPISODE model are the Eulerian grid model and the sub-grid models. The inputs for EPISODE are specified on the periphery. Modules belonging to the CityChem extension are shown with a magenta frame and are numbered: (1) photochemistry on the Eulerian grid, (2) EP10-Plume chemistry in the sub-grid components, (3) simplified street canyon model (SSCM) in the line source sub-grid model and (4) WORM Meteorological Pre-Processor (WMPP) in the point source sub-grid model.
EPISODE–CityChem solves the photochemistry of multiple reactive pollutants on the Eulerian grid by using one of the following chemical schemes: (1) EMEP45 chemistry, (2) EmChem03-mod or (3) the EmChem09-mod. In the sub-grid components, the PSS assumption involving is replaced by the EP10-Plume scheme. Dispersion close to point and line sources is modified in the sub-grid component. In the line source sub-grid model, the simplified street canyon model (SSCM) is integrated to calculate pollutant dispersion in street canyons. In the point source sub-grid model the WMPP (WORM Meteorological Pre-Processor) is integrated to calculate the wind speed at plume height. Figure 1 illustrates the modules and processes of the EPISODE model with the CityChem extension. Modules that belong to the CityChem extension are shown in boxes with a magenta frame.
Byun et al. (1999)Hamer et al. (2019)Bott (1989)Hamer et al. (2019)Smith (1985)Hamer et al. (2019)The recommended configuration of model processes in EPISODE–CityChem is given in Table 1. EPISODE–CityChem has been used with this configuration to simulate the air quality and atmospheric chemistry in the city of Hamburg (Sect. 4).
In EPISODE–CityChem, a regular receptor grid is defined, for which time-dependent surface concentrations of the pollutants at receptor points are calculated by summation of the Eulerian grid concentration of the corresponding grid cell (i.e. the background concentration) and the concentration contributions from the Gaussian sub-grid models due to line source and point source emissions. This way, surface concentration fields of pollutants for the entire city at a horizontal resolution of (currently) 100 m are obtained. The modules of the CityChem extension for photochemistry and source dispersion are described in detail in the remainder of this section.
2.1 Extensions to the photochemistry
Atmospheric gas-phase chemical reactions are described by ordinary differential equations (ODEs). The ODE set of reactions is considered stiff because the chemical e-folding lifetimes of individual gases vary by many orders of magnitude in the urban atmosphere (from approx. 10−6 to 106 s−1; McRae et al., 1982). The non-linear system of the stiff chemical ODEs is solved by the TWOSTEP solver (Verwer and Simpson, 1995; Verwer et al., 1996) using fast Gauss–Seidel iterative techniques, with numerical error control and restart in the case of detected numerical inaccuracies (Walker et al., 2003). The solver is applied to chemical reaction mechanisms available in EPISODE–CityChem for photochemical transformation on the Eulerian grid (EMEP45, EmChem03-mod and EmChem09-mod) and in the sub-grid component (EP10-Plume). For solving the EMEP45 scheme, the Gauss–Seidel iterative technique is used for all compounds except for the oxygen atoms and OH, for which reactions are very fast and we use the steady-state approximation instead (Walker et al., 2003). The relative error tolerances for the solver are set to 0.1 (10 % relative error) for all chemical compounds, while the absolute error tolerances are set in a range from 2.5×108 molecule cm−3 to 1.0×1015 molecule cm−3 depending on the compound. Photodissociation rates are specified as a function of the solar zenith angle and cloud cover, as given in Appendix B. The sink terms for the dry deposition and wet removal of gases and particles are presented in Appendix C.
2.1.1 Development and description of the EMEP45 chemistry scheme
The EMEP45 chemistry scheme developed at NILU (Walker et al., 2003) contains 45 chemical compounds and about 70 chemical reactions compared to 70 compounds and about 140 reactions in the original EMEP mechanism (Simpson, 1992, 1993; Andersson-Sköld and Simpson, 1999).
The intention of the development of EMEP45 was to obtain a condensed chemical scheme for urban areas that still captures the key aspects of the photochemistry in the urban atmosphere. The reduction of the EMEP mechanism was guided by the following considerations: first, the new chemistry scheme is applied in rather polluted urban regions. Second, the residence time of the atmospheric compounds in the urban domain is normally limited to less than a day.
The main simplification in EMEP45 compared to the original EMEP mechanism is the neglect of peroxy radical self-reactions. The self-reactions of peroxy radicals, either between the organic peroxy radical (RO2) and hydroperoxyl radical (HO2) or between two organic peroxy radicals,
are in competition with the reaction of RO2 (or HO2) with NO, leading to photochemical ozone formation:
At the ambient levels of NOx typical of moderately or more polluted areas, Reactions (R1)–(R3) will be negligible compared with Reaction (R4). Thus, all reactions of organic peroxy radicals of type (R2) and (R3) were omitted in the EMEP45 scheme. However, due to their relevance, the reaction of HO2 with the methyl peroxy radical (CH3O2) and the HO2 self-reaction (R1) were included. EMEP45 includes a simple four-reaction scheme for the oxidation of isoprene (C5H8) with the OH radical. All reaction rates and coefficients in EMEP45 are according to the International Union of Pure and Applied Chemistry (IUPAC) 2001 recommendations (Atkinson et al., 2000).
2.1.2 Development of the EmChem03-mod scheme and the EmChem09-mod scheme
The EMEP45 scheme was updated in recent years at the Helmholtz-Zentrum Geesthacht (HZG). All reaction rate constants were updated in accordance with the default chemistry scheme EmChem09 of the EMEP/MSC-W model (Simpson et al., 2012). The resulting scheme is called EmChem03-mod and consists of 45 gas-phase species, 51 thermal reactions and 16 photolysis reactions, as listed in Table S1 in the Supplement. The most important technical change compared to EMEP45 is that the new scheme can be dynamically updated and further extended with new chemical reactions and compounds. The chemical preprocessor of the EMEP/MSC-W model, GenChem, developed at the EMEP group (Simpson et al., 2012), is used to convert lists of input chemical species and reactions to differential equations of the solver in Fortran 90 code. This makes the update and extension of the new scheme entirely flexible.
In the next step, the EmChem09-mod scheme (Table S2) was developed based on the current EMEP chemistry mechanism, EmChem09 (Simpson et al., 2012), by (1) replacing the detailed isoprene chemistry with the simplified isoprene reaction scheme from EMEP45, (2) adding monoterpene oxidation reactions and (3) including semi-volatile organic compounds (SVOCs) as reaction products which can potentially act as precursors for secondary organic aerosol (SOA) constituents.
EmChem09-mod includes reactions between organic peroxy radicals and HO2 as well as other organic peroxy radicals; it is therefore appropriate for low NOx conditions in rural and suburban areas of the city domain. With EmChem09-mod the chemistry of biogenic volatile organic compounds (BVOCs), emitted from urban vegetation, can be simulated. Two monoterpenes, α-pinene and limonene, are model surrogates to represent slower- and faster-reacting monoterpenes (α-pinene: cm3 s molecule−1; limonene: cm3 s molecule−1; for the OH-reaction, both at 298 K). The scheme considers the OH-initiated oxidation of isoprene, as well as the oxidation of α-pinene and limonene by OH, NO3 and O3. Limonene has two reactive sites (double bonds) allowing for a rapid reaction chain to oxidation products with low vapour pressure. The lumped reaction scheme of α-pinene is adopted from Bergström et al. (2012) and that of limonene is based on Calvert et al. (2000). In total, EmChem09-mod includes 70 compounds, 67 thermal reactions and 25 photolysis reactions.
2.1.3 Development and description of the EP10-Plume chemistry scheme
In the sub-grid components, i.e. the Gaussian models for line and point source dispersion, the PSS assumption involving was replaced by the EP10-Plume scheme for computation of the chemistry at the local receptor grid points. EP10-Plume includes only the reactions of O3, NO, NO2, nitric acid (HNO3) and CO, as well as the photochemical oxidation of formaldehyde (HCHO). It contains 10 compounds and 17 reactions; Table S3 provides a list.
Only a small portion of NOx from motor vehicles and combustion sources is in the form of NO2, the main part being NO. The largest fraction of ambient NO2 originates from the subsequent chemical oxidation of NO. The only reactions considered to be relevant in the vicinity of NOx emission sources are
For conditions in northern Europe, an instantaneous equilibrium between the three reactions relating NO, NO2 and O3 is assumed, the so-called PSS, and implemented in the EPISODE model. In EP10-Plume the three reactions are, however, treated explicitly. Reactions occurring with negligible rates at the NOx levels typical of moderately or highly polluted areas were excluded from the scheme. HCHO and acetaldehyde are important constituents of vehicle exhaust gas (e.g. Rodrigues et al., 2012). The photolysis of HCHO is a source of HO2 radicals.
HCHO also reacts with the OH radical to give two HO2 radicals. HO2 competes with ozone for the available NO (Reaction R4), and the reaction between HO2 and NO results in additional NO-to-NO2 conversion. Since the generation of HO2 radicals through HCHO photolysis does not depend on the entrainment of photo-oxidants from the background air, it can trigger the photochemical reaction cycle even in traffic plumes very close to the source. Carbon monoxide (CO) has a lifetime of about 2 months towards OH (at molecules cm−3). Reaction (R10) is therefore not relevant near sources and of very low relevance on the urban scale. For completeness of the OH-to-HO2 cycling, Reaction (R10) was, however, included in EP10-Plume.
2.2 Extensions to the source dispersion
Sub-grid models to resolve dispersion close to point sources and line sources are embedded in the EPISODE model to account for sub-grid variations as a result of emissions along open roads and streets as well as along plume trajectories from elevated point source releases. The sub-grid model for line sources, i.e. open road and urban street traffic, is the Gaussian model HIWAY-2 (Highway Air Pollution Model 2; Petersen, 1980) from the U.S. EPA with modifications. The sub-grid model for point sources, e.g. stacks of industrial plants and power plants, is the Gaussian segmented plume trajectory model SEGPLU (Walker and Grønskei, 1992). SEGPLU computes and keeps a record of subsequent positions of plume segments released from a point source and the corresponding pollutant concentration within each plume segment. The vertical position of the plume segment is calculated from the plume rise of the respective point source. Plume rise for elevated point sources due to momentum or buoyancy is computed based on the plume rise equations originally presented by Briggs (1969, 1971, 1975). A detailed description of the implementation of HIWAY-2 and SEGPLU in the EPISODE model is given in part one (Hamer et al., 2019). In this section, extensions of the sub-grid models for the simulation of dispersion near sources within CityChem are described.
2.2.1 Implementation of a simplified street canyon model (SSCM) for line source dispersion
In CityChem, a simplified street canyon model (SSCM) to compute concentrations for receptor points that are located in street canyons is introduced. The street canyon model follows in most aspects the Operational Street Pollution Model (OSPM; Berkowicz et al., 1997). A fundamental assumption of this model is that when the wind blows over a rooftop in a street canyon, an hourly averaged recirculation vortex is always formed inside the canyon (Hertel and Berkowicz, 1989). The part of the street canyon covered by the vortex of recirculating air is called the recirculation zone.
The concentration at a receptor point located within an urban street canyon is calculated as the sum of the concentration contribution (Cline,s) due to the emissions of the line source s and the urban background concentration, which is taken from the corresponding cell of the Eulerian grid component. The contribution of a line source s is given by the direct contribution (Cscdir,s) from the traffic plume plus a contribution from the recirculation of the traffic plume (Cscrec,s) due to the vortex inside the canyon (Berkowicz et al., 1997):
The leeward receptor inside a street canyon is exposed to direct contribution from the emissions inside the recirculation zone (unless the wind direction is close to parallel) and a recirculation contribution. For the receptor on the windward side, only emissions outside the recirculation zone are considered for the direct contribution. If the recirculation zone extends through the whole canyon, no direct contribution is given to the windward receptor. The length of the recirculation zone, Lrec, is estimated as being twice the average building height of the canyon and limited by the canyon width, Wsc.
The calculation of the direct and recirculation concentration contributions in this simple approach is adopted from the OSPM following the description in Berkowicz et al. (1997) with certain modifications. Simplifications are made with respect to the street canyon geometry, since only general geometries with average street canyon width and height are used. The rate of release Qs in the street assumes that emissions are distributed homogeneously along the line source segment that is inside the street canyon area, which means emissions are assumed to be distributed homogeneously over the street canyon in the full length and width of the canyon (along the dimension of the respective line source object).
The direct contribution is calculated using a Gaussian plume model. The direct concentration contribution at the receptor point Cscdir,s, located at distance x from the line source (i.e. starting from the midline of the street), is obtained by integrating along the wind path at street level. The integration path depends on wind direction, the extension of the recirculation zone and the street canyon length (Hertel and Berkowicz, 1989):
where h0 is a constant that accounts for the height of the initial pollutant dispersion (h0=2 m is used in SSCM), σw is the vertical velocity fluctuation due to mechanical turbulence generated by wind and vehicle traffic in the street, and ustreet is the wind speed at street level, calculated assuming a logarithmic reduction of the wind speed at rooftop towards the bottom of the street. Note that the wind direction at street level in the recirculation zone is mirrored compared to the roof-level wind direction. Outside the recirculation zone, the wind direction is the same as at roof level. The vertical velocity fluctuation is calculated as a function of the street-level wind speed and the traffic-produced turbulence by the following relationship (Berkowicz et al., 1997):
where αs is a proportionality constant empirically assigned a value of 0.1, and σw0 is the traffic-induced turbulence, in SSCM assigned a value of 0.25 m s−1, which is typical for traffic on working days between 08:00 and 19:00 (Central European Time) in situations in which traffic-induced turbulence dominates (Kastner-Klein et al., 2000; Fig. 6 therein).
The integration path for Eq. (2) begins from xstart, which is defined as the distance from the receptor point at which the plume has the same height as the receptor, which is zero in the case that h0 is smaller than or equal to the height of the receptor. The upper integration limit is xend, defined by tabular values in Ottosen et al. (2015, Table 3 therein). The integration is performed along a straight line path against the wind direction. The calculation of the maximum integration path, Lmax, depends on the wind direction with respect to the street axis, θstreet, i.e. the angle between the street and the street-level wind direction (Ottosen et al., 2015).
The recirculation contribution is computed using a simple box model, assuming equality of the inflow and outflow of the pollutant. The cross section of the recirculation zone is modelled as a trapezium with upper length Ltop and baseline length Lbase. Ltop is half of the baseline length, where Lbase is defined as . The length of the hypotenuse of the trapezium is calculated as , assuming the leeward side edge of the recirculation zone to be the vertical building wall, with the length of the building height. It is further assumed that the slant edge of the recirculation zone towards the opposite street side is not intercepted by buildings.
The recirculation concentration contribution is expressed by the relationship (Berkowicz et al., 1997)
where σwt is the ventilation velocity of the canyon as given by Hertel and Berkowicz (1989), and σhyp is the average turbulence at the hypotenuse of the trapezium (slant edge towards the opposite street side).
For a given receptor point, the concentration contribution from a line source is calculated either by HIWAY-2 or by SSCM. HIWAY-2 does not calculate line source concentration contributions to receptors that are upwind of a line source or receptor points that are very close to the line source. For all windward and leeward receptor points (1) located within a model grid cell defined as a street canyon cell (see below), (2) located close enough to a line source (i.e. within the actual street canyon) and (3) located at a road link with length >8 m, the concentration contribution from the street is calculated by SSCM. For all windward receptors which do not fulfil these conditions, the concentration contribution is calculated by HIWAY-2.
The complex and diverse geometry of street canyons is approximated by three generic types for which average street canyon geometry properties are applied (Table 2). Street canyons are identified based on the urban land use classes of TAPM. Each line source for which the geometric midpoint is located in a grid cell with urban land use (land use classes 32–35 defined in TAPM) is identified as a potential street canyon. A disadvantage of this method is that some streets and roads, especially in the sparsely built urban areas outside the inner city, will be classified as street canyons despite being open roads with open spaces between buildings.
Table 2The geometry of three generic street canyon types in CityChem. For street canyons of type “urban medium”, Hsc is taken as the mean value of “urban low” and “urban high”.
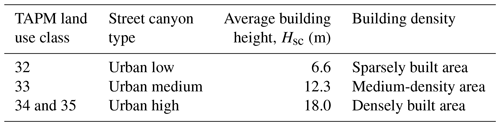
Furthermore, it is assumed that all buildings at the street canyon line source have the same average building height, Hsc, and that there are no gaps between the buildings. The average building heights for the TAPM land use classes were obtained by the intersection of the 3-D city model LoD1-DE Hamburg (LGV, 2014) – which contains individual building heights – with the CORINE (Coordination of Information on the Environment) urban land use information (CLC, 2012). The width of the street canyon, Wsc, is defined as twice the width of the (line source) street width W to account for sidewalks and to avoid canyons that are too narrow. The length of the street canyon, Lsc, corresponds to the length of the line source within the grid cell.
2.2.2 Implementation of the WMPP for point sources
The wind speed profile function of the meteorological preprocessor WMPP is utilized in the CityChem extension to calculate the wind speed at plume height within the point source sub-grid dispersion model. WMPP replaces the previous routine, which calculated the wind speed at plume height using a logarithmic wind speed profile corrected by the stability function for momentum based on Holtslag and de Bruin (1998). WMPP has been developed as part of NILU's WORM open-road line source model (Walker, 2011, 2010) to calculate various meteorological parameters needed by WORM. In the current version of WORM, the profile method is applied using hourly observations of wind speed at one height, e.g. 10 m, and the temperature difference between two heights, e.g. 10 and 2 m, to calculate the other derived meteorological parameters.
Given the above input data and an estimate of the momentum surface roughness, WMPP calculates friction velocity (u∗), temperature scale (θ∗) and inverse Obukhov length (L−1) according to Monin–Obukhov similarity theory. These quantities are calculated by solving the following three non-linear equations:
where κ is Von Kármán's constant (0.41), g is the acceleration of gravity (9.81 m s−2), Δu is the wind speed difference between heights zu2 and zu1, where zu2 is e.g. 10 m, and zu1=z0m, where the wind speed is zero, so that . In the definition of the temperature scale, Δθ is the difference in potential temperature between heights zt2 and zt1, which are e.g. 10 and 2 m, respectively, so that we have , where the +0.01 term is for the conversion from potential temperature to actual temperature. In the definition of the Obukhov length, Tref is a reference temperature, here taken to be the average of T2 m and T10 m.
In Eq. (5), the similarity functions φm and φh are defined as follows (Högström, 1996):
and
where Pr0=0.95 is the Prandtl number for neutral conditions and where the empirical coefficients are defined as , , βm=5.3 and βh=8.2.
This set of similarity functions is then used to calculate vertical profiles of temperature and wind speed. The temperature at a height (in metres above the ground) is thus calculated by
where zref=10 m and cp is the specific heat capacity of air, here set to 1005 J kg−1 K−1. Similarly, the wind speed at height z (m) above the ground is calculated by
In CityChem, WMPP is used in the sub-grid point source model to calculate the wind speed at plume height according to Eq. (9). WMPP can also be used to calculate the convective velocity scale w∗ and the mixing height hmix, but this is not implemented in CityChem.
2.3 Additional modifications
Here we describe the modifications in the CityChem extension to read hourly 3-D boundary concentrations from the output of the CMAQ model and to determine sub-grid concentrations from a regular receptor grid in the surface model layer.
2.3.1 Adapting 3-D boundary conditions from the CMAQ model
CityChem has the option to use the time-varying 3-D concentration field at the lateral and vertical boundaries from the CMAQ model as initial and boundary concentrations for selected chemical species. The adaption of boundary conditions from CMAQ output in the EPISODE model is based on the implementation for boundary conditions from the Copernicus Atmosphere Monitoring Service (CAMS; http://www.regional.atmosphere.copernicus.eu/, last access: 29 July 2019) described in part one (Hamer et al., 2019). The regional background concentrations are adopted for the grid cells (outside the computational domain) directly adjacent to the boundary grid cells of the model domain and for the vertical model layer that is on top of the highest model layer. The outside grid cell directly adjacent to the boundary grid cell is filled with the CMAQ concentration value for inflow conditions and with the concentration value of the boundary grid cell for outflow conditions, i.e. allowing for a zero-concentration gradient at the outflow boundary. More details on the treatment of 3-D boundary conditions are given in Appendix D.
2.3.2 Description of the regular receptor grid
In the CityChem extension, a regular receptor grid is defined, for which time-dependent surface concentrations of the pollutants at receptor points are calculated by summation of the Eulerian grid concentration of the corresponding grid cell (i.e. the background concentration) and the concentration contributions from the sub-grid models due to the dispersion of line source and point source emissions. Regular receptor grids with a typical resolution of 100 × 100 m2 have also been used in earlier versions of EPISODE, but primarily for capturing sub-grid-scale concentration contributions from larger industrial point sources. The establishment of a regular receptor grid is an integral part of CityChem to enable the higher-resolution output required for comparison with monitor data acquired near line sources. Line sources are a major source of pollutant emissions affecting inner-city air quality; thus, the use of the regular receptor grid provides information at much higher spatial resolution than the Eulerian grid output alone. The regular receptor grid in EPISODE–CityChem differs from the downscaling approach by Denby et al. (2014), which allocates sampling points at high density along roads and other line sources but much fewer further away from the line sources. While Denby et al. (2014) interpolate the model-computed high-density set of receptor concentrations to the desired output resolution using ordinary kriging, EPISODE–CityChem gives as output the receptor point concentrations on a regular 2-D grid covering the entire model domain.
The instantaneous concentration Crec in an individual receptor point r∗ of the receptor grid with coordinates (xr, yr, zr) is defined as
where Cm is the main grid concentration of the grid cell (x, y, 1) in which the receptor point is located. The grid (background) concentration Cm used in Eq. (10) corresponds to a modified Eulerian 3-D grid concentration, i.e. , to prevent emissions of point and lines sources from being counted twice. Cpoint,p is the instantaneous concentration contribution of point source p calculated by the point source sub-grid model, and Cline,s is the instantaneous concentration contribution of line source s calculated by the line source sub-grid model. Since Crec is not added to the main grid concentration but kept as a separate (diagnostic) variable, the double-counting of emitted pollutant mass is prevented. In the CityChem extension, receptor point concentrations represent the high-resolution ground concentration of a cell with the grid cell area of the receptor grid.
On the 3-D Eulerian grid, time-dependent concentration fields of the pollutants are calculated by solving the advection–diffusion equation with terms for chemical reactions, dry and wet deposition, and area emissions. The hourly 2-D and 3-D fields of meteorological variables and the hourly 2-D fields of area emissions are given as input to the model with the spatial resolution of the Eulerian grid. As the model steps forward in time, an accurate account of the total pollutant mass from the area, point and line sources is kept within the Eulerian grid model component. Emissions from line sources are added to the Eulerian grid concentrations at each model time step.
For the test of the various model extensions, EPISODE was run as a 1-D column model, with vertical exchange as the only transport process. Emissions were injected into the ground cell (grid centre at UTM coordinates: (X) 568500, (Y) 5935550, 32 N) with an area of 1 × 1 km2 and flat terrain (15 m a.s.l.). Table 3 shows the general setup for the 1-D column and the specific configuration for the tests. Mixing height, surface roughness and friction velocity were kept constant (hmix=250m, z0=0.8 m s−1, m s−1). Hourly varying meteorological variables included air temperature, temperature gradient, relative humidity, sensible and latent heat fluxes, total solar radiation, and cloud fraction. The test simulations are performed for a period of 5 d, and results were taken as an average of the period.
3.1 Test of the photochemistry on the Eulerian grid
3.1.1 Tests of the original EMEP45 photochemistry
When the condensed EMEP45 photochemistry was developed, various tests were carried out to compare the condensed mechanism with the standard EMEP chemical mechanism. Results from box model studies with the two chemical mechanisms revealed that there were generally small differences between the full and the condensed chemical mechanisms. Even for conditions more representative of a rural environment, the difference between the standard EMEP and the condensed mechanism was small. For these more rural conditions, the condensed mechanism gave slightly lower levels of NO and NO2, while the ozone concentration was almost identical in the two mechanisms. For urban conditions, these differences were expected to be significantly smaller.
The EPISODE model with the condensed EMEP45 mechanism furthermore participated in the CityDelta project (Cuvelier et al., 2007) within which it was applied to the city of Berlin. CityDelta was the first in a series of projects (later named EuroDelta) dedicated to photochemical model inter-comparisons. When evaluated against observations of NO2 and O3, the EPISODE model with the EMEP45 chemistry performed favourably when compared to the suite of atmospheric models participating in the CityDelta project (Walker et al., 2003).
3.1.2 Test of ozone formation with EmChem03-mod
The ozone–NOx–VOC sensitivity of the EmChem03-mod scheme in the Eulerian model component was analysed by repeated runs with varying emissions of NOx and non-methane VOCs (NMVOCs) using the daily cycle of mean summer meteorology with clear sky but low wind speed (0.1 m s−1). The ozone net production in the runs was taken at the maximum daily O3 during the simulation.
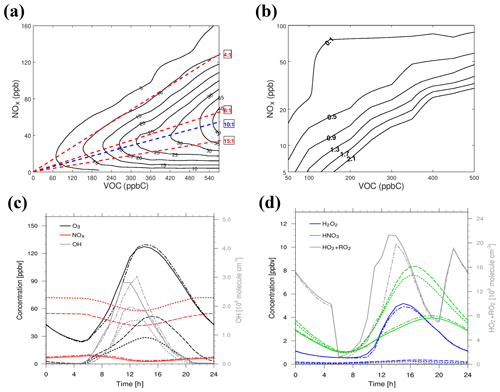
Figure 2Test of relationships between ozone, NOx and NMVOCs in EmChem03-mod: (a) ozone isopleth diagram, (b) isopleth diagram showing the ratio of the production rate of peroxides to the production rate of nitric acid, (c) concentration time series of O3 (black), NOx (red) and OH (grey; second y axis), and (d) concentration time series of H2O2 (blue), HNO3 (green) and HO2+RO2 concentration (grey, second y axis). Daily concentration cycle as an average from a test run with NMVOC emissions of and varying NOx emissions: (solid lines), (dash-dotted lines), (dashed lines) and (dotted lines). Lines of constant VOC∕NOx ratio are annotated with red dashed lines (4 : 1, 8 : 1 and 15 : 1) and the blue dashed line (10 : 1) in panel (a). Note the logarithmic scale of the y axis in panel (b).
An area source of traffic emissions of NOx and NMVOCs in the ground cell of the 1-D column was activated in the test. The variation of ozone precursor emissions from the traffic area source was done in a systematic way in order to derive the ozone isopleth diagram (Fig. 2a), which shows the rate of O3 production (ppb h−1) as a function of NOx and NMVOC concentrations. Compound abundances are given in mixing ratios (ppb) for this test to enable comparison with the literature on ozone formation potentials.
The ozone–precursor relationship in urban environments is a consequence of the fundamental division into NOx-limited and VOC-limited chemical regimes. VOC∕NOx ratios are an important controlling factor for this division of chemical regimes (Sillman, 1999). VOC-limited chemistry generally occurs in urban centres where NO2 concentrations are high due to traffic emissions. Rural areas downwind of the city are typically NOx limited (Ehlers et al., 2016).
The “ridgeline” of the ozone isopleth diagram marks the local maxima of O3 production and differentiates two different photochemical regimes. Below the line is the NOx-limited regime, in which O3 increases with increasing NOx, while it is hardly affected by increasing VOCs. Above the line is the VOC-limited regime, in which O3 increases with increasing VOCs and decreases with increasing NOx. The ridgeline in Fig. 2a follows a line of constant VOC∕NOx ratio; in the case of EmChem03-mod it is close to the ratio 10 : 1, whereas a slope of 8 : 1 is more typically found (e.g. Dodge, 1977). The traffic NMVOC mixture includes a high share of aromatics (35 %) represented by o-xylene in the model. Due to the high reactivity of the NMVOC mixture, the ridgeline is tilted towards higher VOC∕NOx ratios compared to the ozone isopleths for a NMVOC mixture with lower reactivity.
The split into NOx-limited and VOC-limited regimes is closely associated with sources and sinks of odd hydrogen radicals (defined as the sum of OH, HO2 and RO2). Odd hydrogen radicals are produced in the photolysis of ozone and intermediate organics such as formaldehyde. Odd hydrogen radicals are removed by reactions that produce hydrogen peroxide (Reaction R1) and organic peroxides (Reaction R2). They are also removed by reaction with NO2, producing HNO3, according to
When peroxides represent the dominant sink for odd hydrogen, then the sum of peroxy radicals is insensitive to changes in NOx or VOC. This is the case for the concentrations represented as solid and dash-dotted lines in Fig. 2c–d. Doubling NOx emissions from solid lines to dash-dotted lines only marginally changes the peroxy radical sum concentration (Fig. 2d).
When HNO3 is the dominant sink of odd hydrogen, then the OH concentration is determined by equilibrium between the producing reactions (e.g. photolysis of O3) and the loss reaction (R11); it thus decreases with increasing NOx (Fig. 2c–d; from dashed to dotted lines), while it is either unaffected or increases due to the photolysis of intermediate organics with increasing VOCs.
Plotting the isopleths for the ratio of the production rate of peroxides to the production rate of HNO3 (Fig. 2b) shows that this ratio is closely related to the split between NOx-limited and VOC-limited regimes. The ratio is typically 0.9 or higher for NOx-limited conditions and 0.1 or less for VOC-limited conditions (Sillman, 1999). The ridgeline that separates the two regimes should be at a ratio of 0.5 (Sillman, 1999), which is the case in Fig. 2b. However, the curves representing the ratio are shifted towards higher NOx mixing ratios compared to the isopleth diagram for the ratio displayed in Sillman (1999, Fig. 8 therein). For instance, for 100 ppbC NMVOCs and 5 ppb NOx, the ratio is below 0.1 (VOC limited) in the isopleth diagram of Sillman (1999), while it is 1.3 (NOx limited) in Fig. 2b. The reason for this discrepancy is the lack of reactions producing organic peroxides (RO2H) in EmChem03-mod and thus the reduced removal of odd hydrogen in conditions with a high VOC∕NOx ratio. In conditions with NOx below 20 ppbv, EmChem03-mod has an efficiency of NO-to-NO2 conversion via Reaction (R4) that is too high.
3.1.3 Test of EmChem09-mod photochemistry
The EmChem09-mod scheme was compared to the EmChem03-mod scheme for conditions with relatively low levels of NOx (<20 µg m−3). The configuration of the test was the same as in Sect. 3.1.2, with an area source of traffic emissions of NOx (0.043 g s−1 in the 1 × 1 km2 ground cell) and varying emissions of NMVOC corresponding to VOC∕NOx ratios of 4 : 1, 8 : 1 and 15 : 1. The daily cycle of ozone with EmChem09-mod shows O3 concentrations which are lower for a VOC∕NOx ratio of 4 : 1 (VOC limited) than with EmChem03-mod, similar for a VOC∕NOx ratio of 8 : 1 (transition) to EmChem03-mod and higher for a VOC∕NOx ratio of 15 : 1 (NOx limited) than with EmChem03-mod (Fig. 3a). Compared to EmChem03-mod, the EmChem09-mod scheme includes reactions between organic peroxy radicals and HO2, as well as other organic peroxy radicals. In conditions with low levels of NOx, the rates from these reactions will be in competition with the reaction rates of organic peroxy radicals with NO.
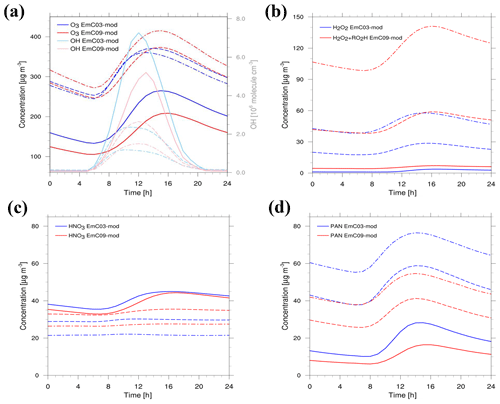
Figure 3Comparison of EmChem09-mod (red lines) with EmChem03-mod (blue lines) for three different VOC∕NOx ratios: (a) O3 and OH (light colours, second y axis); (b) H2O2 and organic peroxides (abbreviated as RO2H); (c) HNO3; and (d) PAN. Daily concentration cycle as an average from a test run with NOx emissions of and NMVOC emissions corresponding to a VOC∕NOx ratio of 4 : 1 (solid lines), 8 : 1 (dashed lines) and 15 : 1 (dash-dotted lines).
The lower O3 with EmChem09-mod in VOC-limited conditions is related to the competition between organic peroxy radical self-reactions and the reaction with NO, preventing additional NO-to-NO2 conversion. Compared to EmChem03-mod, the removal of odd hydrogen through Reaction (R11) to form HNO3 is weakened (Fig. 3b), the formation of H2O2 and organic peroxides is enhanced (Fig. 3c), and the formation of peroxyacetyl nitrate (PAN) is suppressed (Fig. 3d); the latter is due to the competing reaction between the acetyl peroxy radical (CH3COO2) and HO2, which is not included in EmChem03-mod. As a result, less NO2 is lost and the NOx concentrations in EmChem09-mod increase compared to EmChem03-mod (Fig. S1), which reduces ozone production in the VOC-limited regime.
The higher O3 with EmChem09-mod in NOx-limited conditions is related to the much higher production of peroxides and the reduced production of PAN and HNO3 compared to EmChem03-mod. The NOx concentrations in EmChem09-mod are higher, which increases ozone production in the NOx-limited regime.
3.2 Test of EP10-Plume sub-grid photochemistry
The photochemistry in the sub-grid component of EPISODE–CityChem was tested for dispersion from a single line source aligned in the SE–NW diagonal of the 1 × 1 km2 grid cell. The line source was oriented perpendicular to the wind direction, emitting NOx and NMVOCs with a ratio of 1 : 2. The HIWAY-2 line source model was used in the test (SSCM was not activated). Photochemistry tests were made as follows: (1) no chemistry; (2) photochemical steady-state assumption (PSS) for (default); and (3) with EP10-Plume using the numerical solver. Inside the centre cell, ground air concentrations downwind of the line source were recorded using additional receptor points every 10 m up to a distance of 300 m from the line source.
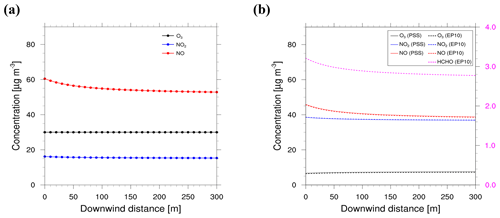
Figure 4Photochemistry downwind of a line source in the SE–NW diagonal of the 1 × 1 km2 grid cell: (a) concentration of O3 (black), NO (red), NO2 (blue) with no chemistry (lines with filled circles), and (b) O3, NO, NO2 and HCHO (magenta, second y axis) with PSS (solid lines) and EP10-Plume (dashed lines and magenta line). Note that lines for PSS and EP10-Plume are overlapping for O3, NO and NO2.
Comparing O3 (black lines), NO2 (red lines) and NO (blue lines) concentrations from the three tests with increasing downwind distance x shows that dilution alone (test with no chemistry; Fig. 4a) leads to a decay of NO, which follows a power function of the form , while O3 remains constant at the level of the background concentration (30 µg m−3).
Applying the PSS reduces O3 immediately at the line source by reaction (R5) to one-fourth of the concentration without chemistry. At the line source (0 m of distance), PSS converts roughly 15 µg m−3 NO to 21 µg m−3 NO2, as deduced from the differences between the no chemistry and the PSS test run. The third option, EP10-Plume, gives very similar results to PSS, with O3, NO and NO2 concentrations deviating by at most 4 % from the solution of the PSS (overlapping lines in Fig. 4b). In EP10-Plume, the line-emitted HCHO during daytime reacts with OH or undergoes photolysis to give HO2 radicals. However, the odd hydrogen radicals are rapidly removed by Reaction (R11), and the effect of emitted HCHO on O3 is negligible. It is noted that HCHO accounts for only 2.7 % of traffic NMVOC emissions. Further testing showed that the share of HCHO has to be increased by a factor of 10 or more (for the same VOC emission rate) in order to exceed the PSS concentration of O3 close to the line source.
3.3 Test of the source dispersion extensions
3.3.1 Test of SSCM for line source dispersion
Tests with the simplified street canyon model (SSCM; see Sect. 2.2.1) were performed for different roof-level wind speeds (0.5, 1.0, 1.5, 2.0, 4.0 and 6.0 m s−1) and compared to results from the HIWAY-2 line source dispersion model. The street canyon was oriented along the SE–NW diagonal of the grid cell, canyon width was 18 m and average building height was 18 m, with no gaps between buildings. Receptor points were placed symmetrically on the northeast side and the southwest side of the canyon 5 m of distance from the street. Time-averaged modelled concentrations of PM10, emitted from the line source as a chemically inert tracer, are shown as a function of wind direction and wind speed in Fig. 5 for the northeast side (left) and southwest side (right) receptor. The wind direction dependency at the two receptors is simply shifted by 180∘ with respect to the other due to the symmetric arrangement. With SSCM, the leeward concentrations are generally higher than the windward concentrations (grey-shaded areas in the figure). For both models, maximum concentrations are calculated for wind direction close to parallel with the street (135 and 315∘).
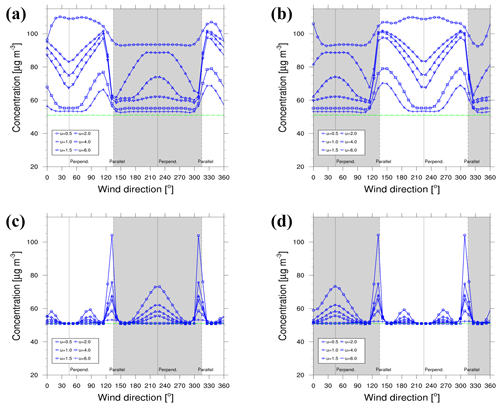
Figure 5Test of the street canyon model: concentration of an inert tracer (PM10) at the northeast side receptor (a, c) and at the southwest side receptor (b, d) as a function of wind direction and wind speed (a, b) from a simulation with SSCM and (c, d) from a simulation with HIWAY-2 (no street canyon). The background concentration, taken from the grid cell at a maximum distance from the line, is shown as a green line. Wind speed (m s−1) is given in the legend. Grey-shaded area indicates when the station is on the windward side of the street canyon. The solid vertical line indicates wind perpendicular to the street, and the dashed vertical line indicates wind parallel to the street.
For this specific street canyon, with an aspect ratio (Wsc∕Hsc) equal to 1, the recirculation zone extends through the whole canyon at high wind speeds and the windward receptor only receives a contribution from the recirculation. At low wind speeds, here at 2 m s−1 or below, the windward side starts to receive a direct contribution because the extension of the vortex decreases at low wind speeds. At wind speeds below 0.5 m s−1, the vortex disappears and traffic-generated turbulence determines the concentration levels. Gaussian models are not designed to simulate dispersion in low-wind conditions. Therefore, a lower limit of the rooftop wind speed was placed at 0.5 m s−1 in this test, preventing the test of lower wind speeds. It is, however, obvious from Fig. 5a that the influence of the wind direction on concentrations at 0.5 m s−1 is much reduced compared to higher wind speeds.
Similar to SSCM, the simulation with HIWAY-2 shows a local maximum at the windward side when the wind is perpendicular to the street and a local minimum at the leeward side when the wind is perpendicular. In HIWAY-2, the pollution from traffic is dispersed freely away from the street because it applies to open road without buildings. In SSCM, the leeward side is influenced directly by the traffic emissions in the street and additionally by the recirculated polluted air. HIWAY-2 neglects the contribution of recirculated polluted air. This is also the reason why the baseline contribution (in addition to the urban background) is higher in SSCM.
3.3.2 Test of WMPP-based point source dispersion
The WMPP model code was extensively tested using meteorological observations from a 4-month measurement campaign at Nordbysletta in Lørenskog, Norway, in 2002 (Walker, 2011, 2010).
WMPP (see Sect. 2.2.2) is used in the plume rise module of SEGPLU (Walker and Grønskei, 1992) for the calculation of the wind speed at (1) stack height, (2) plume heights along the plume trajectory and (3) at final plume height. The modification of the plume rise module is similar to the “NILU plume” parameterization implemented in the WRF–EMEP (Weather Research and Forecasting–European Monitoring and Evaluation) model, as presented in Karl et al. (2014). In comparison with two simple schemes for plume rise calculation, NILU plume gave a lower final plume rise from an elevated point source for all tested atmospheric stability conditions. In neutral conditions, the maximum concentration at the ground (Cmax) was found to be roughly twice as high as for the two simple plume rise schemes. In unstable conditions, all plume rise schemes gave similar effective emission heights.
Table 4Test of the point source dispersion of SO2 (handled as an inert tracer) for different atmospheric stability conditions in flat terrain at a wind speed of 1 m s−1. Hourly concentration is given at the location where the maximum is found for the 5 d average within a radius of 2.0 km around the point source. Parameterization of point source: exhaust gas temperature: 20 ∘C; stack height: 10 m; exit velocity: 5 m s−1; stack radius: 0.5 m (circular opening). Emission rate: 1 g s−1. The default is the standard parameterization in EPISODE.
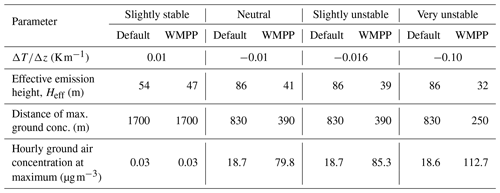
The WMPP integration in the sub-grid point source model for near-field dispersion around a point source was tested in different atmospheric stability conditions and compared to the standard point source parameterization in EPISODE (termed “default” in the following). A single point source was located at the midpoint of the 1 × 1 km2 grid cell. The dispersion of SO2, treated as a non-reactive tracer, released from the point source stack was studied by sampling ground air concentrations from a regular receptor grid with 100 m resolution within a radius of 2 km around the point source. Transport on the Eulerian grid was deactivated so that the test corresponds to a stand-alone test of the Gaussian point source model. Details about the point source and resulting hourly ground concentrations (averaged for 5 d) at the location of maximum impact, Cmax, for different stability conditions (slightly stable, neutral, slightly unstable, very unstable) are summarized in Table 4.
For WMPP, the maximum impact lies within 250 and 400 m downwind of the point source in neutral and unstable conditions. The effective emission height, Heff, in neutral conditions is about half of that computed by the default parameterization. For WMPP, Heff decreases with enhanced instability (from neutral to very unstable), while Cmax increases correspondingly. The increase in Cmax computed by WMPP is 41 %. Cmax should be roughly inversely proportional to the square of the effective emission height (Hanna et al., 1982); thus, the decrease from 41 m (neutral) to 32 m (very unstable) implies a potential increase in Cmax by 25 %. The higher increase in Cmax than expected might be due to continuous wind from one direction (225∘) and the relatively low wind speed (1 m s−1) in the test. For the default, Heff and Cmax are not affected by changing stability in neutral or unstable conditions; computed Cmax is a factor of 4–6 smaller than for WMPP. In stable conditions, Cmax is several orders of magnitude smaller than in neutral and unstable conditions for both plume rise parameterizations. The maximum impact is found at 1700 m of distance from the point source, which is comparable to previous tests with the point source model (Karl et al., 2014).
4.1 Setup of model experiments for the application for AQ modelling in Hamburg
EPISODE–CityChem was run as part of a one-way nested chemistry-transport model chain from the global scale to the urban scale. The APTA (Asthma and Allergies in Changing Climate) global reanalysis (Sofiev et al., 2018) of the Finnish Meteorological Institute (FMI) provided the chemical boundary conditions for the European domain. The CMAQ v5.0.1 CTM (Byun et al., 1999; Byun and Schere, 2006; Appel et al., 2013) was run with a temporal resolution of 1 h over the European domain and an intermediate nested domain over northern Europe with 64 and 16 km horizontal resolution, respectively. CMAQ simulations were driven by the meteorological fields of the COSMO-CLM (COnsortium for SMall-scale MOdeling in CLimate Mode) model version 5.0 (Rockel et al., 2008) for the year 2012 using the ERA-Interim reanalysis of the European Centre for Medium-Range Weather Forecasts (ECMWF) as forcing data (Geyer, 2014). Within the northern Europe domain, an inner domain over the Baltic Sea region with 4 km horizontal resolution was nested (Fig. 6a). The 4 km resolved CMAQ simulation of the Baltic Sea region provided the initial and hourly boundary conditions for the chemical concentrations in the Hamburg model domain.
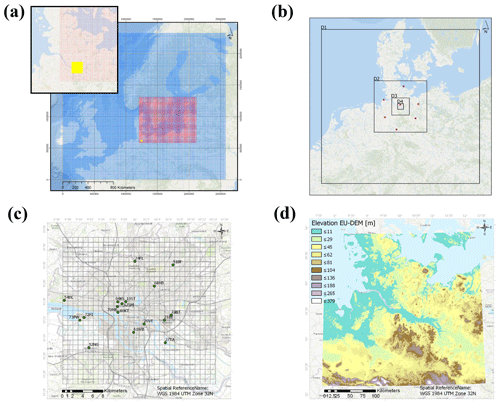
Figure 6Model domains. (a) Nested CTM simulations with CMAQ showing the 16 km (CD16, blue grid) and 4 km (CD04, red grid) resolution nests as well as the study domain (yellow box); inset in the upper left corner shows a zoomed-in view of the study region. (b) Domains used for the nested meteorological simulations within TAPM (D1–D4; black frames); the red dots indicate DWD station locations, from which observation data were assimilated to nudge the wind field predictions in TAPM. (c) Study domain of Hamburg (30 × 30 km2) for simulation with CityChem, which corresponds to the innermost nest (D4) of the nested model chain of both CMAQ and TAPM simulations. Green dots indicate the positions of stations in the air quality monitoring network. (d) Terrain elevation (m a.s.l.) from the Digital Elevation Model over Europe (EU-DEM; https://www.eea.europa.eu/data-and-maps/data/eu-dem, last access: 29 July 2019) for the extent of D2.
The hourly meteorological fields for the study domain of Hamburg (30 × 30 km2) were obtained from the inner domain in a nested simulation with TAPM (Hurley et al., 2005) with a 1 km horizontal resolution (D4 in Fig. 6b). The meteorological component of TAPM is an incompressible, non-hydrostatic, primitive equation model with a terrain-following vertical sigma coordinate for 3-D simulations; it was used for downscaling the synoptic-scale meteorology. The outer domain (D1 in Fig. 6b) was driven by the 3-hourly synoptic-scale ERA5 reanalysis ensemble means on a longitude–latitude grid at 0.3∘ grid spacing. In addition, wind speed and direction observations at seven measurement stations of the German Weather Service (DWD) are used to nudge the predicted solution towards the observations.
TAPM uses a vegetative canopy, soil scheme and an urban scheme with seven urban land use classes (Hurley, 2008) at the surface, while radiative fluxes, both at the surface and at upper levels, are also included. In regions belonging to one of the urban land use classes, specific urban land use characteristics, such as fraction of urban cover, albedo of urban surfaces, thermal conductivity of urban surface materials (e.g. concrete, asphalt, roofs), urban anthropogenic heat flux and urban roughness length, are used to calculate the surface temperature and specific humidity as well as surface fluxes and turbulence. A complete list of the meteorological variables and fields used from TAPM as input to EPISODE–CityChem for the AQ simulations is given in the user's guide for EPISODE–CityChem, which is included in the CityChem distribution.
For a better representation of local features, the coarsely resolved standard land cover classes and elevation heights, which are provided together with TAPM, were updated with 100 m CORINE Land Cover 2012 data (CLC, 2012), and terrain elevation data were adopted from the German Digital Elevation Model (BKG, 2013) at 200 m horizontal resolution.
The procedure to adapt hourly 3-D concentrations of the CMAQ model computed for the 4 km resolution domain as lateral and vertical boundary conditions is described in Sect. 2.3.1 and Appendix D. CMAQ concentrations from the 4 km resolution domain were interpolated to the 1 km resolution (in UTM projection) of the Hamburg study domain, preserving a nesting factor of four (64, 16, 4, 1 km) for the nested model chain. The study domain is located in the southwest part of the 4 km CMAQ domain (CD04); the west border of the study domain is 30 km from the CD04 west border, and the south border is 21 km from the CD04 south border (inset in Fig. 6a). The contribution of the recirculation of NOx from the coarser outer domain to the budget of NOx in the inner domain is very small due to the predominant westerly winds.
4.1.1 Description of the model setup and configuration for Hamburg
The EPISODE–CityChem simulation was performed using the recommended numerical schemes for physics and chemistry, including the new urban parametrization for vertical eddy diffusivity (urban K(z), see part one; Hamer et al., 2019). The segmented plume model SEGPLU with WMPP-based plume rise was used for the point source emissions. The line source model HIWAY-2 with the street canyon option was used for the line source emissions. Table 1 summarizes the chosen model processes and options. The vertical and horizontal structure of the 3-D Eulerian grid in EPISODE–CityChem is determined by the model domain structure of the TAPM simulation. The model input of boundary conditions and gridded area emissions have to be with the same horizontal resolution as the meteorological fields. A horizontal resolution of 1000 m was chosen for the 30 × 30 km2 domain of Hamburg. The horizontal resolution is in practice limited by the available gridded area source emission data. Finer resolution increases the required computational time; for instance, using a horizontal resolution of 500 m for the study domain results in a number of grid cells that is 4 times higher and a halved model time step (dt=300 s instead of dt=600 s), increasing the total computational time for one simulation month by a factor of 2.8 compared to the applied resolution. The EPISODE–CityChem model was set up with the vertical dimension and resolution matching that of TAPM, with a layer top at 3750 m of height above the ground, avoiding the need for vertical interpolation. The layer top heights of the lowest 10 layers were 17.5, 37.5, 62.5, 87.5, 125, 175, 225, 275, 325 and 375 m. Table 5 provides details on the vertical and horizontal structure of EPISODE–CityChem and TAPM (pollution grid) D4 as used for the Hamburg study domain and CMAQ (CD04). The computational time for a 1-month simulation with EPISODE–CityChem is 10.7 h on an Intel® Xeon (R) CPU E5-2637 v3 at 3.50 GHz with 64 GB of RAM.
Table 5Vertical and horizontal structure of the 3-D Eulerian grid of the EPISODE–CityChem model and comparison with TAPM (D4) and CMAQ (CD04) for the simulation of AQ in Hamburg.
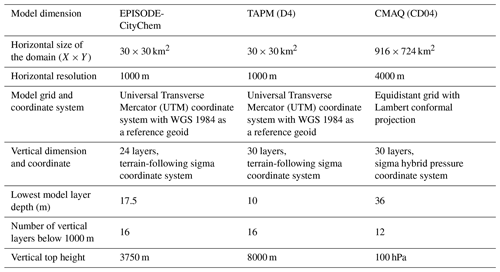
Table 6Emission sector data for the simulation of air quality in Hamburg. Classification according to Selected Nomenclature for sources of Air Pollution (SNAP). The top heights of layers 1, 2, 3 and 4 are 17.5, 37.5, 62.5 and 87.5 m above the ground, respectively. Point source emission data for SNAP categories 01, 04, 05 and 09 were collected from the PRTR database (PRTR, 2017) and from the registry of emission data for point sources in Hamburg as reported under the German Federal Emission Control Act (BImSchV 11).
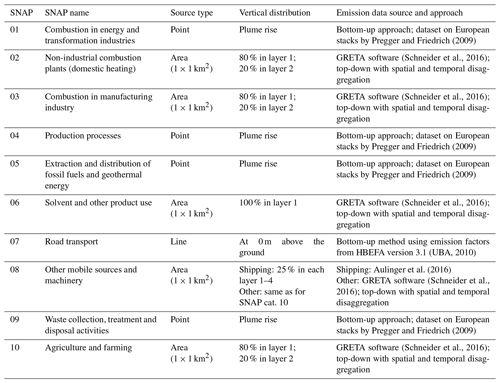
Area, point and line source emissions for the study domain of Hamburg were used from various data sources for the different emission sectors classified by the Selected Nomenclature for sources of Air Pollution (SNAP) of the European Environmental Agency (EEA), applying top-down and bottom-up approaches (Matthias et al., 2018). Table 6 gives an overview. Spatially gridded annual emission totals for area sources with a grid resolution of 1 × 1 km2 were provided by the German Federal Environmental Agency (Umweltbundesamt, UBA). The spatial distribution of the reported annual emission totals was calculated at UBA using the ArcGIS-based software GRETA (Gridding Emission Tool for ArcGIS) (Schneider et al., 2016). Hourly area emissions with a 1 km horizontal resolution for SNAP cat. 03 (commercial combustion), 06 (solvent and other product use), 08 (other mobile sources, not including shipping) and 10 (agriculture and farming) were derived from the UBA area emissions by temporal disaggregation using monthly, weekly and hourly profiles.
For SNAP cat. 02 (domestic heating) the daily average ground air temperature obtained from the TAPM simulation is used to create the annual temporal profiles. The day-to-day variation of domestic heating emissions is based on the heating degree-day concept implemented in the Urban Emission Conversion Tool (UECT) (Hamer et al., 2019). Domestic heating emissions (SNAP cat. 02) for Hamburg are distributed between 32 % district heating, 40 % natural gas, 14 % fuel oil and 14 % electricity (Schneider et al., 2016).
NMVOC emissions in the UBA dataset were distributed over individual VOCs of the chemical mechanism using the VOC split of the EMEP model (Simpson et al., 2012) for all SNAP sectors.
A total of 120 point sources were extracted from the PRTR (Pollutant Release and Transfer Register) database (PRTR, 2017) and from the registry of emission data for point sources in Hamburg, representing the largest individual stack emissions.
The line source emission dataset (emissions of NOx, NO2 and PM10) provided by the city of Hamburg contained 15 816 road links within the study domain. The NOx emission factor from road traffic for the year 2012 was increased by 20 % for all street types because the average NOx emission factor in the new HBEFA (Handbook Emission Factors for Road Traffic) v3.3 for passenger cars is higher by 19.4 % (diesel cars: 21 %) than in HBEFA v3.1 used in the road emission inventory (UBA, 2010). To estimate NMVOC traffic emissions, an average NMVOC∕NOx ratio of 0.588, derived from UBA data for SNAP cat. 07, was used.
An NO2∕NOx ratio of 0.3 was applied to recalculate NO2 emissions for this study because of the expected higher real-world NO2 emissions from diesel vehicles. The applied value is higher than suggested by the reported range (3.2–23.5 vol %) of the primary NO2 emission fraction from vehicular traffic in London (Carslaw and Beevers, 2005) and the NO2∕NOx ratio of 0.22 for passenger cars in urban areas assumed by Keuken et al. (2012) for the Netherlands. The use of the high NO2∕NOx ratio for the Hamburg vehicle fleet is consistent with the higher NO2∕NOx ratio from diesel passenger cars (from 0.12 to >0.5; Carslaw and Rhys-Tyler, 2012) and the review by Grice et al. (2009), who assumed that Euro 4–6 passenger cars emit 55 % of the total NOx as NO2.
4.2 Presentation and evaluation of model results
4.2.1 Setup of the model evaluation and performance analysis
A 1-year simulation with EPISODE–CityChem was performed for the study domain using the model setup as described in Sect. 4.1.1. Model results were compared to results from the standard EPISODE model to assess the total effect of the new implementations of the CityChem extension. In the standard EPISODE model, the PSS approximation is used at the receptor points and on the Eulerian grid; the street canyon model and the WMPP module were deactivated. For the simulation with EPISODE–CityChem and standard EPISODE, the boundary conditions from hourly 3-D concentrations of CMAQ were taken as described in Sect. 2.3.1. In addition, EPISODE–CityChem results were compared to results from the air pollution module of TAPM, which is used as a reference model in this study. The TAPM run was performed with the same horizontal resolution (1 km), as well as identical meteorological fields and urban emissions, but with 2-D boundary conditions instead of 3-D boundary conditions. The hourly 2-D boundary concentrations for TAPM were prepared by using the horizontal wind components on each of the four lateral boundaries to weight the CMAQ concentrations surrounding the Hamburg study domain. Concentrations for the TAPM boundary conditions were taken from the seventh vertical model layer of the CMAQ simulation, with a mid-layer height of approximately 385 m above the ground, where average concentrations are not significantly affected by urban emissions.
Evaluation of the model results for Hamburg was done in a four-stage procedure:
-
statistical performance analysis of the prognostic meteorological model component of TAPM (Sect. 4.2.2);
-
evaluation of the temporal variation of modelled concentrations against observed concentrations (Sect. 4.2.3);
-
evaluation of the spatial variation of the annual mean concentrations (Sect. 4.2.4); and
-
model performance analysis with respect to the objectives set forth in the AQD for the use of the model in policy applications (Sect. 4.2.5).
Statistical indicators of the evaluation included the mean (modelled ∕ observed), standard deviation (SD; modelled ∕ observed), correlation coefficient (Corr), root mean square error (RMSE), overall bias (Bias), normalized mean bias (NMB) and index of agreement (IOA). See Appendix E for the definition of the statistical indicators.
The FAIRMODE (Forum for Air Quality Modelling in Europe) DELTA tool version 5.6 (Thunis et al., 2012a, b, 2013; Pernigotti et al., 2013; FAIRMODE, 2014; Monteiro et al., 2018) was used for the evaluation of model results from air quality simulations for Hamburg. The DELTA tool focuses primarily on the air pollutants regulated in the current AQD (EC, 2008).
4.2.2 Evaluation of downscaled meteorological data
Downscaled meteorological data on temperature, relative humidity, total solar radiation, wind speed and wind direction were examined. Hourly-based data from the meteorological station at the Hamburg airport (Fuhlsbüttel) (operated by DWD) and from the 280 m high Hamburg weather mast at Billwerder (operated by Universität Hamburg) were analysed. Observation data from the DWD station at 10 m of height and from the Hamburg weather mast at 10 and 50 m of height were used in the analysis. TAPM modelled meteorological data from the 1 × 1 km2 grid cell of the D4 domain, where the stations are located, at the corresponding height were extracted for comparison with observations. Table S4 provides an overview of the statistical analysis of TAPM data.
Hourly temperature predicted by TAPM was in excellent agreement with the observed temperature at both stations and both heights, showing high correlation (Corr ≥ 0.98) and small overall bias (≤ 1.00 ∘C). Relative humidity also showed good agreement but with lower correlation (Corr = 0.74). Total solar radiation was predicted by TAPM with high correlation (Corr = 0.86) but a high positive overall bias of 27 W m−2. Situations with reduced solar radiation due to high cloud coverage are often not well captured by TAPM. The IOA for temperature, relative humidity and total solar radiation was 0.99 (average of all observations), 0.86 and 0.92, respectively.
TAPM shows good predictive capabilities for wind speed and direction. Due to the assimilation of wind observations at the DWD Hamburg airport station for nudging wind speed and direction in TAPM meteorological runs, the meteorological performance for wind speed and direction was only compared at the Hamburg weather mast. Modelled hourly data for wind speed at the Hamburg weather mast agreed well with the observations throughout the year at 10 m (Corr = 0.87, Bias = −0.08 m s−1) and 50 m of height (Corr = 0.85, Bias = −0.02 m s−1); they were within the observed variability. Southwest and west are the most frequent wind directions in Hamburg due to prevailing Atlantic winds, followed by winds from the southeast (Bruemmer et al., 2012). Mean wind direction was computed as a circular average (unit vector mean wind direction) for model and observation data. At the Hamburg weather mast, modelled versus observed wind directions show good agreement at 10 and 50 m of height (IOA ≥ 0.89) with a bias in the mean wind direction of 16.9 and 6.2∘ at 10 and 50 m, respectively. The difference is due to a slightly higher frequency of winds from the west predicted by TAPM.
4.2.3 Evaluation of the temporal variation of pollutants
The statistical performance of the models with respect to temporal variation was assessed by comparing modelled concentrations against observed concentrations from the AQ monitoring network of Hamburg. The stations of the monitoring network and the available measurements of pollutant concentrations are listed in Table S5. The monitoring network covers all parts of the city (Fig. 6c). Minimum data availability is required for statistics to be produced at a given AQ monitoring station. In the DELTA tool, the requested percentage of available data over a selected time period (here: 1 year) is 75 %, as defined in the AQD. This has been fulfilled by all stations in Hamburg, except for O3 and PM2.5 measurements at two stations. The statistical analysis included all stations for which the data availability criterion was fulfilled. For the comparison, model output at the exact geographic location of the monitoring stations from the model was used. Concentrations of NO2 and NO were measured at all stations included in this study. The model performance statistics are listed in Table 7 for NO2 (based on hourly values), in Table 8 for O3 (based on daily max. of 8 h running mean) and in Table 9 for PM10 (based on daily mean).
Table 7Model performance statistics of EPISODE–CityChem (EPCC), standard EPISODE (EPIS) and TAPM for the temporal variation of NO2 based on hourly concentration at all stations with sufficient data available in 2012. Bold numbers represent model results with better performance for Corr, RMSE and IOA per station.
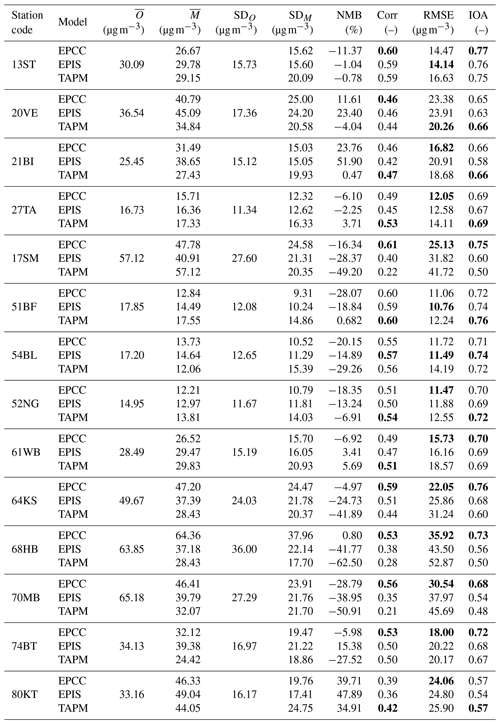
Table 8Model performance statistics of EPISODE–CityChem (EPCC), standard EPISODE (EPIS) and TAPM for the temporal variation of O3 based on the daily maximum of the 8 h running mean concentration at all stations with sufficient data available in 2012. Bold numbers represent model results with better performance for Corr, RMSE and IOA per station.
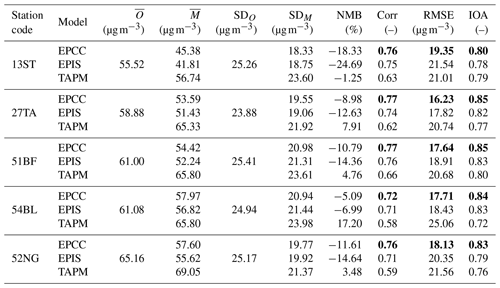
Table 9Model performance statistics of EPISODE–CityChem (EPCC), standard EPISODE (EPIS) and TAPM for the temporal variation of PM10 based on daily mean concentration at all stations with sufficient data available in 2012. Bold numbers represent model results with better performance for Corr, RMSE and IOA per station.
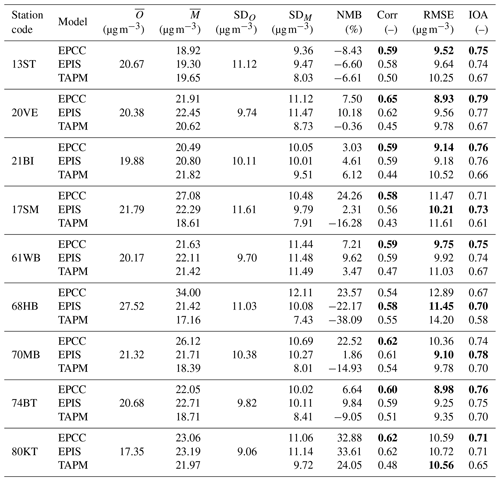
EPISODE–CityChem performs fairly well for NO2 based on hourly values, with an IOA of 0.70 (average of all stations) and correlation of 0.53 (average of all stations). The average performance at the traffic stations (Corr = 0.57, IOA = 0.73 on average) is better than for the other stations. EPISODE–CityChem performs better than EPISODE and TAPM at the traffic stations. This implies that the use of the street canyon model improves the agreement between the model and observations of NO2 at traffic stations. In particular, TAPM shows weak correlation at the traffic sites (Corr = 0.29, IOA = 0.52 on average). On the other hand, TAPM shows low bias and relatively good correlation at the industrial sites and stations influenced by emissions from industry and shipping (20VE, 21BI, 61WB, 80KT). For most urban background stations, EPISODE–CityChem tends to underestimate the observation mean. The NMB is slightly negative for stations of the urban background in suburban areas (NMB = −14.2 % on average), which is indicative of NOx emissions from suburban areas that are too low or dispersion of local emissions that is too efficient. Compared to EPISODE, the EPISODE–CityChem model has a lower positive bias at some industrial and background stations (20VE, 21BI, 74BT, 80KT), probably due to the combined effect of the more advanced photochemistry and WMPP used for point source plumes.
EPISODE–CityChem performs better for the O3 daily maximum of the 8 h running mean than the other two models. The overall performance for ozone is very good, with an IOA of 0.83 (average of all stations), correlation of 0.76 (average of all stations) and small NMB (within ±18 %). The correlation improvement compared to EPISODE is relatively small, and hence the better performance compared to TAPM might be largely from the use of the more comprehensive set of boundary conditions.
The performance of EPISODE–CityChem for PM10 based on daily means is good, with an IOA of 0.74 (average of all stations). Correlation values are generally satisfactory, with a station average of Corr = 0.60; RMSE values are fairly small, within the range 8.9–12.9 µg m−3 for all stations. For the urban background stations, EPISODE–CityChem and EPISODE perform better than TAPM in terms of correlation and IOA, giving a clear indication of the advantage of using 3-D boundary conditions instead of 2-D boundary conditions. Including the street canyon model leads to an overestimation of the observed daily mean PM10 concentrations at the traffic stations. However, it cannot be concluded whether the overestimation is due to shortcomings in the street canyon module or due to inaccurate traffic emissions in the respective streets.
4.2.4 Evaluation of the spatial variation of pollutants
Annual mean concentrations of regulatory air pollutants from the EPISODE–CityChem model output were compared to the available observation data (Fig. 7). The model reproduces the spatial variation of NO and NO2 concentrations (Fig. 7a, b) and the concentration gradients of NO2 and NO between the urban background (80KT, 51BF, 52NG, 13ST; 61WB, 54BL, 27TA, 74BT), traffic stations (68HB, 64KS, 70MB, 17SM) and industrial stations (21BI, 20VE). For most stations the overall bias of annual mean observed NO2 is within ±10 µg m−3. Observed levels of annual mean O3 at the five urban background stations (13ST, 27TA, 51BF, 54BL, 52NG) are captured by EPISODE–CityChem within ±15 % (Fig. 7c). TAPM overestimates annual mean O3 by 10 %–25 %, except for the inner-city background station 13ST. Annual mean SO2 was compared at five stations (Fig. 7d). With the exception of the industrial station 20VE, modelled annual mean SO2 from EPISODE–CityChem agreed with the observed concentrations within a factor of 2. At station 20VE, modelled SO2 is about 6 times higher than observed SO2. Obviously, the models overestimate the influence of SO2 emissions from nearby industrial sources. Modelled annual mean SO2 from EPISODE and TAPM was even higher at this site. The slightly better agreement of EPISODE–CityChem compared to EPISODE might be due to the use of WMPP or to the consideration of the OH reaction of SO2.
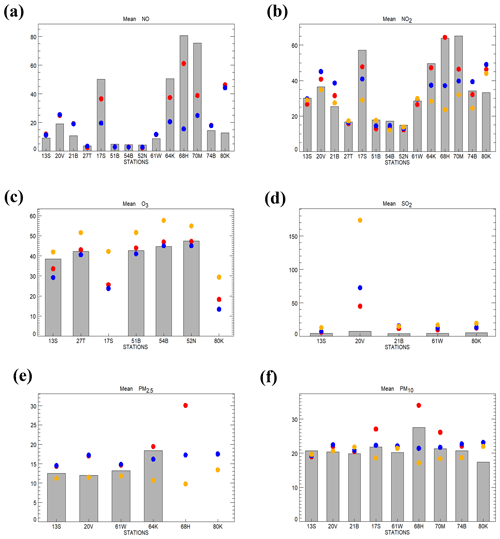
Figure 7Bar plots comparing modelled and observed mean annual concentrations (µg m−3) for monitoring stations in the Hamburg AQ network: (a) NO, (b) NO2, (c) O3, (d) SO2, (e) PM2.5 and (f) PM10. Observed values are represented as grey filled bars, and modelled values from EPISODE–CityChem, standard EPISODE and TAPM are indicated as red, blue and orange filled circles, respectively. Observation data not shown for stations for which data completeness was less than 75 % (for O3: 17SM and 80KT, for PM2.5: 68HB and 80KT). TAPM does not output NO concentrations. Non-visible data points of EPISODE–CityChem overlap EPISODE.
Levels of PM2.5 and PM10 in the models are controlled by the primary emission of particulate matter and its atmospheric dispersion, while secondary aerosol formation is not considered in the models. The comparison of the time series of daily means at station 13ST in Fig. S2 indicates that EPISODE–CityChem is able to capture observed peak events with high PM2.5 and PM10 concentrations during the winter season (DJF). These events are likely related to the short- or long-range transport of anthropogenic emitted PM or secondary produced inorganic PM. Observed levels of annual means of PM2.5 are matched by the model within ±43 % (Fig. 7e). TAPM underestimates observed annual mean PM2.5 and PM10 at the traffic stations. We note that TAPM has no specific treatment of local dispersion at the street scale. Line source emissions in TAPM are released into the volume of the corresponding grid cell in the surface layer (10 m of height) and therefore immediately diluted.
EPISODE–CityChem overestimated annual mean PM10 at three traffic stations (17SM, 68HB and 70MB) by 22 %–24 %, while the agreement with observed PM10 was excellent at the other stations (Fig. 7f). Since EPISODE shows lower modelled PM10 at the traffic stations than EPISODE–CityChem, we conclude that the overestimation of PM10 at the traffic stations is due to the street canyon module. However, the estimation of traffic emissions of PM10 is complicated by coarse particles from non-exhaust emissions, such as tyre abrasion and the resuspension of road dust. In addition, traffic emissions used in the simulations are not based on actual traffic counts, so it is entirely possible that the included PM10 line source emissions in the respective streets were too high.
4.2.5 Model performance analysis for policy support applications
We assessed whether the model results from EPISODE–CityChem have reached a sufficient level of quality for a given policy support application and compared the outcome to EPISODE and TAPM. The model quality objective in the FAIRMODE DELTA tool has been constructed on the basis of the observation uncertainty and describes the minimum level of quality to be achieved by a model to be fit for policy use (Thunis et al., 2012a, b, 2013; Pernigotti et al., 2013). The model quality indicator (MQI) provides a general overview of the model performance. The associated model performance criteria (MPC) for correlation, standard deviation and bias can be used to highlight which of the model performance aspects need to be improved. Details on the MQI and MPC are given in Appendix E.
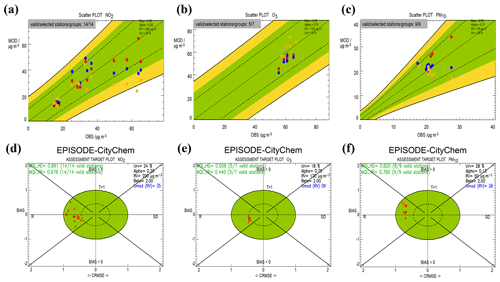
Figure 8Model performance evaluation for monitoring stations in the Hamburg AQ network: (a) scatter diagram for NO2 hourly values, (b) scatter diagram for O3 daily max. of 8 h running mean, (c) scatter diagram for PM10 daily values, (d) target plot for NO2 hourly values, (e) target plot for O3 daily max. of 8 h running mean and (f) target plot for PM10 daily values. Scatter diagrams show model data from EPISODE–CityChem (red dots), standard EPISODE (blue dots) and TAPM (orange dots). In the scatter diagrams, the uncertainty parameters (β, α, RV, ) used to produce the diagram are listed on the top right-hand side; dashed and solid lines indicate NMB ∕ 2RMSU ratios of 0.5 and 1. Target diagrams show the evaluation of EPISODE–CityChem. The target diagrams indicate MQI (MQIHD for hourly and daily values, MQIYR for yearly average) for the station most distant from the origin and the model uncertainty, Umod(RV).
Figure 8 shows the model performance evaluation of EPISODE–CityChem in terms of fitness for purpose in the form of scatter diagrams and target diagrams (Thunis et al., 2012a; Monteiro et al., 2018) for NO2 (hourly), O3 (daily max. of the 8 h running mean) and PM10 (daily mean). For the yearly averaged values shown in the scatter diagrams, the model quality objective is the bias MPC (as defined by Eq. E12). The scatter diagrams related to the bias MPC for yearly averaged NO2, O3 and PM10 in Fig. 8a–c include data from all three models. For EPISODE–CityChem, the data indicate fulfilment (stations lie within green-shaded zone) for all pollutants at all stations. The scatterplot for NO2 reveals that for TAPM two stations are in the orange-shaded zone (fulfilment but error dominated by bias), and one station does not fulfil the bias MPC. EPISODE–CityChem shows scatter around the 1 : 1 line with more stations lying below than above, indicating a slight tendency to underestimate yearly averaged NO2. The scatterplot for O3 indicates fulfilment for all models, with EPISODE–CityChem and TAPM predictions being closer to the 1 : 1 line than EPISODE. For PM10, EPISODE–CityChem and EPISODE gave similar results for most stations (Fig. 7f), and data points therefore overlap in the scatter diagram. EPISODE–CityChem shows values close to the upper measurement uncertainty range at the three traffic stations. TAPM shows an outlier for one traffic station (68HB) with predicted PM10 that is too low; however, it is still within the green-shaded zone.
In the target diagrams (Fig. 8d–f) the centred RMSE (CRMSE) for each monitoring station is plotted against the normalized bias. The distance from the circle origin gives an estimate for the MQI; MQI values below unity (i.e. within the green-shaded area) are considered to comply with the model quality objective required for policy applications. The EPISODE–CityChem model fulfils the model performance objectives for NO2, O3 and PM10 in terms of both bias MPC and MQI (max. MQIs for hourly and daily values are NO2 0.891, O3 0.506, PM10 0.820). All stations are located within the green-shaded zone, indicating fulfilment of the RMSE criteria as defined by Eq. (E10), but outside the dashed circle; i.e. the difference between the model and observations is not within the measurement uncertainty range. In the right quadrant of the target diagram (Fig. 8d–f), the error related to standard deviation dominates the model performance, and in the left quadrant the error related to correlation dominates the model performance. For all three pollutants, deficits in the model performance of EPISODE–CityChem are related to the centred RMSE (abscissa of the target diagram), while bias is small. The error related to correlation dominates the model's performance (all stations are in the left quadrant of the target diagram). The estimated model uncertainty of the predicted hourly NO2 and the predicted daily mean PM10 is 35 % and 36 %, respectively.
Corresponding target diagrams for TAPM and EPISODE are shown in Fig. S3. Notably, for hourly and yearly averaged NO2 the two other models show weaker performance than EPISODE–CityChem. EPISODE fulfils the MQI for hourly values but exceeds the MQI for yearly averages. TAPM exceeds both MQI criteria, mainly due to the weak performance at the traffic stations (see Sect. 4.2.3). For O3 and PM10 the model performance objectives are fulfilled by both EPISODE and TAPM.
4.3 Atmospheric chemistry in the urban area
4.3.1 Mapping of annual mean concentrations
Figure 9 depicts spatial maps of the annual mean concentrations of NO2, O3, total NMVOC, SO2, gaseous sulfuric acid (H2SO4) and PM2.5 from the model output of the receptor grid (resolution 100 × 100 m2). With the exception of PM2.5, concentrations of the aforementioned compounds are modulated by photochemical reactions in the model simulation. Due to the large temporal, spatial and compositional variations of the input from anthropogenic emissions of NOx, NMVOCs and CO within the urban area, the atmospheric chemistry in urban environments is complex. Prevailing winds from the west, on an annual basis, allow for a simplified view of the inflow–outflow pattern for ozone within the study domain of Hamburg. Following the inflow–outflow direction in space from west to east (30 km), the modelled O3 concentration starts with ca. 50 µg m−3 at the western border, is largely reduced in the inner city to 15–30 µg m−3 and gradually increases again over the eastern part to ca. 40 µg m−3.
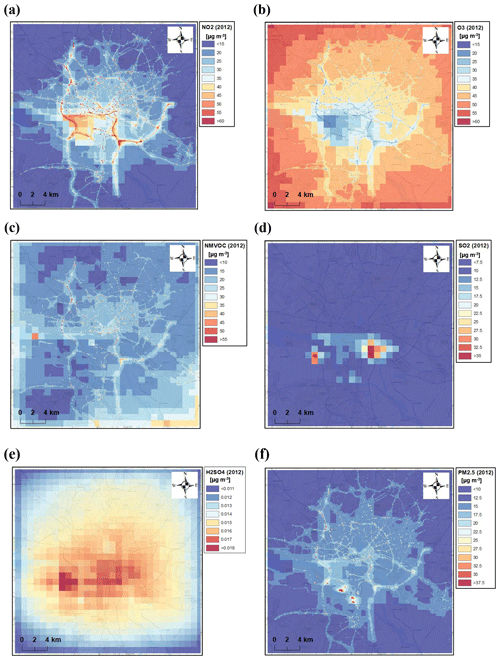
Figure 9Spatial maps of the annual concentration average (µg m−3) for Hamburg from the EPISODE–CityChem model simulation output for the receptor grid (100 × 100 m2): (a) NO2, (b) O3, (c) total NMVOC, (d) SO2, (e) H2SO4 and (f) PM2.5. Maps are created using ESRI® ArcMap™, with an overlay on a topographic base map showing the network of main roads as grey lines.
Modelled and observed annual mean NO2 at the four traffic stations exceeds the annual limit value of 40 µg m−3 in 2012 (see Fig. 7b). The model simulation suggests that there is widespread exceedance of this limit value in the city, mainly at the main streets and road junctions of the inner city and along motorways outside the inner city (Fig. 9a).
Modelled ozone at the outflow border does not reach the level at the inflow border. Within the urban area, traffic-related emissions of NO destroy much of the O3 (mainly at night when O3 is not recycled through the photolysis of NO2), clearly seen as minimum concentrations along the traffic network (Fig. 9b). Thus, the inner urban area provides an efficient sink for ozone, which is qualitatively in accord with the findings of the REPARTEE (Regents Park and Tower Environmental Experiment) measurement campaign carried out in London in the autumn of 2006 and 2007 (Harrison et al., 2012).
The photochemical production of O3 from NOx, NMVOCs and CO, emitted in the urban area, is very limited in the inner city. Main sources of NMVOC in Hamburg are solvent use (SNAP cat. 06) and traffic emissions. NMVOC annual mean concentrations of more than 40 µg m−3 were modelled close to roads in the inner city (Fig. 9c). Ehlers et al. (2016) report the similarity of NMVOC fingerprints in air samples taken in the inner city of Munich and in a road tunnel in Berlin to the fingerprint of petrol cars under cold-start conditions. Our model simulation finds that the loss of NMVOCs by OH-initiated oxidation in the model is inhibited due to the low O3 concentrations in the inner city. In summer, modelled OH midday maximum concentrations are in the range (0.5–2.0) ×106 molecule cm−3 in the inner city. Modelled o-xylene, which is the model surrogate compound for the sum of aromatic VOCs, is 5–10 µg m−3 (1.2–2.2 ppbv) at some distance from the roads. This is in the same range as concentrations measured in central London (ca. 2 ppbv for the sum of aromatics; Valach et al., 2015).
SO2 is an important precursor for secondary aerosol formation. SO2 emissions in Hamburg are mainly from industrial point sources and ship traffic in the harbour area. The highest yearly averaged modelled SO2 concentrations are in the range of 20–40 µg m−3 in proximity to the main sources (Fig. 9d).
In the atmosphere, SO2 reacts with the OH radical and with CH3O2 to give gaseous sulfuric acid (H2SO4). The presence of sulfuric acid in gaseous concentrations of 106–107 molecule cm−3 is necessary in order to observe new particle formation events in the atmosphere (Zhang et al., 2012). In the model, a constant very low boundary condition (BCON) value (10−5 µg m−3) was chosen for H2SO4, leading to a reduced sulfuric acid concentration in the boundary cells (Fig. 9e). Towards the inner domain, H2SO4 quickly increases due to the oxidation of SO2 advected to Hamburg from the regional background. Modelled annual mean H2SO4 peaks in the harbour area with up to 0.018 µg m−3. On spatial average, the H2SO4 annual mean concentration is 0.013 µg m−3, corresponding to 8.2×107 molecule cm−3, which is higher than typical ambient concentrations in the urban atmosphere. For comparison, reported maximum midday H2SO4 concentrations in Beijing are in the range (0.3–1.1) ×107 molecule cm−3 (Wang et al., 2013). Modelled H2SO4 that is too high is explained by the fact that the condensation of sulfuric acid on pre-existing particles is not accounted for in the model. Condensation is the most important atmospheric sink of sulfuric acid and also leads to the formation of particulate sulfate.
Previous studies have shown that levels of PM2.5 in the urban background are to a large extent controlled by atmospheric transport from upwind regions. Modelled PM2.5 levels in the urban background of Hamburg are on annual average 2.5 µg m−3 higher than the regional background. The urban increment due to road traffic exceeds the urban background by 10–20 µg m−3 in the model simulation (Fig. 9f). Hotspots of PM2.5 pollution are found within the harbour area to the southwest of the inner city, where several refineries, power plants and industries are located. These industrial emissions are represented as point source plumes in EPISODE–CityChem.
4.3.2 Modelling of ozone formation
To demonstrate the effect of using an advanced photochemical mechanism for modelling ozone concentrations and ozone production, we compared two EPISODE–CityChem summer (JJA) simulations for Hamburg: one using EmChem09-mod chemistry (EmC09-mod) and the other using the PSS assumption (PSSA) on the Eulerian 3-D grid. The implementation of the PSS involves only three reactions for the equilibrium of (i.e. Reactions R5–R7), while ignoring all other reactions. Spatial maps in Fig. 10a and b show the difference in summer mean concentrations of NO2 and O3 between the runs EmC09-mod and PSSA. In EmC09-mod, additional NO-to-NO2 conversions occur compared to the PSS due to the oxidation of CO and VOCs. The largest impact on NO2, an increase by ca. 1.4–2.3 µg m−3 compared to PSS, happens in the western part of the city, along the river Elbe and in the harbour area south of the river. However, the increased NO2 does not translate into an increase in O3 in this area because modelled NO2 is already high (>35 µg m−3), and additional NO2 decreases the OH concentration via Reaction (R11). Remarkably, photochemical ozone production takes place in the outflow to the southeast of the city and in the urban background southwest of the city. The O3 concentration difference between EmC09-mod and PSSA is in the range 1.6–2.2 µg m−3 in the outflow of polluted air, implying that advanced photochemistry is necessary for a more accurate prediction of O3 in the urban background. The modelled VOC∕NOx ratio in the pollution outflow is ∼0.6, indicating VOC-limited conditions. This is in contrast to the general expectation of finding NOx-limited conditions in the outflow of cities; the low VOC∕NOx ratio might be associated with an overestimation of NOx by the model, as will be shown below. In the inner city and in the vicinity of highly trafficked streets and motorways, photochemical ozone production is very small (difference <0.4 µg m−3), suggesting that the PSS assumption is valid close to the sources of NOx pollution.
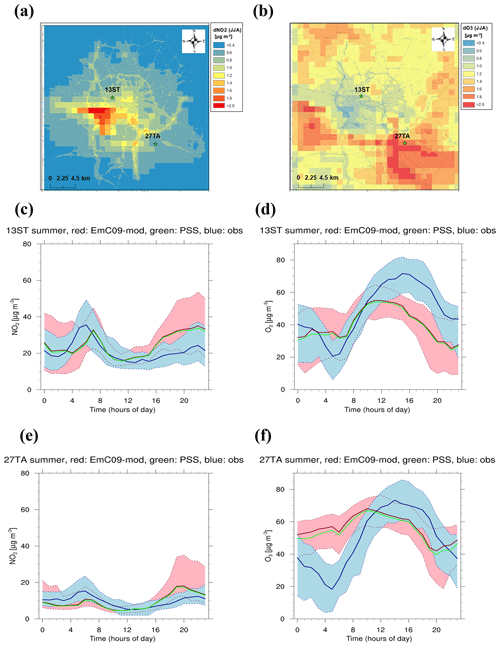
Figure 10Comparison of ozone formation in summer (JJA) with EmChem09-mod (short: EmC09-mod) to the PSS assumption using EPISODE–CityChem in Hamburg: (a) summer mean NO2 concentration difference between a simulation with EmC09-mod and with PSS, (b) summer mean O3 concentration difference between a simulation with EmC09-mod and with PSS, (c) diurnal cycle of summer NO2 at station 13ST, (d) diurnal cycle of summer O3 at station 13ST, (e) diurnal cycle of summer NO2 at station 27TA, (f) diurnal cycle of summer O3 at station 27TA. Modelled median shown as a red line for EmChem09-mod and as a green line for PSS; the observed median is shown as a blue line. Shaded area reflects the bandwidth between the 25th percentile and the 75th percentile (EmChem09-mod, red shaded; observation, blue shaded). Green stars in panels (a) and (b) indicate the locations of stations 13ST and 27TA.
Comparison with measurements was done at two sites: 13ST, an inner-city urban background monitoring site, and 27TA, an urban background station in the SE part of the domain (green stars in Fig. 10a and b). The diurnal cycles of observed median O3 and NO2 concentrations at 13ST for summer (JJA) show a minimum of O3 in the early morning hours between 04:00 and 07:00, as well as a daily maximum of O3 between noon and 16:00, whereas NO2 peaks two times during the day (06:00–08:00 and at 18:00–20:00), coinciding with traffic rush hours (Fig. 10c and d). The diurnal cycles of observations follow a similar pattern at 27TA, but the observed median of NO2 is roughly half that at 13ST (Fig. 10e and f).
At 13ST in the afternoons between 14:00 and 19:00, modelled median O3 concentrations from both EmC09-mod (red line) and PSSA (green line) are below the bandwidth of observed ozone (25th to 75th percentile), and modelled median O3 is ca. 20 µg m−3 lower than the observed median. At both stations, modelled median NO2 in the evening (between 16:00 and 20:00) is higher than the bandwidth of observed NO2. The prediction of an NO2 concentration that is too high in the evening at both sites could be due to several reasons: (1) overestimated emissions of NOx in the urban area; (2) inadequate diurnal profile of traffic emissions regarding the afternoon rush hour; and (3) inaccurate mixing height of the nocturnal BL, leading to enhanced accumulation of NOx emissions in the surface layer of the model.
The future development of the EPISODE model with respect to photochemistry and dispersion near sources is outlined in the following. Specifically, the implementation of the photochemistry in relation to sub-grid modelling and the treatment of aerosol formation on the urban scale will be the focus of the planned development for the next versions of EPISODE–CityChem.
5.1 Photolysis parameterization development
The procedure for calculating photodissociation rates (j values) has not been changed since the original development of the EMEP45 mechanism and is thus as documented by Walker et al. (2003). This procedure was based on the procedure used in the EMEP oxidant model at that time and needs to be revised and updated. The plan is to update the methodology in accordance with the present version of the EMEP model (Simpson et al., 2012). In the present EMEP model, the j values are based on precalculated rates from a detailed radiative-transfer model (PHODIS; Kylling et al., 1998) and interpolated between certain fixed cloud fractions.
5.2 EP10-Plume development
The photochemical steady state might apply in street canyons because the distance between the source and receptor is short there, and hence only the fastest chemical reactions can have a significant influence on photochemical transformation in the street canyon air. On the timescales applying to a street canyon, CO and hydrocarbons can be treated as inert tracers. However, the photochemical scheme applied in the sub-grid component also needs to consider situations with a larger distance between the source and receptor (within a cell of the Eulerian grid).
The PSS relationship is usually not valid in the urban air because organic peroxy radicals compete with O3 to convert NO to NO2 as a result of the oxidation of VOCs in sunlight. EP10-Plume considers only the photochemical degradation of HCHO, but not the oxidation of other reactive VOCs emitted from traffic. It is planned to develop a more detailed chemistry scheme, including VOCs, at the receptor points.
Background ozone concentrations are taken into account in photochemical transformation in the sub-grid component (in both PSS and EP10-Plume). Due to the low ventilation in street canyons, the residence time of pollutants becomes comparable to the timescales of the reactions involved in the PSS assumption, i.e. on the order of tens of seconds. This implies that the exchange rate between the plume from the line source and the background air can become a limiting factor for photochemical transformation in street canyons. The exchange rate is governed by the residence time of the pollutants at the street. However, in the sub-grid line source model, it is assumed that background O3 is instantaneously mixed with NO from the plume of the line source. The rate of reaction (R5) might therefore be overestimated in the sub-grid model, depending on the ambient conditions for plume mixing. Hertel and Berkowicz (1989) take the exchange rate into account for the PSS and suggest that the residence time of pollutants in a street canyon can be approximated by Hsc / σwt, where σwt is the ventilation velocity. The exchange rate of ozone will be considered in the refinement of the photochemistry of the sub-grid component.
5.3 EmChem09-mod developments
5.3.1 Emissions of biogenic VOC
Biogenic emissions of VOCs from trees might be relevant in the VOC-limited regime. Many urban trees, such as European aspen (Populus tremula) and deciduous oaks, emit large amounts of isoprene (Karl et al., 2009). The ozone formation potential of BVOCs emitted from urban trees is sufficiently high to outperform the ozone uptake capacity of the trees (Grote et al., 2016). Missing emissions of BVOCs from trees in urban green parks and at the roadside might partly explain the underestimation of observed O3 in summer in the inner city by the model. Isoprene, in the presence of a sufficiently high level of NOx, can contribute substantially to O3 formation in the urban atmosphere. Further, monoterpenes are considered to be a relevant source of organic peroxy radicals at night (Platt et al., 2002). EmChem09-mod includes chemical reactions for the OH-initiated oxidation of isoprene and for two types of monoterpenes, represented by α-pinene and limonene. It is planned to implement a module for the computation of inline emissions of isoprene and monoterpenes as a function of temperature and solar radiation using data on tree-specific BVOC emission factors by Karl et al. (2009) and high-resolution urban land use information, for example from the Copernicus Urban Atlas.
5.3.2 Secondary formation of particulate matter
Currently, both PM2.5 and PM10 are treated as inert tracers with no secondary aerosol formation. The first step towards a better representation of the particulate phase will be the separation of particulate matter into individual particulate chemical compounds. Traffic emissions of fine (PM2.5) and coarse (PM10–PM2.5) particulate matter will be separated into (non-volatile) primary organic aerosol (POA) and elemental carbon (EC), thereby assuming that any emission of SVOCs and intermediate-volatility volatile organic compounds (IVOCs) immediately undergoes irreversible condensation to the exhaust particulate matter. A clear advantage of the approach is that it avoids the need to discount SVOCs and IVOCs from the city's particulate matter emission inventory. Mineral dust and sea salt imported to the city from the regional background need to be treated as separate compounds. The main focus is on the formation of secondary inorganic aerosol (SIA). Thus, the Model for an Aerosol Reacting System (MARS; Binkowski and Shankar, 1995) could be used for the calculation of the partitioning between gas and fine-mode particles (Simpson et al., 2012). However, MARS does not account for mineral dust components and sea salt; the latter could be relevant for the formation of SIA in coastal cities because it increases the water associated with particulate matter.
5.3.3 Ultrafine particles
Among the emerging, yet unregulated, pollutants in cities are ultrafine particles (UFPs; diameter less than 100 nm). Major sources of UFPs in urban environments are motor vehicle exhaust emissions (e.g. Harrison et al., 2011). Emissions from ships contribute to UFP pollution in harbour cities (Pirjola et al., 2014). High concentrations of ultrafine particles are formed during new particle formation events (Salma et al., 2016). Studies have demonstrated the relevance of episodes of new particle formation in urban environments for cities situated in high insolation regions such as southern Europe (e.g. Pey et al., 2008; Dall'Osto et al., 2013; Brines et al., 2015). UFPs are usually evaluated in terms of particle number (PN) concentrations. In urban environments, UFPs dominate the total PN concentrations, but only make a small contribution to particulate matter. A simplified parameterization for the treatment of the dry deposition and coagulation of particles (Karl et al., 2016) has already been implemented in EPISODE for modelling PN concentrations in Oslo (Kukkonen et al., 2016). The MAFOR aerosol dynamics solver (Karl et al., 2011, 2016) will be implemented in EPISODE–CityChem to compute information on the size distribution of UFPs and the total PN. The solver includes nucleation, coagulation, growth due to the condensation of sulfuric acid, and low volatile and/or semi-volatile organic vapours from biogenic and anthropogenic sources.
5.4 SSCM development
In addition to the dependence on the wind direction (considered through θstreet, the angle between wind direction and road axis), the direct contribution in SSCM is mainly sensitive to the emission intensity of the line source, the street-level wind speed and the integration path. The integration path corresponds to the length of the recirculation zone but extends to Lmax for close-to-parallel wind directions. The length of the recirculation zone (Lrec) depends on the building height along the canyon, while Lmax is a function of the canyon width for large θstreet and a function of the canyon length if θstreet is below 45∘. Both dimensions (Lrec and Lmax) are only roughly estimated in SSCM because it considers only generic street canyon types and not the site-specific street canyon geometry. It is planned to refine SSCM with respect to a better representation of urban street canyon geometry. This could be done, for example, by using spatially resolved information on building height and street canyon width by extracting data from 3-D city building models for each road segment.
5.5 WMPP development
Envisaged future improvements to the WMPP are to extend it to use energy budget methods (Thom et al., 1975; van Ulden and Holtslag, 1985; Fritschen and Simpson, 1989; Tunick, 2006) in combination with net surface radiation and heat flux to determine wind and turbulence profiles and mixing height. The method will be based on data such as solar radiation, cloud cover, air temperature, wind speed, relative humidity, precipitation and surface conditions.
The CityChem extension of the urban AQ model EPISODE (Slørdal et al., 2003, 2008; Hamer et al., 2019) offers a detailed treatment of the atmospheric chemistry in urban areas and a more advanced treatment for dispersion close to point emission sources, such as industrial stacks, and line emissions sources, such as open roads and street canyons. EPISODE consists of a 3-D Eulerian grid CTM with embedded Gaussian dispersion models that track the sub-scale dispersion of pollutants from line and point emission sources until the fine-scale variability becomes unimportant. The EPISODE–CityChem model, which is based on the core of the EPISODE model, integrates the CityChem extension into an urban CTM system with the capability to simulate the photochemistry and dispersion of multiple pollutants on urban scales. Photochemistry on the Eulerian grid is computed using a numerical chemistry solver. Photochemistry in the sub-grid components is solved with a compact reaction scheme, replacing the photo-stationary-state assumption. The integration of SSCM in the sub-grid line source model results in higher concentrations in street canyons because it considers the reduced ventilation inside the canyon and the recirculation of the traffic plume. The integration of WMPP in the sub-grid point source model for dispersion around an elevated point source increases the maximum ground concentration of an inert tracer by a factor of 4 to 6 in neutral and unstable conditions compared to the standard parameterization in EPISODE.
The EPISODE–CityChem model takes into account the fact that long-range transport contributes to urban pollutant levels by using hourly varying pollutant concentrations at the lateral and vertical boundaries from the CMAQ model (Byun and Schere, 2006) as initial and boundary concentrations. The model reads meteorological fields generated by the prognostic meteorology component of TAPM (Hurley, 2008; Hurley et al., 2005) but can also use meteorological fields constrained by observations.
The performance of EPISODE–CityChem was evaluated with a series of tests to study the basic functionalities of the CityChem extension and with a first application to the air quality situation in the city of Hamburg, Germany. The ability to reproduce the temporal variation of major regulated pollutants at AQ monitoring stations in Hamburg was compared to that of the standard EPISODE model and TAPM (AQ model) using identical meteorological fields and emissions. EPISODE–CityChem performs better than EPISODE and TAPM for the prediction of hourly NO2 concentrations at the traffic stations, which is attributable to the street canyon model. EPISODE–CityChem was in better agreement with the observed O3 daily maximum of the 8 h running mean than the other two models. For daily mean PM10 at urban background stations, EPISODE–CityChem and EPISODE gave better results than TAPM, largely due to the use of hourly 3-D boundary conditions from CMAQ. The performance analysis with the FAIRMODE DELTA tool for air quality in Hamburg showed that EPISODE–CityChem fulfils the model performance objectives for NO2 (hourly), O3 (daily max. of the 8 h running mean) and PM10 (daily mean) set forth in the AQD, qualifying the model for use in policy applications.
The effect of using an advanced photochemical mechanism (EmChem09-mod) compared to the PSS assumption for modelling ozone concentrations and ozone production was investigated in summer (JJA) simulations for Hamburg. Photochemical ozone production was found to take place in the outflow of polluted air from the city, implying that advanced photochemistry is necessary for a more accurate prediction of O3 in the urban background. However, the modelled daily maximum O3 in summer afternoons was ca. 25 % lower than observed at an inner-city urban background station. In addition, the model predicted an NO2 concentration in the summer evenings at two urban background stations that is too high. Further investigation of the high modelled evening NOx in summer will require a sensitivity analysis of the various source categories contributing to the NOx levels in the inner city, which remains as a task for future studies.
BVOC emissions from urban parks and forests might partly explain the underestimation of observed ozone in summer. There is evidence that the contribution of BVOC emissions to ozone formation can be up to 60 % during heat waves in densely populated areas (Churkina et al., 2017), depending on the type and amount of urban vegetation. In the future, BVOC emissions in urban areas are expected to become even more important in ozone formation if anthropogenic NMVOC emissions continue to decline as a result of technological progress (Wagner and Kuttler, 2014). The implementation of BVOC emissions as a function of temperature and solar radiation will be the focus of coming developments of the CityChem extension.
Envisaged applications of the EPISODE–CityChem model are urban air quality studies, emission control scenarios in relation to traffic bans introduced in German cities and the source attribution of sector-specific emissions to observed levels of air pollutants. The model can also be utilized in the evaluation of air pollution exposure and in the assessment of adverse health impacts. Features of the model that facilitate its application to urban AQ in cities worldwide include integrated utilities for input preparation and output processing, moderate computational demand, photochemistry options (ozone formation studies), high spatial and temporal resolution, and demonstrated fitness for policy use.
The source codes of the EPISODE–CityChem model version 1.2 and the preprocessing utilities are accessible in release under the RPL licence at https://doi.org/10.5281/zenodo.3063356 (Karl and Ramacher, 2019).
A tar package with example data for a 1-month simulation and the user's guide are included in the release. All preprocessing tools are written in Fortran 90. Software requirements for the utilities and the EPISODE–CityChem model are installation of the GCC–GFortran Fortran 90 compiler (version 4.4. or later) and the NetCDF library (version 3.6.0 or later).
The following datasets are available for download from the HZG FTP server upon request:
-
input data for the 1-year AQ simulation of Hamburg (full set ca. 50 GB);
-
DELTA tool data for comparison of model output and measurements; and
-
model output data from the AQ simulation of Hamburg (full set ca. 100 GB).
Acronym | Description |
AirQUIS | Air quality information system developed at NILU |
AQ | Air quality |
AQD | Air Quality Directive |
APTA | Asthma and Allergies in Changing Climate |
BCON | Boundary condition |
BCONCC | Utility for creating boundary conditions for EPISODE–CityChem |
BL | Boundary layer |
BVOC | Biogenic volatile organic compound |
CAMS | Copernicus Atmosphere Monitoring Service |
CFD | Computational fluid dynamics |
CityChem | City-scale Chemistry extension of the EPISODE model |
CMAQ | Community Multiscale Air Quality |
CO | Carbon monoxide |
CORINE | Coordination of Information on the Environment |
Corr | Correlation coefficient |
COSMO-CLM | COnsortium for SMall-scale MOdeling in CLimate Model |
CRMSE | Centred root mean square error |
CTM | Chemistry-transport model |
DELTA | Evaluation software for diagnostics of air quality model performances |
DWD | German Weather Service |
EC | Elemental carbon |
ECMWF | European Centre for Medium-Range Weather Forecasts |
EMEP45 | EMEP chemistry mechanism with 45 chemical compounds |
EP10-Plume | Compact chemical reaction scheme for the receptor grid |
EPISODE | 3-D Eulerian grid model for urban air quality modelling developed at NILU |
EU | European Union |
FAIRMODE | Forum for Air Quality Modelling in Europe |
FMI | Finnish Meteorological Institute |
GenChem | Chemical preprocessor of the EMEP model |
GRETA | Gridding Emission Tool for ArcGIS |
HBEFA | Handbook Emission Factors for Road Traffic |
HCHO | Formaldehyde |
HIWAY-2 | Highway Air Pollution Model 2 |
HNO3 | Nitric acid |
H2SO4 | Sulfuric acid |
HO2 | Hydroperoxyl radical |
IOA | Index of agreement |
IOAPI | Input/Output Application Programming Interface |
IVOC | Intermediate-volatility organic compound |
IUPAC | International Union of Pure and Applied Chemistry |
JJA | June–July–August |
MAFOR | Multicomponent Aerosol Formation model |
MARS | Model for an Aerosol Reacting System |
MPC | Model performance criteria |
MQI | Model quality indicator |
NILU | Norwegian Institute for Air Research |
NMB | Normalized mean bias |
NMVOC | Non-methane volatile organic compound |
NO | Nitric oxide |
NO2 | Nitrogen dioxide |
NO3 | Nitrate radical |
Acronym | Description |
NOx | Nitrogen oxides (sum of NO and NO2) |
N2O5 | Dinitrogen pentoxide |
NWP | Numerical weather prediction |
O3 | Ozone |
ODE | Ordinary differential equation |
OH | Hydroxyl radical |
OSPM | Operational Street Pollution Model |
PAN | Peroxyacetyl nitrate |
PM | Particulate matter |
PM2.5 | Particulate matter with an aerodynamic diameter of less than 2.5 µm |
PM10 | Particulate matter with an aerodynamic diameter of less than 10 µm |
PN | Particle number |
POA | Primary organic aerosol |
PRTR | Pollutant Release and Transfer Register |
PSS | Photo-stationary state |
REPARTEE | Regents Park and Tower Environmental Experiment |
RMSE | Root mean square error |
RO2 | Organic peroxy radical |
SEGPLU | Gaussian segmented plume trajectory model |
SIA | Secondary inorganic aerosol |
SNAP | Selected Nomenclature for sources of Air Pollution |
SO2 | Sulfur dioxide |
SOA | Secondary organic aerosol |
SON | September–October–November |
SSCM | Simplified street canyon model |
SD | Standard deviation |
SVOC | Semi-volatile organic compound |
TAPM | The Air Pollution Model, developed at CSIRO |
UBA | German Federal Environmental Agency |
UECT | Urban Emission Conversion Tool |
UFP | Ultrafine particle (diameter less than 100 nm) |
UTM | Universal Transverse Mercator |
UV | Ultraviolet |
VOC | Volatile organic compound |
WMPP | WORM Meteorological Pre-Processor |
WORM | Weak-wind Open Road Model |
WRF–EMEP | Weather Research and Forecasting–European Monitoring and Evaluation |
The photodissociation coefficients of photolysis reactions are calculated according to the expression
where θz is the zenith angle, α0 denotes the optical air mass for large zenith angles and CLFn is the cloud correction factor for reaction number n:
The actual fractional cloud cover of low clouds (0.0 to 1.0), CL, is either based on observational data of cloud coverage or the total solar radiation field (calculated by TAPM) using the approximation for the transmission coefficient of shortwave radiation suggested by Burridge and Gadd, as given in Stull (1988). Empirical values for ε1, ε2, ε3 and ε4 for the photolysis reactions are tabulated in Table S1.
C1 Dry deposition
The dry deposition of gases and aerosols is treated based on the resistance analogy, wherein the inverse deposition velocity of gases is the sum of three resistances in series: the aerodynamic resistance Ra (m s−1), the quasi-laminar layer resistance, Rb (m s−1) and the surface (canopy) resistance Rc (m s−1). Gravitational settling of coarse particles is considered for the dry deposition of aerosols. The loss rate of a gaseous species i to the land or water surface, within a volume of unit area and height Δz (here the thickness of the lowermost layer), is given by the product of the deposition velocity Vdry (m s−1) at the reference height zref (here the midpoint height of the lowermost model layer) and the concentration (Ci) at that height:
The dry deposition velocity of gases, Vdry,g, is calculated as (Simpson et al., 2003)
The aerodynamic resistance at zref is calculated based on surface layer similarity theory as a function of the Monin–Obukhov length and the friction velocity:
where ΨH is the influence function for heat transfer, z0 is the surface roughness (for momentum) and L is the Monin–Obukhov length. The quasi-laminar layer resistance is calculated according to the parameterization given by Simpson et al. (2003). The canopy resistance, i.e. deposition due to the capture of pollutants by the surface, is currently only considered by a minimum value, i.e. m s−1. The parameterization of the canopy resistance is complex, since it depends on both surface characteristics and the chemical characteristics of the depositing gas.
The dry deposition velocity of particles, Vdry,p, is calculated as (Simpson et al., 2003)
where υs is the gravitational settling velocity and the other terms are as for gases.
Equation (C4) involves the assumption that all deposited particles stick to the surface so that the surface resistance becomes zero. The dry deposition velocity of atmospheric aerosols depends on their sizes. The current formulation distinguishes between PM2.5 and PM10, which are presently assigned the particle diameters of 0.3 and 4 µm. All the resistances are integrated over the aerosol sizes, assuming a log-normal particle size distribution with geometric standard deviations of 2.0 and 2.2 µm for PM2.5 and PM10, respectively.
C2 Wet deposition
Wet deposition is described as a sink term within the advection–diffusion equation and can be parameterized by , where Ci is the grid concentration of a gaseous or particulate species and Λ is the scavenging coefficient (s−1). Wet scavenging is different from zero in grid cells in which precipitation (rainfall or snowfall) occurs. The chosen crude approach for representing wet deposition treats in-cloud scavenging in the same way as below-cloud scavenging. Further, the cloud base is assumed to be at the model top, which means that scavenging occurs throughout the entire 1-D model column for which the precipitation rate in the surface grid cell is greater than zero. For the short-term estimation of near-ground concentrations in urban areas, below-cloud scavenging is expected to be the dominant wet removal process. A more accurate treatment of below-cloud scavenging requires knowledge of the cloud base height (which is not standard output of TAPM) in order to limit wet deposition to the model layers that are actually affected by raining clouds and to separate between in-cloud and below-cloud scavenging. The scavenging of gases is calculated as (Simpson et al., 2003)
where Wsub is the sub-cloud scavenging coefficient for gases, supplied as a constant value by the model user, Pr (kg m2 s−1) is the precipitation rate, Hsub is the scavenging depth (corresponding to the total vertical depth of the model) and ρw is the water density (1000 kg m−3).
Precipitation is a 2-D surface field, either from observations of precipitation rate or computed by TAPM. The wet deposition rate of particulate compounds is calculated as (Simpson et al., 2003)
where Vdr is the raindrop fall speed (Vdr=5 m s−1), A=5 is an empirical coefficient when the Marshall–Palmer size distribution is assumed for raindrops and is the tabulated size-dependent collection efficiency of aerosols by the raindrops.
The utility BCONCC v2.1 (included in the CityChem distribution) is used to produce EPISODE-format binary files containing hourly varying 3-D boundary concentrations for the most relevant chemical compounds. BCONCC makes use of the IOAPI version 3.1 library (https://www.cmascenter.org/ioapi, last access: 29 July 2019) to access CMAQ output files. The IOAPI (Models-3/EDSS Input/Output Application Programming Interface) provides a variety of data structure types for organizing the data and a set of data access routines.
The preparation of chemical boundary conditions from the CMAQ model output is done in two steps. First, the city's 3-D domain extent plus one grid cell to each side is cut out from the CMAQ model grid, interpolating the hourly concentrations to the horizontal main grid resolution of EPISODE using bilinear interpolation. Second, EPISODE-format binary files for boundary conditions (BCON files) containing background concentrations of all individual CityChem compounds are created for the defined model domain in the required input format. Linear interpolation is used to convert concentrations from the vertical layers of the CMAQ model to the vertical layers of the EPISODE–CityChem model. Temperature and pressure from the METCRO3D file (meteorological input file of the CMAQ simulation) are used to convert the concentrations of gaseous compounds from mixing ratios to mass-based concentrations.
The background concentrations are adopted for the grid cells directly adjacent to the grid cells of the model domain (with nx×ny cells per model layer) and also for the vertical model layer that is on top of the highest model layer. Boundary conditions from CMAQ concentrations are created for the gas-phase chemical compounds: O3, NO, NO2, H2O2, SO2, HCHO, CO, N2O5, HNO3, PAN and the individual VOCs. Boundary conditions for PM2.5 include primary aerosol components: EC, POA, sea salt (NaCl) and mineral dust. They also include secondary inorganic aerosol (SIA) components: sulfate (), ammonium (), nitrate () and SOA (PM2.5 was defined including modes I and J of the CMAQ aerosol components). Since the focus of the AQ study is mainly on photochemistry and fine particulate mass, boundary conditions for PM10 were approximated as [PM10] = [PM2.5] × 1.5.
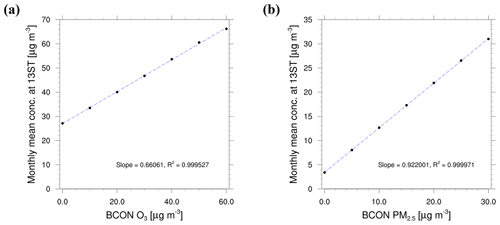
Figure D1Test of the boundary conditions for lateral entrainment into the model domain of Hamburg. Relationship between the monthly mean concentration (July 2012) in the grid cell in which station 13ST is located and the BCON offset added to the CMAQ concentrations at the lateral boundaries: (a) O3 and (b) PM2.5. Zero BCON offset corresponds to the original boundary conditions from CMAQ.
The entrainment of O3 and PM2.5 from the regional background into the model domain and their effect on the concentrations inside the domain was studied with a numerical experiment using the model setup for Hamburg as described in Sect. 4.1.1. A constant concentration offset (BCON offset) was added to the hourly CMAQ concentrations at the lateral and vertical boundaries. In a series of test runs, the BCON offset of O3 was increased from 0 to 60 µg m−3 in steps of 10 µg m−3, and the BCON offset of PM2.5 was increased from 0 to 30 µg m−3 in steps of 5 µg m−3 between the runs. A linear relationship was found between the monthly mean concentration (July 2012) of O3 and PM2.5 in the grid cell in which the inner-city urban background station 13ST is located and the BCON offset (Fig. D1). Fitting a linear regression model of the form to the data gave a slope of 0.66 and 0.93 for O3 and PM2.5, respectively. Since PM2.5 is treated as a chemical non-reactive tracer in the model, the reason for a slope smaller than 1 is removal by dry and wet deposition within the study domain. For ozone, the addition of an offset to the concentrations at the boundaries does not fully propagate into the grid cell concentration at station 13ST due to removal by dry deposition, photolysis by sunlight and the chemical reaction with NOx emitted in the urban area.
In the statistical analysis of the model performance the following statistical indicators are used: overall bias (Bias), normalized mean bias (NMB), standard deviation (SD), root mean square error (RMSE), correlation coefficient (Corr) and index of agreement (IOA).
The overall bias captures the average deviations between the model and observed data and is defined as follows:
where M and O stand for the model and observation results, respectively. The overbars indicate the time average over N time intervals (number of observations).
The normalized mean bias is given by
The root mean square error combines the magnitudes of the errors in predictions for various times into a single measure and is defined as
where subscript i indicates the time step (time of observation values). RMSE is a measure of accuracy to compare prediction errors of different models for particular data and not between datasets, as it is scale dependent.
The correlation coefficient (Pearson r) for the temporal correlation is defined as
SDM and SDO are the standard deviation of the model and observation data, respectively. The standard deviations are
and
The index of agreement is defined as
An IOA value close to 1 indicates agreement between modelled and observed data. The denominator in Eq. (E6) is referred to as the potential error.
The model performance criterion (MPC) for dispersion models is the minimum level of quality that has to be achieved for use in policy support related to AQ regulations. The MPC implemented in the FAIRMODE DELTA tool has been constructed on the basis of the observation uncertainty (Thunis et al., 2012a).
The uncertainty of a single observation value U95(Oi) is expressed as
where represents the relative measurement uncertainty estimated around a reference value, RV, for a given time averaging, e.g. the hourly or daily limit values of the Air Quality Directive (AQD). The fraction of uncertainty around the RV is given by α2. Most commonly, the expanded uncertainty is scaled by using a value of 2 for the coverage factor, k, to achieve a level of confidence of approximately 95 %.
The root mean square of the observation uncertainty (RMSU) is then
A model quality indicator (MQI) is defined as the ratio between the model–observation bias and a quantity proportional to the observation uncertainty as
with β=2 in the DELTA tool.
Using Eq. (E8), the MQI can be generalized to a time series by
The model quality objective (MQO) is fulfilled when the MQI is less than or to equal 1.
A characteristic of the MQI is that errors in Bias, SD and Corr are condensed into a single indicator value, as follows:
From Eq. (E11), the model performance criterion (MPC) for the error of bias, standard deviation and correlation can be derived. The bias MPC is derived from Eq. (E11) assuming Corr = 1 and SDM=SDO, as follows:
The MQI as described by Eq. (E10) is used as the main indicator in the target diagram (Thunis et al., 2012a). In the normalized target diagram, it represents the distance between the origin and a given station point. The normalized bias (first term on the right-hand side of Eq. E11) is used for the y axis, while the centred root mean square error (CRMSE) (sum of the two last terms on the right-hand side of Eq. E11) is used to define the x axis. More details on the normalized target diagram can be found in Thunis et al. (2012a).
The supplement related to this article is available online at: https://doi.org/10.5194/gmd-12-3357-2019-supplement.
MK was mainly responsible for the development of the CityChem extension, development of the research questions, most of the writing, evaluating air concentration data, and creating a framework for data processing, visualization and plotting. SEW drafted the overall structure of the paper, developed EMEP45 and WMPP, which became part of the CityChem extension, and implemented TWOSTEP for solving photochemistry on the Eulerian grid. SS contributed to the development of EMEP45. MOPR prepared input datasets for the air quality study of Hamburg, performed TAPM simulations to produce air concentration data and meteorological input data, evaluated meteorological data from TAPM, tested early versions of the EPISODE–CityChem model, and assisted with the setup and use of the DELTA tool. All co-authors contributed to the writing of the paper and discussion of the model results.
The authors declare that they have no conflict of interest.
This work was prepared as part of the ERA-PLANET transnational project SMURBS (SMart URBan Solutions for air quality, disasters and city growth). We thank the city of Hamburg, Ministry of Environment and Energy (Behörde für Umwelt Energie Hamburg, BUE) for providing AQ monitoring data and the Agency for Emission Control and Plant Installations (Amt für Immissionsschutz und Betriebe, IB) for providing traffic emission data and industrial emission totals. We kindly acknowledge Ingo Lange and the Meteorological Institute of Universität Hamburg for compiling and providing meteorological measurement data. Moreover, we would like to thank Stefan Feigenspan and Stephan Nordmann of the German Federal Environmental Agency (Umweltbundesamt, UBA) for providing gridded emission totals for Hamburg. Peter Hurley (CSIRO) is acknowledged for support with TAPM. Britt Ann Høiskar (NILU) is thanked for permission to distribute the CityChem extension to the EPISODE model under the RPL licence. We thank Paul Hamer (NILU) for the coordination of the two-part series publication on EPISODE and for the graphics in Fig. 1. ECMWF ERA 5 reanalysis ensemble means for 2012 are distributed by the Copernicus Climate Change Service. The air quality model CMAQ is developed and maintained by the U.S. Environmental Protection Agency (U.S. EPA). COSMO-CLM is the community model for German climate research. The simulations with COSMO-CLM and CMAQ were performed at the German Climate Computing Centre (DKRZ) within the project “Regional Atmospheric Modelling” (project ID 0302).
This research has been supported by the ERA-PLANET, EU Horizon 2020 Framework Programme (grant no. 689443).
The article processing charges for this open-access
publication were covered by a Research
Centre of the Helmholtz Association.
This paper was edited by Simone Marras and reviewed by two anonymous referees.
Andersson-Sköld, Y. and Simpson, D.: Comparison of the chemical schemes of the EMEP MSC-W and the IVL photochemical trajectory models, Atmos. Environ., 33, 1111–1129, 1999. a
Appel, K. W., Pouliot, G. A., Simon, H., Sarwar, G., Pye, H. O. T., Napelenok, S. L., Akhtar, F., and Roselle, S. J.: Evaluation of dust and trace metal estimates from the Community Multiscale Air Quality (CMAQ) model version 5.0, Geosci. Model Dev., 6, 883–899, https://doi.org/10.5194/gmd-6-883-2013, 2013. a, b
Atkinson, R., Baulch, D. L., Cox, R. A., Hampson, R. F., Kerr, J. A., Rossi, M. J., and Troe, J.: Evaluated kinetic and photochemical data for atmospheric chemistry: Supplement VIII, Halogen species – IUPAC subcommittee on gas kinetic data evaluation for atmospheric chemistry, J. Phys. Chem. Ref. Data, 29, 167–266, 2000. a
Aulinger, A., Matthias, V., Zeretzke, M., Bieser, J., Quante, M., and Backes, A.: The impact of shipping emissions on air pollution in the greater North Sea region – Part 1: Current emissions and concentrations, Atmos. Chem. Phys., 16, 739–758, https://doi.org/10.5194/acp-16-739-2016, 2016. a
Baklanov, A., Hänninen, O., Slørdal, L. H., Kukkonen, J., Bjergene, N., Fay, B., Finardi, S., Hoe, S. C., Jantunen, M., Karppinen, A., Rasmussen, A., Skouloudis, A., Sokhi, R. S., Sørensen, J. H., and Ødegaard, V.: Integrated systems for forecasting urban meteorology, air pollution and population exposure, Atmos. Chem. Phys., 7, 855–874, https://doi.org/10.5194/acp-7-855-2007, 2007. a
Bergström, R., Denier van der Gon, H. A. C., Prévôt, A. S. H., Yttri, K. E., and Simpson, D.: Modelling of organic aerosols over Europe (2002–2007) using a volatility basis set (VBS) framework: application of different assumptions regarding the formation of secondary organic aerosol, Atmos. Chem. Phys., 12, 8499–8527, https://doi.org/10.5194/acp-12-8499-2012, 2012. a
Berkowicz, R., Hertel, O., Larsen, S. E., Sørensen, N. N., and Nielsen, M.: Modelling traffic pollution in streets, Ministry of Environment and Energy, National Environmental Research Institute, Roskilde, Denmark, available at: http://www2.dmu.dk/1_viden/2_Miljoe-tilstand/3_luft/4_spredningsmodeller/5_OSPM/5_description/ModellingTrafficPollution_report.pdf (last access: 21 October 2018), 1997. a, b, c, d, e
Binkowski, F. and Shankar, U.: The Regional Particulate Matter Model .1. Model description and preliminary results, J. Geophys. Res., 100, 26191–26209, 1995. a
BKG: Digitales Geländemodell Gitterweite 200 m, Bundesamt für Kartographie und Geodäsie, available at: http://www.geodatenzentrum.de/docpdf/dgm200.pdf (last access: 21 October 2018), 2013. a
Borge, R., Lumbreras, J., Perez, J., de la Paz, D., Vedrenne, M., de Andres, J. M., and Rodriguez, M. E.: Emission inventories and modelling requirements for the development of air quality plans. Application to Madrid (Spain), Sci. Total Environ., 466, 809–819, 2014. a
Bott, A.: A positive definite advection scheme obtained by nonlinear renormalization of the advective fluxes, Mon. Weather Rev. 117, 1006–1015, 1989. a
Briggs, G. A.: Plume Rise, US Atomic Energy Commission, Springfield, USA, 1–81, 1969. a
Briggs, G. A.: Some recent analyses of plume rise observation, in: Proceedings of the Second International Clean Air Congress, edited by: Englund, H. M. and Berry, W. T., Academic Press, Washington, USA, 6–11 December 1970, 1029–1032, 1971. a
Briggs, G. A.: Plume rise predictions, in: Lectures on Air Pollution and Environmental Impact Analysis, edited by: Haugen, D. A., Amer. Meteor. Soc., Boston, MA, USA, 59–111, 1975. a
Brines, M., Dall'Osto, M., Beddows, D. C. S., Harrison, R. M., Gómez-Moreno, F., Núñez, L., Artíñano, B., Costabile, F., Gobbi, G. P., Salimi, F., Morawska, L., Sioutas, C., and Querol, X.: Traffic and nucleation events as main sources of ultrafine particles in high-insolation developed world cities, Atmos. Chem. Phys., 15, 5929–5945, https://doi.org/10.5194/acp-15-5929-2015, 2015. a
Bruemmer, B., Lange, I., and Konow, H.: Atmospheric boundary layer measurements at the 280 m high Hamburg weather mast 1995–2011: Mean annual and diurnal cycles, Meteorol. Z., 21, 319–335. https://doi.org/10.1127/0941-2948/2012/0338, 2012. a
Byun, D. W. and Schere, K. L.: Review of the governing equations, computational algorithms and other components of the Models-3 Community Multiscale Air Quality (CMAQ) Modeling System, Appl. Mech. Rev. 59, 51–77, https://doi.org/10.1115/1.2128636, 2006. a, b, c
Byun, D. W., Young, J., Pleim, J., Odman, M. T., and Alapaty, K.: Chapter 7, Numerical Transport Algorithms for the Community Multiscale Air Quality (CMAQ) Chemical Transport Model in Generalized Coordinates, in: Science Algorithms of the EPA Models-3 Community Multiscale Air Quality (CMAQ) Modeling System. EPA/600/R-99/030, U.S. Environmental Protection Agency, Office of Research and Development, Washington, DC, USA, 1999. a, b, c
Calvert, J., Atkinson, J., Kerr, J., Madronich, S., Moortgat, G. K., Wallington, T., and Yarwood, G.: Mechanisms of the atmospheric oxidation of the alkenes, Oxford University Press, New York, NY, USA, 2000. a
Carslaw, D. C. and Beevers, S. D.: Estimations of road vehicle primary NO2 exhaust emission fractions using monitoring data in London, Atmos. Environ., 39, 167–177, 2005. a
Carslaw, D. C. and Rhys-Tyler, G.: New insights from comprehensive on-road measurements of NOx, NO2 and NH3 from vehicle emission remote sensing in London, UK, Atmos. Environ., 81, 339–347, 2012. a
Churkina, G., Kuik, F., Bonn, B., Lauer, A., Grote, R., Tomiak, K., and Butler, T. M.: Effect of VOC emissions from vegetation on air quality in Berlin during a heatwave, Environ. Sci. Technol., 51, 6120–6130, https://doi.org/10.1021/acs.est.6b06514, 2017. a
CLC: Copernicus Land Monitoring Service, available at: http://land.copernicus.eu/pan-european/corine-land-cover/clc-2012/ (last access: 21 October 2018), 2012. a, b
Cuvelier, C., Thunis, P., Vautard, R., Amann, M., Bessagnet, B., Bedogni, M., Berkowicz, R., Brandt, J., Brocheton, F., Builtjes, P., Carnavale, C., Coppalle, A., Denby, B., Douros, J., Graf, A., Hellmuth, O., Hodzic, A., Honoré, C., Jonson, J., Kerschbaumer, A., de Leeuw, F., Minguzzi, E., Moussiopoulos, N., Pertot, C., Peuch, V. H., Pirovano, G., Rouil, L., Sauter, F., Schaap, M., Stern, R., Tarrason, L., Vignati, E., Volta, M., White, L., Wind, P., and Zuber, A.: CityDelta: a model intercomparison study to explore the impact of emission reductions in European cities in 2010, Atmos. Environ., 41, 189–207, 2007. a
Dall'Osto, M., Querol, X., Alastuey, A., O'Dowd, C., Harrison, R. M., Wenger, J., and Gómez-Moreno, F. J.: On the spatial distribution and evolution of ultrafine particles in Barcelona, Atmos. Chem. Phys., 13, 741–759, https://doi.org/10.5194/acp-13-741-2013, 2013. a
Denby, B. R., Sundvor, I., Schneider, P., Thanh, D. V.: Air quality maps of NO2 and PM10 for the region including Stavanger, Sandnes, Randaberg and Sola. Norwegian Institute for Air Research, NILU TR 01/2014, Kjeller, Norway, 2014. a, b
Dodge, M. C.: Effect of selected parameters on predictions of a photochemical model, US Environmental Protection Agency, EPA-600/3-77/048, Research Triangle Park, NC, USA, 1977. a
EC: Directive 2008/50/EC of the European Parliament and of the Council of 21 May 2008 on ambient air quality and cleaner air for Europe, European Commission, Official Journal of the European Union L152, Luxembourg, 2008. a, b
EEA: Air quality in Europe – 2015 Report, European Environment Agency, EEA Report. No. 5/2015, Copenhagen, Denmark, 57 pp., 2015. a
Ehlers, C., Klemp, D., Rohrer, F., Mihelcic, D., Wegener, R., Kiendler-Scharr, A., and Wahner, A.: Twenty years of ambient observations of nitrogen oxides and specified hydrocarbons in air masses dominated by traffic emissions in Germany, Faraday Discuss., 189, 407–437, 2016. a, b
FAIRMODE: Modeling quality objectives in the framework of the FAIRMODE project: working document, edited by: Pernigotti, D., Gerboles, M., and Thunis, P., available at: http://fairmode.jrc.ec.europa.eu/document/fairmode/WG1/Working note_MQO.pdf (last access: 22 October 2018), 2014. a
Fritschen, L. J. and Simpson, J. R.: Surface energy and radiation balance systems: General description and improvements, J. Appl. Meteor., 28, 680–689, 1989. a
Geyer, B.: High-resolution atmospheric reconstruction for Europe 1948–2012: coastDat2, Earth Syst. Sci. Data, 6, 147–164, https://doi.org/10.5194/essd-6-147-2014, 2014. a
Grice, S., Stedman, J., Kent, A., Hobson, M., Norris, J., Abbott, J., and Cooke, S.: Recent trends and projections of primary NO2 emissions in Europe, Atmos. Environ., 43, 2154–2167, 2009. a
Grote, R., Samson, R., Alonso, R., Amorim, J. H., Cariñanos, P., Churkina, G., Fares, S., Thiec, D. L., Niinemets, Ü., Mikkelsen, T. N., Paoletti, E., Tiwary, A., and Calfapietra, C.: Functional traits of urban trees: air pollution mitigation potential, Front Ecol. Environ., 14, 543–550, https://doi.org/10.1002/fee.1426, 2016. a
Hamer, P. D., Walker, S. E., Sousa-Santos, G., Vogt, M., Vo-Thanh, D., Lopez-Aparicio, S., Ramacher, M. O. P., and Karl, M.: The urban dispersion model EPISODE. Part 1: A Eulerian and sub-grid-scale air quality model and its application in Nordic winter conditions, submitted to Geosci. Model Dev., 2019. a, b, c, d, e, f, g, h, i, j
Hanna, S. R., Briggs, G. A., and Hosker Jr., R. P.: Handbook on Atmospheric Diffusion, edited by: Smith, J. S., DOE/TIC-11223, Technical Information Center, US Department of Energy, Springfield, USA, 1982. a
Harrison, R. M., Beddows, D. C. S., and Dall'Osto M.: PMF analysis of wide-range particle size spectra collected on a major highway, Environ. Sci. Technol., 45, 5522–5528, 2011. a
Harrison, R. M., Dall'Osto, M., Beddows, D. C. S., Thorpe, A. J., Bloss, W. J., Allan, J. D., Coe, H., Dorsey, J. R., Gallagher, M., Martin, C., Whitehead, J., Williams, P. I., Jones, R. L., Langridge, J. M., Benton, A. K., Ball, S. M., Langford, B., Hewitt, C. N., Davison, B., Martin, D., Petersson, K. F., Henshaw, S. J., White, I. R., Shallcross, D. E., Barlow, J. F., Dunbar, T., Davies, F., Nemitz, E., Phillips, G. J., Helfter, C., Di Marco, C. F., and Smith, S.: Atmospheric chemistry and physics in the atmosphere of a developed megacity (London): an overview of the REPARTEE experiment and its conclusions, Atmos. Chem. Phys., 12, 3065–3114, https://doi.org/10.5194/acp-12-3065-2012, 2012. a
Hertel, O. and Berkowicz, R.: Modelling Pollution from Traffic in a Street Canyon, Evaluation of Data and Model Development, National Environmental Research Institute, Roskilde, Denmark, 1989. a, b, c, d
Högström, U.: Review of some basic characteristics of the atmospheric surface layer, Bound.-Lay. Meteorol., 78, 215–246, 1996. a
Holtslag, A. A. M. and de Bruin, H. A. R.: Applied modelling of the nighttime surface energy balance over land, J. Appl. Meteorol., 27, 689–704, 1998. a
Hurley P.: TAPM v. 4, Part 1: Technical Description, CSIRO Marine and Atmospheric Research Paper No.25, Aspendale, Vic., Australia, ISBN: 978-1-921424-71-7, 2008. a, b, c
Hurley, P., Physick, W., and Luhar, A.: TAPM – a practical approach to prognostic meteorological and air pollution modelling, Environ. Modell. Softw., 20, 737–752, https://doi.org/10.1016/j.envsoft.2004.04.006, 2005. a, b, c
Karl, M. and Ramacher, M.: City-scale Chemistry Transport Model EPISODE-CityChem (Release version 1.2.1), Zenodo, https://doi.org/10.5281/zenodo.3063356, 2019. a
Karl, M., Guenther, A., Köble, R., Leip, A., and Seufert, G.: A new European plant-specific emission inventory of biogenic volatile organic compounds for use in atmospheric transport models, Biogeosciences, 6, 1059–1087, https://doi.org/10.5194/bg-6-1059-2009, 2009. a, b
Karl, M., Gross, A., Pirjola, L., and Leck, C.: A new flexible multicomponent model for the study of aerosol dynamics in the marine boundary layer, Tellus B, 63, 1001–1025, 2011. a
Karl, M., Castell, N., Simpson, D., Solberg, S., Starrfelt, J., Svendby, T., Walker, S.-E., and Wright, R. F.: Uncertainties in assessing the environmental impact of amine emissions from a CO2 capture plant, Atmos. Chem. Phys., 14, 8533–8557, https://doi.org/10.5194/acp-14-8533-2014, 2014. a, b
Karl, M., Svendby, T., Walker, S.-E., Velken, A. S., Castell, N., and Solberg, S.: Modelling atmospheric oxidation of 2-aminoethanol (MEA) emitted from post-combustion capture using WRF-Chem, Sci. Total Environ., 527–528, 185–202, https://doi.org/10.1016/j.scitotenv.2015.04.108, 2015. a
Karl, M., Kukkonen, J., Keuken, M. P., Lützenkirchen, S., Pirjola, L., and Hussein, T.: Modeling and measurements of urban aerosol processes on the neighborhood scale in Rotterdam, Oslo and Helsinki, Atmos. Chem. Phys., 16, 4817–4835, https://doi.org/10.5194/acp-16-4817-2016, 2016. a, b
Kastner-Klein, P., Berkowicz, R., and Plate, E. J.: Modelling of vehicle induced turbulence in air pollution studies for streets, Int. J. Environ. Pollut., 14, 496–507, https://doi.org/10.1504/IJEP.2000.000573, 2000. a
Keuken, M. P., Roemer, M. G. M., Zandveld, P., Verbeek, R. P., and Velders, G. J. M.: Trends in primary NO2 and exhaust PM emissions from road traffic for the period 2000–2020 and implications for air quality and health in the Netherlands, Atmos. Environ., 54, 313–319, 2012. a
Kukkonen, J., Karl, M., Keuken, M. P., Denier van der Gon, H. A. C., Denby, B. R., Singh, V., Douros, J., Manders, A., Samaras, Z., Moussiopoulos, N., Jonkers, S., Aarnio, M., Karppinen, A., Kangas, L., Lützenkirchen, S., Petäjä, T., Vouitsis, I., and Sokhi, R. S.: Modelling the dispersion of particle numbers in five European cities, Geosci. Model Dev., 9, 451–478, https://doi.org/10.5194/gmd-9-451-2016, 2016. a
Kylling, A., Bais, A. F., Blumthaler, M., Schreder, J., Zerefos, C. S., and Kosmidis, E.: Effect of aerosols on solar UV irradiances during the Photochemical Activity and Solar Radiation campaign, J. Geophys. Res., 103, 26051–26060, https://doi.org/10.1029/98JD02350, 1998. a
LGV: 3-D city model LoD1-DE Hamburg, Freie und Hansestadt Hamburg, Landesbetrieb Geoinformation und Vermessung, available at: https://www.govdata.de/daten/-/details/3d-stadtmodell-hamburg1 (last access: 21 October 2018), 2014. a
Matthias, V., Ramacher, M. O. P., and Quante, M.: Air Quality in Hamburg, Luftqualität in Hamburg, edited by: Fehr, R. and Trojan, A., in: Nachhaltige StadtGesundheit Hamburg, Oekonom, 568 pp., ISBN: 978-3-96238-059-5, Munich, Germany, 2018 (in German). a
McRae, G. J., Goodin, W. R., and Seinfeld, J. H.: Development of a second-generation mathematical model for urban air pollution – 1. Model formulation, Atmos. Environ., 16, 679–696, 1982. a
Monteiro, A., Durka, P., Flandorfer, C., Georgieva, E., Guerreiro, C., Kushta, J., Malherbe, L., Maiheu, B., Miranda, A. I., Santos, G., Stocker, J., Trimpeneers, E., Tognet, F., Stortini, M., Wesseling, J., Janssen, S., and Thunis, P.: Strengths and weaknesses of the FAIRMODE benchmarking methodology for the evaluation of air quality models, Air Qual. Atmos. Hlth., 11, 373–383, https://doi.org/10.1007/s11869-018-0554-8, 2018. a, b
Ottosen, T.-B., Kakosimos, K. E., Johansson, C., Hertel, O., Brandt, J., Skov, H., Berkowicz, R., Ellermann, T., Jensen, S. S., and Ketzel, M.: Analysis of the impact of inhomogeneous emissions in the Operational Street Pollution Model (OSPM), Geosci. Model Dev., 8, 3231–3245, https://doi.org/10.5194/gmd-8-3231-2015, 2015. a, b
Pernigotti, D., Thunis, P., Gerboles, M., and Belis, C.: Model quality objectives based on measurement uncertainty: Part II: PM10 and NO2, Atmos. Environ., 79, 869–878, 2013. a, b
Petersen, W. B.: User's Guide for Hiway-2: A Highway Air Pollution Model, US Environmental Protection Agency, EPA-600/8-80-018, Research Triangle Park, NC, USA, 1980. a
Petetin, H., Beekmann, M., Colomb, A., Denier van der Gon, H. A. C., Dupont, J.-C., Honoré, C., Michoud, V., Morille, Y., Perrussel, O., Schwarzenboeck, A., Sciare, J., Wiedensohler, A., and Zhang, Q. J.: Evaluating BC and NOx emission inventories for the Paris region from MEGAPOLI aircraft measurements, Atmos. Chem. Phys., 15, 9799–9818, https://doi.org/10.5194/acp-15-9799-2015, 2015. a
Pey, J., Rodríguez, S., Querol, X., Alastuey, A., Moreno, T., Putaud, J. P., and Van Dingenen, R.: Variations of urban aerosols in the western Mediterranean, Atmos. Environ., 42, 9052–9062, 2008. a
Pirjola, L., Pajunoja, A., Walden, J., Jalkanen, J.-P., Rönkkö, T., Kousa, A., and Koskentalo, T.: Mobile measurements of ship emissions in two harbour areas in Finland, Atmos. Meas. Tech., 7, 149–161, https://doi.org/10.5194/amt-7-149-2014, 2014. a
Platt, U., Alicke, B., Dubois, R., Geyer, A., Hofzumahaus, A., Holland, F., Martinez, M., Mihelcic, D., Klüppel, T., Lohrmann, B., Pätz, W., Perner, D., Rohrer, F., Schäfer, J., and Stutz, J.: Free radicals and fast photochemistry during BERLIOZ, J. Atmos. Chem., 42, 359–394, 2002. a
Pregger, T. and Friedrich, R.: Effective pollutant emission heights for atmospheric transport modelling based on real-world information, Environ. Pollut., 157, 552–560, https://doi.org/10.1016/j.envpol.2008.09.027, 2009. a, b, c, d
PRTR: Pollutant Release and Transfer Register, PRTR-Gesamtdatenbestand (Stand: 20.09.2017), available at: http://www.thru.de/thrude/downloads/ (last access: 21 October 2018), 2017. a
Querol, X., Alastuey, A., Reche, C., Orio, A., Pallares, M., Reina, F., Dieguez, J. J., Mantilla, E., Escudero, M., Alonso, L., Gangoiti, G., and Millan, M.: On the origin of the highest ozone episodes in Spain, Sci. Total Environ., 572, 379–389, https://doi.org/10.1016/j.scitotenv.2016.07.193, 2016. a
Rockel, B., Will, A., and Hense, A.: The Regional Climate Model COSMO-CLM (CCLM), Meteorol. Z., 17, 347–348, 2008. a
Rodrigues, M. C., Guarieiro, L. L. N., Cardoso, M. P., Carvalho, L. S., da Rocha, G. O., de Andrade, J. B.: Acetaldehyde and formaldehyde concentrations from sites impacted by heavy-duty diesel vehicles and their correlation with the fuel composition: Diesel and diesel/biodiesel blends, Fuel, 92, 258–263, https://doi.org/10.1016/j.fuel.2011.07.023, 2012. a
Salma, I., Németh, Z., Kerminen, V.-M., Aalto, P., Nieminen, T., Weidinger, T., Molnár, Á., Imre, K., and Kulmala, M.: Regional effect on urban atmospheric nucleation, Atmos. Chem. Phys., 16, 8715–8728, https://doi.org/10.5194/acp-16-8715-2016, 2016. a
Sanchez, B., Santiago, J.-L., Martilli, A., Palacios, M., and Kirchner, F.: CFD modeling of reactive pollutant dispersion in simplified urban configurations with different chemical mechanisms, Atmos. Chem. Phys., 16, 12143–12157, https://doi.org/10.5194/acp-16-12143-2016, 2016. a
Schneider, C., Pelzer, M. Toenges-Schuller, N., Nacken, M., and Niederau, A.: Gridding Emission Tool for ArcGIS (GRETA), ArcGIS basierte Lösung zur detaillierten, deutschlandweiten Verteilung (Gridding) nationaler Emissionsjahreswerte auf Basis des Inventars zur Emissionsberichterstattung. Umweltbundesamt, Texte 71/2016, ISSN 1862-4804, 223 pp., Dessau-Rosslau, Germany, 2016 (in German). a, b, c, d, e, f, g
Sillman, S.: The relation between ozone, NOx and hydrocarbons in urban and polluted rural environments, Atmos. Environ., 33, 1821–1845, 1999. a, b, c, d, e
Simpson, D.: Long-period modelling of photochemical oxidants in Europe. Model calculations for July 1985, Atmos. Environ., 26A, 1609–1634, 1992. a
Simpson, D.: Photochemical model calculations over Europe for two extended summer periods: 1985 and 1989. Model results and comparisons with observations, Atmos. Environ., 6, 921–943, https://doi.org/10.1016/0960-1686(93)90009-N, 1993. a
Simpson, D.: Hydrocarbon reactivity and ozone formation in Europe, J. Atmos. Chem., 20, 163–177, 1995. a
Simpson, D., Fagerli, H., Jonson, J. E., Tsyro, S., and Wind, P.: Transboundary Acidification, Eutrophication and Ground Level Ozone in Europe. Part 1: Unified EMEP Model Description, EMEP Status Report 1/2003, ISSN 0806-4520, Norwegian Meteorological Institute, Oslo, Norway, 2003. a, b, c, d, e
Simpson, D., Benedictow, A., Berge, H., Bergström, R., Emberson, L. D., Fagerli, H., Flechard, C. R., Hayman, G. D., Gauss, M., Jonson, J. E., Jenkin, M. E., Nyíri, A., Richter, C., Semeena, V. S., Tsyro, S., Tuovinen, J.-P., Valdebenito, Á., and Wind, P.: The EMEP MSC-W chemical transport model – technical description, Atmos. Chem. Phys., 12, 7825–7865, https://doi.org/10.5194/acp-12-7825-2012, 2012. a, b, c, d, e, f, g
Slørdal, L. H., Solberg, S., and Walker, S. E.: The Urban Air Dispersion Model EPISODE applied in AirQUIS2003, Technical description, Norwegian Institute for Air Research, NILU TR 12/2003, Kjeller, Norway, 2003. a
Slørdal, L. H., McInnes, H., and Krognes, T.: The Air Quality Information System AirQUIS, Info. Techn. Environ. Eng., 1, 21–33, 2008. a, b
Smith, G. D.: Numerical solution of partial differential equations: finite difference methods, Clarendon Press, Oxford, UK, 1985. a
Sofiev, M., Kouznetsov, R., Prank, M., Soares, J., Vira, J., Tarvainen, V., and Sofieva, V.: A long-term re-analysis of atmospheric composition and air quality, edited by: Mensink, C. and Kallos, G., in: Air Pollution Modeling and its Application XXV, Springer Proceedings in Complexity, Springer International Publishing, Cham, Switzerland, 2018. a
Stull, R. B.: An Introduction to Boundary Layer Meteorology, Kluwer Acad. Publ., Dordrecht, the Netherlands, Boston, USA, London, UK, 666 pp., 1988. a
Thom, A. S., Stewart, J. B., Liver, H. R., and Gash, J. H. C.: Comparison of aerodynamic and energy budget estimates of fluxes over a pine forest, Q. J. Roy. Meteor. Soc., 101, 93–105, 1975. a
Thunis, P., Pederzoli, A., and Pernigotti, D.: Performance criteria to evaluate air quality modeling applications, Atmos. Environ., 79, 476–482, 2012a. a, b, c, d, e, f
Thunis, P., Georgieva, E., and Pederzoli, A.: A tool to evaluate air quality model performances in regulatory applications, Environ. Model. Softw., 38, 220–230, https://doi.org/10.1016/j.envsoft.2012.06.005, 2012b. a, b
Thunis, P., Pernigotti, D., andGerboles, M.: Model quality objectives based on measurement uncertainty: Part I: Ozone, Atmos. Environ., 79, 861–868, 2013. a, b
Tunick, A.: A radiation and energy budget algorithm for forest canopies, Meteorol. Atmos. Phys., 91, 237–246, https://doi.org/10.1007/s00703-005-0145-8, 2006. a
UBA: Handbook of Emission Factors for Road Transport, HBEFA version 3.1, Umweltbundesamt Berlin, available at: http://www.hbefa.net/d/ (last access: 22 October 2018), 2010 (in German). a, b
Valach, A. C., Langford, B., Nemitz, E., MacKenzie, A. R., and Hewitt, C. N.: Seasonal and diurnal trends in concentrations and fluxes of volatile organic compounds in central London, Atmos. Chem. Phys., 15, 7777–7796, https://doi.org/10.5194/acp-15-7777-2015, 2015. a
Valverde, V., Pay, M. T., and Baldasano, J. M.: Ozone attributed to Madrid and Barcelona on-road transport emissions: Characterization of plume dynamics over the Iberian Peninsula, Sci. Tot. Environ., 543, 670–682, https://doi.org/10.1016/j.scitotenv.2015.11.070, 2016. a
van Ulden, A. P. and Holtslag, A. A. M.: Estimation of Atmospheric Boundary Layer Parameters for Diffusion Applications, J. Appl. Meteor., 24, 1196–1207, 1985. a
Verwer, J. and Simpson, D.: Explicit methods for stiff ODEs from atmospheric chemistry, Appl. Numer. Math., 18, 413–430, 1995. a
Verwer, J. G., Blom, J. G., and Hundsdorfer, W.: An implicit explicit approach for atmospheric transport-chemistry problems, Appl. Numer. Math., 20, 191–209, 1996. a
Wagner, P. and Kuttler, W.: Biogenic and anthropogenic isoprene in the near-surface urban atmosphere – A case study in Essen, Germany, Sci. Total Environ., 475, 104–115, https://doi.org/10.1016/j.scitotenv.2013.12.026, 2014. a
Walker, S. E.: Probabilistic modelling of air pollution from road traffic, Master Thesis, University of Oslo, available at https://www.duo.uio.no/handle/10852/10784, (last access: 21 October 2018), 2010. a, b
Walker, S. E.: WORM – A new open road line source model for low wind speed conditions, Int. J. Environ. Pollut., 47, 348–357, https://doi.org/10.1504/IJEP.2011.047348, 2011. a, b
Walker, S. E. and Grønskei, K. E.: Spredningsberegninger for on-line overvåkning i Grenland. Programbeskrivelse og brukerveiledning (In Norwegian), Norwegian Institute for Air Research, NILU OR 55/92, Kjeller, Norway, 1992. a, b
Walker, S.-E., Solberg, S., and Denby, B.: Development and implementation of a simplified EMEP photochemistry scheme for urban areas in EPISODE, Norwegian Institute for Air Research, NILU TR 13/2003, Kjeller, Norway, 2003. a, b, c, d, e, f
Wang, Z. B., Hu, M., Mogensen, D., Yue, D. L., Zheng, J., Zhang, R. Y., Liu, Y., Yuan, B., Li, X., Shao, M., Zhou, L., Wu, Z. J., Wiedensohler, A., and Boy, M.: The simulations of sulfuric acid concentration and new particle formation in an urban atmosphere in China, Atmos. Chem. Phys., 13, 11157–11167, https://doi.org/10.5194/acp-13-11157-2013, 2013. a
Zhang, R., Khalizov, A., Wang, L., Hu, M., and Xu, W.: Nucleation and growth of nanoparticles in the atmosphere, Chem. Rev., 112, 1957–2011, https://doi.org/10.1021/cr2001756, 2012. a
- Abstract
- Introduction
- Development and description of EPISODE–CityChem model extensions
- Test of different model extensions
- Application of EPISODE–CityChem to air quality modelling for Hamburg
- Planned improvements to the EPISODE model
- Conclusions
- Code and data availability
- Appendix A: List of acronyms and abbreviations
- Appendix B: Photodissociation rates
- Appendix C: Treatment of deposition on the Eulerian grid
- Appendix D: Treatment of boundary concentration data
- Appendix E: Statistical indicators and model performance indicators
- Author contributions
- Competing interests
- Acknowledgements
- Financial support
- Review statement
- References
- Supplement
- Abstract
- Introduction
- Development and description of EPISODE–CityChem model extensions
- Test of different model extensions
- Application of EPISODE–CityChem to air quality modelling for Hamburg
- Planned improvements to the EPISODE model
- Conclusions
- Code and data availability
- Appendix A: List of acronyms and abbreviations
- Appendix B: Photodissociation rates
- Appendix C: Treatment of deposition on the Eulerian grid
- Appendix D: Treatment of boundary concentration data
- Appendix E: Statistical indicators and model performance indicators
- Author contributions
- Competing interests
- Acknowledgements
- Financial support
- Review statement
- References
- Supplement