the Creative Commons Attribution 4.0 License.
the Creative Commons Attribution 4.0 License.
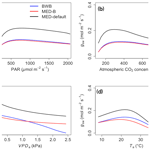
Implementation and evaluation of the unified stomatal optimization approach in the Functionally Assembled Terrestrial Ecosystem Simulator (FATES)
Shawn P. Serbin
Julien Lamour
Kenneth J. Davidson
Kim S. Ely
Alistair Rogers
Stomata play a central role in regulating the exchange of carbon dioxide and water vapor between ecosystems and the atmosphere. Their function is represented in land surface models (LSMs) by conductance models. The Functionally Assembled Terrestrial Ecosystem Simulator (FATES) is a dynamic vegetation demography model that can simulate both detailed plant demographic and physiological dynamics. To evaluate the effect of stomatal conductance model formulation on forest water and carbon fluxes in FATES, we implemented an optimality-based stomatal conductance model – the Medlyn (MED) model – that simulates the relationship between photosynthesis (A) and stomatal conductance to water vapor (gsw) as an alternative to the FATES default Ball–Woodrow–Berry (BWB) model. To evaluate how the behavior of FATES is affected by stomatal model choice, we conducted a model sensitivity analysis to explore the response of gsw to climate forcing, including atmospheric CO2 concentration, air temperature, radiation, and vapor pressure deficit in the air (VPDa). We found that modeled gsw values varied greatly between the BWB and MED formulations due to the different default stomatal slope parameters (g1). After harmonizing g1 and holding the stomatal intercept parameter (g0) constant for both model formulations, we found that the divergence in modeled gsw was limited to conditions when the VPDa exceeded 1.5 kPa. We then evaluated model simulation results against measurements from a wet evergreen forest in Panama. Results showed that both the MED and BWB model formulations were able to capture the magnitude and diurnal changes of measured gsw and A but underestimated both by about 30 % when the soil was predicted to be very dry. Comparison of modeled soil water content from FATES to a reanalysis product showed that FATES captured soil drying well, but translation of drying soil to modeled physiology reduced the models' ability to match observations. Our study suggests that the parameterization of stomatal conductance models and current model response to drought are the critical areas for improving model simulation of CO2 and water fluxes in tropical forests.
- Article
(699 KB) -
Supplement
(1053 KB) - BibTeX
- EndNote
Global climate change has resulted in significant modifications to Earth's ecosystems through changing weather patterns, including an increased frequency and severity of extreme drought and heatwaves, which has resulted in increased risk for terrestrial vegetation (Pachauri et al., 2014; Reichstein et al., 2013; Gatti et al., 2021). The exchange of water vapor and carbon dioxide between plants and the atmosphere is dominated by transport through stomata (Hetherington and Woodward, 2003; Kala et al., 2016). The mechanisms regulating stomatal opening involve complex biochemical and biophysical processes that are currently not represented in land surface models (LSMs) (Lawson et al., 2014; Buckley and Mott, 2013; Blatt, 2000; Davies et al., 2002). However, a range of much simpler, largely empirical formulations that describe the responses of stomata to their environment have been successfully used by LSMs for many years. Most of them require only two parameters, i.e., the intercept parameter (g0), which is the conductance when photosynthesis (A) is zero, and the slope parameter (g1) that describes the relationship between stomatal conductance to water vapor (gsw) and a regressor term that includes A and environmental drivers (Damour et al., 2010; Berry et al., 2010). The most widely used representation of gsw, and the default formulation used in the Functionally Assembled Terrestrial Ecosystem Simulator (FATES), is the Ball–Woodrow–Berry model (BWB, Ball et al., 1987), wherein gsw is based on an empirical relationship with leaf net photosynthesis (Anet), carbon dioxide concentration at the leaf surface (Cs), and relative humidity at the leaf surface (Hs) as Eq. (1):
The optimality-based unified stomatal conductance model (Medlyn model, MED, Medlyn et al., 2011) is based on the assumption that plants will attempt to maximize carbon gain while minimizing water loss (Cowan and Farquhar, 1977). The MED model has been proposed as an alternative representation of gsw in LSMs (De Kauwe et al., 2015; Lawrence et al., 2019). The basic functional form of the MED model is shown in Eq. (2). One important difference between the BWB and MED formulations is that gsw responds to vapor pressure deficit at the leaf surface (VPDs) instead of Hs:
Although the functional form of the MED model is similar to the BWB model, g1 is based on underlying optimization theory and has a strong theoretical link to plant water use efficiency. The MED model is also favored by many plant physiologists given that gsw responds to VPDs rather than Hs (Rogers et al., 2017a). Importantly, the g1 parameter has also been found to vary significantly across a wide range of different plant functional types (PFTs) and climate regions (Lin et al., 2015). Better representation of gsw in LSMs requires efforts to improve the fidelity of g1 parametrization by PFT. The g1 parameter can be estimated through field measurement campaigns (Lin et al., 2015; Wu et al., 2020) or model inversion (Bonan et al., 2011; Fer et al., 2018). For example, De Kauwe et al. (2015) derived a PFT-specific g1 parameterization from Lin et al. (2015) for the CABLE model and found a significant reduction in annual fluxes of transpiration using MED compared with the original model formulation of CABLE (Leuning, 1995). Despite considerable analysis supporting the adoption of the MED model in LSMs (De Kauwe et al., 2015; Lawrence et al., 2019), the formulation has not been widely adopted and is not common in dynamic vegetation models (Fisher et al., 2018).
Exploring plant physiological responses to key environmental variables is emerging as a promising way to understand model representation and evaluate model behaviors (Rogers et al., 2017a; Bonan et al., 2011). Stomatal conductance in the MED and BWB models responds to direct environmental drivers including atmospheric CO2 concentration and VPDs or Hs, as well as indirect drivers like radiation and leaf surface temperature (via photosynthesis) (Franks et al., 2017). Evaluating the BWB and MED formulations in response to changing climate in a complete LSM, wherein atmospheric, ecological, and hydrologic processes are highly coupled, is urgently needed to understand model responses within a larger domain.
Improved projection of the response of ecosystems to global climate change requires improved understanding and model representations of plant responses to a hotter, drier, and CO2-enriched future (Sullivan et al., 2020). To mimic the drought effects on ecosystems, some models have included a soil water stress factor (often denoted as β), which is used to reduce the “base rate” of stomatal model parameters: either g0 (e.g., CLM, Lawrence et al., 2019), g1 (e.g., G'DAY, Comins and McMurtrie, 1993; O-CN, Zaehle and Friend, 2010; CABLE, De Kauwe et al., 2015), or both (e.g., ORCHIDEE, Guimberteau et al., 2018). In some cases, it is also used to lower the maximum carboxylation rate of Rubisco (Vcmax) (e.g., CLM; O-CN; SIBCASA, Schaefer et al., 2008), both Vcmax and the maximum rate of electron transport (Jmax) (e.g., G'DAY), or directly A (e.g., JULES, Best et al., 2011; Clark et al., 2011). Reduction in A will further reduce gsw. Some models also consider the soil water stress on mesophyll conductance (e.g., SIBCASA; ORCHIDEE). However, the application of a β factor to different physiological parameters has not been evaluated against measurements for gsw models. Therefore, evaluating different gsw schemes and parameterization with data collected under normal and stressed conditions may help reveal areas for model improvement.
In this study, we explored the impact of stomatal behavior under simulated and realistic environmental conditions in the FATES model (Koven et al., 2020), into which we implemented the MED formulation as an alternative approach to the default BWB formulation. The FATES model is a dynamic vegetation demography model that simulates leaf to ecosystem-scale carbon, water, and energy fluxes, as well as cohort-level plant growth, competition, and mortality processes, enabling FATES to predict the distribution, structure, and composition of vegetation (Fisher et al., 2015; Koven et al., 2020). FATES itself is not a stand-alone model, but instead is used in conjunction with a host land model, and is currently coupled with the Community Land Model (CLM, Lawrence et al., 2019) and the Energy Exascale Earth System Model (E3SM) Land Model (ELM, Holm et al., 2020). Using FATES and the MED and BWB representations we addressed the following questions: (1) how do projected leaf-level and canopy-level CO2 and water vapor fluxes differ between the BWB and MED formulations in response to key meteorological forcing variables? (2) How do the two model outputs of stomatal conductance and photosynthesis compare to leaf-level gas exchange measurements collected through a dry season in a tropical forest? (3) How does the application of a soil water stress factor affect the simulation of water and carbon cycles during dry periods in a tropical forest?
2.1 Implementation of the Medlyn model into FATES
In FATES, leaf-level photosynthesis (A) in C3 plants is based on the model of Farquhar et al. (1980) as modified by Collatz et al. (1991). A is calculated as the minimum of the RuBP-carboxylase-limited (Rubisco-limited) rate and RuBP regeneration rate (i.e., the light-limited rate). The net photosynthesis rate (Anet) is the difference between A and leaf respiration. Gross primary productivity (GPP) is calculated as the weighted average of the photosynthetic rate from sunlit and shaded leaves, which is integrated through the vertical profile, and finally across all the leaf layers by multiplying exposed leaf area for a given cohort. A cohort is a group of plants with similar disturbance history, height, and PFT type. The leaf area of each cohort is calculated from leaf biomass and specific leaf area (SLA). Leaf biomass is controlled by the processes of phenology, allocation, and turnover. SLA is a PFT-specific parameter.
We implemented the MED stomatal conductance model as an alternative to the BWB model for the calculation of gsw in FATES. Leaf-level gsw is central to the water, CO2, and energy cycles in forests. It not only controls the water and CO2 exchange, but also modifies the energy balance and biochemical processes. Similarly, in FATES, the variable gsw is used to model several processes such as the heat and water transfer and photosynthesis. The calculation of this variable is therefore complex and uses both analytical and numerical solutions to couple the equations describing each process. A detailed description of the implementation can be found in online documentation (FATES Development Team, 2020b). It should be noted that parameters g0 and Vcmax used to calculate Anet in Eqs. (1) and (2) are multiplied by an empirical soil moisture stress factor (β) by default in the FATES model. The β factor ranges from 1 when the soil is wet to zero when the soil is dry. The β factor depends on the soil water potential of each soil layer, the root distribution of the PFT, and a plant-dependent response to soil water stress as shown in Eq. (3):
where wj is a plant wilting factor for layer j and rj is the fraction of roots in layer j. The soil wilting factor is a bounded linear function of soil matric potential defined by two parameters, the soil water potential at (and above) which stomata are fully open and the value at which stomata are fully closed. The soil matric potential is related to the soil water content, soil texture, and organic matter content. The root fraction is determined by PFT-specific root distribution parameters. For more details on the calculation of the plant wilting factor and the fraction of roots, see the CLM version 4.5 (CLM4.5) technical note (Oleson et al., 2013).
2.2 The San Lorenzo, Panama, model test-bed site
Our model simulations were made for a single tropical forest located in Bosque Protector San Lorenzo, Panama (9∘16′51.71′′ N, 79∘58′28.27′′ W, elevation 25 m), which is a part of the Center for Tropical Forest Science (CTFS) – Forest Global Earth Observatory (ForestGEO). The Smithsonian Tropical Research Institute canopy crane provides access to the top of the forest canopy and allows us to compare our simulations with previous measurements of stomatal conductance and net photosynthesis rate (Wu et al., 2020; Rogers et al., 2017c). The site is characterized as a moist tropical forest, with mean annual temperature of 26 ∘C, with only small seasonal variation. The mean annual precipitation is 3300 mm, and 90 % of this precipitation falls during the wet season (May–December). More details about the site can be found in Wright et al. (2003).
For our study, we conducted all model simulations using the FATES model coupled with the CLM version 5.0.34 (CLM5). For all simulations, we initialized the FATES model using real-world forest inventory data that provided information on tree size distribution for the whole forest area (Condit et al., 2009) and enabled us to better compare model outputs with field measurements by matching the internal cohort structure with that observed in inventory data. Inventory data from the most recent census (1999) were used as the initial state for the simulations. For simplicity, in our FATES simulations we assumed that the site is populated entirely by the broadleaf evergreen tropical (BET) tree plant functional type.
2.3 Sensitivity simulations of FATES with synthetic forcing
The MED and BWB stomatal conductance models differ in the representation of atmospheric dryness as well as the g1 values. To isolate the influence of structural and parametric differences on FATES simulations using the MED and BWB stomatal models, we employed three model ensemble simulations associated with a BET tree PFT.
For the BWB configuration we used the BWB model with a default g1 value of 8 (unitless) for the BET tree PFT in FATES. In our MED-default setup, the MED model was parameterized with g1 set to 4.1 kPa0.5 to match the best estimate from Lin et al. (2015). To constrain the model difference to structural difference we also ran FATES with the MED model with a g1 value that was harmonized with the BWB model in FATES, which was abbreviated as MED-B. Here, we assumed g1 for BWB (g1b) to be 8, air temperature to be 25∘, and relative humidity in the air (Ha) to be 0.8 following Franks et al. (2017) in Eq. (4) to obtain a BWB-equivalent g1=2.39 kPa0.5 in the MED-B simulation (g1m, Eq. 4), wherein VPDa is VPD in the air. For all simulations, g0 was fixed at 1000 µmol m−2 s−1.
The FATES model is driven by half-hourly longwave radiation, shortwave radiation, air temperature, specific humidity, precipitation, surface pressure, wind speed, and atmospheric CO2 concentration. These variables modify the leaf conductance by changing the environment at the leaf surface (Hs, VPDs, and Cs in Eqs. 1 and 2). Following model initialization, the model was run with our synthetic climate forcing data in order to reveal model responses to specific climate forcing. For our synthetic climate forcing, each represented the scenarios of a linear increase in VPDa, air temperature (Ta), photosynthetically active radiation (PAR), and atmospheric CO2 concentration (CO2), respectively, while other climate forcing data were kept as constant. The details for these scenarios are listed in Table 1. In addition, we set the precipitation to 1.3 mm d−1, surface pressure to 99 626 Pa, wind speed to 4.8 m s−1, and longwave radiation to 407.4 W m−2 for all these scenarios, which represent annual average conditions at our field site. Given the physical dependence of saturated water vapor on Ta (Ficklin and Novick, 2017), it was necessary to adjust the specific humidity together with Ta to keep the VPDa fixed at 1 kPa for the MED-default and MED-B simulations. For the Ta scenario in the BWB model, Ha was kept at 80 % as Ha rather than VPDa was used in the BWB model (Franks et al., 2017). We then studied the responses of gsw, net photosynthesis (Anet), gross primary productivity (GPP), and evapotranspiration (ET) to these drivers for the top layer (averaged across sunlit and shaded leaves) of the canopy. We also checked the number of plants per hectare (nplant) to ensure that cohort density did not change during our simulations.
2.4 Evaluation of FATES against in situ measurements
We compared the modeled diurnal gsw and Anet of upper canopy layers with measured values (Rogers et al., 2017c; Wu et al., 2020). The data were collected at the San Lorenzo field site at monthly intervals across the dry season and the beginning of the wet season during the strong El Niño–Southern Oscillation (ENSO) year of 2016. The gsw and Anet of top-of-canopy leaves of eight species, which all belonged to the BET PFT, were measured across 4 months starting in February 2016 and ending in May 2016. Measurements of gsw and Anet were made with an LI-6400 (LI-COR Biosciences, Lincoln, NE, USA) for which the conditions of radiation, humidity, CO2 concentration, and temperature surrounding the leaves were closely matched to the ambient conditions. Using this dataset, the g1 values of the BWB and MED models were estimated for each species (see Table 2 in Wu et al., 2020). We used those estimations to parametrize the gsw model in FATES, and we used their gsw and Anet measurements to compare with FATES simulation results. It should be noted that the g1 values we used were varied between 4.43 to 8.3 for the BWB model and between 1.14 and 2.85 kPa0.5 for the MED model; most values were lower than the defaults for evergreen tropical trees in both of the models, as discussed in Wu et al. (2020). Because g1 was estimated for BWB and MED models based on the same measurements, g1 was equivalent for the two models and the simulations resemble MED-B and BWB in Sect. 2.3. An ensemble of simulations with varying measured species-specific g1 values was carried out to evaluate the impact of stomatal slope parameterization on FATES-simulated gsw and Anet. In addition, Vcmax at 25∘ was set to 63 µmol m−2 s−1 based on the curves measured at the same time during the 2016 campaign (Rogers et al., 2017b). Other parameters such as Jmax and leaf dark respiration rate (Rdark) at 25∘ were directly calculated by FATES based on their relationship with Vcmax or leaf nitrogen content.
For our simulations, we used the observed half-hourly weather data including precipitation, air temperature, and humidity from the field site meteorological station as the atmospheric forcing data to drive the FATES simulations (Faybishenko et al., 2019). Atmospheric CO2 concentration was set to a background level of 403.3 ppm based on data from the NOAA Mauna Loa observatory, which is also very close to the CO2 concentration inside the leaf chamber of the gas exchange equipment.
2.5 Drought effects on physiological parameters
In FATES, a soil water stress factor (β) is used to adjust g0 and Vcmax in the original form of the BWB model (Bonan et al., 2011). For the MED approach we implemented, we also applied the β factor in the same manner as the default setting (Sect. 2.1). However, whether the calculation of the β factor can truly reflect soil water conditions is unclear. To the best of our knowledge, the relevance of the β factor has not been rigorously tested for tropical ecosystems or in comparison with measured gsw and Anet. We therefore first compared the modeled soil water content and β factor against soil moisture products from the ECMWF Reanalysis data version 5 (ERA5) (Hersbach et al., 2018). Then we explored whether this formulation of the β factor accurately represents observed physiological responses to soil water stress and whether the stress factor should also be applied to g1 for both the BWB and MED models. To test this, we designed model simulations (Table 2) to assess how the inclusion of the β factor modifies modeled gsw and Anet and the comparison with the measurements. In these simulations, g1 and Vcmax were set as the averages across all the species measured for the BET PFT.
3.1 Model responses to climatic drivers
The responses of gsw and Anet to climatic forcing as modeled by the BWB (BWB model with default g1) and MED-default (MED model with default g1) simulations had similar shapes (Figs. 1 and 2, blue and black lines). The MED-default yielded markedly higher gsw than BWB for all climatic drivers considered with an average difference of 75 % (Fig. 1). The MED-default simulation also resulted in higher estimations of Anet, but the increase over the BWB simulation was much smaller (around 15 % on average) than the increase in gsw (Fig. 2). When the VPDa increased above 1.5 kPa, the two models showed strong additional divergence. At a VPDa of 2.0 kPa projected gsw and Anet from the BWB simulation were 316 % and 86 % lower than projections from the MED-default simulation (Figs. 1c and 2c).
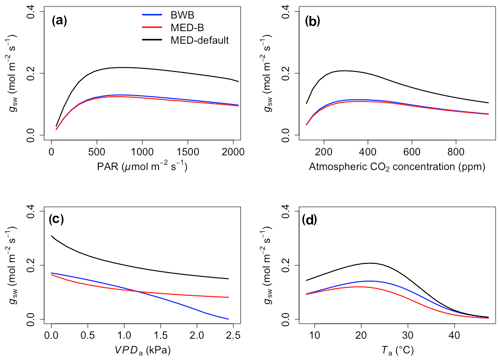
Figure 1The responses of stomatal conductance (gsw) to scenarios with (a) radiation, (b) CO2, (c) VPDa, and (d) Ta for the three model setups: BWB (blue), MED-B (red), and MED-default (black).
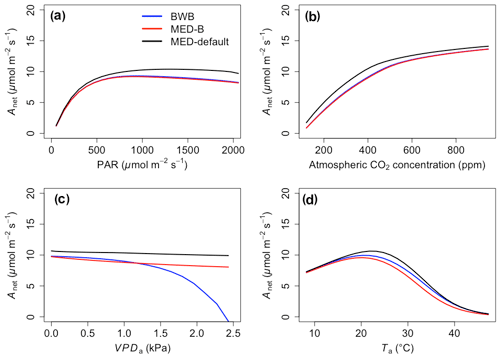
Figure 2The responses of net photosynthesis (Anet) to scenarios with (a) radiation, (b) CO2, (c) VPDa, and (d) Ta for the three model setups: BWB (blue), MED-B (red), and MED-default (black).
The particularly large divergence between the BWB and the MED-default simulations can be explained by a combination of parametric and structural differences. Comparison of the MED-B (for which we used a parameterization equivalent to that of BWB in the MED model) with the BWB limited potential model deviation to structural difference between the two approaches. Both simulations yielded similar responses of gsw and Anet to radiation, temperature, and CO2 (Figs. 1a, b, d and 2a, b, d; blue and red lines), demonstrating that the differences between the BWB and MED-default settings were attributable to the difference in parameterization associated with g1. With a harmonized parameterization of g1 the divergence between the two models above a VPDa of 1.5 kPa was still readily apparent (Figs. 1c and 2c, blue and red lines). The MED-B simulation showed a slight decrease in gsw with high VPDa, while gsw modeled with the BWB simulation decreased more markedly when VPDa was beyond 1.5 kPa. At 2.0 kPa the BWB simulation projected gsw and Anet that were 126 % and 53 % lower than the MED-B simulation. For the temperature response of gsw, BWB and MED-B were very similar although BWB had slightly higher gsw values than MED-B (Fig. 1d, blue and red lines).
In contrast to the gsw response, the differences between BWB and MED-default were generally smaller for Anet, except when VPDa was above 1.5 kPa (Fig. 2, blue and black lines). The use of measured g1 for the MED model (MED-default) did not markedly change the magnitude of Anet compared with MED-B (Fig. 2, red and black lines). When we explored the ecosystem-scale responses (Figs. 3 and 4), we found that the patterns of ET and GPP mirrored the leaf-level responses described above when using our synthetic climatic drivers. The difference between BWB and MED-B was also apparent when VPDa was above 1.5 kPa (Figs. 3c and 4c, blue and red lines).
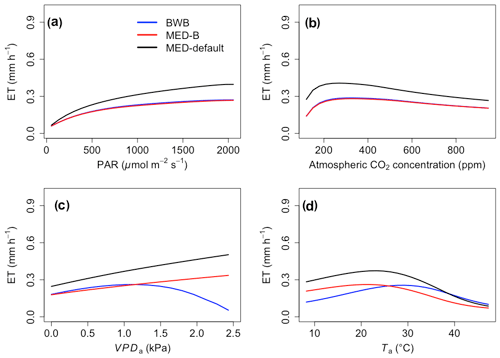
Figure 3The responses of evapotranspiration (ET) to scenarios with (a) radiation, (b) CO2, (c) VPDa, and (d) Ta for the three model setups: BWB (blue), MED-B (red), and MED-default (black).
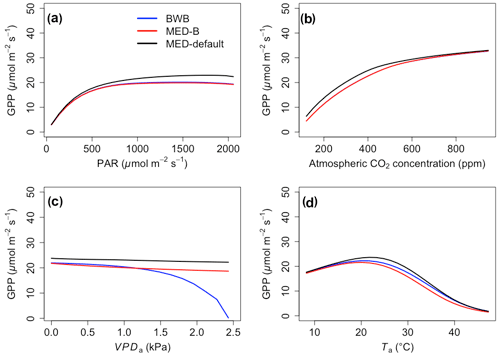
Figure 4The responses of gross primary productivity (GPP) to scenarios with (a) radiation, (b) CO2, (c) VPDa, and (d) Ta for the three model setups: BWB (blue), MED-B (red), and MED-default (black).
To rule out the possibility that these differences were related to changes in underlying plant community structure, we looked for any significant changes in cohort density (number of plants per hectare). Our results showed that there was no significant change (Fig. S1); thus, these ecosystem-scale responses were primarily related to changes in underlying leaf-level physiology.
3.2 Model evaluation against field measurements
Before comparing the results of the BWB and MED model representations within FATES against field measurements, we first evaluated the consistency of the site meteorological measurements used to drive FATES simulations with those measured with our gas exchange instruments during the campaign. We anticipated that the two conditions would be comparable as the environmental controls in the gas exchange instruments were set to mimic the ambient conditions just before the leaf measurements. We found that for PAR, Ha, and CO2 concentration, the atmospheric and leaf chamber conditions at the time of measurements were in reasonably close agreement, while the in situ measured Ta and VPDa were higher than climate data in all months (Fig. 5a–d).
To account for measurement and natural variability of g1 across different species, we ran a series of FATES simulations driven by meteorological forcing data with different g1 values. These experiments showed that FATES Anet and gsw were sensitive to different g1 values for both model formulations (Fig. 5e–l). The MED model ensemble results for Anet and gsw with different g1 values, represented as the envelopes in Fig. 5e–l, generally overlapped with those from the BWB model, with comparable averages. Compared with field measurements, both models captured the diurnal patterns well (Fig. S2) but tended to underestimate Anet and gsw, notably in the month of April by about 30 %, at the peak of the dry season (Fig. 5g and k).
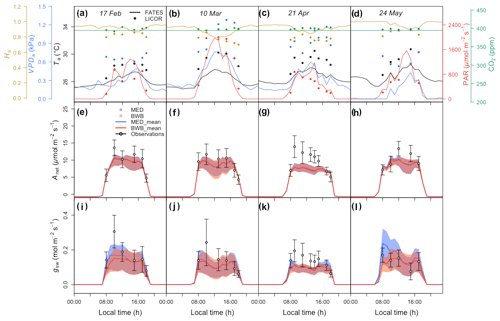
Figure 5(a–d) Diurnal change in climate forcing, (e–h) model–data comparison of net photosynthesis rate (Anet), and (i–l) model–data comparison of stomatal conductance (gsw) for four field campaign dates. In panels (a)–(d), lines and filled points represent climate forcing data used in FATES and in situ measurements, respectively. Different colors are for different types: black for Ta, red for PAR, blue for VPDa, green for atmospheric CO2 concentration, and gold for Ha. In panels (e)–(l) shaded areas represent the range of FATES model ensemble results with different measured g1 values for different species, while lines represent the averages of these ensemble results. Blue shaded areas and lines are for results from the MED model, and red is for the BWB model. Gray filled circles for the measured data represent averages across species. Black error bars for the measured data represent the 95 % confidence interval (CI) across species. Columns correspond to days of measurements and are presented in chronological order for 17 February, 10 March, 21 April, and 24 May 2016.
3.3 Water stress factor on physiological parameters
Compared with the ERA5 soil moisture products, FATES generally captured the magnitude and trend of the observed average soil water content at the San Lorenzo site (Fig. 6). FATES also simulated the soil water content well for different layers of the soil column (Fig. S3). By April 2016, at the peak of the dry season in a dry year, the simulated soil moisture stress factor (averaged over all the soil layers) reached an annual minimum (0.7), corresponding to the observed soil moisture drying trend (Fig. 6). FATES also underestimated gsw and Anet by the largest margin in April when compared to our field measurements (Fig. 5g and k). To explore this further, we conducted additional experiments focused on evaluating the use of the β factor to modify g0, g1, and Vcmax. For the month of April in 2016, we compared a range of different model simulation experiments wherein the β factor was applied in different combinations to g0, g1, and Vcmax (Table 2, Fig. 7). The results from Exp. 1 and Exp. 4 showed high overlap, indicating that considering the β effect on g0 does not influence modeled carbon and water fluxes. However, when applied to Vcmax the β factor reduced gsw and Anet by 15 %–20 % (Exp. 2 vs. Exp. 3, Fig. 7). Applying the β factor to g1 also reduced gsw and Anet by 10 %–50 % (Exp. 1 vs. Exp. 3, Fig. 7). Unsurprisingly, comparing model results with β applied to all or no parameters showed the largest differences (30 %–80 %) (Exp. 2 vs. Exp. 4, Fig. 7). Default simulations with the β factor on g0 and Vcmax underestimated Anet by 29 % and gsw by 26 % for the MED model. However, the results from simulations with no β effects or with β only applied to g0 (Exps. 1 and 4) corresponded best to the observations, in which Anet was only underestimated by 15 % and gsw by 9 % for the MED model (Fig. 7a and c). There was also a significant improvement of performance when the β effects were removed from the equation in the BWB model (Fig. 7b and d).
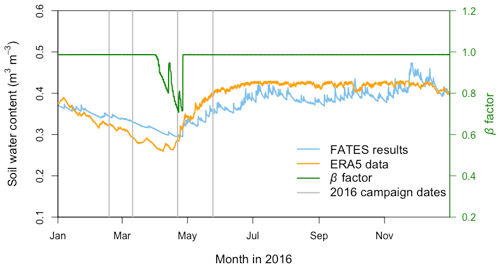
Figure 6Annual cycle of the modeled volumetric soil water content (blue line) and corresponding soil water stress (β) factor (green line) from the FATES simulation as well as ERA5 reanalysis soil water content data (orange line) at the San Lorenzo field site in 2016. The soil water content data are means across all soil layers. For the β factor, 1 represents fully saturated soil, while 0 represents very dry soil. Vertical gray lines indicate the four campaign dates in 2016.
4.1 Advances in understanding model difference
We implemented the MED stomatal model in FATES and compared model projections of CO2 and water vapor exchange to the existing BWB formulation. The two models diverged considerably in the responses of both leaf-level (gsw and Anet, Figs. 1 and 2) and canopy-level (ET and GPP, Figs. 3 and 4) fluxes to a wide range of radiation, air temperature, VPDa, and CO2 concentrations with the default stomatal slope parameter (g1). When parameterization of g1 was harmonized between the MED and BWB formulations, the difference was much smaller in responses to varying radiation, temperature, and CO2 conditions but were markedly apparent at VPDa above 1.5 kPa.
Our analysis of the general model responses to synthetic climate forcing presents some advantages over previous evaluations. First, some studies found that different stomatal conductance models varied considerably in water-limited regions (Knauer et al., 2015; Morales et al., 2005) but were unable to attribute the difference to specific climate forcing as all factors, such as temperature and humidity, are closely related (Galbraith et al., 2010; Rowland et al., 2015). In their recent experimental study of a tropical forest, Smith et al. (2020) found that stomatal response to VPDa, rather than to Ta, is the primary mechanism for high-temperature photosynthetic declines in tropical forests by separating the temperature effect and VPDa effect. This observation, along with our findings, highlights the need to improve the representation of stomatal conductance response to VPDa in models. Second, most previous modeling studies relied on evaluating model performance against benchmarks such as eddy covariance data and remote sensing products (De Kauwe et al., 2015), which were limited to the current climate conditions and ecoregions. To test model behaviors under all possible climate change scenarios, our studies designed simulations driven by a wide range of climate forcing data. Third, understanding model response to synthetic climate forcing (Figs. 1–4) is a powerful diagnostic tool because the model outputs can be evaluated in comparison to known and measurable physiological responses to environmental variation, such as radiation and CO2. The model outputs of GPP and ET also provide insight into how leaf-level responses influence the emergent ecosystem-scale responses, which is relevant for forecasting the responses of ecosystems and biomes to climate change. Fourth, by using the calibrated and default parameters to run the models, we were also able to separate effects of model structure (i.e., stomatal model choice) and parameterization (i.e., g0 and g1) on model differences.
As highlighted previously by Franks et al. (2017), the influence of parameterization dominated potential differences of gsw and ET due to model choice, further emphasizing the need to develop robust approaches to estimate g1 and understand covariance with environmental drivers, such as soil moisture availability, and other leaf traits that may facilitate the use of trait–trait or trait–environment relationships to enable model parameterization (De Kauwe et al., 2015; Héroult et al., 2013; Lin et al., 2015; Wu et al., 2020). However, different g1 values did not markedly change the magnitudes of Anet and GPP, suggesting that the difference of g1 propagates to the simulation of intercellular CO2 first and finally to Anet with attenuated effects. The structural difference is attributable to the different representation of humidity in the BWB and MED models (i.e., Hs vs. VPDs) and is consistent with previous studies (Rogers et al., 2017a; Knauer et al., 2015; Franks et al., 2017). gsw simulated by the power function of the MED model decreases hyperbolically, while that simulated by the linear function of the BWB model drops steeply. The nonlinear response of gsw to VPDa when using the MED model is supported by some observations (Marchin et al., 2016; Héroult et al., 2013; Wang et al., 2009; Domingues et al., 2014), but more measurements of leaf-level VPDa responses would be valuable. Our results suggest that when implemented in a dynamic vegetation demography model (FATES) the choice of stomatal model only has a small effect on projections of leaf- and canopy-level CO2 and water vapor fluxes under conditions of VPDa below 1.5 kPa. Under higher VPDa, higher gsw values were simulated using the MED model compared with the BWB model and led to higher Anet, ET, and GPP. This suggests that the MED formulation would predict tropical evergreen broadleaf forests to be more resistant to extreme atmospheric drought than with the BWB formulation. As the global surface temperature is projected to increase, the VPDa is also expected to increase (Ficklin and Novick, 2017; Yuan et al., 2019; Kolby Smith et al., 2016). Therefore, the difference between the two models under high VPDa conditions will lead to radically different ecosystem carbon and water dynamics under future climate change scenarios.
4.2 Model responses under simulated water stress
Our field campaign, which occurred during the 2016 ENSO event, enables us to evaluate model performance under various climate conditions, including extreme drought. Overall, simulations made with FATES with both gsw models captured the dynamics of measured upper canopy leaf-level fluxes well, confirming the utility of the current stomatal conductance models in LSMs for non-stressed conditions.
However, at the peak of the dry season this underestimation of gsw and Anet was notable and resulted in part from application of a soil water stress (β) factor used to modify leaf physiology in response to reduced soil moisture content. In FATES, the β factor affects g0 and Vcmax through an empirical modification. Experimental evidence about how physiological parameters change in response to soil water conditions is diverse. Some previous studies found that g1 was relatively stable under water stress (Gimeno et al., 2016; De La Motte et al., 2020; Xu and Baldocchi, 2003). Other studies found a range of responses of g1 to drought across different plant species (Miner and Bauerle, 2017; Zhou et al., 2013). For g0, it was reported to decrease under water stress (Miner and Bauerle, 2017; Misson et al., 2004) but also to show no response to drought (Barnard and Bauerle, 2013). Drought nearly universally lowered Vcmax in plants (Zhou et al., 2013). However, some argued that effects of mild and moderate droughts on Vcmax were negligible (Aranda et al., 2012; Bota et al., 2004; Cano et al., 2013), and others showed a range of responses resulting in a 10 %–25 % reduction in Vcmax (Galmés et al., 2007; Grassi and Magnani, 2005; Keenan et al., 2010; Limousin et al., 2010; Misson et al., 2010; Wilson et al., 2000; Zait and Schwartz, 2018).
Despite previous extensive experimental studies of the β effects on plant physiological parameters, understanding of the results of applying β effects in models is still inadequate. The uncertainty of the β calculation is a major challenge. Based on the equations, the β factor is a function of soil water content, modified by parameters related to plant response, root distribution, and soil properties. Due to the lack of in situ measurements, we only used general parameters for the β factor in the simulations. Although soil moisture content was relatively well simulated (Fig. 6), root fraction and other soil properties were difficult to constrain due to scarce observations. In our study, by toggling on and off the β effects on stomatal and photosynthetic parameters, we were able to learn more about how the calculation of β influences model outputs. Overall, we found that the predictions of gsw and Anet were closer to the measurements when the β factor was treated as 1 (i.e., no stress). Similar studies also found that the implementation of the β factor in the CLM overestimated the drought-related productivity loss compared with the observations, biased the transpiration rate, or lacked diurnal variability (Powell et al., 2013; Kennedy et al., 2019; Bonan et al., 2014). To improve models, further systematic evaluation of the β effects on photosynthetic capacity, stomatal conductance, and mesophyll conductance in LSMs is highly recommended (Egea et al., 2011; Vidale et al., 2021). More mechanistic approaches such as representation of hydraulic limitations and chemical signaling through abscisic acid (ABA) are emerging as promising ways to represent the plant response to drought in LSMs but come with significant added complexity (Verhoef and Egea, 2014; Sperry and Love, 2015; Kennedy et al., 2019).
4.3 Implications for evaluating model performance
Our FATES sensitivity analysis used synthetic meteorological forcing to enable us to isolate the impacts of individual abiotic drivers on model behaviors. By adjusting the specific humidity concurrently with air temperature, we were able to isolate the model response to changing air temperature from typically concurrent change in VPDa. We believe that our sensitivity analysis should be included as a routine approach for evaluating changes in model behavior during model development activities. Using similar simulations regularly during development would provide a powerful check on unexpected or unintended changes related to any changes in structure or parameters.
When the models are driven by synthetic climate forcing, special attention should be paid to the change in the environment conditions at the leaf surface, to which plants directly respond. In most LSMs, leaf surface temperature (Tl) is the balance of environmental drivers and leaf biophysical activities, and it is one of the most important variables regulating leaf biochemical responses such as photosynthesis and respiration (Kumarathunge et al., 2019; Leuning, 2002). But as Tl is an emergent variable in FATES we could only control Ta rather than Tl in our sensitivity analysis simulations. In scenarios with changing radiation, although we have fixed Ta, Tl was also increasing (Fig. S4a), which resulted in slight decreasing trends of gsw and Anet in response to radiation as Tl exceeded the temperature optimum of gsw and Anet (Figs. 1d and 2d). But the influence of Tl change was limited for other response curves (Fig. S4).
Parameterization of g0 has been shown to be critical for predicting ecosystem fluxes (De Kauwe et al., 2015; Barnard and Bauerle, 2013). However, there is little agreement on how to parameterize g0 due to different definitions and measurement approaches for this parameter. Whether g0 should be an intercept from data fitting, a minimum threshold when Anet approaches zero, a nighttime gsw, or the cuticular conductance is still an active research topic (Lombardozzi et al., 2017; Duursma et al., 2019; Lamour et al., 2022; Davidson et al., 2022). The slope parameter g1 we used in the model was from Lin et al. (2015), estimated with the assumption that g0 was zero. In our implementation, we not only included a nonzero g0 in the numerical calculation of gsw, but also set a small positive value for g0 to prevent gsw becoming zero or negative when Anet approaches zero. In this way, the leaf stomatal resistance (i.e., the reverse of gsw) will not become infinitive during the simulations. To understand how different g0 values influence gsw and Anet, we tested the sensitivity of gsw, Anet, ET, and GPP to different g0 values with our synthetic climate forcing listed in Table 1. In addition to the simulations with our default value (1000 µmol m−2 s−1), the g0 was set zero or the commonly adopted value of 10 000 µmol m−2 s−1 (Sellers et al., 1996). A comparison of g0=0 and g0=1000 µmol mol−1 showed a very minor effect on the model response of gsw. Using the 10-fold larger estimate for g0 (10 000 µmol mol−1) only resulted in a small effect on the magnitudes of gsw, Anet, ET, and GPP (Figs. S5–S8).
For the model evaluation against site-level measurements, we found it is necessary to check the consistency of climate forcing used in models and that measured by the instruments (Héroult et al., 2013). In our study, the in situ measured Ta and VPDa were higher than those recorded by a nearby meteorological station (Fig. 5a–d). The mismatch was partially related to the challenge of matching leaf chamber conditions with ambient conditions, avoiding condensation in the leaf chamber, or the use of a pump to move air across the leaf surface during gas exchange measurements. The slight deviation of modeled gsw and Anet against measurements when soil was relatively wet (measured in February, March, and May) can be partly attributed to the mismatch of Ta and VPDa used in the model compared with the in situ measurements. In this study we evaluated the inclusion of the MED model in FATES in a tropical forest. However, future efforts could include evaluations at sites of different ecosystem types and at regional and global scales for carbon and water cycles, particularly at sites where VPDa routinely rises above 1.5 kPa.
Implementing new plant physiological theories, such as the optimal stomatal conductance model, into dynamic vegetation models is crucial to keep the models up to date and to enable the exploration of new behaviors and capacities to understand potential ecosystem responses to global change. In this study, we added the optimality-based Medlyn model into the state-of-the-art dynamic vegetation model FATES as an alternative to the default Ball–Woodrow–Berry model and then tested model behaviors in response to key independent climate forcing. Our model evaluation demonstrated that the major difference between the two models was caused by the parameterization of the stomatal slope parameter (g1). When parameters were harmonized, the potential for markedly different projections of water vapor and CO2 fluxes between stomatal conductance models only occurred as VPDa rose above 1.5 kPa. We also compared model performance with gas exchange measurements from an evergreen tropical forest. Modeled CO2 and water vapor fluxes in the dry season of a drought year were similar between models and closely matched observations, except at the peak of the dry season when a soil moisture correction factor was used to adjust physiological parameters. After removing this adjustment, projections for both models improved. Our study showed that the parameterization of g1 and the application of the correction factor associated with decreasing soil moisture content are the key targets for improving model representation of CO2 and water fluxes in tropical forests.
The FATES model is available at https://github.com/NGEET/fates (last access: 30 May 2020) (https://doi.org/10.5281/zenodo.3825474, FATES Development Team, 2020b). The specific FATES version used in this study is the one that merged the Medlyn model with git commit “9a4627a”, and the version corresponds to tag “sci.1.37.0_api.11.2.0” (https://doi.org/10.5281/zenodo.5851984, FATES Development Team, 2020a). FATES was run here within CLM5. The latest release version of CLM5 is available at https://github.com/ESCOMP/ctsm (last access: 30 May 2020) (https://doi.org/10.5281/zenodo.3779821, CTSM Development Team, 2020), which is also the version used in this study. Scripts to run all the model experiments, create synthetic climate forcing, and analyze model outputs are available at https://github.com/Qianyuxuan/Scripts_for_papers/tree/main/Medlyn_model (last access: 16 January 2022) (https://doi.org/10.5281/zenodo.5854740, Li and Serbin, 2022).
All the model outputs and observation data utilized to produce the results used in this paper are archived at https://doi.org/10.5281/zenodo.6595277 (Li, 2022).
The supplement related to this article is available online at: https://doi.org/10.5194/gmd-15-4313-2022-supplement.
QL, AR, and SPS designed the simulations, and QL carried them out. QL, AR, SPS, and JL analyzed the results. QL, AR, SPS, JL, KJD, and KSE prepared the paper together.
The contact author has declared that neither they nor their co-authors have any competing interests.
Publisher's note: Copernicus Publications remains neutral with regard to jurisdictional claims in published maps and institutional affiliations.
This work was supported by the Next-Generation Ecosystem Experiments in the Tropics (NGEE-Tropics) project supported by the US DOE, Office of Science, Office of Biological and Environmental Research, and through the United States Department of Energy with contract no. DE-SC0012704 to Brookhaven National Laboratory.
This work was supported by the Next-Generation Ecosystem Experiments in the Tropics (NGEE-Tropics) project supported by the US DOE, Office of Science, Office of Biological and Environmental Research, and through the United States Department of Energy with contract no. DE-SC0012704 to Brookhaven National Laboratory.
This paper was edited by Christoph Müller and reviewed by two anonymous referees.
Aranda, I., Rodríguez-Calcerrada, J., Robson, T. M., Cano, F. J., Alté, L., and Sánchez-Gómez, D.: Stomatal and non-stomatal limitations on leaf carbon assimilation in beech (Fagus sylvatica L.) seedlings under natural conditions, For. Syst., 21, 405–417, https://doi.org/10.5424/fs/2012213-02348, 2012.
Ball, J. T., Woodrow, I. E., and Berry, J. A.: A Model Predicting Stomatal Conductance and its Contribution to the Control of Photosynthesis under Different Environmental Conditions, in: Progress in Photosynthesis Research: Volume 4 Proceedings of the VIIth International Congress on Photosynthesis Providence, Rhode Island, USA, 10–15 August 1986, edited by: Biggins, J., Springer Netherlands, Dordrecht, 221–224, https://doi.org/10.1007/978-94-017-0519-6_48, 1987.
Barnard, D. M. and Bauerle, W. L.: The implications of minimum stomatal conductance on modeling water flux in forest canopies, J. Geophys. Res.-Biogeo., 118, 1322–1333, https://doi.org/10.1002/jgrg.20112, 2013.
Berry, J. A., Beerling, D. J., and Franks, P. J.: Stomata: key players in the earth system, past and present, Curr. Opin. Plant Biol., 13, 232–239, https://doi.org/10.1016/j.pbi.2010.04.013, 2010.
Best, M. J., Pryor, M., Clark, D. B., Rooney, G. G., Essery, R. L. H., Ménard, C. B., Edwards, J. M., Hendry, M. A., Porson, A., Gedney, N., Mercado, L. M., Sitch, S., Blyth, E., Boucher, O., Cox, P. M., Grimmond, C. S. B., and Harding, R. J.: The Joint UK Land Environment Simulator (JULES), model description – Part 1: Energy and water fluxes, Geosci. Model Dev., 4, 677–699, https://doi.org/10.5194/gmd-4-677-2011, 2011.
Blatt, M. R.: Cellular Signaling and Volume Control in Stomatal Movements in Plants, Annu. Rev. Cell Dev. Biol., 16, 221–241, https://doi.org/10.1146/annurev.cellbio.16.1.221, 2000.
Bonan, G. B., Lawrence, P. J., Oleson, K. W., Levis, S., Jung, M., Reichstein, M., Lawrence, D. M., Swenson, S. C., Bonan, C. :, Lawrence, P. J., Oleson, K. W., Levis, S., Jung, M., Reichstein, M., Lawrence, D. M., and Swenson, S. C.: Improving canopy processes in the Community Land Model version 4 (CLM4) using global flux fields empirically inferred from FLUXNET data, J. Geophys. Res.-Biogeo., 116, G02014, https://doi.org/10.1029/2010JG001593, 2011.
Bonan, G. B., Williams, M., Fisher, R. A., and Oleson, K. W.: Modeling stomatal conductance in the earth system: linking leaf water-use efficiency and water transport along the soil–plant–atmosphere continuum, Geosci. Model Dev., 7, 2193–2222, https://doi.org/10.5194/gmd-7-2193-2014, 2014.
Bota, J., Medrano, H., and Flexas, J.: Is photosynthesis limited by decreased Rubisco activity and RuBP content under progressive water stress?, New Phytol., 162, 671–681, https://doi.org/10.1111/j.1469-8137.2004.01056.x, 2004.
Buckley, T. N. and Mott, K. A.: Modelling stomatal conductance in response to environmental factors, Plant Cell Environ., 36, 1691–1699, https://doi.org/10.1111/pce.12140, 2013.
Cano, F. J., Sánchez-Gómez, D., Rodríguez-Calcerrada, J., Warren, C. R., Gil, L., and Aranda, I.: Effects of drought on mesophyll conductance and photosynthetic limitations at different tree canopy layers, Plant Cell Environ., 36, 1961–1980, https://doi.org/10.1111/pce.12103, 2013.
Clark, D. B., Mercado, L. M., Sitch, S., Jones, C. D., Gedney, N., Best, M. J., Pryor, M., Rooney, G. G., Essery, R. L. H., Blyth, E., Boucher, O., Harding, R. J., Huntingford, C., and Cox, P. M.: The Joint UK Land Environment Simulator (JULES), model description – Part 2: Carbon fluxes and vegetation dynamics, Geosci. Model Dev., 4, 701–722, https://doi.org/10.5194/gmd-4-701-2011, 2011.
Collatz, G. J., Ball, J. T., Grivet, C., and Berry, J. A.: Physiological and environmental regulation of stomatal conductance, photosynthesis and transpiration: a model that includes a laminar boundary layer, Agric. For. Meteorol., 54, 107–136, https://doi.org/10.1016/0168-1923(91)90002-8, 1991.
Comins, H. N. and McMurtrie, R. E.: Long-Term Response of Nutrient-Limited Forests to CO”2 Enrichment; Equilibrium Behavior of Plant-Soil Models, Ecol. Appl. Publ. Ecol. Soc. Am., 3, 666–681, https://doi.org/10.2307/1942099, 1993.
Condit, R., Perez, R., Aguilar, S., and Lao, S.: Sherman 6-ha Forest Census Plot Data, ForestGEO [data set], https://forestgeo.si.edu/sites/neotropics/san-lorenzo/san-lorenzo-sherman-plot-data, (last access: 1 June 2020), 2009.
Cowan, I. R. and Farquhar, G. D.: Stomatal function in relation to leaf metabolism and environment, Symp. Soc. Exp. Biol., 31, 471–505, 1977.
CTSM Development Team: ESCOMP/CTSM: Update documentation for release-clm5.0 branch, and fix issues with no-anthro surface dataset creation, Zenodo [code], https://doi.org/10.5281/zenodo.3779821, 2020.
Damour, G., Simonneau, T., Cochard, H., and Urban, L.: An overview of models of stomatal conductance at the leaf level, Plant Cell Environ., 33, 1419–1438, https://doi.org/10.1111/j.1365-3040.2010.02181.x, 2010.
Davidson, K. J., Lamour, J., Rogers, A., and Serbin, S. P.: Late-day measurement of excised branches results in uncertainty in the estimation of two stomatal parameters derived from response curves in Populus deltoides Bartr. × Populus nigra L., Tree Physiol., tpac006, https://doi.org/10.1093/treephys/tpac006, in press, 2022.
Davies, W. J., Wilkinson, S., and Loveys, B.: Stomatal control by chemical signalling and the exploitation of this mechanism to increase water use efficiency in agriculture, New Phytol., 153, 449–460, https://doi.org/10.1046/j.0028-646X.2001.00345.x, 2002.
De Kauwe, M. G., Kala, J., Lin, Y.-S., Pitman, A. J., Medlyn, B. E., Duursma, R. A., Abramowitz, G., Wang, Y.-P., and Miralles, D. G.: A test of an optimal stomatal conductance scheme within the CABLE land surface model, Geosci. Model Dev., 8, 431–452, https://doi.org/10.5194/gmd-8-431-2015, 2015.
De La Motte, L. G., Beauclaire, Q., Heinesch, B., Cuntz, M., Foltýnová, L., Šigut, L., Kowalska, N., Manca, G., Ballarin, I. G., Vincke, C., Roland, M., Ibrom, A., Lousteau, D., Siebicke, L., Neiryink, J., and Longdoz, B.: Non-stomatal processes reduce gross primary productivity in temperate forest ecosystems during severe edaphic drought: Edaphic drought in forest ecosystems, Philos. Trans. R. Soc. B Biol. Sci., 375, 20190527, https://doi.org/10.1098/rstb.2019.0527, 2020.
Domingues, T. F., Martinelli, L. A., and Ehleringer, J. R.: Seasonal patterns of leaf-level photosynthetic gas exchange in an eastern Amazonian rain forest, Plant Ecol. Divers., 7, 189–203, https://doi.org/10.1080/17550874.2012.748849, 2014.
Duursma, R. A., Blackman, C. J., Lopéz, R., Martin-StPaul, N. K., Cochard, H., and Medlyn, B. E.: On the minimum leaf conductance: its role in models of plant water use, and ecological and environmental controls, New Phytol., 221, 693–705, https://doi.org/10.1111/nph.15395, 2019.
Egea, G., Verhoef, A., and Vidale, P. L.: Towards an improved and more flexible representation of water stress in coupled photosynthesis–stomatal conductance models, Agric. For. Meteorol., 151, 1370–1384, https://doi.org/10.1016/j.agrformet.2011.05.019, 2011.
Farquhar, G. D., von Caemmerer, S., and Berry, J. A.: A biochemical model of photosynthetic CO2 assimilation in leaves of C3 species, Planta, 149, 78–90, https://doi.org/10.1007/BF00386231, 1980.
FATES Development Team: The Functionally Assembled Terrestrial Ecosystem Simulator (commit 9a4627a), Zenodo [code], https://doi.org/10.5281/zenodo.5851984, 2020a.
FATES Development Team: The Functionally Assembled Terrestrial Ecosystem Simulator (FATES) (Version sci.1.35.5_api.11.0.0), Zenodo [code], https://doi.org/10.5281/zenodo.3825474, 2020b.
Faybishenko, B., Paton, S., Knox, R., Varadharajan, C., Agarwal, D., and Powell, T.: San Lorenzo meteorological drivers, Next-Generation Ecosystem Experiments Tropics; STRI; Lawrence Berkeley National Lab, (LBNL), Berkeley, CA (United States) [data set], https://doi.org/10.15486/ngt/1507769, 2019.
Fer, I., Kelly, R., Moorcroft, P. R., Richardson, A. D., Cowdery, E. M., and Dietze, M. C.: Linking big models to big data: efficient ecosystem model calibration through Bayesian model emulation, Biogeosciences, 15, 5801–5830, https://doi.org/10.5194/bg-15-5801-2018, 2018.
Ficklin, D. L. and Novick, K. A.: Historic and projected changes in vapor pressure deficit suggest a continental-scale drying of the United States atmosphere, J. Geophys. Res., 122, 2061–2079, https://doi.org/10.1002/2016JD025855, 2017.
Fisher, R. A., Muszala, S., Verteinstein, M., Lawrence, P., Xu, C., McDowell, N. G., Knox, R. G., Koven, C., Holm, J., Rogers, B. M., Spessa, A., Lawrence, D., and Bonan, G.: Taking off the training wheels: the properties of a dynamic vegetation model without climate envelopes, CLM4.5(ED), Geosci. Model Dev., 8, 3593–3619, https://doi.org/10.5194/gmd-8-3593-2015, 2015.
Fisher, R. A., Koven, C. D., Anderegg, W. R. L., Christoffersen, B. O., Dietze, M. C., Farrior, C. E., Holm, J. A., Hurtt, G. C., Knox, R. G., Lawrence, P. J., Lichstein, J. W., Longo, M., Matheny, A. M., Medvigy, D., Muller-Landau, H. C., Powell, T. L., Serbin, S. P., Sato, H., Shuman, J. K., Smith, B., Trugman, A. T., Viskari, T., Verbeeck, H., Weng, E., Xu, C., Xu, X., Zhang, T., and Moorcroft, P. R.: Vegetation demographics in Earth System Models: A review of progress and priorities, Glob. Change Biol., 24, 35–54, https://doi.org/10.1111/gcb.13910, 2018.
Franks, P. J., Berry, J. A., Lombardozzi, D. L., and Bonan, G. B.: Stomatal function across temporal and spatial scales: Deep-time trends, land-atmosphere coupling and global models, Plant Physiol., 174, 583–602, https://doi.org/10.1104/pp.17.00287, 2017.
Galbraith, D., Levy, P. E., Sitch, S., Huntingford, C., Cox, P., Williams, M., and Meir, P.: Multiple mechanisms of Amazonian forest biomass losses in three dynamic global vegetation models under climate change, New Phytol., 187, 647–665, https://doi.org/10.1111/j.1469-8137.2010.03350.x, 2010.
Galmés, J., Medrano, H., and Flexas, J.: Photosynthetic limitations in response to water stress and recovery in Mediterranean plants with different growth forms, New Phytol., 175, 81–93, https://doi.org/10.1111/j.1469-8137.2007.02087.x, 2007.
Gatti, L. V., Basso, L. S., Miller, J. B., Gloor, M., Gatti Domingues, L., Cassol, H. L. G., Tejada, G., Aragão, L. E. O. C., Nobre, C., Peters, W., Marani, L., Arai, E., Sanches, A. H., Corrêa, S. M., Anderson, L., Von Randow, C., Correia, C. S. C., Crispim, S. P., and Neves, R. A. L.: Amazonia as a carbon source linked to deforestation and climate change, Nature, 595, 388–393, https://doi.org/10.1038/s41586-021-03629-6, 2021.
Gimeno, T. E., Crous, K. Y., Cooke, J., O'Grady, A. P., Ósvaldsson, A., Medlyn, B. E., and Ellsworth, D. S.: Conserved stomatal behaviour under elevated CO2 and varying water availability in a mature woodland, Funct. Ecol., 30, 700–709, https://doi.org/10.1111/1365-2435.12532, 2016.
Grassi, G. and Magnani, F.: Stomatal, mesophyll conductance and biochemical limitations to photosynthesis as affected by drought and leaf ontogeny in ash and oak trees, Plant Cell Environ., 28, 834–849, https://doi.org/10.1111/j.1365-3040.2005.01333.x, 2005.
Guimberteau, M., Zhu, D., Maignan, F., Huang, Y., Yue, C., Dantec-Nédélec, S., Ottlé, C., Jornet-Puig, A., Bastos, A., Laurent, P., Goll, D., Bowring, S., Chang, J., Guenet, B., Tifafi, M., Peng, S., Krinner, G., Ducharne, A., Wang, F., Wang, T., Wang, X., Wang, Y., Yin, Z., Lauerwald, R., Joetzjer, E., Qiu, C., Kim, H., and Ciais, P.: ORCHIDEE-MICT (v8.4.1), a land surface model for the high latitudes: model description and validation, Geosci. Model Dev., 11, 121–163, https://doi.org/10.5194/gmd-11-121-2018, 2018.
Héroult, A., Lin, Y. S., Bourne, A., Medlyn, B. E., and Ellsworth, D. S.: Optimal stomatal conductance in relation to photosynthesis in climatically contrasting Eucalyptus species under drought, Plant Cell Environ., 36, 262–274, https://doi.org/10.1111/j.1365-3040.2012.02570.x, 2013.
Hersbach, H., de Rosnay, P., Bell, B., Schepers, D., Simmons, A., Soci, C., Abdalla, S., Alonso-Balmaseda, M., Balsamo, G., Bechtold, P., Berrisford, P., Bidlot, J.-R., de Boisséson, E., Bonavita, M., Browne, P., Buizza, R., Dahlgren, P., Dee, D., Dragani, R., Diamantakis, M., Flemming, J., Forbes, R., Geer, A., Haiden, T., Hólm, E., Haimberger, L., Hogan, R., Horányi, A., Janiskova, M., Laloyaux, P., Lopez, P., Munoz-Sabater, J., Peubey, C., Radu, R., Richardson, D., Thépaut, J.-N., Vitart, F., Yang, X., Zsótér, E., and Zuo, H.: Operational global reanalysis: progress, future directions and synergies with NWP, ECMWF ERA Report Series, N27, https://www.ecmwf.int/en/elibrary/18765-operational-globalreanalysis-progress-future-directions-and-synergies-nwp (last access: April 2022), 2018.
Hetherington, A. M. and Woodward, F. I.: The role of stomata in sensing and driving environmental change, Nature, 424, 901–908, https://doi.org/10.1038/nature01843, 2003.
Holm, J. A., Knox, R. G., Zhu, Q., Fisher, R. A., Koven, C. D., Lima, A. J. N., Riley, W. J., Longo, M., Negrón-Juárez, R. I., de Araujo, A. C., Kueppers, L. M., Moorcroft, P. R., Higuchi, N., and Chambers, J. Q.: The Central Amazon Biomass Sink Under Current and Future Atmospheric CO2: Predictions From Big-Leaf and Demographic Vegetation Models, J. Geophys. Res.-Biogeo., 125, e2019JG005500, https://doi.org/10.1029/2019JG005500, 2020.
Kala, J., De Kauwe, M. G., Pitman, A. J., Medlyn, B. E., Wang, Y. P., Lorenz, R., and Perkins-Kirkpatrick, S. E.: Impact of the representation of stomatal conductance on model projections of heatwave intensity, Sci. Rep., 6, 23418, https://doi.org/10.1038/srep23418, 2016.
Keenan, T., Sabate, S., and Gracia, C.: The importance of mesophyll conductance in regulating forest ecosystem productivity during drought periods, Glob. Change Biol., 16, 1019–1034, https://doi.org/10.1111/j.1365-2486.2009.02017.x, 2010.
Kennedy, D., Swenson, S., Oleson, K. W., Lawrence, D. M., Fisher, R., Lola da Costa, A. C., and Gentine, P.: Implementing Plant Hydraulics in the Community Land Model, Version 5, J. Adv. Model. Earth Sy., 11, 485–513, https://doi.org/10.1029/2018MS001500, 2019.
Knauer, J., Werner, C., and Zaehle, S.: Evaluating stomatal models and their atmospheric drought response in a land surface scheme: A multibiome analysis, J. Geophys. Res.-Biogeo., 120, 1894–1911, https://doi.org/10.1002/2015JG003114, 2015.
Kolby Smith, W., Reed, S. C., Cleveland, C. C., Ballantyne, A. P., Anderegg, W. R. L., Wieder, W. R., Liu, Y. Y., and Running, S. W.: Large divergence of satellite and Earth system model estimates of global terrestrial CO2 fertilization, Nat. Clim. Change, 6, 306–310, https://doi.org/10.1038/nclimate2879, 2016.
Koven, C. D., Knox, R. G., Fisher, R. A., Chambers, J. Q., Christoffersen, B. O., Davies, S. J., Detto, M., Dietze, M. C., Faybishenko, B., Holm, J., Huang, M., Kovenock, M., Kueppers, L. M., Lemieux, G., Massoud, E., McDowell, N. G., Muller-Landau, H. C., Needham, J. F., Norby, R. J., Powell, T., Rogers, A., Serbin, S. P., Shuman, J. K., Swann, A. L. S., Varadharajan, C., Walker, A. P., Wright, S. J., and Xu, C.: Benchmarking and parameter sensitivity of physiological and vegetation dynamics using the Functionally Assembled Terrestrial Ecosystem Simulator (FATES) at Barro Colorado Island, Panama, Biogeosciences, 17, 3017–3044, https://doi.org/10.5194/bg-17-3017-2020, 2020.
Kumarathunge, D. P., Medlyn, B. E., Drake, J. E., Tjoelker, M. G., Aspinwall, M. J., Battaglia, M., Cano, F. J., Carter, K. R., Cavaleri, M. A., Cernusak, L. A., Chambers, J. Q., Crous, K. Y., De Kauwe, M. G., Dillaway, D. N., Dreyer, E., Ellsworth, D. S., Ghannoum, O., Han, Q., Hikosaka, K., Jensen, A. M., Kelly, J. W. G., Kruger, E. L., Mercado, L. M., Onoda, Y., Reich, P. B., Rogers, A., Slot, M., Smith, N. G., Tarvainen, L., Tissue, D. T., Togashi, H. F., Tribuzy, E. S., Uddling, J., Vårhammar, A., Wallin, G., Warren, J. M., and Way, D. A.: Acclimation and adaptation components of the temperature dependence of plant photosynthesis at the global scale, New Phytol., 222, 768–784, https://doi.org/10.1111/nph.15668, 2019.
Lamour, J., Davidson, K. J., Ely, K. S., Le Moguédec, G., Leakey, A. D. B., Li, Q., Serbin, S. P., and Rogers, A.: An improved representation of the relationship between photosynthesis and stomatal conductance leads to more stable estimation of conductance parameters and improves the goodness-of-fit across diverse data sets, Glob. Change Biol., 28, 3537–3556, https://doi.org/10.1111/gcb.16103, 2022.
Lawrence, D. M., Fisher, R. A., Koven, C. D., Oleson, K. W., Swenson, S. C., Bonan, G., Collier, N., Ghimire, B., van Kampenhout, L., Kennedy, D., Kluzek, E., Lawrence, P. J., Li, F., Li, H., Lombardozzi, D., Riley, W. J., Sacks, W. J., Shi, M., Vertenstein, M., Wieder, W. R., Xu, C., Ali, A. A., Badger, A. M., Bisht, G., van den Broeke, M., Brunke, M. A., Burns, S. P., Buzan, J., Clark, M., Craig, A., Dahlin, K., Drewniak, B., Fisher, J. B., Flanner, M., Fox, A. M., Gentine, P., Hoffman, F., Keppel-Aleks, G., Knox, R., Kumar, S., Lenaerts, J., Leung, L. R., Lipscomb, W. H., Lu, Y., Pandey, A., Pelletier, J. D., Perket, J., Randerson, J. T., Ricciuto, D. M., Sanderson, B. M., Slater, A., Subin, Z. M., Tang, J., Thomas, R. Q., Val Martin, M., and Zeng, X.: The Community Land Model Version 5: Description of New Features, Benchmarking, and Impact of Forcing Uncertainty, J. Adv. Model. Earth Syst., 11, 4245–4287, https://doi.org/10.1029/2018MS001583, 2019.
Lawson, T., Simkin, A. J., Kelly, G., and Granot, D.: Mesophyll photosynthesis and guard cell metabolism impacts on stomatal behaviour, New Phytol., 203, 1064–1081, https://doi.org/10.1111/nph.12945, 2014.
Leuning, R.: A critical appraisal of a combined stomatal-photosynthesis model for C3 plants, Plant Cell Environ., 18, 339–355, https://doi.org/10.1111/j.1365-3040.1995.tb00370.x, 1995.
Leuning, R.: Temperature dependence of two parameters in a photosynthesis model, Plant Cell Environ., 25, 1205–1210, https://doi.org/10.1046/j.1365-3040.2002.00898.x, 2002.
Li, Q.: Model outputs and observation data for “Implementation and evaluation of the unified stomatal optimization approach in the Functionally Assembled Terrestrial Ecosystem Simulator (FATES)”, Zenodo [data set], https://doi.org/10.5281/zenodo.6595277, 2022.
Li, Q. and Serbin, S.: Scripts for running, analyzing, and plotting results for FATES with Medlyn stomatal conductance model, Zenodo [code], https://doi.org/10.5281/zenodo.5854740, 2022.
Limousin, J. M., Misson, L., Lavoir, A. V., Martin, N. K., and Rambal, S.: Do photosynthetic limitations of evergreen Quercus ilex leaves change with long-term increased drought severity?, Plant Cell Environ., 33, 863–875, https://doi.org/10.1111/j.1365-3040.2009.02112.x, 2010.
Lin, Y. S., Medlyn, B. E., Duursma, R. A., Prentice, I. C., Wang, H., Baig, S., Eamus, D., De Dios, V. R., Mitchell, P., Ellsworth, D. S., De Beeck, M. O., Wallin, G., Uddling, J., Tarvainen, L., Linderson, M. L., Cernusak, L. A., Nippert, J. B., Ocheltree, T. W., Tissue, D. T., Martin-StPaul, N. K., Rogers, A., Warren, J. M., De Angelis, P., Hikosaka, K., Han, Q., Onoda, Y., Gimeno, T. E., Barton, C. V. M., Bennie, J., Bonal, D., Bosc, A., Löw, M., Macinins-Ng, C., Rey, A., Rowland, L., Setterfield, S. A., Tausz-Posch, S., Zaragoza-Castells, J., Broadmeadow, M. S. J., Drake, J. E., Freeman, M., Ghannoum, O., Hutley, L. B., Kelly, J. W., Kikuzawa, K., Kolari, P., Koyama, K., Limousin, J. M., Meir, P., Da Costa, A. C. L., Mikkelsen, T. N., Salinas, N., Sun, W., and Wingate, L.: Optimal stomatal behaviour around the world, Nat. Clim. Change, 5, 459–464, https://doi.org/10.1038/nclimate2550, 2015.
Lombardozzi, D. L., Zeppel, M. J. B., Fisher, R. A., and Tawfik, A.: Representing nighttime and minimum conductance in CLM4.5: global hydrology and carbon sensitivity analysis using observational constraints, Geosci. Model Dev., 10, 321–331, https://doi.org/10.5194/gmd-10-321-2017, 2017.
Marchin, R. M., Broadhead, A. A., Bostic, L. E., Dunn, R. R., and Hoffmann, W. A.: Stomatal acclimation to vapour pressure deficit doubles transpiration of small tree seedlings with warming: Stomatal acclimation increases transpiration, Plant Cell Environ., 39, 2221–2234, https://doi.org/10.1111/pce.12790, 2016.
Medlyn, B. E., Duursma, R. A., Eamus, D., Ellsworth, D. S., Prentice, I. C., Barton, C. V. M., Crous, K. Y., De Angelis, P., Freeman, M., and Wingate, L.: Reconciling the optimal and empirical approaches to modelling stomatal conductance, Glob. Change Biol., 17, 2134–2144, https://doi.org/10.1111/j.1365-2486.2010.02375.x, 2011.
Miner, G. L. and Bauerle, W. L.: Seasonal variability of the parameters of the Ball–Berry model of stomatal conductance in maize (Zea mays L.) and sunflower (Helianthus annuus L.) under well-watered and water-stressed conditions, Plant Cell Environ., 40, 1874–1886, https://doi.org/10.1111/pce.12990, 2017.
Misson, L., Panek, J. A., and Goldstein, A. H.: A comparison of three approaches to modeling leaf gas exchange in annually drought-stressed ponderosa pine forests, Tree Physiol., 24, 529–541, https://doi.org/10.1093/treephys/24.5.529, 2004.
Misson, L., Limousin, J. M., Rodriguez, R., and Letts, M. G.: Leaf physiological responses to extreme droughts in Mediterranean Quercus ilex forest, Plant Cell Environ., 33, 1898–1910, https://doi.org/10.1111/j.1365-3040.2010.02193.x, 2010.
Morales, P., Sykes, M. T., Prentice, I. C., Smith, P., Smith, B., Bugmann, H., Zierl, B., Friedlingstein, P., Viovy, N., Sabaté, S., Sánchez, A., Pla, E., Gracia, C. A., Sitch, S., Arneth, A., and Ogee, J.: Comparing and evaluating process-based ecosystem model predictions of carbon and water fluxes in major European forest biomes, Glob. Change Biol., 11, 2211–2233, https://doi.org/10.1111/j.1365-2486.2005.01036.x, 2005.
Oleson, K. W., Lawrence, D. M., Bonan, G. B., Drewniak, B., Huang, M., Levis, S., Li, F., Riley, W. J., Swenson, S. C., Thornton, P. E., Bozbiyik, A., Fisher, R., Heald, C. L., Kluzek, E., Lamarque, F., Lawrence, P. J., Leung, L. R., Muszala, S., Ricciuto, D. M., Sacks, W., Sun, Y., Tang, J., and Yang, Z.-L.: Technical Description of version 4.5 of the Community Land Model (CLM) (No. NCAR/TN-503+STR), https://doi.org/10.5065/D6RR1W7M, 2013.
Pachauri, R. K., Allen, M. R., Barros, V. R., Broome, J., Cramer, W., Christ, R., Church, J. A., Clarke, L., Dahe, Q., Dasgupta, P., Dubash, N. K., Edenhofer, O., Elgizouli, I., Field, C. B., Forster, P., Friedlingstein, P., Fuglestvedt, J., Gomez-Echeverri, L., Hallegatte, S., Hegerl, G., Howden, M., Jiang, K., Jimenez Cisneroz, B., Kattsov, V., Lee, H., Mach, K. J., Marotzke, J., Mastrandrea, M. D., Meyer, L., Minx, J., Mulugetta, Y., O’Brien, K., Oppenheimer, M., Pereira, J. J., Pichs-Madruga, R., Plattner, G.-K., Pörtner, H.-O., Power, S. B., Preston, B., Ravindranath, N. H., Reisinger, A., Riahi, K., Rusticucci, M., Scholes, R., Seyboth, K., Sokona, Y., Stavins, R., Stocker, T. F., Tschakert, P., van Vuuren, D., and van Ypserle, J.-P.: Climate Change 2014: Synthesis Report. Contribution of Working Groups I, II and III to the Fifth Assessment Report of the Intergovernmental Panel on Climate Change, edited by: Pachauri, R. K. and Meyer, L., IPCC, Geneva, Switzerland, 151 pp., http://www.mendeley.com/research/climate-change-2014-synthesis-report-contribution-working-groups-i-ii-iii-fifth-assessment-report-in-20 (last access: 1 January 2021), 2014.
Powell, T. L., Galbraith, D. R., Christoffersen, B. O., Harper, A., Imbuzeiro, H. M. A., Rowland, L., Almeida, S., Brando, P. M., da Costa, A. C. L., Costa, M. H., Levine, N. M., Malhi, Y., Saleska, S. R., Sotta, E., Williams, M., Meir, P., and Moorcroft, P. R.: Confronting model predictions of carbon fluxes with measurements of Amazon forests subjected to experimental drought, New Phytol., 200, 350–365, https://doi.org/10.1111/nph.12390, 2013.
Reichstein, M., Bahn, M., Ciais, P., Frank, D., Mahecha, M. D., Seneviratne, S. I., Zscheischler, J., Beer, C., Buchmann, N., Frank, D. C., Papale, D., Rammig, A., Smith, P., Thonicke, K., van der Velde, M., Vicca, S., Walz, A., and Wattenbach, M.: Climate extremes and the carbon cycle, Nature, 500, 287–295, https://doi.org/10.1038/nature12350, 2013.
Rogers, A., Medlyn, B. E., Dukes, J. S., Bonan, G., Von Caemmerer, S., Dietze, M. C., Kattge, J., Leakey, A. D. B., Mercado, L. M., and Niinemets, Ü.: A roadmap for improving the representation of photosynthesis in Earth system models, New Phytol., 213, 22–42, 2017a.
Rogers, A., Serbin, S., Ely, K., Wu, J., Wolfe, B., Dickman, T., Collins, A., Detto, M., Grossiord, C., McDowell, N., and Michaletz, S.: CO2 response (ACi) gas exchange, calculated Vcmax & Jmax parameters, Feb2016–May2016, PA-SLZ, PA-PNM: Panama, Next-Generation Ecosystem Experiments Tropics; Brookhaven National Lab. (BNL), Upton, NY (United States); Los Alamos National Lab. (LANL), Los Alamos, NM (United States), STRI, NGEE–Tropics [data set], https://doi.org/10.15486/ngt/1411867, 2017b.
Rogers, A., Serbin, S., Ely, K., Wu, J., Wolfe, B., Dickman, T., Collins, A., Detto, M., Grossiord, C., McDowell, N., and Michaletz, S.: Diurnal leaf gas exchange survey, Feb2016–May2016, PA-SLZ, PA-PNM: Panama, NGEE–Tropics [data set], https://doi.org/10.15486/NGT/1411972, 2017c.
Rowland, L., Harper, A., Christoffersen, B. O., Galbraith, D. R., Imbuzeiro, H. M. A., Powell, T. L., Doughty, C., Levine, N. M., Malhi, Y., Saleska, S. R., Moorcroft, P. R., Meir, P., and Williams, M.: Modelling climate change responses in tropical forests: similar productivity estimates across five models, but different mechanisms and responses, Geosci. Model Dev., 8, 1097–1110, https://doi.org/10.5194/gmd-8-1097-2015, 2015.
Schaefer, K., Collatz, G. J., Tans, P., Denning, A. S., Baker, I., Berry, J., Prihodko, L., Suits, N., and Philpott, A.: Combined Simple Biosphere/Carnegie-Ames-Stanford Approach terrestrial carbon cycle model, J. Geophys. Res.-Biogeo., 113, G03034, https://doi.org/10.1029/2007JG000603, 2008.
Sellers, P. J., Randall, D. A., Collatz, G. J., Berry, J. A., Field, C. B., Dazlich, D. A., Zhang, C., Collelo, G. D., and Bounoua, L.: A revised land surface parameterization (SiB2) for atmospheric GCMs. Part I: Model formulation, J. Climate, 9, 676–705, 1996.
Smith, M. N., Taylor, T. C., van Haren, J., Rosolem, R., Restrepo-Coupe, N., Adams, J., Wu, J., de Oliveira, R. C., da Silva, R., de Araujo, A. C., de Camargo, P. B., Huxman, T. E., and Saleska, S. R.: Empirical evidence for resilience of tropical forest photosynthesis in a warmer world, Nat. Plants, 6, 1225–1230, https://doi.org/10.1038/s41477-020-00780-2, 2020.
Sperry, J. S. and Love, D. M.: What plant hydraulics can tell us about responses to climate-change droughts, New Phytol., 207, 14–27, https://doi.org/10.1111/nph.13354, 2015.
Sullivan, M. J. P., Lewis, S. L., Affum-Baffoe, K., Castilho, C., Costa, F., Sanchez, A. C., Ewango, C. E. N., Hubau, W., Marimon, B., Monteagudo-Mendoza, A., Qie, L., Sonké, B., Martinez, R. V., Baker, T. R., Brienen, R. J. W., Feldpausch, T. R., Galbraith, D., Gloor, M., Malhi, Y., Aiba, S. I., Alexiades, M. N., Almeida, E. C., De Oliveira, E. A., Dávila, E. Á., Loayza, P. A., Andrade, A., Vieira, S. A., Aragão, L. E. O. C., AraujoMurakami, A., Arets, E. J. M. M., Arroyo, L., Ashton, P., Gerardo Aymard, C., Baccaro, F. B., Banin, L. F., Baraloto, C., Camargo, P. B., Barlow, J., Barroso, J., Bastin, J. F., Batterman, S. A., Beeckman, H., Begne, S. K., Bennett, A. C., Berenguer, E., Berry, N., Blanc, L., Boeckx, P., Bogaert, J., Bonal, D., Bongers, F., Bradford, M., Brearley, F. Q., Brncic, T., Brown, F., Burban, B., Camargo, J. L., Castro, W., Céron, C., Ribeiro, S. C., Moscoso, V. C., Chave, J., Chezeaux, E., Clark, C. J., De Souza, F. C., Collins, M., Comiskey, J. A., Valverde, F. C., Medina, M. C., Da Costa, L., Dancsák, M., Dargie, G. C., Davies, S., Cardozo, N. D., De Haulleville, T., De Medeiros, M. B., Del Aguila Pasquel, J., Derroire, G., Di Fiore, A., Doucet, J. L., Dourdain, A., Droissart, V., Duque, L. F., Ekoungoulou, R., Elias, F., Erwin, T., Esquivel-Muelbert, A., Fauset, S., Ferreira, J., Llampazo, G. F., Foli, E., Ford, A., Gilpin, M., Hall, J. S., Hamer, K. C., Hamilton, A. C., Harris, D. J., Hart, T. B., Hédl, R., Herault, B., Herrera, R., Higuchi, N., Hladik, A., Coronado, E., and Phillips, O. L.: Long-term thermal sensitivity of earth’s tropical forests, Science, 368, 869–874, https://doi.org/10.1126/science.aaw7578, 2020.
Verhoef, A. and Egea, G.: Modeling plant transpiration under limited soil water: Comparison of different plant and soil hydraulic parameterizations and preliminary implications for their use in land surface models, Agric. For. Meteorol., 191, 22–32, https://doi.org/10.1016/j.agrformet.2014.02.009, 2014.
Vidale, P. L., Egea, G., McGuire, P. C., Todt, M., Peters, W., Müller, O., Balan-Sarojini, B., and Verhoef, A.: On the Treatment of Soil Water Stress in GCM Simulations of Vegetation Physiology, Front. Environ. Sci., 9, 689301, https://doi.org/10.3389/fenvs.2021.689301, 2021.
Wang, S., Yang, Y., Trishchenko, A. P., Barr, A. G., Black, T. A., and Mccaughey, H.: Modeling the response of canopy stomatal conductance to humidity, J. Hydrometeorol., 10, 521–532, https://doi.org/10.1175/2008JHM1050.1, 2009.
Wilson, K. B., Baldocchi, D. D., and Hanson, P. J.: Quantifying stomatal and non-stomatal limitations to carbon assimilation resulting from leaf aging and drought in mature deciduous tree species, Tree Physiol., 20, 787–797, https://doi.org/10.1093/treephys/20.12.787, 2000.
Wright, S. J., Horlyck, V., Basset, Y., Barrios, H., Bethancourt, A., Bohlman, S. A., Gilbert, G. S., Goldstein, G., Graham, E. A., and Kitajima, K.: Tropical canopy biology program, Republic of Panama, in: Studying forest canopies from above: The international canopy crane network, Smithsonian Tropical Research Institute and United Nations Environmental Programme, 137–139, ISBN 978-9962-614-05-0, 2003.
Wu, J., Serbin, S. P., Ely, K. S., Wolfe, B. T., Dickman, L. T., Grossiord, C., Michaletz, S. T., Collins, A. D., Detto, M., McDowell, N. G., Wright, S. J., and Rogers, A.: The response of stomatal conductance to seasonal drought in tropical forests, Glob. Change Biol., 26, 823–839, https://doi.org/10.1111/gcb.14820, 2020.
Xu, L. and Baldocchi, D. D.: Seasonal trends in photosynthetic parameters and stomatal conductance of blue oak (Quercus douglasii) under prolonged summer drought and high temperature, Tree Physiol., 23, 865–877, https://doi.org/10.1093/treephys/23.13.865, 2003.
Yuan, W., Zheng, Y., Piao, S., Ciais, P., Lombardozzi, D., Wang, Y., Ryu, Y., Chen, G., Dong, W., Hu, Z., Jain, A. K., Jiang, C., Kato, E., Li, S., Lienert, S., Liu, S., Nabel, J. E. M. S., Qin, Z., Quine, T., Sitch, S., Smith, W. K., Wang, F., Wu, C., Xiao, Z., and Yang, S.: Increased atmospheric vapor pressure deficit reduces global vegetation growth, Sci. Adv., 5, eaax139, https://doi.org/10.1126/sciadv.aax1396, 2019.
Zaehle, S. and Friend, A. D.: Carbon and nitrogen cycle dynamics in the O-CN land surface model: 1. Model description, site-scale evaluation, and sensitivity to parameter estimates, Global Biogeochem. Cy., 24, GB1005, https://doi.org/10.1029/2009GB003521, 2010.
Zait, Y. and Schwartz, A.: Climate-Related Limitations on Photosynthesis and Drought-Resistance Strategies of Ziziphus spina-christi, Front. For. Glob. Change, 1, 3, https://doi.org/10.3389/ffgc.2018.00003, 2018.
Zhou, S., Duursma, R. A., Medlyn, B. E., Kelly, J. W. G., and Prentice, I. C.: How should we model plant responses to drought? An analysis of stomatal and non-stomatal responses to water stress, Agric. For. Meteorol., 182–183, 204–214, https://doi.org/10.1016/j.agrformet.2013.05.009, 2013.