the Creative Commons Attribution 4.0 License.
the Creative Commons Attribution 4.0 License.
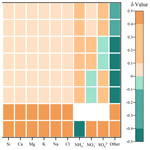
The effect of emission source chemical profiles on simulated PM2.5 components: sensitivity analysis with the Community Multiscale Air Quality (CMAQ) modeling system version 5.0.2
Zhongwei Luo
Yan Han
Kun Hua
Yufen Zhang
Jianhui Wu
Xiaohui Bi
Baoshuang Liu
Yang Chen
Xin Long
A chemical transport model (CTM) is an essential tool for air quality prediction and management, widely used in air pollution control and health risk assessment. However, the current models do not perform very well in reproducing the observations of some major chemical components, for example, sulfate, nitrate, ammonium and organic carbon. Studies have suggested that the uncertainties in the model chemical mechanism, source emission inventory and meteorological field can cause inaccurate simulation results. Still, the emission source profile (used to create speciated emission inventories for CTMs) of PM2.5 has not been fully taken into account in current numerical simulation. Based on the characteristics and variation rules of chemical components in typical PM2.5 sources, different simulation scenarios were designed and the sensitivity of simulated PM2.5 components to the source chemical profile was explored. Our findings showed that the influence of source profile changes on simulated PM2.5 components' concentrations cannot be ignored. Simulation results of some components were sensitive to the adopted source profile in CTMs. Moreover, there was a linkage effect: the variation in some components in the source profile would bring changes to the simulated results of other components. These influences are connected to chemical mechanisms of the model since the variation in species allocations in emission sources can affect the potential composition and phase state of aerosols, chemical reaction priority, and multicomponent chemical balance in thermodynamic equilibrium systems. We also found that the perturbation of the PM2.5 source profile caused variation in simulated gaseous pollutants, which indirectly indicated that the perturbation of source profile would affect the simulation of secondary PM2.5 components. Our paper highlights the necessity of paying enough attention to the representativeness and timeliness of the source profile when using CTMs for simulation.
- Article
(2918 KB) - Full-text XML
-
Supplement
(1835 KB) - BibTeX
- EndNote
Ambient fine particulate matter (PM2.5) pollution in some key regions of China has attracted much attention (Liang et al., 2020; Huang et al., 2021). The chemical components of PM2.5, including elements (Al, Si, Fe, Mn, Ti, Cu, Zn, Pb, etc.), water-soluble ions (SO, NO, Cl−, F−, NH, Na+, K+, Mg2+, Ca2+, etc.) and carbon-containing components (organic carbon, OC; elemental carbon, EC) (Yang et al., 2011; Li et al., 2013), have different physical and chemical properties, such as reactivity, thermal stability, particle size distribution, residence time, optical properties or health hazards (Seinfeld and Pandis, 2006; Tang et al., 2006). According to long-term monitoring results, in most regions of China, SO, NO, NH and OC are the most important species in ambient PM2.5 (Li et al., 2017a, 2021), which has a certain adverse impact on human health (Shi et al., 2018) and ecosystems, such as acid rain in southwest China (Han et al., 2019) or food security (Zhou et al., 2018).
Chemical transport models (CTMs) play an important role in policymaking for regulatory purposes. Based on the scientific understanding of atmospheric physical and chemical processes, CTMs are built to simulate the transport, reaction and removal of pollutants on a certain scale in horizontal and vertical directions. With the development of CTMs, the simulation accuracy of PM2.5 concentration has been significantly improved. Higher requirements have been put forward for the precise simulation of PM2.5 components so as to provide support for the use of CTMs in human health risk assessment, climate effects, pollution sources apportionment and so on (Peterson et al., 2020; Lv et al., 2021). However, the current models do not perform very well in simulating some components (for example, PM2.5-bound sulfate, nitrate, ammonium, trace elements) (Zheng et al., 2015; Fu et al., 2016; Ying et al., 2018; Cao et al., 2021). In the current literature, the correlation coefficient (R) and normalized mean bias (NMB) are highly variable and inconsistent between the simulated and the observed values (listed in Table S1 in the Supplement). This is mainly attributable to the uncertainties in the model chemical mechanism, source emission inventory and meteorological field simulation.
The chemical mechanisms involved in CTMs are derived from parameterized assumptions based on laboratory simulation and field observations. The actual atmospheric chemical processes are very complex, and some reaction mechanisms are still limitedly understood. In addition, the integration of chemical reactions and simplified treatment methods in the model cannot fully reflect the correlation among atmospheric pollutants. For example, in some model mechanisms, important sulfate and nitrate formation pathways through new heterogeneous chemistry were added, including the chemical reaction between SO2 and aerosol, and aerosol (Zheng et al., 2015); nitrous acid oxidizing SO2 to produce sulfate (Zheng et al., 2020); dust particles promoting the oxidation of SO2 (Yu et al., 2020); modifying the uptake coefficients for heterogeneous oxidation of SO2 to sulfate (Zhang et al., 2019); and updating the heterogeneous N2O5 parameterization (Foley et al., 2010). Even though the aforementioned processes can significantly improve the simulation of SO and NO, there is still a gap between the modeled and the actual atmospheric chemical processes.
The uncertainty in meteorological field simulation is another crucial reason for the simulation deviation; especially on heavy-pollution days, the variation trends of PM2.5 chemical components were not well-captured (Ying et al., 2018; Qi et al., 2019; Wang et al., 2022). Precipitation is the key meteorological factor determining wet removal of pollutants; boundary layer height and wind speed are the main factors affecting convection and transport of pollutants; solar radiation, temperature and relative humidity are the key factors affecting the formation of secondary particles (Huang et al., 2019; Chen et al., 2020). Some literature has reported that deviation from precipitation and wind field simulation might lead to underestimation of SO, NO and NH (Cheng et al., 2015; Zhang et al., 2017). Devaluation of the liquid water path and cloud cover causes a decrease in sulfate formation in cloud and ultimately results in significantly underestimated components in simulation values (Sha et al., 2019; Foley et al., 2010). Underestimation of temperature and relative humidity may also cause adverse effects of the temperature-dependent and/or relative-humidity-dependent chemical reaction in the simulation (Sha et al., 2019).
The uncertainty in the source emission inventory also significantly affects the simulation results of PM2.5 components (Shi et al., 2017; Sha et al., 2019). Due to incomplete information or insufficient representativeness, pollutant emissions are sometimes overestimated or underestimated, and the method for temporal and spatial allocation also needs to be improved.
In particular, the emission source profile of PM2.5 (hereinafter referred to as the “source profile”), used to create speciated emission inventories for CTMs (Hsu et al., 2019), has not been fully taken into account in the current numerical simulation. In the reported literature, PM2.5 species allocation coefficients of emission sources are commonly treated in the following ways: (1) allocating PM2.5 components of source emissions by referring to source profile data in published literature or databases like US SPECIATE (Fu et al., 2013; Wang et al., 2014; Ying et al., 2018) and (2) determining chemical profiles from local measurement (Fu et al., 2013; Appel et al., 2013). However, with the development of production technology and the innovation of pollution treatment technology in recent years, some source profiles have changed dramatically (Bi et al., 2019a), such as SO from coal burning: its content in PM2.5 is generally low in coal-fired power plants without desulfurizing facilities, while existing coal-fired power plants use limestone–gypsum wet desulfurization, and the contents of SO in PM2.5 are significantly higher than those without desulfurization facilities (Zhang et al., 2020). The timeliness of PM2.5 species allocation coefficients in current CTMs also needs to be considered.
This paper attempts to answer the following questions: (1) whether the variation in the source profile adopted in the model has an impact on the simulated results of PM2.5 chemical components. (2) How large is that impact? (3) How does the impact work? Aiming to address these problems above, chemical composition and its variation law for typical PM2.5 emission sources are summarized; on this basis, sensitivity tests are designed to identify whether PM2.5 source profiles and species allocation in the model are important parameters that affect the simulation results of chemical components' concentrations in PM2.5. We take the Community Multiscale Air Quality model (CMAQ, one of the most widely used CTMs) and the Multi-resolution Emission Inventory for China (MEIC, a high-resolution inventory of anthropogenic air pollutants in China) as the carriers. The same kind of experiment is also applicable to other CTMs and emission inventories. The aim of this study is to provide support for the effective utilization of source profiles in the CTMs and improvement of the simulation schemes.
2.1 Model configuration
The Weather Research and Forecasting model (WRF 3.7.1), the widely used Community Multiscale Air Quality model (CMAQv5.0.2) (Eder and Yu, 2006; Yu et al., 2014), and the Multi-resolution Emission Inventory for China (MEICv1.3) have been used in this study. MEIC, developed by Tsinghua University, mainly tracked anthropogenic emissions in China including coal-fired power plant, industry, vehicle, residential and agricultural sources (http://meicmodel.org/?page_id=135, last access: 14 November 2023) (Li et al., 2017b; Zheng et al., 2018). The WRF model was used to generate meteorological inputs for the CMAQ model. Three nested modeling domains consisting of 36 km × 36 km (Dom1), 12 km × 12 km (Dom2) and 4 km × 4 km (Dom3) horizontal grid sizes were set, as shown in Fig. 1. The initial and boundary conditions for WRF were based on the North American Regional Reanalysis data archived at National Center for Atmospheric Research (NCAR). In addition, surface and upper-air observations obtained from NCAR were used to further refine the analysis data. The modeling was conducted from 1 to 30 October 2018, and the major configurations we used in CMAQ were as follows: gas-phase chemistry was based on the CB05 mechanism, and the aerosol dynamics and chemistry were based on the AERO6 module (cb05tucl_ae6_aq). The detailed model configurations are shown in Table S2, and the regional distribution of PM2.5 emission sources is shown in Fig. S1.
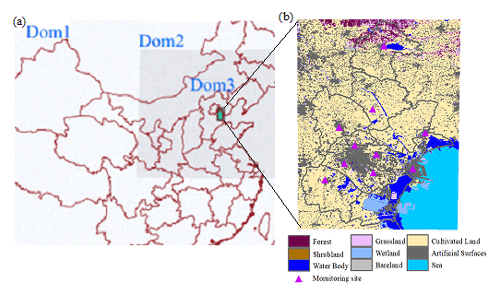
Figure 1Modeling domains of the CMAQ model. (a) The three-domain nested CMAQ domains. (b) Land use and observation sites of Dom3 (data source of land use: GlobeLand30, https://www.webmap.cn/mapDataAction.do?method=globalLandCover, last access: 14 November 2023, National Geomatics Center of China).
2.2 Selection and comparison of the PM2.5 emission source profile
The PM2.5 emission source profiles from the database of Source Profiles of Air Pollution (SPAP) (http://www.nkspap.com:9091/, last access: 14 November 2023) and the US Environmental Protection Agency (EPA) SPECIATE database (https://www.epa.gov/air-emissions-modeling/speciate, last access: 14 November 2023) as well as from the published literature were selected. SPAP was developed by the State Environment Protection Key Laboratory of Urban Particulate Air Pollution Prevention, Nankai University, China. This database contains more than 3000 size-resolved source profiles of stationary combustion sources, industrial processes, vehicle exhaust, biomass burning, dust and other sources, collected from more than 40 cities in China since 2001. In addition to inorganic elements, water-soluble ions, OC, EC and other conventional components, some source profiles also encompass tracer information, such as organic markers, isotopes, single-particle mass spectrometry, volatile organic compounds (VOCs) and other gaseous precursors. Based on species in the aerosol chemical mechanism (AERO6) of CMAQ (Appel et al., 2013; Chapel Hill, 2012), we selected 15 components in PM2.5 source profiles including Al, Ca, Cl, EC, Fe, K, Mg, Mn, Na, OC, Si, Ti, NH, NO and SO; the remaining components are classified as “other”. In the database of Source Profiles of Air Pollution (SPAP) and the US EPA SPECIATE database, the four source categories (coal-fired power plant, industry process, transportation sector and residential coal combustion) contain a series of sub-categories. But MEIC does not include the corresponding sub-categories. So we take the average values of source profiles in each source category as representing the source profile; the details can also be seen in our previous work (Bi et al., 2019a). Then we multiply inventory emissions by profile fraction to get emissions of specific chemical components.
To determine the similarity between the two groups of source profiles. The coefficient divergence (CD) is calculated using the following formula (Wongphatarakul et al., 1998):
where CDjk is the coefficient of divergence of the source profile j and k, p is the number of chemical components in the source profile, xij is the weight percentage for the chemical component i in source profile j, and xik is the weight percentage for i in source profile k (%). The CD value is in the range of 0 to 1; if the two source profiles are similar, the value of CD is close to 0, and if the two are very different, the value is close to 1.
2.2.1 Coal-fired power plant (PP)
Coal-fired power plants remain the main coal consumers in China and accounted for 50.2 % of total coal consumption in 2019 (NBS, 2021). They have gained much attention, especially with the wide implementation of the ultralow emission standards by which PM2.5 emission characteristics have changed accordingly (Wu et al., 2020, 2022). There are obvious differences in PM2.5 source profiles between SPAPPC (SPAP database and published source profiles in China) and SPECIATE (US EPA SPECIATE database); the CD value of these two groups lies between 0.34 and 0.92 (0.64 ± 0.10). Detailed information is shown in Table S3 and Fig. S2 in the Supplement. The percentages of species in PP source profiles are plotted in Fig. 2a. The main components in SPAPPC are sorted by Si, SO, OC and Ca with average values of 8.7 ± 6.8 %, 8.5 ± 11.5 %, 6.8 ± 9.1 % and 6.5 ± 6.9 %, respectively. The SPECIATE data are enriched in SO (16.9 % ± 20.0 %), OC (12.7 ± 21.8 %), Si (9.6 ± 5.0 %) and Ca (9.3 ± 7.3 %), which are higher than SPAPPC. Coal properties, burning conditions, pollution control measures and emission sampling methods are the main reasons for those great percentage fluctuations. Different treatment processes of flue gases, e.g., wet/dry limestone, ammonia and double-alkali flue gas desulfurization, will affect the percentages of components in source profiles (Zhang et al., 2020). It has been reported that the percentage of Ca, Mg, SO and Cl− in PP profiles increased after the limestone–gypsum method was used in coal-fired power plants (Bi et al., 2019a). Besides that, the percentage of Cl− in SPAPPC is obviously higher than that in SPECIATE, which might be attributed to the generally higher Cl− content in raw coal in China (Guo et al., 2004).
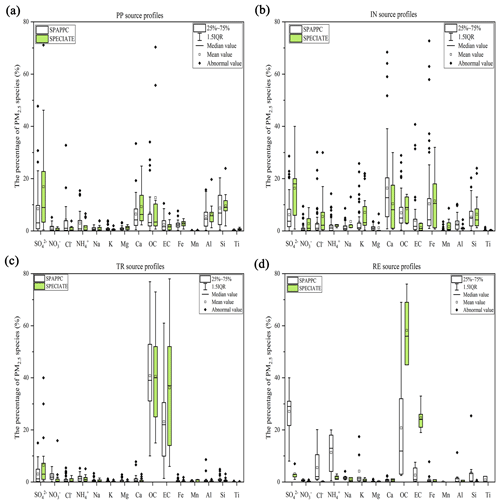
Figure 2Chemical profiles for PM2.5 emitted from (a) coal-fired power plants (PP), (b) industry processes (IN), (c) the transportation sector (TR) and (d) residential coal combustion (RE). Data obtained from SPAPPC (SPAP database and published source profiles in China) and SPECIATE (US EPA SPECIATE database).
2.2.2 Industrial process (IN)
Industrial emissions are one of the major sources of PM2.5 (Hopke et al., 2020); the percentages of Ca, Fe, OC and SO are relatively high in both SPAPPC and SPECIATE, but the shares in different source profile databases vary. Their CD values vary from 0.45 to 0.94 (0.72 ± 0.09) (detailed information is shown in Tables S4–S7 and Fig. S3). In SPAPPC, these four components account for 16.4 ± 14.9 %, 10.4 ± 14.4 %, 6.9 ± 6.1 % and 6.2 ± 6.4 %; the proportions in SPECIATE are 10.4 ± 9.8 %, 11.4 ± 10.6 %, 8.5 ± 4.9 % and 16.3 ± 13.3 %, respectively (Fig. 2b). Large variations in components and their percentages in industrial processes are attributed to the manufacturing processes, raw material, pollution control measures and so on (Ji et al., 2017; Bi et al., 2019a; Gao et al., 2022). For example, Ca, Al, OC and SO are found to have the highest percentages in cement sources (Guo et al., 2021); Fe, Si and SO are the most abundant species in steel industry emissions (Guo et al., 2017).
2.2.3 Transportation sector (TR)
Traffic contributed a large fraction of PM2.5 in many locations (Hopke et al., 2022). It is well-known that the transportation sector makes a dominant contribution of OC and EC. The main components of PM2.5 emitted from traffic sources are OC, EC and SO in both SPAPPC and SPECIATE, but they still vary in a wide range: their CD values fall between 0.33 and 0.86 (0.69 ± 0.09) (detailed information is given in Tables S8–S10 and Fig. S4). In SPAPPC, the percentages of OC, EC and SO are 40.8 ± 15.0 %, 23.1 ± 13.8 % and 3.1 ± 3.7 %, and in SPECIATE, the percentages are 40.6 ± 16.4 %, 36.1 ± 21.5 % and 6.4 ± 9.9 %, respectively (Fig. 2c). These significant differences can mainly be attributed to the vehicle type, fuel quality, mixing ratio between oil and gas, and combustion phase in vehicle engine (Xia et al., 2017).
2.2.4 Residential coal combustion (RE)
Residential coal combustion, as the leading source of global PM2.5 emissions (Weagle et al., 2018), has a much higher emission factor than coal-fired power plants (Wu et al., 2022). The fraction of components varies greatly in the profiles measured from SPAPPC and SPECIATE; their CD values are 0.75 ± 0.10 (detailed information is given in Table S11 and Fig. S5). SO, OC, NH and EC make the main contribution to PM2.5 emitted from residential coal combustion. In SPAPPC, the average percentages of SO, OC, NH and EC are 27.1 ± 10.1 %, 20.7 ± 20.6 %, 11.3 ± 7.7 % and 2.6 ± 2.8 %, respectively. In SPECIATE, the average percentages are 58.2 ± 14.0 % for OC, 24.6 ± 5.4 % for EC, 3.2 ± 2.3 % for SO and 1.6 ± 1.0 % for NH (Fig. 2d). Total percentages of OC and EC in SPECIATE are over 80 %, obviously higher than that in SPAPPC, while higher percentages of SO, Cl−, K and Si are observed in SPAPPC. The coal type and properties and burning conditions are the main factors affecting the percentages of PM2.5 components; for example, the chunk coal burning has relatively high percentages of OC, EC, SO, NO and NH compared to honeycomb briquettes (Wu et al., 2021; Song et al., 2021).
Briefly, many factors can affect PM2.5 source profiles, and with the innovation of manufacturing techniques and pollution control technology and changes in fuel and raw and auxiliary materials, the main chemical components and their percentages can change dramatically. To explore whether the variations in the source profile adopted in the CMAQ model are one of the important factors affecting the simulated PM2.5 component, we designed a series of simulation tests to address the following issues.
In this part, we separately selected source profiles from the SPAPPC and SPECIATE databases and applied them to an emission inventory for simulating PM2.5 and its components with other modeling conditions unchanged, corresponding to cases CMAQ_SPA and CMAQ_SPE. The detailed information about source profiles is shown in Fig. S6.
By comparing the selected SPAPPC source profiles with the selected SPECIATE source profiles, the coefficient divergences for the four main source categories were CDPP(0.67) > CDRE(0.62) > CDTR(0.60) > CDIN(0.60), which meant the selected source profiles in the two simulation cases were quite different. The average simulated concentration of PM2.5 and its components at each ambient air quality monitoring station (Table S12) was extracted from CMAQ outputs. We selected one air quality monitoring station (Site 8 as the selected station here but any site could be available) to explore the effect of emission source chemical profiles on simulated PM2.5 components and then used the nine sites left to further illustrate the conclusions suggested.
The simulation results for PM2.5 species under the CMAQ_SPA and CMAQ_SPE scenarios also showed big differences (as shown in Fig. 3 and Table S13). The largest difference in average simulated concentration was for EC, with CMAQ_SPE higher than CMAQ_SPA by 167 %. For OC and Mn, higher values were also given by CMAQ_SPE than by CMAQ_SPA (45 % and 126 % on average, respectively). For the other components of concern, the simulated concentration by CMAQ_SPE was lower than CMAQ_SPA with Ti (58 %), Na (55 %), Mg (53 %), Ca (51 %), Al (33 %), Cl (31 %), K (29 %), Si (22 %), Fe (16 %), NH (3 %), SO (9 %) and NO (8 %). The simulated PM2.5 concentrations for the two cases were quite close. The influence of source profile variation on the simulated PM2.5 concentration was not significant, but the influence on the simulation of chemical components in PM2.5 could not be ignored.
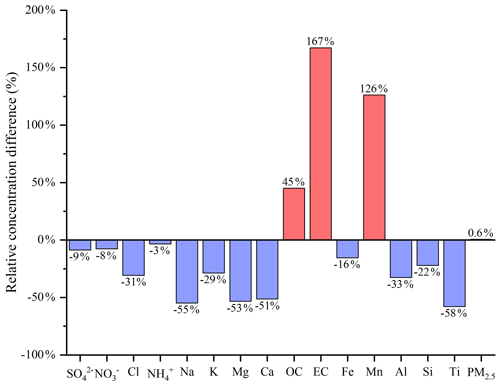
Figure 3The relative concentration difference in average simulated results (PM2.5 and its components) between CMAQ_SPE and CMAQ_SPA (relative to CMAQ_SPA) during the simulation period; PM2.5 source profiles from the SPAPPC and SPECIATE databases were used to create speciated emission inventories for CMAQ, corresponding to cases CMAQ_SPA and CMAQ_SPE, respectively.
To quantitatively characterize how much the source profiles affect the simulation results, we selected the chemical composition of code 000002.5 (variety of different categories used for the overall average composite profiles; Hsu et al., 2019) in the US EPA SPECIATE_5.0_0 database for species allocation of PM2.5 components. The corresponding percentages of EC, OC, Mn, Fe, Ti, Al, Si, Ca, Mg, K, Na, Cl, NH, NO and SO in PM2.5 are shown in Fig. 4 (SGL, base case simulation).
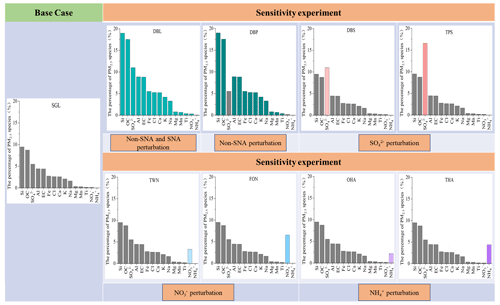
Figure 4The general roadmap of sensitivity tests (the histogram in each case is the speciation profile in CTMs; SNA represents SO, NO and NH; non-SNA represents other components in PM2.5).
Table 1The content of sensitivity experiment cases.
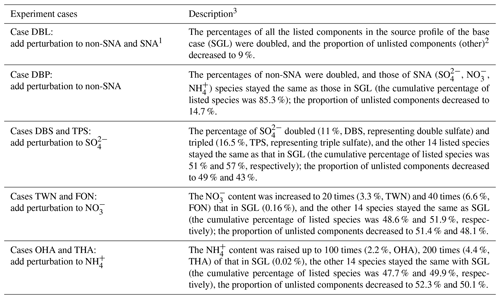
1 SNA represents SO, NO and NH; non-SNA represents the other components in PM2.5. 2 The listed components contained Al, Ca, Cl, EC, Fe, K, Mg, Mn, Na, OC, Si, Ti, NH, NO and SO; unlisted components were classified as other. 3 The source profiles in all cases listed in the table were calculated based on the base case SGL. In the design of simulation cases, the reason why the disturbance amplitude of NH and NO was significantly higher than that of other components such as SO and non-SNA was that the percentages of NH and NO in the base source profile (SGL, based on the chemical composition of code 000002.5 in the EPA SPECIATE_5.0_0 database) were very low, while the percentages of NH and NO in SPAPPC presented in Sect. 2.2 were orders of magnitude higher than those in SGL.
Given the large quantity and complex chemical composition of PM2.5, it was advisable to classify the chemical composition reasonably before designing sensitivity experiments. The DBL case consisted of doubling the percentage of the 15 components mentioned in the above base case (SGL) (details are shown in Fig. 4 and Table 1). As the percentage of these components increased, the proportion of unlisted components (represented by other) decreased to 9 % in order to meet the requirement that the total percentage of all components is 100 %. Then we compared the simulation results before (SGL case) and after (DBL case) perturbation in the species allocation of PM2.5 sources.
In the case of DBL, when the percentages of all the components except those in the other category were doubled in the source profile, the simulated concentrations of Al, Ca, Cl, EC, Fe, K, Mg, Mn, Na, OC, Si and Ti doubled as well, while the simulated concentration of NO3 and SO increased at about 3 % and 10 % and NH decreased by 4 %, respectively, although the simulated concentration of PM2.5 was not obviously changed (detailed simulation results are shown in Table S14). The simulation test results for SNA (SO, NO and NH) and non-SNA were obviously different. Therefore, we divided the components in the source profile into two groups (non-SNA and SNA) and designed a series of sensitivity tests listed in the next section to further explore how species allocation of PM2.5 in emission sources affects the simulation results. A sketch of the sensitivity experiment design idea is shown in Fig. S7.
4.1 Sensitivity tests design
Sensitivity tests were designed by changing the percentages of the target components and related components in the base case (SGL): add the perturbation to each component of non-SNA, to SO, to NO and to NH. A general roadmap of sensitivity tests is shown in Fig. 4, and an illustration of each case is summarized in Table 1. Basic rules must be followed: (a) the perturbation in the percentage of each component in the source profile falls within the variation range of its measured value described in Sect. 2.2. (b) The sum of the percentage of listed non-SNA, SNA and other components in the PM2.5 source profile is 100 %.
4.2 Sensitivity of simulated components to changes in the source profile
We propose the sensitivity coefficient (δ) as an evaluation index. The calculation formula is as follows:
wherein δi,p is the sensitivity coefficient of component i relative to component p, representing the change in the simulated value of its content in ambient PM2.5 corresponding to 1 % perturbation in the source profiles. Ci_case is the simulated concentration of component i in each sensitivity experiment case (µg m−3), Ci_base is the simulated concentration of components i in the base case (µg m−3), is the simulated concentration of PM2.5 in each sensitivity experiment case (µg m−3), is the simulated concentration of PM2.5 in the base case (µg m−3), Pp_case is the percentage of component p in the source profile of the sensitivity experiment case (%), j is the perturbed component j in different source profiles of sensitivity experiment cases and Pp_base is the percentage of component p in the source profile of the base case (%).
A positive value of δ means the simulated concentration of the PM2.5 component increases (decreases) with the increase (decrease) in perturbation in the percentage of components in the source profile, and negative δ is just the opposite. If the absolute value of δ is less than or equal to 0.1, the simulated component is considered to be insensitive to the corresponding variation in the source profile; if the absolute value of δ falls between 0.1 and 0.4 (included), the simulated component is considered to be sensitive to the variation in the source profile; if the absolute value of δ is larger than 0.4, the simulated component is very sensitive to the variation in the source profile. The greater the absolute value of δ, the more obvious the impact of the variation in the source profile adopted in CMAQ on the simulated results of PM2.5 chemical components.
Figure 5 lists the sensitivity coefficients of simulated ambient PM2.5 components according to the perturbation of the source profile under each test case. In the DBL case (double the percentage of the listed components in the source profile of the base case and decrease the proportion of other unlisted components to 9 %), the sensitivity coefficient (δ) of NH was negative and the absolute value was high, indicating that the simulated proportion of NH in ambient PM2.5 decreased and it was very sensitive to the variation in the source profile. Conversely, the sensitivity coefficient of NO was close to 1, which illustrated that the simulated proportion of NO in ambient PM2.5 increased proportionally with the change in source profile. The simulated SO also showed a very sensitive property. The simulated non-SNA concentrations were doubled when compared to the base case (SGL).
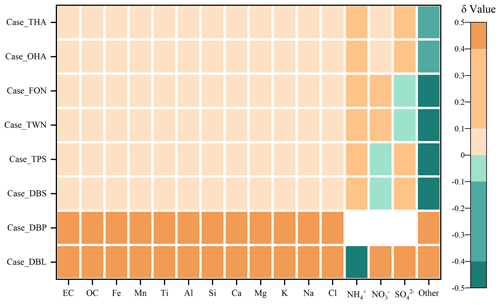
Figure 5The sensitivity coefficients (δ) of simulated components according to the perturbation of the adopted source profile in different cases. Note: each small color box in the figure represents the sensitivity level (indicated by the legend on the right) of PM2.5 components (x coordinate) in different cases (y coordinate). The blank grids in the DBP case indicate no perturbation to SNA in the PM2.5 source profile.
In case DBP, when the percentages of listed non-SNA (Al, Ca, Cl, EC, Fe, K, Mg, Mn, Na, OC, Si and Ti) in the source profile were doubled, the simulated proportions of non-SNA in ambient PM2.5 synchronous increased and were very sensitive to the change in the adopted source profile, with a sensitivity coefficient (δ) of 0.5. Interestingly, the simulated concentration of SNA in ambient PM2.5 also changed although SNA in the source profile did not change; the concentration of NO and SO increased by 2 % and 3 %, respectively, and NH decreased by 10 % (detailed simulation results of each case are shown in Tables S15–S21).
Under SO perturbation cases (Case DBS and Case TPS), we found the simulated results of non-SNA and NO had no obvious variation compared with the base case. In both Case DBS and Case TPS, the δ values of non-SNA and NO were between −0.1 and 0.1. But when the percentage of SO was doubled in source profile (DBS), the simulated concentration of NH and SO increased by 6 % and 8 %, respectively. In Case TPS (the percentage of SO was tripled), the simulated concentrations of NH and SO were increased by 11 % and 16 %, respectively. The δ values of NH and SO were 0.12 and 0.36, sensitive toward the positive direction with the increase in SO in the source profile.
In the situation of NO perturbation in the source profile (Case TWN and Case FON), the simulated non-SNA hardly changes when compared to the base case, while changing patterns of simulated SNA were different. The simulation concentration of NH increased by 2.6 % and 5.4 % compared with the base case, the simulated NO increased by 14 % and 30 %, and the simulated SO decreased slightly and even could be neglected in some observation sites. The simulated concentrations of non-SNA and SO were insensitive to the perturbation of NO in the source profile; NH was sensitive, and NO was very sensitive.
When we add perturbation to NH in the source profile (Case OHA and Case THA), the simulation results of non-SNA were almost not changed, whereas the simulated concentration of SO, NH and NO increased. The δ values of SNA in response to the variation in NH in the source profile were positive, and > > ; SO and NH were sensitive to the NH perturbation in the source profile, but NO was not so sensitive.
In general, the simulation results of components in ambient PM2.5 were affected in one way or another by the change in source profiles adopted by CMAQ. Both of the simulated non-SNA and the simulated SNA were very sensitive to the perturbation of non-SNA in the source profile. When the percentage of SNA changed in the source profile, simulated non-SNA generally changed little, but the simulation results of SNA could change in different patterns: the simulated SO was very sensitive and NH was sensitive to the perturbation of SO in the source profile; simulated NO was very sensitive and NH was sensitive to the perturbation of NO in the source profile; SO and NH were sensitive to the perturbation of NH in the source profile. The simulated component such as SO was influenced not only by the change in SO itself but also by other components like some non-SNA and NH in the source profile. In other words, there was a linkage effect, variation in some components in the source profile would bring changes to the simulated results of other components.
The variation in species allocation in emission sources can directly affect the composition of aerosol systems in CTMs. In CMAQv5.0.2, the aerosol thermodynamic equilibrium process is carried out according to ISORROPIA II, including a SO–NO–Cl−–NH–Na+–K+–Mg2+–Ca2+–H2O system (detailed equilibrium relations are shown in Table S22). Some assumptions have been made in the ISORROPIA model to simplify the simulation system (Fountoukis and Nenes, 2007): (1) because the vapor pressure of sulfuric acid and metal salts (such as Na+, Ca2+, K+, Mg2+) is very low, it is assumed that all the sulfuric acid and metal salts in the system existed in the aerosol phase; (2) for ammonia in the system, it is preferred to have an irreversible reaction with sulfuric acid to produce ammonium sulfate. Only when there is still surplus NH3 after the neutralization of H2SO4 can it have a reversible reaction with HNO3 and HCl to produce NH4NO3 and NH4Cl. (3) For sulfuric acid in the system, if there are metal ions (such as Ca2+, Mg2+, K+, Na+) in the system, sulfuric acid would react with metal ions to produce metal salts. Only in the case of insufficient sodium would sulfuric acid react with ammonia. Based on these assumptions, the ISORROPIA model introduces the following three judgment parameters (R1, R2 and R3) to determine the simulation subsystems; these parameters are calculated by the following formulas:
where [X] denotes the molar concentration of the component (mol m−3) and R1, R2 and R3 are termed as the “total sulfate ratio”, “crustal species and sodium ratio” and “crustal species ratio”, respectively. The numbers of species and equilibrium reactions are determined by the relative abundance of NH3, Na, Ca, K, Mg, HNO3, HCl and H2SO4, as well as the ambient relative humidity and temperature. Guided by the values of R1, R2 and R3, five aerosol composition regimes in ISORROPIA are defined. (Detailed rules are shown in Table S27 and the solving procedure in Fig. S8.) R1, R2 and R3 in each sensitivity test case are shown in Fig. 6. These components achieved thermodynamic equilibrium in the order of preference for more stable salts; obviously, the simulation processes of these components may influence each other.
5.1 General results
Our sensitivity experiment focuses on examining the impact of source profile changes on simulated PM2.5 components. For given meteorological conditions, we analyze the sensitivity of simulated components to variations in the source chemical profile by comparing the simulation results between perturbed cases and the base case.
In the non-SNA perturbation case, when the percentage of non-SNA in the source profile was doubled (Case DBP), there were more Na, K, Mg, Ca and Cl participating in the aerosol chemistry; the model system needed more SO and NO on the basis of charge balance; and the thermodynamic equilibrium shifted to the direction of consuming Ca, Mg, K and Na, which resulted in the increase in the simulated concentration of SO and NO. Meanwhile, according to the rule of anions preferentially binding with nonvolatile cations in ISORROPIA, the increased cations of Na+, K+, Mg2+ and Ca2+ directly led to the decrease in anions binding with NH; there was less reaction dose between SO and NH to form (NH4)2SO4 or NH4HSO4, ultimately resulting in a decrease in the simulated concentration of NH compared with the base case. Because in this case more anions such as SO were passively needed, according to the principle of chemical equilibrium mentioned above, the chemical conversion of SO2 to SO was promoted and the simulated secondary SO increased; this could be proved by the sensitivity coefficient δ of SO2 in Case DBP being negative (shown in Fig. 7; details of other monitoring stations' results are shown in Table S25).
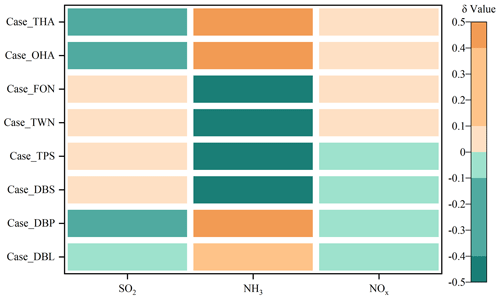
Figure 7The sensitivity coefficients (δ) of simulated gas pollutants according to the change in the adopted source profile in different cases.
Similarly, with the increase in metal ions in the system to bond with anions, the number of anions which can bind to NH decreased. The system needed less NH, and the need for conversion from NH3 to NH was weakened; the simulated NH concentration decreased, while the δ of NH3 was positive and very sensitive. Different trends of the simulated concentration of gaseous pollutants mirrored the rules mentioned above in terms of another aspect. The δ of SO2 and NOx was negative and that of NH3 was positive. We could see the same phenomena in DBL case (Fig. 7). When the percentages of non-SNA in the source profile increased, they affected not only the simulated concentration of non-SNA, but also the secondary SO, NO and NH.
In SO perturbation cases (Case DBS and Case TPS), as the percentage of SO in the source profile increased, for the chemical reactions of sulfate radical consumption (as shown in Table S22), the chemical equilibrium would move toward the products compared with the base case. In contrast, for the chemical reactions of sulfate radical formation (the equations are shown in Table S23), the product was added in and the chemical equilibrium was pushed toward the reactants. The chemical reactions between SO and NH would shift to the direction of (NH4)2SO4 or NH4HSO4 generation, and we could see the simulated concentrations of NH in DBS and TPS were both higher and in NH3 were lower than those in the base case (SGL). In addition, when more SO was added to the system, the conversion of SO2 to SO was affected at some level, less SO2 was consumed than in the base case and simulated SO2 showed an insensitive but positive trend (Fig. 7). The potential solid-phase species in ISORROPIA II under DBS and TPS cases (shown in Table S27) mainly consisted of sulfate salts, so the simulated concentration of NO did not change apparently.
As the percentage of NO in the source profile increased (Case FON and Case TWN), the associated chemical equilibrium shifted toward the consumption of NO, such as NH NO → NH4NO3, which would also result in the consumption of more NH and formation of more ammonium salt and finally the consumption of more NH3 because of NH3(gas) + H2O(aq) → NH(aq) + OH−(aq). The simulation results also showed that the concentration of NH increased while that of NH3 decreased. Based on the assumption of ISORROPIA, the cations like Na+, K+, Mg2+, Ca2+ and NH preferentially react with SO; only if there are cations left after neutralized SO can they react with NO to form salts, so the simulated concentration of SO was not obviously changed. Accordingly, the simulated concentration of NOx and SO2 was almost unchanged (the δ of NOx and SO2 was insensitive).
In the cases of NH perturbation (Case OHA and Case THA), when the percentage of NH in the source profile increased, the related chemical equilibrium shifted toward the direction of NH consumption, such as in 2NH + SO → (NH4)2SO4 or NH + H+, SO → NH4HSO4; more SO was consumed at the same time, which further promoted the conversion of SO2 to SO. The increased NH in OHA and THA would also inhibit the conversion of NH3 to NH compared with the base case. This, in turn, appeared as an increase in the simulated secondary SO and NH3 and a decrease in the simulated SO2.
5.2 Results from stratified analysis
For each case, the distribution of R values was related to meteorological conditions (as shown in Fig. 6). To illustrate the role of meteorological conditions in the mechanism of how the source profile affected the simulated PM2.5 components, stratified analysis was used. The hourly simulation result of temperature and humidity (affecting the ISORROPIA solving procedure) and the wind field (affecting flux in and flux out for each grid) were incorporate into k-means clustering. When the number of clusters was equal to or greater than four, there was a significant inflection point between data points and their assigned cluster centroids (Fig. S9). Hence, four patterns of meteorological conditions were selected for the subsequent analysis.
For patterns I, II, III and IV, as shown in Fig. 8, the rule similar to the general result was observed. From a global view, the subdivisional (category-specific) sensitivity of simulated PM2.5 components to the source chemical profile under different patterns is similar; from a local perspective, their sensitivity levels are slightly different. For example, in pattern II, the simulated NH was very sensitive to the perturbation of SO, while in patterns I, III and VI it was sensitive, but it remained the major component that underwent change (these results are also shown in Table S28 of the Supplement).
When we perturb the source profile, some species/reactants increase (or decrease) in the system and the chemical equilibrium shift to the direction of consuming more (or fewer) reactants, as shown in Fig. S10. According to different patterns of meteorological conditions (determining the values of R), the influence pathways of chemical source profile changes on the simulated PM2.5 components has the same laws as the general results.
In summary, the effects of source profile variation on the simulation results of different components were linked. When the percentages of non-SNA, SO, NO and NH in the source profile changed, they not only affected the simulated concentration of themselves, but also affected the simulation results of some other components. The simulation results of both primary components and secondary components were affected by the change in the source profile; the secondary SO and NH were affected more than the secondary NO.
The influence of source profile variation on the simulated PM2.5 components cannot be ignored, as simulation results of some components are sensitive to the adopted source profile in CTMs; e.g., both the simulated non-SNA and the simulated SNA are sensitive to the perturbation of non-SNA in the source profile, the simulated SO and NH are sensitive to the perturbation of SO, the simulated NO and NH are sensitive to the perturbation of NO, and SO and NH are sensitive to the perturbation of NH. These influences not only are specific to an individual component, but also can be transmitted and linked between components. The influence path is connected to chemical mechanisms in the model, since the variation in species allocation in emission sources directly affects the thermodynamic equilibrium system (ISORROPIA II, SO–NO–Cl−–NH–Na+–K+–Mg2+–Ca2+–H2O system).
It is generally believed that changes in the source profile would have an impact on the simulation result of primary PM2.5, but interestingly, the simulation of secondary components could be affected as well. We found the perturbation of the PM2.5 source profile caused the variation in simulation results of gaseous pollutants by influencing related chemical reactions like gas-phase chemistry of SO2, NOx and NH3. Overall, the emission source profile used in CTMs is one of the important factors affecting the simulation results of PM2.5 chemical components. Additionally, organic species are one of the most important components in PM2.5 and gain much attention in relation to human health. While the number of organic species in the source profile is relatively low, which brings a challenge for simulation test designing, the influence of the source profile on the simulation results of organic species is not taken into account in this study.
With changes in fuel and raw materials, the development of production technology, and the innovation of pollution treatment technology in recent years, some components have changed significantly in source profiles. Given the important role of air quality simulation in decision-making for pollution control and health risk assessment, the representativeness and timeliness of the source profile should be considered.
Our study tentatively discussed the influence of mechanisms of PM2.5 emission source profiles on the simulation results of components in CTMs. The size distribution, mixing state, aging and solubility for different aerosol components might have something to do with source profile; the extent of the influence of source profile changes on the simulation of these physical and chemical process deserves future study.
The source code for CMAQ version 5.0.2 is available at https://github.com/USEPA/CMAQ/tree/5.0.2 (last access: April 2014, https://doi.org/10.5281/zenodo.1079898, US EPA Office of Research and Development, 2014). The source code for WRF version 3.7.1 is available at https://doi.org/10.5065/D68S4MVH (Skamarock et al., 2008).
The input datasets for WRF simulation are available at https://doi.org/10.5065/D6M043C6 (National Center for Atmospheric Research (NCAR), 2000). The PM2.5 emission source profiles are from the database of Source Profiles of Air Pollution (SPAP) (http://www.nkspap.com:9091/ Feng et al., 2020, Nankai University), the SPECIATE database (https://www.epa.gov/sites/default/files/2019-07/speciate_5.0_0.zip, Hsu et al., 2019, the US Environmental Protection Agency (EPA)) and the Mendeley Data repository (https://doi.org/10.17632/x8dfshjt9j.2, Bi et al., 2019b). A tutorial guide for accessing the database of Source Profiles of Air Pollution (SPAP), input and output data, and the emission data repository is available (https://doi.org/10.5281/zenodo.10122628, Luo, 2022). Surface Observational Weather Data can be found at https://doi.org/10.5065/4F4P-E398 (National Centers for Environmental Prediction/National Weather Service/NOAA/U.S. Department of Commerce, 2004) and Upper Air Observational Weather Data at https://doi.org/10.5065/39C5-Z211 (Satellite Services Division/Office of Satellite Data Processing and Distribution/NESDIS/NOAA/U.S. Department of Commerce, and National Centers for Environmental Prediction/National Weather Service/NOAA/U.S. Department of Commerce).
The supplement related to this article is available online at: https://doi.org/10.5194/gmd-16-6757-2023-supplement.
ZL: data curation and collection, writing (original draft). YH: modeling, writing (original draft). KH: data collection. YZ: supervision (review and editing). JW: supervision on the source profile. XB: supervision on the source profile. QD: resources. BL: resources. YC: modification and editing. XL: supervision on modeling. YF: supervision (review and editing).
The contact author has declared that none of the authors has any competing interests.
Publisher's note: Copernicus Publications remains neutral with regard to jurisdictional claims made in the text, published maps, institutional affiliations, or any other geographical representation in this paper. While Copernicus Publications makes every effort to include appropriate place names, the final responsibility lies with the authors.
We would like to thank the National Natural Science Foundation of China (grant number 42177465) for providing funding for the project. We are grateful for the Inventory Spatial Allocation Tool (ISAT) provided by Kun Wang from the Department of Air Pollution Control, Institute of Urban Safety and Environmental Science, Beijing Academy of Science and Technology. We thank Klaus Klingmüller (handling editor), Astrid Kerkweg (executive editor) and the three anonymous referees for the time and effort spent in reviewing the manuscript. We also thank Polina Shvedko, Sarah Schneemann, Sarah Buchmann, Anja Kesting, Lisa Appel, Gerrit Armbrecht, Madeleine Patston and Daniela Dawin for their suggestions and assistance.
This research has been supported by the National Natural Science Foundation of China (grant no. 42177465).
This paper was edited by Klaus Klingmüller and reviewed by three anonymous referees.
Appel, K. W., Pouliot, G. A., Simon, H., Sarwar, G., Pye, H. O. T., Napelenok, S. L., Akhtar, F., and Roselle, S. J.: Evaluation of dust and trace metal estimates from the Community Multiscale Air Quality (CMAQ) model version 5.0, Geosci. Model Dev., 6, 883–899, https://doi.org/10.5194/gmd-6-883-2013, 2013.
Bi, X., Dai, Q., Wu, J., Zhang, Q., Zhang, W., Luo, R., Cheng, Y., Zhang, J., Wang, L., Yu, Z., Zhang, Y., Tian, Y., and Feng, Y.: Characteristics of the main primary source profiles of particulate matter across China from 1987 to 2017, Atmos. Chem. Phys., 19, 3223–3243, https://doi.org/10.5194/acp-19-3223-2019, 2019a.
Bi, X., Dai, Q., Wu, J., Zhang, Q., Zhang, W., Luo, R., Cheng, Y., Zhang, J., Wang, L., Yu, Z., Zhang, Y., Tian, Y., and Feng, Y.: Data for: chemical compositions of the main source profiles of ambient particulate matter across China, Mendeley Data [data set], https://doi.org/10.17632/x8dfshjt9j.2, 2019b.
Cao, J., Qiu, X., Gao, J., Wang, F., Wang, J., Wu, J., and Peng, L.: Significant decrease in SO2 emission and enhanced atmospheric oxidation trigger changes in sulfate formation pathways in China during 2008–2016, J. Clean. Prod., 326, 129396, https://doi.org/10.1016/j.jclepro.2021.129396, 2021.
Chapel Hill, N.: Operational Guidance for the Community Multiscale Air Quality (CMAQ) Modeling System Version 5.0, https://www.airqualitymodeling.org/index.php/CMAQ_version_5.0_(February_2010_release)_OGD#Aerosol_Module, last access: February 2012.
Chen, Z., Chen, D., Zhao, C., Kwan, M., Cai, J., Zhuang, Y., Zhao, B., Wang, X., Chen, B., Yang, J., Li, R., He, B., Gao, B., Wang, K., and Xu, B.: Influence of meteorological conditions on PM2.5 concentrations across China: A review of methodology and mechanism, Environ. Int., 139, 105558, https://doi.org/10.1016/j.envint.2020.105558, 2020.
Cheng, N. L., Meng, F., Wang, J. K., Chen, Y. B., Wei, X., and Han, H.: Numerical simulation of the spatial distribution and deposition of PM2.5 in East China coastal area in 2010, J. Safety Environ., 15, 305–310, https://doi.org/10.13637/j.issn.1009-6094.2015.06.063, 2015 (in Chinese).
Eder, B. K. and Yu, S. C.: A performance evaluation of the 2004 release of Models-3 CMAQ, Atmos. Environ., 40, 4811–4824, https://doi.org/10.1007/978-0-387-68854-1_57, 2006.
Feng, Y., Bi, X., Wu, J., and Zhang, Y.: The state environment protection key laboratory of urban particulate air pollution prevention, Database of Source Profiles of Air pollution (SPAP) [data set], http://www.nkspap.com:9091/ (last access: 14 November 2023), 2020.
Foley, K. M., Roselle, S. J., Appel, K. W., Bhave, P. V., Pleim, J. E., Otte, T. L., Mathur, R., Sarwar, G., Young, J. O., Gilliam, R. C., Nolte, C. G., Kelly, J. T., Gilliland, A. B., and Bash, J. O.: Incremental testing of the Community Multiscale Air Quality (CMAQ) modeling system version 4.7, Geosci. Model Dev., 3, 205–226, https://doi.org/10.5194/gmd-3-205-2010, 2010.
Fountoukis, C. and Nenes, A.: ISORROPIA II: a computationally efficient thermodynamic equilibrium model for K+–Ca2+–Mg2+––Na+–––Cl−–H2O aerosols, Atmos. Chem. Phys., 7, 4639–4659, https://doi.org/10.5194/acp-7-4639-2007, 2007.
Fu, X., Wang, S., Zhao, B., Xing, J., Cheng, Z., Liu, H., and Hao, J.: Emission inventory of primary pollutants and chemical speciation in 2010 for the Yangtze River Delta region, China, Atmos. Environ., 70, 39–50, https://doi.org/10.1016/j.atmosenv.2012.12.034, 2013.
Fu, X., Wang, S. X., Chang, X., Cai, S., Xing, J., and Hao, J. M.: Modeling analysis of secondary inorganic aerosols over China: pollution characteristics, and meteorological and dust impacts, Sci. Rep., 6, 35992, https://doi.org/10.1038/srep35992, 2016.
Gao, S., Zhang, S., Che, X., Ma, Y., Chen, X., Duan, Y., Fu, Q., Wang, S., Zhou, B., Wei, C., and Jiao, Z.: New understanding of source profiles: Example of the coating industry, J. Clean. Prod., 357, 132025, https://doi.org/10.1016/j.jclepro.2022.132025, 2022.
Guo, R., Yang, J., and Liu, Z.: Influence of heat treatment conditions on release of chlorine from Datong coal, J. Anal. Appl. Pyrol., 71, 179–186, https://doi.org/10.1016/S0165-2370(03)00086-X, 2004.
Guo, Y. Y., Gao, X., Zhu, T. Y., Luo, L., and Zheng, Y.: Chemical profiles of PM emitted from the iron and steel industry in northern China, Atmos. Environ., 150, 187–197, https://doi.org/10.1016/j.atmosenv.2016.11.055, 2017.
Guo, Z., Hao, Y., Tian, H., Bai, X., Wu, B., Liu, S., Luo, L., Liu, W., Zhao, S., Lin, S., Lv, Y., Yang, J., and Xiao, Y.: Field measurements on emission characteristics, chemical profiles, and emission factors of size-segregated PM from cement plants in China, Sci. Total Environ., 818, 151822, https://doi.org/10.1016/j.scitotenv.2021.151822, 2021.
Han, Y., Xu, H., Bi, X. H., Lin, F. M., Li, J., Zhang, Y. F., and Feng, Y. C.: The effect of atmospheric particulates on the rainwater chemistry in the Yangtze River Delta, China, J. Air Waste Manage., 69, 1452–1466, https://doi.org/10.1080/10962247.2019.1674750, 2019.
Hopke, P. K., Dai, Q., Li, L., Feng, Y.: Global review of recent source apportionments for airborne particulate matter, Sci. Total Environ., 740, 140091, https://doi.org/10.1016/j.scitotenv.2020.140091, 2020.
Hopke, P. K., Feng, Y. C., and Dai, Q.: Source apportionment of particle number concentrations: A global review, Sci. Total Environ., 819, 153104, https://doi.org/10.1016/j.scitotenv.2022.153104, 2022.
Hsu, Y., Divita, F., and Dorn, J.: SPECIATE 5.0 – Speciation Database Development Documentation, Final Report, Abt Associates Inc./Office of Research and Development/U.S. Environmental Protection Agency Research Triangle Park, NC27711, [data set], https://www.epa.gov/sites/default/files/2019-07/speciate_5.0_0.zip (last access: 14 November 2023), 2019.
Huang, C. H., Hu, J. L., Xue, T., Xu, H., and Wang, M.: High-Resolution Spatiotemporal Modeling for Ambient PM2.5 Exposure Assessment in China from 2013 to 2019, Environ. Sci. Technol., 55, 2152–2162, https://doi.org/10.1021/acs.est.0c05815, 2021.
Huang, Z. J., Zheng, J. Y., Qu, J. M., Zhong, Z. M., Wu, Y. Q., and Shao, M.: A Feasible Methodological Framework for Uncertainty Analysis and Diagnosis of Atmospheric Chemical Transport Models, Environ. Sci. Technol., 53, 3110–3118, https://doi.org/10.1021/acs.est.8b06326, 2019.
Ji, Z., Gan, M., Fan, X., Chen, X., Li, Q., Lv, W., Tian, Y., Zhou, Y., and Jiang, T.: Characteristics of PM2.5 from iron ore sintering process: Influences of raw materials and controlling methods, J. Clean. Prod., 148, 12–22, https://doi.org/10.1016/j.jclepro.2017.01.103, 2017.
Li, J., Wu, Y., Ren, L., Wang, W., Tao, J., Gao, Y., Li, G., Yang, X., Han, Z., and Zhang, R.: Variation in PM2.5 sources in central North China Plain during 2017–2019: Response to mitigation strategies, J. Environ. Manage., 28, 112370, https://doi.org/10.1016/j.jenvman.2021.112370, 2021.
Li, M., Hu, M., Du, B., Guo, Q., Tan, T., Zheng, J., Huang, X., He, L., Wu, Z., and Guo, S.: Temporal and spatial distribution of PM2.5 chemical composition in a coastal city of Southeast China, Sci. Total Environ., 605–606, 337–346, https://doi.org/10.1016/j.scitotenv.2017.03.260, 2017a.
Li, M., Liu, H., Geng, G., Hong, C., Liu, F., Song, Y., Tong, D., Zheng, B., Cui, H., Man, H., Zhang, Q., and He, K.: Anthropogenic emission inventories in China: a review, Natl. Sci. Rev., 4, 834–866, https://doi.org/10.1093/nsr/nwy044, 2017b.
Li, X., He, K., Li, C., Yang, F., Zhao, Q., Ma, Y., Chen, Y., Ouyang, W., and Chen, G.: PM2.5 mass, chemical composition, and light extinction before and during the 2008 Beijing Olympics, J. Geophys. Res., 118, 12158–12167, https://doi.org/10.1002/2013JD020106, 2013.
Liang, F., Xiao, Q., Yang, X., Liu, F., Li, J., Lu, X., Liu, Y., and Gu, D.: The 17-y spatiotemporal trend of PM2.5 and its mortality burden in China, P. Natl. Acad. Sci. USA, 117, 25601–25608, https://doi.org/10.1073/pnas.1919641117, 2020.
Luo, Z.: The effect of emission source chemical profiles on simulated PM2.5 components: sensitivity analysis with CMAQ 5.0.2, Zenodo [data set], https://doi.org/10.5281/zenodo.10122628, 2022.
Lv, L., Wei, P., Li, J., and Hu, J.: Application of machine learning algorithms to improve numerical simulation prediction of PM2.5 and chemical components, Atmos. Pollut. Res., 12, 101211, https://doi.org/10.1016/j.apr.2021.101211, 2021.
National Centers for Environmental Prediction/National Weather Service/NOAA/U.S. Department of Commerce: NCEP FNL Operational Model Global Tropospheric Analyses, Research Data Archive at the National Center for Atmospheric Research, Computational and Information Systems Laboratory [data set], https://doi.org/10.5065/D6M043C6, 2000.
National Centers for Environmental Prediction/National Weather Service/NOAA/U.S. Department of Commerce: NCEP ADP Global Surface Observational Weather Data, Research Data Archive at the National Center for Atmospheric Research, Computational and Information Systems Laboratory [data set], https://doi.org/10.5065/4F4P-E398, 2004.
NBS (National Bureau of Stastistics of China): China Statistical Yearbook 2021, http://www.stats.gov.cn/sj/ndsj/2021/indexch.htm (last access: 14 November 2023), 2021.
Peterson, G., Hogrefe, C., Corrigan, A., Neas, L., Mathur, R., Rappold, A.: Impact of Reductions in Emissions from Major Source Sectors on Fine Particulate Matter–Related Cardiovascular Mortality, Environ. Health Persp., 128, 017005, https://doi.org/10.1289/EHP5692, 2020.
Qi, H., Cui, C., Zhao, T., Bai, Y., and Liu, L.: Numerical simulation on the characteristics of PM2.5 heavy pollution and the influence of weather system in Hubei Province in winter 2015, Meteorological Monthly, 45, 1113–1122, ISBN 1000-0526, 2019 (in Chinese).
Satellite Services Division/Office of Satellite Data Processing and Distribution/NESDIS/NOAA/U.S. Department of Commerce, and National Centers for Environmental Prediction/National Weather Service/NOAA/U.S. Department of Commerce: NCEP ADP Global Upper Air Observational Weather Data, Research Data Archive at the National Center for Atmospheric Research, Computational and Information Systems Laboratory, [data set], https://doi.org/10.5065/39C5-Z211, 2004.
Seinfeld, J. H. and Pandis, S. N.: Atmospheric Chemistry and Physics, from air pollution to climate change, John Wiley & Sons, Inc., Hoboken, New Jersey, 47–61, ISBN9781119221166, 2006.
Sha, T., Ma, X., Jia, H., Tian, R., Chang, Y., Cao, F., and Zhang, Y.: Aerosol chemical component: Simulations with WRF-Chem and comparison with observations in Nanjing, Atmos. Environ., 218, 1–14, https://doi.org/10.1016/j.atmosenv.2019.116982, 2019.
Shi, W., Liu, C., Norback, D., Deng, Q., Huang, C., Qian, H., Zhang, X., Sundell, J., Zhang, Y., Li, B., Kan, H., and Zhao, Z.: Effects of fine particulate matter and its constituents on childhood pneumonia: a cross-sectional study in six Chinese cities, Lancet, 392, s79, https://doi.org/10.1016/S0140-6736(18)32708-9, 2018.
Shi, Z., Li, J., Huang, L., Wang, P., Wu, L., Ying, Q., Zhang, H., Lu, L., Liu, X., Liao, H., and Hu, J.: Source apportionment of fine particulate matter in China in 2013 using a source-oriented chemical transport model, Sci. Total Environ., 601–602, 1476–1487, https://doi.org/10.1016/j.scitotenv.2017.06.019, 2017.
Skamarock, W., Klemp, J., Dudhia, J., Gill, D., Barker, D., Duda, D., Huang, X., Wang, W., and Powers, J.: A Description of the Advanced Research WRF Version 3, NCAR Tech. [code], https://doi.org/10.5065/D68S4MVH, 2008.
Song, S. Y., Wang, Y. S., Wang, Y. L., Wang, T., and Tan, H. Z.: The characteristics of particulate matter and optical properties of Brown carbon in air lean condition related to residential coal combustion, Powder Technol., 379, 505–514, https://doi.org/10.1016/j.powtec.2020.10.082, 2021.
Tang, X. Y., Zhang, Y. H., and Shao, M.: Atmosphere Environment Chemistry, 2nd Edn., Higher Education Press, Beijing, China, 268–329, ISBN 978-7-04-019361-9, 2006 (in Chinese).
US EPA Office of Research and Development: CMAQv5.0.2 (5.0.2), Zenodo [code], https://doi.org/10.5281/zenodo.1079898, 2014.
Wang, C., Zheng, J., Du, J., Wang, G., Klemes, J., Wang, B., Liao, Q., and Liang, Y.: Weather condition-based hybrid models for multiple air pollutants forecasting and minimisation, J. Clean. Prod., 352, 131610, https://doi.org/10.1016/j.jclepro.2022.131610, 2022.
Wang, D., Hu, J., Xu, Y., Lv, D., Xie, X., Kleeman, M., Xing, J., Zhang, H., and Ying, Q.: Source contributions to primary and secondary inorganic particulate matter during a severe wintertime PM2.5 pollution episode in Xi'an, China, Atmos. Environ., 97, 182–194, https://doi.org/10.1016/j.atmosenv.2014.08.020, 2014.
Weagle, C., Sinder, G., Li, C. C., Donkelaar, A., S, P., Bissonnette, P., Burke, I., Jackson, J., Latimer, R., Stone, E., Abboud, I., Akoshile, C., Anh, N., Brook, J., Cohen, A., Dong, J., Gibson, M., Griffith, D., He, K., Holben, B., Kahn, R., Keller, C., Kim, J., Lagrosas, N., Lestari, P., Khian, Y., Liu, Y., Marais, E., Martins, J., Misra, A., Muliane, U., Pratiwi, R., Quel, E., Salam, A., Segey, L., Tripathi, S., Wang, C., Zhang, Q., Brauer, M., Rudich, Y., and Martin, R.: Global Sources of Fine Particulate Matter: Interpretation of PM2.5 Chemical Composition Observed by SPARTAN using a Global Chemical Transport Model, Environ. Sci. Technol., 52, 11670–11681, https://doi.org/10.1021/acs.est.8b01658, 2018.
Wongphatarakul, V., Friedlander, S. K., and Pinto, J. P.: A Comparative Study of PM2.5 Ambient Aerosol Chemical Databases, Environ. Sci. Technol., 32, 3926–3934, https://doi.org/10.1021/es9800582, 1998.
Wu, B., Bai, X., Liu, W., Zhu, C., Hao, Y., Lin, S., Liu, S., Luo, L., Liu, X., Zhao, S., Hao, J., and Tian, H.: Variation characteristics of final size-segregated PM emissions from ultralow emission coal-fired power plants in China, Environ. Pollut., 259, 113886, https://doi.org/10.1016/j.envpol.2019.113886, 2020.
Wu, D., Zheng, H., Li, Q., Jin, L., Lyu, R., Ding, X., Huo, Y., Zhao, B., Jiang, J., Chen, J., Li, X., and Wang, S.: Toxic potency-adjusted control of air pollution for solid fuel combustion, Nat. Energ., 7, 194–202, https://doi.org/10.1038/s41560-021-00951-1, 2022.
Wu, Z. X., Hu, T. F., Hu, W., Shao, L. Y., Sun, Y. Z., Xue, F. L., and Niu, H. Y.: Evolution in physicochemical properties of fine particles emitted from residential coal combustion based on chamber experiment, Gondwana Res., 110, 252–263, https://doi.org/10.1016/j.gr.2021.10.017, 2021.
Xia, Z. Q., Fan, X. L., Huang, Z. J., Liu, Y. C., Yin, X. H., Ye, X., and Zheng, J. Y.: Comparison of Domestic and Foreign PM2.5 Source Profiles and Influence on Air Quality Simulation, Res. Environ. Sci., 30, 359–367, https://doi.org/10.13198/j.issn.1001-6929.2017.01.55, 2017 (in Chinese).
Yang, F., Tan, J., Zhao, Q., Du, Z., He, K., Ma, Y., Duan, F., Chen, G., and Zhao, Q.: Characteristics of PM2.5 speciation in representative megacities and across China, Atmos. Chem. Phys., 11, 5207–5219, https://doi.org/10.5194/acp-11-5207-2011, 2011.
Ying, Q., Feng, M., Song, D. L., Wu, L., Hu, J., Zhang, H., Kleeman, M., and Li, X.: Improve regional distribution and source apportionment of PM2.5 trace elements in China using inventory-observation constrained emission factors, Sci. Total Environ., 624, 355–365, https://doi.org/10.1016/j.scitotenv.2017.12.138, 2018.
Yu, S., Mathur, R., Pleim, J., Wong, D., Gilliam, R., Alapaty, K., Zhao, C., and Liu, X.: Aerosol indirect effect on the grid-scale clouds in the two-way coupled WRF–CMAQ: model description, development, evaluation and regional analysis, Atmos. Chem. Phys., 14, 11247–11285, https://doi.org/10.5194/acp-14-11247-2014, 2014.
Yu, Z. C., Jang, M., Kim, S., Bae, C., Koo, B., Beardsley, R., Park, J., Chang, L., Lee, H., Lim, Y., Cho, J.: Simulating the Impact of Long-Range-Transported Asian Mineral Dust on the Formation of Sulfate and Nitrate during the KORUS-AQ Campaign, Earth Space Chem., 4, 1039–1049, https://doi.org/10.1021/acsearthspacechem.0c00074, 2020.
Zhang, J., Wu, J., Lv, R., Song, D., Huang, F., Zhang, Y., and Feng, Y.: Influence of Typical Desulfurization Process on Flue Gas Particulate Matter of Coal-fired Boilers, Environ. Sci., 41, 4455–4461, https://doi.org/10.13227/j.hjkx.202003193, 2020 (in Chinese).
Zhang, Q., Xue, D., Wang, S., Wang, L., Wang, J., Ma, Y., and Liu, X.: Analysis on the evolution of PM2.5 heavy air pollution process in Qingdao (In Chinese), China Environ. Sci., 37, 3623–3635, https://doi.org/10.3969/j.issn.1000-6923.2017.10.003, 2017.
Zhang, S. P., Xing, J., Sarwar, G., Ge, Y. L., He, H., Duan, F., Zhao, Y., He, K., Zhu, L., and Chu, B.: Parameterization of heterogeneous reaction of SO2 to sulfate on dust with coexistence of NH3 and NO2 under different humidity conditions, Atmos. Environ., 208, 133–140, https://doi.org/10.1016/j.atmosenv.2019.04.004, 2019.
Zheng, B., Tong, D., Li, M., Liu, F., Hong, C., Geng, G., Li, H., Li, X., Peng, L., Qi, J., Yan, L., Zhang, Y., Zhao, H., Zheng, Y., He, K., and Zhang, Q.: Trends in China's anthropogenic emissions since 2010 as the consequence of clean air actions, Atmos. Chem. Phys., 18, 14095–14111, https://doi.org/10.5194/acp-18-14095-2018, 2018.
Zheng, B., Zhang, Q., Zhang, Y., He, K. B., Wang, K., Zheng, G. J., Duan, F. K., Ma, Y. L., and Kimoto, T.: Heterogeneous chemistry: a mechanism missing in current models to explain secondary inorganic aerosol formation during the January 2013 haze episode in North China, Atmos. Chem. Phys., 15, 2031–2049, https://doi.org/10.5194/acp-15-2031-2015, 2015.
Zheng, H., Song, S., Sarwar, G., Gen, M., Wang, S., Ding, D., Chang, X., Zhang, S., Xing, J., Sun, Y. L., Ji, D., Chan, C. K., Gao, J., and Mcelory, M.: Contribution of Particulate Nitrate Photolysis to Heterogeneous Sulfate Formation for Winter Haze in China, Environ. Sci. Technol. Lett., 7, 632–638, https://doi.org/10.1021/acs.estlett.0c00368, 2020.
Zhou, L., Chen, X., and Tian, X.: The impact of fine particulate matter (PM2.5) on China's agricultural production from 2001 to 2010, J. Clean. Prod., 178, 133–141, https://doi.org/10.1016/j.jclepro.2017.12.204, 2018.
- Abstract
- Introduction
- Model and data
- Is there an impact of variation in the source profile on the simulation results?
- How large is the impact?
- How does the impact work?
- Conclusions
- Code availability
- Data availability
- Author contributions
- Competing interests
- Disclaimer
- Acknowledgements
- Financial support
- Review statement
- References
- Supplement
- Abstract
- Introduction
- Model and data
- Is there an impact of variation in the source profile on the simulation results?
- How large is the impact?
- How does the impact work?
- Conclusions
- Code availability
- Data availability
- Author contributions
- Competing interests
- Disclaimer
- Acknowledgements
- Financial support
- Review statement
- References
- Supplement