the Creative Commons Attribution 4.0 License.
the Creative Commons Attribution 4.0 License.
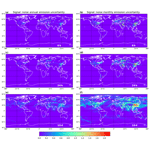
Representing model uncertainty for global atmospheric CO2 flux inversions using ECMWF-IFS-46R1
Joe R. McNorton
Nicolas Bousserez
Anna Agustí-Panareda
Gianpaolo Balsamo
Margarita Choulga
Andrew Dawson
Richard Engelen
Zak Kipling
Simon Lang
Atmospheric flux inversions use observations of atmospheric CO2 to provide anthropogenic and biogenic CO2 flux estimates at a range of spatio-temporal scales. Inversions require prior flux, a forward model and observation errors to estimate posterior fluxes and uncertainties. Here, we investigate the forward transport error and the associated biogenic feedback in an Earth system model (ESM) context. These errors can occur from uncertainty in the initial meteorology, the analysis fields used, or the advection schemes and physical parameterisation of the model. We also explore the spatio-temporal variability and flow-dependent error covariances. We then compare the error with the atmospheric response to uncertainty in the prior anthropogenic emissions. Although transport errors are variable, average total-column CO2 (XCO2) transport errors over anthropogenic emission hotspots (0.1–0.8 ppm) are comparable to, and often exceed, prior monthly anthropogenic flux uncertainties projected onto the same space (0.1–1.4 ppm). Average near-surface transport errors at three sites (Paris, Caltech and Tsukuba) range from 1.7 to 7.2 ppm. The global average XCO2 transport error standard deviation plateaus at ∼0.1 ppm after 2–3 d, after which atmospheric mixing significantly dampens the concentration gradients. Error correlations are found to be highly flow dependent, with XCO2 spatio-temporal correlation length scales ranging from 0 to 700 km and 0 to 260 min. Globally, the average model error caused by the biogenic response to atmospheric meteorological uncertainties is small (<0.01 ppm); however, this increases over high flux regions and is seasonally dependent (e.g. the Amazon; January and July: 0.24±0.18 ppm and 0.13±0.07 ppm). In general, flux hotspots are well-correlated with model transport errors. Our model error estimates, combined with the atmospheric response to anthropogenic flux uncertainty, are validated against three Total Carbon Observing Network (TCCON) XCO2 sites. Results indicate that our model and flux uncertainty account for 21 %–65 % of the total uncertainty. The remaining uncertainty originates from additional sources, such as observation, numerical and representation errors, as well as structural errors in the biogenic model. An underrepresentation of transport and flux uncertainties could also contribute to the remaining uncertainty. Our quantification of CO2 transport error can be used to help derive accurate posterior fluxes and error reductions in future inversion systems. The model uncertainty diagnosed here can be used with varying degrees of complexity and with different modelling techniques by the inversion community.
- Article
(4413 KB) - Full-text XML
-
Supplement
(714 KB) - BibTeX
- EndNote
Since 1750 global atmospheric CO2 concentrations have increased from 277 ppm (Joos and Spahni, 2008) to 2019 values of 410 ppm (Dlugokencky and Tans, 2019). The initial growth in CO2 was primarily caused by land use change and then subsequently by fossil fuel sources. The budget contribution from anthropogenic sources, along with existing ocean and biogenic fluxes, is difficult to disentangle at both short (days) and long (decades) timescales. For example, Le Quéré et al. (2018) found a 2008–2017 budget imbalance of 0.5 GtC yr−1 caused by uncertainties in fossil fuel emissions, land use change and the land–ocean sink.
Atmospheric inversions are often used to estimate both biogenic and anthropogenic CO2 fluxes at a range of spatial and temporal scales (e.g. Gurney et al., 2002; Peylin et al., 2013; Lauvaux et al., 2016). These inversions typically follow a Bayesian framework whereby prior information is used in an atmospheric transport model; those fluxes and uncertainties are then updated based on comparisons with atmospheric observations. Inversion intercomparison studies show that whilst model agreement is improving, large differences remain between different inversion systems (Peylin et al., 2013; Le Quéré et al., 2018; Gaubert et al., 2019). These are caused by a combination of differences in the prior information, transport model and observation networks used to constrain the fluxes.
Bayesian CO2 inversions require combined knowledge of the prior uncertainty, model transport uncertainty, measurement error and representation error to provide an accurate estimation of fluxes (e.g. Engelen et al., 2002). Neglecting these components of uncertainty imposes a hard constraint on the inversion, resulting in unreasonable solutions.
Prior fluxes are typically derived from bottom-up process models and observations. The uncertainty can, in part, be estimated by sampling the prior inventory probability distribution function (PDF), perturbing the meteorological data used to force the process models, using ancillary information on uncertainty estimates (e.g. national energy statistics) or a combination of these. Spatial and temporal prior flux error correlation structures can also be considered (e.g. Wu et al., 2013). The prior uncertainty is often only applied to the biogenic fluxes, with assumed perfect knowledge of the anthropogenic flux, although joint inversions of both biogenic and anthropogenic fluxes require consideration of uncertainties from both.
The observation uncertainty is independent, relatively small and well-known for in situ observations, and the application of this uncertainty to an inverse system is straightforward. For satellite observations, spatially coherent biases might influence uncertainties (Basu et al., 2018).
The representation error consists of two components. The first is the internal model component, which relates to the model inversion resolution being lower than that of the forward model (see Engelen et al., 2002, for more details). The second is the error that arises from spatio-temporal differences between the model and observations: for example, a point measurement compared to a model grid box average. This error is expected to be reduced as both the forward and inverse model resolution increase, and to an extent it can be quantified using multi-resolution models (see Agustí-Panareda et al., 2019, for more details).
Here, we investigate the forward transport error and the associated biogenic feedback in an Earth system model (ESM) context. Model transport error is usually larger than the observation error (Stephens et al., 2007; Law et al., 2008) and often consists of simplified assumptions. Depending on the configuration of the forward model, errors can occur from uncertainty in the initial meteorological conditions, the analysis fields used, or the advection schemes and physical parameterisation of the model.
Uncertainties in the physical parameterisation of land surface and planetary boundary layer schemes can cause errors in the mixed layer (ML) depth, which can lead to errors in the vertical mixing of CO2 (Sarrat et al., 2007; Díaz-Isaac et al., 2018). For CO2, the biogenic flux exchange at the surface correlates with changes in the ML depth, making the issue more complex (Denning et al., 1995). When performing inversions using surface observations, the accurate representation and consideration of any uncertainties in vertical mixing are especially important to avoid biases in estimated fluxes (Yi et al., 2004; Denning et al., 2008; Ahmadov et al., 2009). For aircraft and column observations the errors in the vertical mixing may become less important; for example, Verma et al. (2017) found that inverse flux estimates from aircraft profiles are not sensitive to errors in the ML depth. Similarly, satellite-based inversions, which retrieve total-column CO2 (XCO2), are expected to be less sensitive to vertical mixing errors. However, the issue of sensitivity becomes more complex in this case because the XCO2 signal is smaller than the ML signal (Basu et al., 2018). In addition to vertical mixing, advection errors associated with horizontal wind can result in errors up to 6 ppm (Lin and Gerbig, 2005).
CO2 inversions are performed using either an online model with a full physics scheme used to compute the meteorology or offline using analysis transport fields. Online inversions are computationally expensive, require access to a numerical weather prediction (NWP) system and, without the benefit of analysed transport fields, are limited by the accuracy of the physical forecast model. There is the added logistical challenge of reconciling the relatively short NWP assimilation window length (hours to days) with the typically longer CO2 window length (weeks to years). Typically, online systems have a higher temporal frequency than offline systems, which are limited by the output frequency of the archived analysis fields used. Vertical transport and other subgrid-scale processes, which are missing from the analysis, are computed by offline systems using schemes that are likely to be inconsistent with the original analysis, resulting in further errors (Engelen et al., 2002). Within an online ESM context, biogenic fluxes and surface parameter estimation can be integrated within the inversion system at a high temporal resolution. The advantages of an online inversion system for the attribution of model uncertainty are investigated here.
Ensembles of transport models are often used to quantify transport uncertainty (e.g. Gurney et al., 2002; Baker et al., 2006; Peylin et al., 2013; Basu et al., 2018). Whilst this represents the variability between models, systematic errors inherent within those models remain unaccounted for. For example, several models within an ensemble may use the same planetary boundary layer scheme, resulting in an unrealistic assumption of transport uncertainty. Ensembles using multiple schemes or resolutions may yield different inverse results (Gaubert et al., 2019), but this does not necessarily mean they provide an accurate representation of transport uncertainty. Alternatively, multi-physics ensembles with perturbed parameterisations provide a representation of transport uncertainty caused by parametric uncertainty during the simulation period (Kretschmer et al., 2012; Lauvaux and Davis, 2014; Díaz-Isaac et al., 2019). The stochastic representation of model uncertainty required for reliable ensemble forecasts has been thoroughly researched within the NWP community (e.g. Leutbecher et al., 2017). The ensemble approach may also consist of models which use forcing data taken from the same analysis product, leading to an underestimate in the uncertainty associated with the initial conditions and meteorological fields. A representation of uncertainties in initial meteorological conditions, boundary conditions (for regional models), forcing data and model physics is required to accurately evaluate transport uncertainty. Numerical uncertainty in models, including errors relating to interpolation, diffusion and advection, also contribute to transport uncertainty, although these are not investigated in this study. A complementary approach to quantify transport uncertainty is to perform direct comparisons with modelled and observed meteorological variables, as described by Lin and Gerbig (2005).
Here we use an NWP ensemble forecast system, initialised from an ensemble data assimilation (EDA) system, to investigate transport model uncertainty relating to both the uncertainty in the initial meteorological conditions and in the model physics. Furthermore, we explore the spatio-temporal variability and flow-dependent error covariances. We perform preliminary investigations into the biogenic fluxes associated with the meteorological uncertainty, resulting in a more complete model uncertainty. The biogenic feedbacks here do not account for parameterisation and mapping uncertainties. Finally, we investigate the signal-to-noise ratio for a prospective CO2 flux inversion system by comparing model uncertainties to the atmospheric response to anthropogenic emission uncertainties. The combined XCO2 error from model uncertainty and anthropogenic flux uncertainty is validated against Total Carbon Observing Network (TCCON) observations. If the model uncertainty is comparable to the model–observation error, as given by a control experiment, then it can be reasoned that the estimated model uncertainty is a relatively accurate estimation of the true model uncertainty. Other errors not accounted for, such as the representation error, would further increase this error towards the true model uncertainty.
We have used version 46R1 of the Integrated Forecasting System (IFS), operated and licensed by the European Centre for Medium-Range Weather Forecasts (ECMWF). A detailed scientific and technical description of the IFS can be found at https://www.ecmwf.int/en/forecasts/documentation/evolution-ifs/cycles/summary-cycle-46r1 (last access: 22 September 2019). The IFS primary use is in NWP, although extensions exist for atmospheric CO2 modelling. We used the Ensemble Prediction System (EPS) component of the Integrated Forecasting System (IFS), detailed in Leutbecher and Palmer (2008), to simulate 3-D atmospheric CO2 concentrations given a combination of prescribed and modelled surface fluxes. The EPS is configured to represent the uncertainty in both the initial meteorological conditions and the model formulation. The uncertainty in initial conditions was inherited from an operational EDA, wherein input observations were perturbed with stochastic noise based on a given observation error (Isaksen et al., 2010). In addition to this, both the EPS and EDA use a stochastically perturbed parameterisation tendencies (SPPT) scheme to represent errors caused by uncertainty in physical parameterisations, including subgrid-scale processes (Buizza et al., 1999; Leutbecher et al., 2017). Different from the operational configuration of the EPS we start the ensemble members directly from the EDA members instead of adding perturbations to the deterministic analysis. Furthermore, we do not apply singular vector perturbations to the initial conditions.
All simulations were performed globally for January and July 2015 with 137 vertical levels and at ∼25 km horizontal resolution (TCo399). Instantaneous 3-D model CO2 fields and biogenic fluxes calculated online by CTESSEL, the land surface component of the IFS (Boussetta et al., 2013; Agustí-Panareda et al., 2014, 2016), were output at hourly frequency. The uncertainty in each simulation is represented by the standard error of a 50-member ensemble; the sampling error resulting from the ensemble size is discussed in the following sections. The 3-D CO2 fields for all ensemble members were initialised using the ECMWF operational product, which is provided under the Copernicus Atmosphere Monitoring Service (Agustí-Panareda et al., 2019). Each month-long ensemble member is comprised of 24 h forecasts reinitialised from the operational EDA, with the 3-D CO2 field cycled from the last time step of the previous forecast. As a result, on the first day of the month the ensemble does not include a representation of the initial atmospheric 3-D CO2 uncertainty; however, the error in initial CO2 concentrations for each forecast is established within the ensemble after a few days. To account for this the first 2 d are discarded from all monthly values provided.
Multiple experiments were performed to identify specific contributions to the total ESM uncertainty. Perturbing the initial conditions, model physics and the meteorologically dependent biogenic flux provides a representation of model uncertainty; hereafter, this simulation is referred to as FME. Individually, the uncertainties associated with the initial conditions (IME), the model physics (PME) and the biogenic response to uncertainty in meteorological forcing (BME) were investigated by performing ensemble simulations in which only the target component was perturbed. It is important to note that the biogenic uncertainty shown here only represents the biogenic feedback to uncertainties in meteorology and not the mapping or process uncertainty inherent within the model. A simulation in which both the initial meteorological conditions and model physics were perturbed (TME) represents the transport model uncertainty by using offline biogenic emissions from a control experiment. Hereafter, transport model uncertainty is defined as the uncertainty associated with the initial conditions and model physics during the integration, which is typically simplified in inverse modelling studies, and model uncertainty includes uncertainty in biogenic fluxes associated with meteorological uncertainty. The biogenic response to errors in the forcing is estimated using the member-specific biogenic fluxes from TME as offline fluxes in BME.
Offline biogenic emissions were broadly consistent with online biogenic emissions in that they were generated using CTESSEL; the only difference is in the frequency. The online biogenic emissions were applied at model time step frequency (20 min), whereas the offline biogenic emissions were input at 3 h intervals and interpolated across each time step. Unless otherwise stated offline biogenic emissions were generated using a control forecast. Offline monthly anthropogenic emissions were generated using EDGAR v4.3.2 (Janssens-Maenhout et al., 2019), extended to 2015 with monthly scaling factors derived from 2010. These were regridded to the model grid from a native resolution. Daily mean fire emissions were also regridded from resolution, taken from the Global Fire Assimilation System (GFAS; Kaiser et al., 2012). Monthly mean ocean fluxes were taken from Jena CarboScope v1.6 based on the SOCAT data set of pCO2 observations (Rödenbeck et al., 2014). The uncertainties in fire and ocean fluxes are not considered here.
The forward model component of an ensemble-based CO2 flux inversion provides an estimated PDF of atmospheric CO2 based on a signal (prior emission uncertainty) and noise (model uncertainty). To investigate the signal-to-noise ratio relevant for anthropogenic CO2 inversions, additional simulations were performed using estimated anthropogenic emission uncertainties and are described alongside all other experiment configurations in Table 1 (EXP, PEM and PEA). These estimates are calculated per sector and per country following the error propagation method outlined by the IPCC guidelines (IPCC, 2006) and are based upon uncertainties in emission factors and activity data. The statistical infrastructure development level of the country is also considered, defining all countries as either statistically well-developed or less developed. The most commonly used fuel type is considered for uncertainty calculations when multiple types are used. Uncertainties are assumed to be uncorrelated in time and between sectors and countries. Further details will be discussed in detail in a follow-up paper (Choulga et al., 2020). Anthropogenic emissions were grouped into six sectors: large power plants, the remaining energy sector, manufacturing, transport, settlements and other. National uncertainties for annual and monthly emissions are strongly sector and country dependent, ranging from annual transport uncertainties of ∼4 % for numerous developed nations to monthly other sector uncertainties of ∼330 % for the Democratic Republic of the Congo. Aviation emissions were used as 3-D profiles but remained unperturbed in these simulations.
The uncertainties used here are thought to be relatively modest considering the timescales being investigated. Data availability for several aspects of anthropogenic uncertainties currently limits our ability to diagnose a reasonable atmospheric XCO2 response signal at short timescales. For example, daily uncertainties, which would be required for high-temporal-frequency flux inversions, are expected to be considerably larger than monthly uncertainties. This would provide, in principle, a larger signal. Additionally, a lack of prior information prevented the consideration of uncertainty correlations in prior fluxes. Finally, the diurnal variability in emissions, which is likely to influence the modelled atmospheric response to anthropogenic emissions, is not considered. The missing information in prior uncertainties of anthropogenic fluxes leads to an underestimation of the flux signal and as a result the signal-to-noise ratio.
We used atmospheric XCO2 measurements from the Total Carbon Column Observing Network (TCCON) (Wunch et al., 2011) to evaluate the combined forward model error and the atmospheric response to anthropogenic flux uncertainties. Assuming the 50-member ensemble accurately represents the atmospheric CO2 PDF accounting for all uncertainties, the standard error in EXP should be comparable to the model–observation error. However, the total error is expected to underrepresent the model–observation error because some uncertainties were either missing or underestimated by the ensemble. For example, the representation error is not present in our ensemble, and the prior anthropogenic flux uncertainty is based on monthly estimates and not weekly or daily values.
Here, we focus on model uncertainty relative to prior anthropogenic flux uncertainty. Therefore, three TCCON sites with nearby anthropogenic sources and with available data for 2015 were selected for evaluation: Paris (Té et al., 2014), Caltech (Wennberg et al., 2015) located near Los Angeles and Tsukuba (Morino et al., 2018) near Tokyo. Sounding-specific TCCON averaging kernels were applied to interpolated model output for direct model–observation comparisons.
4.1 TCCON site-specific error representation
All results shown are taken from the January 2015 simulations; results from the July simulations, although discussed here, are shown in the Supplement. The relative contribution to total XCO2 variability from the uncertainties in initial meteorological conditions, model physics and biogenic feedback, as well as the atmospheric response to prior anthropogenic uncertainty, is shown to be location and time dependent (Fig. 1 and Fig. S1 in the Supplement; for illustration purposes only the first 5 d are shown). After 2–3 d the total error stabilises, caused by the impact of atmospheric diffusion (Figs. 2 and S2). All monthly averages are calculated after an initial 2 d spin-up, omitting the first and second of the month.
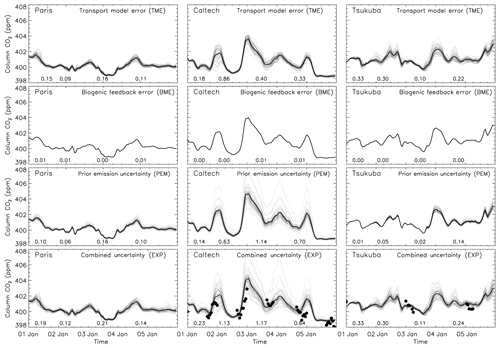
Figure 1IFS model XCO2 (ppm) variability over three TCCON sites for the 50-member ensemble for 1–5 January 2015 from uncertainties in model transport (first row), biogenic feedback from meteorological uncertainty (second row), monthly uncertainties in anthropogenic emissions (third row) and a combination of all uncertainties (fourth row). Individual ensemble members are shown with grey lines, and the ensemble mean is the black line. TCCON observations, when available, are shown for 5 d (black circles). Values denote standard error after 12, 24, 48 and 96 h.
Over Paris the initial meteorology (IME) and model physics (PME) errors are the largest individual components of the total XCO2 variability for January (Table 2). The combined average transport error (TME) increases further, with a January maximum of 0.61 ppm. The biogenic feedback (BME) errors are small. The average atmospheric XCO2 variation associated with annual anthropogenic flux uncertainties (PEA) is relatively small; however, using monthly uncertainties (PEM) this variability increases slightly, but this is still below the derived transport error.
Average initial meteorological error and model physics error also dominate the total error over Tsukuba in January, with a combined average transport error reaching a maximum of 1.01 ppm. The biogenic feedback errors are again smaller in comparison. Monthly and annual anthropogenic emission uncertainties over Tsukuba consistently produce smaller errors than the total transport error.
Over Caltech the January average variability in the atmospheric response to annual anthropogenic emission uncertainties is lower than that from the initial meteorological error, the model physics error and the combined transport error (maximum value of 2.55 ppm). Conversely, the monthly anthropogenic uncertainties produce the largest average error in atmospheric XCO2. The average biogenic feedback error is once again small. For small periods the PEM standard error exceeds the EXP standard error over Caltech; this is thought to either be due to spurious noise generated by the small ensemble size or a compensating reduction in total error caused by other sources of errors (transport and biogenic).
Variability between the three sites is a result of multiple factors including nearby fluxes, regional atmospheric transport and orography. The minor impact of the biogenic feedback, caused by meteorological uncertainty, results in the FME and TME errors being almost identical at all three sites.
Table 2The average IFS model XCO2 (ppm) standard error across the 50-member ensemble over three TCCON sites for seven different model configurations for January 2015.
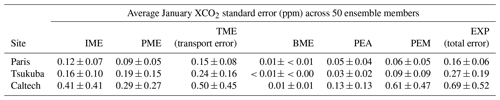
July simulations show comparable model transport errors to January over Paris (0.15±0.06), decreases over Caltech (0.23±0.10 ppm) and increases over Tsukuba (0.38±0.23 ppm), showing site-specific seasonal variability (Figs. S1 and S2). The biogenic feedback error increased over all three sites in July (Paris: 0.02±0.01 ppm, Caltech: 0.02±001 ppm, Tsukuba: 0.04±0.03 ppm) due to Northern Hemisphere summer. This remains smaller than the transport and anthropogenic uncertainty response but is no longer negligible. The July spread in the atmospheric response to monthly anthropogenic flux uncertainties is increased over Paris (0.08±0.05 ppm) and Tsukuba (0.38±0.2 ppm) when compared to January. Over Caltech (0.47±0.19 ppm) the same error is reduced for July.
There is no clear diurnal cycle in the column transport error at any of the three stations; January midnight averages at Paris (0.15±0.08 ppm), Caltech (0.48±0.47 ppm) and Tsukuba (0.29±0.18 ppm) are all comparable to midday averages (0.15±0.07 ppm, 0.46±0.32 ppm and 0.25±0.18 ppm, respectively). For July, only Caltech exhibits a slight diurnal cycle, with midday averages of 0.29±0.13 ppm and midnight averages of 0.18±0.05 ppm. Over Caltech in July, a diurnal cycle is found in the atmospheric XCO2 error as a response to both the biogenic feedback uncertainty and anthropogenic flux uncertainty, with midday averages of 0.02±0.01 ppm and 0.73±0.30 ppm, respectively, and midnight averages of 0.01±0.01 ppm and 0.43±0.18 ppm. Without a diurnal cycle in anthropogenic fluxes, this would suggest that the diurnal meteorological variability causes the observed difference in model error as the magnitude in prior flux and error remains the same for both night and day. Summertime diurnal variability over Caltech has previously been attributed to the sea–mountain breeze, whereby CO2-enhanced air masses peak in the afternoon before being reduced again in the evening (Agustí-Panareda et al., 2019). These enhancements cause an increase in atmospheric CO2 gradients, resulting in an increased transport error. Diurnal variability in emissions is expected to increase the diurnal signal in the atmospheric transport error, with typically lower night-time emissions resulting in lower transport model errors; however, we have not tested this hypothesis here.
Flux inversions, more specifically posterior error reductions, depend on the signal-to-noise ratio, wherein the atmospheric response to prior flux uncertainty is the signal and the remaining errors represent the noise. As previously mentioned, we underestimate the noise here by only accounting for some model uncertainty. Using annual anthropogenic uncertainties to perturb January fluxes generates an average signal-to-noise ratio, after a 2 d spin-up, of 0.38±0.37, 0.27±0.16 and 0.20±0.17 at Paris, Caltech and Tsukuba, respectively (Fig. 2). Over Caltech and Tsukuba, the ratio does not exceed 1 for the whole of January and only exceeds 1 % for 8 % of the month over Paris. Using monthly anthropogenic uncertainties, the signal-to-noise ratio over Paris and Tsukuba after a 2 d spin-up increases to an average of 0.54±0.37 and 0.36±0.21, exceeding 1 % for 9 % and 1 % of the month, respectively. Over Caltech this increases to a ratio of 1.02±0.68, exceeding 1 % for 44 % of the month. The average signal-to-noise ratio, when using monthly uncertainties, increases at all three sites in July to 0.61±0.42 ppm over Paris, 2.48±0.93 ppm over Caltech and 0.94±0.48 ppm over Tsukuba (S2). The ratio exceeds 1 % for 11 % of the month over Paris, >99 % over Caltech and 38 % over Tsukuba. It is reasonable to assume that the uncertainties, and therefore the signal-to-noise ratio, will increase by a similar order of magnitude from monthly to daily uncertainties as the increase seen here from annual to monthly uncertainties; however, no data are currently available for daily anthropogenic flux uncertainties.
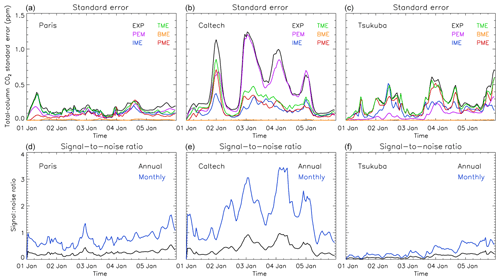
Figure 2IFS model XCO2 (ppm) standard error across the 50-member ensemble over three TCCON sites for seven different model configurations (a, b, c). The XCO2 signal generated by uncertainties in anthropogenic emissions divided by the noise from remaining model error over the same TCCON sites (d, e, f). For acronym definitions, see Table 1.
To evaluate the accuracy of the total error in XCO2 (model uncertainty and atmospheric response to anthropogenic flux uncertainty) the standard error across ensemble members is compared to the control model–observation error from TCCON (Figs. 1 and S1). For January, the mean centred model–observation errors are found to be 1.41 ppm at Caltech and 0.54 ppm at Tsukuba compared to EXP total model uncertainties (transport, biogenic feedback and monthly anthropogenic emission uncertainty) of 0.69±0.52 ppm and 0.27±0.19 ppm, respectively. There are no available TCCON data over Paris for January 2015, and the EXP uncertainty over Paris is 0.16±0.06 ppm. For July, the model–observation errors are 0.92, 0.90 and 1.84 ppm for Paris, Caltech and Tsukuba, respectively, compared to EXP uncertainties of 0.19±0.07 ppm, 0.60±0.23 ppm and 0.56±0.31 ppm. This would suggest that, depending on the time and location, the uncertainties explored here account for 21 %–65 % of the total model uncertainty. As previously mentioned, the monthly uncertainty estimates used here are an underestimation of the uncertainties at the short timescales being investigated here (hourly or daily). It should also be noted that additional sources of model–observation variability, such as observation errors, representation error, numerical errors and biogenic flux errors relating to both processes and mapping, are not considered in these values. Our results show that these additional uncertainties are not negligible and need to be accounted for in addition to the uncertainties derived here.
The vertical error structure for each ensemble configuration at the three TCCON sites over a 24 h period shows column variability (Fig. 3). For all three sites individual errors are typically largest near the surface, where the CO2 gradients are the largest. Both components of the transport error are noticeable in the mid-troposphere, with some model levels exceeding 1 ppm errors for both initial meteorological and model physics errors individually at all sites. On average, the near-surface (∼100 m) transport error over Paris is 1.7±2.7 ppm, with a maximum of 17.6 ppm. Over Caltech noticeable transport errors are typically found in the lower troposphere. The average near-surface error is 7.2±6.2 ppm, with a maximum of 21.8 ppm. Over Tsukuba the initial meteorological condition error is detectable not only near the surface but also in the middle to upper troposphere (∼300 hPa), with averages of 0.41±0.21 ppm. Near-surface average transport errors are 2.2±2.8 ppm, with a maximum of 16.6 ppm.
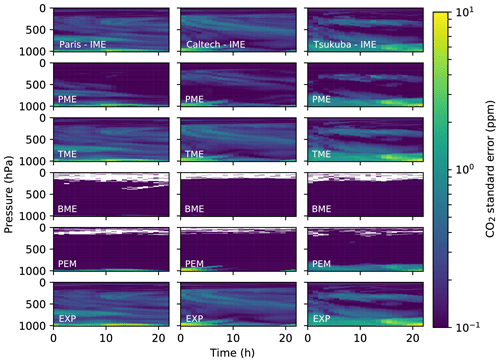
Figure 3Standard error of IFS model CO2 profiles (ppm) across the 50-member ensemble for 5 January 2015 over three TCCON sites. Ensemble configurations consist of perturbed initial meteorological conditions (top row), perturbed model physics (second row), both perturbed initial conditions and physics (third row), perturbed biogenic emissions caused by transport uncertainty (fourth row), perturbed emissions using monthly anthropogenic uncertainties per sector and country (fifth row), perturbations of the combined transport, biogenic feedback, and anthropogenic emission uncertainties (bottom row). Note that the colour scale is logarithmic.
For the near surface, which is typically used for in situ based inversions, average signal-to-noise ratios for monthly anthropogenic uncertainties are 1.4±0.5, 0.8±0.7 and 0.4±0.2 over Paris, Caltech and Tsukuba, respectively. The ratio exceeds 11 % for 78 % of the time over Paris but less frequently over Caltech (36 %) and Tsukuba (0 %).
All three sites do not exhibit a diurnal cycle in the near-surface transport error. For each site the difference in error between day and night is less than 10 %. This assumes that the EDA and SPPT accurately represent transport error by perturbing the boundary layer physics. These results underestimate the diurnal cycle in the transport error by not accounting for diurnal variability in emissions.
4.2 Global and regional model uncertainty
The global XCO2 uncertainty resulting from uncertainties in emissions, biogenic feedback and transport, which includes both initial conditions and physics, is found to be spatially and temporally varying (e.g. January 2015 shown by Fig. 4). As expected, the atmospheric XCO2 signal from monthly anthropogenic emission uncertainties is largest over emission hotspots in eastern China, with smaller signals over North America, Europe and the Middle East (Tables 3 and 4). The global average error for both January and July 2015 is relatively small at 0.01±0.00 ppm, although the error values are heterogenous, with maximum local instantaneous XCO2 errors reaching 9.2 ppm. The error is expected to increase further with uncertainties applied at the hourly or daily timescale, as these currently unavailable values would be larger than both monthly and annual uncertainties.
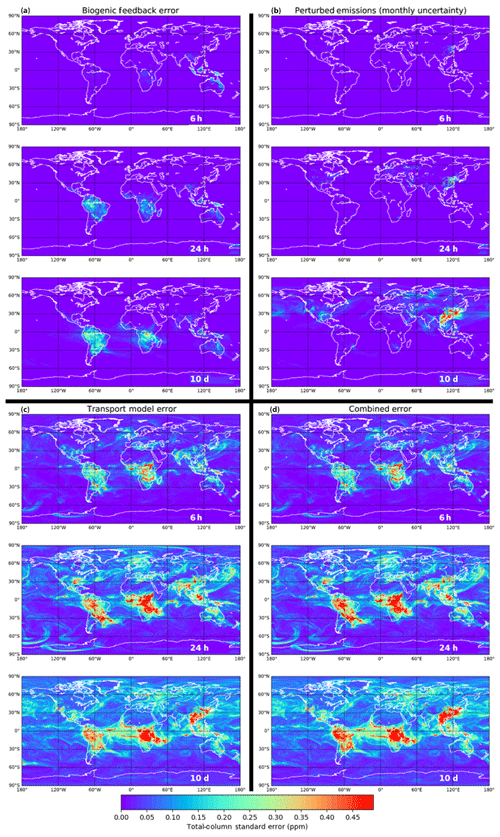
Figure 4Global standard error of IFS model XCO2 (ppm) across the 50-member ensemble after 6 h, 24 h and 10 d. Errors shown are from uncertainties in biogenic emissions caused by meteorological uncertainty (a), monthly anthropogenic emission uncertainties per sector and country (b), model transport uncertainty (c), and a combination of all uncertainties (d).
The XCO2 biogenic feedback error from atmospheric model uncertainty is largest over regions with a high net ecosystem exchange, e.g. the Amazon; (January: 0.16±0.08 ppm, July: 0.06±0.06 ppm) and southern Africa (January: 0.13±0.07 ppm, July: 0.05±0.07 ppm). These are also areas with large atmospheric gradients. The high values in Southern Hemisphere summer suggest a seasonal cycle in the biogenic feedback error. Globally, the average biogenic feedback error is smaller (<0.01 ppm) in January and increases slightly in July (0.02±0.00 ppm), following the seasonal dependence of biogenic fluxes.
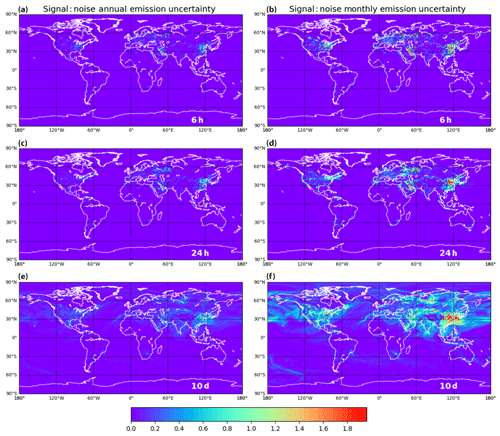
Figure 5Global signal-to-noise ratio of IFS model XCO2 across the 50-member ensemble after 6 h (a, b), 24 h (c, d) and 10 d (e, f). The signal is the atmospheric response to annual (a, c, e) and monthly (b, d, f) anthropogenic emission uncertainty, and the noise is the transport and biogenic feedback error.
The error in atmospheric XCO2 caused by transport model uncertainties correlates with the error caused by both the anthropogenic uncertainties and biogenic feedback uncertainties, as these are the regions with the largest fluxes and, as a result, the largest gradients. The globally averaged XCO2 error resulting from the initial model error, model physics error and combined transport error is 0.06±0.00 ppm, 0.09±0.00 ppm and 0.10±0.01 ppm, respectively. Over regions with a high biogenic flux the average transport error further increases, e.g. the Amazon (January: 0.24±0.18 ppm, July: 0.20±0.15 ppm) and southern Africa (January: 0.30±0.26 ppm, July: 0.18±0.21 ppm). The transport error in these regions exhibits a similar seasonal cycle to the biogenic feedback error, most likely caused by the increased flux in Southern Hemisphere summer. The increase in transport error is also evident over regions with a high anthropogenic flux (Tables 3 and 4). The average transport model error over these hotspots is similar in July (0.32±0.17 ppm) and January (0.32±0.22 ppm). Considering most of the sites are in the Northern Hemisphere this would suggest there is little or no seasonal variability in the average transport error over anthropogenic hotspots, although certain hotspots show some seasonal variability (e.g. Los Angeles). The maximum transport error for all times and locations is 9.2 ppm, although globally for individual grid cells and times the error only exceeds 0.5 ppm for ∼1 % of the time.
The signal-to-noise ratio using monthly and annual anthropogenic uncertainties is location and time dependent, shown in Fig. 5 and for various emission hotspots in Tables 3 and 4. After the initial 2–3 d this ratio is typically below 1 when using prior annual anthropogenic uncertainties, with exceptions over eastern Asia and the Middle East. For prior monthly uncertainties, large parts of North America, Europe, Asia and some Southern Hemisphere hotspots consistently exceed 1. Further work is required to investigate more robust daily, or even hourly, uncertainty estimates for each sector, which is relevant for posterior error reductions at high temporal frequencies. The increased uncertainty in fluxes at higher temporal resolution will result in a more accurate total error, increasing the signal-to-noise ratio and resulting in increased posterior error reductions.
Table 3Average, minimum and maximum total-column model CO2 error statistics for the transport model error and the atmospheric response to monthly emission uncertainties (signal), as well as the signal-to-noise ratio for various emission hotspots for January 2015. Results are calculated from the 50-member IFS ensemble.
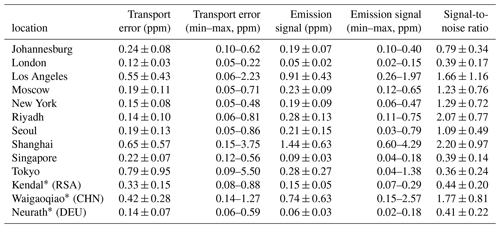
∗ Denotes large power stations.
Table 4Average, minimum and maximum total-column model CO2 error statistics for the transport model error and the atmospheric response to monthly emission uncertainties (signal), as well as the signal-to-noise ratio for various emission hotspots for July 2015. Results are calculated from the 50-member IFS ensemble.
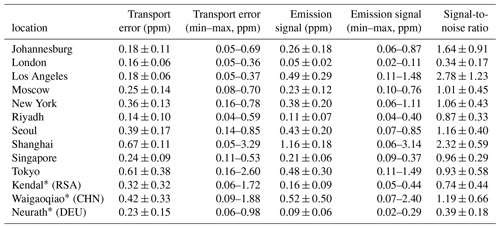
∗ Denotes large power stations.
4.3 Impact of ensemble size
After 2–3 d the global average transport model error reaches a steady state at which the model error growth balances with the atmospheric mixing caused by CO2 gradients (Fig. 6). Afterwards, the global transport model error remains approximately 0.1 ppm for all ensemble sizes. Globally, as ensemble size tends toward 50, the error across all ensemble members converges.
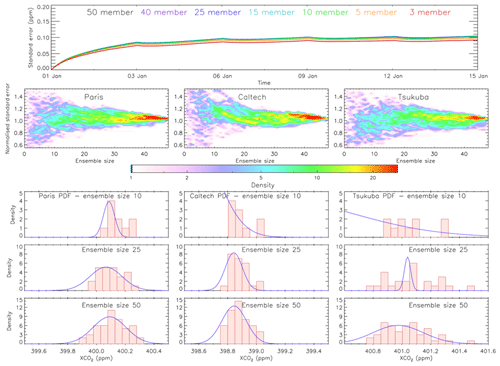
Figure 6Global average XCO2 (ppm) standard error from the IFS model over 15 d for a 3- (red), 5- (orange), 10- (green), 15- (turquoise), 25- (blue), 40- (purple) and 50-member (black) ensemble (top). A binned density plot of the change in normalised error relative to the 50-member ensemble with respect to ensemble size (second row). The normalised error is computed for each ensemble size for 120 different times (January 2015) before being binned. Histogram showing IFS model XCO2 from a 10-, 25- and 50-member ensemble after 5 d. Note that all ensembles shown consist of initial meteorological uncertainty and perturbed model physics (TME).
Here, we investigated the required ensemble size to adequately represent the prior XCO2 PDF using multiple sizes available. The model error is within 5 % of the 50-member ensemble error for ensemble sizes of 40, 39 and 43 for Paris, Caltech and Tsukuba, respectively (Fig. 6). Ensemble sizes <40 provide model error approximations that may not be suitable for use in inversions. Computational cost currently limits the use of larger ensemble sizes, and optimum ensemble size investigations indicated that the 50-member ensemble may provide an adequate sample for meteorological errors (Leutbecher et al., 2017), although CO2 poses more specific challenges and requirements.
To investigate the suitability of representing the transport error with a Gaussian PDF, ensemble members were binned into 0.05 ppm bins and a non-linear least-square fit was applied to provide an estimated Gaussian fit for a PDF with three terms: A0, A1 and A3.
Assuming the prior PDF is Gaussian, results show that ensemble sizes ≤50 can fail to represent a suitable distribution and contain spurious noise. Over Paris and Caltech, a Gaussian distribution is relatively well-captured by a 50-member ensemble; however, for Tsukuba either more ensemble members are required or the PDF is not Gaussian.
4.4 Error correlation
The noise generated by small ensemble sizes creates spurious spatial and temporal error correlations in the XCO2 transport error (Fig. 7). This localisation problem is typically addressed by limiting the distance of correlations considered within the inversion (e.g. Miyazaki et al., 2011) or by applying a decay function (e.g. Chatterjee et al., 2012). Here we propose that temporal filtering, as shown by artificially creating a 150-member ensemble using neighbouring times from a 50-member ensemble, could be used to reduce spurious error correlations. This is only applicable with suitably high-frequency model data. By filtering a small ensemble (10 members) using time smoothing and finding the best fit to a 50-member ensemble, it is typically found that a 2 h smoothing is optimum with our model set-up (T−1, T0, T+1). The optimum filter length, however, is location and time dependent.
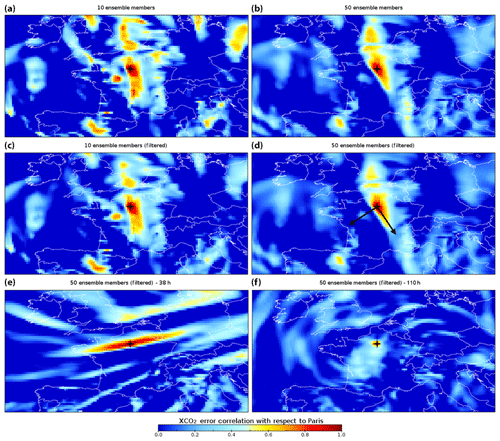
Figure 7A snapshot of regional XCO2 error correlation structure with respect to Paris XCO2 from the 10-member (a) and 50-member (b) IFS model ensemble after 4 d, when the ensemble consists of perturbed initial meteorology and model physics (transport error). (c, d) The same as (a, b) but including the preceding and subsequent model time steps (±1 h), artificially increasing the correlation sample to 30 and 150 members. (e, f) The same correlation calculations as (d) (150 members consisting of ±1 h) but for two different times, highlighting the flow dependence in error correlation structure. The star denotes the column over Paris, and the black arrows denote the downwind and across-wind directions used to calculate further and the shortest correlation lengths for a given time (see Fig. 8).
For a given location we assume that non-spurious correlations are represented by surrounding XCO2 error correlation values, which are both part of the spatial extent of the plume and greater than the derived e-folding correlation length scale (). Here we consider the plume to be represented by correlation values that continuously remain above e−0.5, extending out from the given location. The maximum distance of these correlations from the artificially generated TME 150-member ensemble can range between maximum distances of 30 to 520 km over Paris (Fig. 7). Over Caltech and Tsukuba these range from 0 to 230 km and 30 to 700 km, respectively. The flow dependency suggests that a predefined distance for the correlation filter might limit the available useful information within the inversion system, even when the filter is spatially varying. The application of the flow-dependent structure to inverse systems can be computationally expensive; as a result, offline systems should adopt a simplified approach to represent the errors derived here.
For a given time and location, assuming a Gaussian error correlation structure may cause an underestimation or overestimation of the correlation length scale, depending on direction (Figs. 8 and S3). For most situations, regardless of location, the shortest correlation length scale is close to the average correlation length in all directions; however, the downwind correlation length scale is typically around twice as far. Downwind is defined as the plume direction at model output time.
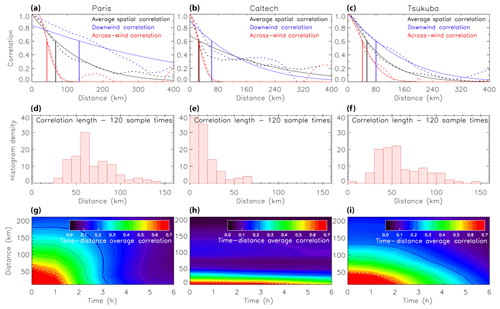
Figure 8A snapshot of XCO2 error correlation with respect to Paris (a, d, g), Caltech (b, e, h) and Tsukuba (c, f, i) as a function of distance for a 50-member IFS model ensemble after 4 d (a, b, c). These panels show the directionally averaged (black dashed line), downwind (blue dashed line) and across-wind (red dashed line) correlation values with a Gaussian fit (solid lines) in addition to the derived correlation length, where (vertical solid lines). The directionally averaged derived correlation lengths for 120 sample times for January 2015 are placed in 10 km bins for all three sites (d, e, f). The directionally averaged and time-averaged error correlation values for the same 120 sample sizes as a function of both time and distance (g, h, i).
For January, the time- and direction-averaged error correlation length scale, assuming a Gaussian distribution, varies across all three sites (Paris 67±24 km, Caltech 17±16 km and Tsukuba 59±26 km). In July, over Paris and Tsukuba, the average correlation length scale is reduced to 61±22 km and 35±16 km, respectively, whereas there is a slight increase over Caltech to 26±14 km. The large decrease in correlation length scale detected over Tsukuba in summer may be a result of dominant mesoscale biogenic fluxes in the region during summer months masking the plume from anthropogenic hotspots. The variability in average correlation length scale is reduced at all three sites during Northern Hemisphere summer, which is also likely to be the result of a more active background biogenic flux limiting the maximum spatial extent of the signal from anthropogenic hotspots. Seasonal variability in local meteorological systems is also likely to cause observed changes in the correlation length scales derived. At all three locations the average error correlation length scale in all directions varies considerably with time, suggesting that flow-dependent information is required and no single length scale should be used (Figs. 8 and S3).
The average error correlation in both time and space simultaneously is also considered, again using a simplistic Gaussian assumption (Figs. 8 and S3). This shows that the time component of the average error correlation varies with location, with an average time correlation length scale decreasing with distance.
For January Paris (80 min) and Tsukuba (150 min) both show a relatively short average time correlation length scale but a long spatial length scale, whereas Caltech (260 min) has a longer time correlation length scale and shorter spatial length scale. For July the correlation length scale increases over both Paris (120 min) and Tsukuba (170 min), with decreases over Caltech (160 min). Differences between locations and seasons are caused by changes in fluxes, meteorology and orography. For instance, over Caltech, shorter spatial correlations and longer time correlations result from the impact of the Los Angeles basin, which reinforces air stagnation during winter. This effect is less pronounced during the summer due to the presence of stronger sea breezes.
We have performed multiple ensemble simulations of CO2 using an online NWP model to quantify sources of atmospheric model uncertainty. We have individually diagnosed the relative contribution of uncertainties from the initial meteorological state and model physics to the total transport error. This work can be used to inform future atmospheric flux inversion studies on the spatio-temporal variability of model transport error, which is typically lacking. By utilising the online capability of the ESM, we have also diagnosed the biogenic flux feedback error associated with uncertainties in atmospheric meteorology. We have performed ensemble simulations using perturbed anthropogenic emissions to investigate the signal-to-noise ratio, which provides a first assessment of the posterior error reductions in an anthropogenic inversion system. Finally, we have diagnosed error correlations and correlation length scales at selected sites. To evaluate the diagnosed error, the results were validated at three TCCON sites. The ensemble-derived uncertainties found here will be used to model transport errors in a proposed future operational global CO2 monitoring system being developed as part of the CO2 Human Emissions project.
The transport error is shown to be spatio-temporally varying and is largest near biogenic and anthropogenic flux hotspots. Transport errors over anthropogenic flux hotspots are on average 0.1–0.8 ppm and 0.1–0.7 ppm for January and July, respectively. This transport error is comparable to uncertainties in the prior monthly anthropogenic emissions projected onto the observation (XCO2) space over the same regions (January: 0.1–1.4 ppm, July: 0.1–1.2 ppm). However, since the proposed future monitoring system will be based on prior flux uncertainties associated with higher temporal resolutions than those used here (daily and hourly), a significant increase in the signal-to-noise ratio is expected. The estimation of high-frequency transport error covariance structures is essential to ensuring the reliability of the future inversion system. With potential future improvements to bottom-up flux estimations the signal-to-noise ratio may further decrease in the future, decreasing the posterior error reduction values that could be expected from such a system. The spatial and temporal variability of errors and resulting signal-to-noise ratios are influenced by neighbouring hotspots, local orography and meteorological variability. Our findings, on a global scale, agree well with the regional study of Chen et al. (2019).
Atmospheric CO2 transport error initially grows and then plateaus after 2–3 d, depending on the location. After this time the error growth from uncertainties in transport balances out with the atmospheric CO2 mixing, resulting in a globally averaged transport error of ∼0.1 ppm.
A noticeable transport error is identified in both the near-surface model levels and in the total-column CO2. As a result, it is likely to impact both satellite- and surface-based atmospheric inversions. These results highlight the importance of including detailed transport error within atmospheric CO2 inversions, as most previous studies either ignore it or use a simplistic representation of model transport error, leading to overconfidence in results. The near-surface errors found here at three sites (1.7–7.2 ppm) are comparable to the 3–4 ppm errors found by Díaz-Isaac et al. (2018).
The atmospheric CO2 error caused by the biogenic feedback error as a response to uncertainty in meteorology is found to be small; however, in regions of high net ecosystem exchange this value increases to an average of 0.16 ppm and requires consideration for high-precision atmospheric inversions in those regions. Both the atmospheric response to prior anthropogenic emission uncertainties and the biogenic feedback errors are found to be seasonally dependent for some locations, caused by seasonal changes in flux and meteorology. This also results in seasonal variability in the model transport error over regions of high net ecosystem exchange. The error associated with biogenic fluxes shown here does not account for uncertainties in the biogenic model or ancillary information (e.g. mapping or plant functional type).
Validation performed with TCCON observations suggests that the uncertainty derived in model XCO2 from transport uncertainty, anthropogenic flux uncertainty and biogenic feedback to meteorological uncertainties accounts for 21 %–65 % of the total model uncertainty, depending on time and location. An underrepresentation of anthropogenic flux uncertainty, by using monthly and not higher-temporal-resolution uncertainties, and other factors including observation errors, numerical errors, the representation error, missing biogenic processes and biogenic mapping errors make up the remaining model uncertainty. These remaining uncertainties are not negligible; for example, a previous study showed that over the same Caltech site used in this study, the model representation error is typically 2 ppm for January (Agustí-Panareda et al., 2019). Future studies should aim to quantify these additional aspects of model uncertainty.
The 50-member ensemble used here is shown to provide a reasonable estimate of the prior PDF; however, for some regions, ensemble sizes larger than 50 members may be required. The computational cost of sufficiently large ensemble sizes to describe the spatial error structures could potentially be overcome by appropriate filtering techniques of smaller ensemble sizes (e.g. Lauvaux et al., 2019).
Spurious noise is evident in the transport error correlation structure of a 50-member ensemble; to address this issue and prevent further computational costs, we apply a simple time filtering to artificially increase the member size to 150 members. Error correlation structures are shown to be strongly flow dependent. Using a simplified Gaussian assumption the average correlation length scale values are found to be between 0 and 700 km in distance and 0 and 260 min in time, with a seasonal dependence based on changes in flux and meteorology.
The transport uncertainty diagnosed here highlights the importance of accounting for all sources of model error when performing inversions. Our results are derived using an online NWP system; however, our findings can be used with various levels of complexity to inform future CO2 offline inversions at both the regional and global scale. It should be noted that whilst these uncertainties can be used in an offline system, several additional errors would also need to be considered, including interpolation errors and inconsistencies between transport parameterisations. The model error PDF, although reasonably well-represented by the 50-member ensemble, requires either additional ensemble members or suitable selection techniques (e.g. Díaz-Isaac et al., 2019), which requires further investigation. For the wider inverse modelling community, gridded total errors are available for the total-column CO2 mixing ratios at 3-hourly intervals for all of 2015, and hourly gridded transport errors are available for both the total column and surface for January and July 2015 at https://doi.org/10.5281/zenodo.3703136.
The IFS source code is available subject to a licence agreement with the ECMWF; see also Leutbecher et al. (2017) for details on the ensemble model description and specific details of the code relevant to this study, including the use of the EDA and SPPT. ECMWF member-state weather services and their approved partners will be granted access. Components of the IFS code relevant to this study (e.g. SPPT), without modules for data assimilation, are also available for educational and academic purposes as part of the OpenIFS project (https://software.ecmwf.int/wiki/display/OIFS/OpenIFS+Home, last access: 9 December 2019; OpenIFS project, 2019). Technical developments specifically related to the work detailed here are available upon request; please contact joe.mcnorton@ecmwf.int. The specific code relevant to this study for emissions perturbations based on given log-normal uncertainties is available at https://doi.org/10.5281/zenodo.3750842 (McNorton et al., 2020).
Model data are available online through the ECMWF Meteorological Archive and Retrieval System (MARS) catalogue, but access may be limited. Model output data are available upon request to joe.mcnorton@ecmwf.int. Ensemble-based error calculations for 2015 are available at https://doi.org/10.5281/zenodo.3750842 (McNorton et al., 2020).
The supplement related to this article is available online at: https://doi.org/10.5194/gmd-13-2297-2020-supplement.
The simulations were performed by JM with coding developments from AA-P, AD, ZK and SL. The experimental design was devised by JM, NB, AA-P, GP, RE and SL. Emission inventories were compiled by JM and AAP, with uncertainties and budgets calculated by JM and MC. The paper was prepared by JM with analysis interpretation from NB as well as input and feedback from AA-P, GP, NB, RE and SL.
The authors declare that they have no conflict of interest.
This research has been supported by Horizon 2020 (CHE (grant no. 776186)).
This paper was edited by Juan Antonio Añel and reviewed by two anonymous referees.
Agustí-Panareda, A., Massart, S., Chevallier, F., Boussetta, S., Balsamo, G., Beljaars, A., Ciais, P., Deutscher, N. M., Engelen, R., Jones, L., Kivi, R., Paris, J.-D., Peuch, V.-H., Sherlock, V., Vermeulen, A. T., Wennberg, P. O., and Wunch, D.: Forecasting global atmospheric CO2, Atmos. Chem. Phys., 14, 11959–11983, https://doi.org/10.5194/acp-14-11959-2014, 2014.
Agustí-Panareda, A., Massart, S., Chevallier, F., Balsamo, G., Boussetta, S., Dutra, E., and Beljaars, A.: A biogenic CO2 flux adjustment scheme for the mitigation of large-scale biases in global atmospheric CO2 analyses and forecasts, Atmos. Chem. Phys., 16, 10399–10418, https://doi.org/10.5194/acp-16-10399-2016, 2016.
Agustí-Panareda, A., Diamantakis, M., Massart, S., Chevallier, F., Muñoz-Sabater, J., Barré, J., Curcoll, R., Engelen, R., Langerock, B., Law, R. M., Loh, Z., Morguí, J. A., Parrington, M., Peuch, V.-H., Ramonet, M., Roehl, C., Vermeulen, A. T., Warneke, T., and Wunch, D.: Modelling CO2 weather – why horizontal resolution matters, Atmos. Chem. Phys., 19, 7347–7376, https://doi.org/10.5194/acp-19-7347-2019, 2019.
Ahmadov, R., Gerbig, C., Kretschmer, R., Körner, S., Rödenbeck, C., Bousquet, P., and Ramonet, M.: Comparing high resolution WRF-VPRM simulations and two global CO2 transport models with coastal tower measurements of CO2, Biogeosciences, 6, 807–817, https://doi.org/10.5194/bg-6-807-2009, 2009.
Baker, D. F., Law, R. M., Gurney, K. R., Rayner, P., Peylin, P., Denning, A. S., Bousquet, P., Bruhwiler, L., Chen, Y. H., Ciais, P., and Fung, I. Y.: TransCom 3 inversion intercomparison: Impact of transport model errors on the interannual variability of regional CO2 fluxes, 1988–2003, Global Biogeochem. Cy., 20, GB1002, https://doi.org/10.1029/2004GB002439, 2006.
Basu, S., Baker, D. F., Chevallier, F., Patra, P. K., Liu, J., and Miller, J. B.: The impact of transport model differences on CO2 surface flux estimates from OCO-2 retrievals of column average CO2, Atmos. Chem. Phys., 18, 7189–7215, https://doi.org/10.5194/acp-18-7189-2018, 2018.
Boussetta, S., Balsamo, G., Beljaars, A., Panareda, A. A., Calvet, J. C., Jacobs, C., van den Hurk, B., Viterbo, P., Lafont, S., Dutra, E., and Jarlan, L.: Natural land carbon dioxide exchanges in the ECMWF Integrated Forecasting System: Implementation and offline validation, J. Geophys. Res.-Atmos., 118, 5923–5946, https://doi.org/10.1002/jgrd.50488, 2013.
Buizza, R., Milleer, M., and Palmer, T. N.: Stochastic representation of model uncertainties in the ECMWF ensemble prediction system, Q. J. Roy. Meteorol. Soc., 125, 2887–2908, https://doi.org/10.1002/qj.49712556006, 1999.
Chatterjee, A., Michalak, A. M., Anderson, J. L., Mueller, K. L., and Yadav, V.: Toward reliable ensemble Kalman filter estimates of CO2 fluxes, J. Geophys. Res.-Atmos., 117, D22306, https://doi.org/10.1029/2012JD018176, 2012.
Chen, H. W., Zhang, F., Lauvaux, T., Davis, K. J., Feng, S., Butler, M. P., and Alley, R. B.: Characterization of Regional-Scale CO2 Transport Uncertainties in an Ensemble with Flow-Dependent Transport Errors, Geophys. Res. Lett., 46, 4049–4058, 2019.
Choulga, M., Janssens-Maenhout, G., Super, I., Agusti-Panareda, A., Balsamo, G., Bousserez, N., Crippa, M., Denier van der Gon, H., Engelen, R., Guizzardi, D., Kuenen, J., McNorton, J., Oreggioni, G., Solazzo, E., and Visschedijk, A.: Global anthropogenic CO2 emissions and uncertainties as prior for Earth system modelling and data assimilation, Earth Syst. Sci. Data Discuss., https://doi.org/10.5194/essd-2020-68, in review, 2020.
Denning, A. S., Fung, I. Y., and Randall, D.: Latitudinal gradient of atmospheric CO2 due to seasonal exchange with land biota, Nature, 376, 240–243, 1995.
Denning, A. S., Zhang, N., Yi, C., Branson, M., Davis, K., Kleist, J., and Bakwin, P.: Evaluation of modeled atmospheric boundary layer depth at the WLEF tower, Agr. Forest Meteorol., 148, 206–215, https://doi.org/10.1016/j.agrformet.2007.08.012, 2008.
Díaz-Isaac, L. I., Lauvaux, T., and Davis, K. J.: Impact of physical parameterizations and initial conditions on simulated atmospheric transport and CO2 mole fractions in the US Midwest, Atmos. Chem. Phys., 18, 14813–14835, https://doi.org/10.5194/acp-18-14813-2018, 2018.
Díaz-Isaac, L. I., Lauvaux, T., Bocquet, M., and Davis, K. J.: Calibration of a multi-physics ensemble for estimating the uncertainty of a greenhouse gas atmospheric transport model, Atmos. Chem. Phys., 19, 5695–5718, https://doi.org/10.5194/acp-19-5695-2019, 2019.
Dlugokencky, E. and Tans, P.: Trends in atmospheric carbon dioxide, National Oceanic & Atmospheric Administration, Earth System Research Laboratory (NOAA/ESRL), available at: http://www.esrl.noaa.gov/gmd/ccgg/trends/global.html, last access: 9 September 2019.
Engelen, R. J., Denning, A. S., and Gurney, K. R.: On error estimation in atmospheric CO2 inversions, J. Geophys. Res.-Atmos., 107, 4635, https://doi.org/10.1029/2002JD002195, 2002.
Gaubert, B., Stephens, B. B., Basu, S., Chevallier, F., Deng, F., Kort, E. A., Patra, P. K., Peters, W., Rödenbeck, C., Saeki, T., Schimel, D., Van der Laan-Luijkx, I., Wofsy, S., and Yin, Y.: Global atmospheric CO2 inverse models converging on neutral tropical land exchange, but disagreeing on fossil fuel and atmospheric growth rate, Biogeosciences, 16, 117–134, https://doi.org/10.5194/bg-16-117-2019, 2019.
Gurney, K. R., Law, R. M., Denning, A. S., Rayner, P. J., Baker, D., Bousquet, P., Bruhwiler, L., Chen, Y. H., Ciais, P., Fan, S., and Fung, I. Y.: Towards robust regional estimates of CO2 sources and sinks using atmospheric transport models, Nature, 415, 626, https://doi.org/10.1038/415626a, 2002.
IPCC 2006: IPCC Guidelines for National Greenhouse Gas Inventories, Prepared by the National Greenhouse Gas Inventories Programme, edited by: Eggleston, H. S., Buendia, L., Miwa, K., Ngara, T., and Tanabe, K., Published: IGES, Japan, 2006.
Isaksen, L., Bonavita, M., Buizza, R., Fisher, M., Haseler, J., Leutbecher, M., and Raynaud, L.: Ensemble of data assimilations at ECMWF, Research Department Technical Memorandum No. 636, ECMWF, Shinfield Park, Reading RG29AX, UK, available at: http://www.ecmwf.int/publications/ (last access: 9 December 2019), 2010.
Janssens-Maenhout, G., Crippa, M., Guizzardi, D., Muntean, M., Schaaf, E., Dentener, F., Bergamaschi, P., Pagliari, V., Olivier, J. G. J., Peters, J. A. H. W., van Aardenne, J. A., Monni, S., Doering, U., Petrescu, A. M. R., Solazzo, E., and Oreggioni, G. D.: EDGAR v4.3.2 Global Atlas of the three major greenhouse gas emissions for the period 1970–2012, Earth Syst. Sci. Data, 11, 959–1002, https://doi.org/10.5194/essd-11-959-2019, 2019.
Joos, F. and Spahni, R.: Rates of change in natural and anthropogenic radiative forcing over the past 20,000 years, P. Natl. Acad. Sci. USA, 105, 1425–1430, https://doi.org/10.1073/pnas.0707386105, 2008.
Kaiser, J. W., Heil, A., Andreae, M. O., Benedetti, A., Chubarova, N., Jones, L., Morcrette, J.-J., Razinger, M., Schultz, M. G., Suttie, M., and van der Werf, G. R.: Biomass burning emissions estimated with a global fire assimilation system based on observed fire radiative power, Biogeosciences, 9, 527–554, https://doi.org/10.5194/bg-9-527-2012, 2012.
Kretschmer, R., Gerbig, C., Karstens, U., and Koch, F.-T.: Error characterization of CO2 vertical mixing in the atmospheric transport model WRF-VPRM, Atmos. Chem. Phys., 12, 2441–2458, https://doi.org/10.5194/acp-12-2441-2012, 2012.
Lauvaux, T. and Davis, K. J.: Planetary boundary layer errors in mesoscale inversions of column-integrated CO2 measurements, J. Geophys. Res.-Atmos., 119, 490–508, https://doi.org/10.1002/2013JD020175, 2014.
Lauvaux, T., Miles, N. L., Deng, A., Richardson, S. J., Cambaliza, M. O., Davis, K. J., Gaudet, B., Gurney, K. R., Huang, J., O'Keefe, D., and Song, Y.: High-resolution atmospheric inversion of urban CO2 emissions during the dormant season of the Indianapolis Flux Experiment (INFLUX), J. Geophys. Res.-Atmos., 121, 5213–5236, https://doi.org/10.1002/2015JD024473, 2016.
Lauvaux, T., Díaz-Isaac, L. I., Bocquet, M., and Bousserez, N.: Diagnosing spatial error structures in CO2 mole fractions and XCO2 column mole fractions from atmospheric transport, Atmos. Chem. Phys., 19, 12007–12024, https://doi.org/10.5194/acp-19-12007-2019, 2019.
Law, R. M., Peters, W., Rödenbeck, C., Aulagnier, C., Baker, I., Bergmann, D. J., Bousquet, P., Brandt, J., Bruhwiler, L., Cameron-Smith, P. J., and Christensen, J. H.: TransCom model simulations of hourly atmospheric CO2: Experimental overview and diurnal cycle results for 2002, Global Biogeochem. Cy., 22, GB3009, https://doi.org/10.1029/2007GB003050, 2008.
Le Quéré, C., Andrew, R. M., Friedlingstein, P., Sitch, S., Hauck, J., Pongratz, J., Pickers, P. A., Korsbakken, J. I., Peters, G. P., Canadell, J. G., Arneth, A., Arora, V. K., Barbero, L., Bastos, A., Bopp, L., Chevallier, F., Chini, L. P., Ciais, P., Doney, S. C., Gkritzalis, T., Goll, D. S., Harris, I., Haverd, V., Hoffman, F. M., Hoppema, M., Houghton, R. A., Hurtt, G., Ilyina, T., Jain, A. K., Johannessen, T., Jones, C. D., Kato, E., Keeling, R. F., Goldewijk, K. K., Landschützer, P., Lefèvre, N., Lienert, S., Liu, Z., Lombardozzi, D., Metzl, N., Munro, D. R., Nabel, J. E. M. S., Nakaoka, S., Neill, C., Olsen, A., Ono, T., Patra, P., Peregon, A., Peters, W., Peylin, P., Pfeil, B., Pierrot, D., Poulter, B., Rehder, G., Resplandy, L., Robertson, E., Rocher, M., Rödenbeck, C., Schuster, U., Schwinger, J., Séférian, R., Skjelvan, I., Steinhoff, T., Sutton, A., Tans, P. P., Tian, H., Tilbrook, B., Tubiello, F. N., van der Laan-Luijkx, I. T., van der Werf, G. R., Viovy, N., Walker, A. P., Wiltshire, A. J., Wright, R., Zaehle, S., and Zheng, B.: Global Carbon Budget 2018, Earth Syst. Sci. Data, 10, 2141–2194, https://doi.org/10.5194/essd-10-2141-2018, 2018.
Leutbecher, M. and Palmer, T. N.: Ensemble forecasting, J. Comput. Phys., 227, 3515–3539, https://doi.org/10.1016/j.jcp.2007.02.014, 2008.
Leutbecher, M., Lock, S. J., Ollinaho, P., Lang, S. T., Balsamo, G., Bechtold, P., Bonavita, M., Christensen, H. M., Diamantakis, M., Dutra, E., and English, S.: Stochastic representations of model uncertainties at ECMWF: state of the art and future vision, Q. J. Roy. Meteorol. Soc., 143, 2315–2339, https://doi.org/10.1002/qj.3094, 2017.
Lin, J. C. and Gerbig, C.: Accounting for the effect of transport errors on tracer inversions, Geophys. Res. Lett., 32, L01802, https://doi.org/10.1029/2004GL021127, 2005.
McNorton, J., Bousserez, N., Agustí-Panareda, A., Balsamo, G., Choulga, M., Dawson, A., Engelen, R., Kipling, Z. and Lang, S.: Representing Model Uncertainty for Global Atmospheric CO2 Flux Inversions Using ECMWF-IFS-46R1, Data set, Geoscientific Model Development, Zenodo, https://doi.org/10.5281/zenodo.3750842, 2020.
Miyazaki, K., Maki, T., Patra, P., and Nakazawa, T.: Assessing the impact of satellite, aircraft, and surface observations on CO2 flux estimation using an ensemble‐based 4‐D data assimilation system, J. Geophys. Res.-Atmos., 116, D16306, https://doi.org/10.1029/2010JD015366, 2011.
Morino, I., Matsuzaki, T., and Horikawa, M.: TCCON data from Tsukuba (JP), 125HR, Release GGG2014.R2, Data set, CaltechDATA, https://doi.org/10.14291/tccon.ggg2014.tsukuba02.r2, 2018.
OpenIFS project: OpenIFS Home, available at: https://software.ecmwf.int/wiki/display/OIFS/OpenIFS+Home, last access: 9 December 2019.
Peylin, P., Law, R. M., Gurney, K. R., Chevallier, F., Jacobson, A. R., Maki, T., Niwa, Y., Patra, P. K., Peters, W., Rayner, P. J., Rödenbeck, C., van der Laan-Luijkx, I. T., and Zhang, X.: Global atmospheric carbon budget: results from an ensemble of atmospheric CO2 inversions, Biogeosciences, 10, 6699–6720, https://doi.org/10.5194/bg-10-6699-2013, 2013.
Rödenbeck, C., Bakker, D. C. E., Metzl, N., Olsen, A., Sabine, C., Cassar, N., Reum, F., Keeling, R. F., and Heimann, M.: Interannual sea–air CO2 flux variability from an observation-driven ocean mixed-layer scheme, Biogeosciences, 11, 4599–4613, https://doi.org/10.5194/bg-11-4599-2014, 2014.
Sarrat, C., Noilhan, J., Lacarrere, P., Donier, S., Lac, C., Calvet, J. C., Dolman, A. J., Gerbig, C., Neininger, B., Ciais, P., and Paris, J. D.: Atmospheric CO2 modeling at the regional scale: Application to the CarboEurope Regional Experiment, J. Geophys. Res.-Atmos., 112, D12105, https://doi.org/10.1029/2006JD008107, 2007.
Stephens, B. B., Gurney, K. R., Tans, P. P., Sweeney, C., Peters, W., Bruhwiler, L., Ciais, P., Ramonet, M., Bousquet, P., Nakazawa, T., and Aoki, S.: Weak northern and strong tropical land carbon uptake from vertical profiles of atmospheric CO2, Science, 316, 1732–1735, https://doi.org/10.1126/science.1137004, 2007.
Té, Y., Jeseck, P., and Janssen, C.: TCCON data from Paris (FR), Release GGG2014.R0, Data set, CaltechDATA, https://doi.org/10.14291/tccon.ggg2014.paris01.r0/1149279, 2014.
Verma, S., Marshall, J., Gerbig, C., Rödenbeck, C., and Totsche, K. U.: The constraint of CO2 measurements made onboard passenger aircraft on surface–atmosphere fluxes: the impact of transport model errors in vertical mixing, Atmos. Chem. Phys., 17, 5665–5675, https://doi.org/10.5194/acp-17-5665-2017, 2017.
Wennberg, P. O., Wunch, D., Roehl, C. M., Blavier, J.-F., Toon, G. C., and Allen, N. T.: TCCON data from Caltech (US), Release GGG2014.R1, Data set, CaltechDATA, https://doi.org/10.14291/tccon.ggg2014.pasadena01.r1/1182415, 2015.
Wu, L., Bocquet, M., Chevallier, F., Lauvaux, T., and Davis, K.: Hyperparameter estimation for uncertainty quantification in mesoscale carbon dioxide inversions, Tellus B, 65, 20894, https://doi.org/10.3402/tellusb.v65i0.20894, 2013.
Wunch, D., Toon, G. C., Blavier, J. F. L., Washenfelder, R. A., Notholt, J., Connor, B. J., Griffith, D. W., Sherlock, V., and Wennberg, P. O.: The total carbon column observing network, Philos. Trans. Roy. Soc. A, 369, 2087–2112, https://doi.org/10.1098/rsta.2010.0240, 2011.
Yi, C., Davis, K. J., Bakwin, P. S., Denning, A. S., Zhang, N., Desai, A., Lin, J. C., and Gerbig, C.: Observed covariance between ecosystem carbon exchange and atmospheric boundary layer dynamics at a site in northern Wisconsin, J. Geophys. Res.-Atmos., 109, D08302, https://doi.org/10.1029/2003JD004164, 2004.