the Creative Commons Attribution 4.0 License.
the Creative Commons Attribution 4.0 License.
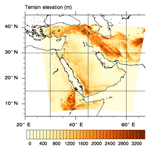
Development of a dynamic dust source map for NMME-DREAM v1.0 model based on MODIS Normalized Difference Vegetation Index (NDVI) over the Arabian Peninsula
Stavros Solomos
Abdelgadir Abuelgasim
Christos Spyrou
Ioannis Binietoglou
Slobodan Nickovic
We developed a time-dependent dust source map for the NMME Dust Regional Atmospheric Model (DREAM v1.0) based on the satellite MODIS Normalized Difference Vegetation Index (NDVI). Areas with NDVI <0.1 are classified as active dust sources. The updated modeling system is tested for dust emission capabilities over SW Asia using a mesoscale model grid increment of for a period of 1 year (2016). Our results indicate significant deviations in simulated aerosol optical depths (AODs) compared to the static dust source approach and general increase in dust loads over the selected domain. Comparison with MODIS AOD indicates a more realistic spatial distribution of dust in the dynamic source simulations compared to the static dust sources approach. The modeled AOD bias is improved from −0.140 to 0.083 for the case of dust events (i.e., for AOD >0.25) and from −0.933 to −0.424 for dust episodes with AOD >1. This new development can be easily applied to other time periods, models, and different areas worldwide for a local fine tuning of the parameterization and assessment of its performance.
- Article
(5694 KB) - Full-text XML
- BibTeX
- EndNote
The importance of natural particles, namely desert dust, in the weather and climate has been underlined in a great number of studies. Dust is a climatic regulator, as it modifies extensively the radiative balance of the atmospheric column (e.g., Torge et al., 2011; Spyrou et al., 2013; Mahowald et al., 2014). At the same time dust aerosols modify the atmospheric water content (Spyrou, 2018), the way clouds are formed by acting as cloud condensation nuclei (CCN) and ice nuclei (IN), and the precipitation process (Kumar et al., 2011; Solomos et al., 2011; Nickovic et al., 2016). In addition, there is a clear connection between dust particles and human health disorders, as the size of the aerosols produced is small enough to cause respiratory and cardiovascular diseases, as well as pathogenic conditions due to the microorganisms that they can potentially carry (Mitsakou et al., 2008; Esmaeil et al., 2014).
The Arabian Peninsula is one of the most important sources of mineral dust worldwide and together with the Sahara and the Gobi contributes to the formation of a Northern Hemisphere “dust belt” as described by Prospero et al. (2002). Severe dust storms over the Peninsula are quite common, especially during long periods without rain, in the spring and summer (Almazrouia et al., 2012). Particles injected into the atmosphere from arid soils, under favorable weather conditions (high wind speeds and dry soil), can affect large areas around the sources but also remote locations like the Eastern Mediterranean (Mamouri et al., 2016; Solomos et al., 2017) and the Indian Ocean (Chakraborty et al., 2006).
Due to the multitude and severe effects of dust particles not only on the weather and the ecosystem but on human health as well, the proper description of the production, transport, and eventual deposition of the dust cycle in numerical weather prediction models (NWPs) is essential. In order to be able to accurately describe the dust life cycle in the atmosphere, we need a clear understanding of the areas which can potentially act as “dust sources”. The definition of such areas dictates the emission strength and therefore the amount of particles inserted into the atmosphere. A proper representation of dust sources is therefore an essential first step, in studying the impacts of mineral particles in the climate and human societies. Usually the definition of the areas that can act as dust sources is made using global datasets. For example Nickovic et al. (2001) used a subjective correspondence between the Olson World Ecosystems (Olson et al., 1983) and the 13 SSib (simplified simple biosphere; Xue et al., 1991) vegetation types to identify arid and semiarid areas. Similarly, Spyrou et al. (2010) used a 30 s global land use/cover database, classified according to the 24 category U.S. Geological Survey (USGS) land use/cover system (Anderson et al., 1976) to define active areas in the SKIRON dust model. Solomos et al. (2011) used the LEAF soil and vegetation sub-model of the Regional Atmospheric Modeling System (RAMS) (Walko et al., 2000) to identify the active dust sources in the RAMS-ICLAMS model.
However, the abovementioned methodologies have some significant drawbacks. The datasets are usually not up to date; therefore, recent land use modifications are not included and not represented. In addition, such “static” databases mean that possible seasonal variations are not taken into account. In order to overcome the above limitations and improving global dust forecasts, Kim et al. (2013) developed a dynamical dust source map for the GOCART dust model by characterizing Normalized Difference Vegetation Index (NDVI) values <0.15 as active dust spots. Similarly Vukovic et al. (2014) combined MODIS land cover types with pixels having NDVI <0.1 to identify the seasonal dust sources that caused the severe Phoenix haboob of July 2011 in the US. Such information can be even more relevant at meso- and local scales for determining land use changes and potential dust sources, especially in heterogeneous regions such as the Arabian Peninsula (which has more diverse soil types than, e.g., the Sahara) and the greater SW Asia. In this context, Solomos et al. (2017), used the Landsat-8 NDVI data (assuming also NDVI <0.1 as active sources) to identify recent changes in land use due to the war in Iraq and Syria resulting in a significantly more realistic simulation of dust properties in the Middle East.
In the current study we present the implementation of a dynamical dust source map in the well-established and widely used the Dust Regional Atmospheric Model (DREAM v1.0) (Nickovic et al., 2001; Pérez et al., 2006). The new development is first tested here for the greater SW Asia but can be extended for use in mesoscale dust modeling applications worldwide. Two experimental simulations are performed for a 1-month period (August 2016) over the greater SW Asia: (1) a control run, where the dust source definition is based on the Ginoux et al. (2001) dataset, and (2) a dynamic source run, where the NDVI values are used to identify the dust sources. The main differences in our approach compared to the previous studies referenced above are that we use a very high-resolution NDVI product (500 m×500 m) in a regional modeling domain (e.g., Kim et al., 2013 used an 8 km×8 km NDVI dataset extrapolated to global modeling domain) and our study is not limited to specific test cases (like, for example, Vukovic et al., 2014 and Solomos et al., 2017), but covers an extended time period, as presented below. The model results from both runs are compared to available satellite observations and station measurements inside the modeling domain. In Sect. 1 we describe the methodological steps regarding the model developments and remote-sensing data; Sect. 2 includes the results of the experimental runs and Sect. 3 is a summary and discussion of the study findings.
2.1 Model description
The modeling system used in this study is NMME-DREAM v1.0. The meteorological core is the NCEP–NMME atmospheric model (Janjic et al., 2001). DREAM v1.0 is a numerical model created with the main purpose of simulating and predicting the atmospheric life cycle of mineral dust using an Euler-type nonlinear partial differential equation for dust mass continuity (Nickovic et al., 2001, 2016; Pérez et al., 2006; Pejanovic et al., 2011). In DREAM the concentration approach is used for dust uplift, where surface concentration is used as a lower boundary condition and used for the calculation of surface fluxes, which in turn depends on the friction velocity (Nickovic et al., 2001). This surface concentration is calculated using Eq. (11) from Nickovic et al. (2001):
where is a constant determined from model experiments, u* and u*t are the friction velocity and the threshold friction velocity for dust production, respectively, and , where γk the ratio between the mass available for uplift and the total mass βk the fractions of clay, silt, and sand for each soil class and a is the desert mask (between 0 and 1) calculated from the Ginoux et al. (2001) dataset. Soil moisture and particle size dictate the threshold friction velocity which initializes dust production. Once particles have been lifted from the ground they are driven by the atmospheric model variables and processes. Therefore, turbulent parameters are used in the beginning of the process, when dust is lifted from the ground, and transported by model winds in the later phases, when dust travels away from the sources. The model handles dust in eight size bins, with effective radii of 0.15, 0.25, 0.45, 0.78, 1.3, 2.2, 3.8, and 7.1 mm. Dust is treated as a passive tracer and does not interact with radiation or clouds. Dust is eventually settled through rainfall and/or dry deposition processes parameterized according to the scheme of Georgi (1986), which includes deposition by surface turbulent and Brownian diffusion, gravitational settling, and impact on surface elements.
In order to test the use of NDVI for source characterization, the model is set up with a horizontal resolution of , covering the Arabian Peninsula parts of SW Asia and parts of NE Africa (Fig. 1). In the vertical we use 28 levels stretching from the surface to the top of the atmosphere. August 2016 has been selected as a test period for the model development due to the significant dust activity and variability in wind properties during this month. One-year runs for all of 2016 have been conducted to evaluate the performance of the static and dynamic database emission maps. The original classification of dust sources in DREAM is based on Ginoux et al. (2001), which takes into account the preferential sources related to topographic depressions and paleolake sediments. The global mapping of dust sources in Ginoux et al. (2001) is determined from the comparison between the elevation of surface grid points at resolution with the surrounding hydrological basins and with the AVHRR (Advanced Very High Resolution Radiometer) vegetation map (DeFries and Townshend, 1994). Recent studies indicated the contribution of both natural and anthropogenic dust sources to the overall dust emissions detected by the MODIS Deep Blue product (Ginoux et al., 2012) and also the relevance of local geomorphological conditions and sediment supply (Parajuli and Zender, 2017) for the global dust emissions. All these advances in dust emissions are based on static map considerations.
In our work, a numerical procedure has been developed to insert the NDVI satellite information into the model and to update such information each time the NDVI changes during the simulation period. We assume that regions with NDVI values from 0 to 0.1 correspond to bare soil and therefore can be efficient sources (“dust points”; DeFries and Townshend, 1994; Solomos et al., 2017). In general it is not easy to define a global threshold value for all satellite NDVI sensors and all vegetation types worldwide. For example Kim et al. (2013) used a threshold of 0.15 to define global dust sources based on AVHRR retrievals (Tucker et al., 2005; Brown et al., 2006). Here we adopt the 0.1 NDVI threshold due to the bareness of the specific modeling domain since a higher value could overestimate the regional dust sources. The NDVI dataset is at a finer resolution than the model grid (500 m×500 m), and in order to find the potential for dust production in each model grid box, we calculate the following ratio:
where #_of_dust_points is the number of points with NDVI values smaller than 0.1. This approach allows for a dynamic description of dust source areas over the model domain to replace the previously used static database. Moreover, the scaling of satellite data over model grid points allows the use of the same algorithm for different model configurations. Several mountains in the area (e.g., the Sarawat Mountains along the Red Sea coast and the Zagros Mountains in Iraq) could be misclassified as dust sources due to low NDVI values. In order to exclude such unrealistic emissions from non-soil bare areas or snow-covered areas we have applied a limit of zero dust production above 2500 m over the entire domain. This simple approach has been selected in order to keep our straightforward NDVI mapping independent of vegetation and soil information. The threshold value of 2500 m does not suppress the emissions from lowlands and hillsides (e.g., the coastal areas of the Hejaz Mountains in the Red Sea that have been identified as dust hot spots by Anisimov et al., 2017).
In Fig. 2a we show the static sources in the original model version with a factor of 0 to 1 depending on the source area strength. Accordingly in Fig. 2b we show the new dynamic sources for 1–16 August 2016. The two dust source patterns present remarkable differences especially over western Saudi Arabia and over Iran and Pakistan where the NDVI classification results in stronger emissions. In order to test the performance of the new methodology, we run the model in two different configurations: (1) using the static Ginoux et al. (2001) dust source database, called DREAM-CTRL run from now on, and (2) using the dynamic NDVI database as described above, called DREAM-NDVI run from now on. Both setups are initialized using the National Centers for Environmental Prediction Global Forecast System (NCEP GFS) analysis files ( at 00:00, 06:00, 12:00, and 18:00 UTC), which were used for boundary conditions as well. The two model configurations are identical except for the dust source database.
2.2 NDVI description
For the purposes of our study we used the 500 m 16-day averaged NDVI from MODIS (Didan, 2015) for the period of interest. The NDVI is a normalized transform of the near-infrared to red reflectance ratio, designed to provide a standard for vegetation, and takes values between −1 and +1. Since it is expressed as a ratio, the NDVI has the advantage of minimizing certain types of band-correlated noise (positively correlated) and influences attributed to variations in irradiance, clouds, atmospheric attenuation, and other parameters (Solano et al., 2010).
To create an accurate time-dependent dust source map, we have utilized the NDVI derived from the MODIS-Terra instrument. NDVI is calculated as the normalized difference of reflectance in the red and near-infrared channels (Rouse Jr. et al., 1974; Huete et al., 2002); i.e.,
where X represents surface reflectance as would be measured at ground level (i.e., corrected for atmospheric gas and aerosol effects) in each channel. The 16-day composite is calculated by ingesting two 8-day composite surface reflectance granules, taking into account pixel quality, presence of clouds, and viewing geometry. This procedure can lead to spatial discontinuities, as it is possible that data from different days are used for adjacent pixels, each representing different measurement conditions. If a pixel had no useful measurements during the 16-day period, historic data are used as fill values (Didan et al., 2015). For terrestrial targets, NDVI will take values near 0.8 for vegetated areas and near 0 for barren soil (Huete et al., 1999). The high-resolution dataset was used to calculate the percentage of barren land in each model grid cells, and this percentage was used to define the effective strength of dust sources in each cell.
2.3 Evaluation datasets and metrics
Model evaluation is carried out with two datasets. First, the MODIS monthly aerosol optical depth (AOD) is use to study the spatial distribution of dust in the model domain. For this we use the Level 3 gridded atmosphere monthly product at 1×1 resolution, MOD08_ME (Platnick et al., 2017). Secondly, we evaluate model performance using AERONET AOD retrievals at eight photometric stations. AERONET is a network of sun or sky photometers that derive aerosol optical and microphysical properties at a large number of stations around the world (Holben et al., 1998). For this evaluation, we use Version 3 AOD retrievals that, in comparison with previous versions, improve automatic cloud screening (Giles et al., 2018). Level 2 datasets were used for all stations apart from Kuwait University, where only Level 1.5 data were available. Both model and AERONET AODs were calculated at 532 nm; this was chosen to facilitate future intercomparing with lidar systems that frequently measure at this wavelength (e.g., Pappalardo et al., 2014). AERONET measurements were converted to this wavelength using the 440–870 Ångström exponent and taking into account AOD measurements at 440, 675, and 870 nm; in the cases where the 440 nm AOD was not available, the 500 nm (Mezaira) or 443 nm (KAUST campus) measurement was used instead.
We evaluation model performance using five metrics: mean bias, root mean square error, correlation coefficient, mean fractional bias, and fractional gross error. Concretely, assuming we have n pairs of model values (mi) and observations (oi), the mean bias (MB) is defined as
where the bar denotes the mean value. The root mean square error (RMSE) is defined as
The correlation coefficient (r) is defined as
The fractional gross error (FGE) is defined as
following Boylan and Russell (2006). Similarly, mean fractional bias (MFB) is defined as
following Chang and Hanna (2004).
The test simulation period is 1–31 August 2016 and the results from both simulations are compared to MODIS and AERONET AOD. A 5-day spin-up model run, prior to the experimental period, is used for establishing the dust background over the domain. After finalizing the experimental model configuration, we perform a complete 1-year run (2016) and evaluate the results against AERONET stations.
3.1 Dust transport during August 2016
The selected 1-month period is characterized by a significant variability in wind speeds and directions (Fig. 3), which allows the evaluation of the new model version under different conditions. During 1–10 August, east winds prevail over the region and increased dust concentrations are found mostly along the central, east and south coastal areas of the Arabian Peninsula. An anticyclonic circulation is established during 10–15 August over the Arabian Desert and increased dust concentrations are mostly found over the central desert areas. On 16–26 August the circulation is mainly from northerly directions and thick dust plumes are advected southwards towards the Arabian Sea. The north winds veer east on 26–31 August, and increased dust loads are found over the Gulf on these dates.
3.2 Comparison with MODIS and AERONET
The monthly average AOD for August 2016 is shown in Fig. 4 for the two experimental runs (Fig. 4a, b). The DREAM-NDVI run results in a significantly modified spatial distribution of dust presenting increased dust loads over the entire domain and most profoundly over the Red Sea and Gulf regions (Fig. 4b). This dust pattern is closer to the MODIS-observed AOD over the same period that is shown in Fig. 4c. The MODIS AOD in this area is mostly related to dust; however, it must be taken into account that other aerosols not parameterized in the model (e.g., sea salt, sulfates, nitrates) may also contribute to the observed MODIS AOD.
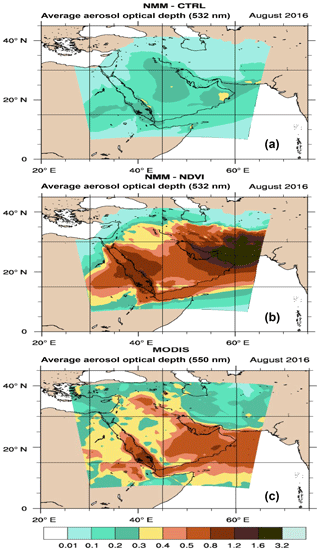
Figure 4Monthly average simulated AOD during August 2016 from the DREAM-CTRL run (a), the DREAM-NDVI run (b) and (c) MODIS.
The first step is to examine how our methodology compares against the monthly average AOD in our study area. Therefore, the monthly average AOD values produced from our two simulations (DREAM-NDVI run and DREAM-CTRL run) are compared. More specifically, the DREAM-NDVI run reproduces the MODIS-observed AOD pattern that is in general characterized by values of 0.3–0.4 in the NW parts of the Arabian Peninsula and by values of 0.4–0.8 in the SE parts. Significant improvement is also evident over the Red Sea and NE Africa. The DREAM-NDVI run captures the maximum observed AOD values reaching up to 1.6 over the Red Sea and also the southwesterly extension of an AOD tongue of 0.3–0.8 towards Sudan. In the eastern parts of the modeling domain, the DREAM-NDVI run again outperforms the DREAM-CTRL run since it reproduces the spatial distribution of AOD 0.4–0.8 over the Arabian Sea and the maximum of 0.8–1.2 at the SE edge of Arabian Peninsula. Over the Gulf, the NDVI run correctly represents the 0.4–0.8 AOD but the dust concentration is overpredicted at the Strait of Hormuz and along the Iran–Pakistan coastline. This is mostly due to the prevailing NE winds during the last days of the August 2016 modeling period and due to a possible misclassification of Iran and Pakistan grid points as effective dust sources, thus favoring unrealistic southeasterly transport towards the Gulf of Oman. The DREAM-NDVI AOD is also higher than MODIS AOD over western Saudi Arabia, indicating a possible overprediction of dust sources in this area.
As a second step we run the same model configurations (CTRL and NDVI) for the entire 2016. The modeled dust optical depth is compared with individual AERONET measurements. The model retrievals are interpolated in time to match the AERONET measurement time considering only dust-relevant measurements with an Ångström coefficient <0.6 (Holben et al., 1998), and the results are shown in Table 1. For completeness we first consider all AERONET stations inside the modeling domain for the evaluation. However the stations that are at the margins of our domain (Cairo_EMA_2, SEDE_BOKER, AgiaMarina_Xyliatou, and El_Farafra) are also affected by other dust source areas (e.g., the Sahara) and their statistics are not representative for Arabian and Middle Eastern sources. Instead, the comparison with Arabian Peninsula stations (Eilat, Kuwait_University, KAUST_Campus, and Mezaira) provides more insight on the effects of the new source characterization. As seen in Fig. 5 and also in Table 2, these stations clearly benefited from the experimental run.
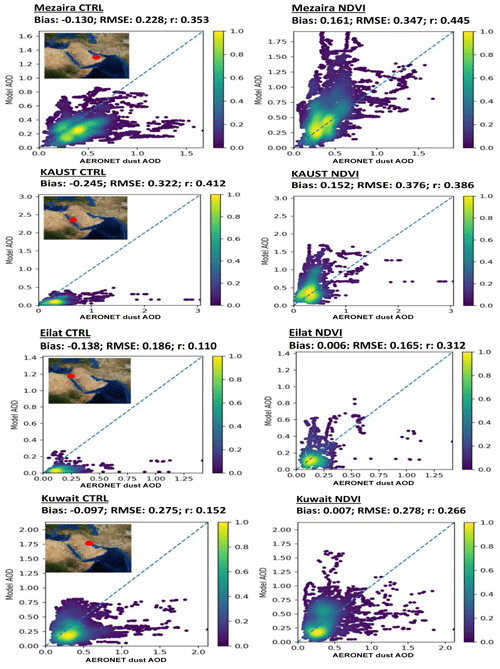
Figure 5Density scatterplots of modeled and AERONET dust AOD at the stations of Mezaira, KAUST, Eilat, and Kuwait for 2016.
Table 1Statistical metrics from the comparison between the annual runs and AERONET.
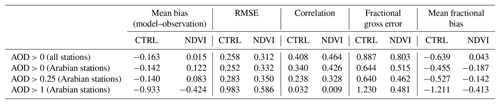
The AERONET stations used in this study are Eilat (29∘ N, 34∘ E), Cairo_EMA_2 (30∘ N, 31∘ E), Kuwait_University (29∘ N, 47∘ E), KAUST_Campus (22∘ N, 39∘ E), SEDE_BOKER (30∘ N, 34∘ E), AgiaMarina_Xyliatou (35∘ N, 33∘ E), Mezaira (23∘ N, 53∘ E), and El_Farafra (27∘ N, 27∘ E).
Table 2Statistical metrics from the annual runs (2016) at AERONET stations. Bold values indicate a correlation coefficient with p<0.01.
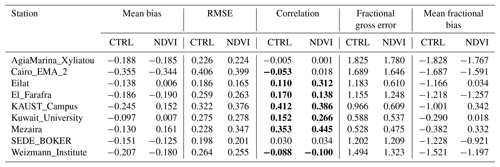
In general the two runs present a significant statistical difference and, more remarkably, a reverse of bias (MODEL-AERONET) from negative in the DREAM-CTRL run to positive in the DREAM-NDVI run. The DREAM-NDVI run produces increased AODs that are neither linearly proportional to the DREAM-CTRL run AODs nor uniformly distributed over the domain. When considering only Arabian stations, the statistical metrics in Table 1 and especially the fractional gross error and bias are improved but the RMSE is increased due to the increase in maximum modeled AODs. In order to investigate the sensitivity of our results towards the severity of dust events we further assume two additional air quality states in Table 1: (i) dust events (AOD >0.25) and (ii) severe dust episodes (AOD >1). Both cases show an improvement in the bias values over the control simulations. When we consider AOD >1, the DREAM-NDVI run still underestimates the observed values but with a lower RMSE (0.586 versus 0.983 of the DREAM-CTRL run). This is clearly evident in Fig. 6, where the NDVI run is indeed more realistic for the Arabian stations but still does not reproduce the extreme AOD during severe episodes. For most of the cases, such high AODs should be attributed to dust storms from convective downdrafts (haboobs). These processes are not resolved at mesoscale model resolutions (Solomos et al., 2012, 2017; Vukovic et al., 2014) and thus cannot be represented here.
In this study we present the development of a dynamic dust source map for implementation in NMME-DREAM v1.0 over the Arabian Peninsula and the greater areas of the Middle East, SW Asia, and NE Africa. Although the major dust sources worldwide are located in permanent deserts where the NDVI is almost always <0.1 (e.g., Bodélé Depression, Gobi, Arabian Desert), the dynamical scaling of dust emissions presented here can be important for providing up-to-date evidence of active dust sources over nonpermanent deserts. These may include dried bogs, marshes, and semidesert areas as well as irrigated and nonirrigated farms where land use changes occur throughout the year. Analysis of the modeling results for a 1-year test period (2016) over SW Asia indicated the improved performance of the new parameterization. The DREAM-NDVI run showed a significant increase in dust loads over the greater Arabian Peninsula area and a more realistic representation of the spatial distribution of AOD compared to the corresponding MODIS satellite retrievals. These findings support the previous results by Kim et al. (2013), who also showed an increase in dust emissions and a more realistic comparison with satellite observations in Saudi Arabia through the introduction of an NDVI-based dynamic source mapping for the GOCART model. Comparison with AERONET measurements also showed significant improvement especially at higher AODs that are also relevant to the model efficiency for air quality purposes (i.e., the model bias is reduced from −0.140 to 0.083 at AOD >0.25 and from −0.933 to −0.424 at AOD >1). However, the model statistics are not improved for all AERONET measuring stations and for all air quality states (Table 2), mainly due to a possible misclassification of dust sources in the highlands of Iran and Pakistan.
The main purpose of our work was the development and first testing of this new modeling version. A major advance of our study is the ability to implement the real-time properties of dust sources in air quality simulations (as represented by the satellite NDVI) and thus capture local or seasonal effects. In general, 1 year is not sufficient for extracting robust statistical results and further analysis is required to examine the performance of the proposed methodology over longer time periods and also over different areas worldwide. For example the simple approach of employing a uniform value of NDVI <0.1 for determining the active dust sources may not be adequate to represent fine-scale land properties, and further adjustments may be required depending on local-scale characteristics. This new approach for the dynamic characterization of active dust sources based on NDVI can be easily implemented in other atmospheric dust models at different configurations and spatial coverage to improve their performance.
All code and data used in this study are available upon request.
SS contributed to the conceptualization, formal analysis, investigation, methodology, project administration, resources, software, validation, visualization, writing of the original draft, review, and editing. AA contributed to the conceptualization, funding acquisition, project administration, supervision, writing, review, and editing. CS contributed to software and data curation, visualization, writing, review, and editing. IB contributed to conceptualization, formal analysis, software, writing, review, and editing. SN contributed to methodology, supervision, writing, review, and editing.
The authors declare that they have no conflict of interest.
This work was funded by a grant from the National Space Science and
Technology Center of the United Arab Emirates University under grant number
NSS Center 7-2017. The authors also acknowledge support from the BEYOND Centre of
Excellence (FP7-REGPOT-2012-2013-1, grant agreement no. 316210) for providing
financial support and computing resources.
Edited by: Samuel Remy
Reviewed by: Enric Terradellas, Sagar Parajuli, and one anonymous referee
Almazrouia, M., NazrulIslama, M., Jonesa, P. D., Athara, H., and AshfaqurRahmana, M.: Recent climate change in the Arabian Peninsula: Seasonal rainfall and temperature climatology of Saudi Arabia for 1979–2009, Atmos. Res., 111, 29–45, 2012.
Anderson, J., Hardy, E., Roach, J., and Witmer, R.: A land use and land cover classification system for use with remote sensing data, U.S. Geological Survey, United States Government Printing Office, Washington, USGS Professional Paper 964, 1976.
Anisimov, A., Tao, W., Stenchikov, G., Kalenderski, S., Prakash, P. J., Yang, Z.-L., and Shi, M.: Quantifying local-scale dust emission from the Arabian Red Sea coastal plain, Atmos. Chem. Phys., 17, 993–1015, https://doi.org/10.5194/acp-17-993-2017, 2017.
Boylan, J. W. and Russell, A. G.: PM and light extinction model performance metrics, goals, and criteria for three-dimensional air quality models, Atmos. Environ., 40, 4946–4959, https://doi.org/10.1016/j.atmosenv.2005.09.087, 2006.
Brown, M. E., Pinzon, J. E., Didan, K., Morisette, J. T., and Tucker, C. J.: Evaluation of the consistency of long-term NDVI time series derived from AVHRR, SPOT-Vegetation, SeaWIFS, MODIS and Land-SAT ETM+, IEEE T. Geosci. Remote, 44, 1787–1793, 2006.
Chakraborty, A., Behera, S. K., Mujumdar, M., Ohba, R., and Yamagata, T.: Diagnosis of tropospheric moisture over Saudi Arabia and influences of IOD and ENSO, Mon. Weather Rev., 134, 598–617, 2006.
Chang, J. C. and Hanna, S. R.: Air quality model performance evaluation, Meteorol. Atmos. Phys., 87, 167–196, https://doi.org/10.1007/s00703-003-0070-7, 2004.
DeFries, R. S. and Townshend, J. R. G.: NDVI-derived land cover classifications at a global scale, Int. J. Remote Sens., 15, 3567–3586, https://doi.org/10.1080/01431169408954345, 1994.
Didan, K. : MOD13A1 MODIS/Terra Vegetation Indices 16-Day L3 Global 500m SIN Grid V006 [Data set], NASA EOSDIS LP DAAC, https://doi.org/10.5067/MODIS/MOD13A1.006, 2015.
Didan, K., Munoz, A. B., Solano, R., and Huete, A.: MODIS Vegetation Index User's Guide (MOD13 Series); Version3.0; University of Arizona, Tucson, AZ, USA, 2015.
Esmaeil, N., Gharagozloo, M., Rezaei, A., and Grunig, G.: Dust events, pulmonary diseases and immune system, American Journal of Clinical and Experimental Immunology, 3, 20–29, 2014.
Georgi, F.: A particle dry-deposition parameterization scheme for in tracer transport models, J. Geophys. Res., 91, 9794–9806, 1986.
Giles, D. M., Sinyuk, A., Sorokin, M. G., Schafer, J. S., Smirnov, A., Slutsker, I., Eck, T. F., Holben, B. N., Lewis, J. R., Campbell, J. R., Welton, E. J., Korkin, S. V., and Lyapustin, A. I.: Advancements in the Aerosol Robotic Network (AERONET) Version 3 database – automated near-real-time quality control algorithm with improved cloud screening for Sun photometer aerosol optical depth (AOD) measurements, Atmos. Meas. Tech., 12, 169–209, https://doi.org/10.5194/amt-12-169-2019, 2019.
Ginoux, P., Chin, M., Tegen, I., Prospero, J. M., Holben, B., Dubovik, O., and Lin, S.-J.: Sources and distributions of dust aerosols simulated with the GOCART model, J. Geophys. Res., 106, 20255–20273. https://doi.org/10.1029/2000JD000053, 2001.
Ginoux, P., Prospero, J. M., Gill, T. E., Hsu, N. C., and Zhao, M.: Global-scale attribution of anthropogenic and natural dust sources and their emission rates based on MODIS Deep Blue aerosol products, Rev. Geophys., 50, RG3005, https://doi.org/10.1029/2012RG000388, 2012.
Holben, B. N., Eck, T. F., Slutsker, I., Tanre, D., Buis, J. P., Setzer, A., Vermote, E., Reagan, J. A., Kaufman, Y., Nakajima, T., Lavenu, F., Jankowiak, I., and Smirnov, A.: AERONET – a federated instrument network and data archive for aerosol characterization, Remote Sens. Environ., 66, 1–16, 1998.
Huete, A. R., Justice, C., and van Leeuwen, W., MODIS Vegetation index (MOD 13): Algorithm Theoretical Basis Document, NASA Goddard Space Flight Center, Greenbelt, Maryland 20771, 1999.
Huete, A., Didan, K., Miura, T., Rodriguez, E. P., Gao, X., and Ferreira, L. G.: Overview of the radiometric and biophysical performance of the MODIS vegetation indices, Remote Sens. Environ., 83, 195–213, 2002.
Janjic, Z. I., Gerrity Jr., J. P., and Nickovic, S.: An Alternative Approach to Nonhydrostatic Modeling, Mon. Weather Rev., 129, 1164–1178, 2001.
Kim, D., Chin, M., Bian, H., Tan, Q., Brown, M. E., Zheng, T., You, R., Diehl, T., Ginoux, P., and Kucsera, T.: The effect of the dynamic surface bareness on dust source function, emission, and distribution, J. Geophys. Res.-Atmos., 118, 871–886, https://doi.org/10.1029/2012JD017907, 2013.
Kumar, P., Sokolik, I. N., and Nenes, A.: Measurements of cloud condensation nuclei activity and droplet activation kinetics of fresh unprocessed regional dust samples and minerals, Atmos. Chem. Phys., 11, 3527–3541, https://doi.org/10.5194/acp-11-3527-2011, 2011.
Mahowald, N., Albani, S., Kok, J. F., Engelstaeder, S., Scanza, R., Ward, D. S., and Flanner, M. G.: The size distribution of desert dust aerosols and its impact on the Earth system, Aeolian Res., 15, 53–71, https://doi.org/10.1016/j.aeolia.2013.09.002, 2014
Mamouri, R.-E., Ansmann, A., Nisantzi, A., Solomos, S., Kallos, G., and Hadjimitsis, D. G.: Extreme dust storm over the eastern Mediterranean in September 2015: satellite, lidar, and surface observations in the Cyprus region, Atmos. Chem. Phys., 16, 13711–13724, https://doi.org/10.5194/acp-16-13711-2016, 2016.
Mitsakou, C., Kallos, G., Papantoniou, N., Spyrou, C., Solomos, S., Astitha, M., and Housiadas, C.: Saharan dust levels in Greece and received inhalation doses, Atmos. Chem. Phys., 8, 7181–7192, https://doi.org/10.5194/acp-8-7181-2008, 2008.
Nickovic, S., Kallos, G., Papadopoulos, A., and Kakaliagou, O.: A model for prediction of desert dust cycle in the atmosphere, J. Geophys. Res., 106, 18113–18129, 2001.
Nickovic, S., Cvetkovic, B., Madonna, F., Rosoldi, M., Pejanovic, G., Petkovic, S., and Nikolic, J.: Cloud ice caused by atmospheric mineral dust – Part 1: Parameterization of ice nuclei concentration in the NMME-DREAM model, Atmos. Chem. Phys., 16, 11367–11378, https://doi.org/10.5194/acp-16-11367-2016, 2016.
Olson, J. S., Watts, J. A., and Allison, L. J.: Carbon in Live Vegetation of Major World Ecosystems, Oak Ridge National Laboratory, Oak Ridge, Tennessee, USA, Report ORNL-5862, 1983.
Pappalardo, G., Amodeo, A., Apituley, A., Comeron, A., Freudenthaler, V., Linné, H., Ansmann, A., Bösenberg, J., D'Amico, G., Mattis, I., Mona, L., Wandinger, U., Amiridis, V., Alados-Arboledas, L., Nicolae, D., and Wiegner, M.: EARLINET: towards an advanced sustainable European aerosol lidar network, Atmos. Meas. Tech., 7, 2389–2409, https://doi.org/10.5194/amt-7-2389-2014, 2014.
Parajuli, S. P. and Zender, C. S.: Connecting geomorphology to dust emission through high-resolution mapping of global land cover and sediment supply, Aeolian Res., 27, 47–65, https://doi.org/10.1016/j.aeolia.2017.06.002, 2017.
Pejanovic, G., Nickovic, S., Vujadinovic, M., Vukovic, A., Djurdjevic, V., and Dacic, M.: Atmospheric deposition of minerals in dust over the open ocean and possible consequences on climate, WCRP OSC Climate Research in Service to Society, 24–28 October 2011, Denver, CO, USA, 2011.
Pérez, C., Nickovic, S., Baldasano, J. M., Sicard, M., Rocadenbosch, F., and Cachorro, V. E.: A long Saharan dust event over the western Mediterranean: Lidar, Sun photometer observations, and regional dust modeling, J. Geophys. Res., 111, D15214, https://doi.org/10.1029/2005JD006579, 2006.
Platnick, S., King, M. D., Meyer, K. G., Wind, G., Amarasinghe, N., Marchant, B., Arnold, G. T., Zhang, Z., Hubanks, P. A., Ridgway, B., and Riedi, J. : MODIS Atmosphere L3 Monthly Product. NASA MODIS Adaptive Processing System, Goddard Space Flight Center, USA, https://doi.org/10.5067/MODIS/MOD08_M3.061, 2017.
Prospero J. M., Ginoux, P., Torres, O., Nicholson, S. E., and Gill, T. E.: Environmental characterization of global sou7rces of atmospheric soil dust identified with the nimbus 7 total ozone mapping spectrometer (TOMS) absorbing aerosol product, Rev. Geophys., 40, 1002, https://doi.org/10.1029/2000RG000095, 2002.
Rouse Jr., R. H., Haas, J. A., Schell, and Deering, D. W.: Monitoring vegetation systems in the Great Plains with ERTS, NASA. Goddard Space Flight Center 3d ERTS-1 Symp., Vol. 1, Sect. A, 309–317, 1974.
Solano, R., Didan, K., Jacobson, A., and Huete, A.: MODIS Vegetation Index User's Guide, ver. 2.0, Vegetation Index and Phenology Lab, The University of Arizona, available at: https://vip.arizona.edu/documents/MODIS/ (last access: 27 February 2019), 2010.
Solomos, S., Kallos, G., Kushta, J., Astitha, M., Tremback, C., Nenes, A., and Levin, Z.: An integrated modeling study on the effects of mineral dust and sea salt particles on clouds and precipitation, Atmos. Chem. Phys., 11, 873–892, https://doi.org/10.5194/acp-11-873-2011, 2011.
Solomos, S., Ansmann, A., Mamouri, R.-E., Binietoglou, I., Patlakas, P., Marinou, E., and Amiridis, V.: Remote sensing and modelling analysis of the extreme dust storm hitting the Middle East and eastern Mediterranean in September 2015, Atmos. Chem. Phys., 17, 4063–4079, https://doi.org/10.5194/acp-17-4063-2017, 2017.
Spyrou, C.: Direct radiative impacts of desert dust on atmospheric water content, Aerosol Sci. Tech., 52, 693–701, https://doi.org/10.1080/02786826.2018.1449940, 2018.
Spyrou, C., Kallos, G., Mitsakou, C., Athanasiadis, P., Kalogeri, C., and Iacono, M. J.: Modeling the radiative effects of desert dust on weather and regional climate, Atmos. Chem. Phys., 13, 5489–5504, https://doi.org/10.5194/acp-13-5489-2013, 2013.
Spyrou, C., Mitsakou, C., Kallos, G., Louka, P., and Vlastou, G.: An improved limited area model for describing the dust cycle in the atmosphere, J. Geophys. Res., 115, D17211, https://doi.org/10.1029/2009JD013682, 2010.
Torge, A., Macke, A., Heinold, B., and Wauer, J.: Solar radiative transfer simulations in Saharan dust plumes: particle shapes and 3-D effect, Tellus B, 63, 770–780, https://doi.org/10.1111/j.1600-0889.2011.00560.x, 2011.
Tucker, C. J., Pinzon, J. E., Brown, M. E., Slayback, D., Pak, E. W., Mahoney, R., Vermote, E., and El Saleous, N.: An extended AVHRR 8-km NDVI data set compatible with MODIS and SPOT vegetation NDVI data, Int. J. Remote Sens., 26, 4485–4498, 2005.
Vukovic, A., Vujadinovic, M., Pejanovic, G., Andric, J., Kumjian, M. R., Djurdjevic, V., Dacic, M., Prasad, A. K., El-Askary, H. M., Paris, B. C., Petkovic, S., Nickovic, S., and Sprigg, W. A.: Numerical simulation of “an American haboob”, Atmos. Chem. Phys., 14, 3211–3230, https://doi.org/10.5194/acp-14-3211-2014, 2014.
Walko, R. L., Band, L. E., Baron, J., Kittel, T. G. F., Lammers, R., Lee, T. J., Ojima, D., Pielke Sr., R. A., Taylor, C., Tague, C., Tremback, C. J., and Vidale, P. J.: Coupled atmosphere-biophysicshydrology models for environmental modeling, J. Appl. Meteorol., 39, 931–944, 2000.
Xue Y., Sellers P. J., Kinter J. L., and Shukla J.: A simplified biosphere model for global climate studies, J. Climate, 4, 345–364, 1991.