the Creative Commons Attribution 4.0 License.
the Creative Commons Attribution 4.0 License.
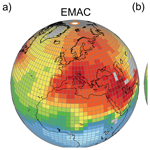
Are contributions of emissions to ozone a matter of scale? – a study using MECO(n) (MESSy v2.50)
Astrid Kerkweg
Volker Grewe
Patrick Jöckel
Robert Sausen
Anthropogenic and natural emissions influence the tropospheric ozone budget, thereby affecting air quality and climate. To study the influence of different emission sources on the ozone budget, often source apportionment studies with a tagged tracer approach are performed. Studies investigating air quality issues usually rely on regional models with a fine spatial resolution, while studies focusing on climate-related questions often use coarsely resolved global models. It is well known that simulated ozone mixing ratios depend on the resolution of the model and the resolution of the emission inventory. Whether the contributions simulated using source apportionment approaches also depend on the model resolution, however, is still unclear. Therefore, this study attempts for the first time to analyse the impact of the model, the model resolution, and the emission inventory resolution on simulated ozone contributions using a diagnostic tagging method. The differences in the ozone contributions caused by these factors are compared with differences that arise from the usage of different emission inventories. To do so, we apply the MECO(n) (MESSy-fied ECHAM and COSMO models nested n times) model system which couples online a global chemistry-climate model with a regional chemistry-climate model equipped with a tagging scheme for source apportionment. The results of the global model (at 300 km horizontal resolution) are compared with the results of the regional model at 50 km (Europe) and 12 km (Germany) resolutions. Besides model-specific differences and biases that are discussed in detail, our results have important implications for other modelling studies and modellers applying source apportionment methods. First, contributions from anthropogenic emissions averaged over the continental scale are quite robust with respect to the model, model resolution, and emission inventory resolution. Second, differences on the regional scale caused by different models and model resolutions can be quite large, and regional models are indispensable for source apportionment studies on the subcontinental scale. Third, contributions from stratospheric ozone transported to the surface differ strongly between the models, mainly caused by differences in the efficiency of the vertical mixing. As stratospheric ozone plays an important role for ground level ozone, but the models show large differences in the amount of downward transported ozone, source apportionment methods should account for this source explicitly to better understand inter-model differences.
- Article
(7993 KB) - Full-text XML
-
Supplement
(32938 KB) - BibTeX
- EndNote
Emissions from land transport, industry, and shipping contribute largely to global budgets of trace gases like NOx and O3, thereby impacting air quality and climate (e.g. Eyring et al., 2007; Matthes et al., 2007; Hoor et al., 2009; Fiore et al., 2012; Young et al., 2013; Hendricks et al., 2017; and Mertens et al., 2018). To quantify the impacts of these emissions, typically source–receptor relationships are calculated using perturbation or source apportionment methods (e.g. Dunker et al., 2002; Emmons et al., 2012; Stock et al., 2013; Matthias et al., 2016; Huang et al., 2017; Clappier et al., 2017; and Butler et al., 2018). Many studies quantifying the influence of anthropogenic and natural emission sources (e.g. land transport emissions or lightning) on the ozone budget exist, but the uncertainties of such analyses are large. There exist three main sources of uncertainty: (1) the emission inventories, (2) the model biases/errors, and (3) the resolutions of the models and/or emission inventories. The influences of the first two factors, emission inventories and model biases, have been investigated by multi-scenario and/or multi-model analyses (e.g. Eyring et al., 2007; Hoor et al., 2009; and Fiore et al., 2009). Even though the influence of the model and emission inventory resolutions on simulated ozone mixing ratios is well known (e.g. Wild and Prather, 2006; Wild, 2007; Tie et al., 2010; Holmes et al., 2014; and Markakis et al., 2015), the impact of the third factor – the model and emission inventory resolutions – on the simulated contributions of specific emission sources to ozone has not yet been systematically investigated in detail. It is important to investigate this third factor, as source apportionment studies focusing on climate usually use rather coarsely resolved global climate models (e.g. Wang et al., 1998; Lelieveld and Dentener, 2000; Grewe, 2006; Matthes et al., 2007; Dahlmann et al., 2011; and Emmons et al., 2012), while air-quality-related studies use more finely resolved regional models (e.g. Dunker et al., 2002; Li et al., 2012; Kwok et al., 2015; Valverde et al., 2016; and Karamchandani et al., 2017). Therefore it is unclear if the results from global and regional models are comparable and how large potential errors, caused by the coarse resolution of global models, are. The present study is a first attempt to investigate the influences of the model and the emission inventory resolutions on the ozone contributions. More precisely, we investigate the influences of four different modelling aspects on source apportionment results for ozone; these aspects are as follows:
-
the applied model,
-
the resolution of the model,
-
the resolution of the emission inventory, and
-
the emission inventory.
We apply the MECO(n) (MESSy-fied ECHAM and COSMO models nested n times; e.g. Kerkweg and Jöckel, 2012b; Mertens et al., 2016) model system together with a detailed source apportionment method (tagging; Grewe et al., 2017). This model system couples, during runtime, the global chemistry-climate model EMAC (ECHAM5/MESSy for Atmospheric Chemistry; Jöckel et al., 2006, 2010) with the regional chemistry-climate model COSMO-CLM/MESSy (Kerkweg and Jöckel, 2012a), which consists of the COSMO-CLM model equipped with the MESSy (Modular Earth Submodel System; Jöckel et al., 2005, 2010) infrastructure. Due to the MESSy infrastructure, we apply identical submodels for calculating the chemical processes and the same source apportionment method in the global and regional model instances. In addition, the global model instance provides consistent boundary conditions for the source apportionment to the regional model instances, allowing a detailed intercomparison of the source apportionment results on different scales. Therefore, this model system is, to our knowledge, the first available model system allowing a seamless contribution analysis from the global to the regional scale. With this model chain we can directly compare the results at the regional and global scale, which allows us to estimate uncertainties of the contribution analyses caused by the model, the model resolution, and emission inventory resolution.
This paper is organised as follows. First, Sect. 2 gives an overview of the model system and discusses the investigation strategy and the performed simulations. In Sect. 3 we present a brief evaluation of the model results compared against ground-level and ozone sonde observations as well as a comparison of the ozone production rates simulated by EMAC and COSMO-CLM/MESSy (Sect. 3.1). In Sect. 4 the differences of the ozone contributions caused by differences of model and emission inventory resolutions are analysed in detail. We provide a more detailed quantification of the differences in specific regions and a further discussion in Sect. 5.
We apply the MECO(n) model system, which couples the global chemistry-climate model EMAC during runtime (i.e. online) with the regional chemistry-climate model COSMO-CLM/MESSy (Kerkweg and Jöckel, 2012b). Both models, EMAC and COSMO-CLM/MESSy, calculate the physical and chemical processes in the atmosphere and their interactions with oceans, land, and human influences. They use the second version of MESSy to link multi-institutional computer codes (Jöckel et al., 2010). The core atmospheric model for EMAC is the fifth-generation European Centre for Medium-Range Weather Forecasts, Hamburg (ECHAM5), general circulation model (Roeckner et al., 2006). The core atmospheric model used in COSMO-CLM/MESSy is the COSMO-CLM model (Rockel et al., 2008), a regional atmospheric climate model that is based on the COSMO (Consortium for Small-scale Modelling) model and jointly further developed by the CLM-Community. In the model system's acronym, MECO(n), “n” denotes the number of COSMO-CLM/MESSy instances nested into the global model framework. The initial and boundary conditions, which are required for each of these nested regional model instances, are provided by the next coarser model instance. This model instance can either be EMAC or COSMO-CLM/MESSy. Due to the online coupling, the boundary conditions for the regional model instances can be provided at every time step of the driving model instance. This is especially important for resolving short-term variations in chemically active species. As EMAC and COSMO-CLM/MESSy calculate both atmospheric dynamics and composition, the meteorological and chemical boundary conditions are as consistent as possible. In addition, the same chemical solver and kinetic mechanism are applied, leading to highly consistent chemical boundary conditions. Therefore, there is no need for lumping (i.e. treating different chemical species with similar chemical formulas as one species), scaling of boundary conditions for specific chemical species, or taking boundary conditions from different models.
More details about the MECO(n) model system are presented in a set of publications including a chemical and meteorological evaluation (Kerkweg and Jöckel, 2012a, b; Hofmann et al., 2012; Mertens et al., 2016; and Kerkweg et al., 2018). The set-up of the simulation applied in the present study is very similar to that described by Mertens et al. (2016). Therefore, we only present the most important details of the model set-up. The complete namelist set-up is part of the Supplement.
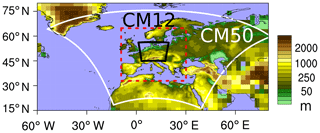
Figure 1Domains of CM50 (white line) and CM12 (black line). Depicted is the topography of the continents (in metres) at the resolution of the corresponding model instance. Outside the CM50 domain the topography of EMAC is displayed. Shown is the entire computational domain including the relaxation area. The dashed red square indicates the region analysed in Sect. 4. The figure is reproduced from Mertens (2017).
A MECO(2) set-up with one COSMO-CLM/MESSy instance over Europe with a resolution of (≈ 50 km) and one instance covering Germany, with a resolution of (≈ 12 km), was applied (see Fig. 1 for the computational domains). For simplicity, we name these two model instances hereafter CM50 and CM12. EMAC, CM50, and CM12 are running simultaneously in the same way as in externally coupled earth system models, with the different earth compartment models running in parallel (see Fig. 2 in Mertens et al. (2016) for the details of the data exchange between the nested model instances). Both COSMO-CLM/MESSy instances use 40 vertical model levels (terrain following) with geometric height as the vertical coordinate. The height of the uppermost model level is at ≈22 km; the damping zone starts at 11 km. The thickness of the lowest model layer is ≈ 20 m. The boundary conditions for CM50 are provided by EMAC, which is operated in the T42L31ECMWF resolution, i.e. with a spherical truncation of T42 (corresponding to a quadratic Gaussian grid of approx. in latitude and longitude) and 31 hybrid pressure levels in the vertical direction up to 10 hPa (corresponding to ≈ 30 km over Europe). The thickness of the lowest model layer corresponds to ≈ 60 m over Europe. The boundary conditions for CM12 are provided by CM50. The applied MESSy version is a modified version of MESSy 2.50, including ECHAM 5.3.02 and COSMO 5.00. All changes are included in MESSy 2.51. To facilitate a one-to-one comparison with observations, EMAC is “nudged” by a Newtonian relaxation of the temperature, the divergence, the vorticity, and the logarithm of surface pressure (Jöckel et al., 2006) towards ERA-Interim (Dee et al., 2011) reanalysis data for the years 2007 to 2010. Sea surface temperature and sea ice coverage are prescribed as boundary conditions for the simulation set-up from ERA-Interim as well.
Due to the MESSy infrastructure, the same diagnostics or chemical process descriptions are applied in all of the model instances. Following the modular structure of MESSy, each diagnostic or process description is coded as a so-called submodel. The applied submodels are listed in Table 1. Besides the name of the submodel and their reference, a short description provides general information on the process or diagnostic represented by the respective submodel. Most importantly, the same kinetic solver (MECCA; Sander et al., 2011) and same TAGGING submodel (Grewe et al., 2017) are applied in each instance.
Dietmüller et al. (2016)Pozzer et al. (2006)Roeckner et al. (2006)Jöckel et al. (2006)Dietmüller et al. (2016)Tost et al. (2006b)Tost et al. (2010)Kerkweg et al. (2006a)Kerkweg and Jöckel (2012b)Roeckner et al. (2003)Landgraf and Crutzen (1998)Jöckel et al. (2006)Tost et al. (2007)Jöckel et al. (2010)Sander et al. (2011)Jöckel et al. (2010)Kerkweg and Jöckel (2012b)Kerkweg et al. (2018)Jöckel et al. (2010)Kerkweg et al. (2006b)Kerkweg et al. (2006b)Dietmüller et al. (2016)Giorgetta and Bengtsson (1999)Jöckel et al. (2006)Dietmüller et al. (2016)Tost et al. (2006a)Kerkweg et al. (2006a)Jöckel et al. (2010)Jöckel et al. (2016)Grewe et al. (2017)Kerkweg et al. (2006b)Jöckel et al. (2006)Table 1Overview of the submodels applied in EMAC and COSMO-CLM/MESSy, respectively. Both COSMO-CLM/MESSy instances use the same set of submodels. MMD* comprises the MMD2WAY submodel and the MMD library.
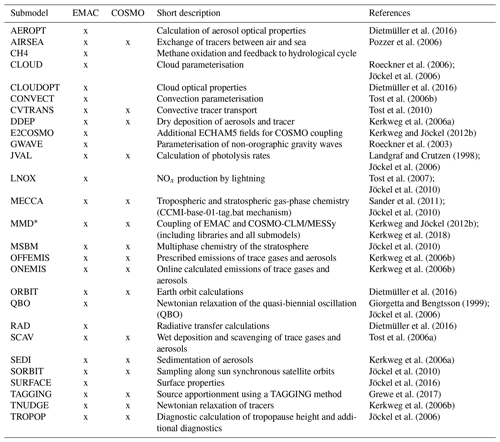
The chemical mechanism used by MECCA considers the chemistry of ozone, methane, and odd nitrogen. While alkynes and aromatics are not considered, alkenes and alkanes are considered up to C4. We use the Mainz Isoprene Mechanism (MIM1; Pöschl et al., 2000) to model the chemistry of isoprene and some non-methane hydrocarbons (NMHCs). The mechanisms of MECCA and of the submodel calculating the scavenging of trace gases by clouds and precipitation (SCAV; Tost et al., 2006a, 2010) are part of the Supplement. The TAGGING submodel calculates the contributions of different emission sources to ozone and the relevant precursors. More details of this tagging approach are given in Sect. 2.1.
The lightning-produced NOx emissions are only calculated in EMAC, using a parameterisation based on Price and Rind (1992) that is scaled to a global nitrogen oxide emission rate of ≈ 5 Tg N a−1 from lightning flashes. In CM50 and CM12 we use the emissions from EMAC (i.e. with same geographical, vertical, and temporal distribution), which are transformed online onto the grids for CM50 and CM12, respectively. This approach was chosen as the calculation of lightning-produced NOx is strongly coupled to the convection parameterisation (e.g. Tost et al., 2007). In different models and/or at different model resolutions convection occurs at different places and/or times and lightning emissions can differ largely. Our approach was chosen to allow for an easier comparison between the results of different model instances.
The calculation of emissions from soil NOx and biogenic isoprene (C5H8) is performed by the MESSy submodel ONEMIS (described as ONLEM by Kerkweg et al., 2006b). Following the parameterisations of Yienger and Levy (1995) and Guenther et al. (1995), the respective emissions depend on the meteorological conditions. In contrast to the lightning NOx emissions, the soil NOx and biogenic emissions are calculated separately by EMAC and CM50. This leads to differences in the soil NOx and C5H8 emissions (see Fig. S17 in the Supplement), influencing the calculation of the contributions. We have chosen this approach because the land–sea masks differ between models and model resolutions. If the emissions calculated by EMAC are used in the COSMO-CLM/MESSy model instances, some of the emissions would occur over the sea (or vice versa). This could lead to artificial errors in the contribution analyses. In EMAC, the isoprene emissions calculated by ONEMIS are scaled by a factor of 0.6 (following Jöckel et al., 2006) and in COSMO-CLM/MESSy by a factor of 0.45 (following Mertens et al., 2016).
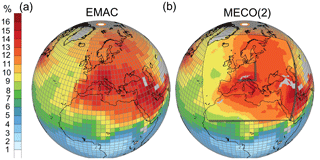
Figure 2Relative contribution (in percent) of land transport emissions to the ozone column up to 850 hPa, averaged over July 2008. (a) The values calculated by the EMAC model and (b) the values calculated by MECO(2) with the two refinements covering Europe and Germany.
2.1 Tagging for source apportionment
We apply the TAGGING submodel described by Grewe et al. (2017) for source apportionment. The tagging method is a diagnostic method; i.e. the atmospheric chemistry calculations are not influenced. To minimise the computational resources (e.g. computing time and memory), the tagging is not performed for the detailed chemistry from MECCA, but for a simplified family concept. The species which are tagged in this family concept are ozone (as odd oxygen family), the NOy family, the NMHC family, CO, PAN, OH, and HO2 (OH and HO2 in a steady-state approach). The tagging method itself is based on the combinatorial ansatz described by Grewe (2013). In the tagging concept the mixing ratios of the considered chemical species and families are fully decomposed into N unique categories, meaning that the sum of mixing ratios over all considered categories is equal the total mixing ratio of the considered species (i.e. the budget is closed),
As an example of the generalised tagging method we consider the production of ozone from the reaction of NO with an organic peroxy radical (RO2) which yields NO2 and an organic oxy radical (RO),
According to Grewe et al. (2017) (Eqs. 13 and 14 therein) this leads to the following fractional apportionment:
PR1 is the production rate of O3 by reaction (R1). NOy and NMHC are the mixing ratios of the corresponding tagged families, while species marked with “tag” represent quantities tagged for a specific category (e.g. stratosphere or land transport). The denominator represents the sum of the mixing ratios over all categories of the respective tagged family/species. Accordingly, the tagging scheme takes into account the specific reaction rates from the full chemistry scheme. Further, the fractional apportionment is inherent to the applied tagging method, as due to the combinatorial ansatz every regarded chemical reaction is decomposed into all possible combinations of reacting tagged species.
The TAGGING submodel is applied in each model instance. At the lateral and top boundaries of CM50 and CM12 the tagged contributions are treated in the same manner as all chemical species; i.e. the mixing ratios of the tagged species of the finer model instance (i.e. the absolute contributions) are relaxed towards the mixing ratios of the tagged species provided by the driving model instance. This is depicted in Fig. 2, showing the relative contribution of the land transport emissions to ozone. EMAC calculates the contributions globally with a rather coarse resolution. With MECO(2) (Fig. 2b) the resolution over Europe and Germany is increased using the two COSMO-CLM/MESSy refinements. As the source apportionment is performed in EMAC, CM50, and CM12 – with the respective boundary conditions provided by the next coarser model instance – this approach allows for a consistent zooming into the area of interest within the global framework. In contrast to our approach, other tagging methods which are usually applied in regional chemistry-climate or chemistry-transport models feature no boundary conditions for the diagnosed contributions (i.e. tagged tracers) at the lateral (and top) boundaries of the regional model domain (e.g. Li et al., 2012; Kwok et al., 2015; and Valverde et al., 2016). Therefore, these approaches have special categories for the contributions from lateral and/or top boundaries. In these cases long-range transported ozone (or other species) is not attributed correctly to the emission sources themselves. Instead, these approaches attribute a given part of the ozone mixing ratios at a specific point to contributions from lateral and/or top boundaries. Therefore, our approach allows for a consistent zooming into the area of interest, including an apportionment of the contribution of emissions from different sources to ozone and its relevant precursors across the lateral and top boundaries of the regional model instances. This is especially important for chemical species with a long lifetime, such as ozone, as large parts of the ozone mixing ratios at a certain place are influenced by long-range transport or subsidence from the stratosphere.
Table 2Overview of the applied MECO(2) simulation set-ups and simulation periods. For the EMAC instance the same set-up is applied in all simulations, but the set-ups of the COSMO-CLM/MESSy instances (CM50 and CM12) are varied systematically. More details are given in the text. The note “calculated by EMAC” in the row “biogenic emissions” means that the emissions, which are calculated by EMAC, are transformed to the COSMO-CLM grid during runtime via the MMD2WAY submodel.
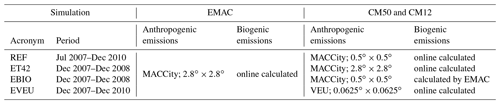
It is important to note that this method is a classical downscaling method and not a grid-refinement technique, which means that with MECO(2) for instance over Germany we calculate the contributions three times, once in each model instance (EMAC, CM50, and CM12). By comparing the results of the different model instances the impact of the model resolution (and the model itself) can be investigated.
2.2 Analysis concept and performed model simulations
The goal of our study is to investigate how diagnosed contributions of different emissions to ozone in Europe are influenced by model uncertainties such as the following:
-
the applied model,
-
the resolution of the model,
-
the resolution of the emission inventory, and
-
the emission inventory.
For this analysis, four different MECO(2) simulations are performed which are named REF, ET42, EBIO, and EVEU (see Table 2). In all simulations the same set-up for the EMAC instance is applied, involving the MACCity emission inventory (Granier et al., 2011) with a resolution of . The set-ups of the CM50 instance and CM12 instance (if applied) are varied systematically between the different simulations. The conceptualisation of these variations is described in the following paragraphs.
For the REF simulation the MACCity emission inventory is applied in EMAC, CM50, and CM12 at its finest available resolution. This means that the MACCity emissions are transformed onto a grid of 2.8∘ × 2.8∘ resolution in EMAC and to a grid of 0.44∘ × 0.44∘ in CM50 (and 0.1∘ × 0.1∘ resolution in CM12). The transformation from the original resolution of the emissions onto the model grid is performed online (i.e. during runtime) via the MESSy submodel GRID (Kerkweg et al., 2018). Here, a conservative remapping approach is used to transform the emissions onto the model grid. We chose this approach because, in this way, we need to store the emission data only once at their original resolution, and we are always using the finest possible resolution. We do not use any proxies for downscaling the emissions on the model grid (e.g. population density). However, due to the different model resolutions, the emissions are distributed differently into the gridboxes. The different geographical distribution of the emissions due to the transformation onto the finer grids is shown in Fig. S16 in the Supplement. This simulation serves as a reference. Differences between the results of EMAC and CM50 (and CM12) can be attributed to model differences: (1) the dynamical core and physical parameterisations between EMAC and COSMO-CLM/MESSy differ; (2) the resolutions of these models differ; and (3) EMAC and COSMO-CLM/MESSy calculate different soil NOx and biogenic C5H8 emissions. The last item is due to the dependencies on meteorology and different soil types in EMAC and COSMO-CLM/MESSy.
The sensitivity simulations help to disentangle these factors. The simulation ET42 applies the identical emissions in CM50 and EMAC, meaning the emissions are first transformed onto the coarse grid of EMAC (2.8∘ × 2.8∘; T42) before they are applied at this coarse resolution in CM50. Accordingly, EMAC and CM50 use the same effective resolution of the anthropogenic emissions. By comparing the CM50 results of REF and ET42, the effect of the emission inventory resolution can be analysed.
In the simulation EBIO, the biogenic C5H8 and soil NOx emissions as calculated by EMAC are transformed down and applied at the resolution of EMAC in CM50. By comparing the results from CM50 of the simulations REF and EBIO, the effect of the differently simulated biogenic emissions can be analysed. These differences in the biogenic emissions are caused by different meteorological conditions simulated by EMAC and CM50.
Finally, the simulation EVEU was performed. In this simulation a different emission inventory is used for the following emission sources: shipping, land transport, and anthropogenic non-traffic emissions. This emission inventory is only available for Europe with a resolution of 0.0625∘ × 0.0625∘ and is an outcome of the DLR project “Verkehrsentwicklung und Umwelt” (VEU; Hendricks et al., 2017). We use the results of the EVEU simulation mainly to compare the impact of the model and the model and the emissions inventory resolutions (REF, EBIO, ET42) with the impact of the uncertainty of emission inventories on the source apportionment results. A full analysis of the differences between the emission inventories is beyond the scope of the present paper and is presented in Mertens et al. (2019). Further, the finer resolution of the emission inventory allows us to compare the results of CM50 and CM12 in order to investigate the effect of an increased model and emission inventory resolution. The total emissions applied in all simulations are given in the Supplement in Tables S3–S11.
The REF simulation covers the period from July 2007 to December 2010. All sensitivity simulations branch off from the REF simulation in December 2007. The simulation period for the EVEU simulation ranges from December 2007 to December 2010. The simulations ET42 and EBIO cover just 1 year, ending in December 2008. Due to the high number of computational resources needed for the CM12 model instance, the CM12 instance is only activated for the period from May to August 2008 and for the simulations REF and EVEU (see also Fig. S15).
All chemical species, as well as the tagging diagnostics, are initialised from a 6-month (from January 2007 to July 2007) spin-up simulation with the EMAC model. This spin-up simulation was initialised with trace gas mixing ratios from the RC1SD-base-10a simulation described in detail by Jöckel et al. (2016). The soil model from COSMO-CLM/MESSy (TERRA) is initialised from a prior simulation for the period January 1983–July 2007 that does not include chemistry. MECO(n) is operated in the so-called quasi chemistry transport model (QCTM) mode (Deckert et al., 2011; Mertens et al., 2016). In this mode, chemistry and dynamics are decoupled to increase the signal-to-noise ratio for small chemical perturbations. This means that even though the emissions differ between the different simulations, each model instance (EMAC, CM50, and CM12) simulates the same meteorology in all simulations, which of course does not imply that the meteorology between the different model instances (EMAC, CM50, and CM12) is the same. In EMAC, the QCTM mode is implemented by applying climatologies for the following processes: (a) the radiation calculations (CO2, CH4, O3, N2O, CFC-11, and CFC-12; submodel RAD), (b) the heterogeneous chemistry calculations (HNO3; submodel MSBM; Multiphase Stratospheric Box Model), and (c) methane oxidation in the stratosphere (OH, O1D, and Cl; submodel CH4). In COSMO-CLM/MESSy only the climatology of nitric acid for the calculation of heterogeneous chemistry is needed. The applied climatologies are monthly mean values from the RC1SD-base-10a simulation.
For our comparison we focus on the period June–August (JJA) when the ozone production is largest. Further, we compare the results on the coarsest grid, to analyse if the finer resolution leads to any added value compared to the coarse resolution.
Table 3Root-mean-square error (RMSE; in micrograms per cubic metre) and normalised mean bias error (MB; in percent) of O3 for EMAC and CM50 in comparison with ground-level observations. Shown are the average values for June to August 2008. The values are calculated from monthly mean values. The model values are height corrected as discussed in detail by Mertens et al. (2016).
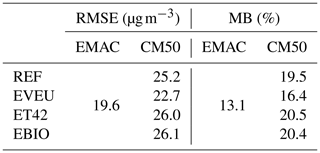
To evaluate the performance of the different model instances and the different simulations, we compare the model results with ground-level observations of ozone and measurements from ozone sondes. For the evaluation we use observations by the European Monitoring and Evaluation Programme (EMEP)(EMEP; http://www.emep.int; last access: 20 January 2020; Tørseth et al., 2012) and ozone sonde data from the world ozone database (WOUDC; http://woudc.org; last access: 20 January 2020). The methodology is described in detail by Mertens et al. (2016). In comparison to Mertens et al. (2016), however, we focus here on average values for JJA 2008 instead of values for June and December 2008. A list of the observation data used is part of the Supplement (Sect. S4).
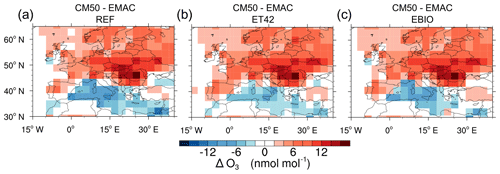
Figure 3Difference (in nanomoles per mole) between JJA average ozone mixing ratios from 2008 as simulated by CM50 and EMAC (“CM50 − EMAC”). (a) REF simulation, (b) ET42 simulation, and (c) EBIO simulation.
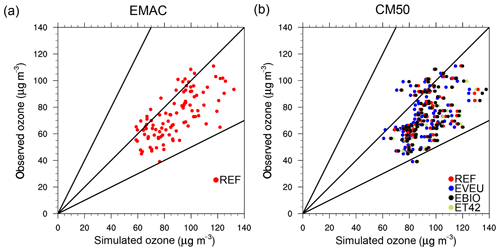
Figure 4Scatter plot of the observed versus simulated ozone concentrations (in micrograms per cubic metre) in (a) EMAC and (b) CM50. Each dot represents a monthly mean value for one station in the period from June to August 2008. The black lines indicate the 1 : 1 line (observed and simulated concentrations are equal) and the range (between lines with slopes of 0.5 and 2, indicating a factor of 2). For EMAC, only the results of the REF simulation are shown, as the set-up of EMAC is identical in all simulations.
For a quantitative evaluation we chose the metrics RMSE (root mean square error) and MB (normalised mean bias error). The definition of both quantities is given in Appendix A. Table 3 lists the RMSE and MB of the EMAC and CM50 instances for each simulation. As the EMAC set-up is identical in all simulations, the model results do not change. Generally, the results from the models are in agreement with the measurements. The RMSE has a range of around 19 to 26 µg m−3 and the MB has a range of 13 % to 21 %. These deviations from the measurements are in the range of comparable model systems (e.g. Knote et al., 2011; Stock et al., 2014). As already noted by Mertens et al. (2016), CM50 exhibits a larger positive ozone bias than EMAC. This bias is mainly caused by a more efficient vertical mixing in COSMO-CLM, as well as by a less stable boundary layer during the night. The latter is a common problem of many models and leads to diurnal cycles with ozone values that are too large during the night, resulting in an overall ozone bias (e.g. Travis and Jacob, 2019). The results of CM12 are not presented here, as the domain only covers Germany, and therefore fewer stations can used for evaluation. The RMSEs and MBs for CM50 and CM12 are given in the Supplement (Table S2) and take into account the measurements at all stations located in the region covered by the CM12 domain.
In general, CM50 simulates larger ozone mixing ratios than EMAC over the continent (see Fig. 3). This ozone bias in the case of CM50 compared to EMAC is caused neither by the finer resolution of the emissions nor by the different biogenic emissions compared to EMAC, because the positive ozone bias for CM50 compared to EMAC is also apparent in the results of ET42 and EBIO. Only over the Mediterranean Sea, lower ozone values are simulated by CM50 compared to EMAC. These lower ozone mixing ratios can be partly attributed to the coarser resolution of the emissions in EMAC compared to CM50, as the difference is lower in the ET42 simulation (Fig. 3b). The simulated ozone mixing ratios in CM50 are up to 7.5 nmol mol−1 larger (in JJA 2008) in ET42 compared to REF. Averaged over the area of the Mediterranean Sea the increase in ozone is around 3 nmol mol−1. The application of the soil NOx and biogenic emissions calculated by EMAC in CM50 (EBIO) leads to an increase in the ozone mixing ratios of 1 to 3 nmol mol−1. The differences are largest over south-eastern Europe, the Mediterranean Sea, and the Iberian Peninsula (Fig. 3c). Overall, the differences in the results of CM50 between REF, EBIO, and ET42 are small compared to the bias between EMAC and CM50. In particular, the positive ozone bias over Serbia and Bulgaria cannot be attributed to different biogenic emissions or the coarser resolution of the emission inventories in EMAC compared to CM50.
Figure 4 shows scatter plots comparing observed and simulated ozone monthly mean concentrations at all considered stations of the EMEP network. The simulated concentrations in all model instances and simulations lie, with one outlier, within a factor of 2 of the measurements. As already discussed, the simulated ozone concentrations at most stations show a positive ozone bias. The simulated ozone concentrations are lower than the measured ozone concentrations only at a few stations . The ozone bias is very similar in all CM50 simulations; EBIO and ET42 show almost the same bias as REF. Only the simulation EVEU shows a slightly lower positive ozone bias. Accordingly, the change in the anthropogenic emission inventory has a larger impact on the model results than the influence of the emission inventory resolution and the geographical distribution of the biogenic emissions.
To evaluate the simulated ozone mixing ratios in the free troposphere, the model results are compared to ozone sonde data (see Sect. S4 in the Supplement for a list of considered stations). In total, 510 individual ozone sonde launches are considered for the year 2008. To compare the ozone sonde data with the model results, the vertical ozone profiles simulated by the model were sampled online at every time step of the model at the location where the ozone sonde was launched. Drifts of the ozone sonde by winds are not taken into account. For every launched ozone sonde, we averaged the simulated vertical profiles in time over the measurement period (usually some hours). These vertical profiles of simulated ozone mixing ratios are compared to the measurements of the ozone sonde data. As the main focus of this comparison is the free troposphere, we restrict this analysis to all data in the pressure range of 600 to 200 hPa.
The probability density functions (PDFs) for the measured and simulated vertical ozone distributions are displayed in Fig. 5. The results show that in the free troposphere both model instances (EMAC, CM50) simulate very similar vertical ozone distributions. Accordingly, the positive ozone bias of CM50 compared to EMAC is confined to the boundary layer. Further, in general a positive ozone bias is apparent, which is will known for EMAC (e.g. Righi et al., 2015; Jöckel et al., 2016).
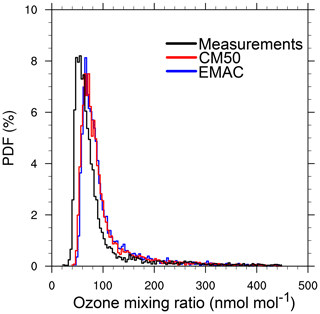
Figure 5Probability density functions (PDFs) of observed (ozone sondes) and simulated vertical ozone mixing ratios in the pressure region between 600 and 200 hPa. Considered are the 510 ozone sonde launches for 2008 in Europe.
3.1 Differences in ozone production
In a next step, the difference between the ozone production simulated by EMAC and CM50 is analysed (for the REF simulation). For this, we consider the net ozone production (), which is calculated as follows:
with the production (ProdO3) and loss rates (LossO3) determined by the chemical solver (for more details see the Supplement of Grewe et al., 2017).
We define as . is largest in the lower troposphere (see Fig. 6a). As indicated by the negative numbers, CM50, in general, simulates lower values of than EMAC. Zonally averaged is around 60 to 80 lower in CM50 than in EMAC, which corresponds to 10 % to 20 %. The largest differences (up to 100 or 40 %) are simulated over the Mediterranean Sea (see also Fig. S1 in the Supplement).
To separate effects caused by the emission inventory resolution from the effects caused by the model resolution and specific model biases, Fig. 6b shows the differences in between ET42 and REF (). The positive values indicate the effect of increased with reduced resolution of the emission inventory, which is caused by the dilution effect of the emissions on the coarse grid (e.g. Tie et al., 2010). The differences are largest in the Mediterranean area, with an increase in in CM50 of up to 40 in ET42 compared to REF. These differences are mainly simulated in areas of the Alboran Sea and Balearic Sea, as well as in areas of the Levantine Sea (see also Fig. S2 in the Supplement). The main reasons for these differences are the dilution of the shipping emissions and the large anthropogenic emissions in Israel when coarse emissions are applied. As the ozone production is strongly nonlinear this dilution of the emissions leads to an artificial increase in the ozone production rate.
The differences, which cannot be attributed directly to the resolution of the anthropogenic emission inventory, are caused by a variety of other model factors which cannot be disentangled in detail. The most important factor in this context is the enhanced vertical mixing in CM50 compared to EMAC, mainly in the boundary layer; it is also due to stronger convective up- and downdraft mass fluxes in CM50 compared to EMAC. The enhanced vertical mixing transports higher amounts of ozone from the free troposphere into the boundary layer, leading to higher ozone mixing ratios in the boundary layer. In addition, ozone precursors are transported more efficiently from the boundary layer into the free troposphere. Further, differences in the land use classes between EMAC and CM50 lead to differences in the calculated dry deposition velocities, which affects also ozone mixing ratios near the surface (see also Mertens et al., 2016).
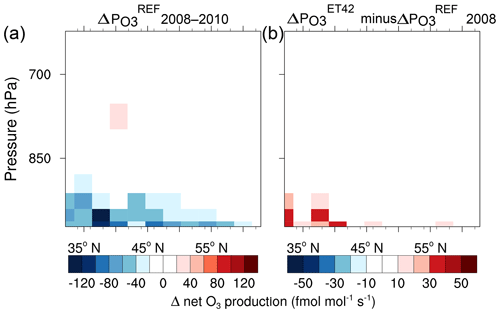
Figure 6Zonally averaged differences in ozone production (), between CM50 and EMAC (in femtomoles per mole per second). (a) calculated from the results of the REF simulation for JJA 2008–2010. (b) Differences in between the ET42 and REF simulations only for the year 2008. The CM50 data have been transformed on the horizontal and vertical grid of EMAC.
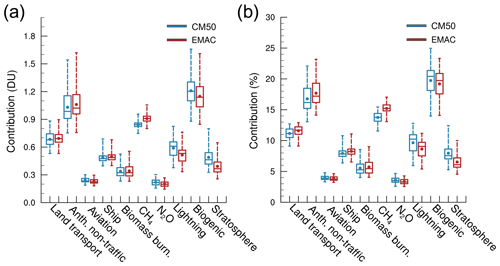
Figure 7Box-and-whisker plot for (a) the absolute (in Dobson units) and (b) relative (in percent) contribution to the ozone column up to 850 hPa. The values are area averaged over the CM50 domain. The lower and upper ends of the boxes indicate the 25th and 75th percentiles, the middle bars represent the medians, the dots represent the average, and the whiskers represent the ranges of the time series for the JJA values from 2008 to 2010.
Figure 7 shows the absolute and relative contributions of different emission sources to the European ozone column up to 850 hPa as simulated by EMAC and CM50 for the REF simulation (see Table S1 in the Supplement for a detailed definition of the tagging categories). The largest absolute and relative ozone contributors are the anthropogenic non-traffic and the biogenic categories, both with contributions of more than 1 DU, corresponding to more than 15 %. Both model instances simulate similar absolute ozone contributions from the categories anthropogenic non-traffic (≈ 1.0 DU), land transport (≈ 0.7 DU), shipping (≈ 0.5 DU), and biomass burning (≈ 0.4 DU). For the biogenic category, CM50 calculates slightly larger absolute contributions compared to EMAC (see Sect. 4.2), but the differences are small compared to the temporal variability in the contributions. Further, CM50 calculates larger absolute contributions from the lightning and stratosphere categories. This mainly affects the categories land transport, anthropogenic non-traffic, shipping, and biomass burning, where EMAC simulates 0.1 to around 1 percentage point larger relative contributions compared to CM50. At the same time, the increased vertical mixing in CM50 leads to an increase in the relative contributions from the categories stratosphere, lightning, and aviation compared to EMAC. Here, the differences are in the range of 0.1 to around 1.5 percentage points.
The positive ozone bias of CM50 compared to EMAC indicates vertical mixing that is too efficient in CM50 (see Sect. 3). Therefore, the larger contributions from the categories stratosphere and lightning in CM50 compared to EMAC are likely an artefact of this too efficient vertical mixing. However, this could partly be a feature of the increased resolution, as individual stratosphere–troposphere exchange (STE) events are better represented in CM50 compared to EMAC due to the increased resolution (Hofmann et al., 2016; Mertens et al., 2016). Generally the correct representation of STE events poses a big challenge in most models (e.g. Zhang et al., 2011; Lin et al., 2012; and Lefohn et al., 2014), and our results suggest a large difference in the contribution of STE to ground-level ozone between the results of different models.
The values which we have discussed so far, however, are averages on the continental scale. On the regional scale the differences can be much larger. Geographical distributions of the differences in the absolute and relative contributions as simulated by EMAC and CM50 are given in the Supplement (Figs. S3 and S4). Exemplarily, we want to focus on the categories land transport, an important anthropogenic emission source, and biogenic emissions. As discussed in Sect. 2, the biogenic emissions are calculated online by both model instances and depend on the meteorology and surface properties. While the total emissions are comparable, the geographical distribution and the area-averaged contribution differ (see Supplement Fig. S17 and Tables S2 to S10). As disparity between online-simulated emissions is a typical inter-model difference, a detailed investigation of the influence of these differences is of interest.
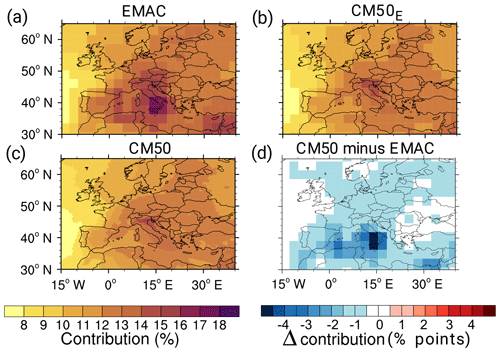
Figure 8Comparison of the JJA average relative contribution of to ground-level O3 (in percent) of EMAC and CM50: (a) results of EMAC, (b) results of CM50 transformed onto the EMAC grid, (c) results of CM50 on the original grid, and (d) difference (“CM50 minus EMAC” in percentage points) on the coarse grid. The comparisons in (a–c) use the same scale. Shown are the results of the REF simulation, averaged over 2008–2010.
4.1 Contribution of land transport emissions to ground-level ozone
Averaged over JJA 2008 and the European area (defined as rectangular box from 10∘ W to 30∘ E and 32∘ to 65∘ N; see red square in Fig. 1) EMAC simulates a relative contribution of the land transport emissions (denoted as ) to ground-level ozone of 13.1 %, while CM50 simulates a contribution of 11.9 %. A decrease in the emission resolution in CM50 increases the relative contribution to 12.1 % (ET42 simulation), and the change in the anthropogenic emission inventory in CM50 increases the contribution to 12.7 % (EVEU simulation). In all cases, similar absolute contributions of are simulated and range between 6.0 and 6.4 nmol mol−1.
The area-averaged values indicate that the inter-model differences between CM50 and EMAC, as discussed in detail in Sect. 3, have a larger influence on the calculated contributions than the change in the anthropogenic emission inventory. The impact of the coarsely resolved emission inventory on the area-averaged values is rather small. In general, the difference in the average contributions of simulated by the two model instances (EMAC and CM50), as well as simulated by CM50 for the four different simulations, is ≈ 10 % at maximum. In comparison, the differences in the contributions to ground-level O3 between EMAC and CM50 from the lightning and stratosphere categories are much larger, ≈20 % and ≈30 %, respectively.
Regionally, the differences in the relative contribution of to ground-level ozone (see Fig. 8) can be larger than the area-averaged differences. In general, both model instances simulate a comparable distribution, with the largest relative contribution of in the Mediterranean region and contributions of around 8 % over the western Atlantic. These values are larger (10 %–18 %) over the continent than over the sea. CM50 simulates a 0.5–1 percentage point lower relative contribution compared to EMAC. As discussed before, this is partly caused by stronger vertical mixing and reduced ozone production () in CM50 compared to EMAC. With increasing altitude the differences between EMAC and CM50 decrease (see Fig. S5 in the Supplement).
The largest differences in the relative contribution of to ground-level ozone are simulated around the Mediterranean area. The differences over the Mediterranean Sea (2 percentage points or more, corresponding to more than 10 %) can partly be attributed to the coarse resolution of the emissions in EMAC compared to CM50. The coarse resolution leads to an artificial increase in (see Sect. 3.1), which in turn leads to an increase in the contribution from (and other anthropogenic categories). Accordingly, the results of CM50 from the ET42 simulation show regionally up to 3 nmol mol−1 and 3 percentage points larger contributions of land transport emissions to ozone than the results from the REF simulation (see also Fig. S7 in the Supplement). However, the large differences over southern Italy and Sicily between CM50 and EMAC especially cannot be attributed to the coarse resolution of the emissions. Here, EMAC simulates the largest contribution (up to 17 %) in the European region (particularly around the Naples region with large land transport emissions), while CM50 simulates contributions of around 13 %. On the coarse EMAC grid most parts of southern Italy are considered as sea, especially affecting the dry deposition calculation in EMAC, as dry deposition of ozone is lower over sea than over land. Therefore, the coarse resolution of the land–sea mask in EMAC compared to CM50 leads to an artificial underestimation of the ozone dry deposition in EMAC. In addition, the coarse land–sea mask leads to differences in the calculation of biogenic emissions. In particular, over Sicily EMAC simulates no biogenic emissions (including soil NOx) while CM50 simulates large emissions there (see Fig. S17 in the Supplement). Accordingly, soil NOx and anthropogenic NOx do not compete in this area in EMAC, and ozone is mostly formed from anthropogenic emissions. Compared to these artificial peaks simulated by EMAC around Naples and over Sicily, CM50 shows the largest contribution (up to 15 %) around the Po Valley. In this region, large amounts of emissions by land transport take place, and ozone production is enhanced by stable and sunny weather conditions. The differences between EMAC and CM50 around the Naples region are even larger (up to 6 percentage points; see Fig. S6 in the Supplement) for the extreme values (95th percentile) than for the mean values which were discussed so far. Accordingly, extreme values are even more strongly deteriorated than the mean values by the coarse land–sea mask problems discussed above.
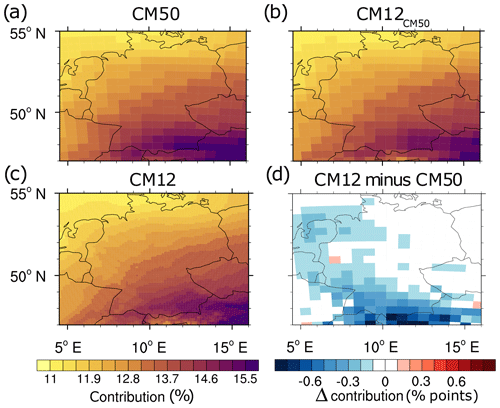
Figure 9Comparison of the JJA average ground-level contribution of to O3 (in percent) of CM50 and CM12: (a) results of CM50, (b) results of CM12 transformed onto the CM50 grid, (c) results of CM12 on the original grid, and (d) difference (“CM12 minus CM50” in percentage points) on the coarse grid. The comparisons (a–c) use the same scale. Shown are the results of the EVEU simulation, averaged over 2008.
The further increase in resolution from 50 km (CM50) to 12 km (CM12) impacts ozone and the contributions of ozone only slightly (see Fig. S11 in the Supplement). In general, we note a decrease in the absolute ozone values, as well as the absolute contributions of anthropogenic emissions (including the land transport category) near the hotspot regions (e.g. Rhine-Ruhr, Munich, and Frankfurt), if the model resolution is increased (REF simulation). The increase in the resolution of the emission inventory (EVEU simulation) intensifies this effect; i.e. near the hotspots ozone values and absolute contributions of decrease further. In southern and eastern Germany, however, the ozone values increase. As a comparison of the contributions of the individual tagging categories shows, this is mainly caused by an increase in the contributions from stratospheric ozone and the CH4 category. The increase in stratospheric ozone is partly caused by the enhanced topography in CM12 compared to CM50 as well as larger convective up- and downdraft mass fluxes in CM12 compared to CM50. The larger contribution of ozone from the CH4 category (meaning more ozone formed by reactions involving CH4 oxidation products) is consistent with the finding of a larger tropospheric oxidation capacity (i.e. lower methane lifetime) in CM12 compared to CM50 by Mertens et al. (2016).
CM12 simulates a lower relative contribution of to ground-level O3 over Germany than CM50 (see Fig. 9). The difference is largest in southern Germany; however it is mostly below 0.5 percentage point (corresponding to less than 5 %). The differences between the mean and 95th percentile (see Fig. S12 in the Supplement) of the contributions of between CM12 and CM50 are much smaller compared to the differences caused by different anthropogenic emissions inventories (e.g. the differences between the results of the REF and EVEU simulation). Accordingly, the differences in emission inventories dominate over differences caused by the resolution of emission inventories and models when comparing the results of CM50 and CM12.
What is not discussed here in detail is the influence of the difference in the shorter-lived species, e.g. NO2 or the tagged contributions to NOy, which differ largely between the two resolutions. Here, maxima (e.g. in Stuttgart or around the Rhine-Ruhr area) are displaced in the coarser resolution (CM50) compared to the finer resolution (CM12). However, the direct influence of displaced precursors on ozone itself is not very large, because ozone formation usually takes place downwind of the source itself. Further, compared to previous studies investigating the influence of the model/emission inventory resolution on ozone (e.g. Wild, 2007; Tie et al., 2010; and Markakis et al., 2015), it is important to note that we apply a chemistry-climate model in which both the chemical processes and the meteorology are calculated on the finer grid. This can alter the results compared to studies applying simpler chemistry-transport models.
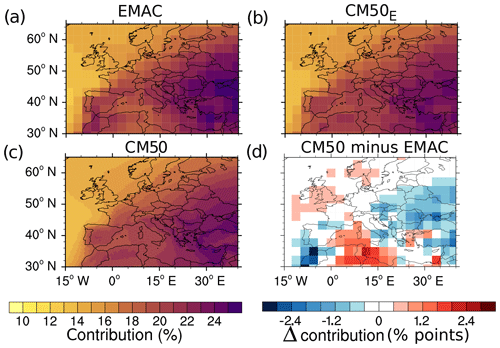
Figure 10Comparison of the JJA average ground-level contribution (in percent) of to O3 of EMAC and COSMO-CLM/MESSy: (a) results of EMAC, (b) results of CM50 transformed onto the EMAC grid, (c) results of CM50 on the original grid, and (d) difference (“CM50 minus EMAC” in percentage points) on the coarse grid. Comparisons in (a–c) use the same scale. Shown are the results of the REF simulation, averaged over 2008–2010.
4.2 Contribution of biogenic emissions to ground-level ozone
The JJA 2008 average relative contribution of ozone from biogenic emissions (mainly soil NOx and biogenic C5H8, denoted as ) to ground-level O3 in Europe (see Sect. 4.1 for the definition) ranges from 19.0 % to 19.6 % in all simulations. Hence, the differences in the relative contribution of to ground-level ozone on the continental scale are rather small (below 5 %). The same is true for the absolute values, ranging from 9.3 to 9.7 nmol mol−1.
With respect to the geographical distribution (Fig. 10) EMAC and CM50 simulate a strong north-west-to-south-east gradient, with relative contributions from of around 10 % over the Atlantic and more than 20 % over south-eastern Europe. In contrast to the contribution of , EMAC simulates not generally larger contributions of than CM50. Instead, EMAC simulates (REF simulation) larger contributions (1–2 percentage points) over south-eastern Europe, Morocco, and the Iberian Peninsula, while CM50 simulates around 1–2 percentage points larger contributions over large parts of the Mediterranean Sea as well as over northern Africa. Also around the British Isles and Scandinavia, CM50 simulates around 0.5 percentage point larger contributions of than EMAC. Averaged over the CM50 domain, CM50 ends up with a 0.5 percentage point larger contribution of than EMAC. Similar to the land transport category, the differences between the results of both model instances decrease with increasing height, but the general pattern remains similar (see Fig. S8 in the Supplement).
The differences between EMAC and CM50 are only partly caused by the different geographical distribution of the biogenic emissions in EMAC compared to CM50. When applying the biogenic emissions as calculated by EMAC in CM50 (EBIO simulation), the relative and absolute contributions of increase mainly in the Mediterranean area, by up to 2 percentage points and 3 nmol mol−1, respectively (see Figs. S9 and S10 in the Supplement). The characteristic dipole pattern, with lower contributions of in south-eastern Europe and higher contributions in southern Europe and northern Africa, in CM50 compared to EMAC is similar. This pattern can partly be attributed to the coarse resolution of the shipping emissions in EMAC, leading to a positive ozone bias in the Mediterranean Sea (see Sect. 3). The dipole pattern, however, is caused neither by the coarse resolution of the emissions nor by the different biogenic emissions, but rather mainly by the differences between the meteorology simulated by EMAC and CM50.
In general, we conclude that regional differences in the relative and absolute contribution of caused by inter-model differences, emission resolution, and different geographical distribution are up to 15 %. Averaged over Europe the differences are lower (10 %). Again, these differences are lower than for example the differences of approximately 30 % of the observed contributions from the stratosphere between the results of EMAC and CM50.
So far, the results indicate that with respect to average values on a continental scale the differences caused by the resolutions of the model/emission inventory are rather small. This confirms findings by Stock et al. (2013), which reported only a small influence of the global redistribution of megacity emissions (which can be seen as a locally decreased emission resolution) on the global ozone budget.
To summarise and quantify these differences in more detail, Fig. 11 shows the (a) absolute and (b) relative contributions of to ground-level ozone averaged over the CM50 domain, as well as for the geographical regions defined in the PRUDENCE project (Christensen et al., 2007). The results of EMAC are not analysed for these geographical regions because, due to the coarse resolution, some regions would only consist of a few grid points.
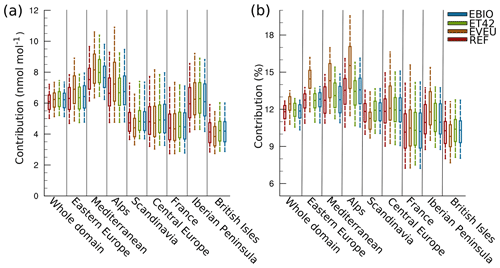
Figure 11Comparison of the contributions of to ground-level ozone for JJA 2008 between the four simulations. (a) The absolute contribution in nanomoles per mole and (b) the relative contribution to ground-level ozone (in percent). All values are area averaged over the respective region and are calculated using the results of the CM50 instance. The lower and upper ends of the box indicate the 25th and 75th percentile, respectively; the bar indicates the median, and the whiskers indicate the 5th and 95th percentiles of the time series for the JJA values from 2008 based on output every 3 h from the model.
Figure 11 also shows that on the scale of smaller regions the absolute and the relative contribution of to ground-level ozone is only slightly influenced by the coarse resolution of anthropogenic emission inventories (ET42) as well as having a different geographical location and resolution of biogenic emissions (EBIO). This holds not only for the mean contributions, but also for the extreme values expressed by the 95th percentile. Further, the simulated differences in the biogenic and shipping categories, which are more affected by the differences in the emission inventories in the two simulations, are also rather small (see Figs. S13 and S14 in the Supplement). The largest simulated differences in the mean contribution of shipping emissions to ground-level ozone between the REF, EBIO, and ET42 simulations are around 0.5 nmol mol−1 and below 0.5 percentage points. The largest change (95th percentile) in the biogenic category in the Iberian Peninsula region is around 0.7 nmol mol−1 and 0.5 percentage point.
Compared to the differences between the contributions of in the REF, ET42, and EBIO, the differences caused by a changed emission inventory (EVEU) are larger. In the Mediterranean region, the mean and 95th percentile of the contributions of increase by 1 nmol mol−1 and 2 percentage points, respectively. In the Alps region, the increase in the mean and 95th percentile of the contributions is up to 1.3 nmol mol−1 and 3 percentage points, respectively. Similarly, for the contribution of shipping emissions the differences are largest with the changed emission inventory (up to 1.5 nmol mol−1 and 1 percentage point). Accordingly, changes in the resolution of the emission inventory or the biogenic emissions can affect the contribution from anthropogenic categories (such as land transport and shipping). However, on the regional scale the main drivers of uncertainties are clearly the anthropogenic emissions and differences caused by the model resolution and/or model differences. For example we found regional differences (see Sect. 4.1) in the contribution of to ground-level O3 between EMAC and CM50 of up to 20 % around the Naples region, which in this case can mainly be attributed to the coarse land–sea mask used in EMAC, leading to land transport emissions to occur over the sea.
The results of the model evaluation, however, are not very helpful in judging which of the two emission inventories are more realistic. Although EVEU shows a smaller ozone bias compared to REF, caused by reduced precursor emissions, it is unclear if lower anthropogenic non-traffic emissions in the VEU compared to MAC emission inventories are realistic.
In the present study, we are focusing on the question: are contributions of emissions to ozone a matter of scale? To answer this question we compare the influences of the model, the model resolution, the emission resolution, and the emission inventory on the results of ozone contribution analyses. For this we apply the MECO(n) model system which combines a global and a regional model by means of an online nesting technique. By applying the identical tagging diagnostics (source apportionment method) in the regional and global model with consistent boundary conditions, we are able to compare the results of model instances with different resolutions to investigate the influence of the model and emission inventory resolutions onto the diagnosed ozone contributions. Such analyses are important for quantifying uncertainties of ozone source apportionment studies, which arise due to limitations of the model and/or computational resources.
For the specific model set-up involving the global model EMAC and the regional model COSMO-CLM/MESSy our results show that simulated differences in ozone contributions on a continental scale (e.g. Europe) are rather small. The largest differences in the contribution of anthropogenic emission sources were up to 10 % for the contribution of land transport emissions to ground-level ozone. However, the contribution of stratospheric ozone to ground-level ozone calculated by EMAC and COSMO differs by up to 30 %. One main reason for this large difference in the contributions of stratospheric ozone between the two models is the existence of enhanced vertical mixing and larger convective up- and downdrafts in COSMO-CLM/MESSy compared to EMAC. Taking the comparison with the measurements into account, the vertical mixing in COSMO-CLM/MESSy and the enhanced stratospheric contribution are likely too large. On the regional scale, the differences between the contributions of anthropogenic emission sources simulated by COSMO-CLM/MESSy and EMAC are much larger. Here, we observed differences of up to 20 % for the contributions of land transport emissions to ground-level ozone. This difference is mainly caused by the coarse land–sea mask used in the global model instance, leading to emissions of land transport emissions over sea, different ozone dry deposition, and missing biogenic emissions. Taking the results of the same model instance (CM50) into account, the largest influence on the results is caused by different emissions inventories. Locally, however, coarsely resolved emission inventories and differences between the biogenic emissions can also lead to differences of up to 20 %. In addition, we showed how the differences in the source apportionment results between different model instances can help to explain model biases and the physical/chemical mechanisms causing these biases.
Apart from the many model specific findings of this study, its results have important implications for other modelling studies and modellers who are applying source apportionment methods. These implications are as follows:
-
First, our study shows that average continental contributions of anthropogenic emissions are quite robust with respect to the model and the model resolution used. This means that global models at coarse resolution can be used to perform ozone source apportionment in the global context.
-
Second, our results also show that on the regional scale, the differences caused either by different models or by model resolution can be larger. These effects arise mainly near hotspot regions like the Po Valley or near major shipping routes in the Mediterranean Sea. However, especially in these areas, contribution analyses of anthropogenic emissions are very important, and spurious effects, such as artificially increased ozone levels and contributions caused by the coarse resolution of models and/or emission inventories should be avoided. Hence, for regional analyses finely resolved models and emission inventories are required.
-
Third, our results clearly indicate how large the spread between models is, with respect to STE. The importance of stratospheric ozone, both in the global and regional model, corroborates the necessity for tracing the contributions of stratospheric ozone to ground-level ozone explicitly by the source apportionment methods. However, only a few currently available methods used on the regional scale account for this process.
Clearly, this study is only a first step in quantifying the driving sources of uncertainties and, particularly, the role of the model and emission inventory resolutions on the results of ozone contribution studies. Especially, as some processes like vertical diffusion or vertical transport can heavily alter the model results, follow-up studies need to take into account more (and different) models to better quantify the uncertainties due to differences in the meteorology simulated by different models. In addition, the two analysed anthropogenic emission inventories clearly do not reflect the whole spectrum of different emission estimates. Further, our analyses only focused on differences near the origin of the emissions. An increased resolution leads to a more realistic chemistry within the plumes downwind of the emission hotspots. This can affect the long-range transport from different precursors and might influence regions far away from the emission region. Especially, calculations of radiative forcings are very sensitive to ozone near the tropopause. In a coarsely resolved model, the overestimated absolute contributions might lead to a biased radiative forcing. This effect, however, is difficult to quantify and would require very finely resolved global chemistry-climate models or two-way nesting capabilities, which feed back information about the contributions from the fine grid back to the coarse grid. For a next step, a further increase in the model and emission resolution should be envisaged. Even if we found only small differences between the 50 and 12 km resolutions this step would be important, as even with a 12 km grid resolution emissions are diluted over large areas. A finer resolution could strongly reduce the dilution . Such an analysis, however, is hindered by two aspects. First, consistent emission inventories (anthropogenic and natural) with a resolution of 1 km over areas that are large enough to compare models on a regional and global scale must be available. Second, requirements with respect to the computational time of chemistry-climate models with ≈1 km resolution over large computational domains are very demanding, hindering the detailed quantification of the differences caused by the resolution over long integration periods.
We define the root mean square error (RMSE) as
where n is the number of data points, the simulated, and the measured ozone concentrations. The normalised mean bias error (MB) is defined as
where and are the simulated and measured ozone concentrations averaged for all stations and months, respectively.
The Modular Earth Submodel System (MESSy) is continuously being further developed and applied by a consortium of institutions. The usage of MESSy and access to the source code is licensed to all affiliates of institutions which are members of the MESSy Consortium. Institutions can become a member of the MESSy Consortium by signing the MESSy Memorandum of Understanding. More information, including on how to become a licensee for the required third-party software, can be found on the MESSy Consortium Website (http://www.messy-interface.org, last access: 20 January 2020). The code presented here was based on MESSy version 2.50 and is available in the official release (version 2.51). The namelist set-up used for the simulations is part of the Supplement. The data used for the Figs. 6 to 11 are part of the Supplement.
Analysis and graphics for the data used were performed using the NCAR Command Language (version 6.4.0) software developed by UCAR NCAR CISL TDD and available online at this site: https://doi.org/10.5065/D6WD3XH5 (NCL, 2017).
The supplement related to this article is available online at: https://doi.org/10.5194/gmd-13-363-2020-supplement.
MM performed the simulations, analysed the data, and drafted the manuscript. AK and PJ developed the model system.VG developed the tagging method. RS drafted the study. All authors contributed to the interpretation of the results and to the text.
The authors declare that they have no conflict of interest.
Mariano Mertens acknowledges funding by the DLR projects “Verkehr in Europa” and “Auswirkungen von NOx”. Furthermore, part of this work is funded by the DLR project “VEU2”.
We thank Roland Eichinger (DLR) and Markus Kilian (DLR) for very valuable comments that improved the manuscript considerably. Further, we acknowledge the comments from three anonymous referees, who improved the manuscript. We acknowledge the Leibniz-Rechenzentrum in Garching for providing computational resources on the SuperMUC Phase 2 under the project ID PR94RI.
This research has been supported by the BMBF (grant no. 01LP1127A).
The article processing charges for this open-access
publication were covered by the Research
Centre of the Helmholtz Association.
This paper was edited by Juan Antonio Añel and reviewed by three anonymous referees.
Butler, T., Lupascu, A., Coates, J., and Zhu, S.: TOAST 1.0: Tropospheric Ozone Attribution of Sources with Tagging for CESM 1.2.2, Geosci. Model Dev., 11, 2825–2840, https://doi.org/10.5194/gmd-11-2825-2018, 2018. a
Christensen, J. H., Carter, T. R., Rummukainen, M., and Amanatidis, G.: Evaluating the performance and utility of regional climate models: the PRUDENCE project, Clim. Change, 81, 1–6, https://doi.org/10.1007/s10584-006-9211-6, 2007. a
Clappier, A., Belis, C. A., Pernigotti, D., and Thunis, P.: Source apportionment and sensitivity analysis: two methodologies with two different purposes, Geosci. Model Dev., 10, 4245–4256, https://doi.org/10.5194/gmd-10-4245-2017, 2017. a
Dahlmann, K., Grewe, V., Ponater, M., and Matthes, S.: Quantifying the contributions of individual NOx sources to the trend in ozone radiative forcing, Atmos. Environ., 45, 2860–2868, https://doi.org/10.1016/j.atmosenv.2011.02.071, 2011. a
Deckert, R., Jöckel, P., Grewe, V., Gottschaldt, K.-D., and Hoor, P.: A quasi chemistry-transport model mode for EMAC, Geosci. Model Dev., 4, 195–206, https://doi.org/10.5194/gmd-4-195-2011, 2011. a
Dee, D. P., Uppala, S. M., Simmons, A. J., Berrisford, P., Poli, P., Kobayashi, S., Andrae, U., Balmaseda, M. A., Balsamo, G., Bauer, P., Bechtold, P., Beljaars, A. C. M., van de Berg, L., Bidlot, J., Bormann, N., Delsol, C., Dragani, R., Fuentes, M., Geer, A. J., Haimberger, L., Healy, S. B., Hersbach, H., Hólm, E. V., Isaksen, L., Kållberg, P., Köhler, M., Matricardi, M., McNally, A. P., Monge-Sanz, B. M., Morcrette, J.-J., Park, B.-K., Peubey, C., de Rosnay, P., Tavolato, C., Thépaut, J.-N., and Vitart, F.: The ERA-Interim reanalysis: configuration and performance of the data assimilation system, Q. J. Roy. Meteor. Soc., 137, 553–597, https://doi.org/10.1002/qj.828, 2011. a
Dietmüller, S., Jöckel, P., Tost, H., Kunze, M., Gellhorn, C., Brinkop, S., Frömming, C., Ponater, M., Steil, B., Lauer, A., and Hendricks, J.: A new radiation infrastructure for the Modular Earth Submodel System (MESSy, based on version 2.51), Geosci. Model Dev., 9, 2209–2222, https://doi.org/10.5194/gmd-9-2209-2016, 2016. a, b, c, d
Dunker, A. M., Yarwood, G., Ortmann, J. P., and Wilson, G. M.: Comparison of Source Apportionment and Source Sensitivity of Ozone in a Three-Dimensional Air Quality Model, Environ. Sci. Technol., 36, 2953–2964, https://doi.org/10.1021/es011418f, 2002. a, b
Emmons, L. K., Hess, P. G., Lamarque, J.-F., and Pfister, G. G.: Tagged ozone mechanism for MOZART-4, CAM-chem and other chemical transport models, Geosci. Model Dev., 5, 1531–1542, https://doi.org/10.5194/gmd-5-1531-2012, 2012. a, b
Eyring, V., Stevenson, D. S., Lauer, A., Dentener, F. J., Butler, T., Collins, W. J., Ellingsen, K., Gauss, M., Hauglustaine, D. A., Isaksen, I. S. A., Lawrence, M. G., Richter, A., Rodriguez, J. M., Sanderson, M., Strahan, S. E., Sudo, K., Szopa, S., van Noije, T. P. C., and Wild, O.: Multi-model simulations of the impact of international shipping on Atmospheric Chemistry and Climate in 2000 and 2030, Atmos. Chem. Phys., 7, 757–780, https://doi.org/10.5194/acp-7-757-2007, 2007. a, b
Fiore, A. M., Dentener, F. J., Wild, O., Cuvelier, C., Schultz, M. G., Hess, P., Textor, C., Schulz, M., Doherty, R. M., Horowitz, L. W., MacKenzie, I. A., Sanderson, M. G., Shindell, D. T., Stevenson, D. S., Szopa, S., Van Dingenen, R., Zeng, G., Atherton, C., Bergmann, D., Bey, I., Carmichael, G., Collins, W. J., Duncan, B. N., Faluvegi, G., Folberth, G., Gauss, M., Gong, S., Hauglustaine, D., Holloway, T., Isaksen, I. S. A., Jacob, D. J., Jonson, J. E., Kaminski, J. W., Keating, T. J., Lupu, A., Marmer, E., Montanaro, V., Park, R. J., Pitari, G., Pringle, K. J., Pyle, J. A., Schroeder, S., Vivanco, M. G., Wind, P., Wojcik, G., Wu, S., and Zuber, A.: Multimodel estimates of intercontinental source-receptor relationships for ozone pollution, J. Geophys. Res.-Atmos., 114, D04301, https://doi.org/10.1029/2008JD010816, 2009. a
Fiore, A. M., Naik, V., Spracklen, D. V., Steiner, A., Unger, N., Prather, M., Bergmann, D., Cameron-Smith, P. J., Cionni, I., Collins, W. J., Dalsøren, S., Eyring, V., Folberth, G. A., Ginoux, P., Horowitz, L. W., Josse, B., Lamarque, J.-F., MacKenzie, I. A., Nagashima, T., O'Connor, F. M., Righi, M., Rumbold, S. T., Shindell, D. T., Skeie, R. B., Sudo, K., Szopa, S., Takemura, T., and Zeng, G.: Global air quality and climate, Chem. Soc. Rev., 41, 6663–6683, https://doi.org/10.1039/C2CS35095E, 2012. a
Giorgetta, M. A. and Bengtsson, L.: Potential role of the quasi-biennial oscillation in the stratosphere-troposphere exchange as found in water vapor in general circulation model experiments, J. Geophys. Res.-Atmos., 104, 6003–6019, https://doi.org/10.1029/1998JD200112, 1999. a
Granier, C., Bessagnet, B., Bond, T., D'Angiola, A., van der Gon, H. D., Frost, G., Heil, A., Kaiser, J., Kinne, S., Klimont, Z., Kloster, S., Lamarque, J.-F., Liousse, C., Masui, T., Meleux, F., Mieville, A., Ohara, T., Raut, J.-C., Riahi, K., Schultz, M., Smith, S., Thompson, A., Aardenne, J., Werf, G., and Vuuren, D.: Evolution of anthropogenic and biomass burning emissions of air pollutants at global and regional scales during the 1980–2010 period, Clim. Change, 109, 163–190, 2011. a
Grewe, V.: The origin of ozone, Atmos. Chem. Phys., 6, 1495–1511, https://doi.org/10.5194/acp-6-1495-2006, 2006. a
Grewe, V.: A generalized tagging method, Geosci. Model Dev., 6, 247–253, https://doi.org/10.5194/gmd-6-247-2013, 2013. a
Grewe, V., Tsati, E., Mertens, M., Frömming, C., and Jöckel, P.: Contribution of emissions to concentrations: the TAGGING 1.0 submodel based on the Modular Earth Submodel System (MESSy 2.52), Geosci. Model Dev., 10, 2615–2633, https://doi.org/10.5194/gmd-10-2615-2017, 2017. a, b, c, d, e, f
Guenther, A., Hewitt, C., E., D., Fall, R. G., C., Graedel, T., Harley, P., Klinger, L., Lerdau, M., McKay, W., Pierce, T., S., B., Steinbrecher, R., Tallamraju, R., Taylor, J., and Zimmermann, P.: A global model of natural volatile organic compound emissions, J. Geophys. Res., 100, 8873–8892, 1995. a
Hendricks, J., Righi, M., Dahlmann, K., Gottschaldt, K.-D., Grewe, V., Ponater, M., Sausen, R., Heinrichs, D., Winkler, C., Wolfermann, A., Kampffmeyer, T., Friedrich, R., Klötzke, M., and Kugler, U.: Quantifying the climate impact of emissions from land-based transport in Germany, Transport Res. D-Tr. E., 65, 825–845, https://doi.org/10.1016/j.trd.2017.06.003, 2017. a, b
Hofmann, C., Kerkweg, A., Wernli, H., and Jöckel, P.: The 1-way on-line coupled atmospheric chemistry model system MECO(n) – Part 3: Meteorological evaluation of the on-line coupled system, Geosci. Model Dev., 5, 129–147, https://doi.org/10.5194/gmd-5-129-2012, 2012. a
Hofmann, C., Kerkweg, A., Hoor, P., and Jöckel, P.: Stratosphere-troposphere exchange in the vicinity of a tropopause fold, Atmos. Chem. Phys. Discuss., https://doi.org/10.5194/acp-2015-949, 2016. a
Holmes, C. D., Prather, M. J., and Vinken, G. C. M.: The climate impact of ship NOx emissions: an improved estimate accounting for plume chemistry, Atmos. Chem. Phys., 14, 6801–6812, https://doi.org/10.5194/acp-14-6801-2014, 2014. a
Hoor, P., Borken-Kleefeld, J., Caro, D., Dessens, O., Endresen, O., Gauss, M., Grewe, V., Hauglustaine, D., Isaksen, I. S. A., Jöckel, P., Lelieveld, J., Myhre, G., Meijer, E., Olivie, D., Prather, M., Schnadt Poberaj, C., Shine, K. P., Staehelin, J., Tang, Q., van Aardenne, J., van Velthoven, P., and Sausen, R.: The impact of traffic emissions on atmospheric ozone and OH: results from QUANTIFY, Atmos. Chem. Phys., 9, 3113–3136, https://doi.org/10.5194/acp-9-3113-2009, 2009. a, b
Huang, M., Carmichael, G. R., Pierce, R. B., Jo, D. S., Park, R. J., Flemming, J., Emmons, L. K., Bowman, K. W., Henze, D. K., Davila, Y., Sudo, K., Jonson, J. E., Tronstad Lund, M., Janssens-Maenhout, G., Dentener, F. J., Keating, T. J., Oetjen, H., and Payne, V. H.: Impact of intercontinental pollution transport on North American ozone air pollution: an HTAP phase 2 multi-model study, Atmos. Chem. Phys., 17, 5721–5750, https://doi.org/10.5194/acp-17-5721-2017, 2017. a
Jöckel, P., Sander, R., Kerkweg, A., Tost, H., and Lelieveld, J.: Technical Note: The Modular Earth Submodel System (MESSy) – a new approach towards Earth System Modeling, Atmos. Chem. Phys., 5, 433–444, https://doi.org/10.5194/acp-5-433-2005, 2005. a
Jöckel, P., Tost, H., Pozzer, A., Brühl, C., Buchholz, J., Ganzeveld, L., Hoor, P., Kerkweg, A., Lawrence, M. G., Sander, R., Steil, B., Stiller, G., Tanarhte, M., Taraborrelli, D., van Aardenne, J., and Lelieveld, J.: The atmospheric chemistry general circulation model ECHAM5/MESSy1: consistent simulation of ozone from the surface to the mesosphere, Atmos. Chem. Phys., 6, 5067–5104, https://doi.org/10.5194/acp-6-5067-2006, 2006. a, b, c, d, e, f, g
Jöckel, P., Kerkweg, A., Pozzer, A., Sander, R., Tost, H., Riede, H., Baumgaertner, A., Gromov, S., and Kern, B.: Development cycle 2 of the Modular Earth Submodel System (MESSy2), Geosci. Model Dev., 3, 717–752, https://doi.org/10.5194/gmd-3-717-2010, 2010. a, b, c, d, e, f, g
Jöckel, P., Tost, H., Pozzer, A., Kunze, M., Kirner, O., Brenninkmeijer, C. A. M., Brinkop, S., Cai, D. S., Dyroff, C., Eckstein, J., Frank, F., Garny, H., Gottschaldt, K.-D., Graf, P., Grewe, V., Kerkweg, A., Kern, B., Matthes, S., Mertens, M., Meul, S., Neumaier, M., Nützel, M., Oberländer-Hayn, S., Ruhnke, R., Runde, T., Sander, R., Scharffe, D., and Zahn, A.: Earth System Chemistry integrated Modelling (ESCiMo) with the Modular Earth Submodel System (MESSy) version 2.51, Geosci. Model Dev., 9, 1153–1200, https://doi.org/10.5194/gmd-9-1153-2016, 2016. a, b, c
Karamchandani, P., Long, Y., Pirovano, G., Balzarini, A., and Yarwood, G.: Source-sector contributions to European ozone and fine PM in 2010 using AQMEII modeling data, Atmos. Chem. Phys., 17, 5643–5664, https://doi.org/10.5194/acp-17-5643-2017, 2017. a
Kerkweg, A. and Jöckel, P.: The 1-way on-line coupled atmospheric chemistry model system MECO(n) – Part 1: Description of the limited-area atmospheric chemistry model COSMO/MESSy, Geosci. Model Dev., 5, 87–110, https://doi.org/10.5194/gmd-5-87-2012, 2012a. a, b
Kerkweg, A. and Jöckel, P.: The 1-way on-line coupled atmospheric chemistry model system MECO(n) – Part 2: On-line coupling with the Multi-Model-Driver (MMD), Geosci. Model Dev., 5, 111–128, https://doi.org/10.5194/gmd-5-111-2012, 2012b. a, b, c, d, e
Kerkweg, A., Buchholz, J., Ganzeveld, L., Pozzer, A., Tost, H., and Jöckel, P.: Technical Note: An implementation of the dry removal processes DRY DEPosition and SEDImentation in the Modular Earth Submodel System (MESSy), Atmos. Chem. Phys., 6, 4617–4632, https://doi.org/10.5194/acp-6-4617-2006, 2006a. a, b
Kerkweg, A., Sander, R., Tost, H., and Jöckel, P.: Technical note: Implementation of prescribed (OFFLEM), calculated (ONLEM), and pseudo-emissions (TNUDGE) of chemical species in the Modular Earth Submodel System (MESSy), Atmos. Chem. Phys., 6, 3603–3609, https://doi.org/10.5194/acp-6-3603-2006, 2006b. a, b, c, d
Kerkweg, A., Hofmann, C., Jöckel, P., Mertens, M., and Pante, G.: The on-line coupled atmospheric chemistry model system MECO(n) – Part 5: Expanding the Multi-Model-Driver (MMD v2.0) for 2-way data exchange including data interpolation via GRID (v1.0), Geosci. Model Dev., 11, 1059–1076, https://doi.org/10.5194/gmd-11-1059-2018, 2018. a, b, c
Knote, C., Brunner, D., Vogel, H., Allan, J., Asmi, A., Äijälä, M., Carbone, S., van der Gon, H. D., Jimenez, J. L., Kiendler-Scharr, A., Mohr, C., Poulain, L., Prévôt, A. S. H., Swietlicki, E., and Vogel, B.: Towards an online-coupled chemistry-climate model: evaluation of trace gases and aerosols in COSMO-ART, Geosci. Model Dev., 4, 1077–1102, https://doi.org/10.5194/gmd-4-1077-2011, 2011. a
Kwok, R. H. F., Baker, K. R., Napelenok, S. L., and Tonnesen, G. S.: Photochemical grid model implementation and application of VOC, NOx, and O3 source apportionment, Geosci. Model Dev., 8, 99–114, https://doi.org/10.5194/gmd-8-99-2015, 2015. a, b
Landgraf, J. and Crutzen, P. J.: An efficient method for online calculations of photolysis and heating rates., J. Atmos. Sci., 55, 863–878, https://doi.org/10.1175/1520-0469, 1998. a
Lefohn, A. S., Emery, C., Shadwick, D., Wernli, H., Jung, J., and Oltmans, S. J.: Estimates of background surface ozone concentrations in the United States based on model-derived source apportionment, Atmos. Environ., 84, 275–288, https://doi.org/10.1016/j.atmosenv.2013.11.033, 2014. a
Lelieveld, J. and Dentener, F. J.: What controls tropospheric ozone?, J. Geophys. Res.-Atmos., 105, 3531–3551, https://doi.org/10.1029/1999JD901011, 2000. a
Li, Y., Lau, A. K.-H., Fung, J. C.-H., Zheng, J. Y., Zhong, L. J., and Louie, P. K. K.: Ozone source apportionment (OSAT) to differentiate local regional and super-regional source contributions in the Pearl River Delta region, China, J. Geophys. Res.-Atmos., 117, D15305, https://doi.org/10.1029/2011JD017340, 2012. a, b
Lin, M., Fiore, A. M., Cooper, O. R., Horowitz, L. W., Langford, A. O., Levy, H., Johnson, B. J., Naik, V., Oltmans, S. J., and Senff, C. J.: Springtime high surface ozone events over the western United States: Quantifying the role of stratospheric intrusions, J. Geophys. Res.-Atmos., 117, D00V22, https://doi.org/10.1029/2012jd018151, 2012. a
Markakis, K., Valari, M., Perrussel, O., Sanchez, O., and Honore, C.: Climate-forced air-quality modeling at the urban scale: sensitivity to model resolution, emissions and meteorology, Atmos. Chem. Phys., 15, 7703–7723, https://doi.org/10.5194/acp-15-7703-2015, 2015. a, b
Matthes, S., Grewe, V., Sausen, R., and Roelofs, G.-J.: Global impact of road traffic emissions on tropospheric ozone, Atmos. Chem. Phys., 7, 1707–1718, https://doi.org/10.5194/acp-7-1707-2007, 2007. a, b
Matthias, V., Aulinger, A., Backes, A., Bieser, J., Geyer, B., Quante, M., and Zeretzke, M.: The impact of shipping emissions on air pollution in the greater North Sea region – Part 2: Scenarios for 2030, Atmos. Chem. Phys., 16, 759–776, https://doi.org/10.5194/acp-16-759-2016, 2016. a
Mertens, M., Kerkweg, A., Jöckel, P., Tost, H., and Hofmann, C.: The 1-way on-line coupled model system MECO(n) – Part 4: Chemical evaluation (based on MESSy v2.52), Geosci. Model Dev., 9, 3545–3567, https://doi.org/10.5194/gmd-9-3545-2016, 2016. a, b, c, d, e, f, g, h, i, j, k, l, m
Mertens, M., Grewe, V., Rieger, V. S., and Jöckel, P.: Revisiting the contribution of land transport and shipping emissions to tropospheric ozone, Atmos. Chem. Phys., 18, 5567–5588, https://doi.org/10.5194/acp-18-5567-2018, 2018. a
Mertens, M., Kerkweg, A., Grewe, V., Jöckel, P., and Sausen, R.: Attributing land transport emissions to ozone and ozone precursors in Europe and Germany, Atmos. Chem. Phys. Discuss., https://doi.org/10.5194/acp-2019-715, in review, 2019. a
Mertens, M. B.: Contribution of road traffic emissions to tropospheric ozone in Europe and Germany, available at: http://nbn-resolving.de/urn:nbn:de:bvb:19-207288 (last access: 20 January 2020), 2017. a
NCL: The NCAR Command Language (Version 6.4.0) [Software], Boulder, Colorado, UCAR/NCAR/CISL/TDD, https://doi.org/10.5065/D6WD3XH5, 2017. a
Pöschl, U., von Kuhlmann, R., Poisson, N., and Crutzen, P.: Development and Intercomparison of Condensed Isoprene Oxidation Mechanisms for Global Atmospheric Modeling, J. Atmos. Chem., 37, 29–152, https://doi.org/10.1023/A:1006391009798, 2000. a
Pozzer, A., Jöckel, P., Sander, R., Williams, J., Ganzeveld, L., and Lelieveld, J.: Technical Note: The MESSy-submodel AIRSEA calculating the air-sea exchange of chemical species, Atmos. Chem. Phys., 6, 5435–5444, https://doi.org/10.5194/acp-6-5435-2006, 2006. a
Price, C. and Rind, D.: A simple lightning parameterization for calculating global lightning distributions, J. Geophys. Res.-Atmos., 97, 9919–9933, https://doi.org/10.1029/92JD00719, 1992. a
Righi, M., Eyring, V., Gottschaldt, K.-D., Klinger, C., Frank, F., Jöckel, P., and Cionni, I.: Quantitative evaluation of ozone and selected climate parameters in a set of EMAC simulations, Geosci. Model Dev., 8, 733–768, https://doi.org/10.5194/gmd-8-733-2015, 2015. a
Rockel, B., Will, A., and Hense, A.: The Regional Climate Model COSMO-CLM (CCLM), Meteorol. Z., 17, 347–348, 2008. a
Roeckner, E., Bäuml, G., Bonaventura, L., Brokopf, R., Esch, M., Giorgetta, M., Hagemann, S., Kirchner, I., Kornblueh, L., Manzini, E., Rhodin, A., Schlese, U., Schulzweida, U., and Tompkins, A.: The atmospheric general circulation model ECHAM5. PART I: Model description, MPI-Report 349, Max Planck Institut für Meteorologie in Hamburg, Deutschland, available at: https://www.mpimet.mpg.de/fileadmin/publikationen/Reports/max_scirep_349.pdf (last access: 18 October 2015), 2003. a
Roeckner, E., Brokopf, R., Esch, M., Giorgetta, M., Hagemann, S., Kornblueh, L., Manzini, E., Schlese, U., and Schulzweida, U.: Sensitivity of Simulated Climate to Horizontal and Vertical Resolution in the ECHAM5 Atmosphere Model, J. Climate, 19, 3771–3791, https://doi.org/10.1175/jcli3824.1, 2006. a, b
Sander, R., Baumgaertner, A., Gromov, S., Harder, H., Jöckel, P., Kerkweg, A., Kubistin, D., Regelin, E., Riede, H., Sandu, A., Taraborrelli, D., Tost, H., and Xie, Z.-Q.: The atmospheric chemistry box model CAABA/MECCA-3.0, Geosci. Model Dev., 4, 373–380, https://doi.org/10.5194/gmd-4-373-2011, 2011. a, b
Stock, Z. S., Russo, M. R., Butler, T. M., Archibald, A. T., Lawrence, M. G., Telford, P. J., Abraham, N. L., and Pyle, J. A.: Modelling the impact of megacities on local, regional and global tropospheric ozone and the deposition of nitrogen species, Atmos. Chem. Phys., 13, 12215–12231, https://doi.org/10.5194/acp-13-12215-2013, 2013. a, b
Stock, Z. S., Russo, M. R., and Pyle, J. A.: Representing ozone extremes in European megacities: the importance of resolution in a global chemistry climate model, Atmos. Chem. Phys., 14, 3899–3912, https://doi.org/10.5194/acp-14-3899-2014, 2014. a
Tie, X., Brasseur, G., and Ying, Z.: Impact of model resolution on chemical ozone formation in Mexico City: application of the WRF-Chem model, Atmos. Chem. Phys., 10, 8983–8995, https://doi.org/10.5194/acp-10-8983-2010, 2010. a, b, c
Tørseth, K., Aas, W., Breivik, K., Fjæraa, A. M., Fiebig, M., Hjellbrekke, A. G., Lund Myhre, C., Solberg, S., and Yttri, K. E.: Introduction to the European Monitoring and Evaluation Programme (EMEP) and observed atmospheric composition change during 1972–2009, Atmos. Chem. Phys., 12, 5447–5481, https://doi.org/10.5194/acp-12-5447-2012, 2012. a
Tost, H., Jöckel, P., Kerkweg, A., Sander, R., and Lelieveld, J.: Technical note: A new comprehensive SCAVenging submodel for global atmospheric chemistry modelling, Atmos. Chem. Phys., 6, 565–574, https://doi.org/10.5194/acp-6-565-2006, 2006a. a, b
Tost, H., Jöckel, P., and Lelieveld, J.: Influence of different convection parameterisations in a GCM, Atmos. Chem. Phys., 6, 5475–5493, https://doi.org/10.5194/acp-6-5475-2006, 2006b. a
Tost, H., Jöckel, P., and Lelieveld, J.: Lightning and convection parameterisations – uncertainties in global modelling, Atmos. Chem. Phys., 7, 4553–4568, https://doi.org/10.5194/acp-7-4553-2007, 2007. a, b
Tost, H., Lawrence, M. G., Brühl, C., Jöckel, P., The GABRIEL Team, and The SCOUT-O3-DARWIN/ACTIVE Team: Uncertainties in atmospheric chemistry modelling due to convection parameterisations and subsequent scavenging, Atmos. Chem. Phys., 10, 1931–1951, https://doi.org/10.5194/acp-10-1931-2010, 2010. a, b
Travis, K. R. and Jacob, D. J.: Systematic bias in evaluating chemical transport models with maximum daily 8 h average (MDA8) surface ozone for air quality applications: a case study with GEOS-Chem v9.02, Geosci. Model Dev., 12, 3641–3648, https://doi.org/10.5194/gmd-12-3641-2019, 2019. a
Valverde, V., Pay, M. T., and Baldasano, J. M.: Ozone attributed to Madrid and Barcelona on-road transport emissions: Characterization of plume dynamics over the Iberian Peninsula, Sci. Total Environ., 543, 670–682, https://doi.org/10.1016/j.scitotenv.2015.11.070, 2016. a, b
Wang, Y., Jacob, D. J., and Logan, J. A.: Global simulation of tropospheric O3-NOx -hydrocarbon chemistry: 3. Origin of tropospheric ozone and effects of nonmethane hydrocarbons, J. Geophys. Res.-Atmos., 103, 10757–10767, https://doi.org/10.1029/98JD00156, 1998. a
Wild, O.: Modelling the global tropospheric ozone budget: exploring the variability in current models, Atmos. Chem. Phys., 7, 2643–2660, https://doi.org/10.5194/acp-7-2643-2007, 2007. a, b
Wild, O. and Prather, M. J.: Global tropospheric ozone modeling: Quantifying errors due to grid resolution, J. Geophys. Res.-Atmos., 111, D11305, https://doi.org/10.1029/2005JD006605, 2006. a
Yienger, J. J. and Levy, H.: Empirical model of global soil-biogenic NOx emissions, J. Geophys. Res.-Atmospheres, 100, 11447–11464, https://doi.org/10.1029/95JD00370, 1995. a
Young, P. J., Archibald, A. T., Bowman, K. W., Lamarque, J.-F., Naik, V., Stevenson, D. S., Tilmes, S., Voulgarakis, A., Wild, O., Bergmann, D., Cameron-Smith, P., Cionni, I., Collins, W. J., Dalsøren, S. B., Doherty, R. M., Eyring, V., Faluvegi, G., Horowitz, L. W., Josse, B., Lee, Y. H., MacKenzie, I. A., Nagashima, T., Plummer, D. A., Righi, M., Rumbold, S. T., Skeie, R. B., Shindell, D. T., Strode, S. A., Sudo, K., Szopa, S., and Zeng, G.: Pre-industrial to end 21st century projections of tropospheric ozone from the Atmospheric Chemistry and Climate Model Intercomparison Project (ACCMIP), Atmos. Chem. Phys., 13, 2063–2090, https://doi.org/10.5194/acp-13-2063-2013, 2013. a
Zhang, L., Jacob, D. J., Downey, N. V., Wood, D. A., Blewitt, D., Carouge, C. C., van Donkelaar, A., Jones, D. B., Murray, L. T., and Wang, Y.: Improved estimate of the policy-relevant background ozone in the United States using the GEOS-Chem global model with horizontal resolution over North America, Atmos. Environ., 45, 6769–6776, https://doi.org/10.1016/j.atmosenv.2011.07.054, 2011. a
- Abstract
- Introduction
- Model description and experimental set-up
- Model evaluation
- Contributors to ozone in Europe
- Discussion
- Summary and conclusions
- Appendix A: Definition of RMSE and MB
- Code and data availability
- Author contributions
- Competing interests
- Acknowledgements
- Financial support
- Review statement
- References
- Supplement
- Abstract
- Introduction
- Model description and experimental set-up
- Model evaluation
- Contributors to ozone in Europe
- Discussion
- Summary and conclusions
- Appendix A: Definition of RMSE and MB
- Code and data availability
- Author contributions
- Competing interests
- Acknowledgements
- Financial support
- Review statement
- References
- Supplement