the Creative Commons Attribution 4.0 License.
the Creative Commons Attribution 4.0 License.
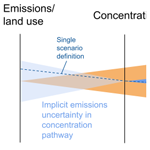
The need for carbon-emissions-driven climate projections in CMIP7
Benjamin M. Sanderson
Ben B. B. Booth
John Dunne
Veronika Eyring
Rosie A. Fisher
Pierre Friedlingstein
Matthew J. Gidden
Tomohiro Hajima
Chris D. Jones
Colin G. Jones
Andrew King
Charles D. Koven
David M. Lawrence
Jason Lowe
Nadine Mengis
Glen P. Peters
Joeri Rogelj
Chris Smith
Abigail C. Snyder
Isla R. Simpson
Abigail L. S. Swann
Claudia Tebaldi
Tatiana Ilyina
Carl-Friedrich Schleussner
Roland Séférian
Bjørn H. Samset
Detlef van Vuuren
Sönke Zaehle
Previous phases of the Coupled Model Intercomparison Project (CMIP) have primarily focused on simulations driven by atmospheric concentrations of greenhouse gases (GHGs), for both idealized model experiments and climate projections of different emissions scenarios. We argue that although this approach was practical to allow parallel development of Earth system model simulations and detailed socioeconomic futures, carbon cycle uncertainty as represented by diverse, process-resolving Earth system models (ESMs) is not manifested in the scenario outcomes, thus omitting a dominant source of uncertainty in meeting the Paris Agreement. Mitigation policy is defined in terms of human activity (including emissions), with strategies varying in their timing of net-zero emissions, the balance of mitigation effort between short-lived and long-lived climate forcers, their reliance on land use strategy, and the extent and timing of carbon removals. To explore the response to these drivers, ESMs need to explicitly represent complete cycles of major GHGs, including natural processes and anthropogenic influences. Carbon removal and sequestration strategies, which rely on proposed human management of natural systems, are currently calculated in integrated assessment models (IAMs) during scenario development with only the net carbon emissions passed to the ESM. However, proper accounting of the coupled system impacts of and feedback on such interventions requires explicit process representation in ESMs to build self-consistent physical representations of their potential effectiveness and risks under climate change. We propose that CMIP7 efforts prioritize simulations driven by CO2 emissions from fossil fuel use and projected deployment of carbon dioxide removal technologies, as well as land use and management, using the process resolution allowed by state-of-the-art ESMs to resolve carbon–climate feedbacks. Post-CMIP7 ambitions should aim to incorporate modeling of non-CO2 GHGs (in particular, sources and sinks of methane and nitrous oxide) and process-based representation of carbon removal options. These developments will allow three primary benefits: (1) resources to be allocated to policy-relevant climate projections and better real-time information related to the detectability and verification of emissions reductions and their relationship to expected near-term climate impacts, (2) scenario modeling of the range of possible future climate states including Earth system processes and feedbacks that are increasingly well-represented in ESMs, and (3) optimal utilization of the strengths of ESMs in the wider context of climate modeling infrastructure (which includes simple climate models, machine learning approaches and kilometer-scale climate models).
- Article
(1784 KB) - Full-text XML
- BibTeX
- EndNote
Past phases of the Coupled Model Intercomparison Project (CMIP; Meehl et al., 2007; Taylor et al., 2012; Eyring et al., 2016) have been the principal source of process-based climate and Earth system modeling outcomes for IPCC assessment reports (ARs; (IPCC, 2021). The vast majority of CMIP experiments have considered boundary conditions where concentrations of greenhouse gases are prescribed, in both the implementation of idealized simulations and future scenarios that inform climate policy (O'Neill et al., 2016; Arnell et al., 2004; van Vuuren et al., 2011; Gillett et al., 2016).
In the two most recent IPCC cycles, scenario experiments have been defined in terms of Representative Concentration Pathways, or RCPs (Moss et al., 2010), which define futures in terms of approximate end-of-century radiative forcing levels to provide a set of consistent scenarios to be used in climate research and to provide multiple model-informed climate impact assessments at different warming levels. In the CMIP6 version of ScenarioMIP, scenarios were defined in terms of Shared Socioeconomic Pathways (SSPs) representing broad socioeconomic background states combined with global mean end-of-century radiative forcing targets (O'Neill et al., 2016; Riahi et al., 2017). IPCC AR6 (IPCC, 2021) adopted the notation of SSPX-Y, where X is one of five SSPs and Y is the radiative forcing level used in the creation of scenarios for ScenarioMIP.
The SSP design is concentration driven, with scenarios defined by their climate response. For example, SSP1-2.6 is a scenario that is designed to achieve a radiative forcing of 2.6 W m−2 in 2100. This is achieved by linking the integrated assessment model (IAM) with a simple climate model (SCM) to solve for a desired climate outcome (Riahi et al., 2017). To meet the predefined climate target, the IAM–SCM integration is iteratively solved with either carbon emissions constraints or carbon price trajectories until the climate target is met with sufficient accuracy (Calvin et al., 2019; van Vuuren et al., 2015; Baumstark et al., 2021). For the SSP design, all IAMs used the same simple climate model (MAGICC6.8) to ensure they reached the same forcing level in 2100 (Riahi et al., 2017). In the CMIP pipeline, the resulting emissions from each IAM SSP scenario are harmonized to a common historical dataset, any missing emissions are infilled (Kikstra et al., 2022; Gidden et al., 2019) and then multi-gas concentration pathways are estimated by a common SCM (Meinshausen et al., 2020) to be used as boundary conditions for ESM simulations in future scenario projections, together with pre-computed spatial information on land use and aerosol emissions (Feng et al., 2020; Hurtt et al., 2020).
Like the CMIP5-era RCPs that predated them (Moss et al., 2010), the SSPs use concentrations as a definitional anchor point. In this framework, Earth system uncertainties as a function of concentrations are estimated by climate models (in practice, by the CMIP ensemble; Fig. 1). This has pragmatic advantages in terms of coordinating research across climate disciplines but excludes uncertainties arising from feedbacks from the carbon cycle back onto atmospheric CO2. The concentration-based framework has no structurally consistent mechanism for representing these uncertainties in a process-resolving fashion – the IPCC AR6 working group 1 (WG1) report relied on emulators that were informed indirectly by CMIP models, where climate and carbon uncertainties were independently calibrated (see cross-chapter box 7.1 in Forster et al., 2023). In some cases, climate assessments bypass the causality chain and express impacts as a function of global mean temperatures (Fig. 1 and cross-chapter box 7.1 in Forster et al., 2023).
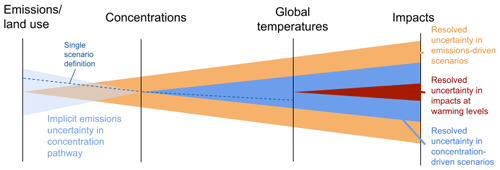
Figure 1A conceptual illustration (in the style of Pfleiderer et al., 2023) of the propagation of uncertainty using concentration- and emissions-based anchor points.
To date, CMIP phases have primarily represented anthropogenic emissions as a residual in concentration-driven simulations (Friedlingstein et al., 2006; Jones et al., 2016), thereby computing compatible carbon emissions consistent with the prescribed concentrations. This is achieved by assessing the residual flux of carbon that would be necessary to balance the internal carbon budget of an ESM simulation that is run in concentration-driven mode. However, in scenarios there are often significant differences between the carbon cycle representations in the original IAM structure and the ESM, such that the compatible emissions are conceptually distinct from the original scenario design (Koven et al., 2022, and Fig. 2). For ambitious mitigation scenarios such as SSP1-1.9, these differences account for a significant variation in the total cumulative emissions consistent with the prescribed concentration pathway (post-2014 cumulative emissions before net zero in SSP1-1.9 range from 200 to 280 GtC; see Fig. A1). As the scenario literature increasingly focuses on mitigation strategy relevant to the Paris Agreement (Rogelj et al., 2019; Sognnaes et al., 2021), it becomes increasingly necessary for ESM simulations to accurately represent both historical emissions and the outcomes of emissions scenarios that are consistent with the socioeconomic trajectories they are meant to represent.
A second issue with compatible emissions is the model-dependent ambiguity in their computation. Because compatible emissions are computed as a residual after accounting for carbon in the land surface, ocean and atmosphere, it is necessary that all models output the needed fields to account for the complete carbon budget. However, CMIP6 models remain inconsistent in their outputting, unit conventions and definitions of component-level carbon fluxes, which complicate analysis. Such issues must be better addressed in emissions-driven simulations where reconstruction of the carbon budget is of first-order importance to understanding the model response. In addition, there is inconsistency in the carbon pools and land use processes represented in different models – confusing the interpretation of the compatible emissions (Liddicoat et al., 2021). Furthermore, compatible emissions can only diagnose the fossil fuel component (Jones et al., 2013). This meant, for example, that IPCC AR6 had to mix ESM output for diagnosed fossil fuel emissions and IAM-based scenario data on land use emissions in creating synthesis figures such as WG1 Fig. SPM.7.
In addition, ESMs calculate land use, land use change and forestry (LULUCF) emissions dynamically based on the changing land use patterns that can markedly differ from the original LULUCF fluxes computed in IAMs (Quesada et al., 2018; Wilkenskjeld et al., 2014), and these differences are manifested in the compatible emissions that, in theory, should represent fossil fuel emissions. This also means that compatible emissions calculated in SCMs are not comparable with ESM estimates because aggregate LULUCF emissions are exogenously prescribed in most SCMs – creating discrepancies between SCM and ESM estimates of remaining carbon budgets for given warming levels (Millar et al., 2017).
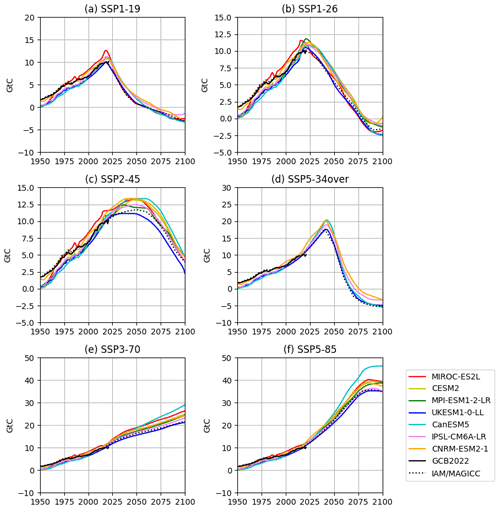
Figure 2Compatible fossil emissions for a range of scenarios and Earth system models in CMIP6, showing MAGICC-calculated CO2 emissions from IAM scenarios (Meinshausen et al., 2020; dotted black lines) and the compatible fossil emissions in CMIP6 ScenarioMIP simulations (colored lines). Historical fossil emissions from the global carbon project (GCB2022; Friedlingstein et al., 2022b) are shown for context.
Assessing compatible emissions for CMIP6 scenarios underlines that there are significant differences in the simulated compatible emissions amongst ESMs (Fig. 2). For example, in the concentration-driven SSP1-2.6 scenario in CMIP6, ESM-simulated net-zero dates measured in terms of compatible fossil fuel emissions ranged from 2076 to 2086, compared with the IAM estimate of 2076 (Gidden et al., 2019; van Vuuren et al., 2017; Fig. 2). The fact that the IAM/MAGICC trajectory lies on the edge of the ESM-compatible emissions distribution is worthy of further consideration, indicating that MAGICC carbon–climate dynamics are either a slight outlier amongst the ESMs or a methodological difference between the compatible emissions in the ESMs and the harmonized emissions trajectory produced in the IAM/MAGICC pipeline (Meinshausen et al., 2020). Differences between compatible emissions are also evident in the historical period, slightly exceeding the historical uncertainty in emissions. For example, 2014 compatible carbon fossil fuel emissions span from 9.1 to 10.9 GtC in CMIP6 concentration-driven models (Fig. 2), compared with the historical estimate of 9.7 ± 0.5 GtC (Friedlingstein et al., 2022b).
The only emissions-driven scenarios in CMIP6 took place as part of C4MIP (Jones et al., 2016), repeating high-emissions scenarios (esm-SSP5-8.5) and an extreme overshoot scenario (esm-SSP5-3.4-over) with a small subset of models. Notably, these scenarios were chosen to inform assessments of carbon feedbacks under high emissions (but they are not themselves considered to represent realistic near-term futures; Hausfather and Peters, 2020b). As a result, multi-model ESM results from the CMIP6 scenario effort as presented in IPCC-AR6-WG1 (e.g., AR6-WG1 Fig. 4.11) exclude an assessment of carbon cycle uncertainty (Tebaldi et al., 2021; IPCC 2021 WG1 Chap. 4). Where carbon–climate feedbacks were included in IPCC consideration of SSP projections (e.g., AR6-WG1 Fig. 4.35), this was achieved by probabilistic SCM ensembles informed by idealized ESM experiments to inform carbon feedback parameter uncertainty (Arora et al., 2020; Masson-Delmotte et al., 2023).
From this perspective, we argue that the increasing sophistication and stability of emissions-driven model configurations relevant for modeling greenhouse gas cycles mean that this approach can now be reassessed. The urgent need for process-based information on the mitigation effectiveness of fossil fuel emission reductions, carbon dioxide removal and land use policies requires a framework for the increased inclusion of emissions-driven experiments in upcoming CMIP cycles in the presence of heterogeneous model complexity, timeline constraints and technological challenges.
These dimensions increasingly dominate many of the most pressing questions in climate policy, and process-resolving ESMs are in a unique position to provide self-consistent assessments of climate policies that have regional, temporal and species dimensions. Constructing scenarios that fully explore these dimensions requires scenario definitions that go beyond the end-of-century forcing or temperature level implied in a concentration pathway. Rather, mitigation strategy needs to be defined in terms of activity and consequence: where human activities include fossil fuel and other industrial emissions, combined with regionally resolved descriptions of land use change and management.
The hybrid approach proposed in this study considers a set of headline experiments in CMIP7 that are preferentially driven by carbon and aerosol emissions, with prescribed values for other atmospheric components. And, for those models capable, dedicated activities to assess process-resolving carbon removal activities, along with the coupled dynamical response of the Earth system to non-CO2 gases such as N2O and CH4, would provide critical groundwork for their eventual representation in following CMIP activities.
Climate policy is framed in terms of emissions – naturally focusing on the elements that can inform mitigation decisions, such as emission benchmarks, carbon budgets and the timing of net zero. In addition, emissions-driven climate metrics (Arora et al., 2020) such as the transient climate response to cumulative emissions of carbon dioxide (TCRE; Allen et al., 2009; Jones and Friedlingstein, 2020; Matthews et al., 2009) and the zero emissions commitment (ZEC; Jones et al., 2019) are important and policy-relevant summary quantifications of the Earth system response to climate mitigation efforts. As of today, countries have committed to achieving climate targets, including net-zero targets under the Paris Agreement that constrain the future emissions space. Consistency of simulations with policy constraints is key to providing policy-relevant information.
However, the dominance of concentration-driven scenarios means that CMIP6 does not contain self-consistent simulations of mitigation strategy and their climate outcome in Earth system models. As a result, although IAM simulations already frame scenarios in terms of emissions pathways (Sognnaes et al., 2021), the simplified internal representation of climate and carbon processes does not allow for a comprehensive assessment of the underlying carbon cycle uncertainties associated with the scenario tradeoffs, generally relying on simple climate models to represent uncertainty in carbon–climate feedbacks (Nauels et al., 2017; Bodman et al., 2016; Damon Matthews et al., 2021; Watson-Parris and Smith, 2022), where idealized ESM results may be indirectly used in the calibration of the simple climate model parameter distributions.
Simple climate models are well-suited to this application – with sufficient structural complexity to emulate more complex models – but sufficiently computationally lightweight to allow rapid sampling of a relatively low parameter space to find model variants that are consistent with observations (Smith et al., 2024; Meinshausen et al., 2011). The increasing use of simple climate models in assessment (Nicholls et al., 2022) as the primary mechanism for representing uncertainty in global-scale climate response allows Earth system model simulations in CMIP to focus on coupled complex process representation. A CMIP ensemble with a primary focus on emissions-driven scenarios, starting with CO2 emissions in CMIP7 but with a longer-term objective to represent human activity through diverse emissions or land management, would allow ESM scenarios to represent real-world climate policy and its outcomes. As emissions and activity-driven processes are improved in ESMs, it is essential that SCMs can emulate any new emergent global coupled dynamics that arise in the ESMs (e.g., nonlinear behavior or tipping points). In short, the presence of a larger model ecosystem including ESMs, SCMs and kilometer-scale models allows for each model class to excel in dimensions that are suited to the platform. For ESMs, the computational efficiency and resolution must balance the need to represent coupled complex processes with the need to be able to calibrate and spin up the coupled system.
2.1 Key science needs for emissions-driven models
This emission-driven CMIP7 strategy would enable four key scientific benefits, which we outline in this section: (1) process-resolved assessment of carbon removal assumptions that underpin the capacity for climate temperature overshoot; (2) tradeoffs between fossil fuel emissions, carbon removals, land use change and short-lived climate forcers on regional scales, including relevant feedbacks; (3) integrated process resolution of system thresholds, nonlinearities, and risks that might exacerbate climate impacts and modify Earth system feedbacks in warmer climates; and (4) relevant simulations to inform the verification of mitigation activity.
2.1.1 Activity-driven representation of carbon removal
The plausibility and effectiveness of the gigaton-scale carbon dioxide removal implied by medium- to high-mitigation scenarios are key uncertainties (Marcucci et al., 2019) for end-of-century warming outcomes, given that the majority of the world's economy has pledged net-zero CO2 or GHG targets that are themselves conditional on significant amounts of carbon dioxide removal (Grant et al., 2021). Increasingly, this assumed feasibility of net global removal of carbon extends to climate overshoot pathways, where the temperature limits of the Paris Agreement are temporarily exceeded. High-level communication of climate science often frames the possibility of a temperature overshoot as a given; for example headline statement B.7 of the IPCC AR6 synthesis report presents the option of temperature overshoot in certain terms: “If warming exceeds a specified level such as 1.5 °C, it could gradually be reduced again by achieving and sustaining net negative global CO2 emissions.”
The plausibility of large-scale carbon dioxide removal (CDR) is subject to both geophysical and technological uncertainties, which vary by method but are not captured in the current IAM and ESM modeling framework. For example, large-scale bioenergy production for bioenergy with carbon capture and sequestration (BECCS) would have potential biophysical and biogeochemical feedbacks on the climate system that are not currently represented by the IAM simple climate models used to define scenarios (Koch et al., 2021; Luyssaert et al., 2018; Melnikova et al., 2023).
For land-based CDR approaches, the carbon sinks assumed within IAMs for a given land use transition are themselves subject to climate-induced risks due to warming (drought, wildfire or insect outbreaks; Anderegg et al., 2022; McDowell and Allen, 2015; McDowell et al., 2020), which are not taken into account in IAM scenarios that rely on approaches such as BECCS for large-scale carbon removal (Kato and Yamagata, 2014; Muri, 2018). In addition, carbon sink strengths themselves respond dynamically to emissions and removals of gases through carbon concentrations, aerosol forcing and surface ozone (Sonntag et al., 2018; Mengis et al., 2019; O'Sullivan et al., 2021; Zhang et al., 2021) – dynamics that can only be represented in an emissions-driven, process-resolving model structure. Ocean-based CDR suggestions such as alkalinity enhancement (Fakhraee et al., 2023; Hartmann et al., 2023) or iron fertilization (Emerson, 2019) are also dependent on the wider climate state and can have significant non-local effects on the wider biosphere (Keller et al., 2014).
We illustrate in Fig. 3 the scale of these potential uncertainties in the feasibility of land-based CDR capacity using a pair of scenarios from CMIP6: the highest-emission member of the ScenarioMIP ensemble (SSP5-8.5) and the extreme overshoot scenario SSP5-3.4-overshoot (Kriegler et al., 2017; Riahi et al., 2017), which assumes that a significant amount of BECCS is deployed in the latter half of the 21st century (with bioenergy crop production of 9 PgC yr−1 by 2100). In CMIP6 ScenarioMIP, both SSP5-8.5 and SSP5-3.4-over input datasets for CMIP were processed by the REMIND-MAGPIE IAM, but experiments were also mirrored in other IAMs. Figure 3a illustrates that the IAMs are more in agreement on the carbon content of current total harvest, but they differ in future projections under the SSP5-3.4-over scenario. Only a small subset of models conducted this simulation in CMIP6, but they are in significant disagreement about the current harvest level – highlighting a potential bias that would require further calibration if BECCS fluxes were calculated internally in ESMs.
We can get some idea of the ESM-simulated additional bioenergy production required for the BECCS-based carbon removal in SSP5-3.4-over by assessing the difference between total harvest in SSP5-8.5 and total harvest in SSP5-3.4-over (Fig. 3b).
The difference in harvest in REMIND-MAGPIE notably exceeds the difference between ESM-simulated harvest flux in SSP5-8.5 (where there is no deployed BECCS) and SSP5-3.4-over in all three of the models considered (difference between purple and red lines, Fig. 3), indicating that none of these models would be able to replicate the level of negative emissions assumed in REMIND-MAGPIE – despite being driven by land use transitions derived from that model. Notably, other IAMs also vary significantly in their assumed harvest fluxes (indicating a varying reliance on BECCS for carbon capture). Again, this highlights that if future climate simulations allowed BECCS fluxes to be calculated internally within the ESMs, there could be significant additional variance in the simulated forcing trajectory of large-overshoot scenarios.
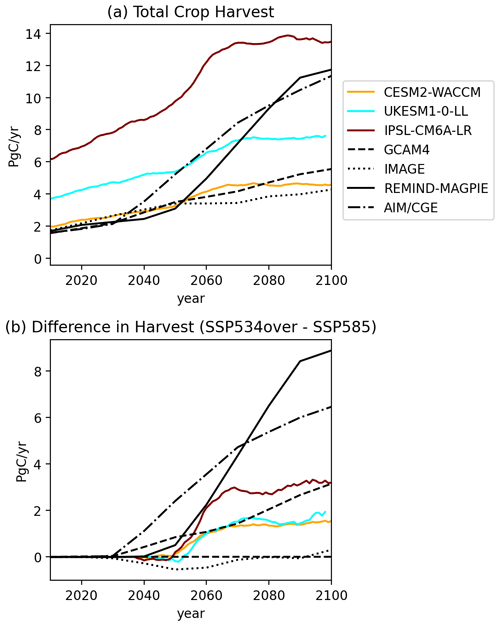
Figure 3(a) An illustration of total harvest carbon flux as simulated in the SSP5-3.4-overshoot scenario as simulated by the SSP5 marker model (REMIND-MAGPIE; solid black) and other integrated assessment models (dotted and dashed black lines) compared with estimates from three Earth system models (colored lines) that completed both simulations. (b) Colored lines show the simulated difference in ESMs (IAMs in black) between harvest carbon flux in SSP5-3.4-overshoot and harvest carbon flux in SSP5-8.5.
Issues related to the feasibility of CDR at scale are compounded by uncertainties in the response of the Earth system to extended periods of net-zero or net-negative emissions. Much of our current understanding stems from highly idealized ESM experiments that have been conducted by only a subset of models (Jones et al., 2019; Keller et al., 2018). Such experiments show that the Earth system response to net-negative emissions is complex and likely asymmetric, but the lack of extensive process-based ESM simulations of response to net-negative emissions leaves significant uncertainties in cases in which SCMs and emulators have not been extensively tested or validated. Such uncertainties have bearing on the feasibility of a temperature overshoot, in terms of both the level of mitigation needed to stabilize warming (Jenkins et al., 2022) and the relative timing of net zero and peak warming (Koven et al., 2023). As such, concentration-driven mitigation scenarios created through the existing modeling chain may assume land use and management carbon fluxes from the IAM that are impossible to achieve with the ESM (and perhaps in reality) due to ecophysiological limitations of vegetation in a changing climate. An activity-driven framework for removals would directly assess these risks associated with land-based carbon mitigation (such as through afforestation, reforestation, forest management, biochar, agricultural soils or BECCS) by providing a range of potential outcomes for the land- and ocean-based removal strategies employed in the scenario that can contextualize and provide uncertainty bounds for the climate trajectory simulated internally within the IAM.
An activity-driven framing is naturally suited to process representation of carbon dioxide removal methods (especially for those methods relying on the manipulation of natural systems that are to some degree resolved within Earth system models). Some of these (such as afforestation) are already represented within most ESMs, while others (BECCS, soil carbon enhancement, terrestrial and marine alkalinity enhancement, blue carbon enhancement) are represented to a lesser degree or not at all. A dedicated activity within CDRMIP could assess the effectiveness of different approaches in a semi-idealized context under different climate background states. Such an activity could aid in the interpretation of emissions-driven scenario simulations in CMIP7 and provide a pathway to the inclusion of a wider range of CDR technologies in CMIP8 and beyond.
2.1.2 Resolving compound tipping points and adaptation challenges as a function of emissions
The potential for nonlinearities and tipping points in the climate system is frequently raised as a motivator for urgent emissions cuts (Lenton et al., 2019) and is often framed in terms of temperature thresholds (for example, in discussion of whether rapid and irreversible changes might be triggered if 1.5 °C of warming above pre-industrial levels is exceeded; Armstrong McKay et al., 2022) – but introducing previously ignored nonlinearities can complicate how thresholds defined in terms of temperature map onto mitigation risks. Some of these previously discussed system thresholds have the potential to alter global-scale carbon–climate feedbacks and dynamics, e.g., cryosphere thresholds may be crossed (Kloenne et al., 2022), forests may be subject to dieback or changes in carbon sink efficacy (Chai et al., 2021), and increased stratification of the ocean may change its heat and carbon uptake dynamics (Bourgeois et al., 2022).
As such, tipping points and emissions are intricately tied together and Earth system models are natural tools for simulating how they might interact, with increasingly complete and sophisticated process resolution for ecosystem, cryosphere and ocean processes. Understanding how these nonlinearities combine and relate to a wider mitigation strategy requires the processes to be simulated in a self-consistent framework in the context of an emissions-driven mitigation scenario where carbon–climate feedbacks are interactively resolved.
This argument extends to adaptation planning, where ESM results from concentration-driven simulations are often currently framed in terms of expected impacts at given warming levels (Jevrejeva et al., 2018; Lwasa et al., 2018; Intergovernmental Panel on Climate Change, 2022; Travis et al., 2018) rather than impacts under given emissions pathways (Drouet et al., 2021; Wiebe et al., 2015). As such, adaptation planners have no simple means of assessing the range of plausible hazards consistent with a given level of climate policy. Emissions-driven simulations could help fill this gap, while still allowing impacts to be framed in terms of warming levels as they are with existing ensembles.
2.1.3 Better assessment of ocean acidification
The IPCC AR6 WG1 report highlighted the limitations of concentration-driven experiments in CMIP6 for projecting future ocean acidification (IPCC, 2021). Inter-model variance in surface pH is very low in a given scenario (Lovenduski et al., 2016), largely because all ocean models experience identical surface CO2 concentrations (Kwiatkowski et al., 2020). Emissions-driven simulations would represent the full joint dynamics of ocean and atmosphere heat and carbon evolution (Terhaar et al., 2023). Such factors would represent an improvement in the categorization of uncertainty in any Earth system processes that are directly or indirectly dependent on atmospheric CO2 concentrations.
2.1.4 Diagnosis of land use emissions
Significant uncertainty remains in both the simulation and the assessment of observed emissions due to land use change (Friedlingstein et al., 2022b). In concentration-driven simulations in CMIP6, land use emissions were calculated internally in each model and were consequential in terms of derived compatible fossil emissions (Liddicoat et al., 2021), and land use emissions are assessed independently in the Land Use Model Intercomparison Project (LUMIP; Lawrence et al., 2016). However, significant uncertainty remains in the definition and quantification of land use fluxes. In the Global Carbon Budget (Friedlingstein et al., 2022b), for example, best estimates of land use emissions are derived from bookkeeping models (Hansis et al., 2015; Houghton and Nassikas, 2017; Quilcaille et al., 2023), which use empirical growth curves to estimate the transient carbon stock response to land use changes. Meanwhile, national inventories use different accounting conventions to those used in IAMS, ESMs and bookkeeping models – including not just transitions in land use but also land sinks in some regions whose usage remains static but are designated as managed (Gidden et al., 2023; Grassi et al., 2021).
2.1.5 Verification of emissions reductions
The 2028 global stocktake will be the next major global assessment of progress towards Paris Agreement goals. This requires increasing understanding of how to quantify and verify national emissions reductions. Existing approaches for the detection and attribution of observed climate changes to different historical anthropogenic activities rely predominantly on models in concentration-driven mode (Hegerl and Zwiers, 2011). However, with increasing focus on mitigation activity and the verification of reductions in terms of climatic variables (such as greenhouse gas concentrations, temperatures or heat uptake; Peters et al., 2017), it makes sense to consider the detection problem in terms of emissions – when can the benefits of mitigation activity be observed?
As climate mitigation ambition ramps up, there is a growing expectation that emissions will change their recent historical trend, initially with slower growth, then a peak followed by a decline. Already, global CO2 emissions have slowed from 3 % yr−1 growth in the 2000s to 1 % yr−1 growth in the 2010s (Friedlingstein et al., 2022a). An increasingly relevant question will then be to what degree any reductions will be detectable in terms of observed climate variables and near-term warming (McKenna et al., 2020; Samset et al., 2022) and potentially in terms of climate impacts themselves (Mendez and Farazmand, 2021; Ciavarella et al., 2017). These questions are of relevance for the justification of climate policy both globally and at the country level; for planning for potential near-term impacts; and for assessments of liability for climate damages.
Modeling to support such activity requires a joint assessment of the land, ocean and atmospheric carbon pool, and human activity in a self-consistent framework (Ilyina et al., 2021). Land sinks are of particular relevance in the context of the global stocktake process, which assesses national-level progress in the context of meeting obligations under the Paris Agreement. In this process, many countries offset a fraction of their emissions using managed land within their borders, which is currently assessed to act as a carbon sink (Grassi et al., 2021). Understanding the robustness of these sinks in present and future divergent climates is thus critical for assessing the degree to which countries can rely on such sinks to substitute for emissions reductions on different timescales (Giebink et al., 2022).
In the atmosphere, efforts to detect emissions reduction from globally averaged atmospheric concentrations have not yet succeeded. It was expected that a 2 % change in the growth rate of CO2 emissions could be detected in the atmosphere with reasonable confidence after about 10 years (Peters et al., 2017). A possible explanation for the lack of signal is our inability to fully model and explain the inter-annual variability in climate–carbon feedbacks, which could be offsetting a part of the expected change in trend (Spring et al., 2020). In the years ahead, when emissions are hopefully declining, there will be a need to understand how the carbon cycle may respond to carbon–climate feedbacks potentially offsetting some of the expected declines in the atmospheric growth rate. Such experiments have to date been idealized (Keller et al., 2018; Jones et al., 2019), but a need for integrated simulation to explore the interaction of natural carbon feedbacks with process-resolving CDR and non-CO2 emission pathways remains.
To date, attempts to verify emissions reductions as a function of atmospheric concentrations have been conducted in simple climate models (Abdulla et al., 2023), by adjustments computed from compatible emissions in Earth system models (Spring et al., 2020) or using atmospheric inversion models to compute emissions consistent with prescribed concentrations (Deng et al., 2022). These estimates would be well-supported by fully self-consistent internally generated representations of the chain of causality from emissions to concentrations, which could be achieved in emissions-driven ESM simulations.
Such questions could be addressed in the Detection and Attribution Model Intercomparison Project (DAMIP; Gillett et al., 2016) or through other activities using a combination of idealized and realistic simulations: (1) idealized experiments where CO2 emissions are reduced at a fixed rate to detect the timing of signal emergence and (2) emissions-driven single-forcer experiments to assess the detectability and linearity of the historical climate response to different anthropogenic emissions. As such, hybrid emissions-driven simulations would provide a critical complement to existing verification efforts, potentially including counterfactual scenarios that could illustrate when mitigation policy implementation becomes detectable in terms of atmospheric concentrations or climate impacts (Tebaldi and Friedlingstein, 2013).
Past CMIP phases designed experiments to exploit the existing modeling capacity in major Earth system modeling centers at the time of experimental design, motivated by dominant uncertainties and pilot studies in the literature (Meehl et al., 2007; Taylor et al., 2012; Eyring et al., 2016). Early climate simulations used atmospheric-only models to diagnose radiative feedbacks (Cess et al., 1989). CMIP2-era coupled experiments generally exploited radiative flux corrections to maintain a stable ocean temperature (Covey et al., 2003), and a parallel Atmospheric Model Intercomparison Project (AMIP) process remained to understand atmospheric feedbacks without the added complexities of ocean coupling (Lawrence Gates et al., 1999). The presence of an intercomparison project fostered rapid improvements in coupled simulation such that by the time of the CMIP3 ensemble (Meehl et al., 2007), there was increasing acceptance that resolving coupled ocean–atmosphere processes was key to understanding climate projections (Frame et al., 2006), and models were rapidly advancing so that they could maintain stable climates without flux corrections.
Over the last 20 years, the scope of process resolution in climate models has further expanded (Fig. 3), and the increasing complexity of both atmospheric chemistry and aerosol treatment has increased the degree to which some emissions are already represented in many climate models and interact with climate feedbacks (Thornhill et al., 2021). The evolution of aerosol treatment from CMIP3 to CMIP5 to CMIP6 has seen a nonuniform tendency for models to represent aerosol indirect effects on clouds and emissions-driven aerosol processes (interactive treatment of aerosols has been included in some fraction of Earth system models since CMIP5; Eyring et al., 2016, and stratospheric aerosols have been included since CMIP3; Meehl et al., 2007). CMIP6 in particular (Eyring et al., 2016) introduced a tiered experimental design that accommodated models with varying levels of aerosol and atmospheric chemistry implementation in scenario experiments, supported by dedicated sub-MIPs to assess processes (in AerChemMIP) and effects of different forcers (in RFMIP).
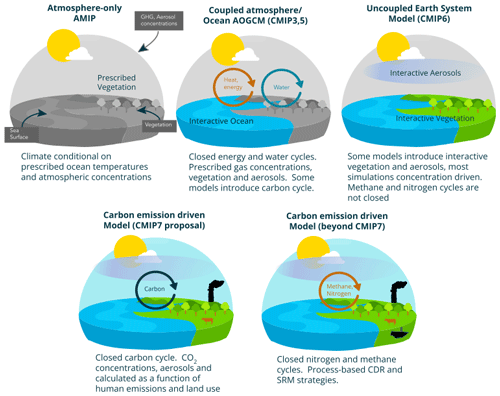
Figure 4The evolving dominant paradigm in different generations of CMIP, including this study's recommendations for CMIP7 and CMIP8.
Past phases of CMIP have defaulted to concentration-driven scenarios, but models capable of running with a closed and interactive carbon cycle have been developed by some centers for over 2 decades (Cox et al., 2000; Joos et al., 1999; Fung et al., 2005), with intercomparison efforts for coupled carbon Earth system models coming soon after (Friedlingstein et al., 2006; Jones, 2020). These early studies established the significance of coupled carbon–climate processes in the wider evolution of the Earth system, with potential interactions between carbon balance and ocean circulation (Joos et al., 1999), feedbacks with the terrestrial biosphere (Cox et al., 2000), and weakening carbon sinks at higher warming levels (Fung et al., 2005).
However, despite increasing acknowledgment of the central role of coupled climate–carbon dynamics in determining the outcome of mitigation policies (Allen et al., 2009; Holden et al., 2018), only 19 out of 82 CMIP6 model configurations participated in the Coupled Climate–Carbon Cycle Model Intercomparison Project (C4MIP) in CMIP6 (Jones et al., 2016), although these models vary in resolved processes (12 resolving carbon–climate interactive feedbacks, 5 resolving phytoplankton biophysical interactions, 3 resolving biogenic aerosol–cloud feedbacks and no models representing non-CO2 biogeochemical cycle feedbacks; Séférian et al., 2020).
Nonetheless, hybrid emissions-driven experiments in the central Diagnostic, Evaluation and Characterization of Kilma (DECK)/historical part of CMIP6 were limited to esm-historical and esm-picontrol (Eyring et al., 2016). Further, the DECK required independent picControl and esm-piControl simulations from an ESM and highlighted the importance of large-ensemble sampling for the historical simulation. In practice, models that conducted the ESM historical simulation esm-hist did so generally without initial condition sampling – presenting an obstacle for the assessment of the role of internal variability in carbon cycle feedbacks and for signal emergence of coupled Earth system processes (Li and Ilyina, 2018) and near-term initialized climate prediction systems (Li et al., 2023a), which enable near-term prediction of atmospheric CO2 concentrations as well as air–sea and air–land carbon fluxes.
The limited ESM–DECK experiments in CMIP6 were supported by process understanding from idealized carbon cycle feedback experiments, including the globally aggregated effects of idealized carbon dioxide removal in CDRMIP (Keller et al., 2018), metrics of carbon cycle feedbacks in C4MIP (Jones et al., 2016) and ZECMIP (Jones et al., 2019), and the physical and carbon effects of land use change in LUMIP (Lawrence et al., 2016) and LS3MIP (van den Hurk et al., 2016). Although C4MIP included some hybrid emissions-driven scenarios (esm-ssp585 and esm-ssp534-over), these represent very large near-term emissions that are distant from contemporary policy discussions (Hausfather and Peters, 2020a).
3.1 A coupled climate–carbon ESM representation for CMIP7
As such, we argue that in order to provide robust information for both adaptation and mitigation, it is equally important to sample inter-model uncertainties in the wider carbon–climate system. This requires a change in prioritization in the DECK, ScenarioMIP and elsewhere in CMIP, with default control, historical and projection simulations run in hybrid emissions-driven configuration, with concentration-driven options used as a fallback for models that cannot process emissions. Such a re-prioritization would enable modeling centers to more efficiently use resources to focus on Earth system uncertainties (including physical and carbon cycle elements) rather than splitting resources.
We argue that carbon–climate interactions and feedbacks are central to how the coupled Earth system will evolve in the future and therefore need to be central to CMIP activities going forwards rather than an optional extra. For CMIP7, this requires that carbon emissions and land-activity-driven simulations become the default for those models that are capable. ESMs in this configuration require the ability to process anthropogenic carbon emissions from fossil fuels and land use change and management in the context of a closed and stable carbon cycle, which represents oceanic and land-based sinks. For these models, CMIP7 historical and scenario experiments could be driven by fossil carbon emissions and land use transitions. For ESMs without the capacity or desire to run in a hybrid emissions-driven configuration, scenarios based on simple climate models could still be computed in the conventional ScenarioMIP structure, with the guidance that the concentration pathway represented within ScenarioMIP is only one potential outcome of climate policies in terms of emissions, atmospheric concentrations, and climate and carbon cycle responses. Alternatively, non-ESM atmosphere–ocean general circulation models (AOGCMs) could be driven by small ensembles of plausible concentration pathways, sampling a range of plausible carbon cycle uncertainty.
Participation in CMIP by models with heterogeneous complexity is not unprecedented. In CMIP5 (Taylor et al., 2012) and CMIP6 (Eyring et al., 2016), only some models were capable of processing aerosol emissions (including aerosol–cloud interactions and feedbacks on natural aerosol emissions such as biomass burning, dust and sea spray), while those without interactive aerosol schemes were driven by predefined loadings (Stevens et al., 2017). In CMIP3 (Meehl et al., 2007), there was a similar coexistence between models with a thermodynamic slab ocean and those with a fully dynamic ocean (although slab oceans were abandoned in CMIP5). These periods of coexistence of model complexity proved a necessary and very successful compromise to allow this diversity on the path towards a successful transition to increased complexity across the CMIP ensemble. We argue that now is the right time for the next planned transition to emissions-driven modeling capability.
3.2 Coordinated effort on activity-driven carbon cycle modeling
The status quo that defined the default configurations in CMIP6 and earlier phases is now changing. Models can increasingly resolve vegetation and soil carbon dynamics including permafrost, as well as marine biogeochemical cycles. For many ESMs, the ability to represent these processes now exists, but relatively little work has been done thus far to comprehensively understand how this complexity impacts the trajectory of climate, especially under deep-mitigation scenarios, geoengineering proposals and overshoots.
ESMs can potentially add self-consistent process resolution to a wide range of carbon processes that are currently resolved in scenarios in an ad hoc and quasi-empirical fashion. ESMs are already well-placed to resolve natural land and ocean carbon sinks and are operationally used to quantify these terms today (Friedlingstein et al., 2022a). But in addition to this, they can directly inform the effectiveness and uncertainty associated with land use and management policy and inform their coupled interaction with natural sinks (Lawrence et al., 2016). Beyond this, many high-ambition scenarios contain significant requirements for explicit representation of carbon dioxide removal (Fuss et al., 2014; Anderson and Peters, 2016) whose plausibility can potentially be assessed when represented in an Earth system model (Muri, 2018). Increasing understanding of how to map between national accounting systems and ESM/IAM output (Gidden et al., 2023; Grassi et al., 2021) can be strengthened with hybrid emissions-driven simulations (combined with well-chosen counterfactual experiments in LUMIP), where ensembles can provide ranges of modeled direct and indirect anthropogenic fluxes from land use change.
A hybrid emissions-driven scenario framework would allow for the explicit representation of different forms of human activity associated with carbon mitigation, and much of this has already been demonstrated using subsets of ESMs. Carbon removal technologies (such as bioenergy carbon capture and storage) could largely use existing models combined with sub-annual harvest cycles, harvest age for woody biomass and a dedicated pool to represent underground carbon storage. Others, such as cultivation and harvesting of oceanic algae (Wu et al., 2023) or ocean alkalinity enhancement (Keller et al., 2014; Ilyina et al., 2013; Burt et al., 2021; González et al., 2018), could be represented with explicit parameterizations (Wu et al., 2023). And, as discussion of the ethics and risks of solar radiation management intensify (Reynolds, 2021; Sovacool, 2021), understanding the interaction between geoengineering and ecosystem processes is of paramount importance (Zarnetske et al., 2021), where coupled ESMs are essential in any comprehensive cost–benefit assessment (Sonntag et al., 2018).
Thus, although there is a large and growing body of work assessing mitigation strategy in the context of emission-driven models, much of this to date has been in the context of isolated ESM experiments that do not capture multi-model uncertainty (with the exception of the idealized adaptive mitigation pathways explored in Silvy et al., 2024). By adopting a hybrid emissions-driven design, CMIP7 could directly inform the coupled system risks associated with the range of carbon removal and geoengineering strategies, which increasingly play an outsized role in the mitigation debate.
3.3 Diagnostic simulations in the CMIP7 fast track
Here we discuss the likely implementation of emissions-driven simulations in CMIP7 at the time of writing. As in CMIP6, CMIP7 will contain a DECK, which (as in CMIP6) will request esm-picontrol as a starting point for emissions-driven simulations. Current plans for CMIP7 will also consider historical and esm-historical as part of the DECK (CMIP phase 7, CMIP7, 2024). In addition, the CMIP7 fast track is a set of high-priority experiments that will be recommended for completion in time to inform assessment reports for the IPCC AR7 cycle (CMIP phase 7, CMIP7, 2024); see Table 1. With a higher focus on emissions-driven experiments, we are recommending (and it is currently planned) that the fast track include both emissions-driven scenarios and diagnostic simulations that will help assess key aspects of emissions-driven response. These idealized carbon-emissions-driven experiments (which will be fully documented in a separate paper) will allow calculation of the key carbon–climate metrics needed to inform climate policy tools such as the IPCC remaining carbon budget for climate stabilization, thus complementing existing concentration-driven metrics. Figure 5 illustrates a proposal for a set of diagnostic emissions-driven experiments, which would provide emissions-driven estimates of the transient climate response to cumulative emissions (TCRE) and zero emissions commitment (ZEC) in the fast track.
Table 1Current plans for the implementation of emissions-driven simulations in the CMIP7 DECK and fast track.
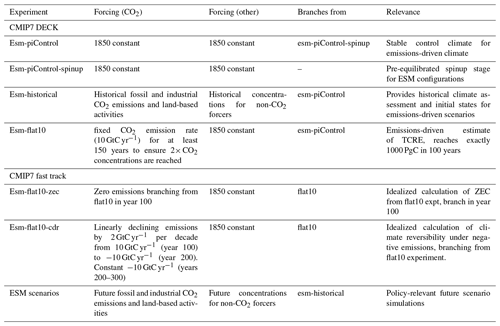
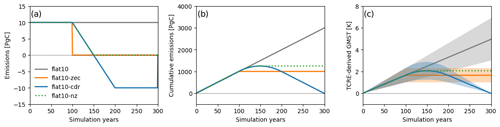
Figure 5Illustrations of recommended idealized diagnostic experiments (Table 1) for the CMIP7 fast track, showing (a) emissions, (b) cumulative emissions and (c) temperature as a function of time. The shaded spread in (c) is defined assuming perfect cumulative emissions and the IPCC AR6 assessed range of TCRE (Masson-Delmotte et al., 2023). Solid lines are recommendations for CMIP7 fast track; dashed lines are additional recommendations for C4MIP in CMIP7.
3.3.1 Esm-flat10 – diagnostic simulation for transient response
Esm-flat10 would consider a constant annual flux of 10 PgC of carbon for 100 years (such that the warming after 100 years would correspond to 1000 PgC of cumulative emissions – as such, a direct measure of TCRE). Unlike for 1pctCO2, compatible emissions do not need to be computed, and the TCRE can be easily calculated as a time average in the experiment, thus providing a clean experiment that can be branched to assess zero-emissions commitment and climate reversibility. Esm-flat10 as a default diagnostic for TCRE would have a number of desirable properties: (1) emissions are constant for all models considered (rather than varying by model under 1pctCO2 – see Fig. A1), (2) emissions are constant at approximately current rates throughout the simulation (rather than weighted towards the end of the simulation as in 1pctCO2) and (3) peak emission rates are more consistent with those of ambitious climate mitigation scenarios than the diagnosed peak emission rates in 1pctCO2 at the point of reaching 1000 PgC cumulative emissions are.
3.3.2 Esm-flat10-zec – diagnostic simulation for zero emissions commitment
We propose a completely emissions-driven alternative derivation for the zero emissions commitment: esm-flat10-zec. The zero emissions commitment is a measure of the path dependence of the temperature on the cumulative emissions relationship (Koven et al., 2023), an estimate of the subsequent global warming that would result after a period of anthropogenic emissions once they are set to zero (Jones et al., 2019; MacDougall et al., 2020). The Zero Emissions Commitment Model Intercomparison Project (ZECMIP; Jones et al., 2019) contains a number of experiments to quantify this behavior, most predominantly with the esm-1pct-brch-1000PgC experiment, which branched from the concentration-driven 1pctCO2 at the point at which 1000 PgC of cumulative emissions had been emitted. Esm-flat10-zec allows for computation of temperature changes after an immediate cessation of emissions, similar to the ZEC concept assessed in Jones et al. (2019).
Esm-flat10-zec would convey a number of both practical and theoretical advantages over 1pctCO2 as a primary diagnostic of zero emissions commitment. (1) The maximum rate of CO2 emissions in esm-flat10 (10 Pg C yr−1 vs. ∼ 20 Pg C yr−1 for 1pctCO2) is closer to the realistic values that are projected for ambitious policy scenarios, where emissions must peak and decline from their present values of ∼ 10 Pg C yr−1 within decades to achieve Paris Agreement-compatible warming targets. (2) Because the experiment is emissions-driven from the outset, it would not require a change in configuration at the branch point. (3) The branch point is identical for all models (unlike in esm-1pct-brch-1000PgC, where the year in which 1000 PgC of compatible cumulative emissions are exceeded must be calculated retrospectively to find the appropriate branch year). (4) This common experimental setup would allow the easier automation of ensembles in the calculation of both TCRE and ZEC, without needing to calculate compatible emissions to find the appropriate branch point.
3.3.3 Esm-flat10-cdr – diagnostic simulation for climate reversibility
An increasingly important feature of the discussion of future Paris Agreement-compatible pathways is an assessment of the reversibility of the climate system, both in a global sense (Zickfeld et al., 2013; Wu et al., 2015) and in terms of regional and subsystem responses (Armour et al., 2011; Martin et al., 2022). In CMIP6, a number of idealized experiments were conducted under the Carbon Dioxide Removal Model Intercomparison Project (CDRMIP; Keller et al., 2018), which included a concentration-driven extension of 1pctCO2 called 1pctCO2-cdr (see Fig. 8) that prescribed a 1 % ramp-down in concentrations at the point at which 1pctCO2 reached quadruple pre-industrial levels. This experiment undergoes a large discontinuity in compatible emissions at the transition from upwards to downwards branches, making it less useful as an indicator of realistic transitions to negative emissions (see Fig. 8; Koven et al., 2023).
Here we propose an emissions-driven extension to esm-flat10 to address this need: esm-flat10-cdr would serve as an emissions-driven idealized experiment to assess the dynamics of climate reversibility under decreasing emissions and net-negative emissions. The experiment would allow for a number of simple idealized diagnostics that would be relevant to the net-zero transition and the response of the system to net-negative emissions. Esm-flat10-cdr would branch from esm-flat10 in year 100 after 1000 PgC of emissions, ramping down emissions linearly over 100 years from +10 to −10 PgC yr−1 and then maintaining a negative flux of −10 PgC yr−1 for an additional 100 years.
This esm-flat10-cdr experiment would provide a number of advantages over 1pctCO2-cdr: (1) an emissions-driven metric of climate reversibility with a continuous emissions time series; (2) an idealized net-zero transition to measure the lags in the climate system in the decades around net zero as emissions pass from net positive to net negative; (3) characterization of asymmetries in the climate response relative to emissions rather than to concentrations, using a symmetric and continuous reversal from positive to negative CO2 emissions; and (4) initial emissions and a decarbonization rate that are comparable to an aggressive mitigation scenario. These features are all also present in the Gaussian cumulative emissions experiment described by Koven et al. (2023), which also features an asymptotic rise in emissions at the start of the industrial period and an asymptotic tapering of negative emissions to zero as cumulative net-zero emissions are achieved. The key advantage of esm-flat10-cdr over esm-restoration for an ESM-DECK is that it allows computational savings by re-using the first common 100 years of esm-flat10 and esm-flat10-zec to form a coherent set of interrelated experiments and metrics.
A final experiment – not recommended for the fast track but for possible inclusion in a CMIP7 satellite MIP such as C4MIP – would be esm-flat10-nz, branching in year 150 from esm-flat10-cdr, allowing an assessment of zero-emissions response under an idealized gradual decline from current emissions rates to net zero. This experiment would provide a companion experiment to esm-flat10-zec, assessing how the zero-emissions response differs between an instantaneous cessation and a gradual approach to net zero (Koven et al., 2023).
3.4 IAMs and scenario development
Emissions-driven simulations to date in CMIP have been highly idealized (e.g., ZECMIP; Jones et al., 2019). An emissions-driven focus allows coupled system processes to be represented in policy-relevant scenarios, but this requires a refinement in the way that scenarios have traditionally been framed and categorized (O'Neill et al., 2016). In hybrid emissions-driven mode, ESM-simulated concentrations, radiative forcing and temperature will differ from that in the scenario definition (currently harmonized SCM simulations combining historical climatic trends and IAM driver data; Fig. 6c). Furthermore, the ability to simulate different types of carbon removal processes and non-CO2 mitigation strategies within the ESM opens the door to having multiple scenarios with comparable best-estimate temperature outcomes in the IAM but with different uncertainty ranges simulated in the ESM ensemble. As such, the naming strategy for emissions-driven scenarios will ultimately need to represent a higher-dimensional space, providing a shorthand for embedded characteristics on decarbonization rate, removal strategy and non-CO2 emissions. This may be more easily achieved with qualitative identifiers than with continuous labels referring to radiative forcing or temperature targets.
In practice, the policy strategies implemented internally in IAMs would still be informed by a climate outcome (e.g., Paris Agreement-compliant scenarios), perhaps assessed using a simple climate model – but process uncertainties represented within the downstream ESM ensemble simulation may illustrate that some policies targeted at a given warming level are more robust than others (e.g., scenarios that rely heavily on afforestation, which may or may not achieve the desired carbon outcomes in all ESMs) or may have different negative impacts on other aspects of the global environment (e.g., air quality or food production capacity).
It is notable that some IAMs already contain process-based land surface models to inform land use emissions estimates (Stevanović et al., 2016). A key distinction in the hybrid emissions-driven framework would be that land use transitions (in addition to fossil CO2 emissions) are provided by the IAM system – allowing a diversity of land use emissions to be simulated in the ESM ensemble (rather than the status quo where a single set of land use emissions is computed by the IAM), thus modeling the uncertainty in climate implications of land use transitions.
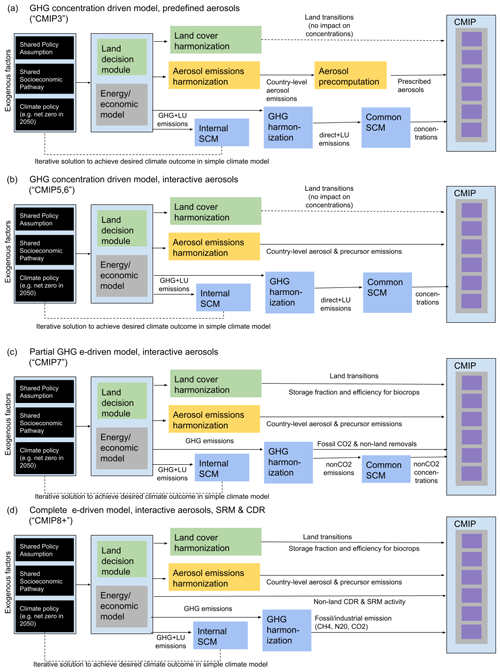
Figure 6Stylized illustrations of the historical (a, b) and proposed (c, d) information flow for CMIP. Panel (a) shows the concentration-driven modeling pipeline with prescribed aerosols common in CMIP3; panel (b) shows the concentration-driven modeling pipeline with interactive aerosols common in CMIP5 and 6; panel (c) is a proposed scenario pipeline for hybrid emissions-driven simulations in CMIP7 with carbon emissions but maintaining concentration definitions for non-CO2 greenhouse gases; and panel (d) is a proposed CMIP8 pipeline with an emissions-driven configuration for CO2, N2O and CH4 and a process-based implementation of CDR and SRM approaches.
4.1 Coupled system biases
A challenge associated with running models in hybrid emissions-driven mode is the additional degrees of freedom associated with calibrating the coupled climate–carbon cycle system to reproduce both the joint evolution in historical concentrations of climate forcers and the historical warming increases. CMIP6 esm-historical simulations show most models (10 out of 13 models in C4MIP) fall within a CO2 concentration range of 40 ppm – representing some 20 years of historical emissions. This is significantly greater than the observational uncertainty (about 0.1 ppm, Friedlingstein et al., 2022a; Lee et al., 2021), WG1 Chap. 4), and we suggest that the remaining outlier models may require greater attention to calibration of historical CO2 concentrations if emissions-driven simulations are the only runs provided. This concentration uncertainty in the present day, however, is likely significantly smaller than the future uncertainty in CO2 concentrations. This is evident from the significantly greater future spread in CO2 concentrations in those models that conducted esm-ssp585 in CMIP6 (200 ppm in 2100 compared with 20 ppm in 2014; Loughran et al., 2023; Masson-Delmotte et al., 2023). This is also supported by the growing spread in cumulative compatible emissions in different Earth system models, with a multi-model range of 50 GtC of cumulative emissions in 2020 compared with 100 GtC in 2100 under ssp119 and a 280 GtC range in 2100 in ssp245 (Fig. A1). As such, the present-day concentration uncertainty is not trivial but is small compared with the future spread in concentrations that arise from the carbon–climate feedback uncertainty.
The spread in present-day concentrations results in a modest increase in the model uncertainty in warming represented by the distribution of historical warming in CMIP6 simulations and their concentration-driven historical analogs for models that completed both experiments (Fig. 7b, c) – and an interquartile range of 0.45 °C for warming in 2005–2014 relative to an 1850–1900 baseline in esm-historical compared with 0.25 °C in the concentration-driven historical experiment. Notably, using a more recent baseline period (1970–1990), the hot model issue of overestimated recent warming (Hausfather et al., 2022) is apparent when considering the concentration-driven historical recent distribution in the context of observations (Fig. 7d), but the higher variance of recent warming in the emissions-driven simulations results in the observed warming lying within the interquartile range of simulated warming. Having only coupled simulations available would likely increase the difficulty of isolating the sources of bias in simulations (i.e., isolating biases in the ecosystem and physical systems). As such, fully coupled simulations would be complemented well by concentration-driven simulations if sufficient computational time is available to assess the role of coupled processes in model bias.
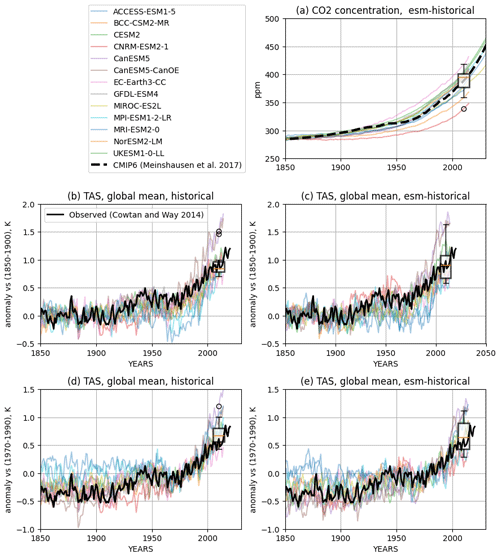
Figure 7Carbon dioxide concentrations (a) and temperature anomalies (c, e) in emissions-driven historical simulations in CMIP6 and temperature anomalies in concentration-driven historical simulations (b, d). The middle and bottom rows are relative to an 1850–1900 and 1970–1990 baseline, respectively. Observed temperature data are from Cowtan and Way (2014). Boxplots show the interquartile range (boxes) and 10–90 percentiles (whiskers) of the model-simulated anomalies for the period 2005–2014.
4.2 Implications for wider MIPs in hybrid emissions-driven simulations
The hybrid approach proposed in this study considers a set of headline experiments in CMIP7 that are preferentially driven by carbon and aerosol emissions, with prescribed values for other atmospheric components. Such an approach would be supported by continued activities in the Radiative Forcing Model Intercomparison Project (RFMIP; Pincus et al., 2016), to provide diagnostics of global aerosol emissions forcing–feedback dynamics, but also in AerChemMIP (Collins et al., 2017), which in CMIP6 assessed the role of aerosol forcing process uncertainty in future simulations.
Some activities that did not exist under the CMIP6 platform could be highly valuable in the increased understanding of emissions-driven processes. A dedicated activity to assess the role of regional aerosol emissions in this uncertainty (Wilcox et al., 2023) would address the growing consensus that shifts in regional emissions intensity have a large and detectable climatic impact (Samset et al., 2019; Liu et al., 2018). Aerosol processes can also be intricately linked with carbon uptake (O'Sullivan et al., 2021; Zhang et al., 2021), impacting both the interpretation of past carbon cycle evolution and future carbon uptake in areas with large aerosol concentrations/surface ozone (e.g., southern Asia/Africa). And, for those models capable, dedicated activities to assess the coupled dynamical response of the Earth system to non-CO2 gases such as N2O and CH4 would provide critical groundwork for their eventual representation in following CMIP activities.
Attribution studies in DAMIP (Gillett et al., 2016) and in general rely on linking cause and effect, where the cause has historically been interpreted as the change in a climate forcer (concentrations of greenhouse gases, solar or volcanic activity, etc.) and the effect is some climate impact variable of interest (large-scale or regional responses in climate impact variables; Hegerl and Zwiers, 2011) or the probability of some specific event (Naveau et al., 2020). Hybrid emissions-driven simulations raise the potential for attribution to be defined in terms of actual emissions – arguably a more useful assessment of the linkage between anthropogenic activity and climate impacts. As such, a perspective paper from the attribution community on the framing of attribution studies in emissions-driven simulations would be a valuable addition to the literature.
4.3 Informing multiple lines of evidence
Longer causal chains from emissions-driven simulations may accelerate a shift away from the use of ESM ensembles in assessment from being an ensemble of opportunity used as a proxy for climate uncertainty. We would argue that this transition has already occurred. IPCC reports up to AR5 relied heavily on ESM ensemble distributions as proxies for climate uncertainty.
However, IPCC AR6 utilized some specific methodologies (Ribes et al., 2021; Brunner et al., 2020) to re-weight ESM distributions of simulations depending on their historical simulated climate change in the context of observations. These methodologies considered primarily the physical response of the climate system to historical concentrations and were used to address the assessed hot model bias (Hausfather et al., 2022), in which the CMIP6 distribution contained some models that notably simulated historical warming beyond that seen in observations. A shift to hybrid emissions-driven simulations would introduce an additional source of potential bias in historical concentrations, which would need proper treatment during any assessment. Any weighting scheme would need to properly represent biases in both physical and carbon cycle elements, together with interactions between those elements (multivariate approaches exist for treating correlated errors, such as errors in CO2 concentrations and global mean temperature; Sanderson et al., 2017b).
As the length of the process chain increases, it will become increasingly unlikely that ESMs will simultaneously reproduce the joint historical evolution of emissions, concentrations and climate response. As such, it might become more useful in assessment to consider ESM ensembles as being sparse samples in a high-dimensional complexity space that is illustrative of potential coupled interactions of the Earth system. Such an interpretation pairs well with the use of meta-models (Nicholls et al., 2022), which can be used to interpolate in a higher-dimensional response space and filter between global-scale projections using observations (Smith et al., 2024).
4.4 Increased computational demand
The operational computational cost of modeling Earth system processes is a factor in development priorities but is not prohibitive. The most notable increase in expense (relative to physics-only simulations) in simulating the carbon cycle arises due to the number of tracers required in the biogeochemical models (Kwiatkowski et al., 2014). As such, an ESM configuration requires some tradeoffs between horizontal and vertical resolution, number of tracers, and the complexity of chemistry and aerosol representation – with the potential for multiple configurations with comparable computational costs with a focus on Earth system processes or resolution, respectively (Dunne et al., 2020). However, because for most CMIP-class models the atmospheric component is significantly more expensive (Danabasoglu et al., 2020; Dunne et al., 2020), land and ocean biogeochemistry (BGC) can be run in parallel with the atmosphere – somewhat increasing the CPU requirements but not the overall runtime of the simulation on parallel high-performance computing (HPC) systems.
Recent ESM development efforts have shown that spinning up oceanic carbon cycles can be achieved on the same timescale as for deep-ocean heat content, which is necessary for any atmosphere–ocean coupled configuration (Lindsay et al., 2014; Yool et al., 2020), although the exact details of how spinup is achieved can impact residual trends (Séférian et al., 2016). Moreover, there are a number of promising efforts to accelerate the spinup of the physical ocean (Lindsay, 2017; Singh et al., 2022) and land (Lu et al., 2020; Sun et al., 2023), further lowering the technical barriers to contributing with stable interactive carbon configurations. Other efforts have improved the parallelization of BGC tracers (Linardakis et al., 2022) and grid coarsening (Berthet et al., 2019) – allowing for the better exploitation of HPC infrastructure to run more comprehensive carbon-resolving simulations without increases in wall-clock time.
Other demands on computation and improvements in model performance for next-generation Earth system models are documented elsewhere and address other key knowledge gaps: the need for kilometer-scale resolution of future climate impacts (Schär et al., 2020), the quantification of parametric uncertainty (Yamazaki et al., 2021), robust sampling of internal variability (Deser et al., 2020), and making the best use of machine learning for computational efficiency and for reducing systematic errors in hybrid Earth system models (Eyring et al., 2024a, b).
Some groups have gone further to suggest that climate modeling efforts must pivot to centralized “digital twins” (X. Li et al., 2023) conducted by a small number of modeling centers to provide global simulations at a kilometer-scale resolution (Bauer et al., 2021). However, such resolutions are not yet tractable in a hybrid emissions-driven configuration, where multi-century simulations are required to spin up the thermal and carbon states of the system. The current highest resolution 3 km “convection-permitting” models achieve 1–10 simulated days per actual day on current high-performance computing architecture (Stevens et al., 2019). Forecast models such as the Integrated Forecast System (IFS) (Roberts et al., 2018) use approximations to achieve longer time steps, which allow an order of magnitude higher throughput, but these approximations are debated for climate applications. Hence, we argue that the current 50–100 km resolution CMIP class of climate and Earth system models remains necessary for long-term emissions-driven climate projections and should continue as a pillar of climate information in parallel to high-resolution activities.
There are a number of highly informative model developments that are likely too ambitious for the CMIP7 timeline but are necessary for a comprehensive process-driven representation of the outcomes of mitigation strategies.
5.1 Closed cycles for water and other major greenhouse gases
Non-CO2 forcers play a significant role in mitigation dynamics and carbon budget uncertainties, in terms of both forcing and scenario uncertainty (Rogelj et al., 2015). However, the capacity of current-generation Earth system models to produce closed and stable cycles for non-CO2 greenhouse gases lags behind that of carbon dioxide (Séférian et al., 2020), which has been demonstrated in historical and scenario simulations in CMIP6 (Arora et al., 2020). While interactive treatment of methane (Heimann et al., 2020; Folberth et al., 2022) and nitrous oxide (Xu-Ri et al., 2012) is being developed in Earth system modeling platforms, no models in CMIP6 have resolved closed cycles for these gases (Séférian et al., 2020). As such, on the timescale of CMIP7, elements of historical and future simulations will, for most models, remain exogenously defined, but developments could be considered for CMIP8 and beyond (Fig. 7d), although it remains likely that some concentration-driven elements will persist – given the large number of minor climate forcers currently handled by SCMs (CFCs, HFCs, PFCs, HCFCs, halons, etc.; Meinshausen et al., 2020).
Closing carbon and nitrogen budgets would require a dedicated joint effort in land and ocean model developments and calibration and the inclusion of potentially absent processes such as lateral transport of dissolved organic carbon and nitrogen (Lauerwald et al., 2017; Lacroix et al., 2021), representation of the coastal ocean dynamics (Mathis et al., 2022), and erosion of coastal permafrost (Nielsen et al., 2022). Similarly, models do not currently close the water cycle. Ice sheets and inland glaciers are a dominant component of sea level rise (itself perhaps the most critical long-term climate adaptation challenge; Hauer et al., 2019), and yet ESMs do not operationally represent them in coupled simulations. Given this, a number of models have a prioritized focus on including ice sheets and glaciers to close the global water cycle (Smith et al., 2021; Lofverstrom et al., 2020).
5.2 Assessment of uncertainty in historical and future land use emissions
A more comprehensive, accurate and consistently diagnosed representation of historical land use emissions and processes is necessary to address both the ensemble bias towards low historical land use emissions as compared to Global Carbon Project estimates in CMIP6 (Friedlingstein et al., 2022b) and the need for a counterfactual no-land-use scenario (Liddicoat et al., 2021). Better land use process representation in an emissions-driven framework must therefore be supported by diagnostic simulations to map between these accounting systems. In a full transient historical or future simulation, it would be difficult to directly isolate the fraction of net land–atmosphere carbon exchange that is associated with land use change and the fraction associated with natural carbon sinks evolving over time under changing climate background states. As such, additional diagnostic counterfactual experiments such as those provided in LUMIP are essential. In CMIP6, these experiments were limited to a concentration-driven framework (e.g., LUMIP experiment hist-noLu, a variant of the concentration-driven historical simulation with no land use change).
In the hybrid emissions-driven model, such diagnostic experiments need to be expanded to include emissions-driven experiments to capture the contribution of land use changes to net transient land use fluxes in the coupled simulation. An esm-hist-noLU, for example, which followed the protocol of esm-historical with fixed land use change, would differ from esm-historical in terms of both the effective land use emissions and any ensuing carbon–climate feedbacks that could modulate the natural emissions as well. As such, a full understanding of the role of land use in the transient land sinks in emissions-driven simulations will require a carefully designed set of complementary diagnostic experiments for both historical and future simulations, likely including both emissions-driven and concentration-driven diagnostic experiments.
5.3 Process-based representation of carbon removal and storage
The objective of interactively resolving the processes associated with carbon removal within the structural framework of Earth system models is a key requirement to providing process uncertainty in carbon dioxide removal (Psarras et al., 2017). Although isolated ESMs have already been used to investigate the potential effectiveness of removals through bioenergy carbon capture and storage (Muri, 2018; Melnikova et al., 2022; Kato and Yamagata, 2014) or potential oceanic CDR approaches through ocean alkalinization (Fröb et al., 2020) or algal cultivation (Wu et al., 2023), these capacities remain experimental and lack representations of and accounting for sequestered carbon in hybrid emissions-driven simulations.
In cases where ESMs resolve some relevant aspects of the carbon removal process (e.g., BECCS or ocean alkalinization), a pipeline must be created for representing how demand for carbon removal strategies in an IAM scenario is translated into appropriate boundary conditions for the ESM (see Fig. 6c, d). Such coupling infrastructure must be urgently defined in order to explore process-based uncertainty in carbon removals in CMIP7.
5.4 Adaptive approaches
The discussion throughout this study has focused on prescribed scenarios, both idealized and quasi-realistic, as generated by integrated assessment models in the CMIP ScenarioMIP exercise. In this model, the ensemble of Earth system models acts as a measure of uncertainty in the coupled carbon–climate response to the emissions pathway. However, the emissions-driven approach opens the door to more interactive treatment of emissions reduction as a function of realized climate change. Since the Paris Agreement, some literature has focused more on adaptive approaches that allow for convergence of a climate model to a target. Such approaches have been used extensively in simple climate models, where it is computationally easy to solve for a given target (Sanderson et al., 2016; Avrutin et al., 2023), and in hybrid mode where simple climate models tuned to reproduce the coupled dynamics of an ESM are used to produce custom emissions pathways for an Earth system model that are consistent with a given temperature target (Goodwin et al., 2018; Sanderson et al., 2017a; Terhaar et al., 2022).
A recent proposal, AERA-MIP (Silvy et al., 2024), has proposed an interactive adaptive approach, where emissions are adjusted in an Earth system model simulation using the relationship between cumulative emissions and temperature (Matthews et al., 2009) to interactively compute an emissions trajectory consistent with any prescribed global warming target. Although this approach is a simplistic model for mitigation policy response to the climate change experienced, it opens the door for more complex adaptive policy scenarios in the future in which two-way couplings between the societal/technological representations exist. It also allows for emission-driven simulations that can stabilize temperature at various global warming levels, enabling the assessment of impacts at different degrees of warming. Such adaptive approaches are increasingly under consideration in the IAM literature (Gambhir et al., 2023), and some groups have succeeded in partial coupling of an ESM and IAM in an integrated framework (Collins et al., 2015). Future efforts could explore more fully the interactions between climate impacts experienced, mitigation ambition and capacity.
Future climate scenarios have been primarily framed in terms of concentrations (or in terms of metrics of global warming) since the Special Report on Emissions Scenarios (SRES) was introduced (Nakicenovic et al., 2000) at the turn of the 21st century. More recently, a parallel process (Moss et al., 2010) advocated defined concentration pathways, with climate effects conditional on concentration pathways assessed by Earth system models while integrated assessment models explore scenarios consistent with the pathways. This approach was chosen pragmatically to allow the two communities to work concurrently and because only a subset of Earth system models have operationally incorporated interactive and closed carbon cycles. However, this framing does not allow carbon cycle uncertainty as represented by diverse, process-resolving Earth system models to be manifested in the scenario outcomes, thus omitting a dominant source of uncertainty in meeting the Paris Agreement (Jones and Friedlingstein, 2020; Holden et al., 2018).
In addition, a rapidly evolving policy landscape increasingly requires information to differentiate between scenarios that represent both different levels of mitigation ambition and different mitigation strategies. A decade earlier in the timing of net-zero CO2 represents a huge economic investment (Nieto, 2022), but at present we do not have scenario outcomes to clearly illustrate the associated climatic benefits in a way that accounts for all uncertainties. Thus, there is no direct and self-consistent simulation of the benefits of mitigation that can be associated with incremental reductions in emissions. On the implementation side, national mitigation policies that (explicitly or implicitly) rely on land use and carbon dioxide removal (CDR) techniques introduce significant uncertainties that remain unsampled in the current ESM scenario framework.
The utility of ESMs is to a large degree shaped by how they are deployed in model intercomparison projects. For example, it has been argued that ESMs can be made more relevant to climate adaptation challenges by resolving and outputting relevant human and ecosystem climate impacts (Bonan and Doney, 2018). Similarly, with the right experimental design, many existing ESMs already include components that can provide valuable insights into the uncertainty surrounding the timing and implementation of net-zero policies.
A draft scenario design document for ScenarioMIP CMIP7 indicates a request for a higher fraction of emissions-driven scenarios (The Scenario Model Intercomparison Project, ScenarioMIP, for CMIP7, 2024), and perspectives on the CMIP7 scenario design have called for higher relevance to Paris Agreement objectives through representative emissions pathways, exploration of CDR risks and potentially counterfactual scenarios (Meinshausen et al., 2024a), while others have called for greater integration with the needs of multiple IPCC working groups and policy relevance (Pirani et al., 2024). Many of these issues can be addressed in a framework enabling an operational assessment of emissions-based policies. This would happen through the explicit representation of carbon dioxide emissions in the context of multiple plausible representations of natural climate system feedbacks. This framework will serve as a structure for incorporating the uncertainties associated with the effectiveness of land use and land- and ocean-based CDR techniques as part of a mitigation portfolio, some of which are already implemented in current-generation Earth system models and some of which require further development beyond the timescale of CMIP7. This framework needs to be flexible enough to accommodate different models at various stages of development and different configurations focusing on different elements of the climate problem, necessitating a hybrid approach for CMIP7.
We propose that the existing CMIP6 model for accommodating a range of aerosol complexity be extended to the simulation of an emissions- and activity-driven carbon cycle. Concentration pathways should still be available for models that require them (and for configurations where carbon cycle feedbacks are not the primary focus, such as high-resolution experiments and some perturbed parameter ensembles). This will need careful communication in the ScenarioMIP framework, as only a subset of models will be subject to carbon cycle uncertainties (although this remains analogous to the CMIP6 treatment of aerosols, where only some models process aerosol emissions directly). It is expected that some climate-relevant forcers such as nitrous oxides and methane will not be represented interactively by a large fraction of models on the timescale of CMIP7; thus exogenous concentrations will still be required in most cases.
Looking ahead to CMIP8 and beyond, ESMs will continue to occupy a critical niche, maximizing the representation of human actions involved in climate mitigation and adaptation in a risk framework relying on deep and diverse process understanding that is uniquely represented in the collective historical and ongoing effort encapsulated in the CMIP ensemble. Future efforts (and their associated computational expense) should be focused on areas where they can add the most value to understanding the Earth system in an ever-widening ecosystem of simple and complex model configurations, which are increasingly well-adapted to different aspects of the climate problem.
We argue that a better understanding and representation of emissions-driven dynamics remain pillars of a wider effort needed to adapt Earth system models to evolving climate challenges. The need for physically realistic, higher-resolution model output has already been documented (Schär et al., 2020; Bauer et al., 2021), but this must be supplemented by lower-resolution operational configurations that are capable of simulating large initial conditions and parametric ensembles of century-driven global response to diverse mitigation strategies. Machine learning may also change this tradeoff – approaches are currently being explored to improve the representation of key resolution-dependent physical processes in global climate models (Gentine et al., 2018), with encouraging results. Such approaches also hold great potential for better utilizing observations to inform future improvement of carbon cycle processes in ESMs (Forkel et al., 2019). Bringing together machine learning (ML) developments for both the physical and carbon cycle components of future emission-driven ESMs offers the potential for a major advance in our ability to model the coupled global climate and carbon cycle (Eyring et al., 2021). However, conceptual problems with overreliance on machine learning for century-scale projections where no training data are available (Watson-Parris, 2021) remain. By requesting that capable centers submit primarily hybrid (i.e., carbon) emissions-driven simulations for CMIP7, the ESM ensemble would become a critically relevant part of the scenario assessment framework, providing the best available process-based estimations of the distribution of potential outcomes resulting from proposed societal transformation pathways. A scenario that achieved a set of policy goals based on the prior generation of models may not achieve those same outcomes with updated models. A default emissions-driven scenario infrastructure would make such comparisons transparent, making it clear when developments in process understanding have measurable impacts on the projected risk associated with a given mitigation strategy.
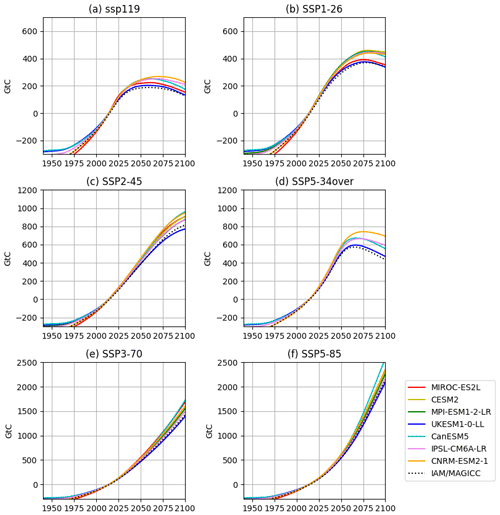
Figure A1Cumulative compatible fossil emissions (relative to 2014) for a range of scenarios and Earth system models in CMIP6, showing MAGICC-calculated CO2 emissions from IAM scenarios (Meinshausen et al., 2020; dotted black lines) and the compatible fossil emissions in CMIP6 ScenarioMIP simulations (colored lines).
All code to reproduce the figures in this study is available at https://doi.org/10.5281/zenodo.12515964 (Sanderson, 2024).
CMIP6 model output is available through the Earth System Grid Foundation (ESGF). CMIP6 scenario data are available at https://greenhousegases.science.unimelb.edu.au/ (Meinshausen et al., 2024b) and https://tntcat.iiasa.ac.at/SspDb (IIASA Energy Program, 2024). Global Carbon Budget data are available at https://www.icos-cp.eu/science-and-impact/global-carbon-budget/2022 (Global Carbon Project, 2022).
BMS wrote the first draft of the paper and produced the figures. Additional analysis was carried out by CK. All authors provided input to, comments on and editing of the various parts of the analysis. In addition, modeling center representatives (JD, VE, CDJ, CJ, DML, RAF, JL, IRS, TI, RS and SZ) were co-responsible for performing subsets of the CMIP6 simulations considered and publishing their model output to the ESGF.
At least one of the (co-)authors is a member of the editorial board of Geoscientific Model Development. The peer-review process was guided by an independent editor, and the authors also have no other competing interests to declare.
Publisher’s note: Copernicus Publications remains neutral with regard to jurisdictional claims made in the text, published maps, institutional affiliations, or any other geographical representation in this paper. While Copernicus Publications makes every effort to include appropriate place names, the final responsibility lies with the authors.
Benjamin M. Sanderson, Veronika Eyring, Roland Séférian, Rosie A. Fisher, Sönke Zaehle, Chris D. Jones and Colin G. Jones acknowledge funding by the European Union's Horizon 2020 (H2020) Research and Innovation program under grant agreement nos. 101003536 (ESM2025 – Earth System Models for the Future) and 821003 (4C, Climate–Carbon Interactions in the Coming Century). Benjamin M. Sanderson and Carl-Friedrich Schleussner acknowledge funding from 101003687 (PROVIDE). Veronika Eyring additionally acknowledges funding by the European Research Council (ERC) Synergy Grant “Understanding and Modeling the Earth System with Machine Learning (USMILE)” under the Horizon 2020 Research and Innovation program (grant agreement no. 855187). Charles D. Koven acknowledges support by the Office of Science, Office of Biological and Environmental Research of the US Department of Energy under contract DE-AC02-05CH11231 through the Regional and Global Model Analysis Program (RUBISCO SFA). Matthew J. Gidden acknowledges funding under the European Union's Horizon Europe Research and Innovation program under grant agreement no. 101056939 (RESCUE). Nadine Mengis acknowledges funding from the Emmy Noether scheme by the German Research Foundation “FOOTPRINTS – From carbOn remOval To achieving the PaRIs agreemeNt's goal: Temperature Stabilisation” (ME 5746/1-1). Abigail L. S. Swann acknowledges support from DOE BER RGMA award DE-SC0021209 to the University of Washington. Chris D. Jones was supported by the Joint UK BEIS/Defra Met Office Hadley Centre Climate Programme (GA01101).
This research has been supported by the Horizon 2020 (grant nos. 101003536, 821003, 855187, 101056939 and 101003687), the Office of Biological and Environmental Research (grant nos. DE-AC02-05CH11231 and DE-SC0021209), and the Deutsche Forschungsgemeinschaft (grant no. ME 5746/1-1).
This paper was edited by Christoph Müller and Paul Ullrich and reviewed by Malte Meinshausen and one anonymous referee.
Abdulla, A., Telschow, F. J. E., Dohner, J., Keeling, R. F., Schwartzman, A., and Victor, D. G.: Atmospheric verification of emissions reductions on paths to deep decarbonization, Environ. Res. Lett., 18, 044003, https://doi.org/10.1088/1748-9326/acbf69, 2023.
Allen, M. R., Frame, D. J., Huntingford, C., Jones, C. D., Lowe, J. A., Meinshausen, M., and Meinshausen, N.: Warming caused by cumulative carbon emissions towards the trillionth tonne, Nature, 458, 1163–1166, https://doi.org/10.1038/nature08019, 2009.
Anderegg, W. R. L., Chegwidden, O. S., Badgley, G., Trugman, A. T., Cullenward, D., Abatzoglou, J. T., Hicke, J. A., Freeman, J., and Hamman, J. J.: Future climate risks from stress, insects and fire across US forests, Ecol. Lett., 25, 1510–1520, https://doi.org/10.1111/ele.14018, 2022.
Anderson, K. and Peters, G.: The trouble with negative emissions, Science, 354, 182–183, https://doi.org/10.1126/science.aah4567, 2016.
Armour, K. C., Eisenman, I., Blanchard-Wrigglesworth, E., McCusker, K. E., and Bitz, C. M.: The reversibility of sea ice loss in a state-of-the-art climate model, Geophys. Res. Lett., 38, L16705, https://doi.org/10.1029/2011gl048739, 2011.
Armstrong McKay, D. I., Staal, A., Abrams, J. F., Winkelmann, R., Sakschewski, B., Loriani, S., Fetzer, I., Cornell, S. E., Rockström, J., and Lenton, T. M.: Exceeding 1.5 °C global warming could trigger multiple climate tipping points, Science, 377, eabn7950, https://doi.org/10.1126/science.abn7950, 2022.
Arnell, N. W., Livermore, M. J. L., Kovats, S., Levy, P. E., Nicholls, R., Parry, M. L., and Gaffin, S. R.: Climate and socio-economic scenarios for global-scale climate change impacts assessments: characterising the SRES storylines, Global Environ. Chang., 14, 3–20, https://doi.org/10.1016/j.gloenvcha.2003.10.004, 2004.
Arora, V. K., Katavouta, A., Williams, R. G., Jones, C. D., Brovkin, V., Friedlingstein, P., Schwinger, J., Bopp, L., Boucher, O., Cadule, P., Chamberlain, M. A., Christian, J. R., Delire, C., Fisher, R. A., Hajima, T., Ilyina, T., Joetzjer, E., Kawamiya, M., Koven, C. D., Krasting, J. P., Law, R. M., Lawrence, D. M., Lenton, A., Lindsay, K., Pongratz, J., Raddatz, T., Séférian, R., Tachiiri, K., Tjiputra, J. F., Wiltshire, A., Wu, T., and Ziehn, T.: Carbon–concentration and carbon–climate feedbacks in CMIP6 models and their comparison to CMIP5 models, Biogeosciences, 17, 4173–4222, https://doi.org/10.5194/bg-17-4173-2020, 2020.
Avrutin, S., Goodwin, P., and Ezard, T. H. G.: Assessing the remaining carbon budget through the lens of policy-driven acidification and temperature targets, Clim. Change, 176, 128, https://doi.org/10.1007/s10584-023-03587-0, 2023.
Bauer, P., Stevens, B., and Hazeleger, W.: A digital twin of Earth for the green transition, Nat. Clim. Change, 11, 80–83, https://doi.org/10.1038/s41558-021-00986-y, 2021.
Baumstark, L., Bauer, N., Benke, F., Bertram, C., Bi, S., Gong, C. C., Dietrich, J. P., Dirnaichner, A., Giannousakis, A., Hilaire, J., Klein, D., Koch, J., Leimbach, M., Levesque, A., Madeddu, S., Malik, A., Merfort, A., Merfort, L., Odenweller, A., Pehl, M., Pietzcker, R. C., Piontek, F., Rauner, S., Rodrigues, R., Rottoli, M., Schreyer, F., Schultes, A., Soergel, B., Soergel, D., Strefler, J., Ueckerdt, F., Kriegler, E., and Luderer, G.: REMIND2.1: transformation and innovation dynamics of the energy-economic system within climate and sustainability limits, Geosci. Model Dev., 14, 6571–6603, https://doi.org/10.5194/gmd-14-6571-2021, 2021.
Berthet, S., Séférian, R., Bricaud, C., Chevallier, M., Voldoire, A., and Ethé, C.: Evaluation of an online grid‐coarsening algorithm in a global eddy‐admitting ocean biogeochemical model, J. Adv. Model. Earth Sy, 11, 1759–1783, 2019.
Bodman, R. W., Rayner, P. J., and Jones, R. N.: How do carbon cycle uncertainties affect IPCC temperature projections?, Atmos. Sci. Lett., 17, 236–242, https://doi.org/10.1002/asl.648, 2016.
Bonan, G. B. and Doney, S. C.: Climate, ecosystems, and planetary futures: The challenge to predict life in Earth system models, Science, 359, 6375, https://doi.org/10.1126/science.aam8328, 2018.
Bourgeois, T., Goris, N., Schwinger, J., and Tjiputra, J. F.: Stratification constrains future heat and carbon uptake in the Southern Ocean between 30° S and 55° S, Nat. Commun., 13, 340, https://doi.org/10.1038/s41467-022-27979-5, 2022.
Brunner, L., Pendergrass, A. G., Lehner, F., Merrifield, A. L., Lorenz, R., and Knutti, R.: Reduced global warming from CMIP6 projections when weighting models by performance and independence, Earth Syst. Dynam., 11, 995–1012, https://doi.org/10.5194/esd-11-995-2020, 2020.
Burt, D. J., Fröb, F., and Ilyina, T.: The Sensitivity of the Marine Carbonate System to Regional Ocean Alkalinity Enhancement, Front. Climate, 3, 624075, https://doi.org/10.3389/fclim.2021.624075, 2021.
Calvin, K., Patel, P., Clarke, L., Asrar, G., Bond-Lamberty, B., Cui, R. Y., Di Vittorio, A., Dorheim, K., Edmonds, J., Hartin, C., Hejazi, M., Horowitz, R., Iyer, G., Kyle, P., Kim, S., Link, R., McJeon, H., Smith, S. J., Snyder, A., Waldhoff, S., and Wise, M.: GCAM v5.1: representing the linkages between energy, water, land, climate, and economic systems, Geosci. Model Dev., 12, 677–698, https://doi.org/10.5194/gmd-12-677-2019, 2019.
Cess, R. D., Potter, G. L., Blanchet, J. P., Boer, G. J., Ghan, S. J., Kiehl, J. T., LE Treut, H., Li, Z. X., Liang, X. Z., Mitchell, J. F., Morcrette, J. J., Randall, D. A., Riches, M. R., Roeckner, E., Schlese, U., Slingo, A., Taylor, K. E., Washington, W. M., Wetherald, R. T., and Yagai, I.: Interpretation of cloud-climate feedback as produced by 14 atmospheric general circulation models, Science, 245, 513–516, https://doi.org/10.1126/science.245.4917.513, 1989.
Chai, Y., Martins, G., Nobre, C., von Randow, C., Chen, T., and Dolman, H.: Constraining Amazonian land surface temperature sensitivity to precipitation and the probability of forest dieback, npj Clim. Atmos. Sci., 4, 1–7, https://doi.org/10.1038/s41612-021-00162-1, 2021.
Ciavarella, A., Stott, P., and Lowe, J.: Early benefits of mitigation in risk of regional climate extremes, Nat. Clim. Change, 7, 326–330, https://doi.org/10.1038/nclimate3259, 2017.
CMIP phase 7 (CMIP7): https://wcrp-cmip.org/cmip7/, last access: 21 June 2024.
Collins, W. D., Craig, A. P., Truesdale, J. E., Di Vittorio, A. V., Jones, A. D., Bond-Lamberty, B., Calvin, K. V., Edmonds, J. A., Kim, S. H., Thomson, A. M., Patel, P., Zhou, Y., Mao, J., Shi, X., Thornton, P. E., Chini, L. P., and Hurtt, G. C.: The integrated Earth system model version 1: formulation and functionality, Geosci. Model Dev., 8, 2203–2219, https://doi.org/10.5194/gmd-8-2203-2015, 2015.
Collins, W. J., Lamarque, J.-F., Schulz, M., Boucher, O., Eyring, V., Hegglin, M. I., Maycock, A., Myhre, G., Prather, M., Shindell, D., and Smith, S. J.: AerChemMIP: quantifying the effects of chemistry and aerosols in CMIP6, Geosci. Model Dev., 10, 585–607, https://doi.org/10.5194/gmd-10-585-2017, 2017.
Covey, C., AchutaRao, K. M., Cubasch, U., Jones, P., Lambert, S. J., Mann, M. E., Phillips, T. J., and Taylor, K. E.: An overview of results from the Coupled Model Intercomparison Project, Global Planet. Change, 37, 103–133, https://doi.org/10.1016/S0921-8181(02)00193-5, 2003.
Cowtan, K. and Way, R. G.: Coverage bias in the HadCRUT4 temperature series and its impact on recent temperature trends, Q. J. Roy. Meteor. Soc., 140, 1935–1944, https://doi.org/10.1002/qj.2297, 2014.
Cox, P. M., Betts, R. A., Jones, C. D., Spall, S. A., and Totterdell, I. J.: Acceleration of global warming due to carbon-cycle feedbacks in a coupled climate model, Nature, 408, 184–187, https://doi.org/10.1038/35041539, 2000.
Damon Matthews, H., Tokarska, K. B., Rogelj, J., Smith, C. J., MacDougall, A. H., Haustein, K., Mengis, N., Sippel, S., Forster, P. M., and Knutti, R.: An integrated approach to quantifying uncertainties in the remaining carbon budget, Commun. Earth Environ., 2, 1–11, https://doi.org/10.1038/s43247-020-00064-9, 2021.
Danabasoglu, G., Lamarque, J. F., Bacmeister, J., Bailey, D. A., DuVivier, A. K., Edwards, J., Emmons, L. K., Fasullo, J., Garcia, R., Gettelman, A., and Hannay, C.: The community earth system model version 2 (CESM2), J. Adv. Model. Earth Sy., 12, e2019MS001916, https://doi.org/10.1029/2019MS001916, 2020.
Deng, Z., Ciais, P., Tzompa-Sosa, Z. A., Saunois, M., Qiu, C., Tan, C., Sun, T., Ke, P., Cui, Y., Tanaka, K., Lin, X., Thompson, R. L., Tian, H., Yao, Y., Huang, Y., Lauerwald, R., Jain, A. K., Xu, X., Bastos, A., Sitch, S., Palmer, P. I., Lauvaux, T., d'Aspremont, A., Giron, C., Benoit, A., Poulter, B., Chang, J., Petrescu, A. M. R., Davis, S. J., Liu, Z., Grassi, G., Albergel, C., Tubiello, F. N., Perugini, L., Peters, W., and Chevallier, F.: Comparing national greenhouse gas budgets reported in UNFCCC inventories against atmospheric inversions, Earth Syst. Sci. Data, 14, 1639–1675, https://doi.org/10.5194/essd-14-1639-2022, 2022.
Deser, C., Lehner, F., Rodgers, K. B., Ault, T., Delworth, T. L., DiNezio, P. N., Fiore, A., Frankignoul, C., Fyfe, J. C., Horton, D. E., and Kay, J. E.: Insights from Earth system model initial-condition large ensembles and future prospects, Nat. Clim. Change, 10, 277–286, 2020.
Drouet, L., Bosetti, V., Padoan, S. A., Aleluia Reis, L., Bertram, C., Dalla Longa, F., Després, J., Emmerling, J., Fosse, F., Fragkiadakis, K., Frank, S., Fricko, O., Fujimori, S., Harmsen, M., Krey, V., Oshiro, K., Nogueira, L. P., Paroussos, L., Piontek, F., Riahi, K., Rochedo, P. R. R., Schaeffer, R., Takakura, J., van der Wijst, K.-I., van der Zwaan, B., van Vuuren, D., Vrontisi, Z., Weitzel, M., Zakeri, B., and Tavoni, M.: Net zero-emission pathways reduce the physical and economic risks of climate change, Nat. Clim. Change, 11, 1070–1076, https://doi.org/10.1038/s41558-021-01218-z, 2021.
Dunne, J. P., Horowitz, L. W., Adcroft, A. J., Ginoux, P., Held, I. M., John, J. G., Krasting, J. P., Malyshev, S., Naik, V., Paulot, F., and Shevliakova, E.: The GFDL Earth System Model version 4.1 (GFDL‐ESM 4.1): Overall coupled model description and simulation characteristics, J. Adv. Model. Earth Sy., 12, e2019MS002015, https://doi.org/10.1029/2019MS002015, 2020.
Emerson, D.: Biogenic Iron Dust: A Novel Approach to Ocean Iron Fertilization as a Means of Large Scale Removal of Carbon Dioxide From the Atmosphere, Front. Mar. Sci., 6, 22, https://doi.org/10.3389/fmars.2019.00022, 2019.
Eyring, V., Bony, S., Meehl, G. A., Senior, C. A., Stevens, B., Stouffer, R. J., and Taylor, K. E.: Overview of the Coupled Model Intercomparison Project Phase 6 (CMIP6) experimental design and organization, Geosci. Model Dev., 9, 1937–1958, https://doi.org/10.5194/gmd-9-1937-2016, 2016.
Eyring, V., Mishra, V., Griffith, G. P., Chen, L., Keenan, T., Turetsky, M. R., Brown, S., Jotzo, F., Moore, F. C., and van der Linden, S.: Reflections and projections on a decade of climate science, Nat. Clim. Change, 11, 279–285, https://doi.org/10.1038/s41558-021-01020-x, 2021.
Eyring, V., Gentine, P., Camps-Valls, G., Lawrence, D. M., and Reichstein, M.: AI-empowered Next-generation Multiscale Climate Modeling for Mitigation and Adaptation, Nat. Geosci., 17, 963–971, https://doi.org/10.1038/s41561-024-01527-w, 2024a.
Eyring, V., Collins, W. D., Gentine, P., Barnes, E. A., Barreiro, M., Beucler, T., Bocquet, M., Bretherton, C. S., Christensen, H. M., ,, Gagne, D J, Hoyer, S., Iglesias-Suarez, F., Lopez-Gomez, I., McGraw, M. C., Meehl, G. A., Molina, M. J., Monteleoni, C., Mueller, J., Pritchard, M. S., Rolnick, D., Runge, J., Stier, P., Watt-Meyer, O., Weigel, K., Yu, R., and Zanna, L.: Pushing the frontiers in climate modeling and analysis with machine learning, Nat. Clim. Change, 14, 916–928, https://doi.org/10.1038/s41558-024-02095-y, 2024b.
Fakhraee, M., Li, Z., Planavsky, N. J., and Reinhard, C. T.: A biogeochemical model of mineral-based ocean alkalinity enhancement: impacts on the biological pump and ocean carbon uptake, Environ. Res. Lett., 18, 044047, https://doi.org/10.1088/1748-9326/acc9d4, 2023.
Feng, L., Smith, S. J., Braun, C., Crippa, M., Gidden, M. J., Hoesly, R., Klimont, Z., van Marle, M., van den Berg, M., and van der Werf, G. R.: The generation of gridded emissions data for CMIP6, Geosci. Model Dev., 13, 461–482, https://doi.org/10.5194/gmd-13-461-2020, 2020.
Folberth, G. A., Staniaszek, Z., Archibald, A. T., Gedney, N., Griffiths, P. T., Jones, C. D., O'Connor, F. M., Parker, R. J., Sellar, A. A., and Wiltshire, A.: Description and Evaluation of an Emission-Driven and Fully Coupled Methane Cycle in UKESM1, J. Adv. Model. Earth Sy., 14, e2021MS002982, https://doi.org/10.1029/2021MS002982, 2022.
Forkel, M., Drüke, M., Thurner, M., Dorigo, W., Schaphoff, S., Thonicke, K., von Bloh, W., and Carvalhais, N.: Constraining modelled global vegetation dynamics and carbon turnover using multiple satellite observations, Sci. Rep., 9, 18757, https://doi.org/10.1038/s41598-019-55187-7, 2019.
Forster, P., Storelvmo, T., Armour, K., Collins, W., Dufresne, J., Frame, D., Lunt, D., Mauritsen, T., Palmer, M., Watanabe, M., Wild, M., and Zhang, H.: The earth's energy budget, climate feedbacks and climate sensitivity, in: Climate Change 2021 – The Physical Science Basis, Cambridge University Press, 923–1054, https://doi.org/10.1017/9781009157896.009, 2023.
Frame, D. J., Stone, D. A., Stott, P. A., and Allen, M. R.: Alternatives to stabilization scenarios, Geophys. Res. Lett., 33, L14707, https://doi.org/10.1029/2006gl025801, 2006.
Friedlingstein, P., Cox, P., Betts, R., Bopp, L., von Bloh, W., Brovkin, V., Cadule, P., Doney, S., Eby, M., Fung, I., Bala, G., John, J., Jones, C., Joos, F., Kato, T., Kawamiya, M., Knorr, W., Lindsay, K., Matthews, H. D., Raddatz, T., Rayner, P., Reick, C., Roeckner, E., Schnitzler, K.-G., Schnur, R., Strassmann, K., Weaver, A. J., Yoshikawa, C., and Zeng, N.: Climate–Carbon Cycle Feedback Analysis: Results from the C4MIP Model Intercomparison, J. Climate, 19, 3337–3353, https://doi.org/10.1175/JCLI3800.1, 2006.
Friedlingstein, P., Jones, M. W., O'Sullivan, M., Andrew, R. M., Bakker, D. C. E., Hauck, J., Le Quéré, C., Peters, G. P., Peters, W., Pongratz, J., Sitch, S., Canadell, J. G., Ciais, P., Jackson, R. B., Alin, S. R., Anthoni, P., Bates, N. R., Becker, M., Bellouin, N., Bopp, L., Chau, T. T. T., Chevallier, F., Chini, L. P., Cronin, M., Currie, K. I., Decharme, B., Djeutchouang, L. M., Dou, X., Evans, W., Feely, R. A., Feng, L., Gasser, T., Gilfillan, D., Gkritzalis, T., Grassi, G., Gregor, L., Gruber, N., Gürses, Ö., Harris, I., Houghton, R. A., Hurtt, G. C., Iida, Y., Ilyina, T., Luijkx, I. T., Jain, A., Jones, S. D., Kato, E., Kennedy, D., Klein Goldewijk, K., Knauer, J., Korsbakken, J. I., Körtzinger, A., Landschützer, P., Lauvset, S. K., Lefèvre, N., Lienert, S., Liu, J., Marland, G., McGuire, P. C., Melton, J. R., Munro, D. R., Nabel, J. E. M. S., Nakaoka, S.-I., Niwa, Y., Ono, T., Pierrot, D., Poulter, B., Rehder, G., Resplandy, L., Robertson, E., Rödenbeck, C., Rosan, T. M., Schwinger, J., Schwingshackl, C., Séférian, R., Sutton, A. J., Sweeney, C., Tanhua, T., Tans, P. P., Tian, H., Tilbrook, B., Tubiello, F., van der Werf, G. R., Vuichard, N., Wada, C., Wanninkhof, R., Watson, A. J., Willis, D., Wiltshire, A. J., Yuan, W., Yue, C., Yue, X., Zaehle, S., and Zeng, J.: Global Carbon Budget 2021, Earth Syst. Sci. Data, 14, 1917–2005, https://doi.org/10.5194/essd-14-1917-2022, 2022a.
Friedlingstein, P., O'Sullivan, M., Jones, M. W., Andrew, R. M., Gregor, L., Hauck, J., Le Quéré, C., Luijkx, I. T., Olsen, A., Peters, G. P., Peters, W., Pongratz, J., Schwingshackl, C., Sitch, S., Canadell, J. G., Ciais, P., Jackson, R. B., Alin, S. R., Alkama, R., Arneth, A., Arora, V. K., Bates, N. R., Becker, M., Bellouin, N., Bittig, H. C., Bopp, L., Chevallier, F., Chini, L. P., Cronin, M., Evans, W., Falk, S., Feely, R. A., Gasser, T., Gehlen, M., Gkritzalis, T., Gloege, L., Grassi, G., Gruber, N., Gürses, Ö., Harris, I., Hefner, M., Houghton, R. A., Hurtt, G. C., Iida, Y., Ilyina, T., Jain, A. K., Jersild, A., Kadono, K., Kato, E., Kennedy, D., Klein Goldewijk, K., Knauer, J., Korsbakken, J. I., Landschützer, P., Lefèvre, N., Lindsay, K., Liu, J., Liu, Z., Marland, G., Mayot, N., McGrath, M. J., Metzl, N., Monacci, N. M., Munro, D. R., Nakaoka, S.-I., Niwa, Y., O'Brien, K., Ono, T., Palmer, P. I., Pan, N., Pierrot, D., Pocock, K., Poulter, B., Resplandy, L., Robertson, E., Rödenbeck, C., Rodriguez, C., Rosan, T. M., Schwinger, J., Séférian, R., Shutler, J. D., Skjelvan, I., Steinhoff, T., Sun, Q., Sutton, A. J., Sweeney, C., Takao, S., Tanhua, T., Tans, P. P., Tian, X., Tian, H., Tilbrook, B., Tsujino, H., Tubiello, F., van der Werf, G. R., Walker, A. P., Wanninkhof, R., Whitehead, C., Willstrand Wranne, A., Wright, R., Yuan, W., Yue, C., Yue, X., Zaehle, S., Zeng, J., and Zheng, B.: Global Carbon Budget 2022, Earth Syst. Sci. Data, 14, 4811–4900, https://doi.org/10.5194/essd-14-4811-2022, 2022b.
Fröb, F., Sonntag, S., Pongratz, J., Schmidt, H., and Ilyina, T.: Detectability of artificial ocean alkalinization and stratospheric aerosol injection in MPI-ESM, Earths Future, 8, e2020EF001634, https://doi.org/10.1029/2020ef001634, 2020.
Fung, I. Y., Doney, S. C., Lindsay, K., and John, J.: Evolution of carbon sinks in a changing climate, P. Natl. Acad. Sci. USA., 102, 11201–11206, https://doi.org/10.1073/pnas.0504949102, 2005.
Fuss, S., Canadell, J. G., Peters, G. P., Tavoni, M., Andrew, R. M., Ciais, P., Jackson, R. B., Jones, C. D., Kraxner, F., Nakicenovic, N., Le Quéré, C., Raupach, M. R., Sharifi, A., Smith, P., and Yamagata, Y.: Betting on negative emissions, Nat. Clim. Change, 4, 850–853, https://doi.org/10.1038/nclimate2392, 2014.
Gambhir, A., Mittal, S., Lamboll, R. D., Grant, N., Bernie, D., Gohar, L., Hawkes, A., Köberle, A., Rogelj, J., and Lowe, J. A.: Adjusting 1.5 degree C climate change mitigation pathways in light of adverse new information, Nat. Commun., 14, 1–13, https://doi.org/10.1038/s41467-023-40673-4, 2023.
Gentine, P., Pritchard, M., Rasp, S., Reinaudi, G., and Yacalis, G.: Could machine learning break the convection parameterization deadlock?, Geophys. Res. Lett., 45, 5742–5751, https://doi.org/10.1029/2018gl078202, 2018.
Gidden, M. J., Riahi, K., Smith, S. J., Fujimori, S., Luderer, G., Kriegler, E., van Vuuren, D. P., van den Berg, M., Feng, L., Klein, D., Calvin, K., Doelman, J. C., Frank, S., Fricko, O., Harmsen, M., Hasegawa, T., Havlik, P., Hilaire, J., Hoesly, R., Horing, J., Popp, A., Stehfest, E., and Takahashi, K.: Global emissions pathways under different socioeconomic scenarios for use in CMIP6: a dataset of harmonized emissions trajectories through the end of the century, Geosci. Model Dev., 12, 1443–1475, https://doi.org/10.5194/gmd-12-1443-2019, 2019.
Gidden, M. J., Gasser, T., Grassi, G., Forsell, N., Janssens, I., Lamb, W. F., Minx, J., Nicholls, Z., Steinhauser, J., and Riahi, K.: Aligning climate scenarios to emissions inventories shifts global benchmarks, Nature, 624, 102–108, https://doi.org/10.1038/s41586-023-06724-y, 2023.
Giebink, C. L., Domke, G. M., Fisher, R. A., Heilman, K. A., Moore, D. J. P., DeRose, R. J., and Evans, M. E. K.: The policy and ecology of forest-based climate mitigation: challenges, needs, and opportunities, Plant Soil, 479, 25–52, https://doi.org/10.1007/s11104-022-05315-6, 2022.
Gillett, N. P., Shiogama, H., Funke, B., Hegerl, G., Knutti, R., Matthes, K., Santer, B. D., Stone, D., and Tebaldi, C.: The Detection and Attribution Model Intercomparison Project (DAMIP v1.0) contribution to CMIP6, Geosci. Model Dev., 9, 3685–3697, https://doi.org/10.5194/gmd-9-3685-2016, 2016.
Global Carbon Project: Supplemental data of Global Carbon Budget 2022 (Version 1.0), Global Carbon Project [data set], https://doi.org/10.18160/gcp-2022, 2022.
González, M. F., Ilyina, T., Sonntag, S., and Schmidt, H.: Enhanced rates of regional warming and ocean acidification after termination of large-scale ocean alkalinization, Geophys. Res. Lett., 45, 7120–7129, https://doi.org/10.1029/2018gl077847, 2018.
Goodwin, P., Brown, S., Haigh, I. D., Nicholls, R. J., and Matter, J. M.: Adjusting mitigation pathways to stabilize climate at 1.5 °C and 2.0 °C rise in global temperatures to year 2300, Earths Future, 6, 601–615, https://doi.org/10.1002/2017ef000732, 2018.
Grant, N., Hawkes, A., Mittal, S., and Gambhir, A.: The policy implications of an uncertain carbon dioxide removal potential, Joule, 5, 2593–2605, https://doi.org/10.1016/j.joule.2021.09.004, 2021.
Grassi, G., Stehfest, E., Rogelj, J., van Vuuren, D., Cescatti, A., House, J., Nabuurs, G.-J., Rossi, S., Alkama, R., Viñas, R. A., Calvin, K., Ceccherini, G., Federici, S., Fujimori, S., Gusti, M., Hasegawa, T., Havlik, P., Humpenöder, F., Korosuo, A., Perugini, L., Tubiello, F. N., and Popp, A.: Critical adjustment of land mitigation pathways for assessing countries' climate progress, Nat. Clim. Change, 11, 425–434, https://doi.org/10.1038/s41558-021-01033-6, 2021.
Hansis, E., Davis, S. J., and Pongratz, J.: Relevance of methodological choices for accounting of land use change carbon fluxes, Global Biogeochem. Cy., 29, 1230–1246, https://doi.org/10.1002/2014gb004997, 2015.
Hartmann, J., Suitner, N., Lim, C., Schneider, J., Marín-Samper, L., Arístegui, J., Renforth, P., Taucher, J., and Riebesell, U.: Stability of alkalinity in ocean alkalinity enhancement (OAE) approaches – consequences for durability of CO2 storage, Biogeosciences, 20, 781–802, https://doi.org/10.5194/bg-20-781-2023, 2023.
Hauer, M. E., Fussell, E., Mueller, V., Burkett, M., Call, M., Abel, K., McLeman, R., and Wrathall, D.: Sea-level rise and human migration, Nat. Rev. Earth Environ., 1, 28–39, https://doi.org/10.1038/s43017-019-0002-9, 2019.
Hausfather, Z. and Peters, G. P.: Emissions – the “business as usual” story is misleading, Nature, 577, 618–620, https://doi.org/10.1038/d41586-020-00177-3, 2020a.
Hausfather, Z. and Peters, G. P.: RCP8.5 is a problematic scenario for near-term emissions, P. Natl. Acad. Sci. USA, 117, 27791–27792, https://doi.org/10.1073/pnas.2017124117, 2020b.
Hausfather, Z., Marvel, K., Schmidt, G. A., Nielsen-Gammon, J. W., and Zelinka, M.: Climate simulations: recognize the “hot model” problem, Nature, 605, 26–29, https://doi.org/10.1038/d41586-022-01192-2, 2022.
Hegerl, G. and Zwiers, F.: Use of models in detection and attribution of climate change, Wires Clim. Change, 2, 570–591, https://doi.org/10.1002/wcc.121, 2011.
Heimann, I., Griffiths, P. T., Warwick, N. J., Abraham, N. L., Archibald, A. T., and Pyle, J. A.: Methane Emissions in a Chemistry-Climate Model: Feedbacks and Climate Response, J. Adv. Model Earth. Sy., 12, e2019MS002019, https://doi.org/10.1029/2019MS002019, 2020.
Holden, P. B., Edwards, N. R., Ridgwell, A., Wilkinson, R. D., Fraedrich, K., Lunkeit, F., Pollitt, H., Mercure, J.-F., Salas, P., Lam, A., Knobloch, F., Chewpreecha, U., and Viñuales, J. E.: Climate–carbon cycle uncertainties and the Paris Agreement, Nat. Clim. Change, 8, 609–613, https://doi.org/10.1038/s41558-018-0197-7, 2018.
Houghton, R. A. and Nassikas, A. A.: Global and regional fluxes of carbon from land use and land cover change 1850–2015, Global Biogeochem. Cy., 31, 456–472, https://doi.org/10.1002/2016gb005546, 2017.
Hurtt, G. C., Chini, L., Sahajpal, R., Frolking, S., Bodirsky, B. L., Calvin, K., Doelman, J. C., Fisk, J., Fujimori, S., Klein Goldewijk, K., Hasegawa, T., Havlik, P., Heinimann, A., Humpenöder, F., Jungclaus, J., Kaplan, J. O., Kennedy, J., Krisztin, T., Lawrence, D., Lawrence, P., Ma, L., Mertz, O., Pongratz, J., Popp, A., Poulter, B., Riahi, K., Shevliakova, E., Stehfest, E., Thornton, P., Tubiello, F. N., van Vuuren, D. P., and Zhang, X.: Harmonization of global land use change and management for the period 850–2100 (LUH2) for CMIP6, Geosci. Model Dev., 13, 5425–5464, https://doi.org/10.5194/gmd-13-5425-2020, 2020.
IIASA Energy Program: SSP database, https://tntcat.iiasa.ac.at/SspDb, last access: 30 October 2024.
Ilyina, T., Wolf-Gladrow, D., Munhoven, G., and Heinze, C.: Assessing the potential of calcium-based artificial ocean alkalinization to mitigate rising atmospheric CO2 and ocean acidification, Geophys. Res. Lett., 40, 5909–5914, https://doi.org/10.1002/2013gl057981, 2013.
Ilyina, T., Li, H., Spring, A., Müller, W. A., Bopp, L., Chikamoto, M. O., Danabasoglu, G., Dobrynin, M., Dunne, J., Fransner, F., Friedlingstein, P., Lee, W., Lovenduski, N. S., Merryfield, W. J., Mignot, J., Park, J. Y., Séférian, R., Sospedra-Alfonso, R., Watanabe, M., and Yeager, S.: Predictable variations of the carbon sinks and atmospheric CO2 growth in a multi-model framework, Geophys. Res. Lett., 48, e2020GL090695, https://doi.org/10.1029/2020gl090695, 2021.
Intergovernmental Panel on Climate Change (IPCC): Global Warming of 1.5 °C: IPCC Special Report on Impacts of Global Warming of 1.5 °C above Pre-industrial Levels in Context of Strengthening Response to Climate Change, Sustainable Development, and Efforts to Eradicate Poverty, Cambridge University Press, https://doi.org/10.1017/9781009157940, 2022.
IPCC: Climate Change 2021: The Physical Science Basis., Contribution of Working Group I to the Sixth Assessment Report of the Intergovernmental Panel on Climate Change, edited by: Masson-Delmotte, V., Zhai, P., Pirani, A., Connors, S. L., Péan, C., Berger, S., Caud, N., Chen, Y., Goldfarb, L., Gomis, M. I., Huang, M., Leitzell, K., Lonnoy, E., Matthews, J. B. R., Maycock, T. K., Waterfield, T., Yelekçi, O., Yu, R., and Zhou, B., Cambridge University Press, Cambridge, United Kingdom and New York, NY, USA, https://doi.org/10.1017/9781009157896, 2021.
Jenkins, S., Sanderson, B., Peters, G., Frölicher, T. L., Friedlingstein, P., and Allen, M.: The multi-decadal response to net zero CO 2 emissions and implications for emissions policy, Geophys. Res. Lett., 49, e2022GL101047, https://doi.org/10.1029/2022gl101047, 2022.
Jevrejeva, S., Jackson, L. P., Grinsted, A., Lincke, D., and Marzeion, B.: Flood damage costs under the sea level rise with warming of 1.5 °C and 2 °C, Environ. Res. Lett., 13, 074014, https://doi.org/10.1088/1748-9326/aacc76, 2018.
Jones, C., Robertson, E., Arora, V., Friedlingstein, P., Shevliakova, E., Bopp, L., Brovkin, V., Hajima, T., Kato, E., Kawamiya, M., Liddicoat, S., Lindsay, K., Reick, C. H., Roelandt, C., Segschneider, J., and Tjiputra, J.: Twenty-First-Century Compatible CO2 Emissions and Airborne Fraction Simulated by CMIP5 Earth System Models under Four Representative Concentration Pathways, J. Climate, 26, 4398–4413, https://doi.org/10.1175/JCLI-D-12-00554.1, 2013.
Jones, C. D.: So What Is in an Earth System Model?, J. Adv. Model. Earth Sy., 12, e2019MS001967, https://doi.org/10.1029/2019MS001967, 2020.
Jones, C. D. and Friedlingstein, P.: Quantifying process-level uncertainty contributions to TCRE and carbon budgets for meeting Paris Agreement climate targets, Environ. Res. Lett., 15, 074019, https://doi.org/10.1088/1748-9326/ab858a, 2020.
Jones, C. D., Arora, V., Friedlingstein, P., Bopp, L., Brovkin, V., Dunne, J., Graven, H., Hoffman, F., Ilyina, T., John, J. G., Jung, M., Kawamiya, M., Koven, C., Pongratz, J., Raddatz, T., Randerson, J. T., and Zaehle, S.: C4MIP – The Coupled Climate–Carbon Cycle Model Intercomparison Project: experimental protocol for CMIP6, Geosci. Model Dev., 9, 2853–2880, https://doi.org/10.5194/gmd-9-2853-2016, 2016.
Jones, C. D., Frölicher, T. L., Koven, C., MacDougall, A. H., Matthews, H. D., Zickfeld, K., Rogelj, J., Tokarska, K. B., Gillett, N. P., Ilyina, T., Meinshausen, M., Mengis, N., Séférian, R., Eby, M., and Burger, F. A.: The Zero Emissions Commitment Model Intercomparison Project (ZECMIP) contribution to C4MIP: quantifying committed climate changes following zero carbon emissions, Geosci. Model Dev., 12, 4375–4385, https://doi.org/10.5194/gmd-12-4375-2019, 2019.
Joos, F., Plattner, G. K., Stocker, T. F., Marchal, O., and Schmittner, A.: Global warming and marine carbon cycle feedbacks on future atmospheric CO2, Science, 284, 464–467, https://doi.org/10.1126/science.284.5413.464, 1999.
Kato, E. and Yamagata, Y.: BECCS capability of dedicated bioenergy crops under a future land-use scenario targeting net negative carbon emissions, Earths Future, 2, 421–439, https://doi.org/10.1002/2014ef000249, 2014.
Keller, D. P., Feng, E. Y., and Oschlies, A.: Potential climate engineering effectiveness and side effects during a high carbon dioxide-emission scenario, Nat. Commun., 5, 3304, https://doi.org/10.1038/ncomms4304, 2014.
Keller, D. P., Lenton, A., Scott, V., Vaughan, N. E., Bauer, N., Ji, D., Jones, C. D., Kravitz, B., Muri, H., and Zickfeld, K.: The Carbon Dioxide Removal Model Intercomparison Project (CDRMIP): rationale and experimental protocol for CMIP6, Geosci. Model Dev., 11, 1133–1160, https://doi.org/10.5194/gmd-11-1133-2018, 2018.
Kikstra, J. S., Nicholls, Z. R. J., Smith, C. J., Lewis, J., Lamboll, R. D., Byers, E., Sandstad, M., Meinshausen, M., Gidden, M. J., Rogelj, J., Kriegler, E., Peters, G. P., Fuglestvedt, J. S., Skeie, R. B., Samset, B. H., Wienpahl, L., van Vuuren, D. P., van der Wijst, K.-I., Al Khourdajie, A., Forster, P. M., Reisinger, A., Schaeffer, R., and Riahi, K.: The IPCC Sixth Assessment Report WGIII climate assessment of mitigation pathways: from emissions to global temperatures, Geosci. Model Dev., 15, 9075–9109, https://doi.org/10.5194/gmd-15-9075-2022, 2022.
Kloenne, U., Nauels, A., Pearson, P., DeConto, R. M., Findlay, H. S., Hugelius, G., Robinson, A., Rogelj, J., Schuur, E. A. G., Stroeve, J., and Schleussner, C.-F.: Only halving emissions by 2030 can minimize risks of crossing cryosphere thresholds, Nat. Clim. Change, 13, 9–11, https://doi.org/10.1038/s41558-022-01566-4, 2022.
Koch, A., Brierley, C., and Lewis, S. L.: Effects of Earth system feedbacks on the potential mitigation of large-scale tropical forest restoration, Biogeosciences, 18, 2627–2647, https://doi.org/10.5194/bg-18-2627-2021, 2021.
Koven, C. D., Arora, V. K., Cadule, P., Fisher, R. A., Jones, C. D., Lawrence, D. M., Lewis, J., Lindsay, K., Mathesius, S., Meinshausen, M., Mills, M., Nicholls, Z., Sanderson, B. M., Séférian, R., Swart, N. C., Wieder, W. R., and Zickfeld, K.: Multi-century dynamics of the climate and carbon cycle under both high and net negative emissions scenarios, Earth Syst. Dynam., 13, 885–909, https://doi.org/10.5194/esd-13-885-2022, 2022.
Koven, C. D., Sanderson, B. M., and Swann, A. L. S.: Much of zero emissions commitment occurs before reaching net zero emissions, Environ. Res. Lett., 18, 014017, https://doi.org/10.1088/1748-9326/acab1a, 2023.
Kriegler, E., Bauer, N., Popp, A., Humpenöder, F., Leimbach, M., Strefler, J., Baumstark, L., Bodirsky, B. L., Hilaire, J., Klein, D., Mouratiadou, I., Weindl, I., Bertram, C., Dietrich, J.-P., Luderer, G., Pehl, M., Pietzcker, R., Piontek, F., Lotze-Campen, H., Biewald, A., Bonsch, M., Giannousakis, A., Kreidenweis, U., Müller, C., Rolinski, S., Schultes, A., Schwanitz, J., Stevanovic, M., Calvin, K., Emmerling, J., Fujimori, S., and Edenhofer, O.: Fossil-fueled development (SSP5): An energy and resource intensive scenario for the 21st century, Global Environ. Chang., 42, 297–315, https://doi.org/10.1016/j.gloenvcha.2016.05.015, 2017.
Kwiatkowski, L., Torres, O., Bopp, L., Aumont, O., Chamberlain, M., Christian, J. R., Dunne, J. P., Gehlen, M., Ilyina, T., John, J. G., Lenton, A., Li, H., Lovenduski, N. S., Orr, J. C., Palmieri, J., Santana-Falcón, Y., Schwinger, J., Séférian, R., Stock, C. A., Tagliabue, A., Takano, Y., Tjiputra, J., Toyama, K., Tsujino, H., Watanabe, M., Yamamoto, A., Yool, A., and Ziehn, T.: Twenty-first century ocean warming, acidification, deoxygenation, and upper-ocean nutrient and primary production decline from CMIP6 model projections, Biogeosciences, 17, 3439–3470, https://doi.org/10.5194/bg-17-3439-2020, 2020.
Lacroix, F., Ilyina, T., Mathis, M., Laruelle, G. G., and Regnier, P.: Historical increases in land-derived nutrient inputs may alleviate effects of a changing physical climate on the oceanic carbon cycle, Glob. Change Biol., 27, 5491–5513, https://doi.org/10.1111/gcb.15822, 2021.
Lauerwald, R., Regnier, P., Camino-Serrano, M., Guenet, B., Guimberteau, M., Ducharne, A., Polcher, J., and Ciais, P.: ORCHILEAK (revision 3875): a new model branch to simulate carbon transfers along the terrestrial–aquatic continuum of the Amazon basin, Geosci. Model Dev., 10, 3821–3859, https://doi.org/10.5194/gmd-10-3821-2017, 2017.
Lawrence, D. M., Hurtt, G. C., Arneth, A., Brovkin, V., Calvin, K. V., Jones, A. D., Jones, C. D., Lawrence, P. J., de Noblet-Ducoudré, N., Pongratz, J., Seneviratne, S. I., and Shevliakova, E.: The Land Use Model Intercomparison Project (LUMIP) contribution to CMIP6: rationale and experimental design, Geosci. Model Dev., 9, 2973–2998, https://doi.org/10.5194/gmd-9-2973-2016, 2016.
Lawrence Gates, W., Boyle, J. S., Covey, C., Dease, C. G., Doutriaux, C. M., Drach, R. S., Fiorino, M., Gleckler, P. J., Hnilo, J. J., Marlais, S. M., Phillips, T. J., Potter, G. L., Santer, B. D., Sperber, K. R., Taylor, K. E., and Williams, D. N.: An Overview of the Results of the Atmospheric Model Intercomparison Project (AMIP I), B. Am. Meteorol. Soc., 80, 29–56, https://doi.org/10.1175/1520-0477(1999)080<0029:AOOTRO>2.0.CO;2, 1999.
Lee, J.-Y., Marotzke, J., Bala, G., Cao, L., Corti, S., Dunne, J. P., Engelbrecht, F., Fischer, E., Fyfe, J. C., Jones, C., Maycock, A., Mutemi, J., Ndiaye, O., Panickal, S., and Zhou, T.: Future Global Climate: Scenario-Based Projections and Near-Term Information. In Climate Change 2021: The Physical Science Basis, Contribution of Working Group I to the Sixth Assessment Report of the Intergovernmental Panel on Climate Change, edited by: Masson-Delmotte, V., Zhai, P., Pirani, A., Connors, S. L., Péan, C., Berger, S., Caud, N., Chen, Y., Goldfarb, L., Gomis, M. I., Huang, M., Leitzell, K., Lonnoy, E., Matthews, J. B. R., Maycock, T. K., Waterfield, T., Yelekçi, O., Yu, R., and Zhou, B., Cambridge University Press, Cambridge, United Kingdom and New York, NY, USA, 553–672, https://doi.org/10.1017/9781009157896.006, 2021.
Lenton, T. M., Rockström, J., Gaffney, O., Rahmstorf, S., Richardson, K., Steffen, W., and Schellnhuber, H. J.: Climate tipping points – too risky to bet against, Nature, 575, 592–595, https://doi.org/10.1038/d41586-019-03595-0, 2019.
Li, H. and Ilyina, T.: Current and future decadal trends in the oceanic carbon uptake are dominated by internal variability, Geophys. Res. Lett., 45, 916–925, https://doi.org/10.1002/2017gl075370, 2018.
Li, H., Ilyina, T., Loughran, T., Spring, A., and Pongratz, J.: Reconstructions and predictions of the global carbon budget with an emission-driven Earth system model, Earth Syst. Dynam., 14, 101–119, https://doi.org/10.5194/esd-14-101-2023, 2023a.
Li, X., Feng, M., Ran, Y., Su, Y., Liu, F., Huang, C., Shen, H., Xiao, Q., Su, J., Yuan, S., and Guo, H.: Big Data in Earth system science and progress towards a digital twin, Nat. Rev. Earth Environ., 4, 319–332, https://doi.org/10.1038/s43017-023-00409-w, 2023b.
Liddicoat, S. K., Wiltshire, A. J., Jones, C. D., Arora, V. K., Brovkin, V., Cadule, P., Hajima, T., Lawrence, D. M., Pongratz, J., Schwinger, J., Séférian, R., Tjiputra, J. F., and Ziehn, T.: Compatible Fossil Fuel CO2 Emissions in the CMIP6 Earth System Models' Historical and Shared Socioeconomic Pathway Experiments of the Twenty-First Century, J. Climate, 34, 2853–2875, https://doi.org/10.1175/JCLI-D-19-0991.1, 2021.
Linardakis, L., Stemmler, I., Hanke, M., Ramme, L., Chegini, F., Ilyina, T., and Korn, P.: Improving scalability of Earth system models through coarse-grained component concurrency – a case study with the ICON v2.6.5 modelling system, Geosci. Model Dev., 15, 9157–9176, https://doi.org/10.5194/gmd-15-9157-2022, 2022.
Lindsay, K.: A Newton–Krylov solver for fast spin-up of online ocean tracers, Ocean Model., 1, 33–43, 2017.
Lindsay, K., Bonan, G. B., Doney, S. C., Hoffman, F. M., Lawrence, D. M., Long, M. C., Mahowald, N. M., Keith Moore, J., Randerson, J. T., and Thornton, P. E.: Preindustrial-control and twentieth-century carbon cycle experiments with the Earth System Model CESM1 (BGC), J. Climate, 15, 8981–9005, 2014.
Liu, L., Shawki, D., Voulgarakis, A., Kasoar, M., Samset, B. H., Myhre, G., Forster, P. M., Hodnebrog, Ø., Sillmann, J., Aalbergsjø, S. G., Boucher, O., Faluvegi, G., Iversen, T., Kirkevåg, A., Lamarque, J.-F., Olivié, D., Richardson, T., Shindell, D., and Takemura, T.: A PDRMIP multi-model study on the impacts of regional aerosol forcings on global and regional precipitation, J. Climate, 31, 4429–4447, https://doi.org/10.1175/JCLI-D-17-0439.1, 2018.
Lofverstrom, M., Fyke, J. G., Thayer-Calder, K., Muntjewerf, L., Vizcaino, M., Sacks, W. J., Lipscomb, W. H., Otto-Bliesner, B. L., and Bradley, S. L.: An Efficient Ice Sheet/Earth System Model Spin-up Procedure for CESM2-CISM2: Description, Evaluation, and Broader Applicability, J. Adv. Model Earth Sy., 12, e2019MS001984, https://doi.org/10.1029/2019MS001984, 2020.
Loughran, T. F., Ziehn, T., Law, R., Canadell, J. G., Pongratz, J., Liddicoat, S., Hajima, T., Ito, A., Lawrence, D. M., and Arora, V. K.: Limited mitigation potential of forestation under a high emissions scenario: Results from multi-model and single model ensembles, J. Geophys. Res. Biogeo., 128, e2023JG007605, https://doi.org/10.1029/2023jg007605, 2023.
Lovenduski, N. S., McKinley, G. A., Fay, A. R., Lindsay, K., and Long, M. C.: Partitioning uncertainty in ocean carbon uptake projections: Internal variability, emission scenario, and model structure, Global Biogeochem. Cy., 30, 1276–1287, https://doi.org/10.1002/2016gb005426, 2016.
Lu, X., Du, Z., Huang, Z., Lawrence, D., Kluzek, E., Collier, N., Lombardozzi, D., Sobhani, N., Schuur, E. A. G., and Luo, Y.: Full implementation of matrix approach to biogeochemistry module of CLM5, J. Adv. Model. Earth Sy., 12, e2020MS002105, https://doi.org/10.1029/2020MS002105, 2020.
Luyssaert, S., Marie, G., Valade, A., Chen, Y.-Y., Njakou Djomo, S., Ryder, J., Otto, J., Naudts, K., Lansø, A. S., Ghattas, J., and McGrath, M. J.: Trade-offs in using European forests to meet climate objectives, Nature, 562, 259–262, https://doi.org/10.1038/s41586-018-0577-1, 2018.
Lwasa, S., Buyana, K., Kasaija, P., and Mutyaba, J.: Scenarios for adaptation and mitigation in urban Africa under 1.5 °C global warming, Curr. Opin. Env. Sust., 30, 52–58, https://doi.org/10.1016/j.cosust.2018.02.012, 2018.
MacDougall, A. H., Frölicher, T. L., Jones, C. D., Rogelj, J., Matthews, H. D., Zickfeld, K., Arora, V. K., Barrett, N. J., Brovkin, V., Burger, F. A., Eby, M., Eliseev, A. V., Hajima, T., Holden, P. B., Jeltsch-Thömmes, A., Koven, C., Mengis, N., Menviel, L., Michou, M., Mokhov, I. I., Oka, A., Schwinger, J., Séférian, R., Shaffer, G., Sokolov, A., Tachiiri, K., Tjiputra , J., Wiltshire, A., and Ziehn, T.: Is there warming in the pipeline? A multi-model analysis of the Zero Emissions Commitment from CO2, Biogeosciences, 17, 2987–3016, https://doi.org/10.5194/bg-17-2987-2020, 2020.
Marcucci, A., Panos, E., Kypreos, S., and Fragkos, P.: Probabilistic assessment of realizing the 1.5 °C climate target, Appl. Energy, 239, 239–251, https://doi.org/10.1016/j.apenergy.2019.01.190, 2019.
Martin, T., Biastoch, A., Lohmann, G., Mikolajewicz, U., and Wang, X.: On timescales and reversibility of the ocean's response to enhanced Greenland ice sheet melting in comprehensive climate models, Geophys. Res. Lett., 49, e2021GL097114, https://doi.org/10.1029/2021gl097114, 2022.
Masson-Delmotte, V., Zhai, P., Pirani, A., Connors, S. L., Péan, C., Berger, S., Caud, N., Chen, Y., Goldfarb, L., Gomis, M. I., Huang, M., Leitzell, K., Lonnoy, E., Matthews, J. B. R., Maycock, T., Waterfield, T., Yelekçi, O., Yu, R., and Zhou, B. (Eds.): Climate Change 2021 – the physical science basis: Working Group I contribution to the Sixth Assessment Report of the Intergovernmental Panel on Climate Change, Cambridge University Press, https://doi.org/10.1017/9781009157896, 2023.
Mathis, M., Logemann, K., Maerz, J., Lacroix, F., Hagemann, S., Chegini, F., Ramme, L., Ilyina, T., Korn, P., and Schrum, C.: Seamless integration of the coastal ocean in global marine carbon cycle modeling, J. Adv. Model. Earth Sy., 14, e2021MS002789, https://doi.org/10.1029/2021ms002789, 2022.
Matthews, H. D., Gillett, N. P., Stott, P. A., and Zickfeld, K.: The proportionality of global warming to cumulative carbon emissions, Nature, 459, 829–832, https://doi.org/10.1038/nature08047, 2009.
McDowell, N. G. and Allen, C. D.: Darcy's law predicts widespread forest mortality under climate warming, Nat. Clim. Change, 5, 669–672, https://doi.org/10.1038/nclimate2641, 2015.
McDowell, N. G., Allen, C. D., Anderson-Teixeira, K., Aukema, B. H., Bond-Lamberty, B., Chini, L., Clark, J. S., Dietze, M., Grossiord, C., Hanbury-Brown, A., Hurtt, G. C., Jackson, R. B., Johnson, D. J., Kueppers, L., Lichstein, J. W., Ogle, K., Poulter, B., Pugh, T. A. M., Seidl, R., Turner, M. G., Uriarte, M., Walker, A. P., and Xu, C.: Pervasive shifts in forest dynamics in a changing world, Science, 368, eaaz9463, https://doi.org/10.1126/science.aaz9463, 2020.
McKenna, C. M., Maycock, A. C., Forster, P. M., Smith, C. J., and Tokarska, K. B.: Stringent mitigation substantially reduces risk of unprecedented near-term warming rates, Nat. Clim. Change, 11, 126–131, https://doi.org/10.1038/s41558-020-00957-9, 2020.
Meehl, G. A., Covey, C., Delworth, T., Latif, M., McAvaney, B., Mitchell, J. F. B., Stouffer, R. J., and Taylor, K. E.: THE WCRP CMIP3 Multimodel Dataset: A New Era in Climate Change Research, B. Am. Meteorol. Soc., 88, 1383–1394, https://doi.org/10.1175/BAMS-88-9-1383, 2007.
Meinshausen, M., Wigley, T. M. L., and Raper, S. C. B.: Emulating atmosphere-ocean and carbon cycle models with a simpler model, MAGICC6 – Part 2: Applications, Atmos. Chem. Phys., 11, 1457–1471, https://doi.org/10.5194/acp-11-1457-2011, 2011.
Meinshausen, M., Nicholls, Z. R. J., Lewis, J., Gidden, M. J., Vogel, E., Freund, M., Beyerle, U., Gessner, C., Nauels, A., Bauer, N., Canadell, J. G., Daniel, J. S., John, A., Krummel, P. B., Luderer, G., Meinshausen, N., Montzka, S. A., Rayner, P. J., Reimann, S., Smith, S. J., van den Berg, M., Velders, G. J. M., Vollmer, M. K., and Wang, R. H. J.: The shared socio-economic pathway (SSP) greenhouse gas concentrations and their extensions to 2500, Geosci. Model Dev., 13, 3571–3605, https://doi.org/10.5194/gmd-13-3571-2020, 2020.
Meinshausen, M., Schleussner, C.-F., Beyer, K., Bodeker, G., Boucher, O., Canadell, J. G., Daniel, J. S., Diongue-Niang, A., Driouech, F., Fischer, E., Forster, P., Grose, M., Hansen, G., Hausfather, Z., Ilyina, T., Kikstra, J. S., Kimutai, J., King, A. D., Lee, J.-Y., Lennard, C., Lissner, T., Nauels, A., Peters, G. P., Pirani, A., Plattner, G.-K., Pörtner, H., Rogelj, J., Rojas, M., Roy, J., Samset, B. H., Sanderson, B. M., Séférian, R., Seneviratne, S., Smith, C. J., Szopa, S., Thomas, A., Urge-Vorsatz, D., Velders, G. J. M., Yokohata, T., Ziehn, T., and Nicholls, Z.: A perspective on the next generation of Earth system model scenarios: towards representative emission pathways (REPs), Geosci. Model Dev., 17, 4533–4559, https://doi.org/10.5194/gmd-17-4533-2024, 2024a.
Meinshausen, M., Nicholls, Z. R., Lewis, J., Gidden, M. J., Vogel, E., Freund, M., Beyerle, U., Gessner, C., Nauels, A., Bauer, N., and Canadell, J. G.: Greenhouse Gas Factsheets, https://greenhousegases.science.unimelb.edu.au/, last access: 30 October 2024b.
Melnikova, I., Boucher, O., Cadule, P., Tanaka, K., Gasser, T., Hajima, T., Quilcaille, Y., Shiogama, H., Séférian, R., Tachiiri, K., Vuichard, N., Yokohata, T., and Ciais, P.: Impact of bioenergy crop expansion on climate–carbon cycle feedbacks in overshoot scenarios, Earth Syst. Dynam., 13, 779–794, https://doi.org/10.5194/esd-13-779-2022, 2022.
Melnikova, I., Ciais, P., Tanaka, K., Vuichard, N., and Boucher, O.: Relative benefits of allocating land to bioenergy crops and forests vary by region, Commun. Earth Environ., 4, 1–12, https://doi.org/10.1038/s43247-023-00866-7, 2023.
Mendez, A. and Farazmand, M.: Investigating climate tipping points under various emission reduction and carbon capture scenarios with a stochastic climate model, Proc. Roy. Soc. A, 477, 20210697, https://doi.org/10.1098/rspa.2021.0697, 2021.
Mengis, N., Keller, D. P., Rickels, W., Quaas, M., and Oschlies, A.: Climate engineering–induced changes in correlations between Earth system variables – implications for appropriate indicator selection, Clim. Change, 153, 305–322, https://doi.org/10.1007/s10584-019-02389-7, 2019.
Millar, R. J., Fuglestvedt, J. S., Friedlingstein, P., Rogelj, J., Grubb, M. J., Matthews, H. D., Skeie, R. B., Forster, P. M., Frame, D. J., and Allen, M. R.: Emission budgets and pathways consistent with limiting warming to 1.5 °C, Nat. Geosci., 10, 741–747, https://doi.org/10.1038/ngeo3031, 2017.
Moss, R. H., Edmonds, J. A., Hibbard, K. A., Manning, M. R., Rose, S. K., van Vuuren, D. P., Carter, T. R., Emori, S., Kainuma, M., Kram, T., Meehl, G. A., Mitchell, J. F. B., Nakicenovic, N., Riahi, K., Smith, S. J., Stouffer, R. J., Thomson, A. M., Weyant, J. P., and Wilbanks, T. J.: The next generation of scenarios for climate change research and assessment, Nature, 463, 747–756, https://doi.org/10.1038/nature08823, 2010.
Muri, H.: The role of largeescale BECCS in the pursuit of the 1.5 °C target: an Earth system model perspective, Environ. Res. Lett., 13, 044010, https://doi.org/10.1088/1748-9326/aab324, 2018.
Nakicenovic, N., Alcamo, J., Davis, G., de Vries, B., Fenhann, J. V., Gaffin, S., Gregory, K., Grübler, A., Jung, T. Y., Kram, T., La Rovere, E. L., Michaelis, L., Mori, S., Morita, T., Pepper, W., Pitcher, H., Price, L., Riahi, K., Roehrl, A., Rogner, H.-H., Sankovski, A., Schlesinger, M., Shukla, P., Smith, S., Swart, R., van Rooijen, S., Victor, N., and Dadi, Z.: Special report on emissions scenarios: A special report of working group III of the intergovernmental panel on climate change, edited by: Nakicenovic, N. and Swart, R., Cambridge University Press, Cambridge, England, 612 pp., ISBN 0 521 80081 1, 2000.
Nauels, A., Rogelj, J., Schleussner, C.-F., Meinshausen, M., and Mengel, M.: Linking sea level rise and socioeconomic indicators under the Shared Socioeconomic Pathways, Environ. Res. Lett., 12, 114002, https://doi.org/10.1088/1748-9326/aa92b6, 2017.
Naveau, P., Hannart, A., and Ribes, A.: Statistical methods for extreme event attribution in climate science, Annu. Rev. Stat. Appl., 7, 89–110, https://doi.org/10.1146/annurev-statistics-031219-041314, 2020.
Nicholls, Z., Meinshausen, M., Lewis, J., Smith, C. J., Forster, P. M., Fuglestvedt, J. S., Rogelj, J., Kikstra, J. S., Riahi, K., and Byers, E.: Changes in IPCC Scenario Assessment Emulators Between SR1.5 and AR6 Unraveled, Geophys. Res. Lett., 49, e2022GL099788, https://doi.org/10.1029/2022GL099788, 2022.
Nielsen, D. M., Pieper, P., Barkhordarian, A., Overduin, P., Ilyina, T., Brovkin, V., Baehr, J., and Dobrynin, M.: Increase in Arctic coastal erosion and its sensitivity to warming in the twenty-first century, Nat. Clim. Change, 12, 263–270, https://doi.org/10.1038/s41558-022-01281-0, 2022.
Nieto, M.: Whatever it Takes to Reach Net Zero Emissions Around 2050 and Limit Global Warming to 1.5c: The Cases of United States, China, European Union and Japan (January 13, 2022), BAFFI CAREFIN Centre Research Paper No. 2022-170, https://doi.org/10.2139/ssrn.4007974, 2022.
O'Neill, B. C., Tebaldi, C., van Vuuren, D. P., Eyring, V., Friedlingstein, P., Hurtt, G., Knutti, R., Kriegler, E., Lamarque, J.-F., Lowe, J., Meehl, G. A., Moss, R., Riahi, K., and Sanderson, B. M.: The Scenario Model Intercomparison Project (ScenarioMIP) for CMIP6, Geosci. Model Dev., 9, 3461–3482, https://doi.org/10.5194/gmd-9-3461-2016, 2016.
O'Sullivan, M., Zhang, Y., Bellouin, N., Harris, I., Mercado, L. M., Sitch, S., Ciais, P., and Friedlingstein, P.: Aerosol–light interactions reduce the carbon budget imbalance, Environ. Res. Lett., 16, 124072, https://doi.org/10.1088/1748-9326/ac3b77, 2021.
Peters, G. P., Le Quéré, C., Andrew, R. M., Canadell, J. G., Friedlingstein, P., Ilyina, T., Jackson, R. B., Joos, F., Korsbakken, J. I., McKinley, G. A., Sitch, S., and Tans, P.: Towards real-time verification of CO2 emissions, Nat. Clim. Change, 7, 848–850, https://doi.org/10.1038/s41558-017-0013-9, 2017.
Pfleiderer, P., Frölicher, T., Kropf, C., Lamboll, R., Lejeune, Q., Lourenco, T., McCaughey, J., Quilcaille, Y., Rogelj, J., Sanderson, B., Smith, C., Sillmann, J., Theokritoff, E., and Schleussner, C.-F.: Reversal of the impact chain for actionable climate information, EarthArXiv [preprint], https://doi.org/10.31223/x5r088, 2023.
Pincus, R., Forster, P. M., and Stevens, B.: The Radiative Forcing Model Intercomparison Project (RFMIP): experimental protocol for CMIP6, Geosci. Model Dev., 9, 3447–3460, https://doi.org/10.5194/gmd-9-3447-2016, 2016.
Pirani, A., Fuglestvedt, J. S., Byers, E., O'Neill, B., Riahi, K., Lee, J.-Y., Marotzke, J., Rose, S. K., Schaeffer, R., and Tebaldi, C.: Scenarios in IPCC assessments: lessons from AR6 and opportunities for AR7, npj Climate Action, 3, 1–7, https://doi.org/10.1038/s44168-023-00082-1, 2024.
Psarras, P., Krutka, H., Fajardy, M., Zhang, Z., Liguori, S., Dowell, N. M., and Wilcox, J.: Slicing the pie: how big could carbon dioxide removal be?, Wiley Interdiscip. Rev. Energy Environ., 6, e253, https://doi.org/10.1002/wene.253, 2017.
Quesada, B., Arneth, A., Robertson, E., and de Noblet-Ducoudré, N.: Potential strong contribution of future anthropogenic land-use and land-cover change to the terrestrial carbon cycle, Environ. Res. Lett., 13, 064023, https://doi.org/10.1088/1748-9326/aac4c3, 2018.
Quilcaille, Y., Gasser, T., Ciais, P., and Boucher, O.: CMIP6 simulations with the compact Earth system model OSCAR v3.1, Geosci. Model Dev., 16, 1129–1161, https://doi.org/10.5194/gmd-16-1129-2023, 2023.
Reynolds, J. L.: Is solar geoengineering ungovernable? A critical assessment of governance challenges identified by the Intergovernmental Panel on Climate Change, Wires. Clim. Change, 12, e690, https://doi.org/10.1002/wcc.690, 2021.
Riahi, K., van Vuuren, D. P., Kriegler, E., Edmonds, J., O'Neill, B. C., Fujimori, S., Bauer, N., Calvin, K., Dellink, R., Fricko, O., Lutz, W., Popp, A., Cuaresma, J. C., Kc, S., Leimbach, M., Jiang, L., Kram, T., Rao, S., Emmerling, J., Ebi, K., Hasegawa, T., Havlik, P., Humpenöder, F., Da Silva, L. A., Smith, S., Stehfest, E., Bosetti, V., Eom, J., Gernaat, D., Masui, T., Rogelj, J., Strefler, J., Drouet, L., Krey, V., Luderer, G., Harmsen, M., Takahashi, K., Baumstark, L., Doelman, J. C., Kainuma, M., Klimont, Z., Marangoni, G., Lotze-Campen, H., Obersteiner, M., Tabeau, A., and Tavoni, M.: The Shared Socioeconomic Pathways and their energy, land use, and greenhouse gas emissions implications: An overview, Global Environ. Chang., 42, 153–168, https://doi.org/10.1016/j.gloenvcha.2016.05.009, 2017.
Ribes, A., Qasmi, S., and Gillett, N. P.: Making climate projections conditional on historical observations, Sci. Adv., 7, eabc0671, https://doi.org/10.1126/sciadv.abc0671, 2021.
Roberts, C. D., Senan, R., Molteni, F., Boussetta, S., Mayer, M., and Keeley, S. P. E.: Climate model configurations of the ECMWF Integrated Forecasting System (ECMWF-IFS cycle 43r1) for HighResMIP, Geosci. Model Dev., 11, 3681–3712, https://doi.org/10.5194/gmd-11-3681-2018, 2018.
Rogelj, J., Meinshausen, M., Schaeffer, M., Knutti, R., and Riahi, K.: Impact of short-lived non-CO2 mitigation on carbon budgets for stabilizing global warming, Environ. Res. Lett., 10, 075001, https://doi.org/10.1088/1748-9326/10/7/075001, 2015.
Rogelj, J., Huppmann, D., Krey, V., Riahi, K., Clarke, L., Gidden, M., Nicholls, Z., and Meinshausen, M.: A new scenario logic for the Paris Agreement long-term temperature goal, Nature, 573, 357–363, https://doi.org/10.1038/s41586-019-1541-4, 2019.
Samset, B. H., Lund, M. T., Bollasina, M., Myhre, G., and Wilcox, L.: Emerging Asian aerosol patterns, Nat. Geosci., 12, 582–584, https://doi.org/10.1038/s41561-019-0424-5, 2019.
Samset, B. H., Zhou, C., Fuglestvedt, J. S., Lund, M. T., Marotzke, J., and Zelinka, M. D.: Earlier emergence of a temperature response to mitigation by filtering annual variability, Nat. Commun., 13, 1578, https://doi.org/10.1038/s41467-022-29247-y, 2022.
Sanderson, B.: benmsanderson/esmperspective: GMD revised manuscript (0.2), Zenodo [code], https://doi.org/10.5281/zenodo.12515964, 2024.
Sanderson, B. M., O'Neill, B. C., and Tebaldi, C.: What would it take to achieve the Paris temperature targets?, Geophys. Res. Lett., 43, 7133–7142, https://doi.org/10.1002/2016gl069563, 2016.
Sanderson, B. M., Xu, Y., Tebaldi, C., Wehner, M., O'Neill, B., Jahn, A., Pendergrass, A. G., Lehner, F., Strand, W. G., Lin, L., Knutti, R., and Lamarque, J. F.: Community climate simulations to assess avoided impacts in 1.5 and 2 °C futures, Earth Syst. Dynam., 8, 827–847, https://doi.org/10.5194/esd-8-827-2017, 2017a.
Sanderson, B. M., Wehner, M., and Knutti, R.: Skill and independence weighting for multi-model assessments, Geosci. Model Dev., 10, 2379–2395, https://doi.org/10.5194/gmd-10-2379-2017, 2017b.
Schär, C., Fuhrer, O., Arteaga, A., Ban, N., Charpilloz, C., Di Girolamo, S., Hentgen, L., Hoefler, T., Lapillonne, X., Leutwyler, D., Osterried, K., Panosetti, D., Rüdisühli, S., Schlemmer, L., Schulthess, T. C., Sprenger, M., Ubbiali, S., and Wernli, H.: Kilometer-Scale Climate Models: Prospects and Challenges, B. Am. Meteorol. Soc., 101, E567–E587, https://doi.org/10.1175/BAMS-D-18-0167.1, 2020.
Séférian, R., Gehlen, M., Bopp, L., Resplandy, L., Orr, J. C., Marti, O., Dunne, J. P., Christian, J. R., Doney, S. C., Ilyina, T., Lindsay, K., Halloran, P. R., Heinze, C., Segschneider, J., Tjiputra, J., Aumont, O., and Romanou, A.: Inconsistent strategies to spin up models in CMIP5: implications for ocean biogeochemical model performance assessment, Geosci. Model Dev., 9, 1827–1851, https://doi.org/10.5194/gmd-9-1827-2016, 2016.
Séférian, R., Berthet, S., Yool, A., Palmiéri, J., Bopp, L., Tagliabue, A., Kwiatkowski, L., Aumont, O., Christian, J., Dunne, J., Gehlen, M., Ilyina, T., John, J. G., Li, H., Long, M. C., Luo, J. Y., Nakano, H., Romanou, A., Schwinger, J., Stock, C., Santana-Falcón, Y., Takano, Y., Tjiputra, J., Tsujino, H., Watanabe, M., Wu, T., Wu, F., and Yamamoto, A.: Tracking Improvement in Simulated Marine Biogeochemistry Between CMIP5 and CMIP6, Curr. Clim. Change Rep., 6, 95–119, https://doi.org/10.1007/s40641-020-00160-0, 2020.
Silvy, Y., Frölicher, T. L., Terhaar, J., Joos, F., Burger, F. A., Lacroix, F., Allen, M., Bernadello, R., Bopp, L., Brovkin, V., Buzan, J. R., Cadule, P., Dix, M., Dunne, J., Friedlingstein, P., Georgievski, G., Hajima, T., Jenkins, S., Kawamiya, M., Kiang, N. Y., Lapin, V., Lee, D., Lerner, P., Mengis, N., Monteiro, E. A., Paynter, D., Peters, G. P., Romanou, A., Schwinger, J., Sparrow, S., Stofferahn, E., Tjiputra, J., Tourigny, E., and Ziehn, T.: AERA-MIP: Emission pathways, remaining budgets and carbon cycle dynamics compatible with 1.5 ºC and 2 ºC global warming stabilization, EGUsphere [preprint], https://doi.org/10.5194/egusphere-2024-488, 2024.
Singh, T., Counillon, F., Tjiputra, J., Wang, Y., and Gharamti, M. E.: Estimation of ocean biogeochemical parameters in an earth system model using the dual one step ahead smoother: A twin experiment, Front. Mar. Sci., 9, 775394, https://doi.org/10.3389/fmars.2022.775394, 2022.
Smith, C., Cummins, D. P., Fredriksen, H.-B., Nicholls, Z., Meinshausen, M., Allen, M., Jenkins, S., Leach, N., Mathison, C., and Partanen, A.-I.: fair-calibrate v1.4.1: calibration, constraining and validation of the FaIR simple climate model for reliable future climate projections, EGUsphere [preprint], https://doi.org/10.5194/egusphere-2024-708, 2024.
Smith, R. S., Mathiot, P., Siahaan, A., Lee, V., Cornford, S. L., Gregory, J. M., Payne, A. J., Jenkins, A., Holland, P. R., Ridley, J. K., and Jones, C. G.: Coupling the U.k. earth System model to dynamic models of the Greenland and Antarctic ice sheets, J. Adv. Model. Earth Sy., 13, e2021MS002520, https://doi.org/10.1029/2021ms002520, 2021.
Sognnaes, I., Gambhir, A., van de Ven, D.-J., Nikas, A., Anger-Kraavi, A., Bui, H., Campagnolo, L., Delpiazzo, E., Doukas, H., Giarola, S., Grant, N., Hawkes, A., Köberle, A. C., Kolpakov, A., Mittal, S., Moreno, J., Perdana, S., Rogelj, J., Vielle, M., and Peters, G. P.: A multi-model analysis of long-term emissions and warming implications of current mitigation efforts, Nat. Clim. Change, 11, 1055–1062, https://doi.org/10.1038/s41558-021-01206-3, 2021.
Sonntag, S., Ferrer González, M., Ilyina, T., Kracher, D., Nabel, J. E. M. S., Niemeier, U., Pongratz, J., Reick, C. H., and Schmidt, H.: Quantifying and comparing effects of climate engineering methods on the earth system, Earths Future, 6, 149–168, https://doi.org/10.1002/2017ef000620, 2018.
Sovacool, B. K.: Reckless or righteous? Reviewing the sociotechnical benefits and risks of climate change geoengineering, Energy Strateg. Rev., 35, 100656, https://doi.org/10.1016/j.esr.2021.100656, 2021.
Spring, A., Ilyina, T., and Marotzke, J.: Inherent uncertainty disguises attribution of reduced atmospheric CO2 growth to CO2 emission reductions for up to a decade, Environ. Res. Lett., 15, 114058, https://doi.org/10.1088/1748-9326/abc443, 2020.
Stevanović, M., Popp, A., Lotze-Campen, H., Dietrich, J. P., Müller, C., Bonsch, M., Schmitz, C., Bodirsky, B. L., Humpenöder, F., and Weindl, I.: The impact of high-end climate change on agricultural welfare, Sci. Adv., 2, e1501452, https://doi.org/10.1126/sciadv.1501452, 2016.
Stevens, B., Fiedler, S., Kinne, S., Peters, K., Rast, S., Müsse, J., Smith, S. J., and Mauritsen, T.: MACv2-SP: a parameterization of anthropogenic aerosol optical properties and an associated Twomey effect for use in CMIP6, Geosci. Model Dev., 10, 433–452, https://doi.org/10.5194/gmd-10-433-2017, 2017.
Stevens, B., Satoh, M., Auger, L., Biercamp, J., Bretherton, C. S., Chen, X., Düben, P., Judt, F., Khairoutdinov, M., Klocke, D., Kodama, C., Kornblueh, L., Lin, S.-J., Neumann, P., Putman, W. M., Röber, N., Shibuya, R., Vanniere, B., Vidale, P. L., Wedi, N., and Zhou, L.: DYAMOND: the DYnamics of the Atmospheric general circulation Modeled On Non-hydrostatic Domains, Prog. Earth Planet. Sci., 6, 61, https://doi.org/10.1186/s40645-019-0304-z, 2019.
Sun, Y., Goll, D. S., Huang, Y., Ciais, P., Wang, Y.-P., Bastrikov, V., and Wang, Y.: Machine learning for accelerating process‐based computation of land biogeochemical cycles, Glob. Change Biol., 29, 3221–3234, 2023.
Taylor, K. E., Stouffer, R. J., and Meehl, G. A.: An Overview of CMIP5 and the Experiment Design, B. Am. Meteorol. Soc., 93, 485–498, https://doi.org/10.1175/BAMS-D-11-00094.1, 2012.
Tebaldi, C. and Friedlingstein, P.: Delayed detection of climate mitigation benefits due to climate inertia and variability, P. Natl. Acad. Sci. USA, 110, 17229–17234, https://doi.org/10.1073/pnas.1300005110, 2013.
Tebaldi, C., Debeire, K., Eyring, V., Fischer, E., Fyfe, J., Friedlingstein, P., Knutti, R., Lowe, J., O'Neill, B., Sanderson, B., van Vuuren, D., Riahi, K., Meinshausen, M., Nicholls, Z., Tokarska, K. B., Hurtt, G., Kriegler, E., Lamarque, J.-F., Meehl, G., Moss, R., Bauer, S. E., Boucher, O., Brovkin, V., Byun, Y.-H., Dix, M., Gualdi, S., Guo, H., John, J. G., Kharin, S., Kim, Y., Koshiro, T., Ma, L., Olivié, D., Panickal, S., Qiao, F., Rong, X., Rosenbloom, N., Schupfner, M., Séférian, R., Sellar, A., Semmler, T., Shi, X., Song, Z., Steger, C., Stouffer, R., Swart, N., Tachiiri, K., Tang, Q., Tatebe, H., Voldoire, A., Volodin, E., Wyser, K., Xin, X., Yang, S., Yu, Y., and Ziehn, T.: Climate model projections from the Scenario Model Intercomparison Project (ScenarioMIP) of CMIP6, Earth Syst. Dynam., 12, 253–293, https://doi.org/10.5194/esd-12-253-2021, 2021.
Terhaar, J., Frölicher, T. L., Aschwanden, M. T., Friedlingstein, P., and Joos, F.: Adaptive emission reduction approach to reach any global warming target, Nat. Clim. Change, 12, 1136–1142, https://doi.org/10.1038/s41558-022-01537-9, 2022.
Terhaar, J., Frölicher, T. L., and Joos, F.: Ocean acidification in emission-driven temperature stabilization scenarios: the role of TCRE and non-CO2 greenhouse gases, Environ. Res. Lett., 18, 024033, https://doi.org/10.1088/1748-9326/acaf91, 2023.
The Scenario Model Intercomparison Project (ScenarioMIP) for CMIP7: https://wcrp-cmip.org/wp-content/uploads/2024/04/24-04-15_ScenarioMIP-CMIP7-proposal_final.pdf, last access: 14 June 2024.
Thornhill, G., Collins, W., Olivié, D., Skeie, R. B., Archibald, A., Bauer, S., Checa-Garcia, R., Fiedler, S., Folberth, G., Gjermundsen, A., Horowitz, L., Lamarque, J.-F., Michou, M., Mulcahy, J., Nabat, P., Naik, V., O'Connor, F. M., Paulot, F., Schulz, M., Scott, C. E., Séférian, R., Smith, C., Takemura, T., Tilmes, S., Tsigaridis, K., and Weber, J.: Climate-driven chemistry and aerosol feedbacks in CMIP6 Earth system models, Atmos. Chem. Phys., 21, 1105–1126, https://doi.org/10.5194/acp-21-1105-2021, 2021.
Travis, W. R., Smith, J. B., and Yohe, G. W.: Moving toward 1.5 °C of warming: implications for climate adaptation strategies, Curr. Opin. Env. Sust., 31, 146–152, https://doi.org/10.1016/j.cosust.2018.03.003, 2018.
van den Hurk, B., Kim, H., Krinner, G., Seneviratne, S. I., Derksen, C., Oki, T., Douville, H., Colin, J., Ducharne, A., Cheruy, F., Viovy, N., Puma, M. J., Wada, Y., Li, W., Jia, B., Alessandri, A., Lawrence, D. M., Weedon, G. P., Ellis, R., Hagemann, S., Mao, J., Flanner, M. G., Zampieri, M., Materia, S., Law, R. M., and Sheffield, J.: LS3MIP (v1.0) contribution to CMIP6: the Land Surface, Snow and Soil moisture Model Intercomparison Project – aims, setup and expected outcome, Geosci. Model Dev., 9, 2809–2832, https://doi.org/10.5194/gmd-9-2809-2016, 2016.
van Vuuren, D. P., Edmonds, J., Kainuma, M., Riahi, K., Thomson, A., Hibbard, K., Hurtt, G. C., Kram, T., Krey, V., Lamarque, J.-F., Masui, T., Meinshausen, M., Nakicenovic, N., Smith, S. J., and Rose, S. K.: The representative concentration pathways: an overview, Clim. Change, 109, 5–31, https://doi.org/10.1007/s10584-011-0148-z, 2011.
van Vuuren, D. P., Kok, M., Lucas, P. L., Prins, A. G., Alkemade, R., van den Berg, M., Bouwman, L., van der Esch, S., Jeuken, M., Kram, T., and Stehfest, E.: Pathways to achieve a set of ambitious global sustainability objectives by 2050: Explorations using the IMAGE integrated assessment model, Technol. Forecast. Soc. Change, 98, 303–323, https://doi.org/10.1016/j.techfore.2015.03.005, 2015.
van Vuuren, D. P., Stehfest, E., Gernaat, D. E. H. J., Doelman, J. C., van den Berg, M., Harmsen, M., de Boer, H. S., Bouwman, L. F., Daioglou, V., Edelenbosch, O. Y., Girod, B., Kram, T., Lassaletta, L., Lucas, P. L., van Meijl, H., Müller, C., van Ruijven, B. J., van der Sluis, S., and Tabeau, A.: Energy, land-use and greenhouse gas emissions trajectories under a green growth paradigm, Global Environ. Chang., 42, 237–250, https://doi.org/10.1016/j.gloenvcha.2016.05.008, 2017.
Watson-Parris, D.: Machine learning for weather and climate are worlds apart, Philos. T. A Math. Phys. Eng. Sci., 379, 20200098, https://doi.org/10.1098/rsta.2020.0098, 2021.
Watson-Parris, D. and Smith, C. J.: Large uncertainty in future warming due to aerosol forcing, Nat. Clim. Change, 12, 1111–1113, https://doi.org/10.1038/s41558-022-01516-0, 2022.
Wiebe, K., Lotze-Campen, H., Sands, R., Tabeau, A., van der Mensbrugghe, D., Biewald, A., Bodirsky, B., Islam, S., Kavallari, A., Mason-D'Croz, D., Müller, C., Popp, A., Robertson, R., Robinson, S., van Meijl, H., and Willenbockel, D.: Climate change impacts on agriculture in 2050 under a range of plausible socioeconomic and emissions scenarios, Environ. Res. Lett., 10, 085010, https://doi.org/10.1088/1748-9326/10/8/085010, 2015.
Wilcox, L. J., Allen, R. J., Samset, B. H., Bollasina, M. A., Griffiths, P. T., Keeble, J., Lund, M. T., Makkonen, R., Merikanto, J., O'Donnell, D., Paynter, D. J., Persad, G. G., Rumbold, S. T., Takemura, T., Tsigaridis, K., Undorf, S., and Westervelt, D. M.: The Regional Aerosol Model Intercomparison Project (RAMIP), Geosci. Model Dev., 16, 4451–4479, https://doi.org/10.5194/gmd-16-4451-2023, 2023.
Wilkenskjeld, S., Kloster, S., Pongratz, J., Raddatz, T., and Reick, C. H.: Comparing the influence of net and gross anthropogenic land-use and land-cover changes on the carbon cycle in the MPI-ESM, Biogeosciences, 11, 4817–4828, https://doi.org/10.5194/bg-11-4817-2014, 2014.
Wu, J., Keller, D. P., and Oschlies, A.: Carbon dioxide removal via macroalgae open-ocean mariculture and sinking: an Earth system modeling study, Earth Syst. Dynam., 14, 185–221, https://doi.org/10.5194/esd-14-185-2023, 2023.
Wu, P., Ridley, J., Pardaens, A., Levine, R., and Lowe, J.: The reversibility of CO2 induced climate change, Clim. Dynam., 45, 745–754, https://doi.org/10.1007/s00382-014-2302-6, 2015.
Xu-Ri, Prentice, I. C., Spahni, R., and Niu, H. S.: Modelling terrestrial nitrous oxide emissions and implications for climate feedback, New Phytol., 196, 472–488, https://doi.org/10.1111/j.1469-8137.2012.04269.x, 2012.
Yamazaki, K., Sexton, D. M., Rostron, J. W., McSweeney, C. F., Murphy, J. M., and Harris, G. R.: A perturbed parameter ensemble of HadGEM3-GC3. 05 coupled model projections: part 2: global performance and future changes, Clim. Dynam., 56, 3437–3471, 2021.
Yool, A., Palmiéri, J., Jones, C. G., Sellar, A. A., De Mora, L., Kuhlbrodt, T., Popova, E. E., Mulcahy, J. P., Wiltshire, A., Rumbold, S. T.,and Stringer, M.: Spin‐up of UK earth system model 1 (UKESM1) for CMIP6, J. Adv. Model. Earth Sy., 12, e2019MS001933, https://doi.org/10.1029/2019MS001933, 2020.
Zarnetske, P. L., Gurevitch, J., Franklin, J., Groffman, P. M., Harrison, C. S., Hellmann, J. J., Hoffman, F. M., Kothari, S., Robock, A., Tilmes, S., Visioni, D., Wu, J., Xia, L., and Yang, C.-E.: Potential ecological impacts of climate intervention by reflecting sunlight to cool Earth, P. Natl. Acad. Sci. USA, 118, e1921854118, https://doi.org/10.1073/pnas.1921854118, 2021.
Zhang, Y., Ciais, P., Boucher, O., Maignan, F., Bastos, A., Goll, D., Lurton, T., Viovy, N., Bellouin, N., and Li, L.: Disentangling the Impacts of Anthropogenic Aerosols on Terrestrial Carbon Cycle During 1850-2014, Earths Future, 9, e2021EF002035, https://doi.org/10.1029/2021EF002035, 2021.
Zickfeld, K., Eby, M., Weaver, A. J., Alexander, K., Crespin, E., Edwards, N. R., Eliseev, A. V., Feulner, G., Fichefet, T., Forest, C. E., Friedlingstein, P., Goosse, H., Holden, P. B., Joos, F., Kawamiya, M., Kicklighter, D., Kienert, H., Matsumoto, K., Mokhov, I. I., Monier, E., Olsen, S. M., Pedersen, J. O. P., Perrette, M., Philippon-Berthier, G., Ridgwell, A., Schlosser, A., Von Deimling, T. S., Shaffer, G., Sokolov, A., Spahni, R., Steinacher, M., Tachiiri, K., Tokos, K. S., Yoshimori, M., Zeng, N., and Zhao, F.: Long-Term Climate Change Commitment and Reversibility: An EMIC Intercomparison, J. Climate, 26, 5782–5809, https://doi.org/10.1175/JCLI-D-12-00584.1, 2013.
- Abstract
- Introduction
- The need for emissions-driven ESM scenarios
- Recommendations for emissions-driven experiments in CMIP7
- Limitations and new challenges
- Towards comprehensive mitigation modeling in CMIP8 and beyond
- Conclusions
- Appendix A
- Code availability
- Data availability
- Author contributions
- Competing interests
- Disclaimer
- Acknowledgements
- Financial support
- Review statement
- References
- Abstract
- Introduction
- The need for emissions-driven ESM scenarios
- Recommendations for emissions-driven experiments in CMIP7
- Limitations and new challenges
- Towards comprehensive mitigation modeling in CMIP8 and beyond
- Conclusions
- Appendix A
- Code availability
- Data availability
- Author contributions
- Competing interests
- Disclaimer
- Acknowledgements
- Financial support
- Review statement
- References