the Creative Commons Attribution 4.0 License.
the Creative Commons Attribution 4.0 License.
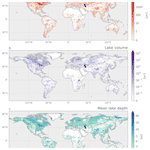
A framework for ensemble modelling of climate change impacts on lakes worldwide: the ISIMIP Lake Sector
Malgorzata Golub
Rafael Marcé
Don Pierson
Inne Vanderkelen
Daniel Mercado-Bettin
R. Iestyn Woolway
Luke Grant
Eleanor Jennings
Benjamin M. Kraemer
Jacob Schewe
Fang Zhao
Katja Frieler
Matthias Mengel
Vasiliy Y. Bogomolov
Damien Bouffard
Marianne Côté
Raoul-Marie Couture
Andrey V. Debolskiy
Bram Droppers
Gideon Gal
Mingyang Guo
Annette B. G. Janssen
Georgiy Kirillin
Robert Ladwig
Madeline Magee
Tadhg Moore
Marjorie Perroud
Sebastiano Piccolroaz
Love Raaman Vinnaa
Martin Schmid
Tom Shatwell
Victor M. Stepanenko
Zeli Tan
Bronwyn Woodward
Huaxia Yao
Rita Adrian
Mathew Allan
Orlane Anneville
Lauri Arvola
Karen Atkins
Leon Boegman
Cayelan Carey
Kyle Christianson
Elvira de Eyto
Curtis DeGasperi
Maria Grechushnikova
Josef Hejzlar
Klaus Joehnk
Ian D. Jones
Alo Laas
Eleanor B. Mackay
Ivan Mammarella
Hampus Markensten
Chris McBride
Deniz Özkundakci
Miguel Potes
Karsten Rinke
Dale Robertson
James A. Rusak
Rui Salgado
Leon van der Linden
Piet Verburg
Danielle Wain
Nicole K. Ward
Sabine Wollrab
Galina Zdorovennova
Empirical evidence demonstrates that lakes and reservoirs are warming across the globe. Consequently, there is an increased need to project future changes in lake thermal structure and resulting changes in lake biogeochemistry in order to plan for the likely impacts. Previous studies of the impacts of climate change on lakes have often relied on a single model forced with limited scenario-driven projections of future climate for a relatively small number of lakes. As a result, our understanding of the effects of climate change on lakes is fragmentary, based on scattered studies using different data sources and modelling protocols, and mainly focused on individual lakes or lake regions. This has precluded identification of the main impacts of climate change on lakes at global and regional scales and has likely contributed to the lack of lake water quality considerations in policy-relevant documents, such as the Assessment Reports of the Intergovernmental Panel on Climate Change (IPCC). Here, we describe a simulation protocol developed by the Lake Sector of the Inter-Sectoral Impact Model Intercomparison Project (ISIMIP) for simulating climate change impacts on lakes using an ensemble of lake models and climate change scenarios for ISIMIP phases 2 and 3. The protocol prescribes lake simulations driven by climate forcing from gridded observations and different Earth system models under various representative greenhouse gas concentration pathways (RCPs), all consistently bias-corrected on a 0.5∘ × 0.5∘ global grid. In ISIMIP phase 2, 11 lake models were forced with these data to project the thermal structure of 62 well-studied lakes where data were available for calibration under historical conditions, and using uncalibrated models for 17 500 lakes defined for all global grid cells containing lakes. In ISIMIP phase 3, this approach was expanded to consider more lakes, more models, and more processes. The ISIMIP Lake Sector is the largest international effort to project future water temperature, thermal structure, and ice phenology of lakes at local and global scales and paves the way for future simulations of the impacts of climate change on water quality and biogeochemistry in lakes.
Please read the corrigendum first before continuing.
-
Notice on corrigendum
The requested paper has a corresponding corrigendum published. Please read the corrigendum first before downloading the article.
-
Article
(2225 KB)
- Corrigendum
-
Supplement
(368 KB)
-
The requested paper has a corresponding corrigendum published. Please read the corrigendum first before downloading the article.
- Article
(2225 KB) - Full-text XML
- Corrigendum
-
Supplement
(368 KB) - BibTeX
- EndNote
There are over 117 million lakes on Earth covering only 3 % of the land surface (Verpoorter et al., 2014), yet freshwater ecosystems in general host 10 % of Earth's known animal species (Reid et al., 2019). Many lakes provide ecosystem services to their local communities including drinking water, fisheries, and transportation, and the number of services provided by lakes has been shown to decrease with deteriorating lake health (Janssen et al., 2021). In addition, lakes are effective as local indicators for both environmental changes at the watershed scale and as “sentinels of climate change” in that they buffer synoptic-scale variability but incorporate information on seasonal cycling, inter-annual variability, and long-term changes in lower atmospheric conditions. Therefore, studying lake impacts across scales is an important field of research for disentangling the global impacts of climate change from the other anthropogenic pressures that climate change interacts with. However, estimates of historical and future lake responses to climate change have, until recently, largely been carried out as site-specific studies with different goals, data, and modelling protocols, which complicates the generalization of simulated impacts at regional and global scales (Settele et al., 2015; IPCC, 2018).
Historical records show that lakes are already responding to climatic change by warming (O'Reilly et al., 2015; Pilla et al., 2020; Gal et al., 2020; Jane et al., 2021; Woolway et al., 2019b), experiencing declining ice cover (Weyhenmeyer et al., 2011; Sharma et al., 2019), shifting thermal habitats (Kraemer et al., 2021), changing mixing regimes (Kraemer et al., 2015; Woolway and Merchant, 2019), and decreasing oxygen levels (Jane et al., 2021). However, long-term monitoring data remain limited to a relatively small number of well-studied lakes, while time series from automated high sampling frequency monitoring buoys are still generally short (Marcé et al., 2016). The existing empirical evidence needs to be combined with lake models to understand how lakes have responded to historical changes (Moras et al., 2019) and how they could behave under future climatic change. Numerous numerical models have been used to assess climate change impacts on lake ecosystems (Schwefel et al., 2016; Hansen et al., 2017; Wang et al., 2018; Zwart et al., 2019; Ayala et al., 2020; Piccolroaz et al., 2020); however, the climate change impacts synthesized in the recent IPCC reports remain limited to a small number of lakes, regions, or specific impact models or climate change scenarios (Kraemer et al., 2015; IPCC, 2018). Multi-model ensemble simulations are increasingly used to obtain more robust assessments of freshwater ecosystem responses to climate change, but so far, only a few lakes have been assessed following a multi-model approach (Perroud et al., 2009; Trolle et al., 2014; Stepanenko et al., 2010, 2013, 2014; Thiery et al., 2014a; Gal et al., 2020; Guseva et al., 2020). To date, no multi-model ensembles have been used over a broad range of lakes to make either hindcast or future climate simulations, which would allow evaluation of the variability in model output related to different model formulations or parametrizations.
The ISIMIP framework (http://www.isimip.org, last access: 23 May 2022) provides a set of climate and socioeconomic forcing data to make consistent historical hindcast and future climate impact projections and evaluate impacts in response to policy-relevant climate change scenarios. ISIMIP is organized in different sectors ranging from hydrology to human health, all of which make use of common and openly provided input data. As part of ISIMIP, we initiated the Lake Sector and developed a lake model simulation protocol to assess climate change impacts on lakes and to provide robust scientific evidence of historical and potential future lake ecosystem changes. To this end, we used two complementary strategies: (i) a local strategy to simulate well-studied lakes where sufficient data were available for lake-specific model parameterization and calibration and (ii) a global strategy that applied lake models to simulate generic lakes for each lake-containing grid cell of the ISIMIP global grid. The simulation set-up described by the protocol enables impacts of climate change on lake characteristics to be projected and attributed. The protocol incorporates uncertainties from the differences in the global climate models (GCMs) providing forcing data, the differences in lake impact model structure, and lake geographical and ecosystem characteristics. The standardized output produced by the hydrodynamic lake models includes vertical profiles of water temperature and metrics describing thermal and ice conditions at daily to annual timescales. This multi-model ensemble provides a systematic overview of plausible future responses of lake ecosystems to a warming climate at an unprecedented geographical coverage. The forcing data from the GCM ensemble further enable the quantification of lake responses to changes in meteorological variables other than air temperature, e.g. from wind velocity or cloud cover (Woolway et al., 2019a), including their potential interactions with increases in air temperature. Completed and ongoing thermal regime simulations will provide the foundation for the modelling of water quality, greenhouse gas emissions, algal blooms, and water level fluctuations to be addressed in future ISIMIP rounds.
Here, we describe the protocol for the global- and local-scale intercomparison of lake model simulations completed for the second phase of ISIMIP (ISIMIP2), as well as the extensions to this protocol that have been implemented for the new phase three simulations (ISIMIP3). The evolution of the modelling protocol from ISIMIP2 to ISIMIP3, and the rationale for these advancements, will be described in individual sections related to the experimental set-up of the Lake Sector, such as changes to lake model forcing datasets and background information on lake mapping. First, we provide an overview of the climate data and climate change scenarios available through ISIMIP that were used as forcing data for lake impact models. Next, we explain the rationale behind the ISIMIP Lake Sector, give an overview of the impact simulations and briefly describe the lake models used at local and global spatial domains. Finally, we highlight some examples of the first lake impact model simulations from the ISIMIP2 simulation phase and illustrate how the simulations allowed us to quantify sources of uncertainty in future projections.
The Lake Sector simulations are the first ensemble projections using a consistent modelling framework to evaluate the impact of climate change on lakes, thereby informing researchers, policymakers, and water managers and enabling comparisons of impacts with other sectors participating in ISIMIP. Given the importance of lake thermal structure in regulating lake processes, ISIMIP2 simulation results from the Lake Sector provide a fundamental contribution to lake specific policy recommendations in reports from organizations like the IPCC, the Intergovernmental Science-Policy Platform on Biodiversity and Ecosystem Services (IPBES), and the UN Environment Programme World Water Quality Alliance (UNEP-WWQA). Furthermore, the work described here paves the way for lake water quality simulations playing a fundamental role in international policy.
The ISIMIP2 simulation framework was divided into two simulation rounds: ISIMIP2a and ISIMIP2b. ISIMIP2a focused on historical simulations, which were forced with gridded climate reanalysis products based on observed meteorological data. The ISIMIP2b simulation round focussed on quantifying the impacts of GCM-derived historical and CMIP5 (Coupled Model Intercomparison Project Phase 5) projected climate change relative to a pre-industrial control (Frieler et al., 2017). For the Lake Sector, ISIMIP2a simulations centred around the calibration of lake models at the local scale that were used for the future climate ensemble simulations in ISIMIP2b. These simulations also evaluated the lake models' ability to simulate observed climate variability and extremes at both local and global scales. The subsequent simulation phase, ISIMIP3a-b, will build on the latest gridded observations and CMIP6 global climate model simulations to provide meteorological forcing and improve the representation of non-climatic input data, such as land use and a dynamic global lake mask, which will be used to produce a new generation of lake model simulations.
The inception of the Lake Sector and the gathering of the first collection of models, modelling teams, and lake data providers greatly benefited from contributions from two global collaborative projects. First, the Lake Model Intercomparison Project (LakeMIP, https://www.unige.ch/climate/lakemip, last access: 23 May 2022) started in 2008 (Stepanenko et al., 2010) with the objective of comparing the thermodynamic regime of lakes (including lake-atmosphere interactions) in a wide range of climatic conditions and mixing regimes as simulated by several one-dimensional lake models (Perroud et al., 2009; Stepanenko et al., 2013, 2014; Thiery et al., 2014a; Guseva et al., 2020). Second, the Global Lake Ecological Observatory Network (GLEON, https://gleon.org, last access: 23 May 2022, Weathers et al., 2013) started in 2005, with the aim of sharing and interpreting lake data to understand, predict, and communicate the role and responses of lakes in a changing global environment.
The simulations followed the network-wide simulation protocols for ISIMIP 2a–b (Frieler et al., 2017; Schewe et al., 2019) and ISIMIP3a–b (see https://www.isimip.org/protocol/, last access: 23 May 2022 for an overview). Here, we describe the rationale and specifics of simulations in the Lake Sector. Lake model simulations were conducted in two spatial domains: local and global (Fig. 1). Climate change impacts were simulated after model calibration for specific lakes in the local domain (see Sect. 3.1), and for “representative” lakes without calibration in the global domain for each lake-containing grid cell in the ISIMIP global grid (see Sect. 3.2). These two complementary spatial domains balanced the need for site-specific information and the need for a global assessment of climate change impacts on lakes. All temperature simulations were conducted under the assumption that the water level of the lakes remained constant and, therefore, the lakes were decoupled from their watersheds. This assumption allowed us to evaluate lake thermal structure based on meteorological forcing data only and was judged acceptable for the existing phases of ISIMIP (see Sect. 3.5.3). It is anticipated that subsequent simulations, especially those that will evaluate changes in lake biogeochemistry, will abandon this simplification and contribute to cross-sectorial collaborations. On a regional scale, coupled hydrologic and lake model simulations to evaluate changes in lake water level (Hanson et al., 2021) and biogeochemistry (Zwart et al., 2019) for 3692 lakes in northern Wisconsin and Michigan have already been developed. Such regional studies can serve as a model for future cross-sectorial simulation in the ISIMIP.
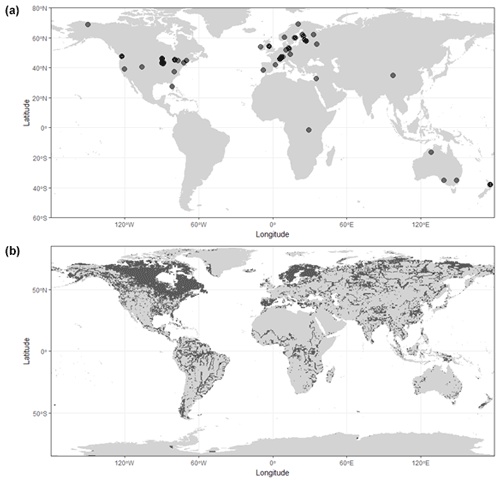
Figure 1Map of lakes at local (a) and global (b) scales participating in the ISIMIP2a/b Lake Sector. In panel (a), the local lake sites are visualized through semi-transparent markers; hence darker markers highlight locations where several lakes are located close to each other.
3.1 Case-study lakes in the local domain
Lakes in the local domain had sufficient information to allow the lake models to be parameterized using individual lake bathymetry and to be calibrated against measured water temperature profiles. Consequently, the local lake dataset was a unique resource for testing and evaluating lake model performance.
For ISIMIP2, bathymetric data and historical data on water temperature from 52 lakes and 10 reservoirs (Fig. 1a, Table S1) were shared among participating modelling teams. Since reservoirs were treated like regular lakes in the simulations, all waterbodies are hereafter called “lakes” (see Sect. 4.3). The geographical distribution of the lakes encompassed a gradient of five major climatic groups in the Köppen–Geiger climate classification, including tropical, arid, temperate, boreal, and polar. Temperate and boreal lakes located in the Northern Hemisphere comprised 87 % of all case-study lakes. The surface area of lakes ranged from 0.011 to 2700 km2, with an average and median area of 121.1 and 8.9 km2, respectively. Two-thirds of lakes covered surface areas between 1 and 100 km2. The average and median mean depths of lakes were 26.3 and 10.8 m (range: 1.7–304.8 m), where 90 % of the lakes were deeper than 3 m. The Secchi depths reported for 49 of the lakes were 4.9 m (average) and 3.5 m (median) and ranged from 0.5 to 32 m, which indicated a wide range of lake trophic status.
In ISIMIP3, the same approach is followed, but the number of lakes has increased. This is achieved through a data call to the research community, and by capitalizing on existing data harmonization efforts (e.g. Pilla et al., 2021).
3.2 Representative lakes in the global domain
Lake simulations in the global domain considered a single, generic lake in each grid cell that contains lakes in the 0.5∘ × 0.5∘ ISIMIP global grid. For a given grid cell, such a lake is termed “representative” because it is assumed to represent real lakes bound by its coordinates by sampling their bathymetric information to perform uncalibrated lake model simulations (Subin et al., 2012). The background data and sampling methods for generating representative lakes has evolved from ISIMIP2 to ISIMIP3.
In the global domain of ISIMIP2, generic lakes in each grid cell used average lake depth and surface area information from a rasterized version of the Global Lake and Wetland Database (see Sect. 3.5.1; Lehner and Döll, 2004). Using the mean lake characteristics in the grid cell could not account for the spatial distribution, variability, and non-uniform distribution in the depth and area of lakes in a grid cell. However, similar representations of lakes were used in Earth system and numerical weather prediction models (Subin et al., 2012; Balsamo et al., 2012; Thiery et al., 2015, 2016, 2017; Vanderkelen et al., 2021), and the grid cell lake representation was a necessary trade-off between computational feasibility and global representativeness. This global-scale lake coverage of 17 500 generic lakes made it possible to represent lakes in all major climatic classes and their subclasses, which was not possible in the local lake domain.
In the global simulations of ISIMIP3, this process was improved with a better method of characterizing generic lakes for each grid cell to represent true lakes with morphological characteristics from newer databases: HydroLAKES (Lehner and Messager, 2016) and Globathy (Khazaei et al., 2022). With this methodology, the 41 449 generic lakes in ISIMIP3 represent true lakes in a more realistic way than for generic lakes defined in ISIMIP2 (see Sect. 3.5.1).
3.3 Lake models participating in ISIMIP
Currently, 10 different lake impact models participate in the ISIMIP Lake Sector, where for some models two different versions were applied (Table 1). There are eight lake models providing calibrated simulations in the local domain: air2water4par, air2water6par, ALBM, FLake, GLM, GOTM5.1, MyLake, and Simstrat. The global ensemble consists of six lake impact models: ALBM, CLM, GOTM5.3, LAKE, Simstrat-UoG, and VIC-LAKE. In the sections below, these models are briefly described. For the models that contribute to both the local and global spatial domains, the global impact model section (Sect. 3.3.2) only describes the differences compared to the local version of the model used.
Table 1Overview of lake impact models participating in the Lake Sector of ISIMIP2a/b. For “Spatial domain”, L defines local or site-specific and G defines global simulations.
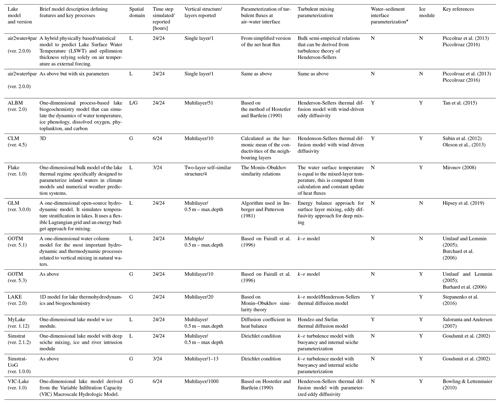
* Y – Yes; N – No.
3.3.1 Lake models for local simulations
air2water is a hybrid of a physically based and statistical model which simulates lake surface water temperature and epilimnion thickness solely based on air temperature as external forcing (Piccolroaz et al., 2013). The model estimates lake temperature in a single layer characterized by a time-varying thickness according to an empirical relationship accounting for the effect of thermal stratification. Within ISIMIP, two different versions of this model provided simulations for local lakes: air2water4par and air2water6par; these two set-ups of the model differ in the number of parameters affecting the lake thermal dynamics (Piccolroaz et al., 2016). The air2water model has been applied in lakes of varying climatic and morphometric conditions worldwide (Toffolon et al., 2014; Prats and Danis, 2019; Piccolroaz et al., 2020).
The Arctic Lake Biogeochemistry Model (ALBM) is a one-dimensional process-based coupled lake hydrodynamic and biogeochemistry model (Tan et al., 2015). The model simulates water temperature dynamics, ice phenology, and phytoplankton as well as dissolved nitrogen, oxygen, carbon dioxide, and methane. ALBM was originally developed for Arctic lakes (Tan et al., 2015, 2017) but has been used for other lakes across the globe (Guo et al., 2020, 2021; Tan et al., 2018). The thermal regimes of lakes are simulated in ALBM using 1D thermal diffusion equations in both water and sediment columns with atmospheric boundary conditions driven by sensible heat, latent heat, thermal radiation, and solar radiation. ALBM simulates 51 irregular lake layers. Snow and ice dynamics are solved using one snow layer, one white/grey ice layer that is formed when too much snow is accumulated, and multiple black ice layers (Tan et al., 2018).
FLake is a one-dimensional model specifically designed to represent the effects of inland waters in climate models and numerical weather prediction systems (Mironov, 2008). FLake uses a two-layer parametric representation of the lake water column. The upper layer is vertically homogeneous, representing the surface layer produced by wind and convective mixing at the lake surface. The lower layer represents the thermally stratified part of the water column. Two additional layers simulate the ice cover and the lake sediment. The vertical temperature distribution in each layer is modelled by a parameterized function of vertical coordinates, derived from a self-similar representation of the temperature profile. For calculation of surface heat fluxes, the model input includes standard meteorological variables describing the air–lake interaction: air temperature and humidity, wind force, and long-wave atmospheric radiation (or cloud amount for its calculation). The short-wave solar radiation enters the model equations as the volumetric source term distributed across the water column. The FLake version used here uses longwave radiation as a direct input, instead of calculating it from cloud cover.
The General Lake Model (GLM, v3.0) is a one-dimensional hydrodynamic lake model, which simulates temperature stratification in lakes (Hipsey et al., 2019). It uses a flexible Lagrangian grid, and an energy budget approach to simulate mixing. The vertical layer structure can change in number and thickness throughout a simulation, following changes in stratification and lake volume. In this study, we based the initial number of layers on the initial water depth. In addition, GLM includes modules for surface heat exchange and ice–snow dynamics, vertical mixing, and water balance dynamics. GLM can be coupled to the Aquatic Ecodynamics Modelling Library (AED) to simulate water quality dynamics and ecosystem interactions.
The General Ocean Turbulence Model (GOTM v5.3) is a one-dimensional model that simulates the most important hydrodynamic and thermodynamic processes related to vertical mixing (Umlauf and Lemmin, 2005). GOTM was developed by Burchard et al. (1999) for modelling turbulence in the oceans, but it has been recently adapted for use in lakes (Sachse et al., 2014). Typically, GOTM is used as a stand-alone model for investigating the dynamics of boundary layers in natural waters, but it can also be coupled to a biogeochemical model using the Framework for Aquatic Biogeochemical Models (FABM; Bruggeman and Bolding, 2014).
MyLake (v1.12) is a one-dimensional process-based model used to simulate physical, chemical, and biological dynamics in lakes (Saloranta and Andersen, 2007). The model simulates thermal stratification, lake ice and snow cover, and phosphorus–phytoplankton dynamics. It also contains a simple sediment box model. MyLake runs at a daily time step using regularly spaced water layers whose vertical resolution is defined by the user. Different versions of the open-source code have been applied to simulate algal blooms (Moe et al., 2016), CO2(g) and CH4(g) (Kiuru et al., 2019), internal phosphorus loads (Markelov et al., 2019), and light attenuation dynamics (Pilla and Couture, 2021).
Simstrat (v2.1.2) is a one-dimensional hydrodynamic model, which specifically includes vertical mixing induced by internal seiches that is not included in most other models (Goudsmit et al., 2002). The model uses layers of fixed depth and supports multiple options for external forcing, comprising several meteorological variables or surface energy fluxes. The model simulates thermal stratification and ice and snow formation (Gaudard et al., 2019). Simstrat has been applied in lakes of varying climatic and morphometric conditions (e.g. Thiery et al., 2014a; Kobler and Schmid, 2019; Mesman et al., 2020) and is operationally applied to provide near-real time, open-access simulation output of the thermal structure and ice cover of all natural Swiss lakes and lake basins greater than 1 km2 and a growing number of reservoirs and small lakes (Gaudard et al., 2019).
3.3.2 Lake models for global simulations
The Community Land Model (CLM) Version 4.5 of CLM (Lawrence et al., 2011; Oleson et al., 2013) is a land surface model that includes simulations with the Lake, Ice, Snow and Sediment Simulator (LISSS; Subin et al., 2012). The CLM4.5 model has been used by multiple ISIMIP sectors with one consistent set-up. CLM4.5 simulations and their outputs have been analysed to assess climate change impacts across a range of indicators within ISIMIP (e.g. Schleussner et al., 2018; Lange et al., 2020; Ito et al., 2020; Gudmundsson et al., 2021; Pokhrel et al., 2021; Gädeke et al., 2021; Reinecke et al., 2021; Thiery et al., 2021).
LAKE is an extended one-dimensional model that simulates thermodynamic, hydrodynamic, and biogeochemical processes in the water column and the bottom sediments of the lakes (Stepanenko et al., 2016). The model simulates vertical heat transfer considering the penetration of short-wave radiation (Heiskanen et al., 2015), ice, snow, and bottom sediments. The model explicitly accounts for the exchange of momentum, heat, and dissolved gases between water and the inclined bottom.
VIC-LAKE is a 1D lake model derived from the Variable Infiltration Capacity (VIC) Macroscale Hydrologic Model (Bowling and Lettenmaier, 2010) and optimized for simulations at a sub-daily timescale. The model is based on a lake energy balance by Hostetler and Bartlein (1990), Hostetler (1991), and Patterson and Hamblin (1988). Turbulent mixing is solved with Henderson-Sellers thermal diffusion models using parametrized eddy diffusivity (Henderson-Sellers, 1985). The model also contains an ice module, which dynamically simulates lake ice and ice snow cover.
Simstrat-UoG v1 is based on Simstrat v1.4 and is an earlier version of the model described above. This version uses an earlier snow and ice formulation from Patterson and Hamblin (1988).
3.4 Input data
3.4.1 ISIMIP2a
The Lake Sector simulation protocol for ISIMIP2a was completed in early 2020. This phase focused on the calibration of eight models in the local domain toward projecting these models with meteorological forcings from the gridded ISIMIP2a observations. The same set of gridded meteorological observations was then used for global lake simulations. However, calibrating these lake models globally was unfeasible because of a lack of measured lake water temperatures at this scale.
Meteorological data from 1979 to 2016 at the ISIMIP grid scale (EWEMBI, “EartH2Observe, WFDEI, and ERA-Interim data Merged and Bias-corrected for ISIMIP”, Lange, 2019a) were used as inputs for calibrating the local lake models. From EWEMBI, the grid cell from each local lake's geographical location (Fig. 1 and Table S1) was used for the model calibration. Since the majority of lakes lacked nearby weather stations, the uniform EWEMBI data allowed us to include a broader diversity of lakes and avoid cumbersome data harmonization. Since the EWEMBI dataset was also used to help bias-correct the future climate scenarios used in the ISIMIP2b simulation round (Frieler et al., 2017; Lange, 2019b), the performance of calibrated lake models can be indicative of their ability to simulate past and future climate change when forced by the ISIMIP bias-corrected data. In addition to the calibration runs that were limited to periods when observed water temperature data were available, the local sector modellers were encouraged to drive their lake models with the complete EWEMBI data record between 1979–2016. This was aimed at evaluating the lake models' abilities to reproduce effects of observed meteorologic variability and extreme events on thermal simulations. These simulations could also be used for benchmarking simulations forced with modelled future climate conditions from GCMs. In addition to the EWEMBI dataset, five other reanalysis datasets were provided in ISIMIP2a for modellers to use as inputs according to their capacities, with the goal of exploring the effect of input data choice on simulation outcomes. All datasets are described and referenced in the simulation protocol document (https://www.isimip.org/protocol/2a/, last access: 23 May 2022).
Data providers supplied historical measured water temperature profiles for 62 lakes (Fig. 1a, Table S1). Lake data had to meet two criteria to be included in the local lake dataset: (1) data needed to overlap with the EWEMBI time span, and (2) temperature profiles needed to encompass at least two consecutive years in the case of (sub)-daily sampling frequency, or at least five consecutive years in the case of (sub)-monthly sampling frequency. These criteria enabled intra- and inter-annual variability to be captured in water and meteorologic conditions in the model calibration procedure. A few lakes from under-represented geographical locations (e.g. tropics) were included despite shorter water temperature records.
Water temperature data were harmonized to a uniform data format and visually quality-controlled to remove outliers. In addition to water temperature, the data providers supplied detailed information of the lake depth and hypsometric data to characterize the morphometry of lake basins, which are required as input to most of the lake models.
3.4.2 ISIMIP2b
ISIMIP2b was designed to compare lake responses to simulated historical and projected future climates relative to pre-industrial climates with a focus on improving the understanding of the effects of global warming in the range of 1.5 to 2 ∘C (Frieler et al., 2017). The lake ensemble included simulations forced by bias-adjusted data from four GCMs, covering historical and up to three representative greenhouse gas concentration pathways (RCPs): a low (RCP2.6), medium–high (RCP6.0), and high emission scenario (RCP8.5). The past and future responses of lakes from these simulations were compared to simulations forced by bias-adjusted pre-industrial control (picontrol) climate data from the same four GCMs to quantify differences from pre-industrial conditions. These differences can be thought to represent the “pure” effect of ongoing changes in climate on simulated lake water temperatures, with minimal confounding effects from changes in further human influences that were identical between the two sets of simulations.
Climate input data for ISIMIP2b were derived from four GCMs, namely GFDL-ESM2M, HadGEM2-ES, IPSL-CM5A-LR, and MIROC5 (Taylor et al., 2012; Frieler et al., 2017) that were available from CMIP5. These GCMs were chosen since they best met the needs of all sectors participating in the ISIMIP and provided the necessary scenario length at daily temporal resolution. They had a wide range of projected warming rates, with GFDL-ESM2M and HadGEM2-ES representing the lower and higher ends of the warming spectrum, respectively. The data management team at the Potsdam Institute for Climate Impact Research (PIK) bias-adjusted the GCM data with a reference dataset of atmospherics observations (EWEMBI, Lange, 2019a) using the statistical transfer functions by Hempel et al. (2013) modified to correct known biases in modelled variables (Frieler et al., 2017). The bias correction was aimed at preserving trends and distributions of modelled variables relative to observed atmospheric observations. All meteorologic data except horizontal wind components were bias corrected. The list of output meteorological variables from GCMs that were used to drive the lake models can be found in Table 2.
Table 2Standard climate forcing variables used to govern lake models to simulate past, present, and future changes in local and global lakes. Dots between brackets indicate optional input variables.
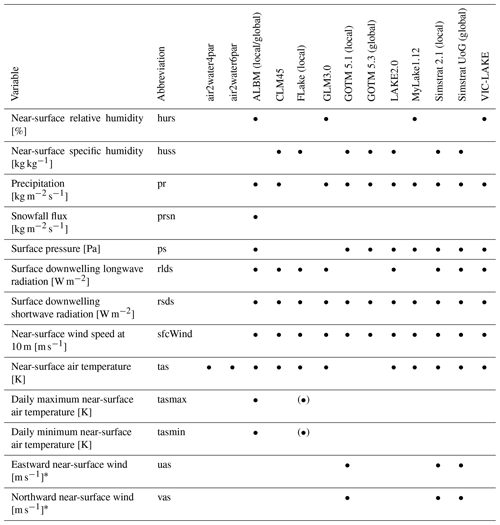
* Uncorrected.
Lake Sector simulations followed the ISIMIP2b protocol (Fig. 1, Frieler et al., 2017, https://www.isimip.org/protocol/#isimip2b, last access: 23 May 2022). To estimate the effects of historical climate warming, lake model simulations forced with data from historical emission scenarios were compared with simulations forced with data from the picontrol scenario. Likewise, to evaluate future climate impacts, lake model simulations were forced with data from the RCP trajectories (RCP2.6, RCP6.0, and RCP8.5) and compared to results from simulations forced with picontrol data. The time spans of different climate change scenarios were 1661–1860 (picontrol), 1861–2005 (picontrol and historical), and 2006–2099 (picontrol, RCP2.6, RCP6.0, and RCP8.5). An extended period between 2100 and 2299 was also used for simulations based on available results for specific emission scenarios (picontrol, RCP2.6) to evaluate longer-term changes in global temperature that meet the Paris Agreement objectives (Frieler et al., 2017).
Since the lake modelling strategy was specifically designed to evaluate only thermal changes resulting from changing atmospheric conditions under the assumption of no watershed inputs (constant lake level), the simulations are not influenced by any changes in land use or socio-economic conditions that would affect watershed inputs to the lakes or changes driven by changes in lake trophic status. The pre-industrial reference simulations (picontrol) assumed fixed socio-economic conditions and land use (1660–1860). CLM4.5 provides additional sets of simulations according to protocols for other ISIMIP sectors (i.e. biomes, agriculture, water (global), permafrost) with a combination of socio-economic (1860-soc and 2005-soc) and CO2 fertilization (2005-co2) scenarios. Lake temperature simulations that were a component of CLM4.5 did not account for these additional scenarios.
3.4.3 ISIMIP3a/b
The protocol for the third simulation round of ISIMIP, ISIMIP3, is currently ongoing. ISIMIP3 is largely similar to the ISIMIP2 protocol but includes counterfactual climate forcing in ISIMIP3a, and the next generation (CMIP6) of climate model forcing and various emission scenarios in ISIMIP3b. Below, we highlight the main differences between both simulation rounds.
In ISIMIP3a, the observational climate forcing covers the period 1901–2016 and consists of the Global Soil and Wetness Project version 3 (GSWP3; Dirmeyer et al., 2006), homogenized to W5E5 for the period 1901–1978 (Lange, 2019a) and a combination of the W5E5 dataset (Lange, 2019b; Cucchi et al., 2020) for the period 1979–2016 and GSWP3 before that. This observational dataset was bias-corrected using observations from the Global Precipitation Climatology Centre (GPCC) (more details at https://climatedataguide.ucar.edu/climate-data/gpcc-global-precipitation-climatology-centre, last access: 23 May 2022) and Climatic Research Unit (CRU) (more details at https://crudata.uea.ac.uk/cru/data/hrg/, last access: 23 May 2022) using the method outlined in Lange (2019b). In addition to providing data for the calibration for local lake models, ISIMIP3a provides counterfactual climate forcing, which is a detrended version of the historical climate forcing (Mengel et al., 2021). Models driven by the counterfactual climate and other historical human pressures provide a baseline to compare with simulations forced by the observational climate forcing to determine climate change impacts, paving the way for IPCC Working Group II style impact attribution (Cramer et al., 2014). In ISIMIP3b, the climate forcing is updated to include the next generation of CMIP6 simulations, which were bias-corrected with a new adjustment routine correcting the simulations towards the W5E5 observational data (https://www.isimip.org/gettingstarted/isimip3b-bias-correction/, last access: 23 May 2022). Climate simulations from five GCMs were provided, namely GDFL-ESM4, UKESM1-0-LL, MPI-ESM1-2-HR, IPSL-CM6A-LR, and MRI-ESM2-0. In addition to the picontrol and historical emissions simulations, future simulations include the SSP1-RCP2.6, SSP3-RCP7, and SSP5-RCP8.5 emission pathway scenarios. Following the CMIP6 protocol, the simulation periods were updated to 1601–1849 for pre-industrial, 1850–2014 for historical, and 2015–2100 for future simulations. Like in ISIMIP2, the GCMs chosen for ISIMIP3 were constrained by data availability, yet they are also a subset of better-performing models relative to the entire CMIP6 ensemble and they contain structurally independent model components (Lange, 2021).
3.4.4 Climate data application
All bias-adjusted meteorologic forcing data provided by ISIMIP sectors have a daily temporal resolution and a spatial resolution matching the ISIMIP grid scale. While most models in the Lake Sector performed simulations at daily time steps, some models required temporally disaggregated forcing data at sub-daily time steps. The modelling teams performed temporal disaggregation using their customary approaches (see Sect. 4). For simulations in the local domain, data were extracted for grid cells corresponding with the lakes' geographic locations. No further downscaling or local corrections were applied to ensure consistency in the forcing data applied to all local lakes.
3.5 Lake parameterization
In ISIMIP2 and ISIMIP3, to account for variations in individual lake responses to meteorological drivers (Heiskanen et al., 2015; Kraemer et al., 2015; Shatwell et al., 2019), there were only two types of data needed by the lake models: a description of the lake bathymetry and information on the lake water transparency, which are necessary for estimating the diffuse attenuation coefficient of incoming shortwave radiation.
3.5.1 Bathymetry
Most lake hydrodynamic models require the hypsographic relationship between depth and surface area, which is critical for determining layer volumes and storage and the vertical transfer of heat. Data providers supplied these bathymetric data for each lake in the local domain. The two versions of air2water models did not require information on lake bathymetry.
For global lake simulations in ISIMIP2, the bathymetry of the representative lakes in each grid cell was derived from a rasterized version of the Global Lake and Wetland Database (GLWD; Lehner and Döll, 2004; Toptunova, 2019). Specifically, for each grid cell, average lake depth and lake surface area values were calculated from all GLWD lake data contained within the grid cell. Lake bathymetry for each generic lake then was assumed to be cylindrical. In the case of the LAKE model, both surface area and mean depth of global lakes were obtained from GLDBv2 (Choulga et al., 2014). In the case of the global simulation model CLM4.5 (see Sect. 3.3.2), all representative lakes had a constant 50 m depth. For all of the lake models, a cylindrical shape was assumed to represent lake bathymetry. The gridded lake masks for the surface area and mean depths can be accessed online (https://data.isimip.org/, last access: 23 May 2022).
In the ISIMIP3 global lake simulations, we selected a representative lake for each grid cell from the 1.4 million lakes included in the HydroLAKES shapefiles (Lehner and Messager, 2016). Here, each lakes was assigned to a single grid cell using the location of the lake centroid. For grid cells containing more than one lake centroid, we selected the lake with the depth corresponding to the depth weighted median (weighted by the area of the lakes) of all the lakes contained in the pixel. Then, for each representative lake selected in the previous step, volume, area, mean and maximum depth, and 11-level area and volume hydrographic curves were extracted from GLOBathy (Khazaei et al., 2022). After applying this procedure, we obtained a total of 41 449 representative lakes across ISIMIP grid cells (see Fig. 3).
3.5.2 Water transparency
Water transparency may mediate a lake's response to climate change (Butcher et al., 2015, Magee et al., 2016; Shatwell et al., 2019). The attenuation coefficient (Kd, m−1) of shortwave radiation is a key parameter to describe water transparency in the lake models (Potes et al., 2012). In all simulations, single non-varying values of Kd were used.
For simulations in the local domain, multi-season and multi-year water transparency data were available from 49 lakes that were used to calculate a diffuse attenuation coefficient (Kd) value or a mean Secchi depth (ZSD, m) (Table S1). When only ZSD measurements were available, Kd was estimated at . (Poole and Atkins, 1929). If both mean ZSD and Kd were provided, the directly measured Kd was used.
When both Kd and ZSD were lacking, we approximated Kd as a function of mean lake depth following the equation derived for 88 Swedish lakes (Håkanson, 1995),
or from maximum depth following the expression derived for 1258 global lakes (Woolway et al., 2021b):
For ISIMIP2a, the different modelling teams defined the best method to parameterize transparency based on the specific lake model requirements and previous protocols developed for calibration and simulation with any given model. Consequently, transparency parameterizations varied both with lake models and the spatial domain of simulations. In the local domain, Kd values derived from ZSD or Hakanson's expression were used in ALBM, Simstrat, and GLM, whereas FLake runs adopted the approach outlined in Woolway et al. (2021b). In GOTM and MyLake, the mean Kd was determined in the calibration process. The two air2water models did not require water transparency. In the global-scale simulations, the grid-varying mean depths of the lakes were used to estimate Kd values from Hakanson's expression in all lake models except for VIC-LAKE. This estimation process is also in the CLM4.5 (Oleson et al., 2013). In the VIC-LAKE model, two-band (visible and near-infrared) Beer's law radiation constants were used to parameterize transparency (Bowling and Lettenmaier, 2010).
3.5.3 Water balance
To simplify lake simulations, the water balance and water inputs and withdrawals were not considered in ISIMIP2 and ISIMIP3. The formulations of some lake models (e.g. air2water or FLake) do not explicitly include hydrological balances. For the rest of the models, the precipitation and evaporation component of water mass exchange was switched off (i.e. only heat exchange occurred) or compensated with a closure term (e.g. CLM4.5). This assumption allowed us to evaluate changes in lake thermal structure in the time frame of the ISIMIP2 and ISIMIP3 simulation periods.
Regional studies assessing the hydrologic responses of lakes to an ensemble of future climate change scenarios show that our omission might variably affect lakes depending on lake type and future climate outcomes for seasonal drying and wetting (Hanson et al., 2021; Hunt et al., 2013). These studies found that drainage lakes in northern Wisconsin, United States, which are hydrologically mediated by lake inflows and outflows, were projected to maintain stable water levels because of competing climatological factors that did not promote a clear drying trend. Under our omission of lake water balances, projections for such lakes could lose reliability where future climate conditions reduce watershed runoff. In the same region, seepage lakes with minimal surface water fluxes and a greater dependence on groundwater inflows, however, were projected to significantly decrease in water level, especially in higher-elevation regions near groundwater divides. These studies are relevant for both our local and global lake simulations. For lakes in the local domain, despite accurate representations of historical changes in lake thermal structure (Table 3), the omission of a water balance could additionally affect the simulated climate change impacts in seven lakes and reservoirs with large water level fluctuations (Table S1); thus caution should be used when evaluating these results. For lake simulations in the global domain, this omission is yet another necessary trade-off between experimental complexity and spatial representativeness (see Sect. 3.2).
Table 3Summary of local domain calibration of lake models participating in the ISIMIP2a/b simulation rounds. Summary statistics for calibrated parameters and models' performance represent the cross-site mean, median [minimum – maximum].
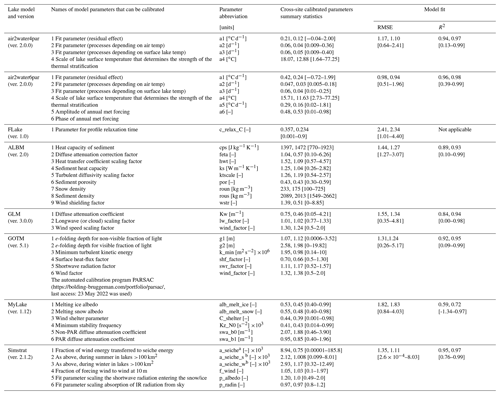
a a_seiche parameter was calibrated for lakes <100 km−2 (n=51). b a_seiche_s and a_seiche_w parameters were calibrated separately for summer and winter months in lakes >100 km−2 (n=8).
3.6 Calibration of local lake models in ISIMIP2a
Eight lake models had specific parameters and coefficients calibrated based on what each modelling group felt was appropriate for use with their specific lake model (Table 3). Each modelling group defined reasonable coefficient ranges based on past experience and the physical constraints that would set limits on the parameter and coefficient values. For each model, the same calibration routine and objective function was applied to all lakes in the local domain. Different objective functions (e.g. RMSE, NSE, Pearson r; see Table S2) were adopted by the different models so that modellers could use their optimal criteria for calibration. In all cases the model performance was optimized by minimization of the difference between simulated and measured water temperature.
The number of calibrated parameters and coefficients in a specific model ranged from one (FLake) to nine (ALBM, Table 3). The calibrated coefficients were mostly related to processes controlling surface heat and energy fluxes, turbulent kinetic energy and wind stress, and light attenuation. Other calibrated coefficients for specific processes were model-specific, including sediment structure and heat fluxes (ALBM), seiches (Simstrat), and ice–snow energy fluxes (MyLake, Simstrat). To allow for comparing the lake models' performance in predicting measured water temperature, for all lake models two common metrics of model fit were calculated in post-processing (not necessarily coincident with the calibration metrics): the root-mean-square error (RMSE) and coefficient of determination (R2, Table 3).
Most lake models were calibrated with the full series of available measured observations. In this majority of cases, no data were withheld for an independent model validation. Considering the relatively short temporal extent of lake measurements, this was done to base parameter estimates on the full range of environmental conditions encountered during simulation for producing robust future projections (Larssen et al., 2007). This is justifiable given extensive research validating the performance of these models outside the calibration period (e.g. Stepanenko et al., 2013; Thiery et al., 2014a) and arguments calling for scepticism of the split-sample approach to calibration and validation (Augusiak et al., 2014; Shen et al., 2022). Exceptionally, ALBM only used the full series of measured observations when the observations were shorter than 5 years. Where measurements exceeded 5 years, modellers running ALBM simulations opted for a split-sample approach to tuning their model and used the first 5 years of measurements for calibration.
3.7 Long-term simulations in ISIMIP2b
The ensemble of lake models in both the local and global domains was forced with the bias-adjusted GCM outputs for the pre-industrial control, historical, and future climate change scenarios. When running the long-term simulations, the calibrated models for each local lake were used, so that each model was optimized for that lake based on the historical calibration described in Sect. 3.6. Spin-up periods used with the local lake models varied and were dependent on the protocols and experience of each modelling group (Table S2). When a spin-up period was used, the spin-up data were created either by repeating the initial year(s) of the scenario input data and then adding these duplicate data to the beginning of the forcing data, or by using a portion of the historical scenario to spin-up future scenario simulations. Initial conditions used for water temperature profiles in the local lake simulations also varied with model and geographical location and were based on either observed temperature profiles, an assumed isothermal 4 ∘C profile, or related to the mean annual air temperature at the local lake location. A more detailed description of the modelling workflows that were used to spin up and initialize each model in the local domain is given in Table S2.
For simulations in the global domain, most lake models used default parameter and coefficient values that were set according to previous experience with each model (see Table 1: “Key references”). Exceptionally, for GOTM, the average values of calibrated coefficients from the GOTM local lakes (Table 3) and default values for the coefficients that were not calibrated (Umlauf and Lemmin, 2005; Sachse et al., 2014) were used for all representative lakes in the global domain. Similarly, the methods for spinning up the models in the global simulations also varied depending on the practices applied by each modelling group. Groups working in the global domain tended to use longer spin-up periods and data from either the picontrol or historical scenarios to create the spin-up data that were added to the scenario forcing data. The initial water temperature profiles used in the global lake simulations also varied. In some cases, the models were initialized as homogenous profiles often based on the mean annual air temperature or linear profiles based on the mean annual air temperature and an assumed 4 ∘C bottom temperature, or linear profiles using a fixed surface and bottom temperature. A description of the modelling workflows that were used to spin-up and initialize each model in the global domain is given in Table S3. More detailed model-specific simulation set-ups can also be found at https://www.isimip.org/impactmodels/ (last access: 23 May 2022).
3.8 Output data format
All outputs from the models were aggregated to daily averages (Tables 4, S4). The vertical resolution of the simulated water temperature profiles in the local domain was reported at 0.5 m intervals for lakes with <50 m maximum depth and at 1 m intervals for lakes >50 m. However, the vertical resolution of simulated temperatures in the global domain was limited by file storage capacity. The number of reported layers depended on the depth of the representative lake and ranged from 1 to 13 (GOTM, LAKE, Simstrat-UoG), from 1 to 50 (ALBM), and from 1 to 1000 (VIC-LAKE). Output from CLM4.5 was grid-invariable, representing water temperature in 10 layers. The remainder of reported variables (thermodepth, surftemp, bottemp) represented a single value, which was either calculated using the approach presented in the simulation protocol (see https://www.isimip.org/protocol/2b/, last access: 23 May 2022) or was directly outputted by the lake model (Tables 4, S4). The Lake Sector simulation protocol provides the model performance metrics used during calibration of lakes in the local spatial domain (Table 3). A full list of variables simulated within ISIMIP2b is summarized in Table S4.
Table 4Common output variables reported by local (L) and global (G) models participating in the Lake Sector of ISIMIP2a/b. The variable watertemp is a full water temperature profile. Naming of lake models and variables are ordered in an alphabetical order (see Table S4 for a list of full variable names).
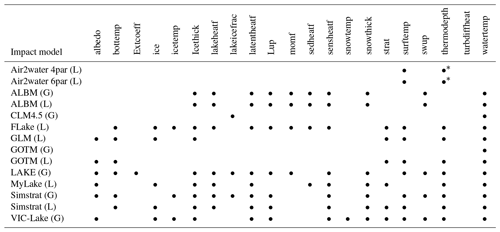
* The model provides a time-varying estimate of the well-mixed surface layer participating to the heat exchanges with the atmosphere.
This diversity of GCM input datasets, emissions scenarios, lake models, and their output variables means that the total ensemble of impact simulations under the Lake Sector requires considerable storage space. In addition, those appreciable computing resources should be anticipated by potential future collaborators. For example, the global lake simulations for ISIMIP2b take up 14 TB of storage space. This means that applications with simulations under multiple GCMs, lake models, and scenarios for a given variable will require high-performance computing resources. For running simulations, computing times may vary depending on the scale of one's contribution. On the one hand, simulating a local, calibrated lake with FLake for a single scenario and GCM combination may take seconds on a laptop (Thiery et al., 2014b), but, on the other hand, global simulations from CLM4.5 for one such scenario and GCM combination will require several weeks using 144 compute cores on a high-performance computer, substantiating both computational costs and resources for dataset storage. These technical prerequisites, in addition to individual model feasibility issues for local versus global domain simulations, explain the discrepancy in model availability across the ISIMIP2 local and global simulations.
4.1 Results from ISIMIP2a
Calibration and performance of local lake models
The simulated water temperatures from the calibrated lake models compared well to the measured water temperature data using the entire record of recorded water temperature data from each local lake. Based on the simulation data from 62 lakes, all eight local lake models were calibrated with a multi-model mean RMSE of 1.50 ∘C that ranged from 0.98 (air2water6par) to 2.41 ∘C (FLake, Table 3). The coefficients of determination (R2) ranged from 0.59 (MyLake) to 0.96 (air2water6par), with the multi-model average value of 0.84. The lake models predicting surface water temperature only, air2water4par and air2water6par, showed lower prediction errors compared to the lake models predicting full water temperature profiles. While the multi-model mean goodness of fit was reasonable for most lakes, 16 % of lakes showed RMSE larger than 2 ∘C, indicating less certain predictions (Tables 3, S1). For individual models, the number of lakes with RMSE exceeding 2 ∘C varied from 3 lakes (5 %, air2water4par) to 40 lakes (65 %, FLake).
Although the ISIMIP2a forcing data used a daily time step at the ISIMIP grid scale, the prediction errors in water temperature were relatively small (Table 3), even though these input data and their resolutions are, in general, less than optimal for the simulations of individual lakes (Bruce et al., 2018). An exception is the air2water model that, owing to its statistical and data-driven calibration of its model's parameters, has been shown to be able to provide the same projections irrespective of the nature of the air temperature dataset used to drive the model (Piccolroaz et al., 2018). It should be noted, however, that inter-model performance comparisons are difficult here. Due to the diverse discretization of lake temperature profiles across models, each model is being evaluated on a derivation of available lake measurements. Therefore, the observations used as a reference in the performance metrics are different across models, reducing the comparability of model performances for a given metric. The average errors in the prediction of water temperature observations were comparable with previous multi-model and/or multi-site modelling studies, where the mean RMSE in water temperature predictions ranged from 1.10 to 2.79 ∘C (Stepanenko et al., 2013; Winslow et al., 2017; Bruce et al., 2018; Piccolroaz et al., 2020). Similarly, the prediction of epilimnetic temperature showed lower errors compared to predictions of hypolimnetic temperature (Winslow et al., 2017; Bruce et al., 2018).
4.2 Results from ISIMIP2b
Impacts of past and future climate change on lakes
Time series of ensemble simulations of lake surface temperature for local lakes over the historical (1851–2005) and future (2006–2100) periods are shown in Fig. 2a. Each ensemble combines the results from 62 well-studied lakes and three separately calibrated lake models. Mean annual surface water temperatures increased by 0.15 ∘C at the end of the historical period (present-day, 1976–2005) relative to the pre-industrial control. These simulations support in situ observations showing that lakes across the globe are already warming (Woolway et al., 2020; O'Reilly et al., 2015). Future projections (2006–2099) accounting for low (RCP2.6) to high (RCP8.5) greenhouse gas emissions under present-day socio-economic conditions, provide ensemble estimates of lake surface warming of 1.38, 2.46, and 3.85 ∘C by the end of the century (2070–2099) relative to pre-industrial control, respectively (Fig. 2a). For example, the strong mitigation measures associated with RCP2.6 resulted in lake surface temperature remaining below 2 ∘C. The ensemble projections consistently show a slower surface water warming under RCP2.6, starting mid-century (Fig. 2a), than for other greenhouse gas emission scenarios.
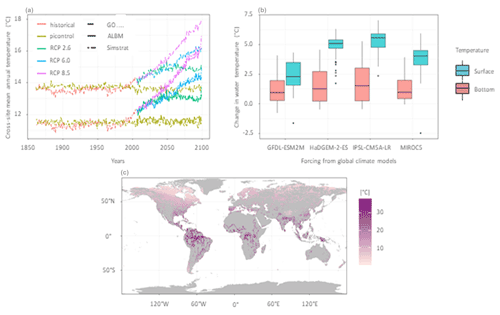
Figure 2Example output data in the ISIMIP2b Lake Sector. (a) Local model time series of mean annual surface temperature derived from ensemble simulations for a period 1861–2100 (historical and future) averaged across lakes and climate models. Colour-coding indicates greenhouse gases scenarios; line types differentiate lake models. Warmer temperatures simulated by the GOTM model are due to the fact that the model version used had only a very rudimentary method for simulating the effects of lake ice on the surface heat exchange. Spring–fall GOTM temperature simulations were compatible with the other models. (b) Temperature changes by 2070–2099 compared to pre-industrial levels in summer mean temperature at two lake depths simulated with GOTM (local) model evaluated with data from four climate models under RCP8.5. (c) Global outlook for the mean annual surface temperature of lakes by the end of the 21st century (2070–2099) under RCP2.6 simulated with GOTM global.
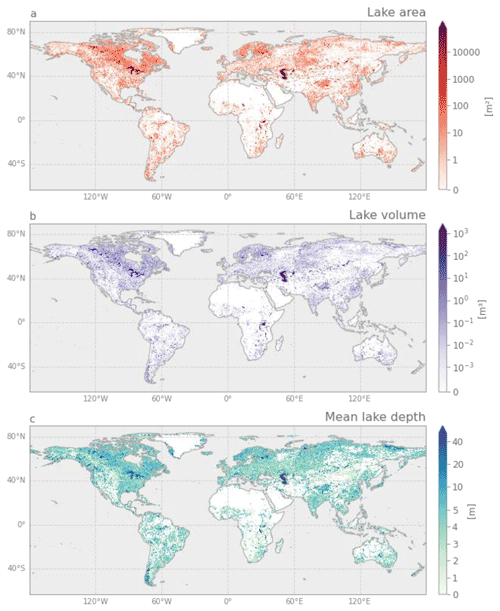
Figure 3Maps of lake area (a), lake volume (b), and mean lake depth (c) used in the ISIMIP3 simulation round. In the input data for ISIMIP3 simulations, a single lake is assigned to each grid cell. However, here we show a modified version of the dataset to delineate large lakes in the global map. The maps are derived from HydroLAKES (Lehner and Messager, 2016) and GLOBathy (Khazaei et al., 2022) datasets using the ISIMIP3 lake mapping methods described in “Code and data availability”.
Based on the anomalies between the pre-industrial control and future scenarios, all lake models showed similar warming rates and trajectories of change. However, the GOTM local simulations were on average 1.75 ∘C warmer than simulations from ALBM and Simstrat, probably because the version of GOTM used for the local lake simulations had only a very rudimentary description of the effects of lake ice on surface heat exchange. These results show the importance of using an ensemble of models to increase the robustness of simulated past and future changes and making interpretations less dependent on a single or small suite of the lake models used (Trolle et al., 2014).
The common fundamental output from all lake models was water temperature, and for most models, this output is in the form of a full vertical profile at a daily time step. These data and other related model output were also aggregated to different metrics describing lake hydrodynamics, e.g. thermocline depth, the onset of stratification, energy and heat fluxes at the air–water interface, and lake ice characteristics and dynamics (Table 4). The methods to calculate these metrics are defined in the Lake Sector protocol (https://www.isimip.org/protocol/, last access: 23 May 2022), and for additional metrics, the full lake water temperature profiles can be further processed by users.
The average changes in surface and bottom water temperature for the 62 lakes for the RCP8.5 greenhouse gas emission scenario, using the GOTM model forced by the four GCM outputs, are shown in Fig. 2b. Results from the ensemble simulations of the local lakes' future responses show faster warming of surface waters (local-lake mean 4.08 ∘C) than bottom waters (1.49 ∘C) by 2070–2099. On average, the difference between the surface and bottom water temperature anomaly was 2.6 ∘C. There was a wide range of lake responses in the local domain (Fig. 2b), with an average range in the change in surface temperatures anomalies derived from the ensemble of 2.28 ∘C and bottom temperature of 3.22 ∘C. These results are consistent with previous findings of the diverse responses in lake surface temperature across the globe (O'Reilly et al., 2015; Pilla et al., 2020) depending on a complex interaction of climate regions (Piccolroaz et al., 2020), lake morphology (Toffolon et al., 2014), and atmospheric conditions (Spence et al., 2013), and changes in the responses of bottom temperatures being influenced by the lake's morphometric characteristics (Kraemer et al., 2015). The Lake Sector local domain provides information on the lake-specific characteristics related to morphometry and water transparency (Table S1) to enable investigation of how the observed differences in responses to climate warming are influenced by lake characteristics.
The variability in Fig. 2b is a result of both variable lake responses and the differences in the forcing associated with the four GCMs. The mean change in surface water temperatures under RCP8.5 until the end of the 21st century ranged from 2.39 ∘C (when forced by GFDL-ESM2M) to 5.34 ∘C (when forced by IPSL-CM5A-LR). A similar pattern was observed for bottom temperature, although the differences were less pronounced (1.19–1.78 ∘C). The changes in the mean surface temperature followed the differences in the air temperature projected by the four GCMs. Sorted from colder to warmer based on simulated impacts on air and water temperature on the local lakes, the GCMs are ranked in the order of GFDL-ESM2M, MIROC5, HADGEM2-ES, and IPSL-CM5A-LR. Similar differences were observed in water temperature and ice changes by Woolway and Merchant (2019). These results indicate that the choice of the GCM has a large effect on the changes predicted by the lake models. Using outputs from several GCMs, following the ISIMIP protocols, therefore, provides the advantage of including ensemble forcing data in simulations of climate change impacts on lakes, increasing the robustness of predictions (Grant et al., 2021).
The results of global domain simulations made with the GOTM model are shown in Fig. S1 for three greenhouse gas emission scenarios and as an ensemble of four GCMs. Under RCP2.6, the emission scenario with the strongest mitigation measures, the global mean annual lake surface temperature was projected to be 12.7 ∘C (range: 3.8–29.4 ∘C) by the end of the 21st century (Figs. 2c, S1a). However, global mean lake surface temperatures of 13.4 ∘C (4.2–30.4 ∘C) and 14.3 ∘C (4.8–31.6 ∘C) were projected for the medium–high emission (RCP6.0) or high-end emission scenario (RCP8.5), respectively (Fig. S1c, e). Mean annual lake temperature was projected to increase by 0.9 ∘C (0.53–1.32 ∘C), 1.7 ∘C (1.0–2.3 ∘C), and 2.6 ∘C (1.6–3.6 ∘C) under these three greenhouse gas emission scenarios relative to the pre-industrial control (Fig. S1b, d, f). Simulations in the global domain allowed the documentation and visualization of spatial variations in lake thermal structure that are not possible using geographically constrained data in the local domain. The most pronounced spatial pattern was a latitudinal gradient of warming of global lakes (Figs. 2c, S1). These results corroborate previous global-scale modelling studies, although here the results are based on ensemble simulations compared to single model simulations.
Existing studies applying these simulations demonstrate the many possibilities for exploring the impacts of climate change on lake physics under the ISIMIP protocol. ISIMIP simulations have been used in a first ever assessment of the global heat uptake by inland waters (Vanderkelen et al., 2020), a relevant addition to existing evaluations of Earth's global heat budget in its land, atmosphere, and oceans. The ISIMIP Lake Sector database has also been used to assess present and future alterations of lake mixing regimes (Woolway and Merchant, 2019) and the shifts in lake stratification and their climatic drivers (Woolway et al., 2021b). Finally, both event and trend attribution of lake heatwaves (Woolway et al., 2021b, 2022) and lake ice cover changes (Grant et al., 2021), respectively, have been undertaken using ISIMIP simulations in combination with global observational datasets to confirm the role of anthropogenic climate change in observed lake changes.
4.3 Future work
4.3.1 Model response to observational vs. simulated forcing data
In addition to simulations using ISIMIP2a forcing, the ALBM and FLake models were also used for simulations forced by EWEMBI observational data (1979–2016). This will allow for assessment of the difference in model output when used with observational forcing data compared to simulations with GCM forcings during the historical time period. Given that impacts under past and future climates are modelled with bias-adjusted GCMs, a comparison with simulations using the observed data used for bias correction will allow an assessment of how simulations forced with the GCM historical inputs compare with those forced using observed (historical) climate (see also Piccolroaz et al., 2018 for a similar analysis). This can give an estimate of the uncertainty in the ISIMIP GCM scenarios and the bias correction method. There are so far no studies for this application of the ISIMIP2a simulations, but the existing simulation output archives are publicly accessible and hold potential for further study.
4.3.2 Lake hydrology and water quality assessments
Current lake modelling activities in ISIMIP are biased towards lake physics and concentrate mostly on water temperature and related variables like ice cover or stratification state. Lake managers require more than that and are usually highly interested in projections for water quantity (i.e. inflow discharges) and water quality and potential effects on the services lakes deliver. Future directions of the lake sector beyond ISIMIP3 are therefore seen in (i) linking the water sector (hydrological models) with the lake sector in order to integrate water quantity projections into lake simulations and (ii) adding water quality descriptors by biogeochemical modelling of lakes. Such modelling can make projections for the future development of ecosystem services and biodiversity in lakes in relation to climate change and socio-economic development. For climate change, such assessments can be directly built on the ISIMIP2 and ISIMIP3 simulations of the Lake Sector but require linkage with the transport of water and nutrients from their catchments (Janssen et al., 2019). For that, nutrient transport models such as IMAGE-GNM (Beusen et al., 2015) or MARINA (Strokal et al., 2016) need to be aligned akin to the ISIMIP approach.
4.3.3 Global-scale calibration and validation
Important steps can be made in the development of a global-scale dataset for calibration and validation purposes. There are a few challenges to overcome in the future (Janssen et al., 2015). First, due to project-based research, long-term measurements are rare as often measurement campaigns stop when projects are over. Second, data are often locked within institutes, meaning that a consistent global database requires corporation between various parties. Similarly, in situ data that have not been properly indexed and stored, sometimes referred to as “dark data”, require rescue efforts to extend back our measurement period of lakes. Third, data are gathered inconsistently, for instance by using different methods, measuring over different periods, or collecting at different spatial scales. Remote sensing could overcome these issues to some extent, as they can provide long-term, global observations.
Remote sensing datasets for lakes are increasing (Dörnhöfer and Oppelt, 2016). Examples of already existing datasets are datasets for lake temperature (Sharma et al., 2015), ice phenology (Wang et al., 2021), and even biological indicators (Fang et al., 2022; Hou et al., 2022). A disadvantage is that remote sensing is limited to proxy values, which still require ground truthing by in situ monitoring data. Moreover, remote sensing performs variably depending on the measurement system and weather conditions and are variable in assessment. While optical imagery is easily obscured by cloud cover, active microwave systems can be used in all-weather conditions for some variables such as ice cover (Kilic et al., 2018; Murfitt and Duguay, 2021). Therefore, satellite observations must be combined with highly spatiotemporally resolved in situ measurements from buoys, field sampling programs, and long-term monitoring networks (Rand et al., 2022). Specifically, in situ measurements are essential for observing lake processes below the water surface (such as stratification and mixing), to improve understanding of complex air–water energy fluxes (such as evaporation), and to maintain long-term perspectives that began prior to the advent of satellites and regardless of weather conditions that adversely impact some satellite measurements. First attempts at such databases are for example the HydroLAKES database, which already has water discharge into lakes (Messager et al., 2016).
Modelling the impact of climate change at a global scale using an ensemble of lake models requires data, vision, ambition, and a strong collaborative network of researchers from a range of disciplines. The first ensemble model simulations in the Lake Sector of the Inter-Sectoral Impact Model Intercomparison Project (ISIMIP) has leveraged such a network to design and execute a protocol that has now provided state-of-the-art scientific evidence of climate change impacts on lakes under low to high greenhouse gas emission scenarios. The Lake Sector protocol in the local domain allows for the calibration of lake models forced with historical ISIMIP2a inputs and parametrized using site-specific data of bathymetry. Comparison of simulated and measured water temperature from 62 well-studied lakes facilitated detailed calibration and evaluation of the models in the local domain. In future global simulations, these locally derived parameter and coefficient values could improve the full ensemble of models that have so far been uncalibrated in their global domain applications. Simulations in the global domain provided daily outputs of lake thermal conditions at the ISIMIP grid scale.
Our simulations at both the local and global resolution quantify past and future changes in water temperature, energy and heat fluxes, and ice with unprecedented geographical coverage. Simulations by the ISIMIP Lake Sector also provide previously unattainable opportunities to evaluate the levels of uncertainty in simulations related to the differences in forcing data between reanalyses, GCMs, emission scenarios, and in model structure and parameterization among lake models.
Simulations by the ISIMIP Lake Sector continue to estimate the range and robustness of plausible lake responses to global warming either at 1.5 ∘C above pre-industrial levels as defined by the ISIMIP2b protocol or for any other future greenhouse gas emission scenarios. This work furthers the state of the art in freshwater science (Vanderkelen et al., 2020; Grant et al., 2021; Woolway et al., 2021b).
Here, we have described the protocol of the Lake Sector in ISIMIP2 and ISIMIP3, which includes the simplifying assumption that hydrologic inputs from the lake watershed had minimal effects on the simulated thermal structure. While this is a reasonable assumption for lake hydrodynamic simulations, it will not be sufficient for simulations of lake biogeochemistry and ecology that strongly depend on the nutrient inputs from the lake watershed. Under the ISIMIP framework's provision of consistent climate forcing datasets and scenarios, the climate change impacts simulated in the Lake Sector are comparable with simulation results from other ISIMIP sectors, supporting cross-sectoral assessments of climate change impacts (Lange et al., 2020; Vanderkelen et al., 2020; Thiery et al., 2021). Ultimately, we expect that the improved simulations of lake hydrodynamics presented here will form a basis for more complex simulations of water quality, lake level fluctuations, and other greenhouse gas emissions scenarios in upcoming simulation rounds, where lake water quality models can be coupled to the hydrologic and biogeochemical outputs from other sectors of the ISIMIP.
The global ISIMIP2a lake sector simulations are available at https://doi.org/10.48364/ISIMIP.530719 (Marcé et al., 2022a). The global ISIMIP2b lake sector simulations are available at https://doi.org/10.48364/ISIMIP.931371 (Marcé et al., 2022b). The local ISIMIP2b lake sector simulations are available at https://doi.org/10.48364/ISIMIP.563533 (Marcé et al., 2022c).
The simulation protocol used in the Lake Sector of the ISIMIP2a-b and ISIMIP3a-b simulation rounds has no common code associated with it. The source codes for specific models are either publicly available or can be requested from the model leaders. A full list of models is available at https://www.isimip.org/impactmodels (The Inter-Sectoral Impact Model Intercomparison Project, 2022). All inputs to ISIMIP impact models and model output simulations can be found here: https://data.isimip.org/ (last access: 23 May 2022). Background information and citations for ongoing ISIMIP3 developments are at https://doi.org/10.48364/ISIMIP.263794.1 (Vanderkelen and Schewe, 2020a) for ISIMIP3a and at https://doi.org/10.48364/ISIMIP.383948 for ISIMIP3b (Vanderkelen and Schewe, 2020b). For global lake mapping in ISIMIP3 (see Sect. 3.5.1, “Bathymetry”), the code for processing lake simulation input data is publicly available and can be found here: https://doi.org/10.5281/zenodo.6457813 (Marcé et al., 2022d).
The supplement related to this article is available online at: https://doi.org/10.5194/gmd-15-4597-2022-supplement.
MGo, WT, RM, DMB, and DP coordinate the lake sector, with key contributions over the past years of IV, RIW, LG, and EJ. JS, FZ, KF, and MMe are part of the ISIMIP core team, provided the meteorological input data, and were the liaisons between the lake sector and the whole project. MGo, WT, DMB, RIW, VYB, RMC, AVD, BD, GG, MGu, ABGJ, GK, RL, MMa, TM, MP, SP, LRV, MS, TS, VMS, ZT, and HY conducted the lake model simulations. WT, DMB, RMC, GG, GK, MMa, TM, MS, HY, RA, MA, OA, LA, KA, LB, CC, KC, EdE, CD, MGr, JH, KJ, IDJ, AL, EBM, IM, HM, CM, DÖ, MP, KR, DR, JAR, RS, LvdL, PV, DW, NKW, SW, and GZ provided in situ lake calibration data. All co-authors read the article and provided comments. LG wrote the response letter and revised the article during the review process.
The contact author has declared that neither they nor their co-authors have any competing interests.
Publisher’s note: Copernicus Publications remains neutral with regard to jurisdictional claims in published maps and institutional affiliations.
We thank and acknowledge the support of Stefan Lange, Matthias Büchner, and Iliusi Vega del Valle for their roles in producing, coordinating, and making available the ISIMIP climate scenarios. We also thank Jorrit Mesman for enabling ISIMIP3 simulations through LakeEnsembleR. Malgorzata Golub thanks Marcus Lundberg and Douglas Scofield at Uppmax for their assistance with codes optimization and software installation, which was made possible through application support provided by SNIC. The Archbold Biological Station, Evelyn Gaiser, Vivienne Sclater, and Monika LaPlante are thanked for sharing the data on Lake Annie. We thank Jacob Zwart of USGS for reviewing this paper. Any use of trade, firm, or product names is for descriptive purposes only and does not imply endorsement by the U.S. Government. Finally, we thank Willem van Verseveld, Bertrand Guenet, and Peter Düben for reviewing this paper and Charles Onyutha for acting as editor.
The funds from the WATExR project (ERA4CS, JPI Climate) provided the financial support to Malgorzata Golub, Rafael Marcé, Don Pierson, Tadhg Moore, Eleanor Jennings, and Daniel Mercado-Bettin. The computations and data handling were enabled by resources provided by the Swedish National Infrastructure for Computing (SNIC) at Uppmax partially funded by the Swedish Research Council through grant agreement no. 2018-05973. Inne Vanderkelen is a research fellow at the Research Foundation Flanders (grant no. FWOTM920). Zeli Tan is supported by the US DOE's Earth System Modeling program through the Energy Exascale Earth System Model (E3SM) project. Annette B. G. Janssen is supported by the NWO talent grant Veni (project number VI.Veni.194.002). Bram Droppers is supported by the Wageningen Institute for Environment and Climate Research (WIMEK; grant no. 5160957551). Hampus Markensten was funded by Department of Public Technology, at Mälardalen University, ABB Västerås, Västmanlands Avfallsaktiebolag, Eskilstuna Energi & Miljö and the EU REFLECT project (Response of European Freshwater Lakes to Environmental and Climatic Change, contract no. ENV4-CT97-0453). Josef Hejzlar received support from the European Regional Development Fund (ERDF) and the European Social Fund (ESF) project Biomanipulation as a tool for improving water quality of dam reservoirs (grant no. CZ.02.1.01/0.0/0.0/16_025/0007417). Partial funding for this work was provided by the Israeli Ministry of Energy grant no. 215-17-017. High-frequency data from Windermere and Esthwaite Water are currently funded by the UK Natural Environment Research Council as part of the UK-SCAPE programme (grant no. refNE/R016429/1). Huaxia Yao and James A. Rusak were supported by the Ontario Ministry of the Environment, Conservation and Parks. Robert Ladwig was supported through an NSF ABI development grant (grant no. DBI 1759865). Victor M. Stepanenko is partially supported by the Russian Ministry for Higher Education and Science (contract no. 075-15-2021-1399). Publisher’s note: the article processing charges for this publication were not paid by a Russian or Belarusian institution. ISIMIP data preparation and curation is supported by the German Federal Ministry of Education and Research (BMBF) under the ISI-Access research project (grant no. 16QK05). Data from lakes Annecy, Bourget, and Geneva were collected and stored with support from the © OLA-IS, AnaEE-France, INRAE of Thonon-les-Bains, CIPEL, SILA, and CISALB (Rimet et al., 2020, https://doi.org/10.4081/jlimnol.2020.1944). Data from Lake Kivu were collected with support from Jean-Pierre Descy and the Belgian Science Policy Office through the research project EAGLES (grant no. CD/AR/02A). Alo Laas and data collection from Estonian lakes was funded by Estonian Research Council (grant nos. PSG32, PRG709, and PRG1167). Data from Lake Burley Griffin were collected by the National Capital Authority, Canberra, Australia. Dale Robertson was supported by the USGS. Fang Zhao is sponsored by the Shanghai Pujiang Program (grant no. 20PJ1403300).
This paper was edited by Charles Onyutha and reviewed by Peter Düben, Willem van Verseveld, and Bertrand Guenet.
Augusiak, J., Van den Brink, P. J., and Grimm, V.: Merging validation and evaluation of ecological models to “evaludation”: A review of terminology and a practical approach, Ecol. Modell., 280, 117–128, https://doi.org/10.1016/j.ecolmodel.2013.11.009, 2014.
Ayala, A. I., Moras, S., and Pierson, D. C.: Simulations of future changes in thermal structure of Lake Erken: proof of concept for ISIMIP2b lake sector local simulation strategy, Hydrol. Earth Syst. Sci., 24, 3311–3330, https://doi.org/10.5194/hess-24-3311-2020, 2020.
Balsamo, G., Salgado, R., Dutra, E., Boussetta, S., Stockdale, T., and Potes, M.: On the contribution of lakes in predicting near-surface temperature in a global weather forecasting model, Tellus A, 64, 15829, https://doi.org/10.3402/tellusa.v64i0.15829, 2012.
Beusen, A. H. W., Van Beek, L. P. H., Bouwman, A. F., Mogollón, J. M., and Middelburg, J. J.: Coupling global models for hydrology and nutrient loading to simulate nitrogen and phosphorus retention in surface water – description of IMAGE–GNM and analysis of performance, Geosci. Model Dev., 8, 4045–4067, https://doi.org/10.5194/gmd-8-4045-2015, 2015.
Bowling, L. C. and Lettenmaier, D. P.: Modeling the effects of lakes and wetlands on the water balance of arctic environments, J. Hydrometeorol., 11, 276–295, https://doi.org/10.1175/2009JHM1084.1, 2010.
Bruce, L. C., Frassl, M. A., Arhonditsis, G. B., Gal, G., Hamilton, D. P., Hanson, P. C., Hetherington, A. L., Melack, J. M., Read, J. S., Rinke, K., Rigosi, A., Trolle, D., Winslow, L., Adrian, R., Ayala, A. I., Bocaniov, S. A., Boehrer, B., Boon, C., Brookes, J. D., Bueche, T., Busch, B. D., Copetti, D., Cortés, A., de Eyto, E., Elliott, J. A., Gallina, N., Gilboa, Y., Guyennon, N., Huang, L., Kerimoglu, O., Lenters, J. D., MacIntyre, S., Makler-Pick, V., McBride, C. G., Moreira, S., Özkundakci, D., Pilotti, M., Rueda, F. J., Rusak, J. A., Samal, N. R., Schmid, M., Shatwell, T., Snorthheim, C., Soulignac, F., Valerio, G., van der Linden, L., Vetter, M., Vinçon-Leite, B., Wang, J., Weber, M., Wickramaratne, C., Woolway, R. I., Yao, H., and Hipsey, M. R.: A multi-lake comparative analysis of the General Lake Model (GLM): Stress-testing across a global observatory network, Environ. Model. Softw., 102, 274–291, https://doi.org/10.1016/j.envsoft.2017.11.016, 2018.
Bruggeman, J. and Bolding, K.: A general framework for aquatic biogeochemical models, Environ. Model. Softw., 61, 249–265, https://doi.org/10.1016/j.envsoft.2014.04.002, 2014.
Burchard, H., Bolding, K., and Villarreal, M. R.: GOTM, a general ocean turbulence model: theory, implementation and test cases, Space Applications Institute, Volume 18745 of EUR/European Commission, 103, https://books.google.be/books/about/GOTM_a_General_Ocean_Turbulence_Model.html?id=zsJUHAAACAAJ&redir_esc=y (last access: 23 May 2022), 1999.
Burchard, H., Bolding, K., Kühn, W., Meister, A., Neumann, T., and Umlauf, L.: Description of a flexible and extendable physical-biogeochemical model system for the water column, J. Marine Syst., 61, 180–211, 2006.
Butcher, J. B., Nover, D., Johnson, T. E., and Clark, C. M.: Sensitivity of lake thermal and mixing dynamics to climate change, Climatic Change, 129, 295–305, https://doi.org/10.1007/s10584-015-1326-1, 2015.
Choulga, M., Kourzeneva, E., Zakharova, E., and Doganovsky, A.: Estimation of the mean depth of boreal lakes for use in numerical weather prediction and climate modelling, Tellus A, 66, 21295, https://doi.org/10.3402/tellusa.v66.21295, 2014.
Cramer, W., Yohe, G. W., Auffhammer, M., Huggel, C., Molau, U., Da Silva Dias, M. A. F., Solow, A., Stone, D. A., and Tibig, L.: Detection and attribution of observed impacts, in: Climate Change 2014: Impacts, Adaptation, and Vulnerability. Part A: Global and Sectoral Aspects. Contribution of Working Group II to the Fifth Assessment Report of the Intergovernmental Panel on Climate, 979–1038, https://doi.org/10.1017/CBO9781107415379.023, 2014.
Cucchi, M., Weedon, G. P., Amici, A., Bellouin, N., Lange, S., Müller Schmied, H., Hersbach, H., and Buontempo, C.: WFDE5: bias-adjusted ERA5 reanalysis data for impact studies, Earth Syst. Sci. Data, 12, 2097–2120, https://doi.org/10.5194/essd-12-2097-2020, 2020.
Dirmeyer, P. A., Gao, X., Zhao, M., Guo, Z., Oki, T., and Hanasaki, N.: GSWP-2: Multimodel analysis and implications for our perception of the land surface, B. Am. Meteorol. Soc., 87, 1381–1397, https://doi.org/10.1175/BAMS-87-10-1381, 2006.
Dörnhöfer, K. and Oppelt, N.: Remote sensing for lake research and monitoring – Recent advances, Ecol. Indic., 64, 105–122, https://doi.org/10.1016/j.ecolind.2015.12.009, 2016.
Fairall, C. W., Bradley, E. F., Rogers, D. P., Edson, J. B., and Young, G. S.: Bulk parameterization of air‐sea fluxes for tropical ocean‐global atmosphere coupled‐ocean atmosphere response experiment, J. Geophys. Res.-Oceans, 101, 3747–3764, 1996.
Fang, C., Song, K., Paerl, H. W., Jacinthe, P.-A., Wen, Z., Liu, G., Tao, H., Xu, X., Kutser, T., Wang, Z., Duan, H., Shi, K., Shang, Y., Lyu, L., Li, S., Yang, Q., Lyu, D., Mao, D., Zhang, B., Cheng, S., and Lyu, Y.: Global divergent trends of algal blooms detected by satellite during 1982–2018, Glob. Chang. Biol., 28, 2327–2340, https://doi.org/10.1111/gcb.16077, 2022.
Frieler, K., Lange, S., Piontek, F., Reyer, C. P. O., Schewe, J., Warszawski, L., Zhao, F., Chini, L., Denvil, S., Emanuel, K., Geiger, T., Halladay, K., Hurtt, G., Mengel, M., Murakami, D., Ostberg, S., Popp, A., Riva, R., Stevanovic, M., Suzuki, T., Volkholz, J., Burke, E., Ciais, P., Ebi, K., Eddy, T. D., Elliott, J., Galbraith, E., Gosling, S. N., Hattermann, F., Hickler, T., Hinkel, J., Hof, C., Huber, V., Jägermeyr, J., Krysanova, V., Marcé, R., Müller Schmied, H., Mouratiadou, I., Pierson, D., Tittensor, D. P., Vautard, R., van Vliet, M., Biber, M. F., Betts, R. A., Bodirsky, B. L., Deryng, D., Frolking, S., Jones, C. D., Lotze, H. K., Lotze-Campen, H., Sahajpal, R., Thonicke, K., Tian, H., and Yamagata, Y.: Assessing the impacts of 1.5 ∘C global warming – simulation protocol of the Inter-Sectoral Impact Model Intercomparison Project (ISIMIP2b), Geosci. Model Dev., 10, 4321–4345, https://doi.org/10.5194/gmd-10-4321-2017, 2017.
Gädeke, A., Langer, M., Boike, J., Burke, E. J., Chang, J., Head, M., Reyer, C. P. O., Schaphoff, S., Thiery, W., and Thonicke, K.: Climate change reduces winter overland travel across the Pan-Arctic even under low-end global warming scenarios, Environ. Res. Lett., 16, 24049, https://doi.org/10.1088/1748-9326/abdcf2, 2021.
Gal, G., Yael, G., Noam, S., Moshe, E., and Schlabing, D.: Ensemble modeling of the impact of climate warming and increased frequency of extreme climatic events on the thermal characteristics of a sub-tropical lake, Water, 12, 1982, https://doi.org/10.3390/w12071982, 2020.
Gaudard, A., Råman Vinnå, L., Bärenbold, F., Schmid, M., and Bouffard, D.: Toward an open access to high-frequency lake modeling and statistics data for scientists and practitioners – the case of Swiss lakes using Simstrat v2.1, Geosci. Model Dev., 12, 3955–3974, https://doi.org/10.5194/gmd-12-3955-2019, 2019.
Goudsmit, G.-H., Burchard, H., Peeters, F., and Wüest, A.: Application of k-ϵ turbulence models to enclosed basins: The role of internal seiches, J. Geophys. Res.-Oceans, 107, 3230, https://doi.org/10.1029/2001JC000954, 2002.
Grant, L., Vanderkelen, I., Gudmundsson, L., Tan, Z., Perroud, M., Stepanenko, V. M., Debolskiy, A. V., Droppers, B., Janssen, A. B. G., Woolway, R. I., Choulga, M., Balsamo, G., Kirillin, G., Schewe, J., Zhao, F., del Valle, I. V., Golub, M., Pierson, D., Marcé, R., Seneviratne, S. I., and Thiery, W.: Attribution of global lake systems change to anthropogenic forcing, Nat. Geosci., 14, 849–854, https://doi.org/10.1038/s41561-021-00833-x, 2021.
Gudmundsson, L., Boulange, J., Do, H. X., Gosling, S. N., Grillakis, M. G., Koutroulis, A. G., Leonard, M., Liu, J., Müller Schmied, H., Papadimitriou, L., Pokhrel, Y., Seneviratne, S. I., Satoh, Y., Thiery, W., Westra, S., Zhang, X., and Zhao, F.: Globally observed trends in mean and extreme river flow attributed to climate change, Science, 371, 1159–1162, https://doi.org/10.1126/science.aba3996, 2021.
Guo, M., Zhuang, Q., Tan, Z., Shurpali, N., Juutinen, S., Kortelainen, P., and Martikainen, P. J.: Rising methane emissions from boreal lakes due to increasing ice-free days, Environ. Res. Lett., 15, 64008, https://doi.org/10.1088/1748-9326/ab8254, 2020.
Guo, M., Zhuang, Q., Yao, H., Golub, M., Leung, L. R., Pierson, D., and Tan, Z.: Validation and Sensitivity Analysis of a 1-D Lake Model Across Global Lakes, J. Geophys. Res.-Atmos., 126, e2020JD033417, https://doi.org/10.1029/2020JD033417, 2021.
Guseva, S., Bleninger, T., Jöhnk, K., Polli, B. A., Tan, Z., Thiery, W., Zhuang, Q., Rusak, J. A., Yao, H., Lorke, A., and Stepanenko, V.: Multimodel simulation of vertical gas transfer in a temperate lake, Hydrol. Earth Syst. Sci., 24, 697–715, https://doi.org/10.5194/hess-24-697-2020, 2020.
Håkanson, L.: Models to predict Secchi depth in small glacial lakes, Aquat. Sci., 57, 31–53, https://doi.org/10.1007/BF00878025, 1995.
Hansen, G. J. A., Read, J. S., Hansen, J. F., and Winslow, L. A.: Projected shifts in fish species dominance in Wisconsin lakes under climate change, Glob. Change Biol., 23, 1463–1476, https://doi.org/10.1111/gcb.13462, 2017.
Hanson, Z. J., Zwart, J. A., Jones, S. E., Hamlet, A. F., and Bolster, D.: Projected changes of regional lake hydrologic characteristics in response to 21st century climate change, Inl. Waters, 11, 335–350, https://doi.org/10.1080/20442041.2021.1924538, 2021.
Heiskanen, J. J., Mammarella, I., Ojala, A., Stepanenko, V. M., Erkkilä, K., Miettinen, H., Eugster, W., Lepparanta, M., Jarvinen, H., Vesala, T., and Nordbo, A.: Effect of water clarity on lake stratification and lake-atmosphere heat exchange, J. Geophys. Res.-Atmos., 120, 7412–7428, https://doi.org/10.1002/2014JD022938, 2015.
Hempel, S., Frieler, K., Warszawski, L., Schewe, J., and Piontek, F.: A trend-preserving bias correction – the ISI-MIP approach, Earth Syst. Dynam., 4, 219–236, https://doi.org/10.5194/esd-4-219-2013, 2013.
Henderson-Sellers, B.: New formulation of eddy diffusion thermocline models, Appl. Math. Model., 9, 441–446, https://doi.org/10.1016/0307-904X(85)90110-6, 1985.
Hipsey, M. R., Bruce, L. C., Boon, C., Busch, B., Carey, C. C., Hamilton, D. P., Hanson, P. C., Read, J. S., de Sousa, E., Weber, M., and Winslow, L. A.: A General Lake Model (GLM 3.0) for linking with high-frequency sensor data from the Global Lake Ecological Observatory Network (GLEON), Geosci. Model Dev., 12, 473–523, https://doi.org/10.5194/gmd-12-473-2019, 2019.
Hostetler, S. W.: Simulation of lake ice and its effect on the late-Pleistocene evaporation rate of Lake Lahontan, Clim. Dynam., 6, 43–48, https://doi.org/10.1007/BF00210581, 1991.
Hostetler, S. W. and Bartlein, P. J.: Simulation of lake evaporation with application to modeling lake level variations of Harney-Malheur Lake, Oregon, Water Resour. Res., 26, 2603–2612, https://doi.org/10.1029/WR026i010p02603, 1990.
Hou, X., Feng, L., Dai, Y., Hu, C., Gibson, L., Tang, J., Lee, Z., Wang, Y., Cai, X., Liu, J., Zheng, Y., and Zheng, C.: Global mapping reveals increase in lacustrine algal blooms over the past decade, Nat. Geosci., 15, 130–134, https://doi.org/10.1038/s41561-021-00887-x, 2022.
Hunt, R. J., Walker, J. F., Selbig, W. R., Westenbroek, S. M., and Regan, R. S.: Simulation of climate-change effects on streamflow, lake water budgets, and stream temperature using GSFLOW and SNTEMP, Trout Lake Watershed, Wisconsin, U.S. Geological Survey Scientific Investigations Report, 2013–5139, Reston, VA, 128 pp., https://doi.org/10.3133/sir20135159, 2013.
Imberger, J. and Patterson, J. C.: Dynamic Reservoir Simulation Model-DYRESM: 5, edited by: Fischer, H. B., 310–361, http://www.arlis.org/docs/vol2/hydropower/APA_DOC_no._534.pdf (last access: 23 May 2022), 1981.
The Inter-Sectoral Impact Model Intercomparison Project: Impact Model Settings & Characteristics, ISIMIP [code], https://www.isimip.org/impactmodels, last access: 23 May 2022.
IPCC: Summary for Policymakers, in: Global warming of 1.5 ∘C. An IPCC Special Report on the impacts of global warming of 1.5 ∘C above pre-industrial levels and related global greenhouse gas emission pathways, in the context of strengthening the global response to the threat of climate change, sustainable development, and efforts to eradicate poverty, edited by: Masson-Delmotte, V., Zhai, P., Pörtner, H. O., Roberts, D., Skea, J., Shukla, P. R., Pirani, A., Moufouma-Okia, W., Péan, C., Pidcock, R., Connors, S., Matthews, J. B. R., Chen, Y., Zhou, X., Gomis, M. I., Lonnoy, E., Maycock, T., Tignor, M., and Waterfield, T., World Meteorological Organization, Geneva, Switzerland, 32 pp., 2018.
Ito, A., Reyer, C. P. O., Gädeke, A., Ciais, P., Chang, J., Chen, M., François, L., Forrest, M., Hickler, T., Ostberg, S., Hao, S., Thiery, W., and Tian, H.: Pronounced and unavoidable impacts of low-end global warming on northern high-latitude land ecosystems, Environ. Res. Lett., 15, 44006, https://doi.org/10.1088/1748-9326/ab702b, 2020.
Jane, S. F., Hansen, G. J. A., Kraemer, B. M., Leavitt, P. R., Mincer, J. L., North, R. L., Pilla, R. M., Stetler, J. T., Williamson, C. E., Woolway, R. I., Arvola, L., Chandra, S., DeGasperi, C., Diemer, L., Dunalska, J., Erina, O., Flaim, G., Grossart, H.-P., Hambright, K. D., Hein, C., Hejzlar, J., Janus, L., Jenny, J.-P., Jones, J., Knoll, L. B., Leoni, B., MacKay, E., Matsuzaki, S.-I., McBride, C., Müller-Navarra, D. C., Paterson, A. M., Pierson, D., Rogora, M., Rusak, J., Sadro, S., Saulnier-Talbot, E., Schmid, M., Sommaruga, R., Thiery, W., Weyhenmeyer, G., Yokota, K., and Rose, K. C.: Widespread deoxygenation of temperate lakes, Nature, 594, 66–70, 2021.
Janssen, A. B. G., Arhonditsis, G. B., Beusen, A., Bolding, K., Bruce, L., Bruggeman, J., Couture, R.-M., Downing, A., Elliott, J. A., Frassl, M. A., Gal, G., Gerla, D. J., Hipsey, M. R., Hu, F., Ives, S. C., Janse, J. H., Jeppesen, E., Jöhnk, K. D., Kneis, D., Kong, X., Kuiper, J. J., Lehmann, M. K., Lemmen, C., Özkundakci, D., Petzoldt, T., Rinke, K., Robson, B., Sachse, R., Schep, S. A., Schmid, M., Scholten, H., Teurlincx, S., Trolle, D., Troost T. A., Van Dam, A. A., Van Gerven, P. A., Weijerman, M., Wells, S. A., and Mooij, W. M.: Exploring, exploiting and evolving diversity of aquatic ecosystem models: a community perspective, Aquat. Ecol., 49, 513–548, https://doi.org/10.1007/s10452-015-9544-1, 2015.
Janssen, A. B. G., Janse, J. H., Beusen, A. H. W., Chang, M., Harrison, J. A., Huttunen, I., Kong, X., Rost, J., Teurlincx, S., Troost, T. A., van Wijk, D., and Mooij, W. M.: How to model algal blooms in any lake on earth, Curr. Opin. Environ. Sustain., 36, 1–10, https://doi.org/10.1016/j.cosust.2018.09.001, 2019.
Janssen, A. B. G., Hilt, S., Kosten, S., de Klein, J. J. M., Paerl, H. W., and Van de Waal, D. B.: Shifting states, shifting services: Linking regime shifts to changes in ecosystem services of shallow lakes, Freshw. Biol., 66, 1–12, https://doi.org/10.1111/fwb.13582, 2021.
Khazaei, B., Read, L. K., Casali, M., Sampson, K. M., and Yates, D. N.: GLOBathy, the global lakes bathymetry dataset, Sci. Data, 9, 36, https://doi.org/10.1038/s41597-022-01132-9, 2022.
Kilic, L., Prigent, C., Aires, F., Boutin, J., Heygster, G., Tonboe, R. T., Roquet, H., Jimenez, C., and Donlon, C.: Expected Performances of the Copernicus Imaging Microwave Radiometer (CIMR) for an All-Weather and High Spatial Resolution Estimation of Ocean and Sea Ice Parameters, J. Geophys. Res.-Oceans, 123, 7564–7580, https://doi.org/10.1029/2018JC014408, 2018.
Kiuru, P., Ojala, A., Mammarella, I., Heiskanen, J., Erkkilä, K.-M., Miettinen, H., Vesala, T., and Huttula, T.: Applicability and consequences of the integration of alternative models for CO2 transfer velocity into a process-based lake model, Biogeosciences, 16, 3297–3317, https://doi.org/10.5194/bg-16-3297-2019, 2019.
Kobler, U. G. and Schmid, M.: Ensemble modelling of ice cover for a reservoir affected by pumped-storage operation and climate change, Hydrol. Process., 33, 2676–2690, https://doi.org/10.1002/hyp.13519, 2019.
Kraemer, B. M., Anneville, O., Chandra, S., Dix, M., Kuusisto, E., Livingstone, D. M., Rimmer, A., Schladow, G., Silow, E., Sitoki, L. M., Tamatamah, R., Vadeboncoeur, Y., and McIntyre, P. B.: Morphometry and average temperature affect lake stratification responses to climate change, Geophys. Res. Lett., 42, 4981–4988, https://doi.org/10.1002/2015GL064097, 2015.
Kraemer, B. M., Pilla, R. M., Woolway, R. I., Anneville, O., Ban, S., Colom-Montero, W., Devlin, S. P., Dokulil, M. T., Gaiser, E. E., Hambright, K. D., Hessen, D. O., Higgins, S. N., Jöhnk, K. D., Keller, W., Knoll, L. B., Leavitt, P. R., Lepori, F., Luger, M. S., Maberly, S. C., Müller-Navarra, D. C., Paterson, A. M., Pierson,, D. C., Richardson, D. C., Rogora, M., Rusak, J. A., Sadro, S., Salmaso, N., Schmid, M., Silow, E. A., Sommaruga, R., Stelzer, J. A. A., Straile, D., Thiery, W., Verburg P., Weyhenmeyer, G. A., and Adrian, R.: Climate change drives widespread shifts in lake thermal habitat, Nat. Clim. Change, 11, 521–529, 2021.
Lange, S.: EartH2Observe, WFDEI and ERA-Interim data Merged and Bias-corrected for ISIMIP (EWEMBI), V.1.1, GFZ Data Serv. [data set], https://doi.org/10.5880/pik.2019.004, 2019a.
Lange, S.: Trend-preserving bias adjustment and statistical downscaling with ISIMIP3BASD (v1.0), Geosci. Model Dev., 12, 3055–3070, https://doi.org/10.5194/gmd-12-3055-2019, 2019b.
Lange, S.: ISIMIP3 bias adjustment fact sheet, https://www.isimip.org/documents/413/ISIMIP3b_bias_adjustment_fact_sheet_Gnsz7CO.pdf (last access: 23 May 2022), 2021.
Lange, S., Volkholz, J., Geiger, T., Zhao, F., Vega, I., Veldkamp, T., Reyer, C. P. O., Warszawski, L., Huber, V., Jägermeyr, J., Schewe, J., Bresch, D. N., Büchner, M., Chang, J., Ciais, P., Dury, M., Emanuel, K., Folberth, C., Gerten, D., Gosling, S. N., Grillakis, M., Hanasaki, N., Henrot, A. J., Hickler, T., Honda, Y., Ito, A., Khabarov, N., Koutroulis, A., Liu, W., Müller, C., Nishina, K., Ostberg, S., Müller Schmied, H., Seneviratne, S. I., Stacke, T., Steinkamp, J., Thiery, W., Wada, Y., Willner, S., Yang, H., Yoshikawa, M., Yue, C., and Frieler, K.: Projecting Exposure to Extreme Climate Impact Events Across Six Event Categories and Three Spatial Scales, Earth's Future, 8, e2020EF001616, https://doi.org/10.1029/2020EF001616, 2020.
Larssen, T., Høgåsen, T., and Cosby, B. J.: Impact of time series data on calibration and prediction uncertainty for a deterministic hydrogeochemical model, Ecol. Modell., 207, 22–33, https://doi.org/10.1016/j.ecolmodel.2007.03.016, 2007.
Lawrence, D. M., Oleson, K. W., Flanner, M. G., Thornton, P. E., Swenson, S. C., Lawrence, P. J., Zeng, X., Yang, Z.-L., Levis, S., Sakaguchi, K., Bonan, G. B., and Slater, A. G.: Parameterization improvements and functional and structural advances in version 4 of the Community Land Model, J. Adv. Model. Earth Syst., 3, M03001, https://doi.org/10.1029/2011MS00045, 2011.
Lehner, B. and Döll, P.: Development and validation of a global database of lakes, reservoirs and wetlands, J. Hydrol., 296, 1–22, https://doi.org/10.1016/j.jhydrol.2004.03.028, 2004.
Lehner, B. and Messager, M.: HydroLAKES, Technical Documentation Version 1.0, 16 pp., https://www.hydrosheds.org/products/hydrolakes (last access: 23 May 2022), 2016.
Magee, M. R., Wu, C. H., Robertson, D. M., Lathrop, R. C., and Hamilton, D. P.: Trends and abrupt changes in 104 years of ice cover and water temperature in a dimictic lake in response to air temperature, wind speed, and water clarity drivers, Hydrol. Earth Syst. Sci., 20, 1681–1702, https://doi.org/10.5194/hess-20-1681-2016, 2016.
Marcé, R., George, G., Buscarinu, P., Deidda, M., Dunalska, J., de Eyto, E., Flaim, G., Grossart, H.-P., Istvanovics, V., Lenhardt, M., Moreno-Ostos, E., Obrador, B., Ostrovsky, I., Pierson, D. C., Potužák, J., Poikane, S., Rinke, K., Rodríguez-Mozaz, S., Staehr, P. A., Šumberová, K., Waajen, G., Weyhenmeyer, G. A., Weathers, K. C., Zion, M., Ibelings, B. W., and Jennings, E.: Automatic high frequency monitoring for improved lake and reservoir management, Environ. Sci. & Technol., 50, 10780–10794, 2016.
Marcé, R., Pierson, D., Mercado-Bettin, D., Thiery, W., Tan, Z., and Schewe, J.: ISIMIP2a Simulation Data from the Global Lakes Sector (v1.0), ISIMIP Repository [data set], https://doi.org/10.48364/ISIMIP.530719, 2022a.
Marcé, R., Pierson, D., Mercado-Bettin, D., Thiery, W., Tan, Z., Seneviratne, S., Golub, M., Debolskiy, A., Stepanenko, V., Perroud, M., Droppers, B., and Schewe, J.: ISIMIP2b Simulation Data from the Global Lakes Sector (v1.0), ISIMIP Repository [data set], https://doi.org/10.48364/ISIMIP.931371, 2022b.
Marcé, R., Pierson, D., Mercado-Bettin, D., Thiery, W., Piccolroaz, S., Woodward, B., Woolway, R. I., Tan, Z., Kirillin, G., Shatwell, T., Couture, R.-M., Côté, M., Bouffard, D., Råman Vinnå, C. L. M., Schmid, M., and Schewe, J.: ISIMIP2b Simulation Data from the Local Lakes Sector (v1.0), ISIMIP Repository [data set], https://doi.org/10.48364/ISIMIP.563533, 2022c.
Marcé, R., Mercado, D., Vanderkelen, I., Tigli, M., Janssen, A. B. G., Kraemer, B., Piccolroaz, S., Thiery, W., Woolway, R. I., Yaghouti, M., and Pierson, D.: Inputs for lake morphology in ISIMIP3 runs of the global lake sector [Data set]. In Geoscientific Model Development (ISIMIP3_LS_v2022), Zenodo [data set], https://doi.org/10.5281/zenodo.6457813, 2022d.
Markelov, I., Couture, R. M., Fischer, R., Haande, S., and Van Cappellen, P.: Coupling Water Column and Sediment Biogeochemical Dynamics: Modeling Internal Phosphorus Loading, Climate Change Responses, and Mitigation Measures in Lake Vansjø, Norway, J. Geophys. Res.-Biogeo., 124, 3847–3866, https://doi.org/10.1029/2019JG005254, 2019.
Mengel, M., Treu, S., Lange, S., and Frieler, K.: ATTRICI v1.1 – counterfactual climate for impact attribution, Geosci. Model Dev., 14, 5269–5284, https://doi.org/10.5194/gmd-14-5269-2021, 2021.
Mesman, J. P., Ayala, A. I., Adrian, R., De Eyto, E., Frassl, M. A., Goyette, S., Kasparian, J., Perroud, M., Stelzer, J. A. A., Pierson, D. C., and Ibelings, B. W.: Performance of one-dimensional hydrodynamic lake models during short-term extreme weather events, Environ. Model. & Softw., 133, 104852, https://doi.org/10.1016/j.envsoft.2020.104852, 2020.
Messager, M. L., Lehner, B., Grill, G., Nedeva, I., and Schmitt, O.: Estimating the volume and age of water stored in global lakes using a geo-statistical approach, Nat. Commun., 7, 13603, https://doi.org/10.1038/ncomms13603, 2016.
Mironov, D. V: Parameterization of lakes in numerical weather prediction. Part 1: Description of a lake model, COSMO Technical Report 11, 47 pp., https://cosmo-model.org/content/model/documentation/techReports/cosmo/docs/techReport11.pdf (last access: 23 May 2022), 2008.
Moe, S. J., Haande, S., and Couture, R. M.: Climate change, cyanobacteria blooms and ecological status of lakes: A Bayesian network approach, Ecol. Modell., 337, 330–347, https://doi.org/10.1016/j.ecolmodel.2016.07.004, 2016.
Moras, S., Ayala, A. I., and Pierson, D. C.: Historical modelling of changes in Lake Erken thermal conditions, Hydrol. Earth Syst. Sci., 23, 5001–5016, https://doi.org/10.5194/hess-23-5001-2019, 2019.
Murfitt, J. and Duguay, C. R.: 50 years of lake ice research from active microwave remote sensing: Progress and prospects, Remote Sens. Environ., 264, 112616, https://doi.org/10.1016/j.rse.2021.112616, 2021.
Oleson, K. W., Lawrence, D. M., Bonan, G. B., Drewniak, B., Huang, M., Charles, D., Levis, S., Li, F., Riley, W. J., Zachary, M., Swenson, S. C., Thornton, P. E., Bozbiyik, A., Fisher, R., Heald, C. L., Kluzek, E., Lamarque, F., Lawrence, P. J., Leung, L. R., Muszala, S., Ricciuto, D. M., and Sacks, W.: Technical description of version 4.5 of the Community Land Model (CLM), NCAR Technical Note, 420 pp., https://opensky.ucar.edu/islandora/object/technotes:515/ (last access: 23 May 2022), 2013.
O'Reilly, C. M., Sharma, S., Gray, D. K., et al.: Rapid and highly variable warming of lake surface waters around the globe, Geophys. Res. Lett., 42, 10–773, https://doi.org/10.1002/2015GL066235, 2015.
Patterson, J. C. and Hamblin, P. F.: Thermal simulation of a lake with winter ice cover, Limnol. Oceanogr., 33, 323–338, https://doi.org/10.4319/lo.1988.33.3.0323, 1988.
Perroud, M., Goyette, S., Martynov, A., Beniston, M., and Anneville, O.: Simulation of multiannual thermal profiles in deep Lake Geneva: A comparison of one-dimensional lake models, Limnol. Oceanogr., 54, 1574–1594, https://doi.org/10.4319/lo.2009.54.5.1574, 2009.
Piccolroaz, S.: Prediction of lake surface temperature using the air2water model: guidelines, challenges, and future perspectives, Adv. Oceanogr. Limnol., 7, https://doi.org/10.4081/aiol.2016.5791, 2016.
Piccolroaz, S., Toffolon, M., and Majone, B.: A simple lumped model to convert air temperature into surface water temperature in lakes, Hydrol. Earth Syst. Sci., 17, 3323–3338, https://doi.org/10.5194/hess-17-3323-2013, 2013.
Piccolroaz, S., Healey, N. C., Lenters, J. D., Schladow, S. G., Hook, S. J., Sahoo, G. B., and Toffolon, M.: On the predictability of lake surface temperature using air temperature in a changing climate: A case study for Lake Tahoe (U.S.A.), Limnol. Oceanogr., 63, 243–261, https://doi.org/10.1002/lno.10626, 2018.
Piccolroaz, S., Woolway, R. I., and Merchant, C. J.: Global reconstruction of twentieth century lake surface water temperature reveals different warming trends depending on the climatic zone, Climatic Change, 160, 427–442, https://doi.org/10.1007/s10584-020-02663-z, 2020.
Pilla, R. M. and Couture, R. M.: Attenuation of photosynthetically active radiation and ultraviolet radiation in response to changing dissolved organic carbon in browning lakes: Modeling and parametrization, Limnol. Oceanogr., 66, 2278–2289, https://doi.org/10.1002/lno.11753, 2021.
Pilla, R. M., Williamson, C. E., Adamovich, B. V, et al.: Deeper waters are changing less consistently than surface waters in a global analysis of 102 lakes, Sci. Rep., 10, 20514, https://doi.org/10.1038/s41598-020-76873-x, 2020.
Pilla, R. M., Mette, E. M., Williamson, C. E., et al.: Global data set of long-term summertime vertical temperature profiles in 153 lakes, Sci. Data, 8, 200, https://doi.org/10.1038/s41597-021-00983-y, 2021.
Pokhrel, Y., Felfelani, F., Satoh, Y., Boulange, J., Burek, P., Gädeke, A., Gerten, D., Gosling, S. N., Grillakis, M., Gudmundsson, L., Hanasaki, N., Kim, H., Koutroulis, A., Liu, J., Papadimitriou, L., Schewe, J., Müller Schmied, H., Stacke, T., Telteu, C. E., Thiery, W., Veldkamp, T., Zhao, F., and Wada, Y.: Global terrestrial water storage and drought severity under climate change, Nat. Clim. Change, 11, 226–233, 2021.
Poole, H. H. and Atkins, W. R. G.: Photo-electric measurements of submarine illumination throughout the year, J. Mar. Biol. Assoc. UK, 16, 297–324, https://doi.org/10.1017/S0025315400029829, 1929.
Potes, M., Costa, M. J., and Salgado, R.: Satellite remote sensing of water turbidity in Alqueva reservoir and implications on lake modelling, Hydrol. Earth Syst. Sci., 16, 1623–1633, https://doi.org/10.5194/hess-16-1623-2012, 2012.
Prats, J. and Danis, P.-A.: An epilimnion and hypolimnion temperature model based on air temperature and lake characteristics, Knowl. Manag. Aquat. Ec., 8, 420, https://doi.org/10.1051/kmae/2019001, 2019.
Rand, J. M., Nanko, M. O., Lykkegaard, M. B., Wain, D., King, W., Bryant, L. D., and Hunter, A.: The human factor: Weather bias in manual lake water quality monitoring, Limnol. Oceanogr. Methods, 20, 288–303, https://doi.org/10.1002/lom3.10488, 2022.
Reid, A. J., Carlson, A. K., Creed, I. F., Eliason, E. J., Gell, P. A., Johnson, P. T. J., Kidd, K. A., MacCormack, T. J., Olden, J. D., Ormerod, S. J., Smol, J. P., Taylor, W. W., Tockner, K., Vermaire, J. C., Dudgeon, D., and Cooke, S. J.: Emerging threats and persistent conservation challenges for freshwater biodiversity, Biol. Rev., 94, 849–873, https://doi.org/10.1111/brv.12480, 2019.
Reinecke, R., Müller Schmied, H., Trautmann, T., Andersen, L. S., Burek, P., Flörke, M., Gosling, S. N., Grillakis, M., Hanasaki, N., Koutroulis, A., Pokhrel, Y., Thiery, W., Wada, Y., Yusuke, S., and Döll, P.: Uncertainty of simulated groundwater recharge at different global warming levels: a global-scale multi-model ensemble study, Hydrol. Earth Syst. Sci., 25, 787–810, https://doi.org/10.5194/hess-25-787-2021, 2021.
Rimet, F., Anneville, O., Barbet, D., Chardon, C., Crépin, L., Domaizon, I., Dorioz, J. M., Espinat, L., Frossard, V., Guillard, J., Goulon, C., Hamelet, V., Hustache, J. C., Jacquet, S., Lainé, L., Montuelle, B., Perney, P., Quetin, P., Rasconi, S., Schellenberger, A., Tran-Khac, V., and Monet, G.: The Observatory on LAkes (OLA) database: Sixty years of environmental data accessible to the public, J. Limnol., 79, 164–178, https://doi.org/10.4081/jlimnol.2020.1944, 2020.
Sachse, R., Petzoldt, T., Blumstock, M., Moreira, S., Pätzig, M., Rücker, J., Janse, J. H., Mooij, W. M., and Hilt, S.: Extending one-dimensional models for deep lakes to simulate the impact of submerged macrophytes on water quality, Environ. Model. Softw., 61, 410–423, https://doi.org/10.1016/j.envsoft.2014.05.023, 2014.
Saloranta, T. M. and Andersen, T.: MyLake-A multi-year lake simulation model code suitable for uncertainty and sensitivity analysis simulations, Ecol. Modell., 207, 45–60, https://doi.org/10.1016/j.ecolmodel.2007.03.018, 2007.
Schewe, J., Gosling, S. N., Reyer, C., Zhao, F., Ciais, P., Elliott, J., Francois, L., Huber, V., Lotze, H. K., Seneviratne, S. I., van Vliet, M. T. H., Vautard, R., Wada, Y., Breuer, L., Büchner, M., Carozza, D. A., Chang, J., Coll, M., Deryng, D., de Wit, A., Eddy, T. D., Folberth, C., Frieler, K., Friend, A. D., Gerten, D., Gudmundsson, L., Hanasaki, N., Ito, A., Khabarov, N., Kim, H., Lawrence, P., Morfopoulos, C., Müller, C., Müller Schmied, H., Orth, R., Ostberg, S., Pokhrel, Y., Pugh, T. A. M., Sakurai, G., Satoh, Y., Schmid, E., Stacke, T., Steenbeek, J., Steinkamp, J., Tang, Q., Tian, H., Tittensor, D. P., Volkholz, J., Wang, X., and Warszawski, L.: State-of-the-art global models underestimate impacts from climate extremes, Nat. Commun., 10, 1005, https://doi.org/10.1038/s41467-019-08745-6, 2019.
Schleussner, C. F., Deryng, D., Müller, C., Elliott, J., Saeed, F., Folberth, C., Liu, W., Wang, X., Pugh, T. A. M., Thiery, W., Seneviratne, S. I., and Rogelj, J.: Crop productivity changes in 1.5 ∘C and 2 ∘C worlds under climate sensitivity uncertainty, Environ. Res. Lett., 13, 064007, https://doi.org/10.1088/1748-9326/aab63b, 2018.
Schwefel, R., Gaudard, A., Wüest, A., and Bouffard, D.: Effects of climate change on deepwater oxygen and winter mixing in a deep lake (Lake Geneva): Comparing observational findings and modeling, Water Resour. Res., 52, 8811–8826, https://doi.org/10.1002/2016WR019194, 2016.
Settele, J., Scholes, R., Betts, R. A., Bunn, S., Leadley, P., Nepstad, D., Overpeck, J. T., and Taboada, M. A.: Terrestrial and Inland water systems, in: Climate Change 2014 Impacts, Adaptation and Vulnerability: Part A: Global and Sectoral Aspects, 271–360, https://doi.org/10.1017/CBO9781107415379.009, 2015.
Sharma, S., Gray, D. K., Read, J. S., O'Reilly, C. M., Schneider, P., Qudrat, A., Gries, C., Stefanoff, S., Hampton, S. E., Hook, S., Lenters, J. D., Livingstone, D. M., McIntyre, P. B., Adrian, R., Allan, M. G., Anneville, O., Arvola, L., Austin, J., Bailey, J., Baron, J. S., Brookes, J., Chen, Y., Daly, R., Dokulil, M., Dong, B., Ewing, K., De Eyto, E., Hamilton, D., Havens, K., Haydon, S., Hetzenauer, H., Heneberry, J., Hetherington, A. L., Higgins, S. N., Hixson, E., Izmest'eva, L. R., Jones, B. M., Kangur, K., Kasprzak, P., Köster, O., Kraemer, B. M., Kumagai, M., Kuusisto, E., Leshkevich, G., May, L., MacIntyre, S., Müller-Navarra, D., Naumenko, M., Noges, P., Noges, T., Niederhauser, P., North, R. P., Paterson, A. M., Plisnier, P. D., Rigosi, A., Rimmer, A., Rogora, M., Rudstam, L., Rusak, J. A., Salmaso, N., Samal, N. R., Schindler, D. E., Schladow, G., Schmidt, S. R., Schultz, T., Silow, E. A., Straile, D., Teubner, K., Verburg, P., Voutilainen, A., Watkinson, A., Weyhenmeyer, G. A., Williamson, C. E., and Woo, K. H.: A global database of lake surface temperatures collected by in situ and satellite methods from 1985-2009, Sci. Data, 2, 150008, https://doi.org/10.1038/sdata.2015.8, 2015.
Sharma, S., Blagrave, K., Magnuson, J. J., O'Reilly, C. M., Oliver, S., Batt, R. D., Magee, M. R., Straile, D., Weyhenmeyer, G. A., Winslow, L., and Woolway, R. I.: Widespread loss of lake ice around the Northern Hemisphere in a warming world, Nat. Clim. Change, 9, 227–231, https://doi.org/10.1038/s41558-018-0393-5, 2019.
Shatwell, T., Thiery, W., and Kirillin, G.: Future projections of temperature and mixing regime of European temperate lakes, Hydrol. Earth Syst. Sci., 23, 1533–1551, https://doi.org/10.5194/hess-23-1533-2019, 2019.
Shen, H., Tolson, B. A., and Mai, J.: Time to Update the Split-Sample Approach in Hydrological Model Calibration, Water Resour. Res., 58, e2021WR031523, https://doi.org/10.1029/2021wr031523, 2022.
Spence, C., Blanken, P. D., Lenters, J. D., and Hedstrom, N.: The Importance of Spring and Autumn Atmospheric Conditions for the Evaporation Regime of Lake Superior, J. Hydrometeorol., 14, 1647–1658, https://doi.org/10.1175/JHM-D-12-0170.1, 2013.
Stepanenko, V., Jöhnk, K. D., and Machulskaya, E.: Simulation of surface energy fluxes and stratification of a small boreal lake by a set of one-dimensional models, Tellus A, 66, 21389, https://doi.org/10.3402/tellusa.v66.21389, 2014.
Stepanenko, V., Mammarella, I., Ojala, A., Miettinen, H., Lykosov, V., and Vesala, T.: LAKE 2.0: a model for temperature, methane, carbon dioxide and oxygen dynamics in lakes, Geosci. Model Dev., 9, 1977–2006, https://doi.org/10.5194/gmd-9-1977-2016, 2016.
Stepanenko, V. M., Goyette, S., Martynov, A., Perroud, M., Fang, X., and Mironov, D.: First steps of a Lake Model Intercomparison Project: LakeMIP, Boreal Environ. Res., 15, 191–202, 2010.
Stepanenko, V. M., Martynov, A., Jöhnk, K. D., Subin, Z. M., Perroud, M., Fang, X., Beyrich, F., Mironov, D., and Goyette, S.: A one-dimensional model intercomparison study of thermal regime of a shallow, turbid midlatitude lake, Geosci. Model Dev., 6, 1337–1352, https://doi.org/10.5194/gmd-6-1337-2013, 2013.
Strokal, M., Kroeze, C., Wang, M., Bai, Z., and Ma, L.: The MARINA model (Model to Assess River Inputs of Nutrients to seAs): Model description and results for China, Sci. Total Environ., 562, 869–888, https://doi.org/10.1016/j.scitotenv.2016.04.071, 2016.
Subin, Z. M., Riley, W. J., and Mironov, D.: An improved lake model for climate simulations: model structure, evaluation, and sensitivity analyses in CESM1, J. Adv. Model. Earth Syst., 4, M02001, https://doi.org/10.1029/2011MS000072, 2012.
Tan, Z., Zhuang, Q., and Walter Anthony, K.: Modeling methane emissions from arctic lakes: Model development and site-level study, J. Adv. Model. Earth Syst., 7, 459–483, https://doi.org/10.1002/2014MS000344, 2015.
Tan, Z., Zhuang, Q., Shurpali, N. J., Marushchak, M. E., Biasi, C., Eugster, W., and Walter Anthony, K.: Modeling CO2 emissions from Arctic lakes: Model development and site-level study, J. Adv. Model. Earth Syst., 9, 2190–2213, https://doi.org/10.1002/2017MS001028, 2017.
Tan, Z., Yao, H., and Zhuang, Q.: A Small Temperate Lake in the 21st Century: Dynamics of Water Temperature, Ice Phenology, Dissolved Oxygen, and Chlorophyll a, Water Resour. Res., 54, 4681–4699, 2018.
Taylor, K. E., Stouffer, R. J., and Meehl, G. A.: An Overview of CMIP5 and the Experiment Design, B. Am. Meteorol. Soc., 93, 485–498, https://doi.org/10.1175/BAMS-D-11-00094.1, 2012.
Thiery, W., Stepanenko, V. M., Fang, X., Jöhnk, K. D., Li, Z., Martynov, A., Perroud, M., Subin, Z. M., Darchambeau, F., Mironov, D., and Van Lipzig, N. P. M.: LakeMIP Kivu: Evaluating the representation of a large, deep tropical lake by a set of one-dimensional lake models, Tellus A, 66, 21390, https://doi.org/10.3402/tellusa.v66.21390, 2014a.
Thiery, W., Martynov, A., Darchambeau, F., Descy, J.-P., Plisnier, P.-D., Sushama, L., and van Lipzig, N. P. M.: Understanding the performance of the FLake model over two African Great Lakes, Geosci. Model Dev., 7, 317–337, https://doi.org/10.5194/gmd-7-317-2014, 2014b.
Thiery, W., Davin, E. L., Panitz, H. J., Demuzere, M., Lhermitte, S., and Van Lipzig, N.: The impact of the African Great Lakes on the regional climate, J. Climate, 28, 4061–4085, https://doi.org/10.1175/JCLI-D-14-00565.1, 2015.
Thiery, W., Davin, E. L., Seneviratne, S. I., Bedka, K., Lhermitte, S., and van Lipzig, N. P. M.: Hazardous thunderstorm intensification over Lake Victoria, Nat. Commun., 7, 12786, https://doi.org/10.1038/ncomms12786, 2016.
Thiery, W., Gudmundsson, L., Bedka, K., Semazzi, F. H. M., Lhermitte, S., Willems, P., van Lipzig, N. P. M., and Seneviratne, S. I.: Early warnings of hazardous thunderstorms over Lake Victoria, Env. Res. Letters, 12, 074012, https://doi.org/10.1088/1748-9326/aa7521, 2017.
Thiery, W., Lange, S., Rogelj, J., Schleussner, C. F., Gudmundsson, L., Seneviratne, S. I., Andrijevic, M., Frieler, K., Emanuel, K., Geiger, T., Bresch, D. N., Zhao, F., Willner, S. N., Büchner, M., Volkholz, J., Bauer, N., Chang, J., Ciais, P., Dury, M., François, L., Grillakis, M., Gosling, S. N., Hanasaki, N., Hickler, T., Huber, V., Ito, A., Jägermeyr, J., Khabarov, N., Koutroulis, A., Liu, W., Lutz, W., Mengel, M., Müller, C., Ostberg, S., Reyer, C. P. O., Stacke, T., and Wada, Y.: Intergenerational inequities in exposure to climate extremes, Science, 374, 158–160, https://doi.org/10.1126/science.abi7339, 2021.
Toffolon, M., Piccolroaz, S., Majone, B., Soja, A. M., Peeters, F., Schmid, M., and Wüest, A.: Prediction of surface temperature in lakes with different morphology using air temperature, Limnol. Oceanogr., 59, 2185–2202, https://doi.org/10.4319/lo.2014.59.6.2185, 2014.
Toptunova, O., Choulga, M., and Kurzeneva, E.: Status and progress in global lake database developments, Adv. Sci. Res., 16, 57–61, https://doi.org/10.5194/asr-16-57-2019, 2019.
Trolle, D., Elliott, J. A., Mooij, W. M., Janse, J. H., Bolding, K., Hamilton, D. P., and Jeppesen, E.: Advancing projections of phytoplankton responses to climate change through ensemble modelling, Environ. Model. Softw., 61, 371–379, 2014.
Umlauf, L. and Lemmin, U.: Interbasin exchange and mixing in the hypolimnion of a large lake: The role of long internal waves, Limnol. Oceanogr., 50, 1601–1611, 2005.
Vanderkelen, I. and Schewe, J.: ISIMIP3a lakes input data, ISIMIP Repository [data set], https://doi.org/10.48364/ISIMIP.263794.1, 2020a.
Vanderkelen, I. and Schewe, J.: ISIMIP3b lakes input data, ISIMIP Repository [data set], https://doi.org/10.48364/ISIMIP.383948, 2020b.
Vanderkelen, I., van Lipzig, N. P. M., Lawrence, D. M., Droppers, B., Golub, M., Gosling, S. N., Janssen, A. B. G., Marcé, R., Schmied, H. M., Perroud, M., Pierson, D., Pokhrel, Y., Satoh, Y., Schewe, J., Seneviratne, S. I., Stepanenko, V. M., Tan, Z., Woolway, R. I., and Thiery, W.: Global Heat Uptake by Inland Waters, Geophys. Res. Lett., 47, e2020GL087867, https://doi.org/10.1029/2020GL087867, 2020.
Vanderkelen, I., van Lipzig, N. P. M., Sacks, W. J., Lawrence, D. M., Clark, M. P., Mizukami, N., Pokhrel, Y., and Thiery, W.: Simulating the Impact of Global Reservoir Expansion on the Present-Day Climate, J. Geophys. Res.-Atmos., 126, e2020JD034485, https://doi.org/10.1029/2020JD034485, 2021.
Verpoorter, C., Kutser, T., Seekell, D. A., and Tranvik, L. J.: A global inventory of lakes based on high-resolution satellite imagery, Geophys. Res. Lett., 41, 6396–6402, https://doi.org/10.1002/2014GL060641, 2014.
Wang, W., Lee, X., Xiao, W., Liu, S., Schultz, N., Wang, Y., Zhang, M., and Zhao, L.: Global lake evaporation accelerated by changes in surface energy allocation in a warmer climate, Nat. Geosci., 11, 410–414, https://doi.org/10.1038/s41561-018-0114-8, 2018.
Wang, X., Qiu, Y., Zhang, Y., Lemmetyinen, J., Cheng, B., Liang, W., and Leppäranta, M.: A lake ice phenology dataset for the Northern Hemisphere based on passive microwave remote sensing, Big Earth Data, 1–19, https://doi.org/10.1080/20964471.2021.1992916, 2021.
Weathers, K., Hanson, P. C., Arzberger, P., Brentrup, J., Brookes, J. D., Carey, C. C., Gaiser, E., Hamilton, D. P., Hong, G. S., Ibelings, B. W., Istvánovics, V., Jennings, E., Kim, B., Kratz, T., Lin, F.-P., Muraoka, K., O'Reilly, C., Piccolo, C., Ryder, E., and Zhu, G.: The Global Lake Ecological Observatory Network (GLEON): the evolution of grassroots network science, Limnol. Oceanogr. Bull., 22, 71–73, 2013.
Weyhenmeyer, G. A., Livingstone, D. M., Meili, M., Jensen, O., Benson, B. J., and Magnuson, J. J.: Large geographical differences in the sensitivity of ice-covered lakes and rivers in the Northern Hemisphere to temperature changes, Glob. Change Biol., 17, 268–275, https://doi.org/10.1111/j.1365-2486.2010.02249.x, 2011.
Winslow, L. A., Read, J. S., Hansen, G. J. A., Rose, K. C., and Robertson, D. M.: Seasonality of change: Summer warming rates do not fully represent effects of climate change on lake temperatures, Limnol. Oceanogr., 62, 2168–2178, https://doi.org/10.1002/lno.10557, 2017.
Woolway, R. I. and Merchant, C. J.: Worldwide alteration of lake mixing regimes in response to climate change, Nat. Geosci., 12, 271–276, https://doi.org/10.1038/s41561-019-0322-x, 2019.
Woolway, R. I., Merchant, C. J., Van Den Hoek, J., Azorin-Molina, C., Nõges, P., Laas, A., Mackay, E. B., and Jones, I. D.: Northern Hemisphere Atmospheric Stilling Accelerates Lake Thermal Responses to a Warming World, Geophys. Res. Lett., 46, 11983–11992, https://doi.org/10.1029/2019GL082752, 2019a.
Woolway, R. I., Weyhenmeyer, G. A., Schmid, M., Dokulil, M. T., de Eyto, E., Maberly, S. C., May, L., and Merchant, C. J.: Substantial increase in minimum lake surface temperatures under climate change, Climatic Change, 155, 81–94, https://doi.org/10.1007/s10584-019-02465-y, 2019b.
Woolway, R. I., Kraemer, B. M., Lenters, J. D., Merchant, C. J., O'Reilly, C. M., and Sharma, S.: Global lake responses to climate change, Nat. Rev. Earth Environ., 1, 388–403, https://doi.org/10.1038/s43017-020-0067-5, 2020.
Woolway, R. I., Jennings, E., Shatwell, T., Golub, M., Pierson, D. C., and Maberly, S. C.: Lake heatwaves under climate change, Nature, 589, 402–407, https://doi.org/10.1038/s41586-020-03119-1, 2021a.
Woolway, R. I., Sharma, S., Weyhenmeyer, G. A., Debolskiy, A., Golub, M., Mercado-Bettín, D., Perroud, M., Stepanenko, V., Tan, Z., Grant, L., Ladwig, R., Mesman, J., Moore, T. N., Shatwell, T., Vanderkelen, I., Austin, J. A., DeGasperi, C. L., Dokulil, M., La Fuente, S., Mackay, E. B., Schladow, S. G., Watanabe, S., Marcé, R., Pierson, D. C., Thiery, W., and Jennings, E.: Phenological shifts in lake stratification under climate change, Nat. Commun., 12, 2318, https://doi.org/10.1038/s41467-021-22657-4, 2021b.
Woolway, R. I., Albergel, C., Frölicher, T. L., and Perroud, M.: Severe Lake Heatwaves Attributable to Human-Induced Global Warming, Geophys. Res. Lett., 49, e2021GL097031, https://doi.org/10.1029/2021gl097031, 2022.
Zwart, J. A., Hanson, Z. J., Read, J. S., Fienen, M. N., Hamlet, A. F., Bolster, D., and Jones, S. E.: Cross-Scale Interactions Dictate Regional Lake Carbon Flux and Productivity Response to Future Climate, Geophys. Res. Lett., 46, 8840–8851, https://doi.org/10.1029/2019GL083478, 2019.
The requested paper has a corresponding corrigendum published. Please read the corrigendum first before downloading the article.
- Article
(2225 KB) - Full-text XML
- Corrigendum
-
Supplement
(368 KB) - BibTeX
- EndNote