the Creative Commons Attribution 4.0 License.
the Creative Commons Attribution 4.0 License.
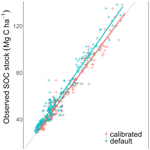
Evaluation and optimisation of the soil carbon turnover routine in the MONICA model (version 3.3.1)
Konstantin Aiteew
Jarno Rouhiainen
Claas Nendel
René Dechow
Simulation models are tools commonly used to predict changes in soil carbon stocks. Prior validation is essential, however, for determining the reliability and applicability of model results. In this study, the process-based biogeochemical model MONICA (Model of Nitrogen and Carbon dynamics on Agro-ecosystems) was evaluated with respect to soil organic carbon (SOC), using long-term monitoring data from 46 German agricultural sites. A revision and parameterisation of equations, encompassing crop- and fertiliser-specific C contents and the abiotic factors of soil temperature, soil water and clay content, were undertaken and included in the model. The modified version was also used for a Morris elementary effects screening method, which confirmed the importance of environmental and management factors to the model's performance. The model was then calibrated by means of Bayesian inference, using the Metropolis–Hastings algorithm. The performance of the MONICA model was compared with that of five established carbon turnover models (CCB, CENTURY, C-TOOL, ICBM and RothC). The original MONICA model systematically overestimated SOC decomposition rates and produced on average a ∼17 % greater mean absolute error (MAE) than the other models. The modification and calibration significantly improved its performance, reducing the MAE by ∼30 %. Consequently, MONICA outperformed CENTURY, CCB and C-TOOL, and produced results comparable with ICBM and RothC. Use of the modified model allowed mostly adequate reproduction of site-specific SOC stocks, while the availability of a nitrogen, plant growth and water submodel enhanced its applicability when compared with models that only describe carbon dynamics.
- Article
(7246 KB) - Full-text XML
- BibTeX
- EndNote
The historic conversion from native to agriculturally managed soils released 116 Pg of soil organic carbon (SOC) as CO2 to the atmosphere (Sanderman et al., 2018). Current projections estimate that unless no measures are taken, the decline in SOC stocks will continue, further intensifying the release of CO2 from soils (Riggers et al., 2021; Zhao et al., 2021). However, the sequestration of atmospheric CO2 in the soil is a climate change mitigation strategy that has substantial potential (Poeplau and Don, 2015; Fuss et al., 2018; Amelung et al., 2020). In particular, croplands are promising landscapes, since they are depleted in SOC and generally accessible for cultivation practices (Poeplau and Don, 2015; Sanderman et al., 2018). Researchers have proposed various agronomic systems, including agroforestry, reduced tilling and perennial and cover cropping, to optimise agricultural opportunities for sequestering carbon and thus partly restore the historically lost SOC (Smith et al., 2005). Estimates of the global capacity of agricultural soils for sequestering carbon range from 2 to 5 Pg C a−1 (Fuss et al., 2018). However, identifying and promoting site-specific measures in a highly diverse agricultural landscape encompassing more than 570 million farms globally and affecting ∼36 % of the Earth's terrestrial surface is a challenge (Lowder et al., 2016; Amundson and Biardeau, 2018). Despite the uncertainty in their practicability, international initiatives, such as the “4 per 1000” initiative, and scientific working groups advise the rapid modification of agricultural systems so that they act as carbon sinks (Minasny et al., 2017; Amelung et al., 2020). In contrast, some scientists focus on the potential for underestimated detrimental effects and advocate a well-thought-out approach to sequestering SOC on a large scale (Sommer and Bossio, 2014; Lugato et al., 2014; Amundson and Biardeau, 2018; Murphy, 2020).
Process-based models are tools that can be used to assess mitigation capabilities of agricultural systems for different environmental and management conditions and to analyse disagreements and accuracy of alternative estimation approaches. They are designed to represent the behaviour of natural systems, based on a selection of mathematical formulations of generally accepted biophysical principles. Carbon turnover models predict the dynamics of SOC in response to management and climate. Agroecosystem models combine carbon and nitrogen turnover routines with algorithms that represent soil water dynamics, greenhouse gas emissions and crop growth and allow the simultaneous prediction of multiple variables from different domains of the soil–plant–atmosphere continuum. Due to the explicit simulation of crop growth in response to weather, site conditions and crop management, agroecosystem models exhibit a clear advantage over simple soil carbon turnover models, as they can translate any changes in conditions that affect biomass input to soil, e.g. climate or crop management, directly to responses in SOC. Potentially, they could also simulate any feedback from those changing SOC dynamics to crop growth, for example, via nitrogen release or immobilisation. The disadvantage of this is that complex models require an accurate reproduction of the carbon input variability, whereas simpler carbon models estimate the carbon inputs from measured yields. Processes that are not described by the model, such as the effects of phosphorus and other nutrients and the effects of plant diseases on plant growth, could further constrain the ability of complex models to simulate adequate carbon inputs. Additionally, complex agroecosystem models are inherently black boxes that make it difficult to understand the various factors and feedback loops. They have as of yet rarely been tested for their ability to do exactly this, and they have just as rarely been compared to simple soil carbon turnover models to identify whether their advantage in applicability comes with a reduced predictive power (Smith et al., 1997; Farina et al., 2021). In any case, to ensure the adequate predictive capability of such models, complex or simple, a comprehensive validation process using measured data with different soil types, management practices and weather conditions is required.
MONICA (Model of Nitrogen and Carbon dynamics on Agro-ecosystems; Nendel et al., 2011, 2014) is a process-based agroecosystem model that simulates the effects of spatiotemporal environmental variability and agricultural practices on crop yields and soil condition of mineral soils (Nendel et al., 2014). It is a successor of the HERMES model, which was initially developed to estimate N dynamics in the soil–crop system (Kersebaum, 1995, 2006). A comprehensive SOC turnover submodule based on the Daisy model was added, replacing the simpler approach in the HERMES model (Hansen et al., 1991; Abrahamsen and Hansen, 2000). The holistic principle of the MONICA model supports research questions on the interlinkage between site conditions, plant development and C and N dynamics in a changing climate. The performance of the MONICA model showed the capability to adequately reproduce crop yields for different crops (Asseng et al., 2013; Bassu et al., 2014; Kothari et al., 2022; Rötter et al., 2012; Salo et al., 2016) and more complex crop rotations (Kollas et al., 2015; Kostková et al., 2021). For SOC dynamics under bare fallow treatment, MONICA performed similarly to simple C turnover models (Farina et al., 2021) but furthermore demonstrated also good performance when simulating short-term high-resolution CO2 exchange in a soil–plant system (Specka et al., 2016). The Daisy model, another agroecosystem model, had already been tested against long-term soil carbon experiments (Smith et al., 1997) and performed comparably well to soil carbon turnover models, such as RothC (Coleman and Jenkinson, 1996) or CANDY (Franko et al., 1995).
The question now is whether the MONICA model with its carbon turnover submodule is able to adequately simulate changes in SOC stocks when considering different agronomic practices. It is also important to establish how the performance of a complex model like MONICA compares with simple C turnover models such as RothC, ICBM, C-TOOL, CENTURY and CCB. To answer these questions, we have the following four objectives: (i) to validate the SOC submodule of the MONICA model with data from 46 agricultural long-term monitoring sites in Germany; (ii) if necessary, to enhance its applicability in view of the diversification of the dataset used for validation; (iii) to identify the main drivers of C sequestration and decomposition in the model; and (iv), if necessary, to improve model performance.
2.1 Data composition and site characteristics
For calibration and validation of the SOC turnover subroutine of the MONICA model, long-term monitoring data collected by the State Office for Mining, Energy and Geology (Landesamt für Bergbau, Energie und Geologie, LBEG) were used. In 1990, the state government of Lower Saxony (Germany) established a programme to permanently observe agricultural and forest soils in the federal territory. Its goal was to monitor soil changes and identify adverse ecologic and economic implications and thus predict the risk of imminent damage for the sustainable utilisation of soils.
The examined area is part of the North German Plain, with highlands up to 971 m in its southern region. It is located at latitudes between 51 and 54∘ N and longitudes between 7 and 11∘ E (Fig. 1a). It is characterised by a maritime climate, with mean annual temperatures ranging from 8.9 ∘C to 10.4 ∘C. Average annual precipitation ranges from 574 to 887 mm, with a positive climatic water balance in spring, autumn and winter and a negative balance in summer.
The database consists of 48 agricultural sites, of which 42 are conventional agricultural systems and 6 are organic. Two sites were removed due to their observation periods being shorter than 10 years, leaving 46 entries for analysis. The observation data consist of 33 sites that have been used for agriculture for more than a century. The remaining 13 sites were converted from forests, meadows or peatlands fewer than 5 decades before the start of the observation period. Soil sampling was generally conducted at regular intervals of between 1 and 5 years (Höper and Meesenburg, 2021). They were sampled at a depth of up to ∼30 cm and consisted of four replicates, which were combined as mixed samples.
In addition, soil horizons were sampled every 10 years at a minimum depth of 1 m. During the soil monitoring programme, a total of 333 soil carbon measurements (replicates not included) were taken at the selected sites from 1992 until the end of 2015. Between the sites, the mineral composition was diverse, with soil textures predominantly sand (20 sites) or silt (21 sites) and less frequently clay soils (five sites; Fig. 1b). The key properties of the examined soils were highly variable between the sites, with SOC ranging from 0.84 % to 4.91 %, from 5.25 to 23.66, bulk density from 1.14 to 1.76 g cm3 and pH from 3.9 to 7.9. A special distinctiveness of the investigated area is the occurrence of so-called black sands, which are characterised by comparably high, stable C contents in sand-dominated locations (Vos et al., 2018). Site-specific details are summarised in the Appendix (Table A1).
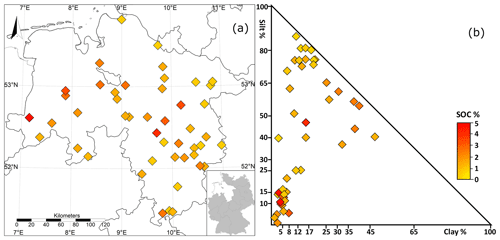
Figure 1Location (a) and soil classification (b) of the 46 agricultural long-term monitoring sites in Lower Saxony, Germany.
The soil and crop management at the sites was undertaken by the landowners or their associates. Therefore, there is a high degree of variability in the agronomic practices performed at the sites, such as tillage depth and type, fertiliser application, irrigation, crop rotation, harvest and crop residue management. The reported management reflects common agricultural practices for the site-specific regions (more details are given by Höper and Meesenburg, 2021). Control treatments were not available for the sites. Simulated soil temperatures and moisture contents were evaluated using data from 11 German long-term field experiments (Table A2; Flessa et al., 1995; Frolking et al., 1998; Schmädeke, 1998; Leidel et al., 2000; Wolf et al., 2014; Flessa et al., 2017; Mallast et al., 2021; Winkhart et al., 2022).
2.2 Model description
The MONICA model is comprised of subroutines that characterise plant growth, C and N dynamics, soil water and soil temperature (for a more detailed description, see Nendel, 2014). Execution of the programme requires comprehensive input data, including weather, soil and management information, as well as parameter values describing model settings. Every model iteration simulates soil and plant conditions in a daily time step for soil depths up to 2 m. Mathematically, the model is one-dimensional (point model), and the spatial representativeness of the model output depends primarily on the calibration data. Generally, the spatial representation ranges between 1 m2 and 1 km2. The soil profile is partitioned into 20 layers, each with 10 cm thickness of homogenous soil conditions. Soil input data are uniformly extrapolated to the relevant depth if the entered soil horizons are shorter than 2 m.
According to the Daisy model, the SOC content is partitioned into three groups composed of dead native soil organic matter (SOM), living soil microbial biomass (SMB) and added organic matter (AOM), each of which contains one rapidly (SOMf, SMBf and AOMf) and one slowly decomposing subpool (Fig. 2; SOMs, SMBs and AOMs; Hansen et al., 1991; Bruun et al., 2003). These compartments are calculated separately for each soil layer.
MONICA is usually initialised using a standard distribution of the SOC pools, which should correspond to an equilibrium at the beginning of the simulation (Bruun and Jensen, 2002). During the initialisation, inert SOC (IOM) is subtracted from the total SOC, based on equations adopted from Falloon et al. (1998). Then the SOM and SMB pools are determined by multiplying the manually entered SOC contents by the corresponding distribution parameters. The AOM pools are initialised and altered by added C contents from crop residues and organic fertilisers. Organic fertiliser and crop types are parameterised with specific N contents (NH and NO), decomposition rate coefficients and pool distributions. Furthermore, plant biomass accumulation is divided into five groups, namely root, leaf, shoot, fruit and sugar. For perennial plants, an additional permanent structure is considered. Each group has its own parameters controlling the growth rate and the biomass that can potentially be harvested or added to the AOM pools. Following a harvest command, accrued crop residues left on an agricultural field are immediately distributed to the AOM pools. Root remains are fragmented into the soil layers, depending on the root mass distribution in the soil profile. During tillage, the SOM content is distributed homogenously to the soil layers affected by tillage depth.
The initialised pools are managed by first-order degradation due to decomposition, with each controlled by related parameters. This process follows a decay rate that is proportional to the pool size (Hansen et al., 1991). In addition, the decomposition rate of organic matter is affected by soil temperature, soil water and clay contents (Bruun et al., 2003; Farina et al., 2021). These environmental conditions influence each pool as rate-modifying factors, with the exception of clay, which solely affects SMBs. Decomposed AOMf and AOMs are subsequently transferred to the SMB pools, from where fractions are respired to the atmosphere as CO2 or alternatively converted to SOMs and SOMf, following a pool-specific carbon use efficiency coefficient. Biologically degraded SOMs and SOMf goes through a similar process and are either respired or returned to the SMB pools. A detailed description of the SOM turnover subroutine is depicted in Hansen et al. (1991) and Nendel (2014).
2.3 Model modification
In total, 16 crop plants (amaranth, asparagus, buckwheat, carrot, flaxseed, field bean, hemp, lupine, serradella, spelt, strawberry, summer onion, sunflower, turnip and vetch) and 8 types of organic fertilisers (biogas slurry, calf slurry, dried poultry manure, hoof and horn meal, lime-stabilised sewage sludge, sheep manure and vinasse) were added to the model. This enabled the reproduction of all occurring land management characteristics in the dataset. Parameter values for crop growth (Tesar, 1984; Jeuffroy and Ney, 1997; Allen et al., 1998), fertiliser nutrient contents (Möller and Schultheiß, 2015; Wiesler et al., 2016), crop residues and organic fertiliser decomposition and partitioning coefficients (Gilmour et al., 1998; Thuriès et al., 2001; Lashermes et al., 2009; Peltre et al., 2012; Semenov et al., 2019) were taken from the literature. Furthermore, a specific C content parameter (CorgContent) was defined for each crop and organic fertiliser, providing a more adequate replication of C inputs in comparison with the original constant (Möller and Schultheiß, 2015; Wiesler et al., 2016; Ma et al., 2018). In addition, an overhaul of existing crop and fertiliser types was undertaken, ensuring distinct and more reasonable values for essential parameters. Crop growth was calibrated according to the measured yield data, while the residue-to-crop product ratio was adapted from the literature and an ensemble of allometric functions (Bolinder et al., 2007; Franko et al., 2011; Jacobs et al., 2020; Taghizadeh-Toosi, 2020). The differences between the allometric functions served as indicators of uncertainty in the estimation of C inputs from crop residues.
The functions that describe the influence of soil temperature, water and clay content on decomposition were revised and parameterised. This enabled all relevant carbon turnover factors to be tested for uncertainty and subsequently calibrated. The optimal values of the parameters were used as the evidential basis for the prior probability distributions, which is necessary for model calibration by means of Bayesian inference. For further explanations, see Sect. 2.5 and Table 1.
Extensive research provided evidence of the influence of temperature on physical soil processes, microbial metabolisms and enzymatic activities (Pietikäinen et al., 2005; Haddix et al., 2006; Meyer et al., 2018). The novel temperature function is described by the following function Eq. (1):
where T is the daily soil temperature in ∘C, Q10 is the Q10 temperature coefficient specified by the parameter QTenFactor, and Topt is the optimal temperature for decomposition in ∘C defined by the parameter TempDecOptimal (Fig. B1). From a literature analysis, a range of 38.2±7.5 ∘C was identified for the optimal temperature (Pietikäinen et al., 2005; Richardson et al., 2012; Menichetti et al., 2015; Liu et al., 2018; Čapek et al., 2019) and 2.6±0.6 for the Q10 temperature coefficient (Haddix et al., 2006; Vanhala et al., 2007; Conant et al., 2008; Gillabel et al., 2010; Meyer et al., 2018).
Microbial aerobic respiration is often driven by changing soil water contents up to a point where water becomes a restricting property for the availability of oxygen (Curiel Yuste et al., 2007; Schimel et al., 2007). Based on an analysis of incubation and field experiments, a Gaussian function was proposed, described by the following Eq. (2):
where WFPSd is the daily water-filled pore space in percent, and Mopt is the optimal water-filled pore space (WFPS) for decomposition in percent defined by the parameter MoistureDecOptimal (Fig. B2). Optimal WFPS for respiration was determined to be around 59.5±9.9 % (Linn and Doran, 1984; Doran et al., 1990; Liebig et al., 1995; Aon et al., 2001; Gabriel and Kellman 2014; Zhang et al., 2015).
Several studies have indicated that increasing soil clay contents have a negative effect on respiration due to the reinforced inaccessibility of SOM for microorganisms (Six and Paustian, 2014; Churchman et al., 2020; Fomina and Skorochod, 2020). In the MONICA model, the modulating effect of clay on the decomposition rates of SMBs is by default adjustable through the parameter LimitClayEffect. An inverse logistic function was proposed, described by the following Eq. (3):
where C is the site-specific clay content (in kg kg−1), and LCE is the limit at which the clay content affects the mineralisation defined by the parameter LimitClayEffect (Fig. B3). Laboratory evidence suggests that soils amended with clay minerals reduce mineralisation rates by % for every percent of clay added to the soil, followed by a gradual reduction in the effectiveness after a certain clay content is exceeded (Nguye and Marschner, 2014; Schapel et al., 2018; Riaz and Marschner, 2020; Liddle et al., 2020).
2.4 Screening of variables relevant to soil carbon turnover
For the purpose of understanding the relationship between input variables, parameters and the predictive proficiency of the MONICA model, a comprehensive sensitivity analysis was conducted using an improved Morris elementary effects screening method (Fig. C1; Morris, 1991; Campolongo et al., 2007; Pujol, 2009). The algorithm demonstrates the capability to determine the importance of parameters in relatively short computing times (Campolongo et al., 2007; Confalonieri et al., 2010). The effect of 51 variables was examined, of which 15 were environmental, 6 were related to the crop, 4 to the management and 26 to SOC turnover. Of the turnover parameters, six were unique for each fertiliser and crop residue type.
Depending on the tangible properties of the variables (factual or conceptual), the upper and lower bounds of each factor were either derived from the literature or intuitively inferred from model characteristics. After building the parameter space, all the factors were scaled to a range from 0–1. During each iteration, one variable was changed to a random value in the defined space (levels = 5; grid jump = 3), while the remaining variables were unaltered. Subsequently, the effect of the modified factor on SOC stocks was assessed by comparing the present iteration with the previous one. Based on the magnitude of the changes and the degree of the interaction with other factors, a parameter importance ranking was identified using the two measures μ∗ and σ (Campolongo et al., 2007).
Common recommendations for the number of model iterations are between 10 to 50 for each variable considered (Campolongo et al., 2007). However, several studies suggest a much higher number of iterations to achieve robust results (Sarrazin et al., 2016; Vanuytrecht et al., 2014; Pianosi et al., 2016). As a base value, 103 iterations and three runs were used in total to accomplish adequate coverage of possible input spaces and to estimate the uncertainty in the elementary effects.
The programming language R (version 4.1.0) with the graphical user interface RStudio (version 1.4.1717) and the sensitivity package (Iooss et al., 2020; version 1.26.0) were used for screening of variables relevant to soil carbon turnover in the MONICA model.
2.5 Parameter calibration
Bayesian inference techniques are an efficient approach for the calibration of process-based models (Vrugt, 2016; Van de Schoot et al., 2021). Common methods for prior elicitation, likelihood specification and posterior distributions have been extensively researched (Van de Schoot et al., 2021). For instance, Bayesian calibration has been applied successfully to forest biomass growth (Van Oijen et al., 2005; Svensson et al., 2008) and agroecosystem models (Lehuger et al., 2009; Gurung et al., 2020).
As a first step, the experimental data were divided into one subset for calibration and one for validation. In the process, the data were clustered into three groups based on their initial SOC stocks in order to achieve a general representation of the variability between the sites. From each of the groups, two-thirds of the sites were randomly sampled for model calibration (totalling 31 sites) and one-third for model validation (totalling 15 sites). The parameter selection process for the calibration was adapted from the results of the sensitivity analysis and the recommendations from Minunno et al. (2013), excluding local and 25 % of the least important parameters.
The calibration of the 14 selected parameters was undertaken by means of Bayesian statistical inference. In Bayesian calibration, the determination of possible parameter values is based on evidential probabilities (Van de Schoot et al., 2021). Accordingly, it was important that the upper and lower limits of the parameters were based on observed, realistic values. Therefore, a comprehensive literature analysis combined with expert knowledge provided the basis for the prior probability distributions, as shown in Sect. 2.3 (Oakley and O'Hagan, 2007). A weakly informative prior probability distribution was constructed, with the mean of all identified measurements as the most likely value and the observed lower and upper bounds as the variance for each parameter (Table 1; Van de Schoot et al., 2021). At the beginning of the calibration, a random initial value (within the prior probability distribution) was generated for each parameter, creating a parameter vector for each iteration. Selection for subsequent parameter values was limited by the minimum and maximum value of the prior probability distribution and a randomly determined step size corresponding to 1 %–25 % of the parameter space (Van Oijen et al., 2005). Accordingly, the subsequent parameter value changes from the previous value by a minimum of 1 % and a maximum of 25 % of the entire parameter space. During each iteration, MONICA was executed using the created parameter vector. Afterwards, the generated output was compared with the measured data using a log–Laplace likelihood function, suggested by Vrugt (2016), which estimated the association between simulated and observed values. This association was a representation of the posterior probability of each parameter vector. The Laplace distribution showed robustness against outliers and random variations, resulting in less biased and more consistent parameter estimates (Schoups and Vrugt, 2010). The Gelman–Rubin diagnostic (Gelman and Rubin, 1992) was used as a measure for the convergence of the calibration and was conducted with the R package coda (Plummer et al., 2006).
Table 1Description of the 14 parameters chosen for the calibration of the C submodel in MONICA. The prior distribution depicts the upper and lower bounds of possible parameter values inferred from the literature; the posterior distribution describes the median value of the posterior and the corresponding standard deviation.
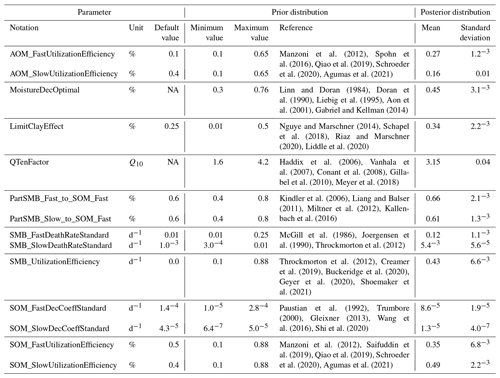
2.6 Quantitative methods and model evaluation specifics
The statistical methods for evaluating the sampling distributions and model performances were conducted with the R packages hydroGOF (Zambrano-Bigiarini, 2020; version 0.4-0) and car (Fox et al., 2019; version 3.0-12). Shapiro–Wilk (Shapiro and Wilk, 1965) and Levene's tests (Levene, 1960) were used on the measured fluxes to test the population for normality and variance equality. The Shapiro–Wilk test resulted in rejection of the null hypothesis for the variables (p<0.001), inferring that the examined datasets were not normally distributed. Levene's test resulted in acceptance of the null hypothesis, implying that the variance in the samples was homoscedastic (p=0.88). Using the interquartile range criterion (Jargowsky and Yang, 2005), 19 outliers were identified in the data, which represents ∼6 % of the cases. Q−Q plots confirmed the assumption that the data points were nonlinear distributed.
To improve representativeness, the years 1992 to 2000 were excluded from the analysis, reducing the observed number of cases from 333 to 223. The reason for this approach was justified by the inherent risk of statistical biases from overfitting of measured and simulated values due to the initialisation of simulated SOC stocks based on measured values, as significant changes in SOC stocks usually take several years. Furthermore, a spin-up period of 20 simulated years was included to ensure a realistic reflection of available fresh matter in the soil, since the AOM pools were initialised equal to zero. The chosen period corresponds to the maximum duration of mineralisation of most AOM (Thuriès et al., 2001; Semenov et al., 2019).
Based on the reduced sample size and the abovementioned statistical assumptions, Kendall's rank correlation coefficient (T; Kendall, 1948) was selected to assess the statistical dependence between the observed and simulated values. Kendall's τ is the preferred method for measuring the ordinal association in data consisting of small sample sizes and outliers and is more robust and efficient than the Pearson correlation coefficient and Spearman's rank correlation coefficient (Bonett and Wright, 2000). In addition, the model results were evaluated with statistical methods like the mean absolute error (MAE; Willmott and Matsuura, 2005) for an analysis of the average error between observed and simulated pairs and with the Nash–Sutcliffe model efficiency coefficient (NSE; Nash and Sutcliffe, 1970) to assess the predictive skill of the simulation model (Fig. E1). Furthermore, the slope intercept was considered in order to better assess the behaviour of the models.
3.1 Simulation of soil and plant growth variables
Soil temperatures were well predicted, with an average error of just under ∼1.8 ∘C (Table 2). Low temperatures were slightly overestimated, and high temperatures were underestimated. In comparison, soil water contents were predicted somewhat less favourably, with an average error of ∼5.4 %. Nonetheless, the predictive power was better than the mean of the observations. The model tended to slightly overestimate moderate water contents and underestimate the measured variance. A comparison of the default with the calibrated plant growth parameters was not performed, since new crops were added to the model in order to simulate all possible systems.
The calibrated plant growth model achieved a good representation of measured yields, with an average error smaller than ∼1.6 Mg DM ha−1 for all crops. Overall, there was a linear relationship between the calibrated and measured yields. However, there was a high variability in the estimation quality between different crop types. Some crops, such as triticale and potatoes, were modelled less adequately than silage maize and sugar beet, which was partly explained by the differences in the number of observations and the variance in yields. The C inputs were compared with the estimated mean of the allometric functions. The model produced an average error that was similar to the calculated difference between the allometric functions (∼1 Mg DM ha−1).
Table 2Statistics describing the performance of the MONICA model regarding soil and plant growth variables. The statistics depicted are Kendall's rank correlation coefficient (T), Nash–Sutcliffe model efficiency coefficient (NSE), mean absolute error (MAE), the slope and intercept for characterising the relationship between measured values (except values for C inputs, which were calculated with allometric functions) and simulated values.
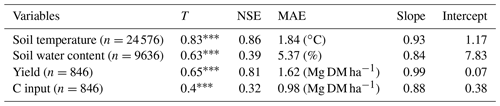
p<0.001.
3.2 Quantification of uncertainty and parameter calibration
A strong relationship was identified between SOC stock change rates and C inputs through organic fertilisation and crop residues (Table F1; OrganicFertilization, AOM_DryMatterContent and CorgContent), given the upper and lower bounds of possible values. Additionally, these factors were less affected by other variables, noticeably by the lower σ on average in comparison with μ*. The climate variables of temperature (Temperature) and precipitation (Daily_Precipitation) were also of greater importance to the SOC turnover rate but had a higher standard deviation of elementary effects. Except for solar irradiance (Global_Radiation), temperature and precipitation, all other climatic factors had an insignificant effect on SOC stocks. Of all the variables that were initialised at the beginning and remained static during the model run, the initial SOC stock (SoilOrganicMatter) was the most influential, followed by the site-specific field capacity (FieldCapacity), soil bulk density (SoilBulkDensity) and pore volume (PoreVolume). Other site-specific variables (Sand, Clay, PermanentWiltingPoint, pH, and Sceleton) were comparably less important. The management factors of tillage (Tillage), mineral fertilisation (MineralFertilization), irrigation (Irrigation) and N content in the added organic matter (CN_Ratio_AOM_Slow, CN_Ratio_AOM_Fast, CN, AOM_NH4Content and AOM_NO3Content) had a small effect on the SOC turnover rate. σ values were generally higher than the overall importance of the input factors, indicating that there is probably an interaction and non-linear effect between the environmental and management variables. In comparison, the sensitivity of the turnover variables was less pronounced and revealed three particularly important parameters, namely one C pool parameter (SOM_SlowDecCoeffStandard) and two weather-regulated decomposition factors (QTenFactor and MoistureDecOptimal). They were similar in significance to precipitation, initial SOC and field capacity. Changes in the parameters QTenFactor and MoistureDecOptimal were more important than in LimitClayEffect, which was in accordance with the influence of clay being limited to the SMBs pool. The effect of the factor describing the optimal temperature for decomposition (TempDecOptimal) was negligible but had a considerable increase in importance when a higher range of possible values for the variable temperature was included. Crop- and fertiliser-specific turnover parameters were also sensitive and coincided with the importance of C inputs for SOC stock change rates. Less pronounced sensitivities involved the parameters describing the microbial dynamic (besides the parameter SMB_UtilizationEfficiency), distinguishable by the SMB in the name. Non-linear effects and interactions were more strongly pronounced in the SOC turnover parameters.
Figure 3 shows the results of the calibration process, with the posterior distribution curve representing the frequency of accepted values and the vertical red line indicating the optimal value as the median of the posterior. Bayesian inference reduced the uncertainty for all parameters, converging the posterior distribution to a normal or lognormal distribution. Throughout the random sampling, the entire plausible parameter space was covered and curve progressions showed that the optimal values were probably within the specified limits. During the calibration process, the posterior distribution of most parameters became narrower in comparison with the prior, confirming the convergence to the optimal values. Similar to Lehuger et al. (2009), the acceptance rate for the proposed parameter values was ∼25 %. Based on the results of the Gelman–Rubin diagnostics, adequate convergence was achieved for all parameters (Fig. G1), signifying the unbiased outcome of the Markov chain Monte Carlo (MCMC). The correlations across parameters remained below a coefficient of 0.2, with the exception of QTenFactor and SOM_SlowDecCoeffStandard (Fig. H1).
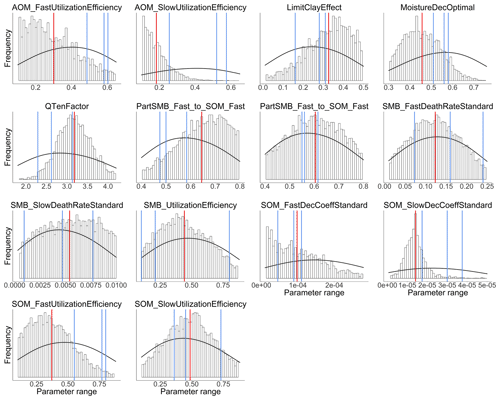
Figure 3Posterior probability distribution (vertical bars) of 14 C submodel parameters calibrated over the range of the prior probability distribution (density curve). The posterior is depicted as the distribution of accepted values determined by random sampling from 105 proposed values. The vertical red lines represent the optimal values as the median of the posterior and the blue lines the initial values of each chain.
3.3 Model performances
The statistical methods showed that the default MONICA model (MONICAdef) was inferior to the RothC, ICBM and C-TOOL models and produced results similar to CENTURY and CCB (Tables 3 and I1; Fig. 4). The mean absolute error was the highest of all the models but was within the margin of error compared with CENTURY and CCB for all sites and with CCB for the validation sites. Kendall's rank correlation coefficient and Nash–Sutcliffe model efficiency coefficient values were within the margin of error for all models and sites and therefore showed insignificant differences in the predictive skill of the models.
Table 3Statistics describing the performance of the default (MONICAdef), the modified uncalibrated (MONICAmod) and the modified calibrated (MONICAcal) soil carbon submodel in MONICA in comparison with five C turnover models for all sites. The first 10 years were excluded from the analysis (n=223). The following statistics are depicted are Kendall's rank correlation coefficient (T), Nash–Sutcliffe model efficiency coefficient (NSE), mean absolute error (MAE) and the slope and intercept for characterising the relationship between measured and simulated C stocks.
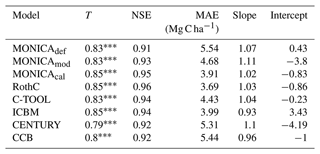
p<0.001.
MONICAdef estimations deviated on average by more than ∼ 10 % from the measurements. Inclusion of a C content parameter for different crop residues and organic fertilisers in the MONICA model (MONICAmod) improved the accuracy of predictions for C stock changes by ∼16 % for all sites and by ∼8 % for the validation sites, while the additional global parameter calibration (MONICAcal) improved MONICA's average estimation error by ∼29 % for all sites and by ∼30 % for the validation sites. Compared to MONICAcal, only RothC achieved a lower MAE for all sites. The differences between RothC and MONICAcal were within the margin of error for the validation but not for all sites. MONICAcal, MONICAmod, RothC, ICBM and C-TOOL produced errors within the average standard deviation between the replicates of the C measurements for the validation (7.76 Mg C ha−1) and for all sites (5.01 Mg C ha−1). In contrast, MONICAdef, CENTURY and CCB had errors that were not within the average standard deviation for all sites. Each model was capable of producing results corresponding to the 95 % confidence interval for the validation sites (6.4 Mg C ha−1). No model achieved the same for all measurements (3.3 Mg C ha−1).
Based on the outcomes of the NSE method, all models performed better than the mean of the measurements, with MONICAdef achieving the lowest and RothC the highest scores. The differences between MONICAcal and MONICAdef were within the margin of error. The Kendall rank coefficient displayed a significant statistical dependence between the simulated and measured data, with MONICAcal, RothC and ICBM achieving the best results and CCB and CENTURY the weakest results. Overall the T coefficients were high, with MONICAdef achieving average results and MONICAcal above-average results, which is in contrast with the other models. Of the six models, MONICAcal generally produced better results than CENTURY, C-TOOL and CCB. The slope and intercept of the statistical analysis indicated that MONICAdef generally overestimated decomposition, while MONICAcal produced results closer to the measured data. Overall, MONICAdef underestimated the SOC stocks at 24 sites and overestimated them at two sites, leaving 20 sites that were simulated within the standard deviation of the measurements. In comparison, MONICAcal underestimated the SOC stocks at six sites and overestimated them at nine sites, leaving 31 sites that were simulated within the standard deviation of the measurements. The calibration resulted in a model behaviour that was comparable with RothC.
4.1 Differences in models and performances
The MONICA model featured a plant growth, nitrogen and soil water submodel, while RothC, CCB, ICBM, CENTURY and C-TOOL did not. Smith et al. (1997) have argued that coupling C turnover with agroecosystem submodels will be necessary to simulate the impact of global change on agricultural production and SOC changes. However, they also assumed that simpler models have an advantage in simulating various land uses due to the lack of adequate data and the necessary parameterisation of complex models. Other literature shows that C turnover models coupled with sophisticated plant growth models do not result in a better performance, highlighting that complex models are more error prone (Mueller et al., 1996; Parton, 1996; Li et al., 1997). One explanation could be that some processes, such as the effect of phosphorus and other nutrients or the impact of plant diseases on crop growth, are not included in the models. In addition, information on crops and plant residues is often not available, so that measured values from other publications must be used to determine the required parameter values for crop development and ultimately the C inputs. This also applied to the data requirements of the MONICA model, which led to the necessary parameterisation of new crop and fertiliser types in order to simulate all sites. Since the data available for this publication did not contain additional information on the organic fertilisers and crops, allometric functions and values of differing genotypes and growing conditions had to be used to compare ingoing C inputs. Although we were able to adequately replicate yields as a proxy for modelling C inputs from crop residues, some discrepancies between model and observed yields remained. This could have led to an inaccurate reproduction of C inputs, since the specific properties of organic fertilisers and crop residues are characterised by high standard deviations (Möller and Schultheiß, 2015; Wiesler et al., 2016; Ma et al., 2018). In contrast, the C input estimation for the simpler models was based on observed crop yields for each site and allometric functions evaluated for Germany in previous studies (Dechow et al., 2019; Riggers et al., 2019). We assumed that a C input estimation based on measured yields should better describe the variability in the C inputs between sites. However, the results of the present study did not confirm the assumption that model complexity is an indicator of predictive ability in process-based models. Notable differences were driven by model characteristics, like SOM pool initialisation or model-specific response functions, some of which were controlled by parameterisation. Each model showed weaknesses depending on the specific conditions at each site (Figs. J1 to J7). For instance, MONICAdef overestimated decomposition in ∼52 % of all sites in the dataset but managed to predict the C losses at the Fuhrberg and Vinnhorst sites better than MONICAcal. These sites had undergone recent land use change and had on average higher initial SOC stocks and losses over the measured period. In temperate agroecosystems, conversion from grassland or forest to arable land is considered to be one of the main reasons for C release (Vos et al., 2019; Poeplau et al., 2020). Correspondingly, sites with recent land use change showed the strongest decline in C stocks and were therefore a good indicator of the downward trend in SOC stocks. Removing sites with a cultivation period <50 years (n=10) resulted in a balanced C budget (0.02 t C ha−1 a−1), which also produced an even better performance by the calibrated MONICA model. The magnitude of changes in SOC stocks for all sites was comparable with other studies conducted in temperate climates, representing a general trend for German arable land (Steinmann et al., 2016; Sanderman et al., 2018; Vos et al., 2019; Seitz et al., 2022).
All models underestimated the magnitude of the variation in the natural SOC dynamics. The best simulation results were generally achieved at sites with slowly changing SOC stocks. Some sites distinguished by dramatic changes in SOC were especially problematic for the models to simulate, in particular the Dinklage site (BDF033_L), which was characterised by high C losses, and in contrast the Reinhausen site (BDF051_L), which was characterised by increasing C stocks. CENTURY and CCB, which under-performed in comparison with the other models, were the best at predicting these highly variable sites. The other models overestimated the SOC stock for BDF033_L and underestimated it for BDF051_L. In MONICAcal, these two sites caused ∼10 % of the mean absolute error.
At site BDF033_L, the farmer was cultivating winter barley and corn as energy crops but experienced declining corn yields over the measured period. This probably led to a negative C balance, even though the field was extensively fertilised with pig and bull slurry. In MONICA, organic fertilisation was the best indicator of SOC changes, while there was no significant relationship between the amount of organic amendments and SOC stock changes in the measured data. Accounting for the quantitative variations in manure application supports the assumption that organic fertilisation is a weak predictor of SOC turnover rates and was correspondingly overestimated in the model. However, numerous studies clearly show a positive effect of organic amendments on SOC stocks (Koishi et al., 2020; Gross and Glaser, 2021; Roß et al., 2022). Since no control treatments were included in the experimental design of the long-term monitoring sites, it was not possible to carry out a comparative analysis of the individual sites with regard to the impact of organic inputs. Another consideration concerned site-specific uncertainties in the necessary model input. The properties of organic fertilisers and residues were unknown and thus had to be supplemented with standard values from the literature. Each fertiliser and crop type had fixed C ratios in the models, while in reality these inputs have a highly variable composition and can vary greatly depending on farm management, animal diet, climate and soil conditions (Schnug et al., 1996; McCartney et al., 2006; Cajamarca et al., 2019). There are three possible reasons for the disparity between the models and observations at site BDF033_L: (i) the fertiliser and crop-specific nutrient contents were inaccurate, leading to an overestimation of C inputs; (ii) the C losses from harvesting were underestimated; or (iii) the site and environmental characteristics and their effect on SOC decomposition were incorrectly simulated.
In comparison, at site BDF051_L, the farmer had transitioned to a management with reduced tillage intensities and no cultivation of root crops and managed to increase the C content in the upper soil layer by ∼30 Mg C ha−1 during the measured time period. Although the effect of conservational tillage is still being debated, numerous studies support the effect measured at site BFD051_L (Freibauer et al., 2004; Luo et al., 2010). In the MONICA model, tillage uniformly distributed SOC in the affected soil layers. This was supported by the sensitivity analysis, which showed that tillage had an effect when there was heterogeneity in the soil profile. However, based on this study's data, it is likely that the model underestimated the effect of tillage on SOC decomposition. Possible reasons for the disparity were either an imbalance of C inputs into the topsoil or missing functions that describe the processes involved, such as the effect of tillage on soil aggregation, hydraulic properties and root growth (Mondal et al., 2020). The effect of tillage on SOC stocks is currently absent from other C turnover models, such as RothC. Jordon and Smith (2022) suggest modifying the decomposition rate to achieve a realistic representation of the effect of tillage on SOC, although they assume a small effect of tillage on SOC stocks.
Another probable reason for the differences between the model and measurements was the climate variables. In the data, only precipitation correlated significantly with SOC stock change rates, while in MONICA this was important but less so than temperature. Sierra et al. (2015) argue that functions in process-based models tend to overestimate the temperature effect on decomposition rates, and this imbalance cannot be offset by the moisture effect. This was also likely in the MONICA model, since the effect of temperature on decomposition was generally twice as strong as moisture. The importance of climate variables is still being debated, with Luo et al. (2017) and Carvalhais et al. (2014) finding major effects on SOC changes, while only minor effects have been established by Fujisaki et al. (2018) and Vos et al. (2019).
4.2 Implications for improving MONICA's soil carbon turnover model
Most biogeochemical models were developed many decades ago. This is also true of the C turnover subroutine in MONICA, which is based on the Daisy model (Hansen et al., 1991; Abrahamsen and Hansen, 2000). Therefore, it is based on the conceptual design of multiple SOC pools with pool-specific turnover rates that are not associated with clear, measurable soil properties (Bruun and Jensen, 2002). This circumstance makes it difficult to clearly define how the model could be optimised using response functions but also the initialisation of the model. Bruun and Jensen (2002) argue that it can be important to use plausible assumptions to initialise the SOM subroutine using radiocarbon measurements or the land use history. Nonetheless, functions in C turnover models need to be improved in order to estimate SOC dynamics across a wide range of spatiotemporal and agronomic conditions (Smith et al., 1997). Correspondingly, general insights into soil processes are needed to justify the introduction of additional or improved functions. There needs to be further examination of existing mechanisms and the interactive effects of site-specific characteristics but also missing factors that affect microbial abundance, diversity and activity (Louis et al., 2016). Microorganisms in particular play a significant role in the soil carbon cycle by controlling the rate of mineralisation and promoting plant growth by improving mineral nutrition or stimulating immune and stress reactions in crops (Tardy et al., 2015; Berg et al., 2017). This could be advantageous for representing the processes like priming or SOC responses on warming. However, microbial models, as reviewed in Chandel et al. (2023), vary widely in terms of model structure, processes considered and parameters required, which makes it clear that there is currently no consensus on which approach is best suited to represent the biosphere. Some of the models are evaluated and compared in Sulman et al. (2018), using experimental data from litter input studies and warming experiments. In evaluating these models, they find high variability in model results and conclude that first-order models already produce divergent projections due to parameter uncertainties and that structural diversity among models would exacerbate these uncertainties. We believe that increasing differences between models due to structural diversity does not represent a degradation in predictive ability but rather a more accurate estimate of predictive uncertainty (Bradford et al., 2016; Lovenduski and Bonan, 2017). It is unclear if the adoption of one of the various microbial approaches could increase the model accuracy of MONICA. Because of the increased model complexity and number of parameters, the evaluation and calibration of an incorporated microbial model for regional conditions would also require a more constraining dataset, preferably including those events where microbial models might outperform models based on first-order kinetics.
This study attempted to introduce two changes to the default MONICA model: (i) a revision, parameterisation and calibration of the functions that describe the influence of soil temperature, water and clay content on decomposition and (ii) an integration of a crop- and fertiliser-specific C content parameter. Alteration of the temperature function involved mathematically reformulating the original exponential function into a surge function. The reason for this change was that the default equations did not consider the decreasing activity of soil microorganisms at temperatures above 30 ∘C (Liu et al., 2018; Fang and Moncrieff, 2001). This could lead to an overestimation of mineralisation, especially under globally increasing temperatures caused by climate change (DWD, 2021). Further improvements could be achieved by incorporating changes in the optimal temperature for decomposition based on site-specific varieties of microbial communities and C qualities (Fierer et al., 2006).
The clay function was changed to an inverse logistic function on the basis of the original equation. Many studies suggest that increasing soil clay contents have an adverse effect on SOC decomposition due to the reinforced inaccessibility of organic substances for decomposers (Six and Paustian, 2014; Churchman et al., 2020; Liddle et al., 2020). Adversely, several studies have found no effect or even a positive effect (Wei et al., 2014; Fissore et al., 2016; Zhang et al., 2021). There are multiple explanations for this disparity, e.g. different clay types, residue qualities and microbial diversities (Nguye and Marschner, 2014; Fissore et al., 2016). Without knowing the exact underlying processes to explain the variability and limitation of the available data, only minor changes were made when modifying the clay function. Removing the clay factor might reduce the complexity of the model but could also negatively impact the predictive capability of the SOC turnover routine. Further evaluation is required to assess the importance of the clay factor for decomposition and to credibly change the function.
Furthermore, the soil moisture factor, which was originally based on the pressure potential derived from the van Genuchten model (Van Genuchten, 1980; Abrahamsen and Hansen, 2000), was simplified. The default moisture function was directly controlled by the soil water content, soil texture, bulk density and SOC stocks, while the revised Gaussian function was only regulated by the soil water content. It is argued that the effect of texture on the decomposing community could be overestimated, since clay as a factor was already included in a function limiting mineralisation. In addition, the influence of soil texture on decomposition proved to be inconsistent, therefore requiring a better understanding of underlying processes (Doran et al., 1990; Scott et al., 1996), while the influence of bulk density was limited by model characteristics as it remained static after initialisation, hampering the reproducibility of management-related soil disturbances on the moisture function. Linn and Doran (1984) showed that the effect of ploughing on bulk density increased the microbial activity and decreased the optimum soil moisture content, while other studies have also determined an impact of soil structure changes on the mineralisation rate (Franzluebbers, 1999; Vilkiene et al., 2016). Another particularity of the default moisture function was that the optimal water-filled pore space for microbial activity was estimated to be between 10 %–25 % for sandy soils and 30 %–35 % for clay soils, while in the literature a general value of ∼60 % is considered the most probable (Linn and Doran, 1984; Doran et al., 1990; Liebig et al., 1995; Aon et al., 2001; Gabriel and Kellman, 2014; Zhang et al., 2015). Nevertheless, the simplification is probably less error-prone and easier to understand but at the same time not optimal for depicting the variance in microbial diversity and their environmental preferences. The inclusion of a more complex moisture function should be based on definite results.
As shown with the crop- and fertiliser-specific C content parameter, increasing the complexity of a model can be important for improving performance. According to the sensitivity analysis and the results, the addition of a C content parameter was necessary for the representation of realistic C inputs. However, the limited availability of data hinders the determination of precise C contents in plant residues and organic fertilisers. As mentioned in Sect. 4.1, values adopted from the literature could have a detrimental effect on the model's predictive capability. Therefore, measurements of C contents in crop residues and organic fertilisers should be part of the experimental design when investigating changes in SOC stocks. To further optimise the model, it is recommended that the C inputs from crop residues be divided into aboveground and belowground biomass. Currently there is no distinction between roots, shoot and leaves in the AOM pool, even though there is good evidence that roots in particular have a much longer mean residence time in the soil (Poeplau et al., 2021; Sokol and Bradford, 2019).
The above suggestions for improvement relate solely to the soil carbon model, but in complex models such as MONICA, the different subroutines can be interdependent, and changes in one could have a relevant impact on another function. Especially with respect to the feedbacks between the C and N cycle, plant growth and soil water contents should be considered in future studies.
The evaluation of the original MONICA model revealed weaknesses in estimating SOC trends. Using the default parameterisation, decomposition was overestimated, and the statistical performance was inferior compared with the C turnover models RothC, ICBM and C-TOOL. MONICA's capabilities of simulating SOC trends increased when more realistic functions were introduced that described the effects of soil temperature, moisture and clay on decomposition, coupled with a better specification of C inputs from organic fertilisation and crop residues. A sensitivity analysis displayed the importance of C inputs and the environmental factors of temperature and precipitation, as well as the corresponding parameters on SOC variation in the model. A successful calibration by means of Bayesian inference improved the parameterisation of the C turnover subroutine. The modified and calibrated MONICA model outperformed CENTURY, C-TOOL and CCB and produced similar results in comparison to RothC and ICBM. With the exception of certain sites, adequate reproduction of SOC stock change rates was achieved. However, none of the investigated models was capable of simulating each site satisfactorily. All the models underestimated the variation in measured SOC dynamics that occurred at sites with particular properties and management practices. This paper reveals that even complex, biogeochemical models are capable of performing similarly or even better than the more simplistic C turnover models. It is likely that with better data availability and continuing optimisation of functions representing natural processes, MONICA's performance can be further enhanced.
Table A1Description of the long-term monitoring sites (n=46) used for calibration and validation of the carbon turnover routine in the MONICA model. SOC is soil organic carbon. MAT is mean annual temperature. MAP is mean annual precipitation.
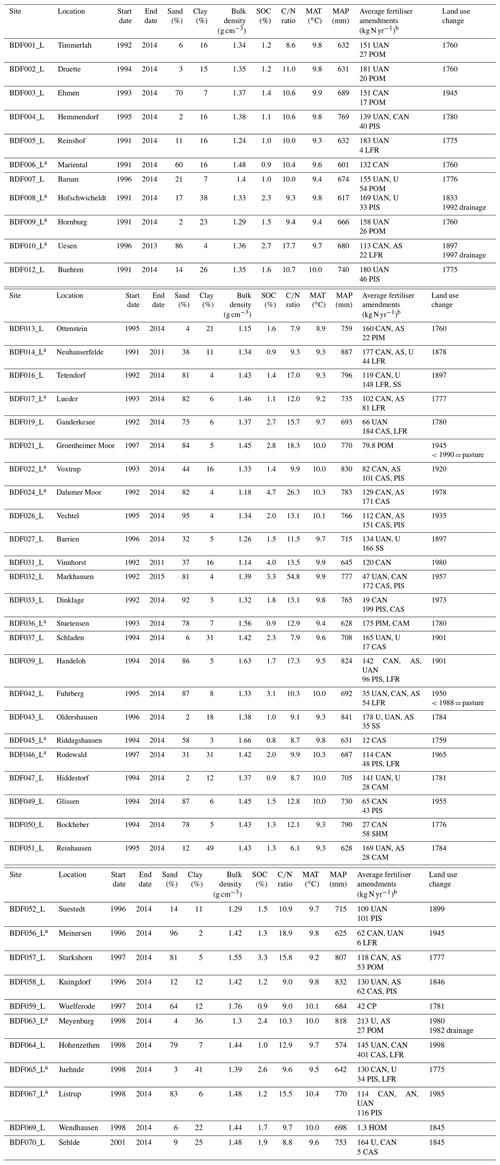
a Sites used for model validation (n=15). b Predominantly applied fertilisers; N values for organic amendments were estimated. UAN is urea ammonium nitrate. U is the urea. CAN is calcium ammonium nitrate. AS is ammonium sulfate. AN is ammonium nitrate. POM is poultry manure. PIS is pig slurry. PIM is pig manure. LFR is liquid fermentation residues. SS is sewage sludge. CAS is cattle slurry. CAM is cattle manure. SHM is sheep manure. CP is compost. HOM is horse manure.
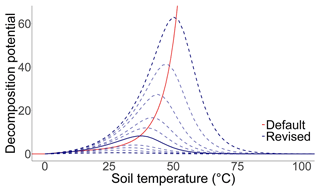
Figure B1The effect of soil temperature on the soil organic carbon decomposition rate. The solid red line describes the standard function that is integrated in the MONICA model. The solid blue line describes the revised function as the best fit curve to the observed data, while the dashed blue lines describe the possible properties of the equation according to the prior probability distribution of the parameters QTenFactor and TempDecOptimal.
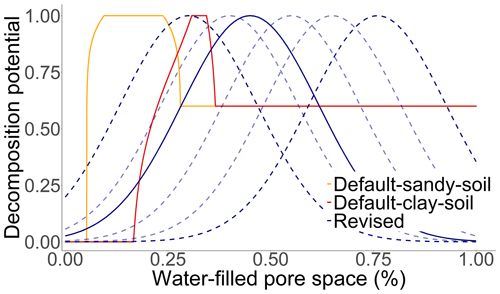
Figure B2The effect of soil moisture on the soil organic carbon decomposition rate. The solid red line describes the standard function for a clay soil (clay = 60 %, sand = 10 %, bulk density = 1.4, and soil organic carbon = 2 %), while the solid yellow line describes the standard function for a sandy soil (clay = 4 %, sand = 80 %, bulk density = 1.4, and soil organic carbon = 1 %). The solid blue line describes the revised function as the best fit curve to the observed data, while the dashed blue lines describe the possible properties of the equation according to the prior probability distribution of the parameter MoistureDecOptimal.
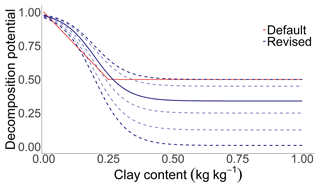
Figure B3The effect of clay on the soil organic carbon decomposition rate. The solid red line describes the standard function that is integrated in the MONICA model. The solid blue line describes the revised function as the best fit curve to the observed data, while the dashed blue lines describe the possible properties of the equation according to the prior probability distribution of the parameter LimitClayEffect.
Table D1Mathematical formulation of the Metropolis–Hastings algorithm and the log–Laplace likelihood function, where θ is the parameter vector, Y the dataset used for calibration, t the number of iterations, σ the standard deviation inferred jointly from the error between observation and simulation, ω the model input, f(ωi;θi) the model run with the parameter vector θ, α the acceptance ratio and δ a random vector from a multivariate distribution.
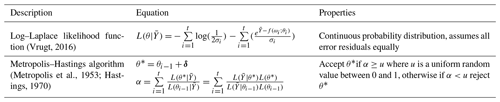
Table F1Results of the Morris method depicting the effects of 51 variables on soil carbon changes. The variables are subdivided into site, management, crop- and fertiliser-specific and carbon turnover factors. The parameters CorgContent (C content of crop residues and organic fertilisers), MoistureDecOptimal (optimal water-filled pore space for decomposition), TempDecOptimal (optimal temperature for decomposition) and QTenFactor(Q10 temperature coefficient) were included as modifications to the model. The overall importance of an input factor is specified by two sensitivity measures, namely the mean (μ*) and the standard deviation of the elementary effect (σ) of each parameter. Higher values in the two sensitivity measures imply a greater importance of the variable for changes in soil carbon stocks. The uncertainty is presented as the standard deviation between the results of three separate Morris elementary effects screening runs.
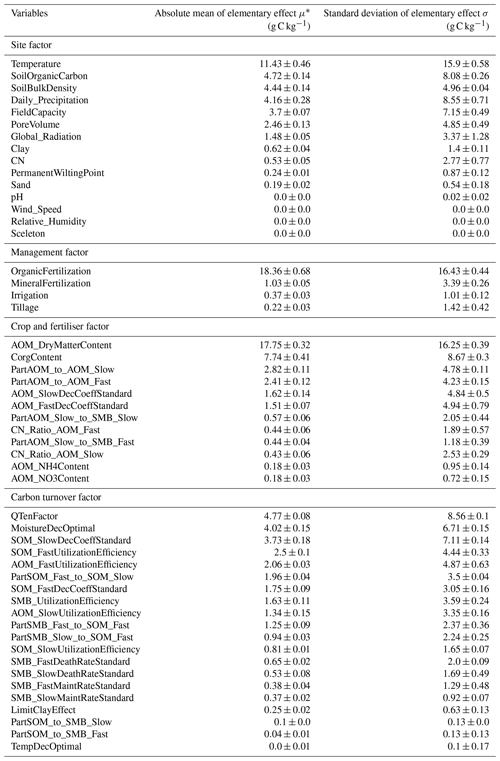
Table I1Statistics describing the performance of the default (MONICAdef), the modified uncalibrated (MONICAmod) and the modified calibrated (MONICAcal) soil carbon submodel in MONICA in comparison with five C turnover models for 15 validation sites. The first 10 years were excluded from the analysis (n=70). The statistics depicted are Kendall's rank correlation coefficient (T), Nash–Sutcliffe model efficiency coefficient (NSE), mean absolute error (MAE) and slope and intercept for characterising the relationship between measured and simulated C stocks.
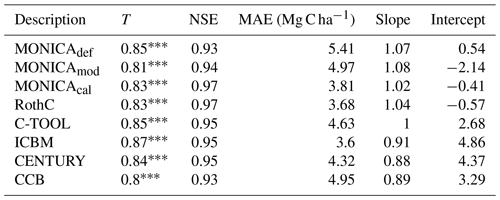
p<0.001.
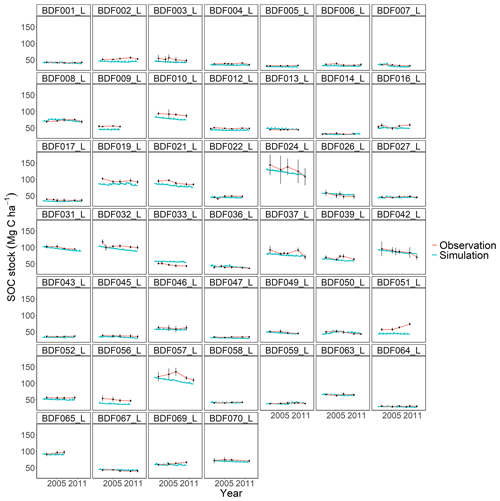
Figure J1Performance of the default MONICA model for each individual site. The first 10 measured years were excluded.
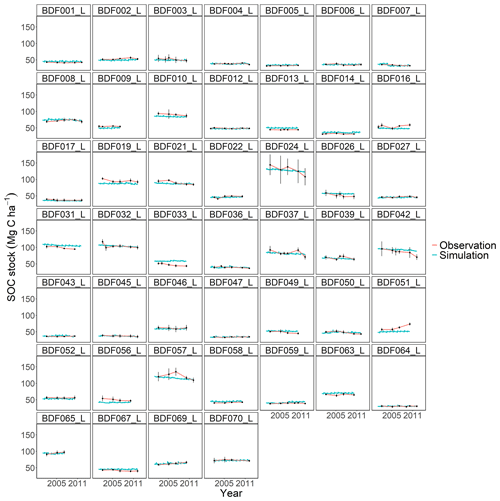
Figure J2Performance of the modified and calibrated MONICA model for each individual site. The first 10 measured years were excluded.
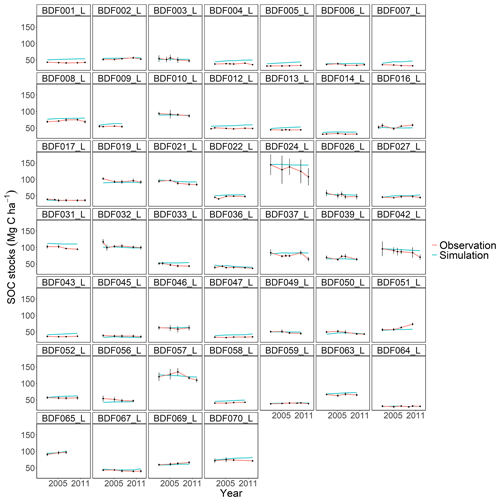
Figure J3Performance of the CCB model for each individual site. The first 10 measured years were excluded.
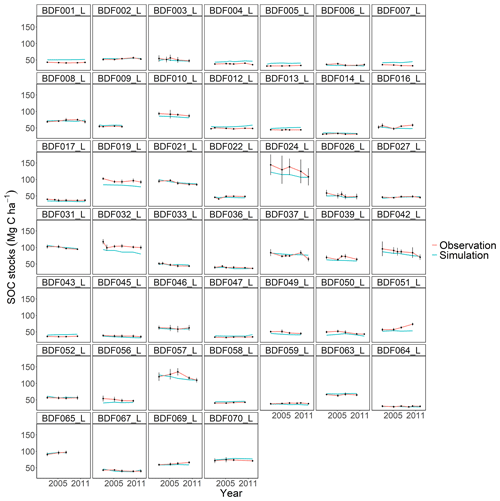
Figure J4Performance of the CENTURY model for each individual site. The first 10 measured years were excluded.
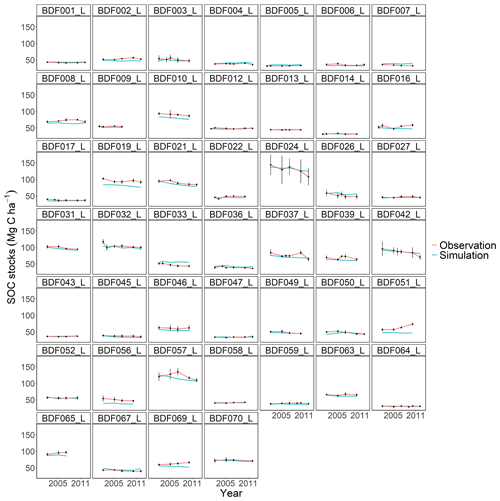
Figure J5Performance of the C-TOOL model for each individual site. The first 10 measured years were excluded.
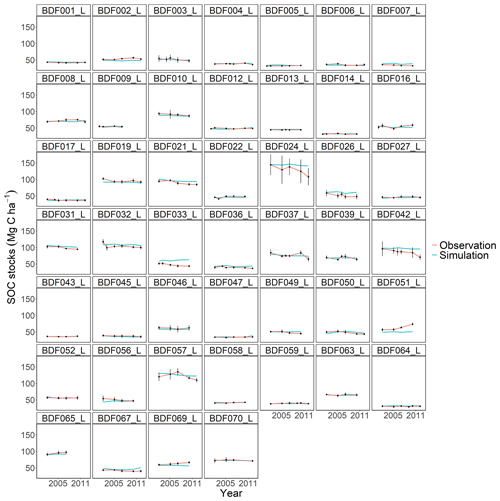
Figure J6Performance of the ICBM model for each individual site. The first 10 measured years were excluded.
The original and modified MONICA model source code is openly available at https://doi.org/10.5281/zenodo.8380341 (Aiteew et al., 2023a). The described long-term soil monitoring data are available from the State Office for Mining, Energy and Geology of the Lower Saxony state government. Restrictions apply to the availability of these data, which were used under license for this study. Data are available from the State Office for Mining, Energy and Geology of the Lower Saxony state government from https://www.lbeg.niedersachsen.de/boden_grundwasser/bodenmonitoring/bodendauerbeobachtung/das-boden-dauerbeobachtungsprogramm-von-niedersachsen-572.html (LBEG, 2023) and via email (bodenkundlicheberatung@lbeg.niedersachsen.de). Additional data are openly available at https://doi.org/10.5281/zenodo.8380332 (Aiteew et al., 2023b). Further information on the Berge, Dedelow, Hohenheim, Hohenschulen and Merbitz data are comprehensively described in the publication by Mallast et al. (2021) (available via email from janine.mallast@thuenen.de). The Deutsche Wetterdienst weather data are openly available from https://opendata.dwd.de/climate_environment/CDC/ (DWD, 2022).
KA prepared the data, developed and implemented the modifications and executed the MONICA model simulations. KA also developed the scripts for model performance evaluation, sensitivity analysis and calibration and also wrote the paper.
JR gave advice in developing the sensitivity analysis algorithm, helped interpret MONICA's source code and supported the drafting of the paper.
CN advised on the execution of the MONICA model and supported in the drafting of the paper.
RD prepared the simulation of the C turnover models CCB, CENTURY, C-TOOL, ICBM and RothC. RD also gave advice, material and support in the drafting of the paper.
The contact author has declared that none of the authors has any competing interests.
Publisher's note: Copernicus Publications remains neutral with regard to jurisdictional claims made in the text, published maps, institutional affiliations, or any other geographical representation in this paper. While Copernicus Publications makes every effort to include appropriate place names, the final responsibility lies with the authors.
We are very grateful to the state government of Lower Saxony and the State Office for Mining, Energy and Geology for collecting and providing the data used in this model evaluation and optimisation. We also like to thank the Chair of Organic Farming and Agronomy, TUM School of Life Sciences, Technical University of Munich, and the Deutsche Wetterdienst for providing additional data. We are also grateful to Michael Berg-Mohnicke for his support regarding questions about the MONICA model. We also thank our colleagues Axel Don, Christopher Poeplau, Felix Seidel, Florian Schneider, Julia Schröder and Balazs Grosz for their support and comments on the paper. We would specifically like to thank the German Federal Ministry of Food and Agriculture and the Agency for Renewable Resources for financially supporting the MASTER project and our work.
This paper was edited by Marko Scholze and reviewed by Livia Rasche and Lorenzo Menichetti.
Abrahamsen, P. and Hansen, S.: Daisy: an open soil-crop-atmosphere system model, Environ. Model. Softw., 15, 313–330, https://doi.org/10.1016/S1364-8152(00)00003-7, 2000.
Agumas, B., Blagodatsky, S., Balume, I., Musyoki, M. K., Marhan, S., and Rasche, F.: Microbial carbon use efficiency during plant residue decomposition: Integrating multi-enzyme stoichiometry and C balance approach, Appl. Soil Ecol., 159, 103820, https://doi.org/10.1016/j.apsoil.2020.103820, 2021.
Aiteew, K., Rouhiainen, J., Nendel, C., and Dechow, R.: Evaluation and optimisation of the soil carbon turnover routine in the MONICA model (version 3.3.1) (3.3.1), Zenodo [code], https://doi.org/10.5281/zenodo.8380341, 2023a.
Aiteew, K., Rouhiainen, J., Nendel, C., and Dechow, R.: Evaluation and optimisation of the soil carbon turnover routine in the MONICA model (version 3.3.1) – MONICA model source code and data, Zenodo [data set], https://doi.org/10.5281/zenodo.8380332, 2023b.
Allen, R. G., Pereira, L. S., Raes, D., and Smith, M.: Crop evapotranspiration-Guidelines for computing crop water requirements-FAO Irrigation and drainage paper 56, Fao, Rome, 300, D05109, M-56, ISBN 92-5-104219-5, 1998.
Amelung, W., Bossio, D., de Vries, W., Kögel-Knabner, I., Lehmann, J., Amundson, R., Bol, R., Collins, C., Lal, R., Leifeld, J., Minasny, B., Pan, G., Paustian, K., Rumpel, C., Sanderman, J., van Groenigen, J. W., Mooney, S., van Wesemael, B., Wander, M., and Chabbi, A.: Towards a global-scale soil climate mitigation strategy, Nat. Commun., 11, 5427, https://doi.org/10.1038/s41467-020-18887-7, 2020.
Amundson, R. and Biardeau, L.: Opinion: Soil carbon sequestration is an elusive climate mitigation tool, P. Natl. Acad. Sci. USA, 115, 11652–11656, 2018.
Aon, M. A., Cabello, M. N., Sarena, D., Colaneri, A., Franco, M., Burgos, J., and Cortassa, S.: I. Spatio-temporal patterns of soil microbial and enzymatic activities in an agricultural soil, Appl. Soil Ecol., 18, 239–254, 2001.
Asseng, S., Ewert, F., Rosenzweig, C., Jones, J. W., Hatfield, J. L., Ruane, A. C., Boote, K. J., Thorburn, P. J., Rötter, R. P., Cammarano, D., Brisson, N., Basso, B., Martre, P., Aggarwal, P. K., Angulo, C., Bertuzzi, P., Biernath, C., Challinor, A. J., Doltra, J., Gayler, S., Goldberg, R., Grant, R., Heng, L., Hooker, J., Hunt, L. A., Ingwersen, J., Izaurralde, R. C., Kersebaum, K. C., Müller, C., Naresh Kumar, S., Nendel, C., O'Leary, G., Olesen, J. E., Osborne, T. M., Palosuo, T., Priesack, E., Ripoche, D., Semenov, M. A., Shcherbak, I., Steduto, P., Stöckle, C., Stratonovitch, P., Streck, T., Supit, I., Tao, F., Travasso, M., Waha, K., Wallach, D., White, J. W., Williams, J. R., and Wolf, J.: Uncertainty in simulating wheat yields under climate change, Nat. Clim. Change, 3, 827–832, https://doi.org/10.1038/nclimate1916, 2013.
Bassu, S., Brisson, N., Durand, J.-L., Boote, K., Lizaso, J., Jones, J. W., Rosenzweig, C., Ruane, A. C., Adam, M., Baron, C., Basso, B., Biernath, C., Boogaard, H., Conijn, S., Corbeels, M., Deryng, D., De Sanctis, G., Gayler, S., Grassini, P., Hatfield, J., Hoek, S., Izaurralde, C., Jongschaap, R., Kemanian, A. R., Kersebaum, K. C., Kim, S.-H., Kumar, N. S., Makowski, D., Müller, C., Nendel, C., Priesack, E., Pravia, M. V., Sau, F., Shcherbak, I., Tao, F., Teixeira, E., Timlin, D., and Waha, K.: How do various maize crop models vary in their responses to climate change factors?, Global Change Biol., 20, 2301–2320, https://doi.org/10.1111/gcb.12520, 2014.
Berg, G., Köberl, M., Rybakova, D., Müller, H., Grosch, R., and Smalla, K.: Plant microbial diversity is suggested as the key to future biocontrol and health trends, FEMS Microbiol. Ecol., 93, fix050, https://doi.org/10.1093/femsec/fix050, 2017.
Bolinder, M. A., Janzen, H. H., Gregorich, E. G., Angers, D. A., and VandenBygaart, A. J.: An approach for estimating net primary productivity and annual carbon inputs to soil for common agricultural crops in Canada, Agricult. Ecosyst. Environ., 118, 29–42, https://doi.org/10.1016/j.agee.2006.05.013, 2007.
Bonett, D. G. and Wright, T. A.: Sample size requirements for estimating pearson, kendall and spearman correlations, Psychometrika, 65, 23–28, https://doi.org/10.1007/BF02294183, 2000.
Bradford, M. A., Berg, B., Maynard, D. S., Wieder, W. R., and Wood, S. A.: Understanding the dominant controls on litter decomposition, J. Ecol., 104, 229–238, https://doi.org/10.1111/1365-2745.12507, 2016.
Bruun, S. and Jensen, L. S.: Initialisation of the soil organic matter pools of the Daisy model, Ecol. Model., 153, 291–295, 2002.
Bruun, S., Christensen, B. T., Hansen, E. M., Magid, J., and Jensen, L. S.: Calibration and validation of the soil organic matter dynamics of the Daisy model with data from the Askov long-term experiments, Soil Biol. Biochem., 35, 67–76, https://doi.org/10.1016/S0038-0717(02)00237-7, 2003.
Buckeridge, K. M., Mason, K. E., McNamara, N. P., Ostle, N., Puissant, J., Goodall, T., Griffiths, R. I., Stott, A. W., and Whitaker, J.: Environmental and microbial controls on microbial necromass recycling, an important precursor for soil carbon stabilization, Commun. Earth Environ., 1, 36, https://doi.org/10.1038/s43247-020-00031-4, 2020.
Cajamarca, S. M. N., Martins, D., da Silva, J., Fontenelle, M. R., Guedes, Í. M. R., de Figueiredo, C. C., and Pacheco Lima, C. E.: Heterogeneity in the Chemical Composition of Biofertilizers, Potential Agronomic Use, and Heavy Metal Contents of Different Agro-Industrial Wastes, Sustainability, 11, 1995, 2019.
Campolongo, F., Cariboni, J., and Saltelli, A.: An effective screening design for sensitivity analysis of large models, Environ. Model. Softw., 22, 1509–1518, https://doi.org/10.1016/j.envsoft.2006.10.004, 2007.
Čapek, P., Starke, R., Hofmockel, K. S., Bond-Lamberty, B., and Hess, N.: Apparent temperature sensitivity of soil respiration can result from temperature driven changes in microbial biomass, Soil Biol. Biochem., 135, 286–293, https://doi.org/10.1016/j.soilbio.2019.05.016, 2019.
Carvalhais, N., Forkel, M., Khomik, M., Bellarby, J., Jung, M., Migliavacca, M., Mu, M., Saatchi, S., Santoro, M., Thurner, M., Weber, U., Ahrens, B., Beer, C., Cescatti, A., Randerson, J. T., and Reichstein, M.: Global covariation of carbon turnover times with climate in terrestrial ecosystems, Nature, 514, 213–217, https://doi.org/10.1038/nature13731, 2014.
Chandel, A. K., Jiang, L., and Luo, Y.: Microbial Models for Simulating Soil Carbon Dynamics: A Review, J. Geophys. Res.-Biogeo., 128, e2023JG007436, https://doi.org/10.1029/2023JG007436, 2023.
Churchman, G. J., Singh, M., Schapel, A., Sarkar, B., and Bolan, N.: Clay minerals as the key to the sequestration of carbon in soils, Clays Clay Miner., 68, 135–143, https://doi.org/10.1007/s42860-020-00071-z, 2020.
Coleman, K. and Jenkinson, D. S.: RothC-26.3 - A Model for the turnover of carbon in soil, Evaluation of Soil Organic Matter Models, Berlin, Heidelberg, 237–246, 1996.
Conant, R. T., Drijber, R. A., Haddix, M. L., Parton, W. J., Paul, E. A., Plante, A. F., Six, J., and Steinweg, J. M.: Sensitivity of organic matter decomposition to warming varies with its quality, Global Change Biol., 14, 868–877, 2008.
Confalonieri, R., Bellocchi, G., Bregaglio, S., Donatelli, M., and Acutis, M.: Comparison of sensitivity analysis techniques: A case study with the rice model WARM, Ecol. Model., 221, 1897–1906, https://doi.org/10.1016/j.ecolmodel.2010.04.021, 2010.
Creamer, C. A., Foster, A. L., Lawrence, C., McFarland, J., Schulz, M., and Waldrop, M. P.: Mineralogy dictates the initial mechanism of microbial necromass association, Geochim. Cosmochim. Ac., 260, 161–176, https://doi.org/10.1016/j.gca.2019.06.028, 2019.
Curiel Yuste, J., Baldocchi, D. D., Gershenson, A., Goldstein, A., Misson, L., and Wong, S.: Microbial soil respiration and its dependency on carbon inputs, soil temperature and moisture, Global Change Biol., 13, 2018–2035, https://doi.org/10.1111/j.1365-2486.2007.01415.x, 2007.
Dechow, R., Franko, U., Kätterer, T., and Kolbe, H.: Evaluation of the RothC model as a prognostic tool for the prediction of SOC trends in response to management practices on arable land, Geoderma, 337, 463–478, https://doi.org/10.1016/j.geoderma.2018.10.001, 2019.
Doran, J., Mielke, L., and Power, J.: Microbial activity as regulated by soil water-filled pore space, Transactions 14th International Congress of Soil Science, Kyoto, Japan, Volume III, 94–99, August 1990.
DWD: Climate predictions and climate projections, Deutscher Wetterdienst, 36, 2021.
DWD: Index of /climate_environment/CDC/, Climate Data Center [data set], https://opendata.dwd.de/climate_environment/CDC/, last access: 17 October 2022.
Falloon, P., Smith, P., Coleman, K., and Marshall, S.: Estimating the size of inert organic matter pool from total soil organic carbon content for use the Rothamsted Carbon Model, Soil Biol. Biochem., 30, 1207–1211, https://doi.org/10.1016/S0038-0717(97)00256-3, 1998.
Fang, C. and Moncrieff, J. B.: The dependence of soil CO2 efflux on temperature, Soil Biol. Biochem., 33, 155–165, https://doi.org/10.1016/S0038-0717(00)00125-5, 2001.
Farina, R., Sándor, R., Abdalla, M., Álvaro-Fuentes, J., Bechini, L., Bolinder, M. A., Brilli, L., Chenu, C., Clivot, H., De Antoni Migliorati, M., Di Bene, C., Dorich, C. D., Ehrhardt, F., Ferchaud, F., Fitton, N., Francaviglia, R., Franko, U., Giltrap, D. L., Grant, B. B., Guenet, B., Harrison, M. T., Kirschbaum, M. U. F., Kuka, K., Kulmala, L., Liski, J., McGrath, M. J., Meier, E., Menichetti, L., Moyano, F., Nendel, C., Recous, S., Reibold, N., Shepherd, A., Smith, W. N., Smith, P., Soussana, J.-F., Stella, T., Taghizadeh-Toosi, A., Tsutskikh, E., and Bellocchi, G.: Ensemble modelling, uncertainty and robust predictions of organic carbon in long-term bare-fallow soils, Global Change Biol., 27, 904–928, https://doi.org/10.1111/gcb.15441, 2021.
Fierer, N., Colman, B. P., Schimel, J. P., and Jackson, R. B.: Predicting the temperature dependence of microbial respiration in soil: A continental-scale analysis, Global Biogeochem. Cycles, 20, 1–10, https://doi.org/10.1029/2005GB002644, 2006.
Fissore, C., Jurgensen, M. F., Pickens, J., Miller, C., Page-Dumroese, D., and Giardina, C. P.: Role of soil texture, clay mineralogy, location, and temperature in coarse wood decomposition – a mesocosm experiment, Ecosphere, 7, e01605, https://doi.org/10.1002/ecs2.1605, 2016.
Flessa, H., Dörsch, P., and Beese, F.: Seasonal variation of N2O and CH4 fluxes in differently managed arable soils in southern Germany, J. Geophys. Res.-Atmos., 100, 23115–23124, https://doi.org/10.1029/95JD02270, 1995.
Flessa, H., Fuß, R., Andres, M., Augustin, J., Christen, O., Dittert, K., Hegewald, H., Heilmann, H., Huth, V., Kage, H., Kern, J., Kesenheimer, K., Knieß, A., Köbke, S., Lewandowski, I., Mallast, J., Moffat, A. M., Mühling, K.-H., Öhlschläger, G., and Stichnothe, H.: Minderung von Treibhausgasemissionen im Rapsanbau unter besonderer Berücksichtigung der Stickstoffdüngung, Johann Heinrich von Thünen-Institut, Braunschweig, 2017.
Fomina, M. and Skorochod, I.: Microbial Interaction with Clay Minerals and Its Environmental and Biotechnological Implications, Minerals, 10, 861, 2020.
Fox, J., Weisberg, S., Price, P., Adler, D., Bates, D., Baud-Bovy, G., Bolker, B., Ellison, S., Firth, D., Friendly, M., Gorjanc, G., Graves, S., Heiberger, R., Krivitsky, P., Fox, J., Weisberg, S., Price, P., Adler, D., Bates, D., Baud-Bovy, G., Bolker, B., Ellison, S., Firth, D., Friendly, M., Gorjanc, G., Graves, S., Heiberger, R., Krivitsky, P., Laboissiere, R., Maechler, M., Monette, G., Murdoch, D., Nilsson, H., Ogle, D., Ripley, B., Venables, W., Walker, S., Winsemius, D., and Zeileis, A.: car: Companion to Applied Regression, Sage [code], https://r-forge.r-project.org/projects/car/ (last access: 5 April 2022), 2019.
Franko, U., Oelschlägel, B., and Schenk, S.: Simulation of temperature-, water- and nitrogen dynamics using the model CANDY, Ecol. Model., 81, 213–222, https://doi.org/10.1016/0304-3800(94)00172-E, 1995.
Franko, U., Kolbe, H., Thiel, E., and Ließ, E.: Multi-site validation of a soil organic matter model for arable fields based on generally available input data, Geoderma, 166, 119–134, https://doi.org/10.1016/j.geoderma.2011.07.019, 2011.
Franzluebbers, A. J.: Microbial activity in response to water-filled pore space of variably eroded southern Piedmont soils, Appl. Soil Ecol., 11, 91–101, https://doi.org/10.1016/S0929-1393(98)00128-0, 1999.
Freibauer, A., Rounsevell, M. D. A., Smith, P., and Verhagen, J.: Carbon sequestration in the agricultural soils of Europe, Geoderma, 122, 1–23, https://doi.org/10.1016/j.geoderma.2004.01.021, 2004.
Frolking, S. E., Mosier, A. R., Ojima, D. S., Li, C., Parton, W. J., Potter, C. S., Priesack, E., Stenger, R., Haberbosch, C., Dörsch, P., Flessa, H., and Smith, K. A.: Comparison of N2O emissions from soils at three temperate agricultural sites: simulations of year-round measurements by four models, Nutr. Cycl. Agroecosys., 52, 77–105, https://doi.org/10.1023/A:1009780109748, 1998.
Fujisaki, K., Chevallier, T., Chapuis-Lardy, L., Albrecht, A., Razafimbelo, T., Masse, D., Ndour, Y. B., and Chotte, J.-L.: Soil carbon stock changes in tropical croplands are mainly driven by carbon inputs: A synthesis, Agricul. Ecosyst. Environ., 259, 147–158, https://doi.org/10.1016/j.agee.2017.12.008, 2018.
Fuss, S., Lamb, W. F., Callaghan, M. W., Hilaire, J., Creutzig, F., Amann, T., Beringer, T., de Oliveira Garcia, W., Hartmann, J., Khanna, T., Luderer, G., Nemet, G. F., Rogelj, J., Smith, P., Vicente, J. L. V., Wilcox, J., del Mar Zamora Dominguez, M., and Minx, J. C.: Negative emissions – Part 2: Costs, potentials and side effects, Environ. Res. Lett., 13, 063002, https://doi.org/10.1088/1748-9326/aabf9f, 2018.
Gabriel, C.-E. and Kellman, L.: Investigating the role of moisture as an environmental constraint in the decomposition of shallow and deep mineral soil organic matter of a temperate coniferous soil, Soil Biol. Biochem., 68, 373–384, https://doi.org/10.1016/j.soilbio.2013.10.009, 2014.
Gelman, A. and Rubin, D. B.: Inference from iterative simulation using multiple sequences, Stat. Sci., 7, 457–472, 1992.
Geyer, K., Schnecker, J., Grandy, A. S., Richter, A., and Frey, S.: Assessing microbial residues in soil as a potential carbon sink and moderator of carbon use efficiency, Biogeochemistry, 151, 237–249, https://doi.org/10.1007/s10533-020-00720-4, 2020.
Gillabel, J., Cebrian-Lopez, B., Six, J., and Merckx, R.: Experimental evidence for the attenuating effect of SOM protection on temperature sensitivity of SOM decomposition, Global Change Biol., 16, 2789–2798, https://doi.org/10.1111/j.1365-2486.2009.02132.x, 2010.
Gilmour, J. T., Norman, R. J., Mauromoustakos, A., and Gale, P. M.: Kinetics of Crop Residue Decomposition: Variability among Crops and Years, Soil Sci. Soc. Am. J., 62, 750–755, https://doi.org/10.2136/sssaj1998.03615995006200030030x, 1998.
Gleixner, G.: Soil organic matter dynamics: a biological perspective derived from the use of compound-specific isotopes studies, Ecol. Res., 28, 683–695, 2013.
Gross, A. and Glaser, B.: Meta-analysis on how manure application changes soil organic carbon storage, Sci. Rep.-UK, 11, 5516, https://doi.org/10.1038/s41598-021-82739-7, 2021.
Gurung, R. B., Ogle, S. M., Breidt, F. J., Williams, S. A., and Parton, W. J.: Bayesian calibration of the DayCent ecosystem model to simulate soil organic carbon dynamics and reduce model uncertainty, Geoderma, 376, 114529, https://doi.org/10.1016/j.geoderma.2020.114529, 2020.
Haddix, M., Steinweg, M., Conant, R., Plante, A., Paul, E., and Six, J.: Effect of Temperature on the Dynamics of Different Soil Organic Matter Fractions, The 18th World Congress of Soil Science, Philadelphia, Pennsylvania, USA, 15 July 2006.
Hansen, S., Jensen, H., Nielsen, N., and Svendsen, H.: Simulation of nitrogen dynamics and biomass production in winter wheat using the Danish simulation model DAISY, Fert. Res., 27, 245–259, 1991.
Hastings, W. K.: Monte Carlo sampling methods using Markov chains and their applications, Biometrika, 57, 97–109, https://doi.org/10.1093/biomet/57.1.97, 1970.
Höper, H. and Meesenburg, H.: 30 Jahre Bodendauerbeobachtung in Niedersachsen, GeoBerichte, 39, 275 pp., https://doi.org/10.48476/geober_39_2021, 2021.
Iooss, B., Da Veiga, S., Janon, A., and Pujol, G.: Sensitivity: Global Sensitivity Analysis of Model Outputs, R package version 1.26.0, 2020.
Jacobs, A., Poeplau, C., Weiser, C., Fahrion-Nitschke, A., and Don, A.: Exports and inputs of organic carbon on agricultural soils in Germany, Nutr. Cycl. Agroecosys., 118, 249–271, https://doi.org/10.1007/s10705-020-10087-5, 2020.
Jargowsky, P. A. and Yang, R.: Descriptive and Inferential Statistics, in: Encyclopedia of Social Measurement, edited by: Kempf-Leonard, K., Elsevier, New York, 659–668, https://doi.org/10.1016/B0-12-369398-5/00145-6, 2005.
Jeuffroy, M.-H. and Ney, B.: Crop physiology and productivity, Field Crops Research, 53, 3–16, https://doi.org/10.1016/S0378-4290(97)00019-1, 1997.
Joergensen, R. G., Brookes, P. C., and Jenkinson, D. S.: Survival of the soil microbial biomass at elevated temperatures, Soil Biol. Biochem., 22, 1129–1136, https://doi.org/10.1016/0038-0717(90)90039-3, 1990.
Jordon, M. W. and Smith, P.: Modelling soil carbon stocks following reduced tillage intensity: A framework to estimate decomposition rate constant modifiers for RothC-26.3, demonstrated in north-west Europe, Soil Till. Res., 222, 105428, https://doi.org/10.1016/j.still.2022.105428, 2022.
Kallenbach, C. M., Frey, S. D., and Grandy, A. S.: Direct evidence for microbial-derived soil organic matter formation and its ecophysiological controls, Nat. Commun., 7, 13630, https://doi.org/10.1038/ncomms13630, 2016.
Kendall, M. G.: A New Measure of Rank Correlation, Biometrika, 30, 81–93, https://doi.org/10.2307/2332226, 1938.
Kendall, M. G.: Rank correlation methods, Oxford University Press, London 1948.
Kersebaum, K. C.: Application of a simple management model to simulate water and nitrogen dynamics, Ecol. Model., 81, 145–156, https://doi.org/10.1016/0304-3800(94)00167-G, 1995.
Kersebaum, K. C.: Modelling nitrogen dynamics in soil–crop systems with HERMES, Nutr. Cycl. Agroecosys., 77, 39–52, 2006.
Kindler, R., Miltner, A., Richnow, H.-H., and Kästner, M.: Fate of gram-negative bacterial biomass in soil – mineralization and contribution to SOM, Soil Biol. Biochem., 38, 2860–2870, https://doi.org/10.1016/j.soilbio.2006.04.047, 2006.
Koishi, A., Bragazza, L., Maltas, A., Guillaume, T., and Sinaj, S.: Long-Term Effects of Organic Amendments on Soil Organic Matter Quantity and Quality in Conventional Cropping Systems in Switzerland, Agronomy, 10, 1977, https://doi.org/10.3390/agronomy10121977, 2020.
Kollas, C., Kersebaum, K. C., Nendel, C., Manevski, K., Müller, C., Palosuo, T., Armas-Herrera, C. M., Beaudoin, N., Bindi, M., Charfeddine, M., Conradt, T., Constantin, J., Eitzinger, J., Ewert, F., Ferrise, R., Gaiser, T., Cortazar-Atauri, I. G. d., Giglio, L., Hlavinka, P., Hoffmann, H., Hoffmann, M. P., Launay, M., Manderscheid, R., Mary, B., Mirschel, W., Moriondo, M., Olesen, J. E., Öztürk, I., Pacholski, A., Ripoche-Wachter, D., Roggero, P. P., Roncossek, S., Rötter, R. P., Ruget, F., Sharif, B., Trnka, M., Ventrella, D., Waha, K., Wegehenkel, M., Weigel, H.-J., and Wu, L.: Crop rotation modelling – A European model intercomparison, Eur. J. Agron., 70, 98–111, https://doi.org/10.1016/j.eja.2015.06.007, 2015.
Kostková, M., Hlavinka, P., Pohanková, E., Kersebaum, K. C., Nendel, C., Gobin, A., Olesen, J. E., Ferrise, R., Dibari, C., Takáč, J., Topaj, A., Medvedev, S., Hoffmann, M. P., Stella, T., Balek, J., Ruiz-Ramos, M., Rodríguez, A., Hoogenboom, G., Shelia, V., Ventrella, D., Giglio, L., Sharif, B., Oztürk, I., Rötter, R. P., Balkovič, J., Skalský, R., Moriondo, M., Thaler, S., Žalud, Z., and Trnka, M.: Performance of 13 crop simulation models and their ensemble for simulating four field crops in Central Europe, J. Agric. Sci., 159, 69–89, https://doi.org/10.1017/S0021859621000216, 2021.
Kothari, K., Battisti, R., Boote, K. J., Archontoulis, S. V., Confalone, A., Constantin, J., Cuadra, S. V., Debaeke, P., Faye, B., Grant, B., Hoogenboom, G., Jing, Q., van der Laan, M., Macena da Silva, F. A., Marin, F. R., Nehbandani, A., Nendel, C., Purcell, L. C., Qian, B., Ruane, A. C., Schoving, C., Silva, E. H. F. M., Smith, W., Soltani, A., Srivastava, A., Vieira, N. A., Slone, S., and Salmerón, M.: Are soybean models ready for climate change food impact assessments?, Eur. J. Agron., 135, 126482, https://doi.org/10.1016/j.eja.2022.126482, 2022.
Lashermes, G., Nicolardot, B., Parnaudeau, V., Thuriès, L., Chaussod, R., Guillotin, M. L., Linères, M., Mary, B., Metzger, L., Morvan, T., Tricaud, A., Villette, C., and Houot, S.: Indicator of potential residual carbon in soils after exogenous organic matter application, Eur. J. Soil Sci., 60, 297–310, https://doi.org/10.1111/j.1365-2389.2008.01110.x, 2009.
LBEG (Landesamt für Bergbau, Energie und Geologie): Das Boden-Dauerbeobachtungsprogramm von Niedersachsen, https://www.lbeg.niedersachsen.de/boden_grundwasser/bodenmonitoring/bodendauerbeobachtung/das-boden-dauerbeobachtungsprogramm-von-niedersachsen-572.html, LBEG [data set], last access: 24 November 2023.
Lehuger, S., Gabrielle, B., Oijen, M. v., Makowski, D., Germon, J. C., Morvan, T., and Hénault, C.: Bayesian calibration of the nitrous oxide emission module of an agro-ecosystem model, Agric. Ecosyst. Environ., 133, 208–222, https://doi.org/10.1016/j.agee.2009.04.022, 2009.
Leidel, S., Augustin, J., Köppen, D., and Merbach, W.: Einfluss Unterschiedlicher Organisch-Mineralischer N-Düngung auf die Lachgas- und Methanemission eines ackerbaulich genutzten standortes Norddeutschlands, Arch. Agron. Soil Sci., 45, 453–469, https://doi.org/10.1080/03650340009366141, 2000.
Levene, H.: Robust Test for Equality of Variances, in: Contributions to Probability and Statistics: Essays in Honor of Harold Hotelling, edited by: Olkin, I., Stanford University Press, Palo Alto, 278–292, 1960.
Li, C., Frolking, S., Crocker, G. J., Grace, P. R., Klír, J., Körchens, M., and Poulton, P. R.: Simulating trends in soil organic carbon in long-term experiments using the DNDC model, Geoderma, 81, 45–60, https://doi.org/10.1016/S0016-7061(97)00080-3, 1997.
Liang, C. and Balser, T. C.: Microbial production of recalcitrant organic matter in global soils: implications for productivity and climate policy, Nat. Rev. Microbiol., 9, 75, https://doi.org/10.1038/nrmicro2386-c1, 2011.
Liddle, K., McGonigle, T., and Koiter, A.: Microbe Biomass in Relation to Organic Carbon and Clay in Soil, Soil Syst., 4, 41, https://doi.org/10.3390/soilsystems4030041, 2020.
Liebig, M., Jones, A., Doran, J., and Mielke, L.: Potential soil respiration and relationship to soil properties in ridge tillage, Soil Sci. Soc. Am. J., 59, 1430–1435, 1995.
Linn, D. M. and Doran, J. W.: Effect of water-filled pore space on carbon dioxide and nitrous oxide production in tilled and nontilled soils, Soil Sci. Soc. Am. J., 48, 1267–1272, 1984.
Liu, Y., He, N., Wen, X., Xu, L., Sun, X., Yu, G., Liang, L., and Schipper, L. A.: The optimum temperature of soil microbial respiration: Patterns and controls, Soil Biol. Biochem., 121, 35–42, https://doi.org/10.1016/j.soilbio.2018.02.019, 2018.
Louis, B. P., Maron, P.-A., Viaud, V., Leterme, P., and Menasseri-Aubry, S.: Soil C and N models that integrate microbial diversity, Environ. Chem. Lett., 14, 331–344, https://doi.org/10.1007/s10311-016-0571-5, 2016.
Lovenduski, N. S. and Bonan, G. B.: Reducing uncertainty in projections of terrestrial carbon uptake, Environ. Res. Lett., 12, 044020, https://doi.org/10.1088/1748-9326/aa66b8, 2017.
Lowder, S. K., Skoet, J., and Raney, T.: The Number, Size, and Distribution of Farms, Smallholder Farms, and Family Farms Worldwide, World Development, 87, 16–29, https://doi.org/10.1016/j.worlddev.2015.10.041, 2016.
Lugato, E., Bampa, F., Panagos, P., Montanarella, L., and Jones, A.: Potential carbon sequestration of European arable soils estimated by modelling a comprehensive set of management practices, Global Change Biol., 20, 3557–3567, https://doi.org/10.1111/gcb.12551, 2014.
Luo, Z., Wang, E., and Sun, O. J.: Can no-tillage stimulate carbon sequestration in agricultural soils? A meta-analysis of paired experiments, Agric. Ecosyst. Environ., 139, 224–231, https://doi.org/10.1016/j.agee.2010.08.006, 2010.
Luo, Z., Feng, W., Luo, Y., Baldock, J., and Wang, E.: Soil organic carbon dynamics jointly controlled by climate, carbon inputs, soil properties and soil carbon fractions, Global Change Biol., 23, 4430–4439, https://doi.org/10.1111/gcb.13767, 2017.
Ma, S., He, F., Tian, D., Zou, D., Yan, Z., Yang, Y., Zhou, T., Huang, K., Shen, H., and Fang, J.: Variations and determinants of carbon content in plants: a global synthesis, Biogeosciences, 15, 693–702, https://doi.org/10.5194/bg-15-693-2018, 2018.
Mallast, J., Stichnothe, H., Flessa, H., Fuß, R., Lucas-Moffat, A., Petersen-Schlapkohl, U., Augustin, J., Hagemann, U., Kesenheimer, K., Ruser, R., Suárez, T., Prochnow, A., Dittert, K., Köbke, S., Huth, V., Glatzel, S., Räbiger, T., Knieß, A., Kage, H., and Christen, O.: Multi-variable experimental data set of agronomic data and gaseous soil emissions from maize, oilseed rape and other energy crops at eight sites in Germany, Open Data Journal for Agricultural Research, 7, 11–19, https://doi.org/10.18174/odjar.v7i0.16124, 2021.
Manzoni, S., Taylor, P., Richter, A., Porporato, A., and Ågren, G. I.: Environmental and stoichiometric controls on microbial carbon-use efficiency in soils, New Phytologist, 196, 79–91, https://doi.org/10.1111/j.1469-8137.2012.04225.x, 2012.
McCartney, D. H., Block, H. C., Dubeski, P. L., and Ohama, A. J.: Review: The composition and availability of straw and chaff from small grain cereals for beef cattle in western Canada, Can. J. Anim. Sci., 86, 443–455, https://doi.org/10.4141/A05-092, 2006.
McGill, W. B., Cannon, K. R., Robertson, J. A., and Cook, F. D.: Dynamics of soil microbial biomass and water-soluble organic C in Breton L after 50 years of cropping to two rotations, Can. J. Soil Sci., 66, 1–19, 1986.
Menichetti, L., Reyes Ortigoza, A. L., García, N., Giagnoni, L., Nannipieri, P., and Renella, G.: Thermal sensitivity of enzyme activity in tropical soils assessed by the Q10 and equilibrium model, Biol. Fert. Soils, 51, 299–310, https://doi.org/10.1007/s00374-014-0976-x, 2015.
Metropolis, N., Rosenbluth, A. W., Rosenbluth, M. N., Teller, A. H., and Teller, E.: Equation of state calculations by fast computing machines, J. Chem. Phys., 21, 1087–1092, 1953.
Meyer, N., Welp, G., and Amelung, W.: The Temperature Sensitivity (Q10) of Soil Respiration: Controlling Factors and Spatial Prediction at Regional Scale Based on Environmental Soil Classes, Global Biogeochem. Cycles, 32, 306–323, https://doi.org/10.1002/2017GB005644, 2018.
Miltner, A., Bombach, P., Schmidt-Brücken, B., and Kästner, M.: SOM genesis: microbial biomass as a significant source, Biogeochemistry, 111, 41–55, https://doi.org/10.1007/s10533-011-9658-z, 2012.
Minasny, B., Malone, B. P., McBratney, A. B., Angers, D. A., Arrouays, D., Chambers, A., Chaplot, V., Chen, Z.-S., Cheng, K., Das, B. S., Field, D. J., Gimona, A., Hedley, C. B., Hong, S. Y., Mandal, B., Marchant, B. P., Martin, M., McConkey, B. G., Mulder, V. L., O'Rourke, S., Richer-de-Forges, A. C., Odeh, I., Padarian, J., Paustian, K., Pan, G., Poggio, L., Savin, I., Stolbovoy, V., Stockmann, U., Sulaeman, Y., Tsui, C.-C., Vågen, T.-G., van Wesemael, B., and Winowiecki, L.: Soil carbon 4 per mille, Geoderma, 292, 59–86, https://doi.org/10.1016/j.geoderma.2017.01.002, 2017.
Minunno, F., Van Oijen, M., and Pereira, J.: Selecting Parameters for Bayesian Calibration of a Process-Based Model: A Methodology Based on Canonical Correlation Analysis, Journal on Uncertainty Quantification, 1, 370–385, https://doi.org/10.1137/120891344, 2013.
Möller, K. and Schultheiß, U.: Chemical characterization of commercial organic fertilizers, Arch. Agron. Soil Sci., 61, 989–1012, https://doi.org/10.1080/03650340.2014.978763, 2015.
Mondal, S., Chakraborty, D., Bandyopadhyay, K., Aggarwal, P., and Rana, D. S.: A global analysis of the impact of zero-tillage on soil physical condition, organic carbon content, and plant root response, Land Degrad. Dev., 31, 557–567, https://doi.org/10.1002/ldr.3470, 2020.
Morris, M. D.: Factorial Sampling Plans for Preliminary Computational Experiments, Technometrics, 33, 161–174, https://doi.org/10.1080/00401706.1991.10484804, 1991.
Mueller, T., Jensen, L. S., Hansen, S., and Nielsen, N. E.: Simulating soil carbon and nitrogen dynamics with the soil-plant-atmosphere system model DAISY, Evaluation of Soil Organic Matter Models, Berlin, Heidelberg, 275–281, 1996.
Murphy, B.: Soil Carbon Sequestration as an Elusive Climate Mitigation Tool, in: No-till Farming Systems for Sustainable Agriculture: Challenges and Opportunities, edited by: Dang, Y. P., Dalal, R. C., and Menzies, N. W., Springer International Publishing, Cham, 337–353, https://doi.org/10.1007/978-3-030-46409-7_20, 2020.
Nash, J. E. and Sutcliffe, J. V.: River flow forecasting through conceptual models part I – A discussion of principles, J. Hydrol., 10, 282–290, https://doi.org/10.1016/0022-1694(70)90255-6, 1970.
Nendel, C.: MONICA: A Simulation Model for Nitrogen and Carbon Dynamics in Agro-Ecosystems, in: Novel Measurement and Assessment Tools for Monitoring and Management of Land and Water Resources in Agricultural Landscapes of Central Asia, edited by: Mueller, L., Saparov, A., and Lischeid, G., Springer International Publishing, Cham, 389–405, https://doi.org/10.1007/978-3-319-01017-5_23, 2014.
Nendel, C., Berg, M., Kersebaum, K. C., Mirschel, W., Specka, X., Wegehenkel, M., Wenkel, K., and Wieland, R.: The MONICA model: Testing predictability for crop growth, soil moisture and nitrogen dynamics, Ecol. Model., 222, 1614–1625, 2011.
Nendel, C., Kersebaum, K. C., Mirschel, W., and Wenkel, K. O.: Testing farm management options as climate change adaptation strategies using the MONICA model, Eur. J. Agron., 52, 47–56, https://doi.org/10.1016/j.eja.2012.09.005, 2014.
Nguye, T.-T. and Marschner, P.: Respiration in mixes of sandy and clay soils: influence of clay type and addition rate, J. Soil Sci. Plant Nutr,, 14, 881–887, 2014.
Oakley, J. E. and O'Hagan, A.: Uncertainty in prior elicitations: a nonparametric approach, Biometrika, 94, 427–441, https://doi.org/10.1093/biomet/asm031, 2007.
Parton, W. J.: Ecosystem model comparisons: science or fantasy world?, Evaluation of Soil Organic Matter Models, Berlin, Heidelberg, 133–142, 1996.
Paustian, K., Parton, W. J., and Persson, J.: Modeling soil organic matter in organic-amended and nitrogen-fertilized long-term plots, Soil Sci. Soc. Am. J., 56, 476–488, 1992.
Peltre, C., Christensen, B., Dragon, S., Icard, C., Kätterer, T., and Houot, S.: RothC simulation of carbon accumulation in soil after repeated application of widely different organic amendments, Soil Biol. Biochem., 52, 49–60, https://doi.org/10.1016/j.soilbio.2012.03.023, 2012.
Pianosi, F., Beven, K., Freer, J., Hall, J. W., Rougier, J., Stephenson, D. B., and Wagener, T.: Sensitivity analysis of environmental models: A systematic review with practical workflow, Environ. Model. Softw., 79, 214–232, https://doi.org/10.1016/j.envsoft.2016.02.008, 2016.
Pietikäinen, J., Pettersson, M., and Bååth, E.: Comparison of temperature effects on soil respiration and bacterial and fungal growth rates, FEMS Microbiol Ecol, 52, 49–58, https://doi.org/10.1016/j.femsec.2004.10.002, 2005.
Plummer, M., Best, N., Cowles, K., and Vines, K.: CODA: convergence diagnosis and output analysis for MCMC, R news, 6, 7–11, 2006.
Poeplau, C. and Don, A.: Carbon sequestration in agricultural soils via cultivation of cover crops – A meta-analysis, Agriculture, Ecosyst. Environ., 200, 33–41, https://doi.org/10.1016/j.agee.2014.10.024, 2015.
Poeplau, C., Jacobs, A., Don, A., Vos, C., Schneider, F., Wittnebel, M., Tiemeyer, B., Heidkamp, A., Prietz, R., and Flessa, H.: Stocks of organic carbon in German agricultural soils – Key results of the first comprehensive inventory, J. Plant Nutr. Soil Sci., 183, 665–681, https://doi.org/10.1002/jpln.202000113, 2020.
Poeplau, C., Don, A., and Schneider, F.: Roots are key to increasing the mean residence time of organic carbon entering temperate agricultural soils, Global Change Biol., 27, 4921–4934, https://doi.org/10.1111/gcb.15787, 2021.
Pujol, G.: Simplex-based screening designs for estimating metamodels, Reliability Engineering & System Safety, 94, 1156–1160, https://doi.org/10.1016/j.ress.2008.08.002, 2009.
Qiao, Y., Wang, J., Liang, G., Du, Z., Zhou, J., Zhu, C., Huang, K., Zhou, X., Luo, Y., Yan, L., and Xia, J.: Global variation of soil microbial carbon-use efficiency in relation to growth temperature and substrate supply, Sci. Rep.-UK, 9, 5621, https://doi.org/10.1038/s41598-019-42145-6, 2019.
Riaz, M. and Marschner, P.: Sandy Soil Amended with Clay Soil: Effect of Clay Soil Properties on Soil Respiration, Microbial Biomass, and Water Extractable Organic C, J. Soil Sci. Plant Nutr., 20, 2465–2470, https://doi.org/10.1007/s42729-020-00312-z, 2020.
Richardson, J., Chatterjee, A., and Darrel Jenerette, G.: Optimum temperatures for soil respiration along a semi-arid elevation gradient in southern California, Soil Biol. Biochem., 46, 89–95, https://doi.org/10.1016/j.soilbio.2011.11.008, 2012.
Riggers, C., Poeplau, C., Don, A., Bamminger, C., Höper, H., and Dechow, R.: Multi-model ensemble improved the prediction of trends in soil organic carbon stocks in German croplands, Geoderma, 345, 17–30, https://doi.org/10.1016/j.geoderma.2019.03.014, 2019.
Riggers, C., Poeplau, C., Don, A., Frühauf, C., and Dechow, R.: How much carbon input is required to preserve or increase projected soil organic carbon stocks in German croplands under climate change?, Plant Soil, 460, 417–433, https://doi.org/10.1007/s11104-020-04806-8, 2021.
Roß, C.-L., Baumecker, M., Ellmer, F., and Kautz, T.: Organic Manure Increases Carbon Sequestration Far beyond the “4 per 1000 Initiative”; Goal on a Sandy Soil in the Thyrow Long-Term Field Experiment DIV.2, Agriculture, 12, 170, 2022.
Rötter, R. P., Palosuo, T., Kersebaum, K. C., Angulo, C., Bindi, M., Ewert, F., Ferrise, R., Hlavinka, P., Moriondo, M., and Nendel, C.: Simulation of spring barley yield in different climatic zones of Northern and Central Europe: a comparison of nine crop models, Field Crops Res., 133, 23–36, 2012.
Saifuddin, M., Bhatnagar, J. M., Segrè, D., and Finzi, A. C.: Microbial carbon use efficiency predicted from genome-scale metabolic models, Nat. Commun., 10, 3568, https://doi.org/10.1038/s41467-019-11488-z, 2019.
Salo, T. J., Palosuo, T., Kersebaum, K. C., Nendel, C., Angulo, C., Ewert, F., Bindi, M., Calanca, P., Klein, T., and Moriondo, M.: Comparing the performance of 11 crop simulation models in predicting yield response to nitrogen fertilization, J. Agric. Sci., 154, 1218–1240, 2016.
Sanderman, J., Hengl, T., and Fiske, G. J.: Correction for Sanderman et al., Soil carbon debt of 12,000 years of human land use, P. Natl. Acad. Sci. USA, 115, E1700–E1700, https://doi.org/10.1073/pnas.1800925115, 2018.
Sarrazin, F., Pianosi, F., and Wagener, T.: Global Sensitivity Analysis of environmental models: Convergence and validation, Environ. Model. Softw., 79, 135–152, https://doi.org/10.1016/j.envsoft.2016.02.005, 2016.
Schapel, A., Marschner, P., and Churchman, J.: Clay amount and distribution influence organic carbon content in sand with subsoil clay addition, Soil Till. Res., 184, 253–260, https://doi.org/10.1016/j.still.2018.08.001, 2018.
Schimel, J., Balser, T. C., and Wallenstein, M.: Microbial stress-response physiology and its implications for ecosystem function, Ecology, 88, 1386–1394, https://doi.org/10.1890/06-0219, 2007.
Schmädeke, F.: Lachgas- und Methaneinflüsse eines Gley-Auenbodens unter dem Einfluss einer Rapsfruchtfolge und in Abhängigkeit von der N-Düngung, Dissertation, Fakultät für Forstwissenschaften und Waldökologie, Georg-August-Universität Göttingen, 149 pp., https://doi.org/10.53846/goediss-2312, 1998.
Schnug, E., Oswald, P., and Haneklaus, S.: Organic manure management and efficiency: Role of organic fertilizers and their management practices, in: Fertilizers and Environment: Proceedings of the International Symposium “Fertilizers and Environment”, Salamanca, Spain, 26–29 September 1994, edited by: Rodriguez-Barrueco, C., Springer Netherlands, Dordrecht, 259–265, https://doi.org/10.1007/978-94-009-1586-2_44, 1996.
Schoups, G. and Vrugt, J. A.: A formal likelihood function for parameter and predictive inference of hydrologic models with correlated, heteroscedastic, and non-Gaussian errors, Water Resour. Res., 46, 1–17, https://doi.org/10.1029/2009WR008933, 2010.
Schroeder, J., Jannoura, R., Beuschel, R., Pfeiffer, B., Dyckmans, J., Murugan, R., Chavannavar, S., Wachendorf, C., and Joergensen, R. G.: Carbon use efficiency and microbial functional diversity in a temperate Luvisol and a tropical Nitisol after millet litter and N addition, Biol. Fert. Soils, 56, 1139–1150, https://doi.org/10.1007/s00374-020-01487-4, 2020.
Scott, N. A., Cole, C. V., Elliott, E. T., and Huffman, S. A.: Soil Textural Control on Decomposition and Soil Organic Matter Dynamics, Soil Sci. Soc. Am. J., 60, 1102–1109, https://doi.org/10.2136/sssaj1996.03615995006000040020x, 1996.
Seitz, D., Fischer, L. M., Dechow, R., Wiesmeier, M., and Don, A.: The potential of cover crops to increase soil organic carbon storage in German croplands, Plant Soil, 488, 157–173, https://doi.org/10.1007/s11104-022-05438-w, 2022.
Semenov, V., Pautova, N., Lebedeva, T., Khromychkina, D., Semenova, N., and lopes de Gerenyu, V.: Plant Residues Decomposition and Formation of Active Organic Matter in the Soil of the Incubation Experiments, Eurasian Soil Science, 52, 1183–1194, https://doi.org/10.1134/S1064229319100119, 2019.
Shapiro, S. S. and Wilk, M. B.: An Analysis of Variance Test for Normality (Complete Samples), Biometrika, 52, 591–611, https://doi.org/10.2307/2333709, 1965.
Shi, Z., Allison, S. D., He, Y., Levine, P. A., Hoyt, A. M., Beem-Miller, J., Zhu, Q., Wieder, W. R., Trumbore, S., and Randerson, J. T.: The age distribution of global soil carbon inferred from radiocarbon measurements, Nat. Geosci., 13, 555–559, https://doi.org/10.1038/s41561-020-0596-z, 2020.
Shoemaker, W. R., Jones, S. E., Muscarella, M. E., Behringer, M. G., Lehmkuhl, B. K., and Lennon, J. T.: Microbial population dynamics and evolutionary outcomes under extreme energy limitation, P. Natl. Acad. Sci. USA, 118, e2101691118, https://doi.org/10.1073/pnas.2101691118, 2021.
Sierra, C. A., Trumbore, S. E., Davidson, E. A., Vicca, S., and Janssens, I.: Sensitivity of decomposition rates of soil organic matter with respect to simultaneous changes in temperature and moisture, J. Adv. Model. Earth Sy., 7, 335–356, https://doi.org/10.1002/2014MS000358, 2015.
Six, J. and Paustian, K.: Aggregate-associated soil organic matter as an ecosystem property and a measurement tool, Soil Biol. Biochem., 68, A4–A9, https://doi.org/10.1016/j.soilbio.2013.06.014, 2014.
Smith, P., Smith, J., Powlson, D., McGill, W., Arah, J., Chertov, O., Coleman, K., Franko, U., Frolking, S., and Jenkinson, D.: A comparison of the performance of nine soil organic matter models using datasets from seven long-term experiments, Geoderma, 81, 153–225, 1997.
Smith, P., Andrén, O., Karlsson, T., Perälä, P., Regina, K., Rounsevell, M., and Van Wesemael, B.: Carbon sequestration potential in European croplands has been overestimated, Global Change Biol., 11, 2153–2163, https://doi.org/10.1111/j.1365-2486.2005.01052.x, 2005.
Sokol, N. W. and Bradford, M. A.: Microbial formation of stable soil carbon is more efficient from belowground than aboveground input, Nat. Geosci., 12, 46–53, https://doi.org/10.1038/s41561-018-0258-6, 2019.
Sommer, R. and Bossio, D.: Dynamics and climate change mitigation potential of soil organic carbon sequestration, J. Environ. Manage., 144C, 83–87, https://doi.org/10.1016/j.jenvman.2014.05.017, 2014.
Specka, X., Nendel, C., Hagemann, U., Pohl, M., Hoffmann, M., Barkusky, D., Augustin, J., Sommer, M., and van Oost, K.: Reproducing CO2 exchange rates of a crop rotation at contrasting terrain positions using two different modelling approaches, Soil Till. Res., 156, 219–229, https://doi.org/10.1016/j.still.2015.05.007, 2016.
Spohn, M., Pötsch, E. M., Eichorst, S. A., Woebken, D., Wanek, W., and Richter, A.: Soil microbial carbon use efficiency and biomass turnover in a long-term fertilization experiment in a temperate grassland, Soil Biol. Biochem., 97, 168–175, https://doi.org/10.1016/j.soilbio.2016.03.008, 2016.
Steinmann, T., Welp, G., Wolf, A., Holbeck, B., Große-Rüschkamp, T., and Amelung, W.: Repeated monitoring of organic carbon stocks after eight years reveals carbon losses from intensively managed agricultural soils in Western Germany, J. Plant Nutr. Soil Sci., 179, 355–366, https://doi.org/10.1002/jpln.201500503, 2016.
Sulman, B. N., Moore, J. A. M., Abramoff, R., Averill, C., Kivlin, S., Georgiou, K., Sridhar, B., Hartman, M. D., Wang, G., Wieder, W. R., Bradford, M. A., Luo, Y., Mayes, M. A., Morrison, E., Riley, W. J., Salazar, A., Schimel, J. P., Tang, J., and Classen, A. T.: Multiple models and experiments underscore large uncertainty in soil carbon dynamics, Biogeochemistry, 141, 109–123, 2018.
Svensson, M., Jansson, P.-E., Gustafsson, D., Kleja, D. B., Langvall, O., and Lindroth, A.: Bayesian calibration of a model describing carbon, water and heat fluxes for a Swedish boreal forest stand, Ecol. Model., 213, 331–344, https://doi.org/10.1016/j.ecolmodel.2008.01.001, 2008.
Taghizadeh-Toosi, A., Cong, W.-F., Eriksen, J., Mayer, J., Olesen, J. E., Keel, S. G., Glendining, M., Kätterer, T., and Christensen, B. T.: Visiting dark sides of model simulation of carbon stocks in European temperate agricultural soils: allometric function and model initialization, Plant Soil, 450, 255–272, https://doi.org/10.1007/s11104-020-04500-9, 2020.
Tardy, V., Spor, A., Mathieu, O., Lévèque, J., Terrat, S., Plassart, P., Regnier, T., Bardgett, R. D., van der Putten, W. H., Roggero, P. P., Seddaiu, G., Bagella, S., Lemanceau, P., Ranjard, L., and Maron, P.-A.: Shifts in microbial diversity through land use intensity as drivers of carbon mineralization in soil, Soil Biol. Biochem., 90, 204–213, https://doi.org/10.1016/j.soilbio.2015.08.010, 2015.
Tesar, M. B. (Ed.): Physiological Basis of Crop Growth and Development, American Society of Agronomy, Inc., and the Crop Science Society of America, Inc., Madison, Wisconsin, USA, https://doi.org/10.2135/1984.physiologicalbasis, 1984.
Throckmorton, H. M., Bird, J. A., Dane, L., Firestone, M. K., and Horwath, W. R.: The source of microbial C has little impact on soil organic matter stabilisation in forest ecosystems, Ecol. Lett., 15, 1257–1265, 2012.
Thuriès, L., Pansu, M., Feller, C., Herrmann, P., and Rémy, J. C.: Kinetics of added organic matter decomposition in a Mediterranean sandy soil, Soil Biol. Biochem., 33, 997–1010, https://doi.org/10.1016/S0038-0717(01)00003-7, 2001.
Trumbore, S.: Age of soil organic matter and soil respiration: Radiocarbon constraints on belowground C dynamics, Ecol. Appl., 10, 399–411, https://doi.org/10.1890/1051-0761(2000)010[0399:AOSOMA]2.0.CO;2, 2000.
van de Schoot, R., Depaoli, S., King, R., Kramer, B., Märtens, K., Tadesse, M. G., Vannucci, M., Gelman, A., Veen, D., Willemsen, J., and Yau, C.: Bayesian statistics and modelling, Nature Reviews Methods Primers, 1, 1, https://doi.org/10.1038/s43586-020-00001-2, 2021.
van Genuchten, M. T.: A Closed-form Equation for Predicting the Hydraulic Conductivity of Unsaturated Soils, Soil Sci. Soc. Am. J., 44, 892–898, https://doi.org/10.2136/sssaj1980.03615995004400050002x, 1980.
Van Oijen, M., Rougier, J., and Smith, R.: Bayesian calibration of process-based forest models: bridging the gap between models and data, Tree Physiol., 25, 915–927, https://doi.org/10.1093/treephys/25.7.915, 2005.
Vanhala, P., Karhu, K., Tuomi, M., Sonninen, E., Jungner, H., Fritze, H., and Liski, J.: Old soil carbon is more temperature sensitive than the young in an agricultural field, Soil Biol. Biochem., 39, 2967–2970, 2007.
Vanuytrecht, E., Raes, D., and Willems, P.: Global sensitivity analysis of yield output from the water productivity model, Environ. Model. Softw., 51, 323–332, https://doi.org/10.1016/j.envsoft.2013.10.017, 2014.
Vilkienė, M., Ambrazaitienė, D., Karčauskienė, D., and Dabkevičius, Z.: Assessment of soil organic matter mineralization under various management practices, Acta Agr. Scand. B-S. P., 66, 641–646, https://doi.org/10.1080/09064710.2016.1162845, 2016.
Vos, C., Jaconi, A., Jacobs, A., and Don, A.: Hot regions of labile and stable soil organic carbon in Germany – Spatial variability and driving factors, SOIL, 4, 153–167, https://doi.org/10.5194/soil-4-153-2018, 2018.
Vos, C., Don, A., Hobley, E. U., Prietz, R., Heidkamp, A., and Freibauer, A.: Factors controlling the variation in organic carbon stocks in agricultural soils of Germany, Eur. J. Soil Sci., 70, 550–564, https://doi.org/10.1111/ejss.12787, 2019.
Vrugt, J. A.: Markov chain Monte Carlo simulation using the DREAM software package: Theory, concepts, and MATLAB implementation, Environ. Model. Softw., 75, 273–316, https://doi.org/10.1016/j.envsoft.2015.08.013, 2016.
Wang, J., Xiong, Z., and Kuzyakov, Y.: Biochar stability in soil: meta-analysis of decomposition and priming effects, GCB Bioenergy, 8, 512—523, https://doi.org/10.1111/gcbb.12266, 2016.
Wei, H., Guenet, B., Vicca, S., Nunan, N., Asard, H., AbdElgawad, H., Shen, W., and Janssens, I. A.: High clay content accelerates the decomposition of fresh organic matter in artificial soils, Soil Biol. Biochem., 77, 100–108, https://doi.org/10.1016/j.soilbio.2014.06.006, 2014.
Wiesler, F., Hund-Rinke, K., Gäth, S. G., Eckhard, Greef, J. M., Hölzle, L. E., Holz, F. H., Kurt-Jürgen, Rudolf, P., Severin, K., Frede, H.-G., Blum, B., Schenkel, H., Horst, W., Dittert, K., Ebertseder, T., Osterburg, B., Philipp, W., and Pietsch, M.: Anwendung von organischen Düngern und organischen Reststoffen in der Landwirtschaft, Bundesministerium für Landwirtschaft und Ernährung, Berichte über Landwirtschaft, https://doi.org/10.12767/buel.v94i1.124, 2016.
Willmott, C. J. and Matsuura, K.: Advantages of the mean absolute error (MAE) over the root mean square error (RMSE) in assessing average model performance, Climate Res., 30, 79–82, 2005.
Winkhart, F., Mösl, T., Schmid, H., and Hülsbergen, K.-J.: Effects of Organic Maize Cropping Systems on Nitrogen Balances and Nitrous Oxide Emissions, Agriculture, 12, 907, https://doi.org/10.3390/agriculture12070907, 2022.
Wolf, U., Fuß, R., Höppner, F., and Flessa, H.: Contribution of N2O and NH3 to total greenhouse gas emission from fertilization: results from a sandy soil fertilized with nitrate and biogas digestate with and without nitrification inhibitor, Nutr. Cycl. Agroecosys., 100, 121–134, 2014.
Zambrano-Bigiarini, M.: hydroGOF: Goodness-of-fit functions for comparison of simulated and observed hydrological time series (0.5-4), Zenodo [code], https://doi.org/10.5281/zenodo.839854, 2020.
Zhang, Y., Liu, Q., Zhang, W., Wang, X., Mao, R., Tigabu, M., and Ma, X.: Linkage of aggregate formation, aggregate-associated C distribution, and microorganisms in two different-textured ultisols: A short-term incubation experiment, Geoderma, 394, 114979, https://doi.org/10.1016/j.geoderma.2021.114979, 2021.
Zhang, Z.-S., Dong, X.-J., Xu, B.-X., Chen, Y.-L., Zhao, Y., Gao, Y.-H., Hu, Y.-G., and Huang, L.: Soil respiration sensitivities to water and temperature in a revegetated desert, J. Geophys. Res.-Biogeo., 120, 773-787, https://doi.org/10.1002/2014JG002805, 2015.
Zhao, F., Wu, Y., Hui, J., Sivakumar, B., Meng, X., and Liu, S.: Projected soil organic carbon loss in response to climate warming and soil water content in a loess watershed, Carbon Balance and Management, 16, 24, https://doi.org/10.1186/s13021-021-00187-2, 2021.
- Abstract
- Introduction
- Materials and methods
- Results
- Discussion
- Conclusions
- Appendix A: General properties of each monitoring and experimental site
- Appendix B: Revised environmental decomposition factors
- Appendix C: Morris elementary effects screening method
- Appendix D: Metropolis–Hastings algorithm
- Appendix E: Model evaluation methods
- Appendix F: Results of the Morris elementary effects screening method
- Appendix G: Gelman and Rubin shrink factor
- Appendix H: Correlations between C turnover parameters
- Appendix I: Model performance
- Appendix J: Model performances
- Code and data availability
- Author contributions
- Competing interests
- Disclaimer
- Acknowledgements
- Review statement
- References
- Abstract
- Introduction
- Materials and methods
- Results
- Discussion
- Conclusions
- Appendix A: General properties of each monitoring and experimental site
- Appendix B: Revised environmental decomposition factors
- Appendix C: Morris elementary effects screening method
- Appendix D: Metropolis–Hastings algorithm
- Appendix E: Model evaluation methods
- Appendix F: Results of the Morris elementary effects screening method
- Appendix G: Gelman and Rubin shrink factor
- Appendix H: Correlations between C turnover parameters
- Appendix I: Model performance
- Appendix J: Model performances
- Code and data availability
- Author contributions
- Competing interests
- Disclaimer
- Acknowledgements
- Review statement
- References