the Creative Commons Attribution 4.0 License.
the Creative Commons Attribution 4.0 License.
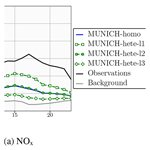
Modelling concentration heterogeneities in streets using the street-network model MUNICH
Alice Maison
Yelva Roustan
Matthias Ketzel
Steen Solvang Jensen
Youngseob Kim
Christophe Chaillou
Populations in urban areas are exposed to high local concentrations of pollutants, such as nitrogen dioxide and particulate matter, because of unfavourable dispersion conditions and the proximity to traffic. To simulate these concentrations over cities, models like the street-network model MUNICH (Model of Urban Network of Intersecting Canyons and Highways) rely on parameterizations to represent the air flow and the concentrations of pollutants in streets. In the current version, MUNICH v2.0, concentrations are assumed to be homogeneous in each street segment. A new version of MUNICH, where the street volume is discretized, is developed to represent the street gradients and to better estimate peoples' exposure. Three vertical levels are defined in each street segment. A horizontal discretization is also introduced under specific conditions by considering two zones with a parameterization taken from the Operational Street Pollution Model (OSPM). Simulations are performed over two districts of Copenhagen, Denmark, and one district of greater Paris, France. Results show an improvement in the comparison to observations, with higher concentrations at the bottom of the street, closer to traffic, of pollutants emitted by traffic (NOx, black carbon, organic matter). These increases reach up to 60 % for NO2 and 30 % for PM10 in comparison to MUNICH v2.0. The aspect ratio (ratio between building height and street width) influences the extent of the increase of the first-level concentrations compared to the average of the street. The increase is higher for wide streets (low aspect ratio and often higher traffic) by up to 53 % for NOx and 18 % for PM10. Finally, a sensitivity analysis with regard to the influence of the street network highlights the importance of using the model MUNICH with a network rather than with a single street.
- Article
(7185 KB) - Full-text XML
- BibTeX
- EndNote
Pollution is estimated to have been responsible for approximately 9 million premature deaths in 2015 (Landrigan et al., 2018). This figure remains valid in 2019 despite an improvement in the types of pollution associated with extreme poverty (e.g. household air pollution and water pollution) (Fuller et al., 2022). This is partly due to an increase in the number of premature deaths attributable to ambient air pollution. The consequences of air pollution are particularly substantial in urban areas, where individuals are exposed to local high concentrations of air pollutants due to unfavourable dispersion conditions and proximity to traffic. As more than half of the world's population already lives in urban areas, with this being expected to rise to 68 % by 2050 (United Nations, 2019), it is crucial to estimate as accurately as possible the exposure of the population to atmospheric pollutant concentrations in urban areas. For many years, various modelling approaches have been developed to contribute to the understanding of the phenomena that drive the concentrations of pollutants in the atmosphere and to provide decision support tools (Collett and Oduyemi, 1997; Vardoulakis et al., 2003; El-Harbawi, 2013; Conti et al., 2017; Khan and Quamrul, 2021).
Regional-scale chemistry transport models, such as Polair3D (Mallet et al., 2007; Sartelet et al., 2018), CHIMERE (Menut et al., 2021; Falasca and Curci, 2018), and CMAQ (Wong et al., 2012; de la Paz et al., 2015), represent the urban background concentrations by solving the chemistry transport equation for spatial resolutions down to 1 km2. However, they cannot represent street concentrations, which are often higher than background concentrations for pollutants such as NO2 and particles (Lugon et al., 2020). To represent these concentrations, local-scale models are thus developed with different approaches of variable complexity and computational cost. Models based on computational fluid dynamics (CFD), such as code_saturne (Archambeau et al., 2004; Gao et al., 2018), OpenFoam (Lin et al., 2023), and PALM (Wolf et al., 2020), are able to represent the dispersion of pollutants and the physicochemical processes taking place in urban districts and streets with a fine spatial resolution by solving the Navier–Stokes equations and mass conservation equations for pollutants. However, they suffer from high computational cost as they use fine meshes to describe the morphology of buildings and streets. Other models use approaches that are less accurate than CFD but that run faster. They are typically based on a Gaussian or an Eulerian approach (Vardoulakis et al., 2003; Liang et al., 2023). Among these, the following can be mentioned: ADMS-Urban (McHugh et al., 1997; Hood et al., 2021), SIRANE (Soulhac et al., 2011, 2023), AERMOD (Cimorelli et al., 2004; Rood, 2014), EPISODE (Karl et al., 2019), and CALIOPE-Urban (Benavides et al., 2019). Either they consider each street independently of the others with exchanges between the street and the background concentrations above the street (Berkowicz, 2000b), or they consider a street network with incoming and/or outcoming flows between streets at intersections (Soulhac et al., 2009; Kim et al., 2022). The Operational Street Pollution Model (OSPM) couples a Gaussian plume model for traffic emissions and a box model for the recirculation in the street (Berkowicz, 2000b). It is thus able to represent concentration heterogeneities in the street but cannot include complex chemistry. The Model of Urban Network of Intersection Canyons and Highways (MUNICH) uses solely an Eulerian box model approach (Lugon et al., 2020). As MUNICH is coupled with the SSH-aerosol model (Sartelet et al., 2020), the formation and ageing of primary and secondary gas and particles in streets are represented. In the current version of MUNICH (v2.0) (Kim et al., 2022), concentrations are considered to be homogeneous in each street segment. However, as shown by several on-site and modelling studies, the concentrations are very heterogeneous in streets with traffic emissions (Xie et al., 2003; Vardoulakis et al., 2011; Lateb et al., 2016; Sanchez et al., 2016; Amato et al., 2019; Lin et al., 2023). They are higher near the ground than at the top of the street, especially for primary pollutants.
A heterogeneous version of MUNICH is developed in this study, with the aim of representing the concentration heterogeneities in the street while keeping the Eulerian approach of MUNICH to retain its ability to accurately model chemistry and aerosol dynamics. The street volume is discretized vertically in three subvolumes. Traffic emissions are not instantaneously diluted in the whole street volume, as in MUNICH v2.0, but only in the first subvolume, i.e. the one closest to the ground. With this discretization we do not aim to reproduce finely the vertical profile of concentrations. The main objective is to improve the representation of concentrations close to the ground by avoiding the excessive dilution associated with the homogeneity assumption. To represent the streets' horizontal heterogeneities, a recirculation zone in the shape of a trapeze is defined based on a parameterization of OSPM. It depends on the meteorological conditions and the street morphology, and it is applied under specific conditions in MUNICH that are described later.
A description of the differences between the homogeneous version of MUNICH (v2.0) and the new heterogeneous version is presented in Sect. 2. The applications to two street networks in Copenhagen, Denmark, with comparisons to observations of NO2 and CO, and to concentrations simulated by OSPM are discussed in Sect. 3. MUNICH is applied in Sect. 4 to the street network near Paris, France, used to validate MUNICH v2.0 (Kim et al., 2022), and the impacts of the heterogeneous version on concentrations of NO2 and particles are studied. To estimate the impact of the modelling hypothesis on the transport of pollutants between streets in the new version of MUNICH, a sensitivity analysis with regard to the presence of a street network is performed in Sect. 5.
The homogeneous version of MUNICH (v2.0) and the new heterogeneous version (see Fig. 1) are briefly described in this section, with a focus on the differences between the two versions. The heterogeneous version was developed from MUNICH v2.0. In the following, the homogeneous and heterogeneous versions of MUNICH are referred to as MUNICH-homo and MUNICH-hete, respectively. For a complete description of MUNICH, please refer to Kim et al. (2018, 2022) and Lugon et al. (2020, 2021a).
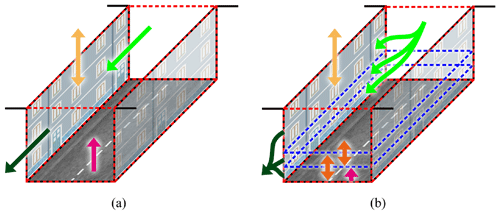
Figure 1Representation of the processes in the homogeneous version (a) and the heterogeneous version (b) of MUNICH. The dotted red lines represent the street volume. In (a), the street canopy is represented by a single volume, whereas in (b), it is divided into three subvolumes delimited by the dotted blue lines. The rose arrows represent the traffic emissions (including brake, tyre, and road wear), the light-green arrows represent the fluxes entering the street via the upwind intersection, and the dark-green arrows represent the fluxes leaving the street via the downwind intersection. The yellow arrows symbolize the vertical turbulent exchanges with the background, and in (b), the orange arrows symbolize the vertical exchanges among the subvolumes.
In order to solve the evolution equation of the street concentrations Cstr, a first-order operator splitting between transport and chemistry is performed:
The transport term includes advection from one street to another and vertical transport between the street and the background above it. The chemistry includes gas-phase chemistry, as well as aerosol dynamics (coagulation, condensation, and evaporation). As deposition and resuspension processes have minor effects compared to transport and chemistry (Lugon et al., 2021b; Kim et al., 2022), they are omitted in the rest of this study.
2.1 Homogeneous approach
Using a box model approach, the concentrations are assumed to be homogeneous in the whole street volume, and the effects of the processes on the concentrations are represented by the following equation:
where V is the volume of the rectangular cuboid street, Qem is the traffic emission flux, Qinflow is the flux entering the street via the upwind intersection, Qoutflow is the flux leaving the street via the downwind intersection, and Qvert is the vertical turbulent flux between the background and the street.
The street volume is defined as V=HWL, with H being the average building height over the street segment, W being the mean street width, and L being its length. MUNICH considers it to be that buildings on each side of the street are continuous, thus not representing inflow and/or outflow that could be induced by gaps in the buildings. The inflow term Qinflow is obtained from the computation of the fluxes at the upwind intersection (Kim et al., 2018; Soulhac et al., 2009) (see Sect. 5 for a brief description). The outflow term Qoutflow is expressed as follows:
with ustr being the mean horizontal wind speed in the street.
The vertical turbulent flux Qvert between the street and the overlying atmosphere is
with qvert being the vertical transfer coefficient and Cbkgd being the background concentration.
The vertical transfer coefficient and the horizontal wind speed are the key parameters representing the dispersion of concentrations. As their formulation differs between the homogeneous and the heterogeneous versions of MUNICH, they are now detailed.
2.1.1 Vertical transfer coefficient for turbulent flux
Three parameterizations are implemented in MUNICH to determine the vertical transfer coefficient qvert between the street and the overlying atmosphere (Maison et al., 2022). However, currently, only the parameterization adapted from Wang (2014) is designed to provide vertical profiles for both wind speed and mixing length within the street. We therefore limit our analysis to the latter.
In MUNICH-homo, the vertical transfer coefficient at the roof level is expressed as follows:
where σw is the standard deviation of the vertical wind velocity at roof level, and lm is the mixing length defined as a harmonic mean between two length scales (Coceal and Belcher, 2004), namely (i) κz, with the Von Kármán constant (κ=0.42), and (ii) lc, which is a characteristic length of the street chosen to be equal to 0.5W (Maison et al., 2022).
2.1.2 Mean horizontal wind speed in the street
As for the calculation of the vertical transfer coefficient, three parameterizations are proposed in MUNICH to determine the mean horizontal wind speed in the street (Maison et al., 2022). In the Wang parameterization, the mean horizontal wind speed in the street ustr is equal to
with being the wind speed at roof level in the direction of the street and z0s being the wall and ground roughness length in the street (fixed to 0.01 m). I0 and K0 are the first- and second-kind modified Bessel functions of order 0. J1 and J2 are integration coefficients equal to
The function g(z) is calculated as follows:
with being the aspect ratio of the street and CB being a coefficient that is dependent on the wind angle with the street and the aspect ratio (Maison et al., 2022).
2.2 Heterogeneous approach
In MUNICH-hete, the street is divided into three vertical levels to limit the artificial dilution of the traffic emissions and the concentrations in the whole street volume (see Figs. 1b and 2). Levels are ordered from the ground to the top of the street. The first level (i=1) contains the traffic emissions. The thickness h1 is taken as 2 m, which corresponds to a zone where the traffic producing turbulence mixes and dilutes traffic emissions (Solazzo et al., 2008). This traffic-induced turbulence is not explicitly considered in the model. The second-level (i=2) thickness h2 is also of 2 m. It acts as a buffer zone between the first level, where traffic emissions are, and the third level, where exchanges with the background take place. Starting at 4 m, the third level (i=3) goes to the roof level ( m). The minimum street height considered in the model is set at 6 m. The three levels of the heterogeneous version are referred to as MUNICH-hete-l1, MUNICH-hete-l2, and MUNICH-hete-l3. Each level i is thus associated with a specific volume Vi, and the evolution equations may be written as follows:
where is the traffic emission flux (only in the first level (i=1)), is the flux entering the level via the upwind intersection, is the flux leaving the level via the downwind intersection, is the vertical turbulent flux between the levels i and i+1 (for i=3, it is exchanged with the background), and is the vertical turbulent flux between the levels i−1 and i (equal to zero if i=1).
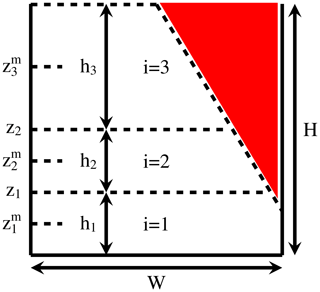
Figure 2Schematic of the discretization in MUNICH-hete. The red triangle represents the ventilation zone that is present under specific conditions, and the white trapeze represents the recirculation zone. is the middle height of level i.
Note that more than three vertical levels could be defined in MUNICH-hete as the vertical variations within the streets of winds and mixing lengths are parameterized when discretizing the streets. However, the first vertical level at the bottom of the street should not be too thin because of mixing due to traffic turbulence. A minimum height for the first layer of 1.5 m seems reasonable.
In OSPM, the flow that develops into a vortex in the street between buildings is represented by a recirculation zone. It occupies the whole street volume for narrow streets, and it has the shape of a trapeze for wider streets (Berkowicz et al., 1997; Berkowicz, 2000b; Ottosen et al., 2015). When the recirculation zone does not occupy the whole street volume, there is a ventilation zone (see Fig. 2) where concentrations of pollutants emitted by traffic are usually lower than in the recirculation zone. In MUNICH-homo, the recirculation zone is not explicit, whatever the street ratio (H/W) is. In MUNICH-hete, the volume of the recirculation zone is computed as in OSPM, as detailed in Appendix A. For now, concentrations in the ventilation zone are considered to be homogeneous and equal to the background concentrations, i.e. concentrations above the street. The ventilation zone is thus taken into account when it is not affected by traffic emissions. In practice, this means that the width of the trapeze base can only be equal to or larger than the width W of the street (see Appendix A). The width Wi of the level i can thus be inferior to the width W of the street, reducing the level volume (see Fig. 2). Appendix A presents the algorithm implemented in MUNICH-hete to consider the volume reduction of the ventilation zone. Further work is needed to differentiate the two zones for cases where the ventilation zone develops further into the street.
To quantify mass transfer through the intersections, the fluxes are assumed to be vertically homogeneous and remain determined as proposed by Soulhac et al. (2009) as the shapes of the intersections may differ from one to another, and turbulence is not quantified. The flux Qinflow entering the street is assumed to be the same for each vertical level. The flux Qoutflow leaving a street is a surface-weighted average of the fluxes leaving each vertical level of the street:
with being the vertical surface of the level i, as presented in Appendix A, and ui being the mean horizontal wind speed of the level i (see Sect. 2.2.2).
2.2.1 Vertical turbulent fluxes
To compute the vertical turbulent transfer at the interface of the vertical levels i and i+1, Eq. (4) is modified to represent the vertical exchanges between the levels i and i+1:
where Ci and Ci+1 are the concentrations in the levels i and i+1 respectively; Wi is the width of the level i that is inferior or equal to the width W of the street; and is the difference in altitude between the middles of the levels i+1 and i, which are as noted , , and (see Fig. 2). For i=3, i.e. the highest level, is taken as the symmetrical of to the roof level and is noted as . It gives an approximation of the volume that effectively exchanges with the third level.
Among the three parameterizations implemented in MUNICH to determine the vertical transfer coefficient qvert, only the Wang parameterization is adapted to the discretization. It is thanks to its explicit vertical dependency and validity for a wide range of street canyon and wind characteristics (Maison et al., 2022). To compute the vertical turbulent transfer , the vertical transfer coefficient is taken at the height of the interface between the two vertical levels considered, and Eq. (5) is now written as follows:
where zi is the height of the interface between levels i and i+1. The influence of the atmospheric stability on vertical mixing is taken into account by modifying the standard deviation of the vertical wind velocity at roof level and thus the vertical transfer rate, depending on the length of Monin–Obukhov, as in MUNICH-homo.
By combining Eqs. (11) and (12), the vertical turbulent transfer can be written for each level as follows:
with C1, C2, C3, and Cbkgd being the concentrations of the three levels and the background respectively.
2.2.2 Mean horizontal wind speed in the street
As for the vertical turbulent flux, the Wang parameterization is preferred among the three parameterizations available in MUNICH to compute the mean horizontal wind speeds in the street. This is thanks to its explicit vertical dependency and the no-slip condition at the ground that is always satisfied () (Maison et al., 2022). Therefore, the mean horizontal wind speed can be computed at each level in the street by modifying Eq. (6) to integrate vertically between the level heights:
with z1 and z2 being the limits of the first two levels, as presented in Fig. 2.
This section presents two applications of MUNICH-hete to assess its capabilities compared to MUNICH-homo and OSPM. Simulations are performed over the year 2019 to generate hourly concentrations for two street networks in Copenhagen, Denmark. The first street network is centred around H. C. Andersens Boulevard, and the second is centred around Jagtvej street. They are respectively named HCAB and JGTV in the following. These street networks have been selected as observational data of CO, NO2, NOx, and O3 are available for HCAB, and observational data of NO2 and NOx are available for JGTV, both over the whole year. OSPM simulations were also performed to compare model performances. As OSPM does not represent air fluxes at intersections, OSPM simulations are performed only for the streets where there are observations. Thanks to its coupled approach of a Gaussian plume model and a box model, OSPM is able to calculate concentrations on the two sides of the street (Berkowicz et al., 1997; Berkowicz, 2000b; Ottosen et al., 2015). Two receptors are used to compare with the observed concentrations on each side of the street: OSPM-R1 and OSPM-R2. The height of the receptors is taken as 2 m to correspond roughly to the height at which observations were performed. They are compared to the concentrations simulated at the first two levels of MUNICH-hete, which are representative of the concentrations at 1 and 3 m.
MUNICH and OSPM use the same input data to estimate the street concentrations. The meteorological parameters originate from simulations performed with the Weather Research and Forecasting model (WRF, Skamarock et al., 2008). The background concentrations are simulated using the Urban Background Model (UBM, Berkowicz, 2000a), which is “a multiple source model that applies a Gaussian approach for horizontal dispersion and a linear approach for vertical dispersion up to the boundary layer” (Jensen et al., 2016). Traffic emissions are generated using the procedure implemented in the local-scale Gaussian air pollution model OML-Highway (Olesen et al., 2015), allowing for precise information for each street segment. Traffic data (average daily traffic, travel speed, and share of heavy-duty vehicles) are used to generate emissions by use of the European emission model COPERT IV. Traffic emissions include exhaust emissions of gases and particles and non-exhaust emissions of particles. Non-exhaust emissions consist of brake, tyre, and road wear. The street parameters (building height and street width) used in MUNICH originate from the OSPM setups.
OSPM represents NO2 and O3 chemical transformations using a system of two reactions (Berkowicz et al., 1997; Berkowicz, 2000b). The first one describes the production of NO2 due to the reaction of NO with O3, and the second one describes the photodissociation of NO2 that leads to the reproduction of NO and O3. For a fair comparison, MUNICH is configured to run with a simple chemistry scheme, the Leighton photostationary state for O3 (Leighton, 1961; Kim et al., 2018):
3.1 H. C. Andersens Boulevard
H. C. Andersens Boulevard is a wide, densely trafficked boulevard, with an aspect ratio of about 0.2 (H=9.9 m, W=50 m). It is open on one side with trees instead of buildings. This configuration can be represented in OSPM. However, in MUNICH, a mean building height is defined for each street. Here, it is estimated by averaging the building and the tree heights. The simulated street network is composed of 86 street segments centred around the street where the observation station is located (see brown cross in Fig. 4). Figure 3 presents the monthly averaged concentrations of CO and NO2 from the OSPM and MUNICH simulations compared to observations. Appendix C contains monthly averaged concentrations of NOx and O3 and statistical indicators of the comparison for all four pollutants.
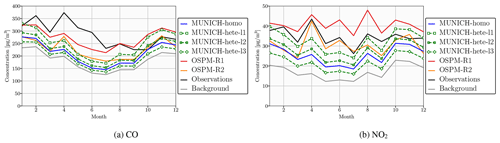
Figure 3Monthly averaged concentrations (in µg m−3) of CO (a) and NO2 (b) at HCAB monitoring station. The solid blue line represents the homogeneous version of MUNICH. The three dashed green lines represents the three levels of the heterogeneous version of MUNICH – the lowest level (l1) is shown with square markers, the intermediate level (l2) is shown with crossed markers, and the top level (l3) is shown with diamond markers. The solid red line represents the OSPM receptor that is close to the measurement station, and the solid orange line represents the second OSPM receptor located on the other side of the street. The observations are in solid black, and the background concentrations are in solid grey.
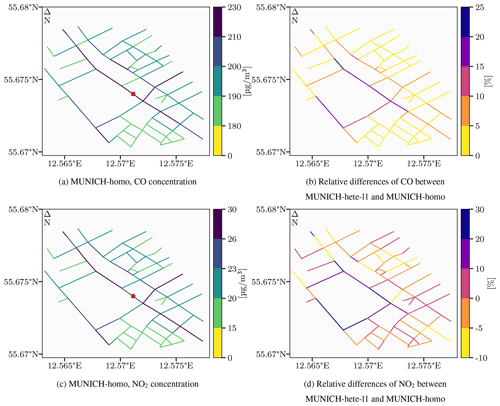
Figure 4CO and NO2 time-averaged concentrations (in µg m−3) for MUNICH-homo are shown in the upper- and lower-left panels (a, c) respectively for the HCAB street network. Relative differences (in %) between the first level of MUNICH-hete and MUNICH-homo for CO and NO2 are shown in the upper- and lower-right panels (b, d) respectively for the HCAB street network. A positive relative difference indicates higher concentrations for MUNICH-hete. The brown cross in panels (a) and (c) represents the position of the measurement station.
OSPM-R1 is the receptor that is close to the measurement station and is thus better suited to be compared to observations. Differences in the concentrations between OSPM-R1 and OSPM-R2 highlight the importance of taking into account the horizontal heterogeneities in the street. Observations lie between the concentrations simulated at the two receptors, except in the case of CO, for which OSPM slightly underestimates concentrations in the first half of the year. This underestimation could be linked to underestimation of sources other than traffic, e.g. biomass burning, at the regional scale but could also be due to the absence of volatile organic compounds in the simulation. Overall, the concentrations are well estimated with OSPM, with errors between 26 % and 33 % for CO and between 34 % and 46 % for NO2.
For CO, NO2, and NOx, which are emitted by traffic in the bottom of the street, the concentrations are higher in the first level, MUNICH-hete-l1, near the bottom and are lower in the third level, MUNICH-hete-l3, near the roof level. For O3, the opposite behaviour is observed as it is mainly imported by the atmosphere above the street, and it is titrated by NO near the ground. The first two levels, MUNICH-hete-l1 and MUNICH-hete-l2, have higher concentrations of CO, NO2, and NOx than munich-homo, thus improving the comparison to observations and OSPM concentrations. For CO, the error is improved from 36 % in MUNICH-homo to 28 % in MUNICH-hete-l1, and for NO2, it is improved from 48 % in MUNICH-homo to 35 % in MUNICH-hete-l1. Although the concentrations of NO2 and CO are underestimated in MUNICH-homo compared to observations, they are well modelled in the first level, MUNICH-hete-l1, with low errors and biases (see Appendix C). For CO, the concentrations simulated in the first level, MUNICH-hete-l1, lie between the two OSPM receptors for most of the year. This is the case for NO2 as well, except between April and August, when NO2 concentrations are underestimated in MUNICH-hete compared to observations and OSPM concentrations. The formation of NO2 in the lower levels (see Reaction R3) could be limited with the current model setup because volatile organic compounds are not taken into account in the Danish cases. However, they may participate in O3 and NO2 formation through HO2 and RO2 radicals (Atkinson, 2000; Kwak and Baik, 2012; Zhong et al., 2017; Dai et al., 2021). Furthermore, the vertical discretization is coarse, limiting O3 transport deep into the street.
Over the whole street network, as presented in Fig. 4, the differences between the concentrations simulated in the first vertical level of MUNICH-hete and those simulated in MUNICH-homo vary. For wide streets and avenues with dense traffic, the concentrations are higher in MUNICH-hete-l1 than in MUNICH-homo, with an increase of up to 23 % for CO and 30 % for NO2. This increase is lower in more narrow and less frequented streets. Although the concentrations in MUNICH-hete-l1 are always higher than those in MUNICH-homo for CO, for NO2, in narrow streets, the concentrations are lower in MUNICH-hete-l1. These lower NO2 concentrations are probably related to the limited transport of O3 from the background to the bottom of the street, limiting the titration of NO.
3.2 Jagtvej
Jagtvej is a conventional street canyon with an aspect ratio of about 0.8 (H=20.3 m, W=26.2 m). The simulated street network is composed of 265 street segments centred around the street where the observation station is located (see brown cross in Fig. 6). The monthly averaged concentrations of CO and NO2 from the OSPM and MUNICH simulations compared to observations for NO2 only are presented in Fig. 5. Appendix D contains monthly averaged concentrations of NOx and O3 and statistical indicators of the comparison for NO2 and NOx.
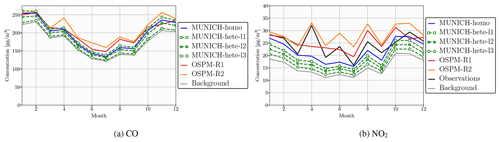
Figure 5Monthly averaged concentrations (in µg m−3) of CO (a) and NO2 (b) at JGTV monitoring station. The solid blue line represents the homogeneous version of MUNICH. The three dashed green lines represent the three levels of the heterogeneous version of MUNICH – the lowest level (l1) is shown with square markers, the intermediate level (l2) is shown with crossed markers, and the top level (l3) is shown with diamond markers. The solid orange line represents the OSPM receptor that is close to the measurement station, and the solid red line represents the second OSPM receptor located on the other side of the street. The observations (only available for NO2) are in solid black, and the background concentrations are in solid grey.
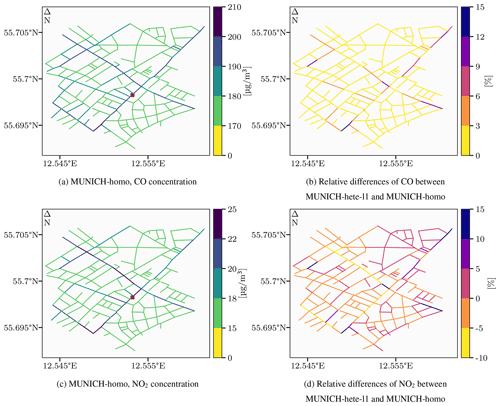
Figure 6CO and NO2 time-averaged concentrations (in µg m−3) for MUNICH-homo are shown in the upper- and lower-left panels (a, c) respectively for the JGTV street network. Relative differences (in %) between the first level of MUNICH-hete and MUNICH-homo for CO and NO2 are shown in the upper- and lower-right panels (b, d) respectively for JGTV. A positive relative difference indicates higher concentrations for MUNICH-hete. The brown cross in panels (a) and (c) represents the position of the measurement station.
OSPM-R2 is the receptor that is close to the measurement station and is thus better suited to be compared to observations. The differences between the concentrations of the two OSPM receptors are lower in JGTV than in HCAB (see Fig. 3). JGTV is narrower, with higher buildings on both sides, thus limiting the ventilation zone and the penetration into the street of background concentrations that would reduce the concentrations at the downwind receptor. OSPM tends to slightly overestimate NO2 and NOx concentrations (with errors between 41 % and 53 % and between 42 % and 63 % respectively).
Concentrations from the homogeneous version of MUNICH are close to OSPM concentrations for CO. They are lower than OSPM concentrations for NO2, but they compare well to observations, with a bias of −3 % and an error of 46 %. These lower NO2 concentrations are linked to lower NOx concentrations (with a bias of −19 % compared to observations and an error of 53 %). As for HCAB, the MUNICH-hete concentrations decrease from the bottom to the top of the street. For CO and NOx, only the first level has concentrations higher than MUNICH-homo, while for NO2, all three levels have concentrations lower than MUNICH-homo. O3 concentrations are also higher for the three levels (see Fig. D1). The NOx concentrations of MUNICH-hete-l1 compare slightly better to observations than MUNICH-homo, while MUNICH-homo is slightly better for NO2. However, the statistics of the two models are quite close (Appendix D).
Conclusions are similar for the whole street network (see Fig. 6). The CO concentrations are slightly higher in the first level of MUNICH-hete than in MUNICH-homo. However, NO2 concentrations at the bottom of the street in the first level of MUNICH-hete tend to be lower than in MUNICH-homo. In some specific street segments of the network, the differences in the concentrations for both CO and NO2 are higher in MUNICH-hete-l1 than in MUNICH-homo. This is due to a mix of different traffic emissions and street morphologies which favour the transport of pollutants.
The impacts of the discretization on gas and particle concentrations are evaluated over the street network near Paris, France, which was used to validate MUNICH v2.0 (Kim et al., 2022) and in several sensitivity studies (Lugon et al., 2021b; Sarica et al., 2022, 2023b). The street network represents a district of Le Perreux-sur-Marne, a suburb 13 km east of Paris, France. It is composed of 577 street segments (see Fig. 8). The street parameters (building height and street width) were obtained from the BD TOPO database (https://geoservices.ign.fr/bdtopo, last access: 12 September 2023). Simulations were performed from 22 March to 15 June 2014 to generate hourly concentrations with input data (emissions, including exhaust emissions and brake, tyre, and road wear; meteorological parameters; and background concentrations) from the reference simulation SCN0 of Sarica et al. (2023b). For this case, MUNICH is coupled to SSH-aerosol (Sartelet et al., 2020) to represent gas-phase chemistry and aerosol dynamics. Observational data are available for the whole simulation period for NO2, NOx, PM2.5, PM10, and black carbon (BC) for a segment of Boulevard d'Alsace Lorraine (see brown cross in Fig. 8). The simulations were performed at a height of about 2 m, and they are thus to be compared to the concentrations of the first two levels (i=1 and i=2) of MUNICH-hete. The segment has an aspect ratio of about 0.3 (H=8.6 m, W=26 m).
The average daily profiles of NOx and PM10 concentrations are shown in Fig. 7, and NO, NO2, and the statistical indicators are shown in Appendix E1. As in the Danish streets, for pollutants emitted by traffic, the concentrations are higher in MUNICH-hete at the bottom of the street, decreasing to the roof level. The concentrations of PM10, NO2, NOx, and BC are higher on average by 12 %, 21 %, 40 %, and 30 % respectively in MUNICH-hete-l1 than in MUNICH-homo. The lower concentration difference for PM10 than for the other compounds reflects the fact that non-traffic sources are more important for PM10, and inversely, they are small for BC. Despite the higher concentrations in MUNICH-hete-l1, BC concentrations remain strongly underestimated compared to observations, in agreement with the CFD simulations of Lin et al. (2023). For PM10, NO2, and NOx, the concentrations compare well to observations; e.g. the error is 33 % for NO2 and 37 % for PM10, with slightly better statistics using MUNICH-hete-l1 than MUNICH-homo. For all pollutants, the concentrations of the intermediate level (i=2) are very close to the concentrations of MUNICH-homo. This is due to a mixture of different parameters such as street morphology and dispersion conditions over the simulated period.
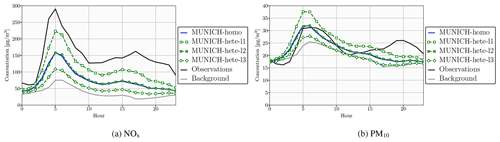
Figure 7Average daily profile of the concentrations of NOx (a) and PM10 (b) over the simulation period at the Boulevard d'Alsace Lorraine monitoring station. The solid blue line represents the homogeneous version of MUNICH. The three dashed green lines represent the three levels of the heterogeneous version of MUNICH – the lowest level (l1) is shown with square markers, the intermediate level (l2) is shown with crossed markers, and the top level (l3) is shown with diamond markers. The observations are in solid black, and the background concentrations are in solid grey.
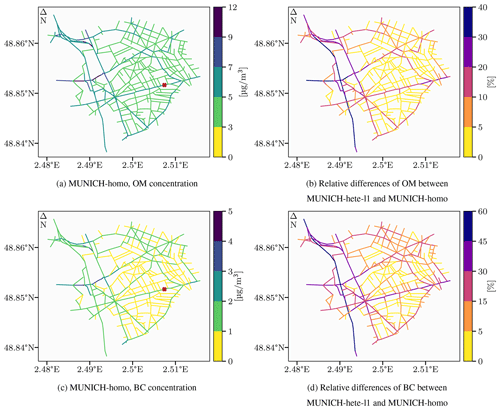
Figure 8OM and BC time-averaged concentrations (in µg m−3) for MUNICH-homo are shown in the upper- and lower-left panels (a, c) respectively for the district of Le Perreux-sur-Marne. Relative differences (in %) between the first level of MUNICH-hete and MUNICH-homo for OM and BC are shown in the upper- and lower-right panels (b, d) respectively for the district of Le Perreux-sur-Marne. A positive relative difference indicates higher concentrations for MUNICH-hete. The brown cross in panels (a) and (c) represents the position of the measurement station.
In streets, both BC and organic matter (OM) exhibit higher concentrations than in the background (Lugon et al., 2021a). OM consists of primary and secondary aerosols that are formed from the oxidation of volatile organic compounds and/or the condensation of semi-volatile organic compounds. Concentrations of both BC and OM simulated in MUNICH-homo and in the first level of MUNICH-hete are compared in Fig 8 (see Fig. E2 for NO2 and PM10). The concentrations simulated in MUNICH-hete-l1 are always higher than in MUNICH-homo. In most streets of the network that are narrow and limited in terms of traffic, the increase in concentrations is limited. It is more important for more open and frequented streets, such as the Boulevard d'Alsace Lorraine.
With BC being a primary inert pollutant emitted by traffic, its concentrations are strongly influenced by the discretization as emissions are no longer artificially diluted in the whole street volume. They are now constraint at the bottom of the street, inducing an average increase of 30 % compared to the homogeneous version. For OM and PM10, the increase is lower (16 % and 12 % respectively) because of the stronger influence of non-traffic sources.
In this section, the influence of the aspect ratio ar on the concentrations in the heterogeneous version of MUNICH is studied. The sensitivity of the heterogeneous version of MUNICH to the presence of a street network, which influences the concentrations entering the street via the upwind intersection, is also estimated.
5.1 Influence of the aspect ratio
The aspect ratio ar, defined as the ratio between building height H and street width W, is used to determine general behaviours of streets with similar geometries. When ar is small, i.e. when W is larger than H, the street is wide, such as in the case of a boulevard or an avenue. On the other hand, when ar is large, i.e. when H is larger than W, the street is narrow and closer to a typical street canyon. In the three cases presented in this study in greater Paris and Copenhagen, streets with small ar are associated with higher traffic emissions.
Table 1 and Appendix F present the influence of the aspect ratio on concentrations in the first vertical level of the heterogeneous version of MUNICH, MUNICH-hete-l1, compared to the homogeneous version. It is quantified using the normalized mean bias (NMB). The homogeneous version of MUNICH presents higher concentrations of pollutants in wide streets (interval [0,0.5[) in greater Paris due to higher traffic emissions compared to in narrower streets. The increase in the concentration in the first level as a result of using the heterogeneous version of the model is more important for wide streets (up to 53 % for NOx). Concerning particles, PM10 concentrations are increased by up to 18 %, while the increase is about 42 % and 23 % for BC and OM respectively. In streets with larger ar (interval [1,1.5[), NOx concentrations are only 12 % higher than in the homogeneous version, and the PM10 concentrations are only 2.5 % higher.
Concerning the two cases in Copenhagen, CO and NOx concentrations present similar behaviour in comparison to the greater Paris case. For the JGTV street network, the increase is limited compared to the HCAB network – 3 % against 11.5 % respectively for CO. Wide streets (interval [0,0.5[) have higher concentrations of NO2 compared to the homogenous version of MUNICH, whereas for the other intervals of ar, they are lower. Thus, O3 concentrations are lower for wide streets and larger for the other intervals. This could be linked to the lack of volatile organic compounds in these two Copenhagen cases.
Table 1Statistical indicators of the influence of the aspect ratio on concentrations in MUNICH-hete-l1 for the street network in greater Paris. Indicators are presented in Appendix B.
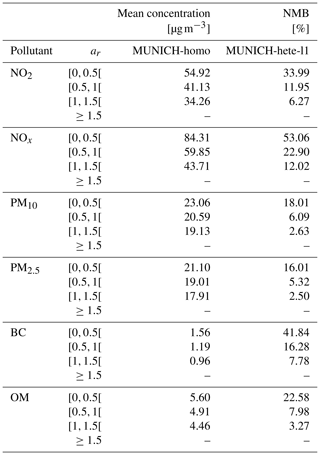
5.2 Sensitivity to the street network
The computation of inflow and outflow fluxes at intersections is performed by estimating the balance of fluxes entering and leaving the intersection from the different street segments attached to it. If this balance is not perfect, there are exchanges with the atmosphere above the intersection. When the total flux entering the intersection is higher than the one leaving it, the flow overload is directed to the atmosphere. When the total flux leaving the intersection is higher, a flux from the atmosphere to the intersection is considered. A further explanation is available in Kim et al. (2018, 2022).
Without a street network around the street segment of interest, the pollutant mass fluxes entering the street are determined from the background concentration. The contribution of the neighbouring streets is thus not taken into account, and concentrations of pollutants emitted in streets are expected to be lower than when there is a street network.
Table 2 and Appendix G present the influence of the neighbouring streets for the three cases of the study. It is quantified using the normalized mean error (NME) and NMB between the simulations without and with the neighbouring streets. As expected, without the street network, the concentrations are lower. The bias is between 12 % and 21 % for NO2, between 18 % and 27 % for NOx, and 14 % for BC. Biases are lower for OM and PM (2 %) because of the stronger influence of background concentrations for those compounds.
Table 2Statistical indicators of the influence of the street network on concentrations simulated with MUNICH-hete for the street segment of the Boulevard d'Alsace Lorraine with the monitoring station. Indicators are presented in Appendix B.
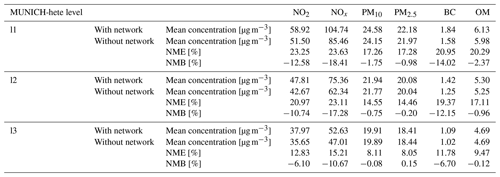
The street-network model MUNICH v2.0 has been modified to introduce concentration heterogeneities in the street and to better represent population exposure. To model the vertical gradients frequently observed, the streets were discretized with three levels, thus limiting the artificial dilution of emissions and concentrations. Based on a parameterization from OSPM, a ventilation zone is considered under specific conditions to represent horizontal heterogeneities. In order to test these developments, the heterogeneous version of MUNICH (MUNICH-hete) has been applied to two cases in Copenhagen, Denmark, with comparisons to OSPM, and to one case near Paris, France. Overall, MUNICH-hete improves the comparison to observations compared to the homogeneous version. The errors compared to observations are reduced by up to 20 % for NOx and up to 15 % for BC.
As expected, in MUNICH-hete, concentrations of compounds emitted by traffic (CO, NO2, NOx, PM10, BC, and OM) are higher at the bottom of the street than at the top. These increases can reach up to 60 % and 30 % for NO2 and PM10 respectively. The intermediate level, serving as a buffer, presents concentrations higher than or similar to the homogeneous version (MUNICH-homo). Finally, concentrations in the highest level, in direct contact with the atmosphere above the street, are the lowest of the street. For the Danish cases, the low NO2 concentrations observed in the lower levels could be related to the absence of volatile organic compounds in the model setup and the coarse vertical discretization limiting O3 transport deep into the street.
A sensitivity study of the influence of the street network on concentrations in the streets shows the importance of considering neighbouring streets in MUNICH. When no network is considered, concentrations in the street are lower due to the overestimated impact of the atmosphere above. At the bottom of the street, concentrations of NO2 and BC are reduced by up to 28 % and 14 % respectively without a network. PM and OM are less impacted, with a reduction of about 2 % due to a strong influence of non-traffic sources.
For the next step, the ventilation zone will be fully discretized vertically to facilitate the penetration of background concentrations into the bottom of the street. The horizontal exchange fluxes between the two zones will also be modelled. Deposition and resuspension processes that were not considered in this development will be added. The fluxes that are currently assumed to be vertically homogeneous will be discretized. Finally, chemistry of volatile organic compounds could be added in the Danish cases if the background concentrations and emissions are available.
The algorithm used in the heterogeneous version of MUNICH to compute the volumes of the recirculation and ventilation zones is based on the parameterization of OSPM (Berkowicz et al., 1997; Berkowicz, 2000b; Ottosen et al., 2015). This algorithm is applied at the beginning of each time step as the size of the recirculation zone is dependent on wind speed and direction. Figure A1 presents the shape of the recirculation zone and the associated parameters.
The first step is to compute the length of the vortex in the direction of the wind:
with H being the height of the street, and
with uroof being the wind speed at roof level.
The width of the trapeze base is the projection of Lvortex in the street:
with θ being the angle between the wind direction and the street orientation.
The width of the trapeze top is equal to half of the base:
Knowing these lengths and using algebraic considerations, the widths W1 and W2 can be calculated:
with .
The horizontal surfaces for vertical exchanges between the levels and with the concentrations above the street are calculated as follows:
with L being the street length.
The vertical surfaces for advection via intersections are determined with the following:
Finally, the volumes associated with each level of the recirculation zone are
In the current version of MUNICH-hete, it is assumed that traffic emissions are all affected to the recirculation zone. Therefore, its base Wbase has to be superior or equal to the street width W. If this is not the case, the recirculation zone is considered to fill the whole street volume.
When considered, the other widths are also limited by the street width:
For evaluation of the simulations compared to observations, the following statistical indicators are used. o and s represent the observed and the simulated concentrations respectively. The overbar represents the average.
-
Mean fractional error (MFE):
-
Mean fractional bias (MFB):
-
Factor of 2 (FAC2): fraction of data that satisfy .
For comparison of the simulations, the normalized mean bias (NMB) and normalized mean error (NME) are used. X represents concentrations, with X0 being the reference simulation and Xi being the compared simulation. The overbar represents the average.
-
Normalized mean bias:
-
Normalized mean error:
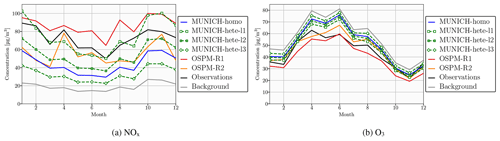
Figure C1Monthly averaged concentrations (in µg m−3) of NOx (a) and O3 (b) at HCAB monitoring station. The solid blue line represents the homogeneous version of MUNICH. The three dashed green lines represent the three levels of the heterogeneous version of MUNICH – the lowest level (l1) is shown with square markers, the intermediate level (l2) is shown with crossed markers, and the top level (l3) is shown with diamond markers. The solid red line represents the OSPM receptor that is close to the measurement station, and the solid orange line represents the second OSPM receptor located on the other side of the street. The observations are in solid black, and the background concentrations are in solid grey.
Table C1Statistical indicators of the evaluation of the hourly simulated concentrations compared to observations at HCAB monitoring station. Indicators are presented in Appendix B.
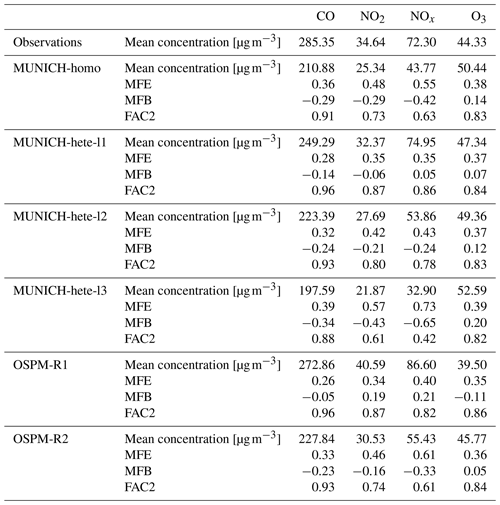
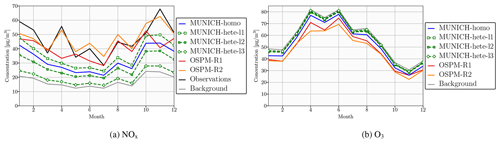
Figure D1Monthly averaged concentrations (in µg m−3) of NOx (a) and O3 (b) at JGTV monitoring station. The solid blue line represents the homogeneous version of MUNICH. The three dashed green lines represent the three levels of the heterogeneous version of MUNICH – the lowest level (l1) is shown with square markers, the intermediate level (l2) is shown with crossed markers, and the top level (l3) s shown with diamond markers. The solid orange line represents the OSPM receptor that is close to the measurement station, and the solid red line represents the second OSPM receptor located on the other side of the street. The observations are in solid black, and the background concentrations are in solid grey.
Table D1Statistical indicators of the evaluation of the hourly simulated concentrations compared to observations at JGTV monitoring station. Indicators are presented in Appendix B.
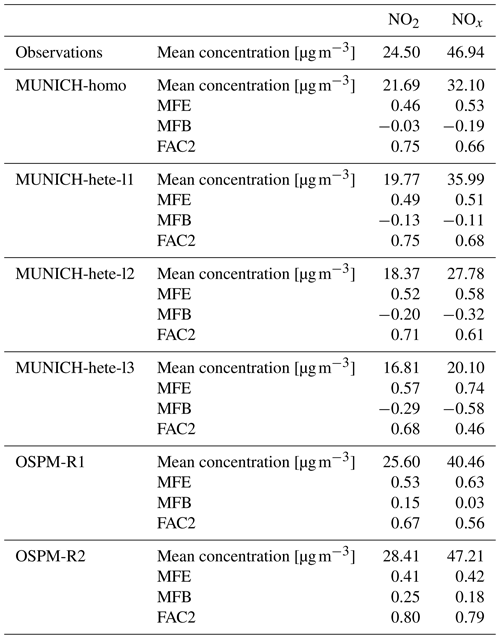
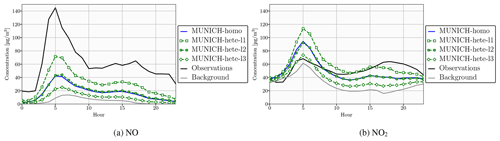
Figure E1Average daily profile of the concentrations of NO (a) and NO2 (b) over the simulation period at the Boulevard d'Alsace Lorraine monitoring station. The solid blue line represents the homogeneous version of MUNICH. The three dashed green lines represent the three levels of the heterogeneous version of MUNICH – the lowest level (l1) is shown with square markers, the intermediate level (l2) is shown with crossed markers, and the top level (l3) is shown with diamond markers. The observations are in solid black, and the background concentrations are in solid grey.
Table E1Statistical indicators of the evaluation of the hourly simulated concentrations to observations at the Boulevard d'Alsace Lorraine monitoring station. Indicators are presented in Appendix B.
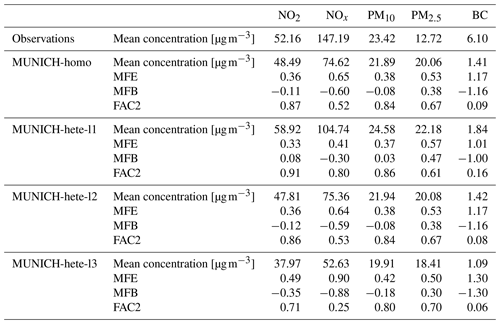
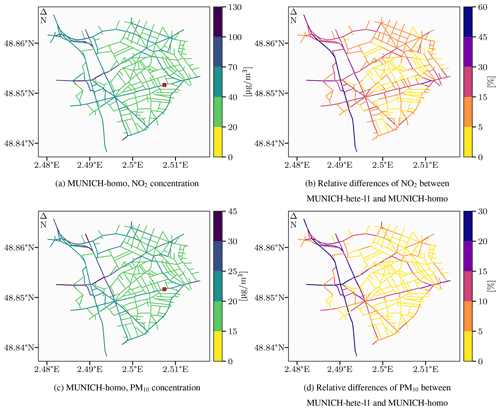
Figure E2NO2 and PM10 time-averaged concentrations (in µg m−3) for MUNICH-homo in the left panel (a, c) for the district of Le Perreux-sur-Marne. Relative differences (in %) between the first level of MUNICH-hete and MUNICH-homo for NO2 and PM10 in the right panel (b, d) for the district of Le Perreux-sur-Marne. A positive relative difference indicates higher concentrations for MUNICH-hete. The brown cross in panels (a) and (c) represents the position of the measurement station.
Table F1Statistical indicators of the influence of the aspect ratio on concentrations in MUNICH-hete-l1 for the street network around HCAB. Indicators are presented in Appendix B.
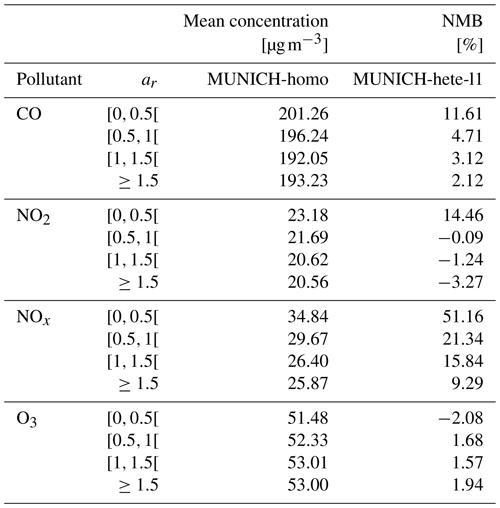
Table F2Statistical indicators of the influence of the aspect ratio on concentrations in MUNICH-hete-l1 for the street network around JGTV. Indicators are presented in Appendix B.
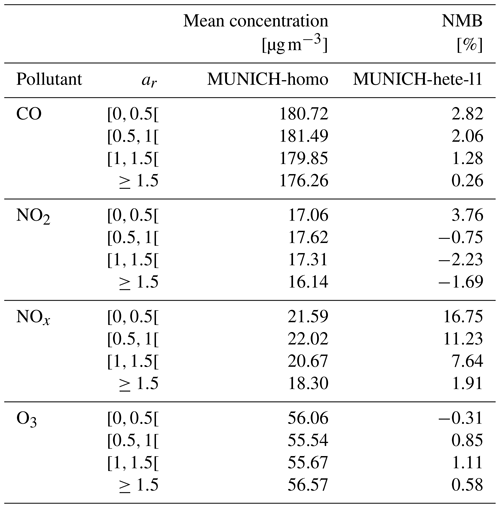
Table G1Statistical indicators of the influence of the street network on concentrations simulated with MUNICH-hete for the street segment HCAB with the monitoring station. Indicators are presented in Appendix B.
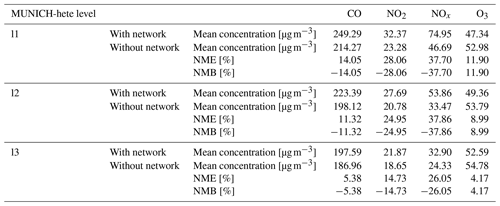
Table G2Statistical indicators of the influence of the street network on concentrations simulated with MUNICH-hete for the street segment JGTV with the monitoring station. Indicators are presented in Appendix B.
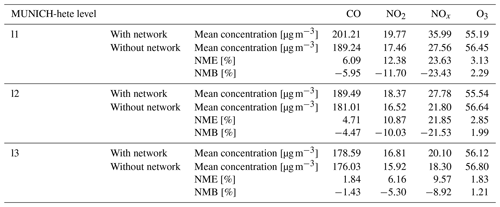
MUNICH-hete is available at https://doi.org/10.5281/zenodo.7778271 (Sarica et al., 2023a). The configuration files, the input data, and the scripts to generate the figures and statistics are available at Sarica et al. (2023a).
TS, AM, KS, and YR conceptualized the development of the study. TS, KS, and YK developed the software, and TS performed the simulations. MK and SSJ provided help for the simulations with OSPM and the data for the Danish cases. TS, KS, and YR performed the analysis. KS, YR, MK, and SSJ supervised the study. KS and CC were responsible for funding acquisition. TS and KS wrote the original draft, and all the authors reviewed and edited the final draft.
The contact author has declared that none of the authors has any competing interests.
Publisher's note: Copernicus Publications remains neutral with regard to jurisdictional claims in published maps and institutional affiliations.
This article is part of the special issue “Air quality research at street level – Part II (ACP/GMD inter-journal SI)”. It is not associated with a conference.
This paper was edited by Christoph Knote and reviewed by two anonymous referees.
Amato, F., Pérez, N., López, M., Ripoll, A., Alastuey, A., Pandolfi, M., Karanasiou, A., Salmatonidis, A., Padoan, E., Frasca, D., Marcoccia, M., Viana, M., Moreno, T., Reche, C., Martins, V., Brines, M., Minguillón, M., Ealo, M., Rivas, I., van Drooge, B., Benavides, J., Craviotto, J., and Querol, X.: Vertical and horizontal fall-off of black carbon and NO2 within urban blocks, Sci. Total Environ., 686, 236–245, https://doi.org/10.1016/j.scitotenv.2019.05.434, 2019. a
Archambeau, F., Méchitoua, N., and Sakiz, M.: Code Saturne: A Finite Volume Code for the computation of turbulent incompressible flows – Industrial Applications, International Journal on Finite Volumes, 1, https://hal.science/hal-01115371 (last access: 12 September 2023), 2004. a
Atkinson, R.: Atmospheric chemistry of VOCs and NOx, Atmos. Environ., 34, 2063–2101, https://doi.org/10.1016/S1352-2310(99)00460-4, 2000. a
Benavides, J., Snyder, M., Guevara, M., Soret, A., Pérez García-Pando, C., Amato, F., Querol, X., and Jorba, O.: CALIOPE-Urban v1.0: coupling R-LINE with a mesoscale air quality modelling system for urban air quality forecasts over Barcelona city (Spain), Geosci. Model Dev., 12, 2811–2835, https://doi.org/10.5194/gmd-12-2811-2019, 2019. a
Berkowicz, R.: A simple model for urban background pollution, Environ. Monit. Assess., 65, 259–267, https://doi.org/10.1023/a:1006466025186, 2000a. a
Berkowicz, R.: OSPM – a parameterised street pollution model, Environ. Monit. Assess., 65, 323–331, https://doi.org/10.1023/A:1006448321977, 2000b. a, b, c, d, e, f
Berkowicz, R., Hertel, O., Larsen, S., Sørensen, N., and Nielsen, M.: Modelling traffic pollution in streets, https://backend.orbit.dtu.dk/ws/portalfiles/portal/128001317/Modelling_traffic_pollution_in_streets.pdf (last access: 13 February 2023), 1997. a, b, c, d
Cimorelli, A., Perry, S., Venkatram, A., Weil, J., Paine, R., Wilson, R., Lee, R., Peters, W., Brode, R., and Paumier, J.: AERMOD: description of model formulation, US Environmental Protection Agency, Tech. rep., EPA-454/R-03-004, https://gaftp.epa.gov/Air/aqmg/SCRAM/models/preferred/aermod/aermod_mfd_454-R-03-004.pdf (last access: 12 September 2023), 2004. a
Coceal, O. and Belcher, S.: A canopy model of mean winds through urban areas, Q. J. Roy. Meteor. Soc., 130, 1349–1372, https://doi.org/10.1256/qj.03.40, 2004. a
Collett, R. S. and Oduyemi, K.: Air quality modelling: a technical review of mathematical approaches, Meteorol. Appl., 4, 235–246, https://doi.org/10.1017/S1350482797000455, 1997. a
Conti, G. O., Heibati, B., Kloog, I., Fiore, M., and Ferrante, M.: A review of AirQ Models and their applications for forecasting the air pollution health outcomes, Environ. Sci. Pollut. R., 24, 6426–6445, https://doi.org/10.1007/s11356-016-8180-1, 2017. a
Dai, Y., Cai, X., Zhong, J., and MacKenzie, A. R.: Modelling chemistry and transport in urban street canyons: Comparing offline multi-box models with large-eddy simulation, Atmos. Environ., 264, 118709, https://doi.org/10.1016/j.atmosenv.2021.118709, 2021. a
de la Paz, D., Borge, R., Vedrenne, M., Lumbreras, J., Amato, F., Karanasiou, A., Boldo, E., and Moreno, T.: Implementation of road dust resuspension in air quality simulations of particulate matter in Madrid (Spain), Front. Environ. Sci., 3, https://doi.org/10.3389/fenvs.2015.00072, 2015. a
El-Harbawi, M.: Air quality modelling, simulation, and computational methods: a review, Environ. Rev., 21, 149–179, https://doi.org/10.1139/er-2012-0056, 2013. a
Falasca, S. and Curci, G.: High-resolution air quality modeling: Sensitivity tests to horizontal resolution and urban canopy with WRF-CHIMERE, Atmos. Environ., 187, 241–254, https://doi.org/10.1016/j.atmosenv.2018.05.048, 2018. a
Fuller, R., Landrigan, P. J., Balakrishnan, K., Bathan, G., Bose-O'Reilly, S., Brauer, M., Caravanos, J., Chiles, T., Cohen, A., Corra, L., Cropper, M., Ferraro, G., Hanna, J., Hanrahan, D., Hu, H., Hunter, D., Janata, G., Kupka, R., Lanphear, B., Lichtveld, M., Martin, K., Mustapha, A., Sanchez-Triana, E., Sandilya, K., Schaefli, L., Shaw, J., Seddon, J., Suk, W., Téllez-Rojo, M. M., and Yan, C.: Pollution and health: a progress update, Lancet Planet. Health, 6, e535–e547, https://doi.org/10.1016/S2542-5196(22)00090-0, 2022. a
Gao, Z., Bresson, R., Qu, Y., Milliez, M., de Munck, C., and Carissimo, B.: High resolution unsteady RANS simulation of wind, thermal effects and pollution dispersion for studying urban renewal scenarios in a neighborhood of Toulouse, Urban Clim., 23, 114–130, https://doi.org/10.1016/j.uclim.2016.11.002, 2018. a
Hood, C., Stocker, J., Seaton, M., Johnson, K., O'Neill, J., Thorne, L., and Carruthers, D.: Comprehensive evaluation of an advanced street canyon air pollution model, J. Air Waste Manage., 71, 247–267, https://doi.org/10.1080/10962247.2020.1803158, 2021. a
Jensen, S. S., Ketzel, M., Brandt, J., Becker, T., Plejdrup, M., Winther, M., Ellermann, T., Christensen, J. H., Nielsen, O.-K., Hertel, O., and Fuglsang, M. W.: 04 – Air Quality at Your Street – Public Digital Map of Air Quality in Denmark, in: Proceedings, COST Association, Brussels, Belgium, Sixth Scientific Meeting EuNetAir 5–7 October 2016, Academy of Sciences, Prague, Czech Republic, 14–17, https://doi.org/10.5162/6eunetair2016/04, 2016. a
Karl, M., Walker, S.-E., Solberg, S., and Ramacher, M. O. P.: The Eulerian urban dispersion model EPISODE – Part 2: Extensions to the source dispersion and photochemistry for EPISODE–CityChem v1.2 and its application to the city of Hamburg, Geosci. Model Dev., 12, 3357–3399, https://doi.org/10.5194/gmd-12-3357-2019, 2019. a
Khan, S. and Quamrul, H.: Review of developments in air quality modelling and air quality dispersion models, J. Environ. Eng. Sci., 16, 1–10, https://doi.org/10.1680/jenes.20.00004, 2021. a
Kim, Y., Wu, Y., Seigneur, C., and Roustan, Y.: Multi-scale modeling of urban air pollution: development and application of a Street-in-Grid model (v1.0) by coupling MUNICH (v1.0) and Polair3D (v1.8.1), Geosci. Model Dev., 11, 611–629, https://doi.org/10.5194/gmd-11-611-2018, 2018. a, b, c, d
Kim, Y., Lugon, L., Maison, A., Sarica, T., Roustan, Y., Valari, M., Zhang, Y., André, M., and Sartelet, K.: MUNICH v2.0: a street-network model coupled with SSH-aerosol (v1.2) for multi-pollutant modelling, Geosci. Model Dev., 15, 7371–7396, https://doi.org/10.5194/gmd-15-7371-2022, 2022. a, b, c, d, e, f, g
Kwak, K.-H. and Baik, J.-J.: A CFD modeling study of the impacts of NOx and VOC emissions on reactive pollutant dispersion in and above a street canyon, Atmos. Environ., 46, 71–80, https://doi.org/10.1016/j.atmosenv.2011.10.024, 2012. a
Landrigan, P. J., Fuller, R., Acosta, N. J. R., Adeyi, O., Arnold, R., Basu, N. N., Baldé, A. B., Bertollini, R., Bose-O'Reilly, S., Boufford, J. I., Breysse, P. N., Chiles, T., Mahidol, C., Coll-Seck, A. M., Cropper, M. L., Fobil, J., Fuster, V., Greenstone, M., Haines, A., Hanrahan, D., Hunter, D., Khare, M., Krupnick, A., Lanphear, B., Lohani, B., Martin, K., Mathiasen, K. V., McTeer, M. A., Murray, C. J. L., Ndahimananjara, J. D., Perera, F., Potočnik, J., Preker, A. S., Ramesh, J., Rockström, J., Salinas, C., Samson, L. D., Sandilya, K., Sly, P. D., Smith, K. R., Steiner, A., Stewart, R. B., Suk, W. A., van Schayck, O. C. P., Yadama, G. N., Yumkella, K., and Zhong, M.: The Lancet Commission on pollution and health, Lancet, 391, 462–512, https://doi.org/10.1016/S0140-6736(17)32345-0, 2018. a
Lateb, M., Meroney, R., Yataghene, M., Fellouah, H., Saleh, F., and Boufadel, M.: On the use of numerical modelling for near-field pollutant dispersion in urban environments – A review, Environ. Pollut., 208, 271–283, https://doi.org/10.1016/j.envpol.2015.07.039, 2016. a
Leighton, P. A.: Photochemistry of air pollution, Academic Press, New York, ISBN 9780323156455, 1961. a
Liang, M., Chao, Y., Tu, Y., and Xu, T.: Vehicle Pollutant Dispersion in the Urban Atmospheric Environment: A Review of Mechanism, Modeling, and Application, Atmosphere, 14, 279, https://doi.org/10.3390/atmos14020279, 2023. a
Lin, C., Wang, Y., Ooka, R., Flageul, C., Kim, Y., Kikumoto, H., Wang, Z., and Sartelet, K.: Modeling of street-scale pollutant dispersion by coupled simulation of chemical reaction, aerosol dynamics, and CFD, Atmos. Chem. Phys., 23, 1421–1436, https://doi.org/10.5194/acp-23-1421-2023, 2023. a, b, c
Lugon, L., Sartelet, K., Kim, Y., Vigneron, J., and Chrétien, O.: Nonstationary modeling of NO2, NO and NOx in Paris using the Street-in-Grid model: coupling local and regional scales with a two-way dynamic approach, Atmos. Chem. Phys., 20, 7717–7740, https://doi.org/10.5194/acp-20-7717-2020, 2020. a, b, c
Lugon, L., Sartelet, K., Kim, Y., Vigneron, J., and Chrétien, O.: Simulation of primary and secondary particles in the streets of Paris using MUNICH, Faraday Discuss., 226, 432–456, https://doi.org/10.1039/D0FD00092B, 2021a. a, b
Lugon, L., Vigneron, J., Debert, C., Chrétien, O., and Sartelet, K.: Black carbon modeling in urban areas: investigating the influence of resuspension and non-exhaust emissions in streets using the Street-in-Grid model for inert particles (SinG-inert), Geosci. Model Dev., 14, 7001–7019, https://doi.org/10.5194/gmd-14-7001-2021, 2021b. a, b
Maison, A., Flageul, C., Carissimo, B., Tuzet, A., and Sartelet, K.: Parametrization of Horizontal and Vertical Transfers for the Street-Network Model MUNICH Using the CFD Model Code_Saturne, Atmosphere, 13, 527, https://doi.org/10.3390/atmos13040527, 2022. a, b, c, d, e, f
Mallet, V., Quélo, D., Sportisse, B., Ahmed de Biasi, M., Debry, É., Korsakissok, I., Wu, L., Roustan, Y., Sartelet, K., Tombette, M., and Foudhil, H.: Technical Note: The air quality modeling system Polyphemus, Atmos. Chem. Phys., 7, 5479–5487, https://doi.org/10.5194/acp-7-5479-2007, 2007. a
McHugh, C., Carruthers, D., and Edmunds, H.: ADMS and ADMS–Urban, Int. J. Environ. Pollut., 8, 438–440, https://doi.org/10.1504/IJEP.1997.028193, 1997. a
Menut, L., Bessagnet, B., Briant, R., Cholakian, A., Couvidat, F., Mailler, S., Pennel, R., Siour, G., Tuccella, P., Turquety, S., and Valari, M.: The CHIMERE v2020r1 online chemistry-transport model, Geosci. Model Dev., 14, 6781–6811, https://doi.org/10.5194/gmd-14-6781-2021, 2021. a
Olesen, H., Ketzel, M., Jensen, S., Løfstrøm, P., Im, U., and Becker, T.: User Guide to OML-Highway. A tool for air pollution assessments along highways., Tech. rep., Aarhus University, DCE – Danish Centre for Environment and Energy, https://dce2.au.dk/pub/TR59.pdf (last access: 14 February 2023), 2015. a
Ottosen, T.-B., Kakosimos, K. E., Johansson, C., Hertel, O., Brandt, J., Skov, H., Berkowicz, R., Ellermann, T., Jensen, S. S., and Ketzel, M.: Analysis of the impact of inhomogeneous emissions in the Operational Street Pollution Model (OSPM), Geosci. Model Dev., 8, 3231–3245, https://doi.org/10.5194/gmd-8-3231-2015, 2015. a, b, c
Rood, A. S.: Performance evaluation of AERMOD, CALPUFF, and legacy air dispersion models using the Winter Validation Tracer Study dataset, Atmos. Environ., 89, 707–720, https://doi.org/10.1016/j.atmosenv.2014.02.054, 2014. a
Sanchez, B., Santiago, J.-L., Martilli, A., Palacios, M., and Kirchner, F.: CFD modeling of reactive pollutant dispersion in simplified urban configurations with different chemical mechanisms, Atmos. Chem. Phys., 16, 12143–12157, https://doi.org/10.5194/acp-16-12143-2016, 2016. a
Sarica, T., Sartelet, K., Roustan, Y., Kim, Y., Lugon, L., André, M., Marques, B., D'Anna, B., Chaillou, C., and Larrieu, C.: Modelling Pollutant Concentrations in Streets: A Sensitivity Analysis to Asphalt and Traffic Related Emissions, in: Air Pollution Modeling and its Application XXVIII, edited by: Mensink, C. and Jorba, O., Springer International Publishing, Cham, 287–293, https://doi.org/10.1007/978-3-031-12786-1_39, 2022. a
Sarica, T., Maison, A., Roustan, Y., Ketzel, M., Jensen, S. S., Kim, Y., and Sartelet, K.: The Model of Urban Network of Intersecting Canyons and Highways (MUNICH), Zenodo [code and data set], https://doi.org/10.5281/zenodo.7778271, 2023a. a, b
Sarica, T., Sartelet, K., Roustan, Y., Kim, Y., Lugon, L., Marques, B., D'Anna, B., Chaillou, C., and Larrieu, C.: Sensitivity of pollutant concentrations in urban streets to asphalt and traffic-related emissions, Environ. Pollut., 332, 121955, https://doi.org/10.1016/j.envpol.2023.121955, 2023b. a, b
Sartelet, K., Zhu, S., Moukhtar, S., André, M., André, J., Gros, V., Favez, O., Brasseur, A., and Redaelli, M.: Emission of intermediate, semi and low volatile organic compounds from traffic and their impact on secondary organic aerosol concentrations over Greater Paris, Atmos. Environ., 180, 126–137, https://doi.org/10.1016/j.atmosenv.2018.02.031, 2018. a
Sartelet, K., Couvidat, F., Wang, Z., Flageul, C., and Kim, Y.: SSH-Aerosol v1.1: A Modular Box Model to Simulate the Evolution of Primary and Secondary Aerosols, Atmosphere, 11, 525, https://doi.org/10.3390/atmos11050525, 2020. a, b
Skamarock, W., Klemp, J., Dudhia, J., Gill, D., Barker, D., Wang, W., Huang, X.-Y., and Duda, M.: A Description of the Advanced Research WRF Version 3, Tech. rep., UCAR/NCAR, https://doi.org/10.5065/D68S4MVH, 2008. a
Solazzo, E., Cai, X., and Vardoulakis, S.: Modelling wind flow and vehicle-induced turbulence in urban streets, Atmos. Environ., 42, 4918–4931, https://doi.org/10.1016/j.atmosenv.2008.02.032, 2008. a
Soulhac, L., Garbero, V., Salizzoni, P., Mejean, P., and Perkins, R.: Flow and dispersion in street intersections, Atmos. Environ., 43, 2981–2996, https://doi.org/10.1016/j.atmosenv.2009.02.061, 2009. a, b, c
Soulhac, L., Salizzoni, P., Cierco, F.-X., and Perkins, R.: The model SIRANE for atmospheric urban pollutant dispersion; part I, presentation of the model, Atmos. Environ., 45, 7379–7395, https://doi.org/10.1016/j.atmosenv.2011.07.008, 2011. a
Soulhac, L., Fellini, S., Nguyen, C. V., and Salizzoni, P.: Evaluation of Photostationary and Non-Photostationary Operational Models for NOX Pollution in a Street Canyon, Atmos. Environ., 297, 119589, https://doi.org/10.1016/j.atmosenv.2023.119589, 2023. a
United Nations: World Urbanization Prospects: The 2018 Revision (ST/ESA/SER.A/420), Tech. rep., United Nations, https://population.un.org/wup/Publications/Files/WUP2018-Report.pdf (last access: 12 September 2023), 2019. a
Vardoulakis, S., Fisher, B., Pericleous, K., and Gonzalez-Flesca, N.: Modelling air quality in street canyons: a review, Atmos. Environ., 37, 155–182, https://doi.org/10.1016/S1352-2310(02)00857-9, 2003. a, b
Vardoulakis, S., Solazzo, E., and Lumbreras, J.: Intra-urban and street scale variability of BTEX, NO2 and O3 in Birmingham, UK: Implications for exposure assessment, Atmos. Environ., 45, 5069–5078, https://doi.org/10.1016/j.atmosenv.2011.06.038, 2011. a
Wang, W.: Analytically modelling mean wind and stress profiles in canopies, Bound.-Lay. Meteorol., 151, 239–256, https://doi.org/10.1007/s10546-013-9899-6, 2014. a
Wolf, T., Pettersson, L. H., and Esau, I.: A very high-resolution assessment and modelling of urban air quality, Atmos. Chem. Phys., 20, 625–647, https://doi.org/10.5194/acp-20-625-2020, 2020. a
Wong, D. C., Pleim, J., Mathur, R., Binkowski, F., Otte, T., Gilliam, R., Pouliot, G., Xiu, A., Young, J. O., and Kang, D.: WRF-CMAQ two-way coupled system with aerosol feedback: software development and preliminary results, Geosci. Model Dev., 5, 299–312, https://doi.org/10.5194/gmd-5-299-2012, 2012. a
Xie, S., Zhang, Y., Qi, L., and Tang, X.: Spatial distribution of traffic-related pollutant concentrations in street canyons, Atmos. Environ., 37, 3213–3224, https://doi.org/10.1016/S1352-2310(03)00321-2, 2003. a
Zhong, J., Cai, X.-M., and Bloss, W. J.: Large eddy simulation of reactive pollutants in a deep urban street canyon: Coupling dynamics with O3-NOx-VOC chemistry, Environ. Pollut., 224, 171–184, https://doi.org/10.1016/j.envpol.2017.01.076, 2017. a
- Abstract
- Introduction
- Model description
- Application to street networks in Copenhagen with comparison to OSPM
- Application to a street network in greater Paris
- Sensitivity analysis
- Conclusions
- Appendix A: Volumes of the recirculation and ventilation zones
- Appendix B: Statistical indicators
- Appendix C: Additional information for HCAB
- Appendix D: Additional information for JGTV
- Appendix E: Additional information for the district of Le Perreux-sur-Marne
- Appendix F: Additional information for the influence of the aspect ratio
- Appendix G: Additional information for the sensitivity to street network
- Code and data availability
- Author contributions
- Competing interests
- Disclaimer
- Special issue statement
- Review statement
- References
- Abstract
- Introduction
- Model description
- Application to street networks in Copenhagen with comparison to OSPM
- Application to a street network in greater Paris
- Sensitivity analysis
- Conclusions
- Appendix A: Volumes of the recirculation and ventilation zones
- Appendix B: Statistical indicators
- Appendix C: Additional information for HCAB
- Appendix D: Additional information for JGTV
- Appendix E: Additional information for the district of Le Perreux-sur-Marne
- Appendix F: Additional information for the influence of the aspect ratio
- Appendix G: Additional information for the sensitivity to street network
- Code and data availability
- Author contributions
- Competing interests
- Disclaimer
- Special issue statement
- Review statement
- References