the Creative Commons Attribution 4.0 License.
the Creative Commons Attribution 4.0 License.
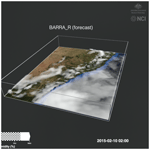
BARRA v1.0: kilometre-scale downscaling of an Australian regional atmospheric reanalysis over four midlatitude domains
Chun-Hsu Su
Nathan Eizenberg
Dörte Jakob
Paul Fox-Hughes
Peter Steinle
Christopher J. White
Charmaine Franklin
Regional reanalyses provide a dynamically consistent recreation of past weather observations at scales useful for local-scale environmental applications. The development of convection-permitting models (CPMs) in numerical weather prediction has facilitated the creation of kilometre-scale (1–4 km) regional reanalysis and climate projections. The Bureau of Meteorology Atmospheric high-resolution Regional Reanalysis for Australia (BARRA) also aims to realize the benefits of these high-resolution models over Australian sub-regions for applications such as fire danger research by nesting them in BARRA's 12 km regional reanalysis (BARRA-R). Four midlatitude sub-regions are centred on Perth in Western Australia, Adelaide in South Australia, Sydney in New South Wales (NSW), and Tasmania. The resulting 29-year 1.5 km downscaled reanalyses (BARRA-C) are assessed for their added skill over BARRA-R and global reanalyses for near-surface parameters (temperature, wind, and precipitation) at observation locations and against independent 5 km gridded analyses. BARRA-C demonstrates better agreement with point observations for temperature and wind, particularly in topographically complex regions and coastal regions. BARRA-C also improves upon BARRA-R in terms of the intensity and timing of precipitation during the thunderstorm seasons in NSW and spatial patterns of sub-daily rain fields during storm events. BARRA-C reflects known issues of CPMs: overestimation of heavy rain rates and rain cells, as well as underestimation of light rain occurrence. As a hindcast-only system, BARRA-C largely inherits the domain-averaged bias pattern from BARRA-R but does produce different climatological extremes for temperature and precipitation. An added-value analysis of temperature and precipitation extremes shows that BARRA-C provides additional skill over BARRA-R when compared to gridded observations. The spatial patterns of BARRA-C warm temperature extremes and wet precipitation extremes are more highly correlated with observations. BARRA-C adds value in the representation of the spatial pattern of cold extremes over coastal regions but remains biased in terms of magnitude.
- Article
(4102 KB) - Companion paper
-
Supplement
(1394 KB) - BibTeX
- EndNote
At horizontal kilometre scales (1–4 km), convection-permitting models (CPMs) have provided a step change in weather forecasting capabilities, particularly for forecasting rainfall and cloud cover (e.g. Lopez et al., 2009; Mailhot et al., 2010; Brousseau et al., 2016; Clark et al., 2016) over local regions with complex terrain or land–sea boundaries (Calmet et al., 2018). Similarly, CPMs have provided new insights for regional climate projections (e.g. Argüeso et al., 2014; Prein et al., 2015; Kendon et al., 2017; 2019) beyond current global models. For instance, regional CPMs have suggested that future increases in short-duration precipitation extremes are larger than what can be expected from increases in atmospheric moisture alone (Kendon et al., 2021, and references therein). Major efforts are underway toward refining the horizontal resolution of global climate models to the kilometre scale (Schär et al., 2020). Extreme weather events such as thunderstorms, damaging winds, and hailstorms are better represented in higher-resolution models (Walsh et al., 2016). Current general practice is that grid spacings less than about 4 km are required to explicitly model small convective cloud processes, replacing parameterizations of moist convection. This avoids several issues seen in parameterized convection schemes used in models with a grid spacing greater than 10 km (Lean et al., 2008) and the “grey zone” issues in mesoscale (4–10 km) models (Gerard et al., 2009). A common assumption of traditional convective parameterizations is that cloud fields adjust so much more rapidly than the processes forcing them that this adjustment can be modelled as instantaneous. Such schemes thus have no “memory” of the meteorological flow, leading to unrealistic model behaviours. Models with parameterized convection exhibit premature convective initiation, a misrepresented diurnal cycle of precipitation, overestimation of drizzle occurrence, underestimation of extreme rainfall (Lean et al., 2008; Clark et al., 2016), fewer identifiable mesoscale convective systems with less structure (Done et al., 2004), and rainfall coastal locking whereby precipitation generated over the sea does not penetrate inland (Bureau of Meteorology, 2018). When the parameterization scheme is used at a resolution finer than 10 km, it also tends to produce intermittent on–off behaviour of deep convection (Gerard et al., 2009).
By contrast, CPMs can represent deep convection and mesoscale convective organization explicitly on the model grid. Explicit modelling of convection better captures precipitation persisting across orographic or land–sea boundaries by the advection of clouds and precipitation. Better representation of topography in CPMs also leads to improved wind circulation patterns and resulting vertical velocities (e.g. Fosser et al., 2015). Improved modelling of the interactions between storm cells and their organizations should improve the estimation of damaging winds. Many studies have found a better diurnal cycle of tropical convection over land, cloud vertical structure, and coupling between moisture convection and convergence in CPMs (Stein et al., 2015; Leutwyler et al., 2017). A finer grid resolution can improve the flow and wind simulation over the recirculation zone behind the escarpment of a hill, and higher vertical grid resolution improves simulation on the lee side of hills (Ma and Liu, 2017).
These benefits from using CPMs are yet to be fully realized in many atmospheric reanalyses. Atmospheric reanalyses combine prior knowledge of physical processes captured in the models with observations from a diverse range of instruments to form spatially complete representations of the historical atmospheric conditions. They are therefore invaluable for applications concerned with local weather processes, climate signals, or events that were not fully observed such as climate monitoring and change assessments (Kendon et al., 2017; 2019), renewable energy assessment (e.g. Frank et al., 2020), and hazard management (e.g. Vitolo et al., 2019). Global-scale reanalyses have advanced in quality and quantity during the past 3 decades with improvements to models, data assimilation methods, the number of observations, and ensemble methods (Kalnay et al., 1996; Ebita et al., 2011; Gelaro et al., 2017; Dee et al., 2011), as well as with increasing spatial resolution. The latest addition, ERA5 (Hersbach et al., 2020), has a horizontal spacing of 31 km. Users of reanalyses have called for development towards finer spatial and temporal scales, i.e. below 10 km horizontal spacing and sub-daily time intervals (Gregow et al., 2016). Such scales are needed in localized climate monitoring for which local-scale mechanisms influenced by complex topography, coastlines, and convective processes are responsible for local climate features and feedbacks.
Departing markedly from global reanalyses are the regional reanalyses that use limited-area models at higher horizontal resolutions over sub-regions, e.g. North America (Mesinger et al., 2006), the Arctic polar region (Bromwich et al., 2016), Europe (Borsche et al., 2015, and references therein), India (Mahmood et al., 2018), and Australia (Su et al., 2019). These reanalyses use grid lengths of the order of 10 km to improve the representation of sub-daily variability and near-surface weather. These are generally produced with global atmosphere model configurations that include convection parameterizations (e.g. Su et al., 2019). Recently, Wahl et al. (2017) overcame this with a 7-year 2 km reanalysis over Germany with the assimilation of conventional observations and radar-derived rain rates and showed improved spatiotemporal variability and intensity frequency of precipitation. Such a direction in the development of the reanalyses, combined with higher-resolution regional projections, can offer a more accurate picture of changes in regional meteorology and extreme weather in the changing climate.
Dynamical downscaling is frequently used to estimate the dynamic variables at scales finer than those of coarser-resolution climate or weather models. This approach is undertaken at the Bureau of Meteorology (Bureau) in Australia to produce kilometre-scale weather forecasts and/or ensemble forecasts over major cities, and a 1.5 km forecast-only model has been used since 2017 for added value over the Bureau's lower-resolution global system. This goal is also pursued in the Bureau of Meteorology Atmospheric high-resolution Regional Reanalysis for Australia (BARRA; Jakob et al., 2017) project. Within this context, this paper is a companion paper to Su et al. (2019) wherein an Australian regional 12 km reanalysis system (BARRA-R) was presented. Here we describe dynamical downscaling of BARRA-R using the UK Met Office (UKMO) Unified Model (UM) at a 1.5 km horizontal grid length over four midlatitude sub-regions of Australia (Fig. 1) over 29 years from January 1990 to February 2019. These regions are chosen in partnership with state fire and emergency management agencies because of the important advantages that dynamically downscaled reanalyses can provide for local-scale planning and management to reduce future risks due to extreme weather events such as bushfires. The four downscaling models, collectively referred to as BARRA-C, yield gridded products that include a variety of 10 min to hourly surface parameters describing both weather and land surface conditions as well as hourly upper-air parameters covering the troposphere and stratosphere with a 40 km model top on 70 model levels and 37 pressure levels.
This paper describes the model and the experimental design in Sect. 2, and Sect. 3 provides the first assessment of the downscaled reanalysis with a focus on screen-level temperature, 10 m wind, and precipitation. Comparisons with BARRA-R and global reanalyses are also made to illustrate the added value of BARRA-C. Our findings are further discussed in Sect. 4, with an overall summary in Sect. 5.
The development of BARRA is based on the Bureau's operational deterministic numerical weather prediction (NWP) forecasting over the Australian region using the Australian Community Climate and Earth-System Simulator systems ACCESS-R and ACCESS-C (Puri et al., 2013). The operational version at the time (Australian Parallel Suite 2) of ACCESS-R is the national 12 km 6-hourly analysis–assimilation and 3 d forecasting system (Bureau of Meteorology, 2016). ACCESS-R has provided the initial and boundary conditions to initialize and constrain ACCESS-C over six smaller domains centred at the Australian cities until 2020 (Bureau of Meteorology, 2018). The APS2 ACCESS-C dynamically downscales ACCESS-R to provide 6-hourly, 1.5 d forecasts at 1.5 km horizontal resolution. The relation between BARRA-R and BARRA-C mirrors this system but is implemented with a shorter forecast (or hindcast) range and a newer version of the meteorological forecast model and science configuration (Sect. 2.1). In particular, BARRA-R is nested in ERA-Interim reanalysis (Dee et al., 2011) and includes four assimilation and hindcast cycles per day (Su et al., 2019). BARRA-C is a hindcast-only system that inherits the analysis from BARRA-R as initial conditions. While BARRA-C refers to the collection of the four sub-domain models, we use BARRA-AD, BARRA-PH, BARRA-SY, and BARRA-TA to denote individual domains centred at Adelaide (South Australia, AD), Perth (Western Australia, PH), Sydney (New South Wales, SY), and Tasmania (TA) (Fig. 1).
The PH and AD domains are similar in terms of climate, having arid deserts north of their domains, temperate dry hot or warm summers near coasts, and arid steppe climate in between (Peel et al., 2007). SY has a temperate climate with warm to hot summers and lacks a dry season, while TA differs with a cooler summer. Cool-season perennial grass (C3) is the dominant vegetation over the southwestern region of PH and the near-coast region of AD, and broadleaf trees are widespread in the SY and TA domains (Fig. S1 in the Supplement). There are several large ephemeral salt lakes (e.g. Lake Torrens, Lake Gairdner) in the AD domain, and these are modelled as land points with bare soil. Of the four domains, only SY has a distinct thunderstorm season, which occurs during November–March. Thunderstorms are far less frequent in the other three domains due to lower incidence of warm, humid air masses and also prevalent stable conditions during the potentially favourable warmer months owing to a subtropical high-pressure belt over or near these areas (Kuleshov et al., 2002). In contrast to PH and AD, the SY and TA domains are topographically complex. The Great Dividing Range extends north to south through the SY domain, and the TA domain features low mountains and a landscape of plateaus.
2.1 Forecast model
The UM (Davies et al., 2005; version 10.6) is the grid-point atmospheric model used in BARRA and ACCESS. It uses a non-hydrostatic, fully compressible, deep atmosphere formulation, and its dynamical core (Even Newer Dynamics for General atmospheric modelling of the environment, ENDGame) solves the equations of motion using mass-conserving, semi-implicit, semi-Lagrangian (SL), time integration methods (Wood et al., 2014). The prognostic variables are three-dimensional wind components, virtual dry potential temperature and Exner pressure, dry density, and mixing ratios of moist quantities. These variables are discretized horizontally onto a regular longitude–latitude grid with Arakawa-C staggering (Arakawa and Lamb, 1977) and vertically with the Charney–Phillips staggered grid (Charney and Phillips, 1953). The BARRA-C model has a horizontal spacing of 0.0135∘ × 0.0135∘ (about 1.5 km at the Equator), and its vertical levels follow the modelled orography at the surface and relax to surfaces of uniform radial height after 62 model levels (∼ 17 km above ground) in the upper atmosphere, with the model top height at 40 km. At this resolution, the model is run with an integration time step of 60 s.
Table 1An overview of major differences between BARRA-C, BARRA-R, and the midlatitude version of RAL1 (RAL1-M). The configurations for BARRA-R are described in Su et al. (2019) and Walters et al. (2017), and those for RAL1-M are described in Bush et al. (2020).
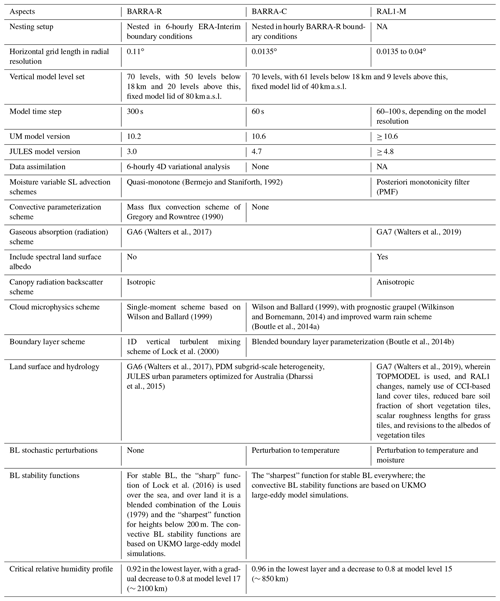
The science configuration of the model in BARRA-C is based on the UK Met Office operational suite OS36, while BARRA-R is based on the Global Atmosphere (GA6) configuration of Walters et al. (2017). While the OS36 model configurations preceded the release of the first UM Regional Atmosphere and Land (RAL1) configuration of Bush et al. (2020), BARRA-C implements some of the improvements from RAL1. Table 1 summarizes the differences between BARRA-C, BARRA-R, and RAL1. The physical parameterization schemes common to BARRA-C and BARRA-R include a variant of Wilson and Ballard (1999) for mixed-phase cloud microphysics, the large-scale cloud scheme of Smith (1990), and the radiation scheme of Edwards and Slingo (1996), all of which have been improved since publication. BARRA-R uses a convection parameterization scheme based on Gregory and Rowntree (1990), which is not used in BARRA-C. With a grid length of 1.5 km, the horizontal grid length approaches the depth of the boundary layer (Hanley et al., 2015), and as such it is no longer appropriate to use the 1D boundary layer parameterization that restricts mixing to the vertical. BARRA-C therefore uses a blended boundary layer parameterization (Boutle et al., 2014b) whereby the scheme transitions from the 1D vertical turbulence scheme of Lock et al. (2000) to a 3D subgrid turbulence scheme based on Smagorinsky (1963) as a function of the grid length to the turbulent length scale (Halliwell et al., 2007). The mixing length, which can be tuned to control the smoothness of the fields and the number of small cells, is taken as 300 m, which is used in operational systems.
The cloud scheme uses a profile of critical relative humidity values (RHcrit), above which a grid box contains some cloud if the relative humidity is exceeded. Based on the assumption that there should be less subgrid variability in humidity for smaller grid boxes, BARRA-C uses higher RHcrit values that BARRA-R in the lowest few layers, decreasing smoothly above to 0.8.
Without the convection parameterization scheme, BARRA-C relies on the model dynamics to represent convective motions. While convection remains unresolved in 1.5 km models, removal of the cumulus parameterization has been shown to result in more realistic behaviour (Clark et al., 2016). In particular, the model can explicitly capture processes with convective-like characteristics, which can subsequently drive scales that the model can properly resolve. BARRA-C also reduces the appearance of unrealistically strong vertical velocities and “grid-point storms” seen in BARRA-R due to the inability of convective parameterization to stabilize the air column (Su et al., 2019). Nevertheless, convection can remain under-resolved, leading to cases of small, shallow showers that are too early or no rain at all. The midlatitude version of RAL1 therefore includes stochastic perturbations of temperature and moisture as well as relatively weak turbulent mixing to encourage the model fields to be less uniform and help convection to initiate. BARRA-C does not use stochastic perturbations for moisture and may thus still suffer from convection initiation issues.
Another distinguishing feature of BARRA-C is the handling of mass conservation during the advection of moisture prognostic variables. This is one of the key science developments in RAL1. BARRA-C and RAL1 use the zero lateral flux scheme of Zerroukat and Shipway (2017) for moisture conservation at the model's lateral boundaries, avoiding spurious extreme precipitation caused by the SL treatment of moisture variables near partially resolved convection.
BARRA-C is missing some of the configuration improvements introduced in RAL1 because production runs had already commenced. BARRA-C does not include a set of changes to the representation of the land surface and the canopy radiation model, which improve the damped diurnal cycle issue in near-surface temperatures. BARRA-C also does not benefit from the improved treatment of gaseous absorption in both longwave and shortwave regimes in GA7 and RAL1, which improves interaction with band-by-band aerosol and cloud forcing.
BARRA uses the land surface scheme of Best et al. (2011), implemented in the Joint UK Land Environment and Simulator (JULES). It describes a 3 m four-layer soil column with subsurface temperature updated using a heat diffusion equation and with vertical moisture flux estimated using the Richard's equation and Darcy's law. The soil hydraulics are computed using the van Genuchten equation. It uses a nine-tile approach to represent subgrid-scale heterogeneity in land cover, with the surface of each land point subdivided into five vegetation types (broadleaf trees, needle-leaved trees, temperate cool-season (C3) grass, tropical warm-season (C4) grass, and shrubs) and four non-vegetated surface types (urban, inland water, bare soil, and land ice). Urban surfaces are represented only by a single urban tile such that street canyons and roofs are not distinguished.
The characteristics of the lower boundary, climatological fields, and natural and anthropogenic emissions are specified using static ancillary fields. These are created as per Bush et al. (2020; Table A1), with the exception of ancillaries for the land–sea mask, canopy tree heights, and land usage. The land–sea mask is created from the 1 km resolution International Geosphere–Biosphere Programme (IGBP) land cover data (Loveland et al., 2000) for SY and TA and from Shuttle Radar Topography Mission (SRTM) orography data for AD and PH. Land cover data based on the Climate Change Initiative (CCI; Hartley et al., 2017) are not adopted here as their mapping to the nine land surface tiles over the Australian region remains untested. The canopy tree heights are derived from satellite light detection and ranging (lidar; Simard et al., 2011; Dharssi et al., 2015). The land usage ancillary, created from IGBP, is modified for AD and PH to match the water fractions in the Water Observations from Space (WOfS; Mueller et al., 2016). Aerosol absorption and scattering in the radiation scheme assume climatological aerosol properties. A climatological ozone field is also prescribed.
2.2 Initial and boundary conditions
The BARRA-C model hindcast is re-initialized with 6-hourly initial conditions at the synoptic hours t0 = 00:00, 06:00, 12:00, and 18:00 UTC created by downscaling from BARRA-R analyses (Fig. S2 in the Supplement). These fields are taken from the centre of BARRA-R's 6 h analysis windows. A two-component reconfiguration approach is used in which BARRA-R winds, moisture, and temperature are downscaled separately with different resolution topography sets to remove model instability due to large horizontal topography gradients. BARRA-C is further constrained by BARRA-R at the lateral boundaries without nudging based on the prescription described in Bush et al. (2020) and a boundary rim width of 0.34∘. The boundary conditions force the development of the larger-scale features within the BARRA-C domains. These setups follow the Bureau's NWP system and ensure that the benefits of the BARRA-R analysis are inherited by BARRA-C, wherein the nested model is treated as a physically consistent interpolator of the driving model.
The JULES soil moisture and temperature are prescribed by BARRA-R. Consistent with BARRA-R, daily sea surface temperature and sea ice 0.05∘ × 0.05∘ analysis from reprocessed (1985–2007; Roberts-Jones et al., 2012) and near-real-time Operational Sea Surface Temperature and Ice Analysis (OSTIA; Donlon et al., 2012) are used as lower boundaries over the water after being interpolated to the BARRA-C grid. The near-real-time data are used from January 2007.
Each hindcast in BARRA-C is a 9 h simulation but only 6 h are used. The model data during the first 3 h are discarded as the fine detail is only partially established from the coarse-resolution initial conditions due to model spin-up. Therefore, the hindcast fields between t0 + 4 h and t0 + 9 h form the BARRA-C datasets. Such a hindcast length is considered short but is chosen to meet computational constraints when regular re-initialization is needed for running the model for such an extended period. One clear limitation of our setup is that model spin-up artefacts are expected to still be present, particularly for convective clouds and rain.
Our assessment focuses on near-surface variables and precipitation as the aim of BARRA-C is to capture small-scale local weather phenomena which are most apparent near the surface. BARRA-C hindcasts are evaluated against point-scale station observations for screen-level temperature, 10 m wind speed (Sect. 3.1), and precipitation (Sect. 3.3). They are also compared with gridded daily analyses of these observations for temperature (Sects. 3.2 and 3.6) and precipitation (Sects. 3.4 and 3.6). Added skill in BARRA-C is illustrated by comparing these variables against BARRA-R, ERA-Interim hindcasts, and ERA5 hourly analyses (ERA5 hindcasts only for precipitation). To increase the diversity of models used in our intercomparison, we also include the Modern-Era Retrospective analysis for Research and Applications-2 (MERRA-2; Gelaro et al., 2017) hindcasts. A scale-selective evaluation of extreme storms is conducted in Sect. 3.5 using radar observations available over the SY domain. Finally, an added-value (AV) method is used to quantify improvements between BARRA-C and BARRA-R in the representation of extreme daily maximum and minimum temperature as well as daily rainfall from gridded observations. Readers are referred to Sect. A in the Supplement for details of the various reference datasets considered in our assessment.
3.1 Point evaluation of screen temperature, 10 m wind speed, surface pressure
The t0 + 6 h model hindcasts of screen-level temperature, 10 m wind speed, and surface pressure are evaluated against land station observations during the 2010–2012 period, following the approach of Su et al. (2019). These observations have no direct relation to BARRA-C, since there is no analysis in BARRA-C, and they are not used in the associated BARRA-R cycle t0. These fields are interpolated from the model levels using surface similarity theory (Walters et al., 2017). Our benchmarks include BARRA-R and ERA-Interim t0 + 6 h hindcasts, the MERRA-2 hourly time-averaged hindcast fields, and the ERA5 hourly analysis. The models are interpolated to be coincident with the observed locations and times. As the observations are irregularly distributed in time, all observations within a t0 + 5 to t0 + 7 h time window for t0 = 00:00 and 12:00 UTC are considered. The root mean square difference (RMSD), Pearson's linear correlation, additive bias, and variance bias are calculated at each station between observed (do) and model (dm) data. Additive bias is defined as Bias , where E(⋅) is the expectation operator, and the variance bias as so as to capture differences in the dispersion, where var(⋅) computes the variance in time. This assessment does not serve to provide information on the true quality of the various reanalyses at their native resolutions; rather, it indicates whether the models contain finer-scale information captured by point measurements. Based on Di Luca et al. (2016), we distinguish three distinct regions with characteristics of complex topography (stations with an elevation higher than 500 m – topo), land–sea contrasts (stations that are within 1.5∘ of the coast – coast), or relatively smooth terrain (stations far from the coast – flat) (Fig. S3 in the Supplement).
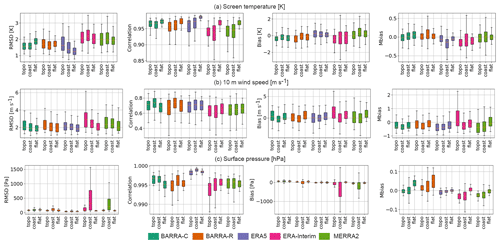
Figure 2Box plots showing the distribution of evaluation scores of various models for (a) screen-level temperature, (b) 10 m wind speed, and (c) surface pressure across the four BARRA-C domains. Three regions are analysed separately: coastal (“coast”), complex topography (“topo”), and flat, and the models are distinguished by colours. The scores are calculated from model hindcasts valid between 05:00–07:00 UTC and 17:00–19:00 UTC against observations during 2010–2012.
The comparisons of scores across all BARRA-C domains are shown in Fig. 2. For temperature, BARRA (i.e. BARRA-R and BARRA-C) and ERA5 show better agreement with the station data than the other coarser reanalyses for most metrics. For instance, BARRA-C shows lower RMSD than ERA-Interim at 80 % of stations. BARRA shows greater contrast from the global reanalyses than between them. ERA5 shows a warm (additive) bias, while BARRA appears cooler. ERA-Interim and ERA5 generally show less variability in temperature than observations (Mbias < 0), while the other models tend to have more similar temperature variability with observations. This is related to the cold bias in ERA during high temperature (shown in the next section). On average, BARRA scores lower for RMSD than ERA5 at elevated stations (e.g. Snowy Mountains in SY) and smaller for Mbias at near-coast stations. In general, BARRA-C shows more visible improvements to BARRA-R at stations near coasts or over complex topography in terms of RMSD, correlation, and Mbias (Fig. S3 in the Supplement). Consequently, BARRA-TA scores higher than BARRA-R on average. However, BARRA-C shows higher RMSD in the flat regions than in the other regions, unlike the other reanalyses. The degradation is small (within 0.6 K in terms of RMSD), and for AD, this is related to overdispersion (Mbias > 1).
For 10 m wind speed, BARRA-C, BARRA-R, and ERA5 similarly exhibit lower RMSD and higher correlation with the station data than the other global reanalyses, and the differences between these three models are not pronounced. BARRA's largest enhancement to ERA-Interim is found at elevated stations and near coasts, benefitting Tasmania particularly. Contrasting with BARRA-R, BARRA-C tends to show lower RMSD at these stations (Fig. S3, Supplement), and while we observe higher RMSD in BARRA-C, the difference is within 1 m s−1. The wind estimated by all the models tends to be underdispersed (Mbias < 1), relating to a positive (negative) bias during light (strong) wind conditions. Such a model underdispersion is more striking in the TA and SY domains than in the other domains as well as over coastal regions.
For surface pressure, the higher-resolution models, including ERA5, show markedly lower RMSD near coasts. There is very good agreement between ERA5 and the observations. BARRA-C shows some improvements over BARRA-R in correlation and Mbias as well as over coastal regions and mountains.
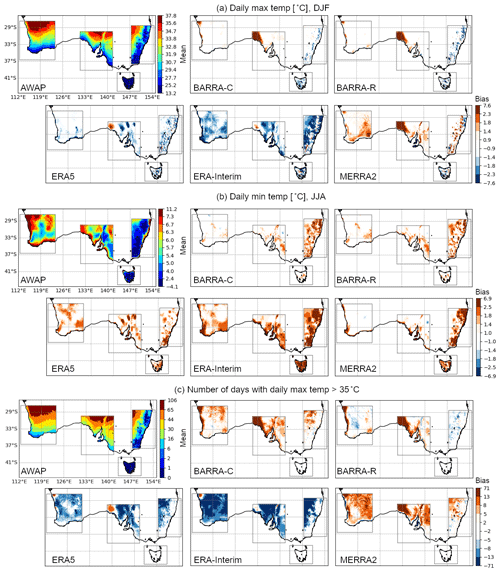
Figure 3Mean difference in (a) summer (DJF) daily maximum temperature, (b) winter (JJA) daily minimum temperature, and the (c) number of days with temperature exceeding 35 ∘C in various models during 1990–2018 with respect to AWAP. The models are regridded onto the AWAP grid using nearest-neighbour interpolation.
3.2 Comparison with gridded analysis of daily maximum and minimum screen temperature
The reanalyses are compared against a gridded daily 0.05∘ × 0.05∘ analysis of observed maximum and minimum screen temperature from the Australian Water Availability Project (AWAP; Jones et al., 2009) in Fig. 3. BARRA outperforms the driving model ERA-Interim in reducing the cold (warm) bias during summer DJF (winter JJA), particularly over the SY and TA domains. BARRA-C shows a smaller extent of summer cold bias in daily maximum temperature over the Great Dividing Range than both BARRA-R and ERA5, but it shares a similar bias with BARRA-R elsewhere. BARRA and the global reanalyses also exhibit a considerable warm bias in the northwest of the AD domain, the Nullarbor Plain, but this is likely an artefact of the AWAP station density and is discussed later.
The warm bias in daily minimum temperature in winter is also similar between BARRA-C and BARRA-R. BARRA-C has largely inherited the biases from BARRA-R but with small local-scale differences. Despite such similarities in summer bias, there are more hot days (i.e. days exceeding 35 ∘C or 308.15 K) in Fig. 3c in BARRA-C than in BARRA-R over inland Australia. By contrast, the summer cold temperature bias in both ERA reanalyses is also reflected by fewer hot days and vice versa for MERRA-2. Further analysis of the temperature extremes is considered in Sect. 3.6.
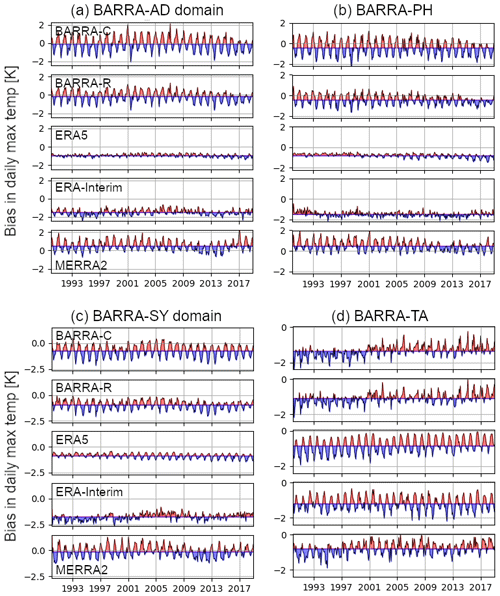
Figure 4Time series of monthly mean difference in daily maximum temperature averaged over various BARRA-C domains with respect to AWAP. The time series are shaded around their individual 1990–2018 means.
Figures 4 and 5 examine the inter-seasonal and inter-annual variations in temperature bias with respect to AWAP for daily maximum and minimum temperature, respectively. They are similar between BARRA-C and BARRA-R, with BARRA-C showing slightly wider inter-seasonal variability. The inter-seasonal range of bias in BARRA is around 2 K, which is similar to ERA-Interim and MERRA-2 in most domains but is larger than ERA5 with the exception of TA. For AD and PH, the daily maximum temperature is positively biased during summer months (DJF) and is negatively biased during winter (JJA). The negative bias in daily maximum temperature is smallest during summer for SY and TA and is largest during winter for SY. For daily minimum temperature these are reversed; e.g. the associated positive bias peaks during winter for AD, PH, and SY, and the negative bias is maximum during summer for AD and PH.
There is both inter-annual variability and a trend of the temperature biases in BARRA. For daily maximum temperature bias, there is a cooling trend in AD and PH and a warming trend in TA. These trends can also be seen in ERA5 and MERRA-2. For daily minimum temperature bias, trends in BARRA are less apparent than in ERA5 and MERRA-2. We also observe in the TA domain that BARRA shows a small warming trend with respect to AWAP.
This analysis of variability in the bias is repeated for the standard deviation of the modelled temperature and AWAP in Figs. S4 and S5 in the Supplement. BARRA-C shows a slightly wider dispersion of daily maximum temperatures than AWAP (by 0.4 K) and BARRA-R (by 0.1 K), with the exception of the TA domain. For BARRA-TA, the standard deviation of BARRA is similar to AWAP and is higher than the global reanalyses. For daily minimum temperature, both BARRA versions are similar and generally underdispersed by 0.3 K compared to AWAP.
3.3 Comparison with rain gauges over Sydney
Hourly modelled precipitation from BARRA and ERA5 is compared against observations from 27 rain gauges within a 1∘ radius around Sydney during the warmer months (NDJF) in 2008–2013 in Fig. 6. During these months convection processes dominate and can produce a distinct diurnal distribution in thunderstorm activity. The greatest frequency of severe thunderstorms occurs in November and December (Griffiths et al., 1993). ERA5 and to a lesser extent BARRA-R both underestimate the frequency of heavy rain rates > 8 mm h−1. By contrast, BARRA-C underestimates the frequency of light rain rate and overestimates heavy rates. BARRA and ERA5 also distribute rainfall differently over a day. BARRA-C shows a bimodal distribution similar to the observations despite showing too much rain leading up to the 06:00 UTC peak and too little rain during the daily minimum around 18:00 UTC. BARRA-R shows less diurnal variation in rainfall with too much rain distributed during 00:00–06:00 UTC, whereas ERA5 shows a pronounced early timing bias.
3.4 Comparison with daily rainfall analysis
Figure 7a compares the modelled precipitation against the daily rain-gauge analysis from AWAP including MERRA-2's hourly time-averaged precipitation (PRECTOTCORR) product. BARRA-C shows a wet bias over the Great Dividing Range and the southeast area of the AD domain but improves the dry bias in BARRA-R and ERA reanalyses over the eastern and western seaboards and the Fleurieu and Yorke peninsulas of South Australia. BARRA-C also shows dry biases on the western borders of the AD and SY domains, possibly due to inconsistencies with the zero lateral moisture mass flux on the boundary conditions (Sect. 2.1). A striking difference between BARRA and the global reanalyses is over western Tasmania where the latter displays a dry bias.
In Fig. 7b, BARRA-R, ERA5, and ERA-Interim show too few heavy rain days (> 10 mm d−1) over the coastlines, SA peninsula, and western Tasmania. BARRA-C improves on this but generally simulates more heavy rain days than other reanalyses and too few moderate–light rain days (< 10 mm d−1, not shown) in all domains. BARRA-R and MERRA-2 generally show too many light rain days, and the ERA reanalyses show too many light rain days in SY and eastern Tasmania and too few in AD, PH, and western Tasmania.
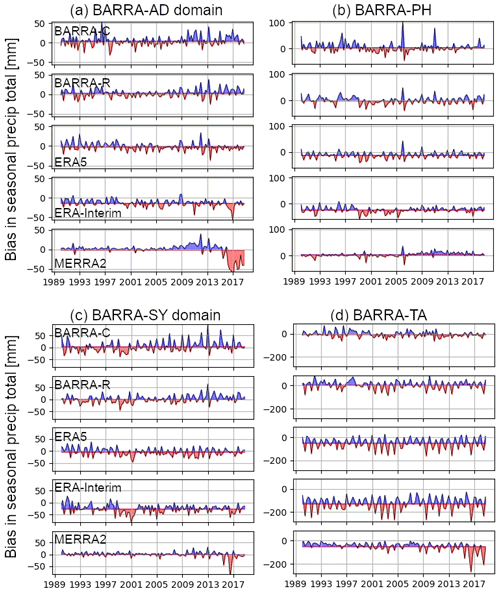
Figure 8Mean difference in seasonal precipitation totals over various BARRA-C domains with respect to AWAP. Black curves are shaded around the 1990–2018 means. Note that the y axes in (a–d) are different.
The inter-seasonal and inter-annual variations in precipitation bias with respect to AWAP are plotted in Fig. 8. As with temperature (Fig. 4), they are similar between BARRA-R and BARRA-C, although the latter shows a larger range in all BARRA-C domains except TA. In particular, a wet bias is generally observed during the wet season (JJA for AD, DJF for PH), wetter months (JJA for TA), and thunderstorm season (DJF for SY). A dry bias generally occurs during the dry season or drier months, i.e. SON for AD, PH and TA. This is consistent with the tendency of BARRA-C to overestimate heavy rain rates and underestimate light rain occurrence. Some of the inter-annual variations in bias are clearly common amongst BARRA and the global reanalyses, e.g. in AD and PH domains during the Millennium Drought (1996–2009) when the various models share a dry bias. BARRA also shows different trends to the global reanalyses. There is a wetting trend post-2009 for BARRA in AD, but this is opposite for the other models. In SY, BARRA also displays a wetting trend, while ERA trends are drier.
It should, however, be noted that, as is often found for gridded interpolated data, AWAP tends to underestimate the intensity of extreme rainfall events and overestimate the frequency and intensity of low rainfall events (King et al., 2012). The errors are larger at high elevations (SY and TA) where gauges are fewer, when there is frozen precipitation, and/or topography is exposed to prevailing winds (Chubb et al., 2016).
3.5 Storms over Sydney
The point gauge-based assessment in Sect. 3.3 is harsher to higher-resolution models than coarser models due to the compound error of space and time near-misses, which increases as the grid cells shrink. Therefore, we compare the simulated rainfall from BARRA-SY with the Bureau's radar nowcasting rainfall product (Rainfields2; Seed et al., 2007) and use the fractions skill score (FSS) to allow assessment at different spatial scales following the approach described in Roberts and Lean (2007), Jermey and Renshaw (2016), and Acharya et al. (2020). The FSS provides an evaluation of the rainfall skill as a function of spatial resolution. The radar product, blended with gauge observations using conditional merging (Sinclair and Pegram, 2005), is available from 2014 onwards on a mosaic grid consisting of the domains of multiple radars. Following Acharya et al. (2020), the largest 36 storm events during 2014–2016 are selected based on domain-averaged daily precipitation.
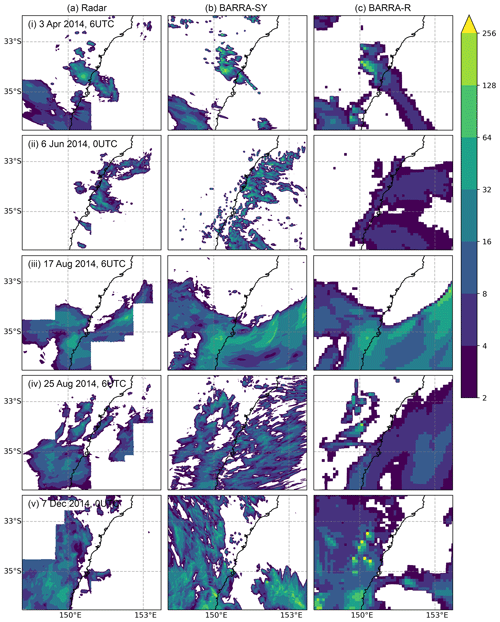
Figure 9Simulated 6 h rainfall accumulation [mm] in BARRA-SY and BARRA-R compared with rainfall derived from the composite radar network around the Sydney area for five events.
FSS is categorized as a “neighbourhood verification” metric (Ebert, 2009) in which fractional coverages of grid cells close to observations are valued equally. The FSS tallies the relative number of “hits” between the model and the observation at different spatial scales and different rain thresholds. An FSS of 1 represents a perfect forecast wherein the number of cells with precipitation above a threshold within a neighbourhood is identical between the model and observation grids for all possible neighbourhoods. Here, BARRA hourly rain rates are regridded to the radar grid of 1.5 km, and the accumulated rain amounts over moving 6 h windows are analysed. From the 36 multi-day storm event set, 1323 different 6 h events are produced using a moving window. FSS is computed for each 6 h event for each model, and then the scores are aggregated to give an average for all events. Given that inherent bias between the observation and the models exists due to differences in their representativity, and also to focus on the spatial accuracy of the models, we use percentile-based thresholds computed across all the storm events. This ensures that the model and observed rain fields have an identical fraction of rain events for each threshold value (explained further in Sect. E in the Supplement). Figure 9 illustrates the striking differences between BARRA-R and BARRA-SY for five events in 2014. BARRA-SY can show more realistic organization in the 1.5 km model owing to the explicit modelling of convection and can produce higher rainfall intensity. The event on 7 December 2014 in Fig. 9v illustrates a summer storm case in which BARRA-R shows rainfall accumulation lacking the spatial pattern common to convective organization that is evident in BARRA-SY and in observations. BARRA-R also shows excessive grid-point precipitation over the mountains, which is absent in both observations and BARRA-SY. At the same time, BARRA-SY can show too many cells (Fig. 9ii), which can produce streaks of light rainfall (Fig. 9iv).
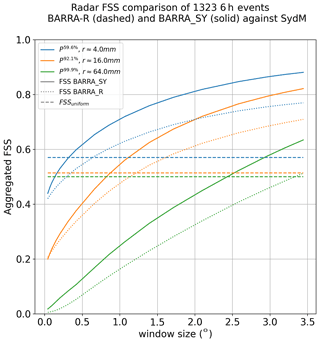
Figure 10Aggregated FSS across 1323 6 h storm events as a function of neighbourhood distance (degrees) for 6 h rainfall above three percentile thresholds (distinguished by colours, percentile values, and observed amount in mm). The solid curves indicate the score for BARRA-SY, dotted curves for BARRA-R, and the dashed horizontal lines the uniform score (FSSuniform) for each threshold as specified by Roberts and Lean (2007).
The FSS results in Fig. 10 show that BARRA-SY is more skilful over all scales than BARRA-R for all threshold levels. FSSuniform is the FSS of a forecast field with a uniform fractional coverage equal to the fraction of points observed with any rain (> 0.2 mm h−1). Scores greater than FSSuniform are considered skilful. For the lowest threshold (56 %, i.e. 4 mm in the observed radar values), the uniform score (FSSuniform) is reached at scales of 0.3∘ (BARRA-SY) and 0.65∘ (BARRA-R). At the highest threshold (99.9 %, 64 mm), the uniform score is reached at scales of 2.4∘ and 3.35∘, respectively. The contrast between the two BARRA FSSs is therefore greater at the higher precipitation thresholds. FSSs for higher rainfall thresholds are also generally lower as the area of rain being sampled becomes more localized and is more challenging to reproduce correctly in the models.
3.6 Added-value analysis for temperature and rainfall extremes
We apply an approach similar to Di Luca et al. (2015) to quantify the added value (AV) in the representation of climatological extremes from BARRA-C by comparing its skill to the skill in BARRA-R. The warm extremes of daily maximum temperature, the cold extremes of daily minimum temperature, and the wet extremes of daily precipitation are assessed against AWAP. The statistics for extremes (X) are given by the percentiles of the daily temperature and precipitation values over the 29-year time period. We use from Di Luca et al. (2016), where d defines a distance metric between the model-derived and AWAP-derived statistics computed across the grid cells. To capture both the total errors and spatial patterns of the statistics, we let = E[(A−B)2] to define the mean squared error and also use = , with R as Pearson's correlation. Larger positive AV values suggest smaller errors in BARRA-C than in BARRA-R and thus substantial added value by the downscaling of BARRA-R.
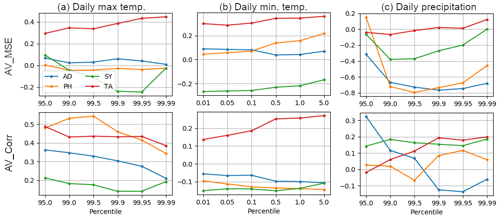
Figure 11Added-value (AV) analysis of the (a) warm extreme of daily maximum temperature, (b) cold extreme of daily minimum temperature, and (c) wet extreme of daily precipitation for all four BARRA-C domains.
Figure 11 plots AV scores for different BARRA-C domains, showing that AV is not gained consistently across the percentiles, variables, and domains. For warm extremes of daily maximum temperature, BARRA-C shows positive AVMSE over BARRA-R in the TA and AD domains. Low or negative AVMSE for AD, PH, and SY (inland region) is mainly due to the warm and wet bias in BARRA-C seen in Figs. 3c and 6a and b. The positive AVCorr indicates that BARRA-C captures the spatial patterns of the warm extremes across the domains, particularly over the coastal and high-topography regions (see also Fig. S6 in the Supplement).
For cold extremes in Fig. 11b, BARRA-C still shows positive AVMSE over all domains except SY. This AV is mostly contributed by coastal regions, as seen in Fig. S6. Negative AVMSE in SY is related to warmer cold extremes, particularly over the Great Dividing Range. Positive AVCorr is seen in TA but not in the other domains. However, it should be noted that the BARRA versions are generally strongly correlated with AWAP, with R mostly between 0.7 and 0.9.
AV from BARRA-C for wet extremes of precipitation relates more to the spatial patterns of the extremes (Fig. 11c). There is negative AVMSE for all domains except TA, which remains near zero, highlighting the BARRA-C rainfall bias. On the other hand, the AVCorr is positive for all domains except AD for the three highest rainfall percentiles, which indicates better spatial correlation of rainfall than BARRA-R.
The BARRA-C 1.5 km models are strongly forced by BARRA-R with both initial conditions every 6 h and hourly boundary conditions. BARRA-C has therefore inherited much of the same quality of BARRA-R; however, it does provide additional information about local near-surface meteorological conditions. BARRA-C provides better representative point-scale estimates of screen temperature, 10 m wind speed, and surface pressure at some areas with complex topography or near coastlines, and it mainly inherits the skills of BARRA-R over other areas. The degradation from BARRA-R is slight, within (RMSD) 0.6 K for temperature and 1 m s−1 for wind speed.
BARRA-C also shows a 10 m wind speed bias that is positive (negative) during light (strong) wind conditions, similar to the bias in BARRA-R. Many factors such as boundary layer mixing, form drag for subgrid orography, and surface properties can influence wind estimation over land. The representation of the stable boundary layer remains challenging due to the multiplicity of physical processes and their complex interactions, i.e. turbulence, radiation, land surface coupling and heterogeneity, and turbulent orographic form drag. Models typically suffer biases in 2 m temperature and wind speed under such conditions (Steeneveld, 2014, and references therein).
BARRA-C also inherits the domain-averaged biases in daily maximum and minimum temperature from BARRA-R. It reduces some bias over the Great Dividing Range but simulates more hot days than seen in observations, particularly over inland Australia. However, in some inland regions the AWAP analyses are poorer quality due to low observing station density. For example, in the northwest of the AD domain – the Nullarbor Plain – both BARRA and the global models show a large warm bias in daily maximum temperature; however, the station density used in AWAP is less than two per square degree (Sect. A in the Supplement).
The daily temperature bias varies differently in time between the four domains, with AD and PH showing a change of sign in bias between summer and winter months, while SY and TA show a persisting negative (positive) bias for daily maximum (minimum) temperatures. Such similarities between the domains may be related to their similarities in terms of climate and land cover. Bush et al. (2020) discussed the fact that changes in RAL1 for land surface representations (Table 1, Sect. 2.1) are important to improve the diurnal biases in pre-RAL1 configurations. These could benefit the biases seen over vegetated areas, particularly for daily minimum temperature in SY and TA.
Differences in land classification between BARRA and ERA reanalyses can explain some of the differences seen in the comparison of gridded daily maximum and minimum temperatures seen in Fig. 3. BARRA avoids the bias in ERA over the salt lakes in SA by modelling them with land characteristics based on IGBP, whereas ERA uses CCI.
The dry bias of higher rain rates seen in the coarser-scale models during the thunderstorm seasons in the SY domain is alleviated by BARRA-C. The underestimation of the peak rain rates in BARRA-R and ERA5 was expected from the lack of convection organization due to the use of a cumulus parameterization, whereas BARRA-C evidently shows more realistic organization and does not underestimate peak rain rates. However, the latter also exhibits too much heavy rain and not enough light rain, which is likely due to the still under-resolved convection and the model's inability to resolve detrainment from convective updrafts. This is consistent with the findings reported in other studies. For example, Lean et al. (2008) and Hanley et al. (2015) found that 1 km grid length UM simulations tend to produce cells that are too intense, too far apart, and with not enough light rain. The latter also noted insufficient small storms in both shower cases and large storm cases, as well as too many large cells in shower cases.
The short hindcast length in BARRA-C (Sect. 2.2) poses a further limitation. The rainfall excess could result from model spin-up. Extra energy (i.e. CAPE) builds up during the early time steps when there is insufficient convection, which is finally released in the form of convective precipitation in later time steps (Lean et al., 2008). Champion and Hodges (2014) have also noted that modelled precipitation intensities are most accurate when the model is initialized 12 h before the rain maxima. The moisture-conserving zero lateral mass flux boundary conditions in BARRA-C exacerbate this issue. Moisture variables are not advected across boundaries and are instead allowed to develop via physical processes in the model. These processes take some time to spin up in each hindcast, leading to a near-boundary downstream moisture bias, e.g. the western boundary of the annual rainfall maps of the AD and SY domains (Fig. 7a). These issues of precipitation with short hindcasts can be improved with an assimilation system that will allow high-resolution features to propagate from one hindcast cycle to the next (Dixon et al., 2009). In spite of these limitations, we find that BARRA-C provides a more representative rainfall climatology for heavy rain days near the coastal and mountainous regions, as well as better sub-daily rain spatial patterns.
BARRA-C simulates peaks in the diurnal distribution of precipitation better than BARRA-R and ERA5. However, we also find that precipitation may be initiated too early and grow too rapidly. Consequently, BARRA-C under-represents off-peak rain rates, resulting in an overly pronounced diurnal cycle, as seen in Fig. 4(b) for BARRA-SY in summer. This is contrary to the expectation for all models to initiate too late since subgrid-scale initial plumes cannot be represented. The early initiation bias in BARRA-R is due to the CAPE-based trigger mechanism of the convection scheme (Lean et al., 2008). In the case of the kilometre-scale UM, there are likely several reasons. Hanley et al. (2015) partly attributed timing bias in convection initiation, which is too early in shower cases and too late in the larger storm cases, to unresolved convection at the kilometre-scale grid length. Other reasons may be that stochastic perturbations (Sect. 2.1) or model responses to the pre-convective profile are too strong or that the profile has inadequate convective inhibition (CIN). The various aspects (intensity, size, and timing) of simulated cells have been shown to improve with adjustments to the mixing length used in the subgrid turbulence scheme, but not all aspects improve simultaneously (Hanley et al., 2015).
There are trends and/or inter-annual variability of the bias in BARRA against analyses of temperature and precipitation observations, and some of these trends are also apparent in the global reanalyses. BARRA-C has similar bias variability as BARRA-R, and its magnitude is similar to or less than the global reanalyses. Spurious trends or artificial shifts in reanalyses could result from abrupt changes to the amount of data assimilated, e.g. at the start and end of satellite missions or the various observational data archives. In BARRA-R, corrections were also made to the observation screening and thinning rules mid-production (Su et al., 2019). However, it is outside the scope of this work to assess the impacts of various observational changes.
BARRA-C shows better agreement with the pattern and the relative distribution of radar-derived rainfall during storms over Sydney. This improvement is due to the use of explicit convection (Sect. 2.1) and a higher-resolution model and is consistent with earlier studies with UM (e.g. Lean et al., 2008). Comparisons of FSS from the same events including ERA5 show that its lower resolution leads to larger representation errors and lower FSS than BARRA-R despite both parameterizing convection (Fig. S6, the Supplement). While BARRA-C still shows considerable bias compared to both rain gauges and radar observations, it adds value to BARRA-R and ERA by providing more realistic and accurate spatial representations of rainfall during storms at various spatial scales and percentile thresholds.
The AV analysis of temperature and precipitation extremes shows that BARRA-C provides some value over BARRA-R in various aspects including the spatial patterns of the warm temperature extremes and wet precipitation extremes as well as the bias in cold extremes over coastal regions. Low AV can be related to temperature and precipitation biases, which differ between the regions. For example, the BARRA-C wet bias relative to AWAP, particularly over the PH domain (Fig. 7b), is responsible for the low AVMSE for rainfall. The positive AVCorr for precipitation in BARRA-SY agrees with the above FSS analysis, which somewhat avoids the wet bias issue through percentile-based thresholding.
Assessing AV for wet extremes may also be problematic with AWAP. As an interpolated dataset, AWAP tends to underestimate the intensity of extreme heavy rainfall observed at stations, and the issue is more pronounced at locations with sparse observational sampling or high topography, particularly in SY and TA (Chubb et al. 2016; King et al., 2012).
While this analysis suggests that limited value is added by the downscaling of BARRA-R for these extremes, the true AV of BARRA-C at its native resolution is not assessed here given the limited resolution of AWAP and can be explored further with the scale-dependent AV analysis of Di Luca et al. (2016). Determining AV at the kilometre scale is also expected to be challenging as more accurate and representative observational datasets are needed.
The recent development of CPMs in NWP has facilitated the creation of kilometre-scale regional reanalysis and climate projections. BARRA is the first regional reanalysis that focuses on the Australasian region. It has been developed with significant co-investment from state-level emergency service agencies across Australia. BARRA-C is the critical component of the project that provides these agencies with the means to develop a deeper understanding of past extreme weather at local scales, especially in areas that were not adequately served by observation networks (e.g. Fig. S3, the Supplement). The four midlatitude domains of BARRA-C are designed to address these needs, and BARRA-R is needed to establish a driving model for BARRA-C and utilize more of the Australian local observations (Su et al., 2019). Completed in June 2019, the 29-year BARRA-R reanalysis (1990 to February 2019) and its downscaled counterpart BARRA-C form a collection of high-resolution gridded meteorological datasets with 12 and 1.5 km horizontal grid lengths and 10 min to hourly time resolution, produced using systems closely related to the Bureau's present (as of October 2020) regional NWP systems. The hybrid model-level and pressure-level gridded data from BARRA-C are also available to drive or force sub-kilometre weather or non-weather models.
This paper describes the experimental configuration of BARRA-C and provides a preliminary assessment to illustrate its skills over BARRA-R and the global reanalyses at their subgrid scales. As expected from a hindcast-only system, it inherits the domain-averaged biases from BARRA-R. On the other hand, our added-value analysis shows that BARRA-C simulated different climatological extremes for temperature. Altogether, there is added skill at the local scale for temperature and wind, particularly in topographically complex regions in SY and TA, as well as coastal regions in all domains. As expected, the contrasts in skills and biases are most apparent between BARRA and the coarser-scale reanalyses (ERA-Interim, MERRA-2). BARRA-R and BARRA-C produce more distinctive precipitation estimates for intensity, sub-daily timing, and hourly spatial patterns that are characteristics of their physical schemes. BARRA-C also provides a different spatial distribution of precipitation over complex terrains and more skilful representations of sub-daily rainfall fields. The latter suggests that BARRA-C is more suited for studies of extreme rainfall events, although it still has a high rainfall bias. The high rainfall bias also manifests in the climatological extremes of precipitation. These findings highlight the fact that improvements are still needed for future kilometre-scale downscaled reanalysis, e.g. adding kilometre-scale data assimilation and further model development. At this stage, BARRA-R and BARRA-C can be used conjunctively to improve individual estimates of temperature and precipitation. Some of their biases, including for 10 m wind, could also be addressed via post-processing using multivariate regression models or quantile matching methods such as those of Glahn and Lowry (1972) and Cattoën et al. (2020). Users of BARRA are strongly encouraged to undertake a local evaluation to ascertain the skills of BARRA-C for their regions and parameters of interest.
BARRA lays some of the important groundwork for future reanalysis-related activities and developing national climate risk services at the Bureau. Some of the issues identified in this work are being actively researched by collaborating national meteorological centres and academic institutions within the “regional atmosphere” configuration development framework (Bush et al., 2020). Future reanalyses will also benefit from the recent advances in the Bureau's NWP, with an assimilation system (Rennie et al., 2020) and ensemble introduced in its upcoming kilometre-scale models to allow propagation of high-resolution information between hindcast cycles and estimation of uncertainties.
All code, including the UM (version 10.6) and JULES (version 4.7), used to produced BARRA-C is version-controlled under the Met Office Science Repository Service. The UM is available for use under license at http://www.metoffice.gov.uk/research/modelling-systems/unified-model (last access: 31 August 2020). JULES is available under licence free of charge at http://jules-lsm.github.io/access_req/JULES_access.html (last access: 31 August 2020). The infrastructure for building and running UM–JULES simulations uses the Rose suite engine (https://metomi.github.io/rose/doc/html/index.html, last access: 31 August 2020) and scheduling using the Cylc workflow engine (https://cylc.github.io/ (last access: 31 August 2020), Oliver et al., 2019). Both Rose and Cylc are available under Version 3 of the GNU General Public License. The BARRA-C Rose/Cylc suite, with an identifier u-ak499, is version-controlled under the Met Office Science Repository Service and contains the UM–JULES science namelist and simulation configurations. Output from the model simulations was converted from UM fieldsfile format to NetCDF4 format using Iris (https://scitools-iris.readthedocs.io/en/stable/, last access: 31 August 2020).
The BARRA datasets for the period of January 1990 to February 2019 are available for academic use. Readers are referred to http://www.bom.gov.au/research/projects/reanalysis (last access: 31 August 2020; Bureau of Meteorology, 2020) for information on available parameters, access, and licensing. The BARRA-R datasets used to initialize and constrain BARRA-C at the boundaries and the BARRA-C ancillary files can be requested by contacting the authors directly and are subject to the same licensing conditions.
The supplement related to this article is available online at: https://doi.org/10.5194/gmd-14-4357-2021-supplement.
PS, DJ, PFH, and CJW conceived and/or designed BARRA. NE, CHS, and PS developed the BARRA-C system with inputs from CF. NE performed the production, and CHS and NE performed the evaluation. CHS and NE prepared the paper with contributions from all co-authors.
The authors declare that they have no conflict of interest.
Publisher's note: Copernicus Publications remains neutral with regard to jurisdictional claims in published maps and institutional affiliations.
Funding for this work was provided by emergency service agencies (New South Wales Rural Fire Service, Western Australia Department of Fire and Emergency Services, South Australia Country Fire Service, South Australia Department of Environment, Water and National Resources) and research institutions (Antarctic Climate and Ecosystems Cooperative Research Centre – ACE CRC – and the University of Tasmania). Funding from Tasmania is supported by the Tasmanian Government and the Australian Government, provided under the Tasmanian Bushfire Mitigation Grants Program.
BARRA-C is set up with assistance from the UKMO colleagues (Stuart Webster) and many colleagues at the Bureau of Meteorology (Greg Kociuba, Gary Dietachmayer, Hongyan Zhu, Yimin Ma, Ilia Bermous, Robin Bowen), the Commonwealth Scientific and Industrial Research Organisation (CSIRO; Martin Dix), and National Computational Infrastructure (NCI; Dale Roberts, Grant Ward). The FSS analysis with the Rainfields2 product is undertaken with assistance from Susan Rennie, Kevin Cheong, Alan Seed (Bureau of Meteorology), and Suwash Acharya (University of Melbourne). We also thank Mitchell Black and Vinodkumar for their feedback on drafts of the paper. The BARRA project was undertaken with resources and services from NCI, which is supported by the Australian Government. This study uses the ERA-Interim and ERA5 data provided through the ARC Centre of Excellence for Climate System Science (Paola Petrelli) at NCI.
ERA-Interim can be retrieved from the ECMWF at https://www.ecmwf.int/en/forecasts/datasets/archive-datasets/reanalysis-datasets/era-interim (last access: 31 August 2020). ERA5 can be retrieved from the Copernicus Climate Data Store at https://cds.climate.copernicus.eu/ (last access: 31 August 2020). The AWAP data can be requested from http://www.bom.gov.au/climate (last access: 31 August 2020). The Rainfields2 radar product is retrieved from the Rainfields Archiving System provided by the Bureau of Meteorology.
This paper was edited by Steven Phipps and reviewed by two anonymous referees.
Acharya, S. C., Nathan, R., Wang, Q. J., Su, C.-H., and Eizenberg, N.: Ability of an Australian reanalysis dataset to characterise sub-daily precipitation, Hydrol. Earth Syst. Sci., 24, 2951–2962, https://doi.org/10.5194/hess-24-2951-2020, 2020.
Arakawa, A. and Lamb, V. R.: Computational design of the basic dynamical processes of the UCLA general circulation model, Methods in Computational Physics: Advances in Research and Applications, 17, 173–265, https://doi.org/10.1016/B978-0-12-460817-7.50009-4, 1977.
Argüeso, D., Evans, J. P., Fita, L., and Bormann, K. J.: Temperature response to future urbanization and climate change, Clim. Dynam., 42, 2183–2199, https://doi.org/10.1007/s00382-013-1789-6, 2014.
Bermejo, R. and Staniforth, A.: The conversion of semi-Lagrangian advection schemes to quasi-monotone schemes, Mon. Weather. Rev., 120, 2622–2632, https://doi.org/10.1175/1520-0493(1992)120<2622:TCOSLA>2.0.CO;2, 1992.
Best, M. J., Pryor, M., Clark, D. B., Rooney, G. G., Essery, R. L. H., Ménard, C. B., Edwards, J. M., Hendry, M. A., Porson, A., Gedney, N., Mercado, L. M., Sitch, S., Blyth, E., Boucher, O., Cox, P. M., Grimmond, C. S. B., and Harding, R. J.: The Joint UK Land Environment Simulator (JULES), model description – Part 1: Energy and water fluxes, Geosci. Model Dev., 4, 677–699, https://doi.org/10.5194/gmd-4-677-2011, 2011.
Borsche, M., Kaiser-Weiss, A. K., Undén, P., and Kaspar, F.: Methodologies to characterize uncertainties in regional reanalyses, Adv. Sci. Res., 12, 207–218, https://doi.org/10.5194/asr-12-207-2015, 2015.
Boutle, I. A., Abel, S. J., Hill, P. G., and Morcrette, C. J.: Spatial variability of liquid cloud and rain: observations and microphysical effects, Q. J. Roy. Meteor. Soc., 140, 583–594, https://doi.org/10.1002/qj.2140, 2014a.
Boutle, I. A., Eyre, J. E. J., and Lock, A. P.: Seamless stratocumulus simulation across the turbulent gray zone, Mon. Weather Rev., 142, 1655–1668, https://doi.org/10.1175/MWR-D-13-00229.1, 2014b.
Bromwich, D. H., Wilson, A. B., Bai, L., Moore, G. W. K., and Bauer, P.: A comparison of the regional Arctic System Reanalysis and the global ERA-Interim Reanalysis for the Arctic, Q. J. Roy. Meteor. Soc., 142, 644–658, https://doi.org/10.1002/qj.2527, 2016.
Brousseau, P., Seity, Y., Ricard, D., and Léger, J.: Improvement of the forecast of convective activity from the AROME-France system, Q. J. Roy. Meteor. Soc., 142, 2231–2243, https://doi.org/10.1002/qj.2822, 2016.
Bureau of Meteorology: APS2 upgrade of the ACCESS-R numerical weather prediction system, NOC Operations Bulletin Number 107, available at: http://www.bom.gov.au/australia/charts/bulletins/apob107-external.pdf (last access: 31 August 2020), 2016.
Bureau of Meteorology: APS2 upgrade of the ACCESS-C numerical weather prediction system, NOC Operations Bulletin Number 114, available at: http://www.bom.gov.au/australia/charts/bulletins/BNOC_Operations_Bulletin_114.pdf (last access: 31 August 2020), 2018.
Bureau of Meteorology: Atmospheric high-resolution regional reanalysis for Australia, available at: http://www.bom.gov.au/research/projects/reanalysis (last access: 1 May 2020), 2020.
Bush, M., Allen, T., Bain, C., Boutle, I., Edwards, J., Finnenkoetter, A., Franklin, C., Hanley, K., Lean, H., Lock, A., Manners, J., Mittermaier, M., Morcrette, C., North, R., Petch, J., Short, C., Vosper, S., Walters, D., Webster, S., Weeks, M., Wilkinson, J., Wood, N., and Zerroukat, M.: The first Met Office Unified Model–JULES Regional Atmosphere and Land configuration, RAL1, Geosci. Model Dev., 13, 1999–2029, https://doi.org/10.5194/gmd-13-1999-2020, 2020.
Calmet, I., Mestayer, P. G., van Eijk, A. M. J., and Herlédant, O.: A coastal day summer breeze study, Part 2: High-resolution numerical simulation of sea-breeze local influences, Bound.-Lay. Meteorol., 167, 27–51, https://doi.org/10.1007/s10546-017-0319-1, 2018.
Cattoën, C., Robertson, D. E., Bennett, J. C., Wang, Q. J., and Carey-Smith, T. K.: Calibrating Hourly Precipitation Forecasts with Daily Observations, J. Hydrometeorol., 21, 1655–1673, https://doi.org/10.1175/JHM-D-19-0246.1, 2020.
Champion, A. J. and Hodges, K.: Importance of resolution and model configuration when downscaling extreme precipitation, Tellus A, 66, 23993, https://doi.org/10.3402/tellusa.v66.23993, 2014.
Charney, J. G. and Phillips, N. A.: Numerical integration of the quasi-geostrophic equations for barotropic and simple baroclinic flows, J. Meteorol., 10, 71–99, https://doi.org/10.1175/1520-0469(1953)010<0071:NIOTQG>2.0.CO;2, 1953.
Chubb, T., Manton, M., Siems, S., and Peace, A. D.: Evaluation of the AWAP daily precipitation spatial analysis with an independent gauge network in the Snowy Mountains, Journal of Southern Hemisphere Earth Systems Science, 66, 55–67, https://doi.org/10.22499/3.6601.006, 2016.
Clark, P., Roberts, N., Lean, H., Ballard, S. P., and Charlton-Perez, C.: Convection-permitting models: a step-change in rainfall forecasting, Meteorol. Appl., 23, 165–181, https://doi.org/10.1002/met.1538, 2016.
Davies, T., Cullen, M. J. P., Malcolm, A. J., Mawson, M. H., Staniforth, A., White, A. A., and Wood, N.: A new dynamical core for the Met Office's global and regional modelling of the atmosphere, Q. J. Roy. Meteor. Soc., 131, 1759–1782, https://doi.org/10.1256/qj.04.101, 2005.
Dee, D. P., Uppala, S. M., Simmons, A. J., Berrisford, P., Poli, P., Kobayashi, S., Andrae, U., Balmaseda, M. A., Balsamo, G., Bauer, P., Bechtold, P., Beljaars, A. C. M., van de Berg, L., Bidlot, J., Bormann, N., Delsol. C., Dragani, R., Fuentes, M., Geer, A. J., Haimberger, L., Healy, S. B., Hersbach, H., Holm, E. V., Isaksen, L., Kallberg, P., Kohler, M., Matricardi, M., McNally, A. P., Monge-Sanz, B. M., Morcrette, J. J., Park, B. K., Peubey, C., de Rosnay, P., Tavolato, C., Thepaut, J. N., and Vitart, F.: The Era-Interim reanalysis: Configuration and performance of the data assimilation system, Q. J. Roy. Meteor. Soc., 137, 553–597, https://doi.org/10.1002/qj.828, 2011.
Dharssi, I., Steinle, P., and Fernon, J.: Improved numerical weather predictions by using optimised urban model parameter values and satellite derived tree heights, in: MODSIM2015, 21st International Congress on Modelling and Simulation, edited by: Weber, T., McPhee, M. J., and Anderssen, R. S., Modelling and Simulation Society of Australia and New Zealand, December 2015, 1161–1167, ISBN: 978-0-9872143-5-5, available at: https://www.mssanz.org.au/modsim2015/M4/dharssi.pdf (last access: 31 August 2020), 2015.
Di Luca, A., de Elía, R., and Laprise, R.: Challenges in the quest for added value of regional climate dynamical downscaling, Current Climate Change Reports, 1, 10–21, https://doi.org/10.1007/s40641-015-0003-9, 2015.
Di Luca, A., Argüeso, D., Evans, J. P., de Elía, R., and Laprise, R.: Quantifying the overall added value of dynamical downscaling and the contribution from different spatial scales, J. Geophys. Res.-Atmos., 121, 1575–1590, https://doi.org/10.1002/2015JD024009, 2016.
Dixon, M., Li, Z., Lean, H., Roberts, N., and Ballard, S.: Impact of data assimilation on forecasting convection over the United Kingdom using a high resolution version of the Met Office Unified Model, Mon. Weather Rev., 137, 1562–1584, https://doi.org/10.1175/2008MWR2561.1, 2009.
Done, J., Davis, C. A., and Weisman, M.: The next generation of NWP: explicit forecasts of convection using the weather research and forecasting (WRF) model, Atmos. Sci. Lett., 5, 110–117, https://doi.org/10.1002/asl.72, 2004.
Donlon, C. J., Martin, M., Stark, J. D., Roberts-Jones, J., Fiedler, E., and Wimmer, W.: The Operational Sea Surface Temperature and Sea Ice analysis (OSTIA) system, Remote Sens. Environ., 116, 140–158, https://doi.org/10.1016/j.rse.2010.10.017, 2012.
Ebert, E. E.: Neighborhood verification: A strategy for rewarding close forecasts, Weather Forecast., 24, 1498–1510, https://doi.org/10.1175/2009WAF2222251.1, 2009.
Ebita, A., Kobayashi, S., Ota, Y., Moriya, M., Kumabe, R., Onogi, K., Harada, Y., Yasui, S., Miyaoka, K., Takahashi, K., Kamahori, H., Kobayashi, C., Endo, H., Soma, M., Oikawa, Y., and Ishimizu, T.: The Japanese 55-year reanalysis JRA-55: An interim report, SOLA, 7, 149–152, https://doi.org/10.2151/sola.2011-038, 2011.
Edwards, J. M. and Slingo, A.: Studies with a flexible new radiation code. I: Choosing a configuration for a largescale model, Q. J. Roy. Meteor. Soc., 122, 689–719, https://doi.org/10.1002/qj.49712253107, 1996.
Fosser, G., Khodayar, S., and Berg, P: Benefit of convection permitting climate model simulations in the representation of convective precipitation, Clim. Dynam., 44, 45–60, https://doi.org/10.1007/s00382-014-2242-1, 2015.
Frank, C. W., Pospichal, B., Wahl, S., Keller, J. D., Hence, A., and Crewell, S.: The added value of high resolution regional reanalyses for wind power applications, Renew. Energ., 148, 1094–1109, https://doi.org/10.1016/j.renene.2019.09.138, 2020.
Gelaro, R., McCarty, W., Suárez, M. J., Todling, R., Molod, A., Takacs, L., Randles, C. A., Darmenov, A., Bosilovich, M. G., Reichle, R., Wargan, K., Coy, L., Cullather, R., Draper, C., Akella, S., Buchard, V., Conaty, A., da Silva, A. M., Gu, W., Kim, G., Koster, R., Lucchesi, R., Merkova, D., Nielsen, J. E., Partyka, G., Pawson, S., Putman, W., Rienecker, M., Schubert, S. D., Sienkiewicz, M., and Zhao, B.: The Modern-Era Retrospective Analysis for Research and Applications, Version 2 (MERRA-2), J. Climate, 30, 5419–5454, https://doi.org/10.1175/JCLI-D-16-0758.1, 2017.
Gerard, L., Piriou, J., Brožková, R., Geleyn, J., and Banciu, D.: Cloud and precipitation parameterization in a meso-gamma-scale operational weather prediction model, Mon. Weather Rev., 137, 3960–3977, https://doi.org/10.1175/2009MWR2750.1, 2009.
Glahn, H. R. and Lowry, D. A.: The use of model output statistics (MOS) in objective weather forecasting, J. Appl. Meteorol., 11, 1203–1211, https://doi.org/10.1175/1520-0450(1972)011<1203:TUOMOS>2.0.CO;2, 1972.
Gregory, D. and Rowntree, P. R.: A mass flux convection scheme with representation of cloud ensemble characteristics and stability-dependent closure, Mon. Weather Rev., 118, 1483–1506, https://doi.org/10.1175/1520-0493(1990)118<1483:AMFCSW>2.0.CO;2, 1990.
Gregow, H., Jylhä, K., Mäkelä, H. M., Aalto, J., Manninen, T., Karlsson, P., Kaiser-Weiss, A. K., Kaspar, F., Poli, P., Tan, D. G., Obregon, A., and Su, Z.: Worldwide survey of awareness and needs concerning reanalyses and respondents views on climate services, B. Am. Meteorol. Soc., 97, 1461–1473, https://doi.org/10.1175/BAMS-D-14-00271.1, 2016.
Griffiths, D. J., Colquhoun, J. R., Batt, K. L., and Casinader, T. R.: Severe thunderstorms in New South Wales: Climatology and means of assessing the impact of climate change, Climatic Change, 25, 369–388, https://doi.org/10.1007/BF01098382, 1993.
Halliwell, C., Boutle, I., and Hanley, K.: Subgrid turbulence scheme, Unified Model Documentation Paper 28, Met Office, Exeter, UK, 2007.
Hanley, K. E., Plant, R. S., Stein, T. H. M., Hogan, R. J., Nicol, J. C., Lean, H. W., Halliwell, C. and Clark, P. A.: Mixing-length controls on high-resolution simulations of convective storms, Q. J. Roy. Meteor. Soc., 141, 272-284, https://doi.org/10.1002/qj.2356, 2015.
Hartley, A., MacBean, N., Georgievski, G., and Bontemps, S.: Uncertainty in plant functional type distributions and its impact on land surface models, Remote Sens. Environ., 203, 71–89, https://doi.org/10.1016/j.rse.2017.07.037, 2017.
Hersbach, H., Bell, B., Berrisford, P., Hirahara, S., Horányi, A., Muñoz-Sabater, J., Nicolas, J., Peubey, C., Radu, R., Schepers, D., Simmons, A., Soci, C., Abdalla, S., Abellan, X., Balsamo, G., Bechtold, P., Biavati, G., Bidlot, J., Bonavita, M., Chiara, G. D., Dahlgren, P., Dee, D., Diamantakis, M., Dragani, R., Flemming, J., Forbes, R., Fuentes, M., Geer, A., Haimberger, L., Healy, S., Hogan, R. J., Hólm, E., Janisková, M., Keeley, S., Laloyaux, P., Lopez, P., Lupu, C., Radnoti, G., de Rosnay, P., Rozum, I., Vamborg, F., Villaume, S., and Thépaut, J.-N.: The ERA5 global reanalysis, Q. J. Roy. Meteor. Soc., 146, 1999– 2049, https://doi.org/10.1002/qj.3803, 2020.
Jakob, D., Su, C.-H., Eizenberg, N., Kociuba, G., Steinle, P., Fox-Hughes, P., and Bettio, L.: An atmospheric high-resolution regional reanalysis for Australia, B. Aus. Meteorol. Oceanog. Soc., 30, 16–23, 2017.
Jermey, P. M. and Renshaw, R. J.: Precipitation representation over a two-year period in regional reanalysis, Q. J. Roy. Meteor. Soc., 142, 1300-1310, https://doi.org/10.1002/qj.2733, 2016.
Jones, D. A., Wang, W., and Fawcett, R.: High-quality spatial climate data-sets for Australia, Aust. Meteorol. Ocean., 58, 233–248, 2009.
Kalnay, E., Kanamitsu, M., Kistler, R., Collins, W., Deaven, D., Gandin, L., Iredell, M., Saha, S., White, G., Woollen, J., Zhu, Y., Chelliah, M., Ebisuzaki, W., Higgins, W., Janowiak, J., Mo, K. C., Ropelewski, C., Wang, J., Leetmaa,A., Reynolds, R., Jenne, R., and Joseph, D.: The NCEP/NCAR 40-Year Reanalysis Project, B. Am. Meteorol. Soc., 77, 437–472, https://doi.org/10.1175/1520-0477(1996)077<0437:TNYRP>2.0.CO;2, 1996.
Kendon, E. J., Ban, N., Roberts, N. M., Fowler, H. J., Roberts, M. J., Chan, S. C., Evans, J. P., Fosser, G., and Wilkinson, J. M.: Do convection-permitting regional climate models improve projections of future precipitation change?, B. Am. Meteorol. Soc., 98, 79–93, https://doi.org/10.1175/BAMS-D-15-0004.1, 2017.
Kendon, E. J., Stratton, R. A., Tucker, S., Marsham, J. H., Berthou, S., Rowell, D. P., and Senior, C. A.: Enhanced future changes in wet and dry extremes over Africa at convection-permitting scale, Nat Commun., 10, 1794, https://doi.org/10.1038/s41467-019-09776-9, 2019.
Kendon, E. J., Prein, A. F., and Senior, C. A.: Challenges and outlook for convective-permitting climate modelling, Philos. T. R. Soc. A, 379, 20190547, https://doi.org/10.1098/rsta.2019.0547, 2021.
King, A. D., Alexander, L. V., and Donat, M. G.: The efficacy of using gridded data to examine extreme rainfall characteristics: a case study for Australia, Int. J. Climatol., 33, 2376–2387, https://doi.org/10.1002/joc.3588, 2012.
Kuleshov, Y., de Hoedt, G., Wright, W., and Brewster, A.: Thunderstorm distribution and frequency in Australia, Aust. Meteorol. Mag., 51, 145–154, 2002.
Louis, J.-F.: A parametric model of vertical eddy fluxes in the atmosphere, Bound.-Lay. Meteorol., 17, 187–202, 1979.
Lean, H. W., Clark, P. A., Dixon, M., Roberts, N. M., Fitch, A., Forbes, R., Halliwell, C.: Characteristics of high-resolution versions of the Met Office Unified Model for forecasting convection over the United Kingdom, Mon. Weather Rev., 136, 3408–3424, https://doi.org/10.1175/2008MWR2332.1, 2008.
Leutwyler, D., Lüthi, D., Ban, N., Fuhrer, O., and Schär, C.: Evaluation of the convection-resolving climate modeling approach on continental scales, J. Geophys. Res.-Atmos., 122, 5237–5258, https://doi.org/10.1002/2016JD026013, 2017.
Lock, A. P., Brown, A. R., Bush, M. R., Martin, G. M., and Smith, R. N. B.: A new boundary layer mixing scheme. Part I: Scheme description and single-column model tests, Mon. Weather Rev., 128, 3187–3199, https://doi.org/10.1175/1520-0493(2000)128<3187:ANBLMS>2.0.CO;2, 2000.
Lock, A., Edwards, J., and Boutle, I.: The parametrization of boundary layer processes, Unified Model Documentation Paper 024, vn10.6, 2016.
Lopez, M. A., Hartmann, D. L., Blossey, P. N., Wood, R., Bretherton, C. S., and Kubar, T. L.: A test of the simulation of tropical convective cloudiness by a cloud-resolving model, J. Climate, 22, 2834–2849, https://doi.org/10.1175/2008JCLI2272.1, 2009.
Loveland, T. R., Reed, B. C., Brown, J. F., Ohlen, D. O., Zhu, Z., Yang, L., and Merchant, J. W.: Development of a global land cover characteristics database and IGBP DISCover from 1 km AVHRR data, Int. J. Remote Sens., 21, 1303–1330, https://doi.org/10.1080/014311600210191, 2000.
Ma, Y. and Liu, H.: Large-eddy simulations of atmospheric flows over complex terrain using the immersed-boundary method in the Weather Research and Forecasting model, Bound.-Lay. Meteorol., 165, 421–445, https://doi.org/10.1007/s10546-017-0283-9, 2017.
Mahmood, S., Davie, J., Jermey, P., Renshaw, R., George, J. P., Rajagopal, E. N., and Rani, S. I.: Indian monsoon data assimilation and analysis regional reanalysis: Configuration and performance, Atmos. Sci. Lett., 19, e808, https://doi.org/10.1002/asl.808, 2018.
Mailhot, J., Bélair, S., Charron, M., Doyle, C., Joe, P., Abrahamowicz, M., Bernier, N. B., Denis, B., Erfani, A., Frenette, R., Giguére, A., Issac, G. A., McLennan, N., McTaggart-Cowan, R., Milbrandt, J., and Tong, L.: Environment Canada's experimental numerical weather prediction systems for the Vancouver 2010 Winter Olympic and Paralympic Games, B. Am. Meteorol. Soc., 91, 1073–1086, https://doi.org/10.1175/2010BAMS2913.1, 2010.
Mesinger, F., DiMego, G., Kalnay, E., Mitchell, K., Shafran, P. C., Ebisuzaki, W., Jović, D., Woollen, J., Rogers, E., Berbery, E. H., Ek, M. B., Fan, Y., Grumbine, R., Higgins, W., Li, H., Lin, Y., Manikin, G., Parrish, D., and Shi, W.: North American Regional Reanalysis, B. Am. Meteorol. Soc., 87, 343–360, https://doi.org/10.1175/BAMS-87-3-343, 2006.
Mueller, N., Lewis, A., Roberts, D., Ring, S., Melrose, R., Sixsmith, J., Lymburner, L., McIntyre, A., Tan, P., Curnow, S., and Ip, A.: Water observations from space: Mapping surface water from 25 years of Landsat imagery across Australia, Remote Sens. Environ., 174, 341–352, https://doi.org/10.1016/j.rse.2015.11.003, 2016.
Oliver, H., Shin, M., Matthews, D., Sanders, O., Bartholomew, S., Clark, A., Fitzpatrick, B., van Haren, R., Hut, R., and Drost, N.: Workflow automation for cycling systems, Comput. Sci. Eng., 21, 7–21, https://doi.org/10.1109/MCSE.2019.2906593, 2019.
Peel, M. C., Finlayson, B. L., and McMahon, T. A.: Updated world map of the Köppen–Geiger climate classification, Hydrol. Earth Syst. Sci., 11, 1633–1644, https://doi.org/10.5194/hess-11-1633-2007, 2007.
Prein, A. F., Langhans, W., Fosser, G., Ferrone, A., Ban, N., Goergen, K., Keller, M., Tölle, M., Gutjahr, O., Feser, F., Brisson, E., Kollet, S., Schmidli, J., Van Lipzig, N. P. M, and Leung, R.: A review on regional convection-permitting climate modeling: Demonstrations, prospects, and challenges, Rev. Geophys., 53, 323–361, https://doi.org/10.1002/2014RG000475, 2015.
Puri, K., Dietachmayer, G., Steinle, P., Dix, M., Rikus, L., Logan,L., Naughton, M., Tingwell, C., Xiao, Y., Barras, V., Bermous, I., Bowen, R., Deschamps, L., Franklin, C., Fraser, J., Glowacki, T., Harris, B., Lee, J., Le, T., Roff, G., Sulaiman, A., Sims, H., Sun, X., Sun, Z., Zhu, H., Chattopadhyay, M. and Engel, C.: Implementation of the initial ACCESS numerical weather prediction system, Aust. Meteorol. Ocean., 63, 265–284, 2013.
Rennie, S., Rikus, L., Eizenberg, N., Steinle, P., and Krysta, M.: Impact of Doppler radar wind observations on Australian high-resolution numerical weather prediction, Weather Forecast., 35, 309–324, https://doi.org/10.1175/WAF-D-19-0100.1, 2020.
Roberts, N. M. and Lean, H. W.: Scale-selective verification of rainfall accumulations from high-resolution forecasts of convective events, Mon. Weather Rev., 136, 78–97, https://doi.org/10.1175/2007MWR2123.1, 2007.
Roberts-Jones, J., Fiedler, E. K., and Martin, M. J.: Daily, global, high-resolution SST and sea ice reanalysis for 1985–2007 Using the OSTIA system, J. Climate, 25, 6215–6232, https://doi.org/10.1175/JCLI-D-11-00648.1, 2012.
Schär, C., Fuhrer, O., Arteaga, A., Ban, N., Charpilloz, C., Di Girolamo, S., Hentgen, L., Hoefler, T., Lapillonne, X., Leutwyler, D., Osterried, K., Panosetti, D., Rüdisühli, S., Schlemmer, L., Schulthess, T. C., Sprenger, M., Ubbiali, S., and Wernli, H.: Kilometer-scale climate models: Prospects and challenges, B. Am. Meteorol. Soc., 101, E567–E587, https://doi.org/10.1175/BAMS-D-18-0167.1, 2020.
Seed, A., Duthie, E., and Chumchean, S.: Rainfields: The Australian Bureau of Meteorology system for quantitative precipitation estimation, Abstract P6B.8, Proceedings of the 33rd AMS Conference on Radar Meteorology, Cairns, Australia, 6–10 August 2007.
Simard, M., Pinto, N., Fisher, J. B., and Baccini, A.: Mapping forest canopy height globally with spaceborne lidar, J. Geophys. Res.-Biogeo., 116, G04021, https://doi.org/10.1029/2011JG001708, 2011.
Sinclair, S. and Pegram, G.: Combining radar and rain gauge rainfall estimates using conditional merging, Atmos. Sci. Lett., 6, 19–22, https://doi.org/10.1002/asl.85, 2005.
Smagorinsky, J.: General circulation experiments with the primitive equations. I: The basic experiment, Mon. Weather Rev., 91, 99–164, 1963.
Smith, R. N. B.: A scheme for predicting layer cloud and their water content in a general circulation model, Q. J. Roy. Meteor. Soc., 116, 435–460, https://doi.org/10.1002/qj.49711649210, 1990.
Steeneveld, G.-J.: Current challenges in understanding and forecasting stable boundary layers over land and ice, Front. Environ. Sci., 2, 41, https://doi.org/10.3389/fenvs.2014.00041, 2014.
Stein, T. H. M., Hogan, R. J., Clark, P. A., Halliwell, C. E., Hanley, K. E., Lean, H. W., Nicol, J. C., and Plant, R. S.: The DYMECS Project: A statistical approach for the evaluation of convective storms in high-resolution NWP models, B. Am. Meteorol. Soc., 96, 939–951, https://doi.org/10.1175/BAMS-D-13-00279.1, 2015.
Su, C.-H., Eizenberg, N., Steinle, P., Jakob, D., Fox-Hughes, P., White, C. J., Rennie, S., Franklin, C., Dharssi, I., and Zhu, H.: BARRA v1.0: the Bureau of Meteorology Atmospheric high-resolution Regional Reanalysis for Australia, Geosci. Model Dev., 12, 2049–2068, https://doi.org/10.5194/gmd-12-2049-2019, 2019.
Vitolo, C., Napoli, C. D., Giuseppe, F. D., Cloke, H. L., and Pappenberger, F.: Mapping combined wildfire and heat stress hazards to improve evidence-based decision making, Environ. Internat., 127, 21–34, https://doi.org/10.1016/j.envint.2019.03.008, 2019.
Wahl, S., Bollmeyer, C., Crewell, S., Figura, C., Friederichs, P., Hense, A., Keller, J. D., and Ohlwein, C.: A novel convective-scale regional reanalysis COSMO-REA2: Improving the representation of precipitation, Meteorol. Z., 26, 345–361, https://doi.org/10.1127/metz/2017/0824, 2017.
Walsh, K. J. E, White, C. J., McInnes, K. L, Holmes, J., Schuster, S., Richter, H., Evans, J. P., Di Luca, A. and Warren, R. A.: Natural hazards in Australia: storms, wind and hail, Climatic Change, 139, 55–67, https://doi.org/10.1007/s10584-016-1737-7, 2016.
Walters, D., Boutle, I., Brooks, M., Melvin, T., Stratton, R., Vosper, S., Wells, H., Williams, K., Wood, N., Allen, T., Bushell, A., Copsey, D., Earnshaw, P., Edwards, J., Gross, M., Hardiman, S., Harris, C., Heming, J., Klingaman, N., Levine, R., Manners, J., Martin, G., Milton, S., Mittermaier, M., Morcrette, C., Riddick, T., Roberts, M., Sanchez, C., Selwood, P., Stirling, A., Smith, C., Suri, D., Tennant, W., Vidale, P. L., Wilkinson, J., Willett, M., Woolnough, S., and Xavier, P.: The Met Office Unified Model Global Atmosphere 6.0/6.1 and JULES Global Land 6.0/6.1 configurations, Geosci. Model Dev., 10, 1487–1520, https://doi.org/10.5194/gmd-10-1487-2017, 2017.
Walters, D., Baran, A. J., Boutle, I., Brooks, M., Earnshaw, P., Edwards, J., Furtado, K., Hill, P., Lock, A., Manners, J., Morcrette, C., Mulcahy, J., Sanchez, C., Smith, C., Stratton, R., Tennant, W., Tomassini, L., Van Weverberg, K., Vosper, S., Willett, M., Browse, J., Bushell, A., Carslaw, K., Dalvi, M., Essery, R., Gedney, N., Hardiman, S., Johnson, B., Johnson, C., Jones, A., Jones, C., Mann, G., Milton, S., Rumbold, H., Sellar, A., Ujiie, M., Whitall, M., Williams, K., and Zerroukat, M.: The Met Office Unified Model Global Atmosphere 7.0/7.1 and JULES Global Land 7.0 configurations, Geosci. Model Dev., 12, 1909–1963, https://doi.org/10.5194/gmd-12-1909-2019, 2019.
Wilkinson, J. M. and Jorge Bornemann, F.: A lightning forecast for the London 2012 Olympics opening ceremony, Weather, 69, 16–19, https://doi.org/10.1002/wea.2176, 2014.
Wilson, D. R. and Ballard, S. P.: A microphysically based precipitation scheme for the UK Meteorological Office Unified Model, Q. J. Roy. Meteor. Soc., 125, 1607–1636, https://doi.org/10.1002/qj.49712555707, 1999.
Wood, N., Staniforth, A., White, A., Allen, T., Diamantakis, M., Gross, M., Melvin, T., Smith, C., Vosper, S., Zerroukat, M., and Thuburn, J.: An inherently mass-conserving semi-implicit semi-Lagrangian discretization of the deep-atmosphere global nonhydrostatic equations, Q. J. Roy. Meteor. Soc., 140, 1505–1520, https://doi.org/10.1002/qj.2235, 2014.
Zerroukat, M. and Shipway, B. J.: ZLF (Zero Lateral Flux): a simple mass conservation method for semi-Lagrangian-based limited-area models, Q. J. Roy. Meteor. Soc, 143, 2578–2584, https://doi.org/10.1002/qj.3108, 2017.