the Creative Commons Attribution 4.0 License.
the Creative Commons Attribution 4.0 License.
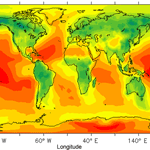
ORACLE 2-D (v2.0): an efficient module to compute the volatility and oxygen content of organic aerosol with a global chemistry–climate model
Alexandra P. Tsimpidi
Vlassis A. Karydis
Andrea Pozzer
Spyros N. Pandis
Jos Lelieveld
A new module, ORACLE 2-D, simulating organic aerosol formation and evolution in the atmosphere has been developed and evaluated. The module calculates the concentrations of surrogate organic species in two-dimensional space defined by volatility and oxygen-to-carbon ratio. It is implemented into the EMAC global chemistry–climate model, and a comprehensive evaluation of its performance is conducted using an aerosol mass spectrometer (AMS) factor analysis dataset derived from almost all major field campaigns that took place globally during the period 2001–2010. ORACLE 2-D uses a simple photochemical aging scheme that efficiently simulates the net effects of fragmentation and functionalization of the organic compounds. The module predicts not only the mass concentration of organic aerosol (OA) components, but also their oxidation state (in terms of O : C), which allows for their classification into primary OA (POA, chemically unprocessed), fresh secondary OA (SOA, low oxygen content), and aged SOA (highly oxygenated). The explicit simulation of chemical OA conversion from freshly emitted compounds to a highly oxygenated state during photochemical aging enables the tracking of hygroscopicity changes in OA that result from these reactions. ORACLE 2-D can thus compute the ability of OA particles to act as cloud condensation nuclei and serves as a tool to quantify the climatic impact of OA.
Atmospheric aerosols adversely affect human health and play a significant role in climate change on regional and global scales. Depending on their composition, aerosols affect the energy budget of the Earth's atmosphere by scattering and absorbing solar radiation (direct effect) and by influencing the reflective properties of clouds, their lifetime, and precipitation formation (indirect effects). In addition, climate change can play a vital and complex role in the formation and removal of atmospheric particles (Trail et al., 2013, 2014). Organic aerosol (OA) is an important constituent of atmospheric particles contributing 20 %–90 % to the total submicron particulate mass, depending on the region (Zhang et al., 2007).
Primary OA (POA) has been traditionally treated as nonvolatile and inert in global-scale chemistry–climate models (CCMs). Robinson et al. (2007) demonstrated that OA emissions are semi-volatile and most of the emitted OA moves to the gas phase after emission due to dilution and evaporation. On the other hand, all organic vapors are subject to photochemical reactions with OH in the gas phase, forming organic products with lower volatility that can recondense to the particulate phase as secondary organic aerosol (SOA). To describe the OA gas–aerosol partitioning, Donahue et al. (2006) developed the volatility basis set (VBS) framework, in which OA is assumed to be semi-volatile and photochemically reactive and is distributed in logarithmically spaced volatility bins. With this innovative approach, semi-volatile primary emissions, chemical aging, and SOA formation were unified within a common framework that is ideally suited for regional and global chemical modeling. Since 2006, many regional (Lane et al., 2008; Murphy and Pandis, 2009; Tsimpidi et al., 2010, 2011; Ahmadov et al., 2012; Athanasopoulou et al., 2013; Koo et al., 2014; Fountoukis et al., 2014; Ciarelli et al., 2017; Gao et al., 2017) and global (Pye and Seinfeld, 2010; Jathar et al., 2011; Jo et al., 2013; Tsimpidi et al., 2014; Hodzic et al., 2016) modeling studies have used the VBS to account for the semi-volatile nature and chemical aging of organic compounds, demonstrating improvements in reproducing the OA budget and its chemical resolution.
The chemical aging of OA results in significant changes to its physical and chemical properties due to the addition of oxygen atoms from reaction with OH. This increase in OA oxygen content is important for its impact on climate through changes in cloud condensation nuclei (CCN) and ice nuclei (IN) activity. In fact, oxygen content, expressed by the ratio of oxygen to carbon atoms (O : C), influences OA hygroscopic growth (Chang et al., 2010; Lambe et al., 2011) which affects CCN activity. In addition, the phase-state changes of SOA during its atmospheric lifetime, which can impact the IN activity, is also influenced by the O : C of OA (Shiraiwa et al., 2017). Donahue et al. (2011) extended the original one-dimensional VBS framework to two dimensions (2-D VBS), tracking not only the saturation concentration but also the oxygen content of OA during atmospheric transport. This approach further improved the description of the atmospheric evolution of OA and its precursor gases that become increasingly more oxidized, less volatile, and more hygroscopic during their atmospheric aging. However, the large number of additional surrogate organic compounds required by the 2-D VBS framework has hindered implementation in three-dimensional atmospheric models (Napier et al., 2014). Therefore, the 2-D VBS approach has been mostly adopted in box and 1-D Lagrangian models (Murphy et al., 2011, 2012; Chacon-Madrid et al., 2013; Zhao et al., 2015; Paciga et al., 2016). Koo et al. (2014) introduced a hybrid VBS approach for use in three-dimensional chemical transport models (CTMs) that combines the simplicity of the VBS with the ability to track the evolution of OA in the 2-D space of volatility and oxygen content.
In this work, a computationally efficient module for the description of OA composition and evolution in the atmosphere (ORACLE; Tsimpidi et al., 2014) has been extended to allow for the first time in a global CCM the description of both the volatility and oxygen content of OA based on the 2-D VBS approach. Similar to ORACLE v1.0, the interface of the new version allows the user to have full control of the complexity of the OA scheme by adjusting the number of species and reactions (i.e., number of compounds, volatility bins, O : C bins) to optimize the computational cost according to the application and the desired chemical resolution. The updated ORACLE module can provide valuable information about the physicochemical evolution of OA during its atmospheric lifetime in support of modeling studies and help quantify the climatic impact of OA.
2.1 EMAC model
The ECHAM/MESSy Atmospheric Chemistry (EMAC) model is a numerical chemistry and climate simulation system that includes submodels describing lower and middle atmospheric processes and their interaction with oceans, land, and human influences. EMAC consists of the Modular Earth Submodel System and an advanced version of the 5th generation of the European Centre Hamburg (ECHAM) general circulation model. ECHAM5 (Roeckner et al., 2006) serves as the atmospheric dynamic core that simulates atmospheric flow and is integrated in the base model layer of MESSy. The interface structure of MESSy links the base model with several atmospheric submodels that online simulate gas-phase chemistry (MECCA; Sander et al., 2011), inorganic aerosol microphysics and dynamics (GMXe; Pringle et al., 2010), organic aerosol formation and growth (ORACLE; Tsimpidi et al., 2014), emissions (ONLEM and OFFLEM; Kerkweg et al., 2006b), dry deposition and sedimentation (DRYDEP and SEDI; Kerkweg et al., 2006a), cloud scavenging (SCAV; Tost et al., 2006), cloud microphysics (CLOUD; Jöckel et al., 2006; Bacer et al., 2018), and aerosol optical properties (AEROPT; Lauer et al., 2007). EMAC has been extensively described and evaluated against ground-based and satellite observations (Pozzer et al., 2012; Tsimpidi et al., 2014, 2016, 2017; Karydis et al., 2016, 2017). In this study, the applied spectral resolution of the EMAC model is T63L31, corresponding to a horizontal grid resolution of and 31 vertical layers extending to 18 km of altitude (10 hPa). EMAC is applied for 11 years covering the period 2000–2010 and the first year is used as spin-up.
2.2 ORACLE module
2.2.1 Module description
ORACLE is a computationally efficient module for the description of organic aerosol composition and evolution in the atmosphere (Tsimpidi et al., 2014) that has been incorporated into the EMAC model. The original version of ORACLE (V1.0) simulated the volatility distribution of a wide variety of semi-volatile organic surrogate compounds using bins of logarithmically spaced effective saturation concentrations. Organic emissions from multiple anthropogenic and natural sources are taken into account using distinct surrogate species for each source category. These surrogates can be subdivided into groups of organic compounds based on their volatility: low-volatility organic compounds (LVOCs, µg m−3), semi-volatile organic compounds (SVOCs, 10 µg m−3), intermediate-volatility organic compounds (IVOCs, µg m−3), and volatile organic compounds (VOCs, µg m−3). These organic compounds are allowed to partition between the gas and aerosol phases, resulting in the formation of OA. The change in aerosol mass of each size mode after the phase partitioning is determined by using a weighting factor as described in Tsimpidi et al. (2014). Gas-phase photochemical reactions that modify the volatility of the organics are taken into account and the oxidation products of each group of precursors are simulated separately in the module to keep track of their origin. The model results for the different organic components in the particulate phase were compared with factor analysis results derived from a comprehensive dataset of aerosol mass spectrometer (AMS) measurements from multiple field campaigns across the Northern Hemisphere. The resulting good agreement between campaign average concentrations and model predictions supports the capability of the model to capture the spatial and temporal characteristics of OA levels. Tsimpidi et al. (2017) conducted an extensive sensitivity analysis of the EMAC OA predictions to uncertain parameters in the ORACLE module. One of the major conclusions of their analysis was that the model performance can be improved by assuming that freshly emitted organic compounds are relatively hydrophobic and become increasingly hygroscopic due to oxidation. As a first step to achieve this goal, the ORACLE module has been further developed here to account for the oxidation state of the organic surrogate compounds. The new version is called ORACLE 2-D.
2.2.2 Emission inventory of OA precursors
Emissions of biogenic VOCs (i.e., isoprene and monoterpenes) are calculated online by EMAC with the ONLEM submodel. The open biomass burning emissions of organic compounds from savanna and forest fires are based on the Global Fire Emissions Database (GFED version 3.1; van der Werf et al., 2010) and are distributed into LVOCs (20 %), SVOCs (40 %), and IVOCs (40 %) based on the emission factors proposed by May et al. (2013). Emissions of anthropogenic LVOCs, SVOCs, IVOCs, and VOCs (i.e., aromatics, alkanes, olefins) from fossil and biofuel combustion are derived from the CMIP5 emission inventory for the RCP4.5 scenario (Thomson et al., 2011). The volatility distribution of anthropogenic emissions to LVOCs (8 %), SVOCs (72 %), and IVOCs (170 %) is based on the findings of Robinson et al. (2007) and includes increased factors (the sum of the emission factors is 250 %) to account for missing IVOC emissions from the traditional inventories. More details about the organic compound emissions used here can be found in Tsimpidi et al. (2016).
3.1 Module overview
The original ORACLE v1.0 (called hereafter ORACLE) uses saturation concentration bins to describe the volatility distribution of the major OA components. In this work, ORACLE is extended to also resolve the oxygen content of OA expressed by the O : C ratio (ORACLE v2.0; called hereafter ORACLE 2-D). The volatility dimension is discretized in up to 10 logarithmically spaced volatility bins separating the organic compounds into low-volatility (LVOCs, expressed by the volatility bins of 10−3, 10−2, and 10−1 µg m−3), semi-volatile (SVOCs, C* equal to 100, 101, and 102 µg m−3), and those of intermediate volatility (IVOCs, C* equal to 103, 104, 105, and 106 µg m−3). Extremely low-volatility organic compounds (ELVOCs, ) can be formed by the ozonolysis of monoterpenes and sesquiterpenes (Liggio et al., 2010; Sun et al., 2009) playing an important role for the formation and growth of new particles created in situ in the atmosphere by nucleation (Ehn et al., 2014). The production of ELVOCs from biogenic VOCs is not currently included in ORACLE since the simulation of new particle formation is outside the scope of the current article and part of work in progress. In addition, the oxygen content dimension is discretized in up to 20 linearly spaced O : C bins subdividing the organic compounds into fresh emissions (expressed by the O : C bins of 0.1 and 0.2), less oxygenated organic compounds (O : C equal to 0.3, 0.4, 0.5, and 0.6), moderately oxygenated organic compounds (O : C equal to 0.7, 0.8, 0.9, and 1.0), and highly oxygenated species with O : C > 1. The first bin also includes hydrocarbons with zero oxygen. The O : C range can be extended up to 2 (for CO2). However, there are only a few atmospheric organic compounds with O : C higher than unity and the observed O : C of ambient OA rarely exceeds 1.1 (Ng et al., 2010; Kroll et al., 2011). The ability of ORACLE 2-D to simulate the degree of oxidation of OA allows for the simulation of its hygroscopicity by using proposed parameterizations that link the hygroscopicity parameter kappa with the O : C of OA (Chang et al., 2010; Lambe et al., 2011; Kuwata and Lee, 2017). In the current application, the hygroscopicity of each OA compound is represented by a linear function of the form κorg=0.18 (O : C) + 0.03 (Lambe et al., 2011).
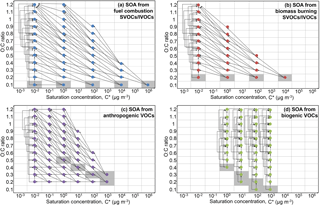
Figure 1The 2-D grid space in the ORACLE 2-D module with saturation concentration (in µg m−3) on the x axis and the O : C ratio on the y axis. For each cell an organic surrogate compound is defined with a specific carbon number calculated as a function of effective saturation concentration and O : C ratio. The formation and evolution of SOA from (a) fuel combustion SVOCs and IVOCs, (b) biomass burning SVOCs and IVOCs, (c) anthropogenic VOCs and, (d) biogenic VOCs are shown. The arrows correspond to aging reactions and the grey grids to the initial chemical state of the species prior to aging.
ORACLE 2-D has a flexible interface in which the user can choose the resolution (number of bins used in each dimension) of the 2-D VBS space through a namelist file depending on the desired application and scientific goals. The namelist file includes the variables that control the desired chemical resolution of OA (number of volatility and O : C bins, number of size modes, saturation concentrations, molecular weights, etc.) and the desired emission factors for the distribution of POA emissions into LVOC, SVOC, and IVOC volatility bins (and the corresponding O : C bins). Changes to the gas-phase chemistry (e.g., photochemical reaction rate constants) need to be made in the MECCA submodel by modifying the ORACLE replacement file, which will automatically update the gas-phase chemistry used by EMAC. Then, the interface layer of ORACLE 2-D reads the namelist variables and automatically (i) defines the new tracers for organic compounds, (ii) performs the coupling with the emission modules and with the gas-phase chemistry module (MECCA), and (iii) calls the core layer of the ORACLE 2-D module. The core layer calculates the bulk equilibrium gas and aerosol concentrations and distributes the change in the bulk aerosol concentration into size modes.
In this work, we employ ORACLE 2-D based on the sensitivity analysis results of Tsimpidi et al. (2017) and using the 2-D VBS at a resolution suitable for medium-term simulations with global chemistry–climate models. This chemical resolution includes 150 organic aerosol surrogate compounds compared to 34 OA compounds in ORACLE, which results in a 16 % increase in the overall EMAC computational burden. The performance reduction comes mainly from the gas-phase chemistry and secondarily from the atmospheric transport of the additional tracers, since ORACLE 2-D uses an efficient method to minimize the computational cost of the phase partitioning calculations as described in Sect. 3.4.
3.2 Constructing the two-dimensional grid
For the current application, ORACLE 2-D distributes the OA surrogate species into logarithmically spaced volatility bins with C* varying from 10−2 to 106 µg m−3 and linearly spaced oxygen content bins with O : C varying from 0.1 to 1.2 (Fig. 1). Each of the OA surrogate species in this C*–O : C 2-D space is characterized by a representative number of carbon atoms per molecule (nC) and a molecular weight (MW). Donahue et al. (2011) used structure activity relationships (Pankow and Asher, 2008) to express C* as a function of nC and the number of oxygens per molecule (nO):
Given that nO is a function of O : C and nC:
nC can be expressed as a function of C* and O : C:
Assuming that the organic compounds consist entirely of carbon, oxygen, and hydrogen atoms (i.e., ignoring nitrogen and sulfur), the MW of each surrogate species is a function of nC, nO, and the number of hydrogen atoms per molecule (nH) and can therefore be calculated as
where
H : C is the atomic ratio of hydrogen to carbon approximated by Heald et al. (2010) as
Combining Eqs. (4), (2), and (5), we get
Given that nC decreases as C* increases (Eq. 3), the MW calculated by Eq. (7) is consistent with the molecular corridor approach (Shiraiwa et al., 2014), which suggests a tight inverse correlation between volatility and molar mass constrained by boundary lines of low and high O : C ratios.
Organic compound emissions from anthropogenic fuel combustion and open biomass burning include LVOCs (with C* at 298 K equal to 10−2 µg m−3), SVOCs (with C* at 298 K equal to 100 and 102 µg m−3), and IVOCs (with C* at 298 K equal to 104 and 106 µg m−3). Their corresponding emissions are estimated using the emission factors of Tsimpidi et al. (2016). Freshly emitted LVOCs, SVOCs, and IVOCs from anthropogenic and open biomass burning sources are assigned an initial O : C of 0.1 (Fig. 1a) and 0.2 (Fig. 1b), respectively (Donahue et al., 2011). We distinguish anthropogenic and biogenic VOCs, and their first-generation oxidation products are distributed in four volatility bins (with C* at 298 K equal to 100, 101, 102, and 103 µg m−3) by using the aerosol mass yields by Tsimpidi et al. (2014). The O : C distributions of the first-generation VOC oxidation products are given by Murphy et al. (2011) and vary with volatility (Fig. 1c and d).
3.3 New photochemical aging scheme
Similar to ORACLE, multiple generations of homogeneous gas-phase reactions with OH are simulated for all OA compounds treated by ORACLE 2-D. After each oxidation step, oxygen atoms are added to the reacting organic gas, resulting in an increase in oxygen content and a change in volatility due to functionalization (reducing the volatility) or fragmentation (increasing the volatility). To minimize the computational cost and at the same time simulate the net effect of both fragmentation and functionalization, ORACLE 2-D uses an approach similar to the aging scheme proposed by Murphy et al. (2011). ORACLE 2-D assumes a net average decrease in volatility of aSOA-v (SOA from the oxidation of anthropogenic VOCs) reacting with OH by a factor of 10 and the addition of one or two oxygen atoms with the same probability of 50 % (Fig. 1c). Assuming volatility transformations only into one direction (functionalization) and neglecting fragmentation can lead to increasingly higher OA concentrations in lower-volatility bins. This may result in an overestimation of OA at long aging timescales up to thousands of kilometers downwind of the source regions. However, this overestimation can be partially offset by the conservative aging scheme used in ORACLE 2-D compared to the relatively aggressive functionalization scheme proposed by Murphy et al. (2012). The same addition of oxygen atoms (one or two) is assumed for bSOA-v (SOA from the oxidation of biogenic VOCs); however, its volatility remains unchanged due to a balancing of fragmentation and functionalization effects (Murphy et al., 2012) (Fig. 1d). The OH reaction rate constant for both aSOA-v and bSOA-v is cm3 molecule−1 s−1 (Donahue et al., 2006; Tsimpidi et al., 2010). The reaction of SOA-sv (SOA from the oxidation of SVOCs) and SOA-iv (SOA from the oxidation of IVOCs) with OH with a rate constant of cm3 molecule−1 s−1 (Tsimpidi et al., 2014) results in the addition of two or three oxygen atoms (with equal probability) and the reduction of their volatilities by a factor of 100 (Fig. 1a and b). The number of added oxygen atoms (nO+) due to reaction with OH is then expressed as an increase in O : C in the 2-D space. Each of the OA surrogate compounds in the 2-D space described in Sect. 3.3 has a representative carbon number per molecule (nC). Assuming that carbon is conserved during the reaction with OH, the O : C of the product is calculated as follows:
If the (O : C)product has more than one decimal place, then it is distributed between the two adjacent O : C bins of the 2-D space by using linear interpolation. Finally, since carbon is conserved, the increase in organic mass (OM) due to the added oxygen after each oxidation reaction is calculated as
where, following Murphy et al. (2011),
3.4 Phase partitioning calculations
ORACLE 2-D uses the core layer of the ORACLE module to calculate the partitioning of organic compounds between the gas and particle phases by assuming bulk equilibrium (Tsimpidi et al., 2014). However, the computational time required for phase partitioning increases super-linearly with the number of species. As the condensation and/or evaporation of organic compounds depends only on their saturation concentration and not their O : C ratio, only one equilibrium calculation is performed per volatility bin of each category (i.e., fuel combustion, biomass burning, anthropogenic VOC products, and biogenic VOC products). This approach significantly reduces the number of equations to be solved and the corresponding computational cost of the phase partitioning calculations.
The ORACLE core layer calculates the aerosol composition at equilibrium by solving the following set of n nonlinear equations.
Ct,i and Ca,i are the total and aerosol-phase concentrations of product i in µg m−3, respectively, is the effective saturation concentration of species i, xi is the mole fraction of product i in the absorbing organic phase, and Mi is the molecular weight of product i, which corresponds to the weighted-average molecular weight of the species with the same saturation concentration.
The temperature dependence of the saturation concentrations is described by the Clausius–Clapeyron equation:
where and are the saturation concentrations at temperature T and T0, respectively, R is the gas constant, and ΔH is the enthalpy of vaporization. Here, an effective ΔH of 30 kJ mol−1 is used for all aSOA-v and bSOA-v species based on data for α-pinene (Pathak et al., 2007). A ΔH of 112, 100, 88, 76, and 64 kJ mol−1 is used for the 10−2, 100, 102, 104, and 106 µg m−3 volatility bins, respectively, of all organic compounds from fuel combustion and biomass burning sources based on data for large saturated species commonly found in primary emissions (Donahue et al., 2006).
Water uptake by the particulate organic phase under high relative humidity conditions can increase the aerosol mass and decrease the mole fraction of individual organic products, which may allow for the additional condensation of SOA (Jathar et al., 2016). However, Eq. (12) calculates the mole fraction of organic products only in the absorbing organic phase without taking into account the presence of water in the aerosol phase. This simplification can result in an underestimation of SOA in areas with high humidity and significant SOA concentrations.
Assuming that the distribution of species in the same volatility bin in the different O : C bins does not change due to condensation or evaporation, the gas and aerosol concentrations of each compound in the 2-D space after the phase partitioning calculations are given by
and
where SOG and SOA are the gas and aerosol concentrations of each compound, respectively, i and j are the volatility and O : C bin index in the 2-D space, respectively, m is the total number of O : C bins, and t and t+Δt are the times before and after the phase partitioning calculations, respectively. and for each volatility bin i in Eqs. (14) and (15) correspond to () and Ca,i at equilibrium calculated from Eqs. (11) and (12).
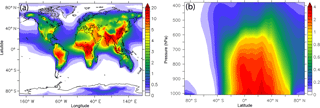
Figure 2Predicted average (a) surface and (b) zonal concentrations of total OA (in µg m−3) during the years 2001–2010.
4.1 Total OA and O : C
Simulated total OA concentrations are high over regions affected by anthropogenic fuel combustion and open biomass burning (Fig. 2a). The highest annual average OA concentrations at the surface are predicted over the densely populated areas of eastern China, northern India, and Bangladesh (10–28 µg m−3), as well as over the tropical forest in the Congo Basin (12–22 µg m−3). Considerably high OA concentrations are also predicted over the tropical forests of Southeast Asia and the Amazon (6–17 µg m−3). Strong fossil fuel combustion sources over the Arabian Peninsula result in annual average OA concentrations of 6–10 µg m−3. The calculated zonal average concentrations of total OA at the surface peak over the midlatitudes of the Northern Hemisphere and the tropics (∼4 µg m−3), remaining relatively high (>2 µg m−3) up to 750 hPa of altitude (Fig. 2b). Over Europe, predicted OA annual average concentrations at the ground level are in the 3–7 µg m−3 range. Over North America, the highest OA concentration is simulated over southern California, the Mexico City metropolitan area, and the southeastern USA (2–8 µg m−3). OA concentrations in the 0.5–1 µg m−3 range are predicted over parts of the oceans due to the long-range transport of OA from adjacent continental sources. Non-negligible concentrations of OA can be found over Greenland and Antarctica due to the long-range transport of organic compounds and their condensation in the particulate phase under very low temperatures. The calculated total tropospheric OA burden is 3.3 Tg, which is higher compared to the calculated tropospheric burden of ORACLE v1.0 (2 Tg; Tsimpidi et al., 2016) but is within the range of OA tropospheric burdens (0.7 to 3.8 Tg) from 31 global CTMs reported by Tsigaridis et al. (2014).
Figure 3a depicts the annual average simulated O : C ratio of total OA at the surface. Lower values of O : C are predicted close to OA sources, i.e., over industrialized areas in the Northern Hemisphere and over tropical and boreal forests. The lowest average values occur over boreal forests (as low as 0.3) due to the limited photochemical activity over these regions in contrast to tropical forests, where O : C is around 0.5. Over the densely populated areas of Asia, Europe, and North America, the O : C is about 0.3–0.4, i.e., close to the anthropogenic sources. O : C levels increase rapidly (in excess of 0.6) downwind of the sources due to photochemical aging of the transported OA. The highest O : C values are calculated over the Sahara Desert and the remote Atlantic and Pacific Ocean (0.8–1); however, over these regions, OA concentrations are low (Fig. 2). O : C ratios increase significantly with altitude according to the model (Fig. 3b) since organic vapors transported vertically react with OH, forming products with higher oxygen content. Over the midlatitudes of the Northern Hemisphere, the average O : C ratio near the surface is 0.55 and increases as the air masses are transported aloft by approximately 0.05 for every 100 hPa (Fig. 3b).
4.2 POA and SOA
Figure 4a depicts the decadal average simulated POA concentrations at the surface. POA concentrations are high over densely populated areas in the Northern Hemisphere due to strong fuel combustion emissions from the industrial, energy, residential, and transport sectors. The highest concentrations are calculated over eastern China (3–13 µg m−3), Bangladesh (2–8 µg m−3), and eastern Europe (1–3 µg m−3). Open biomass burning emissions from forest, woodland, peatland, and savanna fires result in high POA concentrations over the tropics (3–8 µg m−3 over the Congo Basin) and boreal forests (1–6 µg m−3 over Russia). However, a large fraction of POA evaporates due to dilution as the air masses travel downwind from the sources, resulting in significant reduction of the concentration (Fig. 4a). Then, the material that is transferred to the gas phase can be oxidized and recondense to the aerosol phase, forming SOA that persists even far from the sources (Fig. 4b). This results in a relatively homogeneous regional distribution of SOA with a continental background of 2 µg m−3 and high concentrations even far downwind from anthropogenic (e.g., 7–22 µg m−3 over south and eastern Asia) and open biomass burning (e.g., 9–17 µg m−3 over Central Africa) sources. Lower concentrations are predicted over boreal forests (∼1 µg m−3) due to minor photochemical activity.
The tropospheric burden of POA and SOA calculated by ORACLE 2-D is 0.25 and 3.05 Tg, respectively. While the value of POA is very similar to the corresponding tropospheric burden of ORACLE (0.24 Tg), the tropospheric burden of SOA has increased significantly (1.74 Tg in ORACLE). The tropospheric burden of POA calculated by ORACLE 2-D is much lower than most global CTMs in the AEROCOM intercomparison study (mean value of 0.85 Tg) (Tsigaridis et al., 2014). This difference is due to the evaporation of POA and its conversion into SOA in ORACLE 2-D, given that this dynamic behavior of POA is not taken into account by most global CTMs. Consequently, the ORACLE 2-D calculated SOA tropospheric burden is higher than most CTMs from the AEROCOM intercomparison study (mean value of 1 Tg) (Tsigaridis et al., 2014) due to its stronger chemical production. As indicated by Tsimpidi et al. (2016), the POA burden is underestimated by our model, especially in the urban environment in the winter. This underestimation was partially attributed to missing residential wood burning emissions in our inventory (van der Gon et al., 2015).
4.3 Fresh and aged SOA
The major advantage, at least in this initial phase, of extending the ORACLE module to describe the oxygen content of OA is the model's ability to quantify the degree of photochemical processing of OA. The model can distinguish fresh SOA that is relatively less oxygenated from highly aged and oxygenated SOA. As a first approximation, OA compounds with O : C ≤ 0.6 are considered “fresh SOA” and OA compounds with O : C > 0.6 are considered “aged SOA”.
Figure 5 depicts the average concentrations of fresh and aged SOA and their fractional contributions to total SOA at the surface. Fresh SOA exceeds aged SOA close to the source areas (Fig. 5c). On the other hand, the spatial distribution of aged SOA extends further from the sources with relatively high concentrations even over remote continental (e.g., Sahara) and oceanic (e.g., North Pacific) regions (Fig. 5b). Aged SOA maximizes over north India since southerly monsoon winds, prevailing during summer, favor the transport of aged organic compounds to the north where they can accumulate at the foothills of the Himalaya mountain range before being removed by convection and precipitation. The fraction of aged SOA is higher over industrialized regions (0.4–0.7), where IVOCs comprise 70 % of total fuel combustion emissions, and lower over the tropics (0.1–0.5), where IVOCs represent 40 % of total open biomass burning emissions (Fig. 5d). Overall, the tropospheric burden of fresh SOA is 1.26 Tg and of aged SOA 1.79 Tg.
The simulated vertical profiles of fresh and aged SOA are quite different (Fig. 6). Both fresh and aged SOA concentrations are high near the surface with zonal averages of 0.9–1.7 µg m−3 over the northern midlatitudes and the tropics. However, at higher altitudes the oxidation of fresh SOA continues, which leads to transformation into aged SOA. Therefore, above 960 hPa of altitude the zonal average concentrations of fresh SOA decrease gradually (below 1 µg m−3) and those of aged SOA increase, exceeding in some cases 1.5 µg m−3 at 850 hPa of altitude. Further aloft in the atmosphere (above 700 hPa of altitude) the levels of both fresh and aged SOA are reduced significantly due to dilution and removal.
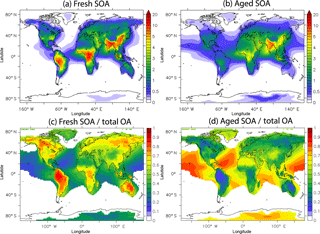
Figure 5Predicted average surface concentration (in µg m−3) of (a) fresh SOA and (b) aged SOA and surface fraction of (c) fresh SOA and (d) aged SOA to total OA during the years 2001–2010.
4.4 2-D space distribution
In this section, we present a new feature that comes along with the upgraded ORACLE 2-D. Since ORACLE 2-D explicitly describes the concentration of organic surrogate species in two-dimensional space, defined by their volatility and O : C ratio, it can also provide as output its distribution in this 2-D space. As an example, Fig. 7 depicts the 2-D distribution of the average total OA concentrations during the years 2001–2010 over central Europe and the Amazon basin. The first left column represents all the species with µg m−3, while the top line represents all the species with O : C ≥ 1.2.
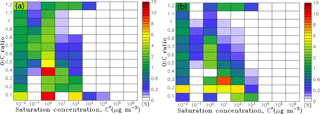
Figure 7Predicted average fraction of OA concentration in each cell of the ORACLE 2-D grid space to total OA concentration over (a) Europe and (b) the Amazon basin during the years 2001–2010.
Over Europe, approximately 50 % of OA has µg m−3. About 10 % of the OA is emitted directly in this volatility bin as POA with O :C = 0.1, while the rest, 40 %, is SOA with higher O : C from the aging of more volatile compounds. The volatility bin with µg m−3 is also important, containing 18 % of the total OA. On the other hand, volatility bins with contain less than 1 % of total OA since organic species in these high-volatility bins exist mainly in the gas phase. Furthermore, about 30 % of the total OA over Europe has O : C ≤ 0.2, mainly from direct emissions of POA from fuel combustion sources with O : C = 0.1 (20 % of total OA). Organic species with O :C = 0.4 are also important, representing 15 % of the total OA. Overall, 40 % of OA over Europe has 0.3 ≤ O : C ≤ 0.6 and 30 % is highly oxidized with O : C ≥ 0.7.
Over the Amazon, similar to Europe, approximately 50 % of total OA has µg m−3, while bins with contain only 2 % of total OA. However, volatility bins with are more important compared to Europe containing about 30 % of the total OA concentrations. This discrepancy can be attributed to the high bSOA-v concentrations over the Amazon rainforest, which remain within higher-volatility bins during their photochemical aging compared to aSOA-v. Furthermore, similar to Europe, about 30 % of total OA over the Amazon has O : C ≤ 0.2, mainly affected by direct aerosol emissions from biomass burning with O : C = 0.2 (∼20 % of total OA). OA species with 0.3 ≤ O : C ≤ 0.6 represent 55 % of the total OA with mostly O : C = 0.4 (35 % of total OA). On the other hand, only 15 % of the total OA is highly oxidized with O : C ≥ 0.7. Comparing these results to Europe shows that OA over biomass burning areas (e.g., the Amazon) are estimated to be less oxidized than OA over anthropogenic areas (e.g., Europe), despite the higher photochemical activity over the tropics. This perhaps unexpected result can be explained by the higher fraction of IVOC emissions over industrialized regions that can increase the overall oxidation state of OA through their multi-generational aging.
The mean absolute gross error (MAGE), mean bias (MB), normalized mean error (NME), normalized mean bias (NMB), and root mean square error (RMSE) are used to assess the model performance of the simulations of OA components (i.e., POA, fresh and aged SOA) and O : C (Tsimpidi et al., 2016).
5.1 Organic aerosol concentrations
The simulated POA and SOA can be compared against AMS factor analysis results from 61 field campaigns performed during the period 2001–2010 over urban downwind and rural environments in the Northern Hemisphere (Fig. 8). Information for each of these campaigns is given in Tsimpidi et al. (2016). Factor analysis techniques classify OA into hydrocarbon-like OA (HOA), biomass burning OA (BBOA), and oxygenated OA (OOA). HOA is assumed to correspond to POA from fossil fuel combustion, and BBOA corresponds to POA from biomass burning (Crippa et al., 2014). Therefore, simulated POA is compared here against the sum of AMS HOA and BBOA (Table 1). OOA corresponds to modeled SOA (Table 2) and can be further classified into two subtypes, semi-volatile OOA (SVOOA) and low-volatility OOA (LVOOA) (Crippa et al., 2014). Recent studies have suggested that the main difference between these two OOA types is often not so much their volatility, but mostly their oxygen content (Kostenidou et al., 2015; Louvaris et al., 2017). These two OOA types represent distinct oxidation states with O : C of 0.33–0.67 for SVOOA and 0.67–1.00 for LVOOA (Donahue et al., 2012). Therefore, SVOOA can be compared at least as a zero-order approximation against the simulated fresh SOA (Table 3) and LVOOA against the simulated aged SOA (Table 4).
Table 1Statistical evaluation of EMAC POA against AMS POA (sum of HOA and BBOA) from 61 datasets worldwide during 2001–2010.
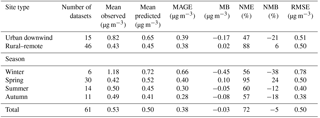
Table 2Statistical evaluation of EMAC SOA against AMS OOA from 61 datasets worldwide during 2001–2010.
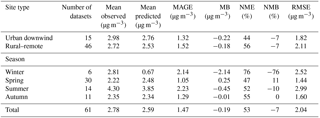
Table 3Statistical evaluation of EMAC aged SOA against AMS LVOOA from 41 datasets worldwide during 2001–2010.
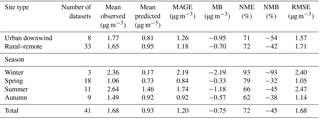
Table 4Statistical evaluation of EMAC fresh SOA against AMS SVOOA from 41 datasets worldwide during 2001–2010.
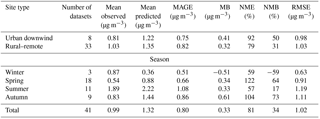
The model reproduces the observed campaign average POA concentrations within a factor of 2 in 40 % of the cases over urban downwind and rural locations (Table 1, Fig. 8a). The average simulated POA concentration over the urban downwind regions is around 0.65 µg m−3 and it decreases further after continued transport from the sources to 0.45 µg m−3 over rural areas. Compared to AMS HOA concentrations, modeled POA is unbiased over rural environments; however, it is underestimated downwind of urban areas (MB = −0.17 µg m−3). The model has the best performance during summer with MB = −0.05 µg m−3 and RMSE = 0.40 µg m−3. Compared to ORACLE (Tsimpidi et al., 2016), ORACLE 2-D produces almost identical concentrations of POA, and hence the model performance is unchanged.
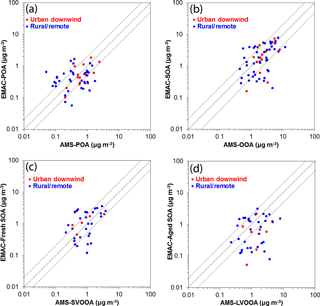
Figure 8Scatterplots comparing model predictions to AMS measurements and their PMF analysis for (a) POA, (b) OOA, (c) SVOOA, and (d) LVOOA concentrations (in µg m−3) in the Northern Hemisphere during 2001–2010. Each point represents the dataset average value over urban downwind (in red) and rural–remote (in blue) sites. Also shown are the 1:1, 2:1, and 1:2 lines.
Calculated SOA concentrations are higher than POA both downwind of major urban centers (2.76 µg m−3) and rural locations (2.53 µg m−3). The model reproduces the observed campaign average SOA concentrations within a factor of 2 in 65 % of the cases over urban downwind and rural locations. Simulated average SOA concentrations are slightly low-biased compared to AMS OOA measurements downwind of urban areas (MB = −0.22 µg m−3) and over rural areas (MB = −0.18 µg m−3). While the model performs well during spring (NMB = 11 %), summer (NMB = −10 %), and autumn (NMB = 0 %), it strongly underestimates SOA concentrations during winter (NMB = −76 %). This underestimation of SOA by the model is mostly due to missing LVOOA. For the 41 campaigns in which both OOA types were identified, EMAC reproduces fresh SOA concentrations well (compared to SVOOA) with an MB of 0.41 and 0.32 µg m−3 downwind of urban and in rural locations, respectively (Table 4). Furthermore, except during winter, the model is also able to capture the seasonal variations of the fresh SOA concentration (Table 4). However, the model underpredicts aged SOA concentrations (compared to AMS measured LVOOA) with an MB of −0.95 and −0.70 µg m−3 over urban downwind and rural locations, respectively. This aged SOA underestimation is also evident during the four seasons of the year, especially during winter (NMB = −93 %). This result may indicate that the model underestimates the atmospheric aging rate of SOA or misses processes forming highly oxidized OA, e.g., highly oxidized ELVOCs from the ozonolysis of terpenes (Ehn et al., 2014; Jokinen et al., 2016). Therefore, another useful feature of ORACLE 2-D is that detailed AMS measurements can be used to gain further insights into what causes biases and errors in its OA predictions. Compared to ORACLE (Tsimpidi et al., 2016), ORACLE 2-D tends to produce higher fresh SOA concentrations and slightly lower aged SOA concentrations. However, this discrepancy may be partially due to the fact that in ORACLE fresh SOA is assumed to correspond only to first-generation oxidation products, while in ORACLE 2-D fresh SOA is defined based on the O : C ratio and includes higher-generation oxidation products as well. Overall, the simulated average total SOA concentrations in ORACLE 2-D (2.59 µg m−3) are higher than ORACLE (1.91 µg m−3), reducing the gap with the corresponding AMS OOA (2.78 µg m−3).
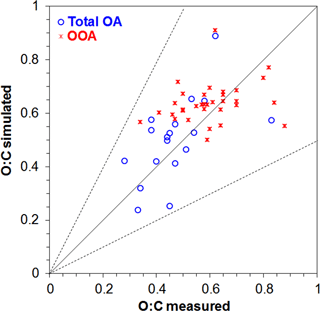
Figure 9Scatterplot comparing model predictions to measurements for O : C ratio of total OA (in blue) and OOA (in red) over nonurban areas in the Northern Hemisphere during 2001–2010. Each point represents the dataset average value over a specific measurement site. Also shown are the 1:1, 2:1, and 1:2 lines.
5.2 O : C ratio
The simulated O : C ratio of total OA and SOA is compared against observed O : C of OA and OOA from 30 and 57 field campaigns, respectively, performed during the period 2001–2010 in the Northern Hemisphere (Tables 5 and 6). Given that global models, including EMAC, underestimate concentrations of POA and SOA over urban locations (Tsigaridis et al., 2014; Tsimpidi et al., 2016), AMS data from these locations are not included for the statistical evaluation of calculated O : C (Fig. 9, Tables 7 and 8). In fact, the model tends to overestimate the O : C of total OA compared to observations from urban locations (e.g., Mexico City, Barcelona, New York, Riverside, Paris; Table 5). This overprediction can be attributed to the coarse spatial resolution and the difficulty to represent freshly emitted (or formed) OA on a local scale. On the other hand, the model performs remarkably well in reproducing the O : C ratio of both total OA (NMB = 7 %, RMSE = 0.13) and SOA (NMB = 5 %, RMSE = 0.12) in nonurban areas.
The model tends to overestimate the O : C ratio of total OA compared to observations from field campaigns close to the coasts (e.g., western coast of Chile, Mace Head). The model reproduces the low O : C ratios of total OA during winter and the higher values during spring and autumn very well (Table 7). Even if the model substantially underpredicts all types of OA during wintertime, the calculated OA O : C is similar to observations because the relative contribution of each OA compound is captured by the model. The slightly larger underprediction of aged SOA (compared to fresh SOA and POA) during winter results in a small underprediction of total OA O : C (NMB = −7 %). This indicates that the missing OA during winter cannot be attributed only to missing POA sources (e.g., residential biofuel use) but also to missing SOA formation pathways (e.g., multiphase oxidation). On the other hand, it overestimates the O : C of total OA during summer (MB = 0.14). Field campaigns conducted during the summer months provide O : C of total OA with surprisingly low O : C values for this time of the year (0.3–0.4), which are not captured by the model. Overall, the O : C of total OA is slightly overestimated by the model (MB = 0.04).
Simulated O : C of SOA is relatively high (0.5–0.7) at most observational sites, which agrees well with measurements. The model also captures the very high O : C values (larger than 0.7) observed over very remote areas (e.g., Okinawa, Finokalia). On the other hand, the low O : C ratios (lower than 0.5) reported by a few field campaigns are overestimated by the model (Duke Forest, Rhine Valley, Jiaxing). Remarkably, the model performance during winter is unbiased for the O : C ratio of SOA (Table 8). This is in contrast to its inability to reproduce SOA concentrations during winter (Tsimpidi et al., 2016). During the other seasons the model slightly overestimates the O : C ratio of SOA with an MB ranging from 0.02 (during spring) to 0.07 (during summer and autumn).
Furthermore, the O : C ratios of total OA presented here can be used to calculate the OM ∕ OC based on Eqs. (6) and (10). The average calculated OM ∕ OC is 1.8 compared to the observed 1.75. This is also in accordance with the OM ∕ OC value of 1.84 reported by Canagaratna et al. (2015) obtained from a large dataset of chamber and ambient OA measurements. The calculated OM ∕ OC ranges from 1.6 during winter to 2 during autumn, while the observed O : C ranges from 1.6 during summer to 1.8 during spring and autumn.
The ORACLE module for the description of OA composition and evolution in the atmosphere (Tsimpidi et al., 2014) has been extended to simultaneously simulate the volatility and the oxygen content of OA that results from atmospheric aging. Similar to ORACLE v1.0, the new version is implemented in the EMAC CCM and considers the formation of OA from emissions and chemical aging of LVOCs, SVOCs, and IVOCs from fossil fuel, biofuel, and biomass burning sources, as well as from the oxidation of anthropogenic and biogenic VOC precursors. The updated ORACLE module employs the 2-D VBS framework that uses logarithmically spaced effective saturation concentration bins to describe the volatility of organic compounds and linearly spaced oxygen-per-carbon-ratio bins to describe their oxygenation state.
Table 7Statistical evaluation of EMAC O : C ratio of total OA against observations from 18 datasets worldwide during 2001–2010.
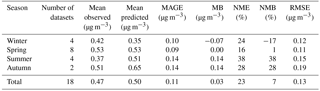
Table 8Statistical evaluation of EMAC O : C ratio of OOA against observations from 32 datasets worldwide during 2001–2010.
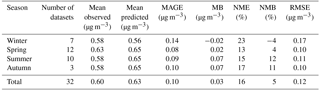
The simulated concentrations of OA and its components (i.e., POA and SOA) are similar to ORACLE v1.0 with relatively high concentrations over industrialized areas in the Northern Hemisphere and biomass burning areas in the tropics. The tropospheric burdens of POA and SOA are calculated to be 0.25 and 3.05 Tg, respectively, the latter being higher than with ORACLE v1.0. The new ORACLE 2-D module additionally allows for the calculation of the oxidation state of OA (in terms of O : C) and therefore its classification into fresh SOA (with O : C lower than 0.6) and aged SOA (with O : C higher than 0.6). O : C is calculated to be relatively low close to source regions and at high latitudes. The predicted O : C ratio is as low as 0.3 over boreal forests, 0.3–0.4 close to the anthropogenic sources in the Northern Hemisphere, 0.5 over the tropical forests, and higher than 0.6 downwind of source areas and at altitudes aloft. Accordingly, fresh SOA concentrations are higher close to sources, while aged SOA increases as the air masses are transported away from the sources and to higher altitudes. The estimated tropospheric burden of fresh SOA is 1.26 Tg and of aged SOA 1.79 Tg. The analysis of model results regarding the distribution of OA in the 2-D space of volatility and oxygen content showed that half of OA has µg m−3 over both anthropogenic areas (e.g., Europe) and tropical forests (e.g., Amazon). Furthermore, over Europe OA compounds are more strongly oxidized than over the Amazon and consist of 40 % fresh OA and 30 % aged SOA (compared to 55 % and 15 %, respectively, over the Amazon). The remaining 30 % in both areas consists of POA or very low oxidized material with O : C ≤ 0.2.
The simulated OA components (POA, fresh, aged, and total SOA) have been compared with observed subtypes of OA (sum of HOA and BBOA, SVOOA, LVOOA, and OOA) derived from AMS factor analysis results from 61 field campaigns performed during the period 2001–2010 over urban downwind and rural environments in the Northern Hemisphere. The model reproduces the POA concentrations over both types of environment with low bias. While the model appears to capture the fresh SOA concentrations reasonably well, it underestimates the concentrations of aged SOA, resulting in an overall underprediction of total SOA. While this aged SOA underestimation is evident throughout the year, it is more pronounced during winter. The underestimation of aged SOA by the model emphasizes the need to better describe chemical aging processes and further explore the effect of ELVOCs on the formation of SOA. Furthermore, the model realistically reproduces the observed O : C ratio of total OA over urban downwind and rural areas. It adequately captures the low values during winter and the higher values during spring and autumn; however, it overestimates the O : C of total OA during summer. In addition, the model slightly overestimates the O : C ratio of total OOA (NMB = 5 %), except during winter when modeled OOA O : C is unbiased.
ORACLE 2-D is a flexible module that efficiently describes organic aerosol composition and evolution in the atmosphere by simulating changes in OA volatility and oxygen content throughout its lifetime in the atmosphere. The ability of ORACLE 2-D to simulate the degree of OA oxidation can help determine changing OA hygroscopicity during atmospheric aging. ORACLE 2-D can potentially provide valuable insights into the composition and reactivity of OA and the physicochemical evolution during atmospheric transport of OA, which can help reduce aerosol-related uncertainties that persist in global atmospheric chemistry and climate modeling.
The complete ORACLE 2-D code can be obtained by applying for an EMAC license or upon request by emailing the first author. To use ORACLE 2-D as part of EMAC, please first apply for an ECHAM5 and a MESSy license. The GCM ECHAM5 has been developed at the Max Planck Institute for Meteorology in Hamburg (see http://www.mpimet.mpg.de/en/science/models/mpi-esm/echam/, ECHAM5, 2018). The Modular Earth Submodel System (MESSy) is developed and applied by a consortium of institutions initiated by the Max Planck Institute for Chemistry. The usage of MESSy and access to the source code is licensed to all affiliates of institutions that are members of the MESSy Consortium. Institutions can become a member of the MESSy Consortium by signing the MESSy Memorandum of Understanding. More information can be found on the MESSy Consortium website (https://www.messy-interface.org/, Messy, 2018). The measurement data used for the evaluation of the model can be found in Tables 5 and 6 of this paper and Tables S6 and S7 in the Supplement of Tsimpidi et al. (2016) (available online at https://doi.org/10.5194/acp-16-8939-2016-supplement).
The authors declare that they have no conflict of interest.
Alexandra P. Tsimpidi acknowledges support from a DFG individual grant program
(project reference TS 335/2-1), and Vlassis A. Karydis acknowledges support from
an
FP7 Marie Curie Career Integration Grant (project reference 618349).
The article processing charges for this open-access
publication
were covered by the Max Planck Society.
Edited by: Axel Lauer
Reviewed by: two anonymous referees
Ahmadov, R., McKeen, S. A., Robinson, A. L., Bahreini, R., Middlebrook, A. M., de Gouw, J. A., Meagher, J., Hsie, E. Y., Edgerton, E., Shaw, S., and Trainer, M.: A volatility basis set model for summertime secondary organic aerosols over the eastern United States in 2006, J. Geophys. Res.-Atmos., 117, D06301, https://doi.org/10.1029/2011jd016831, 2012.
Aiken, A. C., Decarlo, P. F., Kroll, J. H., Worsnop, D. R., Huffman, J. A., Docherty, K. S., Ulbrich, I. M., Mohr, C., Kimmel, J. R., Sueper, D., Sun, Y., Zhang, Q., Trimborn, A., Northway, M., Ziemann, P. J., Canagaratna, M. R., Onasch, T. B., Alfarra, M. R., Prevot, A. S. H., Dommen, J., Duplissy, J., Metzger, A., Baltensperger, U., and Jimenez, J. L.: O ∕ C and OM ∕ OC ratios of primary, secondary, and ambient organic aerosols with high-resolution time-of-flight aerosol mass spectrometry, Environmen. Sci. Technol., 42, 4478–4485, 2008.
Allan, J. D., Williams, P. I., Morgan, W. T., Martin, C. L., Flynn, M. J., Lee, J., Nemitz, E., Phillips, G. J., Gallagher, M. W., and Coe, H.: Contributions from transport, solid fuel burning and cooking to primary organic aerosols in two UK cities, Atmos. Chem. Phys., 10, 647–668, https://doi.org/10.5194/acp-10-647-2010, 2010.
Athanasopoulou, E., Vogel, H., Vogel, B., Tsimpidi, A. P., Pandis, S. N., Knote, C., and Fountoukis, C.: Modeling the meteorological and chemical effects of secondary organic aerosols during an EUCAARI campaign, Atmos. Chem. Phys., 13, 625–645, https://doi.org/10.5194/acp-13-625-2013, 2013.
Bacer, S., Sullivan, S. C., Karydis, V. A., Barahona, D., Krämer, M., Nenes, A., Tost, H., Tsimpidi, A. P., Lelieveld, J., and Pozzer, A.: Implementation of a comprehensive ice crystal formation parameterization for cirrus and mixed-phase clouds into the EMAC model (based on MESSy 2.53), Geosci. Model Dev. Discuss., https://doi.org/10.5194/gmd-2018-62, in review, 2018.
Canagaratna, M. R., Jimenez, J. L., Kroll, J. H., Chen, Q., Kessler, S. H., Massoli, P., Ruiz, L. H., Fortner, E., Williams, L. R., Wilson, K. R., Surratt, J. D., Donahue, N. M., Jayne, J. T., and Worsnop, D. R.: Elemental ratio measurements of organic compounds using aerosol mass spectrometry: characterization, improved calibration, and implications, Atmos. Chem. Phys., 15, 253–272, https://doi.org/10.5194/acp-15-253-2015, 2015.
Carbone, S., Aurela, M., Saarnio, K., Saarikoski, S., Timonen, H., Frey, A., Sueper, D., Ulbrich, I. M., Jimenez, J. L., Kulmala, M., Worsnop, D. R., and Hillamo, R. E.: Wintertime Aerosol Chemistry in Sub-Arctic Urban Air, Aerosol Sci. Tech., 48, 313–323, https://doi.org/10.1080/02786826.2013.875115, 2014.
Chacon-Madrid, H. J., Henry, K. M., and Donahue, N. M.: Photo-oxidation of pinonaldehyde at low NOx: from chemistry to organic aerosol formation, Atmos. Chem. Phys., 13, 3227–3236, https://doi.org/10.5194/acp-13-3227-2013, 2013.
Chang, R. Y. W., Slowik, J. G., Shantz, N. C., Vlasenko, A., Liggio, J., Sjostedt, S. J., Leaitch, W. R., and Abbatt, J. P. D.: The hygroscopicity parameter (kappa) of ambient organic aerosol at a field site subject to biogenic and anthropogenic influences: relationship to degree of aerosol oxidation, Atmos. Chem. Phys., 10, 5047–5064, https://doi.org/10.5194/acp-10-5047-2010, 2010.
Ciarelli, G., El Haddad, I., Bruns, E., Aksoyoglu, S., Mohler, O., Baltensperger, U., and Prevot, A. S. H.: Constraining a hybrid volatility basis-set model for aging of wood-burning emissions using smog chamber experiments: a box-model study based on the VBS scheme of the CAMx model (v5.40), Geosci. Model Dev., 10, 2303–2320, https://doi.org/10.5194/gmd-10-2303-2017, 2017.
Crippa, M., El Haddad, I., Slowik, J. G., DeCarlo, P. F., Mohr, C., Heringa, M. F., Chirico, R., Marchand, N., Sciare, J., Baltensperger, U., and Prevot, A. S. H.: Identification of marine and continental aerosol sources in Paris using high resolution aerosol mass spectrometry, J. Geophys. Res.-Atmos., 118, 1950–1963, https://doi.org/10.1002/jgrd.50151, 2013.
Crippa, M., Canonaco, F., Lanz, V. A., Äijälä, M., Allan, J. D., Carbone, S., Capes, G., Ceburnis, D., Dall'Osto, M., Day, D. A., DeCarlo, P. F., Ehn, M., Eriksson, A., Freney, E., Hildebrandt Ruiz, L., Hillamo, R., Jimenez, J. L., Junninen, H., Kiendler-Scharr, A., Kortelainen, A.-M., Kulmala, M., Laaksonen, A., Mensah, A. A., Mohr, C., Nemitz, E., O'Dowd, C., Ovadnevaite, J., Pandis, S. N., Petäjä, T., Poulain, L., Saarikoski, S., Sellegri, K., Swietlicki, E., Tiitta, P., Worsnop, D. R., Baltensperger, U., and Prévôt, A. S. H.: Organic aerosol components derived from 25 AMS data sets across Europe using a consistent ME-2 based source apportionment approach, Atmos. Chem. Phys., 14, 6159–6176, https://doi.org/10.5194/acp-14-6159-2014, 2014.
Docherty, K. S., Aiken, A. C., Huffman, J. A., Ulbrich, I. M., DeCarlo, P. F., Sueper, D., Worsnop, D. R., Snyder, D. C., Peltier, R. E., Weber, R. J., Grover, B. D., Eatough, D. J., Williams, B. J., Goldstein, A. H., Ziemann, P. J., and Jimenez, J. L.: The 2005 Study of Organic Aerosols at Riverside (SOAR-1): instrumental intercomparisons and fine particle composition, Atmos. Chem. Phys., 11, 12387–12420, https://doi.org/10.5194/acp-11-12387-2011, 2011.
Donahue, N. M., Robinson, A. L., Stanier, C. O., and Pandis, S. N.: Coupled partitioning, dilution, and chemical aging of semivolatile organics, Environ. Sci. Technol., 40, 2635–2643, 2006.
Donahue, N. M., Epstein, S. A., Pandis, S. N., and Robinson, A. L.: A two-dimensional volatility basis set: 1. organic–aerosol mixing thermodynamics, Atmos. Chem. Phys., 11, 3303–3318, https://doi.org/10.5194/acp-11-3303-2011, 2011.
ECHAM5: available at: http://publications.copernicus.org/, last access: 14 August 2018.
Ehn, M., Thornton, J. A., Kleist, E., Sipila, M., Junninen, H., Pullinen, I., Springer, M., Rubach, F., Tillmann, R., Lee, B., Lopez-Hilfiker, F., Andres, S., Acir, I.-H., Rissanen, M., Jokinen, T., Schobesberger, S., Kangasluoma, J., Kontkanen, J., Nieminen, T., Kurten, T., Nielsen, L. B., Jorgensen, S., Kjaergaard, H. G., Canagaratna, M., Maso, M. D., Berndt, T., Petaja, T., Wahner, A., Kerminen, V.-M., Kulmala, M., Worsnop, D. R., Wildt, J., and Mentel, T. F.: A large source of low-volatility secondary organic aerosol, Nature, 506, 476–479, https://doi.org/10.1038/nature13032, 2014.
Fountoukis, C., Megaritis, A. G., Skyllakou, K., Charalampidis, P. E., Pilinis, C., van der Gon, H., Crippa, M., Canonaco, F., Mohr, C., Prevot, A. S. H., Allan, J. D., Poulain, L., Petaja, T., Tiitta, P., Carbone, S., Kiendler-Scharr, A., Nemitz, E., O'Dowd, C., Swietlicki, E., and Pandis, S. N.: Organic aerosol concentration and composition over Europe: insights from comparison of regional model predictions with aerosol mass spectrometer factor analysis, Atmos. Chem. Phys., 14, 9061–9076, https://doi.org/10.5194/acp-14-9061-2014, 2014.
Gao, C. Y., Tsigaridis, K., and Bauer, S. E.: MATRIX-VBS (v1.0): implementing an evolving organic aerosol volatility in an aerosol microphysics model, Geosci. Model Dev., 10, 751–764, https://doi.org/10.5194/gmd-10-751-2017, 2017.
Ge, X. L., Setyan, A., Sun, Y. L., and Zhang, Q.: Primary and secondary organic aerosols in Fresno, California during wintertime: Results from high resolution aerosol mass spectrometry, J. Geophys. Res.-Atmos., 117, D19301, https://doi.org/10.1029/2012jd018026, 2012.
Gong, Z. H., Lan, Z. J., Xue, L., Zeng, L. W., He, L. Y., and Huang, X. F.: Characterization of submicron aerosols in the urban outflow of the central Pearl River Delta region of China, Front. Environ. Sci. Eng., 6, 725–733, https://doi.org/10.1007/s11783-012-0441-8, 2012.
Guo, H. Y., Liu, J. M., Froyd, K. D., Roberts, J. M., Veres, P. R., Hayes, P. L., Jimenez, J. L., Nenes, A., and Weber, R. J.: Fine particle pH and gas-particle phase partitioning of inorganic species in Pasadena, California, during the 2010 CalNex campaign, Atmos. Chem. Phys., 17, 5703–5719, https://doi.org/10.5194/acp-17-5703-2017, 2017.
Hawkins, L. N., Russell, L. M., Covert, D. S., Quinn, P. K., and Bates, T. S.: Carboxylic acids, sulfates, and organosulfates in processed continental organic aerosol over the southeast Pacific Ocean during VOCALS-REx 2008, J. Geophys. Res.-Atmos., 115, D12304, https://doi.org/10.1029/2009jd013276, 2010.
Hayes, P. L., Ortega, A. M., Cubison, M. J., Froyd, K. D., Zhao, Y., Cliff, S. S., Hu, W. W., Toohey, D. W., Flynn, J. H., Lefer, B. L., Grossberg, N., Alvarez, S., Rappenglueck, B., Taylor, J. W., Allan, J. D., Holloway, J. S., Gilman, J. B., Kuster, W. C., De Gouw, J. A., Massoli, P., Zhang, X., Liu, J., Weber, R. J., Corrigan, A. L., Russell, L. M., Isaacman, G., Worton, D. R., Kreisberg, N. M., Goldstein, A. H., Thalman, R., Waxman, E. M., Volkamer, R., Lin, Y. H., Surratt, J. D., Kleindienst, T. E., Offenberg, J. H., Dusanter, S., Griffith, S., Stevens, P. S., Brioude, J., Angevine, W. M., and Jimenez, J. L.: Organic aerosol composition and sources in Pasadena, California, during the 2010 CalNex campaign, J. Geophys. Res.-Atmos., 118, 9233–9257, https://doi.org/10.1002/jgrd.50530, 2013.
He, L. Y., Huang, X. F., Xue, L., Hu, M., Lin, Y., Zheng, J., Zhang, R. Y., and Zhang, Y. H.: Submicron aerosol analysis and organic source apportionment in an urban atmosphere in Pearl River Delta of China using high-resolution aerosol mass spectrometry, J. Geophys. Res.-Atmos., 116, D21305, https://doi.org/10.1029/2010jd014566, 2011.
Heald, C. L., Kroll, J. H., Jimenez, J. L., Docherty, K. S., DeCarlo, P. F., Aiken, A. C., Chen, Q., Martin, S. T., Farmer, D. K., and Artaxo, P.: A simplified description of the evolution of organic aerosol composition in the atmosphere, Geophys. Res. Lett., 37, L08803, https://doi.org/10.1029/2010GL042737, 2010.
Hodzic, A., Kasibhatla, P. S., Jo, D. S., Cappa, C. D., Jimenez, J. L., Madronich, S., and Park, R. J.: Rethinking the global secondary organic aerosol (SOA) budget: stronger production, faster removal, shorter lifetime, Atmos. Chem. Phys., 16, 7917–7941, https://doi.org/10.5194/acp-16-7917-2016, 2016.
Holzinger, R., Kasper-Giebl, A., Staudinger, M., Schauer, G., and Rockmann, T.: Analysis of the chemical composition of organic aerosol at the Mt. Sonnblick observatory using a novel high mass resolution thermal-desorption proton-transfer-reaction mass-spectrometer (hr-TD-PTR-MS), Atmos. Chem. Phys., 10, 10111–10128, https://doi.org/10.5194/acp-10-10111-2010, 2010.
Hu, W. W., Hu, M., Hu, W., Jimenez, J. L., Yuan, B., Chen, W. T., Wang, M., Wu, Y. S., Chen, C., Wang, Z. B., Peng, J. F., Zeng, L. M., and Shao, M.: Chemical composition, sources, and aging process of submicron aerosols in Beijing: Contrast between summer and winter, J. Geophys. Res.-Atmos., 121, 1955–1977, https://doi.org/10.1002/2015jd024020, 2016.
Huang, X. F., He, L. Y., Hu, M., Canagaratna, M. R., Sun, Y., Zhang, Q., Zhu, T., Xue, L., Zeng, L. W., Liu, X. G., Zhang, Y. H., Jayne, J. T., Ng, N. L., and Worsnop, D. R.: Highly time-resolved chemical characterization of atmospheric submicron particles during 2008 Beijing Olympic Games using an Aerodyne High-Resolution Aerosol Mass Spectrometer, Atmos. Chem. Phys., 10, 8933–8945, https://doi.org/10.5194/acp-10-8933-2010, 2010.
Huang, X. F., He, L. Y., Hu, M., Canagaratna, M. R., Kroll, J. H., Ng, N. L., Zhang, Y. H., Lin, Y., Xue, L., Sun, T. L., Liu, X. G., Shao, M., Jayne, J. T., and Worsnop, D. R.: Characterization of submicron aerosols at a rural site in Pearl River Delta of China using an Aerodyne High-Resolution Aerosol Mass Spectrometer, Atmos. Chem. Phys., 11, 1865–1877, https://doi.org/10.5194/acp-11-1865-2011, 2011.
Huang, X. F., Xue, L., Tian, X. D., Shao, W. W., Sun, T. L., Gong, Z. H., Ju, W. W., Jiang, B., Hu, M., and He, L. Y.: Highly time-resolved carbonaceous aerosol characterization in Yangtze River Delta of China: Composition, mixing state and secondary formation, Atmos. Environ., 64, 200–207, https://doi.org/10.1016/j.atmosenv.2012.09.059, 2013.
Jathar, S. H., Farina, S. C., Robinson, A. L., and Adams, P. J.: The influence of semi-volatile and reactive primary emissions on the abundance and properties of global organic aerosol, Atmos. Chem. Phys., 11, 7727–7746, https://doi.org/10.5194/acp-11-7727-2011, 2011.
Jathar, S. H., Mahmud, A., Barsanti, K. C., Asher, W. E., Pankow, J. F., and Kleeman, M. J.: Water uptake by organic aerosol and its influence on gas/particle partitioning of secondary organic aerosol in the United States, Atmos. Environ., 129, 142–154, https://doi.org/10.1016/j.atmosenv.2016.01.001, 2016.
Jo, D. S., Park, R. J., Kim, M. J., and Spracklen, D. V.: Effects of chemical aging on global secondary organic aerosol using the volatility basis set approach, Atmos. Environ., 81, 230–244, 2013.
Jöckel, P., Tost, H., Pozzer, A., Brühl, C., Buchholz, J., Ganzeveld, L., Hoor, P., Kerkweg, A., Lawrence, M. G., Sander, R., Steil, B., Stiller, G., Tanarhte, M., Taraborrelli, D., van Aardenne, J., and Lelieveld, J.: The atmospheric chemistry general circulation model ECHAM5/MESSy1: consistent simulation of ozone from the surface to the mesosphere, Atmos. Chem. Phys., 6, 5067–5104, https://doi.org/10.5194/acp-6-5067-2006, 2006.
Jokinen, T., Kausiala, O., Garmash, O., Perakyla, O., Junninen, H., Schobesberger, S., Yan, C., Sipila, M., and Rissanen, M. P.: Production of highly oxidized organic compounds from ozonolysis of beta-caryophyllene: laboratory and field measurements, Boreal Environ. Res., 21, 262–273, 2016.
Karydis, V. A., Tsimpidi, A. P., Pozzer, A., Astitha, M., and Lelieveld, J.: Effects of mineral dust on global atmospheric nitrate concentrations, Atmos. Chem. Phys., 16, 1491–1509, https://doi.org/10.5194/acp-16-1491-2016, 2016.
Karydis, V. A., Tsimpidi, A. P., Bacer, S., Pozzer, A., Nenes, A., and Lelieveld, J.: Global impact of mineral dust on cloud droplet number concentration, Atmos. Chem. Phys., 17, 5601–5621, https://doi.org/10.5194/acp-17-5601-2017, 2017.
Kerkweg, A., Buchholz, J., Ganzeveld, L., Pozzer, A., Tost, H., and Jöckel, P.: Technical Note: An implementation of the dry removal processes DRY DEPosition and SEDImentation in the Modular Earth Submodel System (MESSy), Atmos. Chem. Phys., 6, 4617–4632, https://doi.org/10.5194/acp-6-4617-2006, 2006a.
Kerkweg, A., Sander, R., Tost, H., and Jöckel, P.: Technical note: Implementation of prescribed (OFFLEM), calculated (ONLEM), and pseudo-emissions (TNUDGE) of chemical species in the Modular Earth Submodel System (MESSy), Atmos. Chem. Phys., 6, 3603–3609, https://doi.org/10.5194/acp-6-3603-2006, 2006b.
Koo, B., Knipping, E., and Yarwood, G.: 1.5-Dimensional volatility basis set approach for modeling organic aerosol in CAMx and CMAQ, Atmos. Environ., 95, 158–164, https://doi.org/10.1016/j.atmosenv.2014.06.031, 2014.
Kostenidou, E., Florou, K., Kaltsonoudis, C., Tsiflikiotou, M., Vratolis, S., Eleftheriadis, K., and Pandis, S. N.: Sources and chemical characterization of organic aerosol during the summer in the eastern Mediterranean, Atmos. Chem. Phys., 15, 11355–11371, https://doi.org/10.5194/acp-15-11355-2015, 2015.
Kroll, J. H., Donahue, N. M., Jimenez, J. L., Kessler, S. H., Canagaratna, M. R., Wilson, K. R., Altieri, K. E., Mazzoleni, L. R., Wozniak, A. S., Bluhm, H., Mysak, E. R., Smith, J. D., Kolb, C. E., and Worsnop, D. R.: Carbon oxidation state as a metric for describing the chemistry of atmospheric organic aerosol, Nat. Chem., 3, 133–139, 2011.
Kuwata, M. and Lee, W. C.: 1-octanol-water partitioning as a classifier of water soluble organic matters: Implication for solubility distribution, Aerosol Sci. Tech., 51, 602–613, https://doi.org/10.1080/02786826.2017.1283004, 2017.
Lambe, A. T., Onasch, T. B., Massoli, P., Croasdale, D. R., Wright, J. P., Ahern, A. T., Williams, L. R., Worsnop, D. R., Brune, W. H., and Davidovits, P.: Laboratory studies of the chemical composition and cloud condensation nuclei (CCN) activity of secondary organic aerosol (SOA) and oxidized primary organic aerosol (OPOA), Atmos. Chem. Phys., 11, 8913–8928, https://doi.org/10.5194/acp-11-8913-2011, 2011.
Lane, T. E., Donahue, N. M., and Pandis, S. N.: Simulating secondary organic aerosol formation using the volatility basis-set approach in a chemical transport model, Atmos. Environ., 42, 7439–7451, 2008.
Lauer, A., Eyring, V., Hendricks, J., Jöckel, P., and Lohmann, U.: Global model simulations of the impact of ocean-going ships on aerosols, clouds, and the radiation budget, Atmos. Chem. Phys., 7, 5061–5079, https://doi.org/10.5194/acp-7-5061-2007, 2007.
Liggio, J., Li, S. M., Vlasenko, A., Sjostedt, S., Chang, R., Shantz, N., Abbatt, J., Slowik, J. G., Bottenheim, J. W., Brickell, P. C., Stroud, C., and Leaitch, W. R.: Primary and secondary organic aerosols in urban air masses intercepted at a rural site, J. Geophys. Res.-Atmos., 115, 15002, https://doi.org/10.1029/2010jd014426, 2010.
Louvaris, E. E., Florou, K., Karnezi, E., Papanastasiou, D. K., Gkatzelis, G. I., and Pandis, S. N.: Volatility of source apportioned wintertime organic aerosol in the city of Athens, Atmos. Enviro., 158, 138–147, 2017.
Martin, S. T., Andreae, M. O., Althausen, D., Artaxo, P., Baars, H., Borrmann, S., Chen, Q., Farmer, D. K., Guenther, A., Gunthe, S. S., Jimenez, J. L., Karl, T., Longo, K., Manzi, A., Muller, T., Pauliquevis, T., Petters, M. D., Prenni, A. J., Poschl, U., Rizzo, L. V., Schneider, J., Smith, J. N., Swietlicki, E., Tota, J., Wang, J., Wiedensohler, A., and Zorn, S. R.: An overview of the Amazonian Aerosol Characterization Experiment 2008 (AMAZE-08), Atmos. Chem. Phys., 10, 11415–11438, https://doi.org/10.5194/acp-10-11415-2010, 2010.
May, A. A., Levin, E. J. T., Hennigan, C. J., Riipinen, I., Lee, T., Collett, J. L., Jimenez, J. L., Kreidenweis, S. M., and Robinson, A. L.: Gas-particle partitioning of primary organic aerosol emissions: 3. Biomass burning, J. Geophys. Res.-Atmos., 118, 11327–11338, https://doi.org/10.1002/jgrd.50828, 2013.
MESSy: available at: https://www.messy-interface.org/, last access: 14 August 2018.
Mohr, C., DeCarlo, P. F., Heringa, M. F., Chirico, R., Slowik, J. G., Richter, R., Reche, C., Alastuey, A., Querol, X., Seco, R., Penuelas, J., Jimenez, J. L., Crippa, M., Zimmermann, R., Baltensperger, U., and Prevot, A. S. H.: Identification and quantification of organic aerosol from cooking and other sources in Barcelona using aerosol mass spectrometer data, Atmos. Chem. Phys., 12, 1649–1665, https://doi.org/10.5194/acp-12-1649-2012, 2012.
Murphy, B. N. and Pandis, S. N.: Simulating the formation of semivolatile primary and secondary organic aerosol in a regional chemical transport model, Environ. Sci. Technol., 43, 4722–4728, 2009.
Murphy, B. N., Donahue, N. M., Fountoukis, C., and Pandis, S. N.: Simulating the oxygen content of ambient organic aerosol with the 2D volatility basis set, Atmos. Chem. Phys., 11, 7859–7873, https://doi.org/10.5194/acp-11-7859-2011, 2011.
Murphy, B. N., Donahue, N. M., Fountoukis, C., Dall'Osto, M., O'Dowd, C., Kiendler-Scharr, A., and Pandis, S. N.: Functionalization and fragmentation during ambient organic aerosol aging: application of the 2-D volatility basis set to field studies, Atmos. Chem. Phys., 12, 10797–10816, https://doi.org/10.5194/acp-12-10797-2012, 2012.
Napier, W. J., Ensberg, J. J., and Seinfeld, J. H.: Insight into the numerical challenges of implementing 2-dimensional SOA models in atmospheric chemical transport models, Atmos. Environ., 96, 331–344, https://doi.org/10.1016/j.atmosenv.2014.07.048, 2014.
Ng, N. L., Canagaratna, M. R., Zhang, Q., Jimenez, J. L., Tian, J., Ulbrich, I. M., Kroll, J. H., Docherty, K. S., Chhabra, P. S., Bahreini, R., Murphy, S. M., Seinfeld, J. H., Hildebrandt, L., Donahue, N. M., DeCarlo, P. F., Lanz, V. A., Prévôt, A. S. H., Dinar, E., Rudich, Y., and Worsnop, D. R.: Organic aerosol components observed in Northern Hemispheric datasets from Aerosol Mass Spectrometry, Atmos. Chem. Phys., 10, 4625–4641, https://doi.org/10.5194/acp-10-4625-2010, 2010.
Paciga, A., Karnezi, E., Kostenidou, E., Hildebrandt, L., Psichoudaki, M., Engelhart, G. J., Lee, B. H., Crippa, M., Prevot, A. S. H., Baltensperger, U., and Pandis, S. N.: Volatility of organic aerosol and its components in the megacity of Paris, Atmos. Chem. Phys., 16, 2013–2023, https://doi.org/10.5194/acp-16-2013-2016, 2016.
Pankow, J. F. and Asher, W. E.: SIMPOL.1: a simple group contribution method for predicting vapor pressures and enthalpies of vaporization of multifunctional organic compounds, Atmos. Chem. Phys., 8, 2773–2796, https://doi.org/10.5194/acp-8-2773-2008, 2008.
Pathak, R. K., Presto, A. A., Lane, T. E., Stanier, C. O., Donahue, N. M., and Pandis, S. N.: Ozonolysis of α-pinene: parameterization of secondary organic aerosol mass fraction, Atmos. Chem. Phys., 7, 3811–3821, https://doi.org/10.5194/acp-7-3811-2007, 2007.
Pozzer, A., Zimmermann, P., Doering, U. M., van Aardenne, J., Tost, H., Dentener, F., Janssens-Maenhout, G., and Lelieveld, J.: Effects of business-as-usual anthropogenic emissions on air quality, Atmos. Chem. Phys., 12, 6915–6937, https://doi.org/10.5194/acp-12-6915-2012, 2012.
Pringle, K. J., Tost, H., Message, S., Steil, B., Giannadaki, D., Nenes, A., Fountoukis, C., Stier, P., Vignati, E., and Leieved, J.: Description and evaluation of GMXe: a new aerosol submodel for global simulations (v1), Geosci. Model Dev., 3, 391–412, https://doi.org/10.5194/gmd-3-391-2010, 2010.
Pye, H. O. T. and Seinfeld, J. H.: A global perspective on aerosol from low-volatility organic compounds, Atmos. Chem. Phys., 10, 4377–4401, https://doi.org/10.5194/acp-10-4377-2010, 2010.
Robinson, A. L., Donahue, N. M., Shrivastava, M. K., Weitkamp, E. A., Sage, A. M., Grieshop, A. P., Lane, T. E., Pierce, J. R., and Pandis, S. N.: Rethinking organic aerosols: Semivolatile emissions and photochemical aging, Science, 315, 1259–1262, 2007.
Roeckner, E., Brokopf, R., Esch, M., Giorgetta, M., Hagemann, S., Kornblueh, L., Manzini, E., Schlese, U., and Schulzweida, U.: Sensitivity of simulated climate to horizontal and vertical resolution in the ECHAM5 atmosphere model, J. Climate, 19, 3771–3791, https://doi.org/10.1175/jcli3824.1, 2006.
Saarikoski, S., Carbone, S., Decesari, S., Giulianelli, L., Angelini, F., Canagaratna, M., Ng, N. L., Trimborn, A., Facchini, M. C., Fuzzi, S., Hillamo, R., and Worsnop, D.: Chemical characterization of springtime submicrometer aerosol in Po Valley, Italy, Atmos. Chem. Phys., 12, 8401–8421, https://doi.org/10.5194/acp-12-8401-2012, 2012.
Sander, R., Baumgaertner, A., Gromov, S., Harder, H., Jöckel, P., Kerkweg, A., Kubistin, D., Regelin, E., Riede, H., Sandu, A., Taraborrelli, D., Tost, H., and Xie, Z.-Q.: The atmospheric chemistry box model CAABA/MECCA-3.0, Geosci. Model Dev., 4, 373–380, https://doi.org/10.5194/gmd-4-373-2011, 2011.
Shiraiwa, M., Berkemeier, T., Schilling-Fahnestock, K. A., Seinfeld, J. H., and Pöschl, U.: Molecular corridors and kinetic regimes in the multiphase chemical evolution of secondary organic aerosol, Atmos. Chem. Phys., 14, 8323–8341, https://doi.org/10.5194/acp-14-8323-2014, 2014.
Shiraiwa, M., Li, Y., Tsimpidi, A. P., Karydis, V. A., Berkemeier, T., Pandis, S. N., Lelieveld, J., Koop, T., and Pöschl, U.: Global distribution of particle phase state in atmospheric secondary organic aerosols, Nat. Commun., 8, 15002, https://doi.org/10.1038/ncomms15002, 2017.
Sun, Y., Zhang, Q., Macdonald, A. M., Hayden, K., Li, S. M., Liggio, J., Liu, P. S. K., Anlauf, K. G., Leaitch, W. R., Steffen, A., Cubison, M., Worsnop, D. R., van Donkelaar, A., and Martin, R. V.: Size-resolved aerosol chemistry on Whistler Mountain, Canada with a high-resolution aerosol mass spectrometer during INTEX-B, Atmos. Chem. Phys., 9, 3095–3111, https://doi.org/10.5194/acp-9-3095-2009, 2009.
Sun, Y. L., Zhang, Q., Schwab, J. J., Demerjian, K. L., Chen, W. N., Bae, M. S., Hung, H. M., Hogrefe, O., Frank, B., Rattigan, O. V., and Lin, Y. C.: Characterization of the sources and processes of organic and inorganic aerosols in New York city with a high-resolution time-of-flight aerosol mass apectrometer, Atmos. Chem. Phys., 11, 1581–1602, https://doi.org/10.5194/acp-11-1581-2011, 2011.
Thomson, A. M., Calvin, K. V., Smith, S. J., Kyle, G. P., Volke, A., Patel, P., Delgado-Arias, S., Bond-Lamberty, B., Wise, M. A., Clarke, L. E., and Edmonds, J. A.: RCP4.5: a pathway for stabilization of radiative forcing by 2100, Climatic Change, 109, 77–94, 2011.
Timonen, H., Carbone, S., Aurela, M., Saarnio, K., Saarikoski, S., Ng, N. L., Canagaratna, M. R., Kulmala, M., Kerminen, V. M., Worsnop, D. R., and Hillamo, R.: Characteristics, sources and water-solubility of ambient submicron organic aerosol in springtime in Helsinki, Finland, J. Aerosol Sci., 56, 61–77, https://doi.org/10.1016/j.jaerosci.2012.06.005, 2013.
Tost, H., Jöckel, P., Kerkweg, A., Sander, R., and Lelieveld, J.: Technical note: A new comprehensive SCAVenging submodel for global atmospheric chemistry modelling, Atmos. Chem. Phys., 6, 565–574, https://doi.org/10.5194/acp-6-565-2006, 2006.
Trail, M., Tsimpidi, A. P., Liu, P., Tsigaridis, K., Hu, Y., Nenes, A., and Russell, A. G.: Downscaling a global climate model to simulate climate change over the US and the implication on regional and urban air quality, Geosci. Model Dev., 6, 1429–1445, https://doi.org/10.5194/gmd-6-1429-2013, 2013.
Trail, M., Tsimpidi, A. P., Liu, P., Tsigaridis, K., Rudokas, J., Miller, P., Nenes, A., Hu, Y., and Russell, A. G.: Sensitivity of air quality to potential future climate change and emissions in the United States and major cities, Atmos. Environ., 94, 552–563, https://doi.org/10.1016/j.atmosenv.2014.05.079, 2014.
Tsigaridis, K., Daskalakis, N., Kanakidou, M., Adams, P. J., Artaxo, P., Bahadur, R., Balkanski, Y., Bauer, S. E., Bellouin, N., Benedetti, A., Bergman, T., Berntsen, T. K., Beukes, J. P., Bian, H., Carslaw, K. S., Chin, M., Curci, G., Diehl, T., Easter, R. C., Ghan, S. J., Gong, S. L., Hodzic, A., Hoyle, C. R., Iversen, T., Jathar, S., Jimenez, J. L., Kaiser, J. W., Kirkevag, A., Koch, D., Kokkola, H., Lee, Y. H., Lin, G., Liu, X., Luo, G., Ma, X., Mann, G. W., Mihalopoulos, N., Morcrette, J. J., Mueller, J. F., Myhre, G., Myriokefalitakis, S., Ng, N. L., O'Donnell, D., Penner, J. E., Pozzoli, L., Pringle, K. J., Russell, L. M., Schulz, M., Sciare, J., Seland, O., Shindell, D. T., Sillman, S., Skeie, R. B., Spracklen, D., Stavrakou, T., Steenrod, S. D., Takemura, T., Tiitta, P., Tilmes, S., Tost, H., van Noije, T., van Zyl, P. G., von Salzen, K., Yu, F., Wang, Z., Wang, Z., Zaveri, R. A., Zhang, H., Zhang, K., Zhang, Q., and Zhang, X.: The AeroCom evaluation and intercomparison of organic aerosol in global models, Atmos. Chem. Phys., 14, 10845–10895, https://doi.org/10.5194/acp-14-10845-2014, 2014.
Tsimpidi, A. P., Karydis, V. A., Zavala, M., Lei, W., Molina, L., Ulbrich, I. M., Jimenez, J. L., and Pandis, S. N.: Evaluation of the volatility basis-set approach for the simulation of organic aerosol formation in the Mexico City metropolitan area, Atmos. Chem. Phys., 10, 525–546, https://doi.org/10.5194/acp-10-525-2010, 2010.
Tsimpidi, A. P., Karydis, V. A., Zavala, M., Lei, W., Bei, N., Molina, L., and Pandis, S. N.: Sources and production of organic aerosol in Mexico City: insights from the combination of a chemical transport model (PMCAMx-2008) and measurements during MILAGRO, Atmos. Chem. Phys., 11, 5153–5168, https://doi.org/10.5194/acp-11-5153-2011, 2011.
Tsimpidi, A. P., Karydis, V. A., Pozzer, A., Pandis, S. N., and Lelieveld, J.: ORACLE (v1.0): module to simulate the organic aerosol composition and evolution in the atmosphere, Geosci. Model Dev., 7, 3153–3172, https://doi.org/10.5194/gmd-7-3153-2014, 2014.
Tsimpidi, A. P., Karydis, V. A., Pandis, S. N., and Lelieveld, J.: Global combustion sources of organic aerosols: model comparison with 84 AMS factor-analysis data sets, Atmos. Chem. Phys., 16, 8939–8962, https://doi.org/10.5194/acp-16-8939-2016, 2016.
Tsimpidi, A. P., Karydis, V. A., Pandis, S. N., and Lelieveld, J.: Global-scale combustion sources of organic aerosols: sensitivity to formation and removal mechanisms, Atmos. Chem. Phys., 17, 7345–7364, https://doi.org/10.5194/acp-17-7345-2017, 2017.
van der Gon, H., Bergstrom, R., Fountoukis, C., Johansson, C., Pandis, S. N., Simpson, D., and Visschedijk, A. J. H.: Particulate emissions from residential wood combustion in Europe revised estimates and an evaluation, Atmos. Chem. Phys., 15, 6503–6519, https://doi.org/10.5194/acp-15-6503-2015, 2015.
van der Werf, G. R., Randerson, J. T., Giglio, L., Collatz, G. J., Mu, M., Kasibhatla, P. S., Morton, D. C., DeFries, R. S., Jin, Y., and van Leeuwen, T. T.: Global fire emissions and the contribution of deforestation, savanna, forest, agricultural, and peat fires (1997–2009), Atmos. Chem. Phys., 10, 11707–11735, https://doi.org/10.5194/acp-10-11707-2010, 2010.
Xu, L., Guo, H. Y., Boyd, C. M., Klein, M., Bougiatioti, A., Cerully, K. M., Hite, J. R., Isaacman-VanWertz, G., Kreisberg, N. M., Knote, C., Olson, K., Koss, A., Goldstein, A. H., Hering, S. V., de Gouw, J., Baumann, K., Lee, S. H., Nenes, A., Weber, R. J., and Ng, N. L.: Effects of anthropogenic emissions on aerosol formation from isoprene and monoterpenes in the southeastern United States, P. Natl. Acad. Sci. USA, 112, 37–42, https://doi.org/10.1073/pnas.1417609112, 2015.
Zhang, Q., Jimenez, J. L., Canagaratna, M. R., Allan, J. D., Coe, H., Ulbrich, I., Alfarra, M. R., Takami, A., Middlebrook, A. M., Sun, Y. L., Dzepina, K., Dunlea, E., Docherty, K., DeCarlo, P. F., Salcedo, D., Onasch, T., Jayne, J. T., Miyoshi, T., Shimono, A., Hatakeyama, S., Takegawa, N., Kondo, Y., Schneider, J., Drewnick, F., Borrmann, S., Weimer, S., Demerjian, K., Williams, P., Bower, K., Bahreini, R., Cottrell, L., Griffin, R. J., Rautiainen, J., Sun, J. Y., Zhang, Y. M., and Worsnop, D. R.: Ubiquity and dominance of oxygenated species in organic aerosols in anthropogenically-influenced Northern Hemisphere midlatitudes, Geophys. Res. Lett., 34, L13801, https://doi.org/10.1029/2007gl029979, 2007.
Zhao, B., Wang, S. X., Donahue, N. M., Chuang, W. N., Hildebrandt Ruiz, L., Ng, N. L., Wang, Y. J., and Hao, J. M.: Evaluation of One-Dimensional and Two-Dimensional Volatility Basis Sets in Simulating the Aging of Secondary Organic Aerosol with Smog-Chamber Experiments, Environ. Sci. Technol., 49, 2245–2254, https://doi.org/10.1021/es5048914, 2015.