the Creative Commons Attribution 4.0 License.
the Creative Commons Attribution 4.0 License.
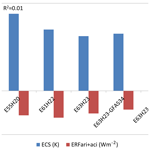
The global aerosol–climate model ECHAM6.3–HAM2.3 – Part 2: Cloud evaluation, aerosol radiative forcing, and climate sensitivity
David Neubauer
Sylvaine Ferrachat
Colombe Siegenthaler-Le Drian
Philip Stier
Daniel G. Partridge
Ina Tegen
Isabelle Bey
Tanja Stanelle
Harri Kokkola
Ulrike Lohmann
The global aerosol–climate model ECHAM6.3–HAM2.3 (E63H23) as well as the previous model versions ECHAM5.5–HAM2.0 (E55H20) and ECHAM6.1–HAM2.2 (E61H22) are evaluated using global observational datasets for clouds and precipitation. In E63H23, the amount of low clouds, the liquid and ice water path, and cloud radiative effects are more realistic than in previous model versions. E63H23 has a more physically based aerosol activation scheme, improvements in the cloud cover scheme, changes in the detrainment of convective clouds, changes in the sticking efficiency for the accretion of ice crystals by snow, consistent ice crystal shapes throughout the model, and changes in mixed-phase freezing; an inconsistency in ice crystal number concentration (ICNC) in cirrus clouds was also removed. Common biases in ECHAM and in E63H23 (and in previous ECHAM–HAM versions) are a cloud amount in stratocumulus regions that is too low and deep convective clouds over the Atlantic and Pacific oceans that form too close to the continents (while tropical land precipitation is underestimated). There are indications that ICNCs are overestimated in E63H23.
Since clouds are important for effective radiative forcing due to aerosol–radiation and aerosol–cloud interactions (ERFari+aci) and equilibrium climate sensitivity (ECS), differences in ERFari+aci and ECS between the model versions were also analyzed. ERFari+aci is weaker in E63H23 (−1.0 W m−2) than in E61H22 (−1.2 W m−2) (or E55H20; −1.1 W m−2). This is caused by the weaker shortwave ERFari+aci (a new aerosol activation scheme and sea salt emission parameterization in E63H23, more realistic simulation of cloud water) overcompensating for the weaker longwave ERFari+aci (removal of an inconsistency in ICNC in cirrus clouds in E61H22).
The decrease in ECS in E63H23 (2.5 K) compared to E61H22 (2.8 K) is due to changes in the entrainment rate for shallow convection (affecting the cloud amount feedback) and a stronger cloud phase feedback.
Experiments with minimum cloud droplet number concentrations (CDNCmin) of 40 cm−3 or 10 cm−3 show that a higher value of CDNCmin reduces ERFari+aci as well as ECS in E63H23.
- Article
(19676 KB) - Full-text XML
- Companion paper
-
Supplement
(6105 KB) - BibTeX
- EndNote
Clouds are the largest modulators of radiation in Earth's atmosphere. Cloud hydrometeors are generally shorter lived than other modulators of radiation in the atmosphere like aerosol particles, greenhouse gases, or changes in surface albedo through changes in land use. Also, the spatial structure of multiple clouds shows a large variability on different scales as it depends not only on large-scale motions of the air but also on convective and turbulent motions at different scales. These convective and turbulent motions in turn are driven in large part by diabatic heating (and cooling) and radiative cooling (and heating) involving cloud and precipitation hydrometeors, leading to a tight coupling between clouds and circulation (e.g., Wood, 2012; Voigt et al., 2014; Vial et al., 2016). The range of microphysical properties of cloud droplets and ice crystals adds to the complexity of clouds in Earth's atmosphere. This complexity makes clouds difficult to observe and to simulate using models, substantially contributing to the current large uncertainties in future climate projections. Therefore, it is necessary to have an increasingly realistic representation of clouds in global climate models to be able to study past and present climate forcings and to strengthen the reliability of climate projections. It is crucial to evaluate clouds in these models with reliable observations and account for the complexity in clouds in the process.
In this study we use current satellite products to evaluate the aerosol–climate model ECHAM6.3–HAM2.3 and the two precursor model versions ECHAM6.1–HAM2.2 and ECHAM5.5–HAM2.0. One problem in using satellite products is that they are produced with retrieval algorithms that have to make assumptions about the nature of the clouds (e.g., assumptions about the vertical cloud profile; Miller et al., 2016) (and other modulators of radiation) that will not always fit optimally for every cloud in the observed satellite pixels. Accordingly, current satellite products include measures of uncertainty in the retrieved cloud properties. We use these uncertainty measures to limit the evaluation only to regions where the observations are reliable. Furthermore, we apply the CFMIP (Cloud Feedback Model Intercomparison Project) Observation Simulator Package (COSP) where appropriate to account for limitations in the satellite observations (e.g., clouds cannot be observed below the level of full lidar signal attenuation by spaceborne lidar; Chepfer et al., 2010) and the different scales of the model grid compared to the satellite data as well as to ensure a comparison of exactly the same variables in the model output as in the satellite products.
To further limit the impact of observational uncertainties we use several products from independent instruments and aim to identify model biases in several of them. We also perform some of the analyses for different regions to study biases for different cloud types.
For studying past and present climate forcings it is indispensable to constrain the effective anthropogenic aerosol forcing due to aerosol–radiation and aerosol–cloud interactions (ERFari+aci). Because of the large impact of clouds on radiation, the representation of clouds in a global model can have an impact on ERFari+aci. Therefore, we also investigate the difference in ERFari+aci in the three ECHAM–HAM model versions and how they compare to differences in the simulations of clouds. As cloud feedbacks will have a large impact on temperature in a warmer climate we compare the equilibrium climate sensitivity (ECS) and cloud feedbacks of the different model versions.
Section 2 gives a short description of the representation of clouds in ECHAM6.3–HAM2.3 and of the observational products applied in the model evaluation. In Sect. 3 the results of the cloud evaluation and the comparison of ERFari+aci, RFari, and ECS in the ECHAM–HAM model versions are presented and discussed. The results are summarized in Sect. 4 and conclusions are drawn.
2.1 Model description
The global aerosol–climate model ECHAM–HAM is a combination of the global climate model ECHAM with the aerosol microphysics module HAM (Stier et al., 2005). The ECHAM5 and ECHAM6 model versions used in this study are described in Roeckner et al. (2003) and Stevens et al. (2013), respectively. The ECHAM–HAM model versions and configurations used in this study are described in separate studies: ECHAM5.5–HAM2.0 in Zhang et al. (2012), ECHAM6.1–HAM2.2 in Neubauer et al. (2014), and ECHAM6.3–HAM2.3 in Tegen et al. (2019). For the sake of brevity, in the following ECHAM5.5–HAM2.0 will be referred to as E55H20, ECHAM6.1–HAM2.2 as E61H22, and ECHAM6.3–HAM2.3 as E63H23. In contrast to the one-moment cloud microphysics scheme in the ECHAM base model (Lohmann and Roeckner, 1996), ECHAM–HAM uses a two-moment cloud microphysics scheme. The two-moment cloud microphysics scheme is described in Lohmann et al. (2007) and Lohmann and Hoose (2009), with recent changes and improvements applied in E63H23 in Lohmann and Neubauer (2018). A two-moment cloud microphysics scheme is required to study aerosol–cloud interactions. In ECHAM–HAM cloud droplet activation and ice crystal nucleation from cloud condensation nuclei and ice-nucleating particles are computed along with in-cloud and below-cloud scavenging. Therefore, ECHAM–HAM simulates aerosol–cloud interactions in liquid, mixed-phase, and ice clouds. However, a two-moment cloud microphysics scheme is not only a prerequisite for simulating aerosol–cloud interactions, but the additional information from the prognostic cloud droplet and ice crystal number concentrations can also improve the simulation of clouds compared to a one-moment cloud microphysics scheme. The general representation of clouds in ECHAM–HAM is described in the literature given in this section but is briefly repeated in the subsections below for the convenience of the reader.
2.1.1 Liquid stratiform clouds and wet scavenging
The scheme for stratiform clouds comprises prognostic variables for water vapor, cloud liquid and cloud ice, a cloud microphysics scheme, and a diagnostic cloud cover scheme (based on Sundqvist et al., 1989). Cloud microphysics is represented by a two-moment scheme described in Lohmann et al. (2007), Lohmann and Hoose (2009), and Lohmann and Neubauer (2018). Optionally available but not used in this study is the one-moment scheme by Lohmann and Roeckner (1996). In ECHAM6.3 changes were made in the diagnostic cloud cover scheme to enhance the cloud cover for marine stratocumulus clouds (Mauritsen et al., 2019). Condensation of cloud liquid water is based on moisture convergence (from transport by advection, turbulence, and convection) and subsequent saturation adjustment. Evaporation of cloud liquid water (or sublimation of cloud ice) occurs when the cloud cover decreases or by the transport of cloud liquid (or ice) mass into the cloud-free part of a grid box. For aerosol activation in liquid clouds the Köhler-theory-based Abdul-Razzak and Ghan (2000) scheme is used. Its implementation is described in Stier (2016). Optionally available is the Lin and Leaitch (1997) aerosol activation scheme. Precipitation is computed diagnostically. The autoconversion of cloud droplets to rain follows Khairoutdinov and Kogan (2000). The accretion of cloud droplets by rain (Khairoutdinov and Kogan, 2000) and evaporation of rain below clouds (based on Rotstayn, 1997) are also computed. Size-dependent wet scavenging of aerosol particles in-cloud and below-cloud follows Croft et al. (2009, 2010). The below-cloud collection efficiencies as a function of aerosol and raindrop or snow crystal size are read from a lookup table. The in-cloud scavenging scheme takes the nucleation scavenging and impaction scavenging of aerosol particles with cloud droplets and ice crystals into account. For nucleation scavenging the number of scavenged aerosol particles is computed for liquid cloud droplets from the cloud droplet number concentration (CDNC) (after the computation of cloud droplet evaporation and precipitation formation), and the fraction of activated aerosol particles (computed by the activation scheme). For ice crystals the aerosol particles are scavenged progressively from the largest to the smallest modes until the number concentration of scavenged aerosol particles is equal to the ice crystal number concentration (ICNC) (after the computation of ice crystal sublimation and precipitation formation) of the grid box.
A downward scavenging tracer flux is computed for each model column each model time step. In-cloud and below-cloud scavenging are sources for the downward scavenging tracer flux, while the evaporation and sublimation of precipitation are sinks for the downward scavenging tracer flux. When the sink term is larger than the source term of the downward scavenging tracer flux in a model level, aerosol mass and number concentrations will be transferred to the respective atmospheric tracers; i.e., aerosol is released from evaporating–sublimating precipitation at this model level back to the atmosphere.
2.1.2 Mixed-phase and cirrus stratiform clouds
The cirrus scheme follows Kärcher and Lohmann (2002) and details are given in Lohmann et al. (2008). The ice crystals in cirrus clouds form by homogenous nucleation of supercooled liquid droplets. The scheme by Joos et al. (2010) for orographic cirrus clouds can optionally be applied to account for the higher updraft velocities in orographic cirrus clouds but was not used in this study. Supersaturation with respect to ice is allowed for cirrus clouds, and therefore the depositional growth equation is solved for cirrus ice crystals (Kärcher and Lohmann, 2002). For mixed-phase clouds the heterogeneous nucleation of supercooled cloud droplets is computed via immersion and contact freezing following Lohmann and Diehl (2006). Depositional growth of cloud ice in mixed-phase clouds is computed analogous to liquid clouds based on moisture convergence and subsequent saturation adjustment. In addition, the growth of ice crystals at the expense of cloud droplets via the Wegener–Bergeron–Findeisen process (Wegener, 1911; Bergeron, 1935; Findeisen, 1938) is implemented following Korolev (2007). Snow forms by the aggregation of ice crystals, the riming of cloud droplets by snow, and the accretion of ice crystals by snow. Sedimentation of ice crystals follows Rotstayn (1997). The sublimation and melting of ice crystals and snow below clouds is also computed. Ice multiplication via rime splintering (Hallett–Mossop process) following Levkov et al. (1992) is optional (not used in this study).
2.1.3 Convective clouds
The convective parameterization from Tiedtke (1989) with modifications for deep convection from Nordeng (1994) and for the triggering of convection from Stevens et al. (2013) is used. The convective parameterization uses only a one-moment cloud microphysics scheme. Detrained condensate of convective clouds is added to stratiform clouds if they exist at the level of detrainment. Whether the condensate is detrained as liquid or ice is based on the same criteria as in the two-moment stratiform cloud microphysics scheme in ECHAM–HAM. To obtain CDNC for the detrained condensate, several simplifications are applied. It is assumed that cloud droplets of convective clouds will form at cloud base. The number of activated cloud condensation nuclei (CCN) at the convective cloud base is computed using the vertical velocity from large-scale and turbulent fluxes as described in Sect. 2.1.4. It is further assumed that CDNC will be constant throughout the vertical extension of the convective clouds. At the level of detrainment these CDNCs from the convective clouds will either evaporate or be added to stratiform clouds if these exist at the level of detrainment. In the latter case a weighted average of the stratiform CDNC and detrained CDNC is computed by weighting stratiform CDNC with the stratiform liquid water content and detrained CDNC with detrained liquid water content. CDNC of the stratiform cloud is not allowed to decrease by this procedure, since cloud droplets will not evaporate in a supersaturated environment. The detrained ICNC is computed from the temperature-dependent empirical relationship of Boudala et al. (2002). An alternative convection scheme based on the Convective Cloud Field Model (CCFM) (Wagner and Graf, 2010) with representation of aerosol–convection interactions is available (Kipling et al., 2017; Labbouz et al., 2018) but not used in this study.
2.1.4 Other processes
The sulfur cycle model of Feichter et al. (1996) forms the base of the sulfur chemistry module. Three sulfur species are treated prognostically: sulfur dioxide, dimethyl sulfide (DMS), and sulfate (the latter not only in the gas phase but also as an aerosol). Three-dimensional climatological fields for oxidants are used, i.e., ozone (O3), OH, H2O2, NO2, and NO3. The nucleation scheme was implemented by Kazil et al. (2010) and is based on Kazil and Lovejoy (2007). Organic nucleation following Kulmala et al. (2006) or Kuang et al. (2008) can optionally be used. Sea salt, dust, and DMS emissions are computed online based on near-surface wind speeds (Stier et al., 2005; Tegen et al., 2002). The Long et al. (2011) sea salt parameterization (temperature dependent; Sofiev et al., 2011) is used in E63H23 and the Guelle et al. (2001) sea salt parameterization is used in E55H20 and E61H22. Aerosol water uptake is computed via kappa-Köhler theory (Petters and Kreidenweis, 2007) as described in Zhang et al. (2012).
Radiative transfer is computed with the two-stream model PSrad (Pincus and Stevens, 2013). Turbulent fluxes in the atmosphere are computed with the turbulent kinetic energy (TKE) scheme of Brinkop and Roeckner (1995). The subgrid-scale vertical velocity that is needed for many cloud microphysical processes (e.g., cloud droplet activation, ice crystal nucleation, Wegener–Bergeron–Findeisen process) is computed from the TKE (Lohmann et al., 2007). Next to a single characteristic updraft velocity for a grid box that is based on TKE, there is also the option to represent the subgrid-scale variability of updraft velocities by a Gaussian probability density function (PDF) of updraft velocities (West et al., 2014). The subgrid-scale variability is again assumed to be due to turbulence, and the width of the Gaussian PDF is therefore a function of TKE. The impact of the width of the Gaussian PDF on ERFari+aci is discussed in West et al. (2014). The PDF approach by West et al. (2014) is optionally available but not used in this study. The physics part of ECHAM6.3 and the two-moment microphysics scheme for stratiform clouds in E63H23 are now energy conserving.
2.1.5 Changes and improvements in E63H23
Changes and improvements in E63H23 are also described in Lohmann and Neubauer (2018) and Tegen et al. (2019), and they are repeated here shortly for the convenience of the reader. From ECHAM6.1 to ECHAM6.3 the following improvements were made (Mauritsen et al., 2019):
-
new PSrad radiation scheme (Pincus and Stevens, 2013), which uses the Monte Carlo independent column approximation for fractional cloudiness and has the option for spectrally sparse but temporally dense calculations;
-
update of the fractional cloud cover scheme, which improves the low bias of marine stratocumulus clouds (this is motivated by the difficulty of representing the strong inversions of stratocumulus-topped marine boundary layers in global climate models; Mauritsen et al., 2019);
-
update of the land model JSBACH (Reick et al., 2013), which uses a new five-layer soil hydrology scheme; and
-
removal of inconsistencies in the convection scheme, convective detrainment, and the vertical diffusion scheme to conserve the atmospheric energy budget.
The aerosol microphysics scheme HAM2.3 received the following improvements compared to HAM2.0:
-
update of mineral dust emission parameterization, which makes use of a satellite-based source mask for Saharan dust sources (Heinold et al., 2016);
-
new sea salt emission parameterization based on Long et al. (2011), which uses a temperature dependence following Sofiev et al. (2011);
-
the latest version of the sectional aerosol module SALSA2.0 is implemented (described in Kokkola et al., 2018) (not used in this study); and
-
new emission datasets have been made available in an input file distribution for E63H23 for anthropogenic aerosol emissions (Global Fire Assimilation System (GFAS): Kaiser et al., 2012; Community Emissions Data System (CEDS): Hoesly et al., 2018; the latter is not used in this study).
Aerosol–cloud interactions were improved from HAM2.0 to HAM2.3 by the following changes.
-
The Köhler-theory-based Abdul-Razzak and Ghan (2000) cloud droplet activation scheme (described in Stier, 2016) replaces the empirical Lin and Leaitch (1997) activation scheme.
-
The in-cloud scavenging scheme by Croft et al. (2010) combines a diagnostic nucleation scavenging scheme with a size-dependent impaction scavenging parameterization and replaces prescribed (size-dependent) aerosol scavenging fractions.
-
There is a changed treatment of detrained cloud water mass and number concentrations from convective clouds: CDNC from detrained cloud water added (weighted average) to CDNC of a stratiform cloud cannot decrease the CDNC of the stratiform cloud; the split between liquid water and ice of detrained condensate is made consistent between mass and number concentrations.
-
In mixed-phase clouds the heterogeneous freezing by immersion freezing of black carbon particles is limited to particles in the accumulation mode and coarse mode.
The two-moment stratiform cloud microphysics scheme in ECHAM–HAM received the following improvements from Lohmann and Hoose (2009) to E63H23.
-
Ice crystals are assumed to have a shape of hexagonal plates, which covers the whole size range of ice crystals, and the shape is consistent in all modules.
-
Sticking efficiency used in the accretion of ice crystals by snow has been changed to the one used in Seifert and Beheng (2006).
-
Two settings for minimum CDNC are available: 40 cm−3 or 10 cm−3.
Further technical improvements, bug fixes, and minor corrections in E63H23 include the following:
-
removal of an inconsistency in the fractional cloud cover and cloud microphysics schemes in ECHAM6.3, which had led cloud cover to be either 0 or 1 in ECHAM6.1;
-
removal of inconsistencies in the kappa-Köhler water uptake in HAM2.3;
-
modularization of the two-moment stratiform cloud microphysics scheme;
-
removal of an inconsistency for convective detrainment in the two-moment stratiform cloud microphysics scheme to conserve the atmospheric energy budget;
-
removal of an inconsistency in the two-moment stratiform cloud microphysics scheme, which led to homogeneous freezing of dry aerosol particles, independent of availability of water vapor below −35 ∘C;
-
CDNC–ICNC can no longer grow and in the same time step evaporate or sublimate;
-
no more CDNC at temperatures colder than 238.15 K and no more ICNC at temperatures warmer than 273.15 K; and
-
and update of default settings, run templates, and run organization (the vertical resolution is by default 47 vertical model layers; the reference year and reference period for present-day simulations are 2008 and 2003–2012, respectively).
2.2 Experiment description
For each of the three model configurations, E55H20, E61H22, and E63H23, three types of experiments were conducted to evaluate the clouds in the present-day climate, ERFari+aci, and ECS (Table 1). The simulation setup was chosen to be as similar as possible for the three model versions to minimize the impact of boundary conditions on the model version comparison. The setup represents the standard setup of E63H23, which is a compromise between which processes are represented in the model and the computational performance. Climatological monthly mean mixing ratios of oxidants from an 8-year (2003–2010) mean Monitoring Atmospheric Composition and Climate (MACC) reanalysis (Inness et al., 2013) are used in E61H22 and E63H23. For E55H20 the climatological monthly mean mixing ratios of oxidants are from simulations with MOZART (the Model for OZone And Related chemical Tracers) for present-day conditions (Horowitz et al., 2013).
2.2.1 Present-day climate simulation (PD)
The 10-year simulations for PD conditions were done for all model versions. Previous studies using E55H20 or E61H22 often used the year 2000 as the reference year or 2000–2009 as the reference period for present-day simulations (Zhang et al., 2012; Neubauer et al., 2014); therefore, we also use the period 2000–2009 for the PD simulations of E55H20 and E61H22. For E63H23 the default model setup has changed and the reference year and reference period for present-day simulations are now 2008 and 2003–2012, respectively. This has become necessary because of the relatively large changes in aerosol emissions in recent years in several regions (Hoesly et al., 2018) and was aided by the availability of new boundary condition datasets. Time-varying (RCP8.5) ACCMIP MACCity (AeroCom II ACCMIP) aerosol emissions were used for E63H23 and E61H22. The biomass burning emissions are based on observations until 2008 in ACCMIP MACCity, and afterwards the biomass burning emissions are from the RCP8.5 emission scenario. For E55H20 the AeroCom I emissions for the year 2000 are applied for all years. The greenhouse gas concentrations are set to the year 2008 (RCP8.5) concentrations in all model versions. All model versions also use a climatology for monthly values of sea surface temperature (SST) and sea ice cover (SIC) derived from AMIP data (Taylor et al., 2000) of the years 2000–2015. The spectral horizontal resolution is T63 () in all model versions. For E55H20 and E61H22, 31 vertical model layers (L31) are used (as in the default configuration of these ECHAM–HAM model versions), and the model top is 10 hPa. For E63H23, 47 vertical model layers (L47) are used (new default configuration of E63H23), with a model top at 0.01 hPa. The lowermost levels of L31 and L47 (up to about 100 hPa) are identical. A comparison of E63H23 simulations with L31 and L47 showed only minor differences (see Table S1 and Fig. S1 in the Supplement). Therefore, we also expect no large differences by using different vertical grids for the different model versions.
2.2.2 ERFari+aci and RFari simulations (PDaer / PIaer)
To compute ERFari+aci two 20-year simulations were conducted, one with present-day aerosol emissions (PDaer) and one with preindustrial aerosol emissions (PIaer). The simulations were otherwise identical. For the PDaer simulation the PD simulation was extended to cover the years 2000–2002 and 2013–2019 (or the years 2010–2019 for E55H20 and E61H22). The same greenhouse gas concentrations and SST and SIC climatology as in the PD simulations have been used. For the time period 2013–2019 the ACCMIP MACCity (AeroCom-II-ACCMIP) aerosol emissions for the year 2008 were used for all years for E63H23 and E61H22. For E55H20 the AeroCom I emissions for the year 2000 are applied for all years (2000–2019) for the PD simulation. For the PIaer simulation the aerosol emissions for the year 1850 from ACCMIP MACCity (AeroCom-II-ACCMIP) were used for E63H23 and E61H22, and the ones for the year 1750 are from AeroCom I for E55H20. ERFari+aci is computed as the difference in top-of-atmosphere (TOA) net radiative flux (netTOA) between the PDaer and PIaer simulation:
The simulation time was 20 years to increase the signal of ERFari+aci compared to variations in TOA net radiative flux due to the internal variability of the atmosphere. The use of an identical climatology for SST–SIC in all simulations reduces the internal variability compared to simulations with a global climate model (GCM) coupled to a full ocean model.
The radiative forcing due to aerosol–radiation interactions (RFari) is computed from the same pair of simulations as ERFari+aci (PDaer / PIaer). The direct aerosol radiative effect is computed by double calls to the radiation, once with the prognostic aerosol and once without aerosol. RFari is computed as the difference of the direct aerosol radiative effect between the PDaer and the PIaer simulations at TOA, once for all-sky and once for clear-sky (CS) conditions:
Note that we did not follow the protocol in Myhre et al. (2013) for all-sky conditions; therefore, our all-sky RFari is somewhat affected by changes in clouds from preindustrial to present-day simulations. This has no large impact on the regional analysis for RFari our study. The reason why we did not follow Myhre et al. (2013) is that we include indirect aerosol effects in our simulations.
2.2.3 ECS simulations (1×CO2∕2×CO2)
To compute ECS, ECHAM–HAM was coupled to a mixed-layer ocean to compute two 50-year simulations, one with preindustrial CO2 concentrations (1×CO2) and one with doubled preindustrial CO2 concentrations (2×CO2). The first 25 years of the simulations were used as spin-up time for the (50 m deep) mixed-layer ocean. ECS was then computed from the difference in global mean surface temperature (Ts) between 2×CO2 and 1×CO2 from the last 25 years of the simulations:
Preindustrial concentrations for well-mixed greenhouse gases other than CO2 were used in all simulations, as were preindustrial aerosol emissions (similar to PIaer). The ocean heat flux corrections required by the mixed-layer ocean to maintain present-day sea surface temperatures were computed for each model version by extending the respective PDaer simulations another 5 years to a total of 25 years.
2.3 Tuning strategy
Following Hourdin et al. (2017), who argue that estimating uncertain parameters in model development is an important process that should be made transparent, we document our tuning strategies and targets. Tuning is needed mainly to ensure that the TOA radiative fluxes are balanced, and model tuning is limited to adjusting global mean properties. We start from the ECHAM6.3 parameter settings and adapt mainly parameters related to the cloud and convection scheme for tuning E63H23. The tuning strategy and parameters for ECHAM6, as well as the impact of these parameters on the model climate, are described in Mauritsen et al. (2012, 2019). The tuning parameters for ECHAM6–HAM2 and their impact on climate are described in Lohmann and Ferrachat (2010). The parameters that were used in the tuning of the ECHAM–HAM versions and that have different values in E55H20, E61H22, and E63H23 are shown in Table 2.
Table 2Parameter settings for E55H20, E61H22 ,and E63H23. The parameters used to tune the ECHAM–HAM versions are a scaling factor for stratiform rain formation rate by autoconversion (γr), a scaling factor for stratiform snow formation rate by aggregation (γs), critical relative humidity at the surface, which is used in the cloud cover scheme (γc), the entrainment rate for shallow convection (ϵs), the entrainment rate for deep convection (ϵd), the convective conversion rate from cloud water to rain (γcr), an inhomogeneity factor for ice clouds (γi), and the minimum cloud droplet number concentration (CDNCmin).
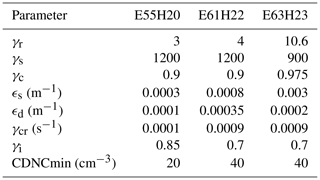
The primary tuning target for E63H23 is to match the global mean observed shortwave (SW) and longwave (LW) TOA fluxes within the range of uncertainty of the observations along with a TOA net radiative imbalance close to the observed present-day value. The secondary tuning target is that the SW, LW, and TOA net cloud radiative effect (CRE) are within the range of uncertainty of the observations. Cloud cover (CC), liquid water path (LWP), ice water path (IWP), total precipitation (P), and aerosol optical depth (AOD) should also be close to the range of observed values (see Table 3).
Table 3Global mean values of the PD simulations. Radiative fluxes are at the top of the atmosphere. Values from observations (OBS) and multi-model means (MMMs) for aerosol burdens are shown next to those of the three model versions. ERFari+aci and ECS are from the PDaer ∕ PIaer and 1xCO2∕2xCO2 simulations, respectively.
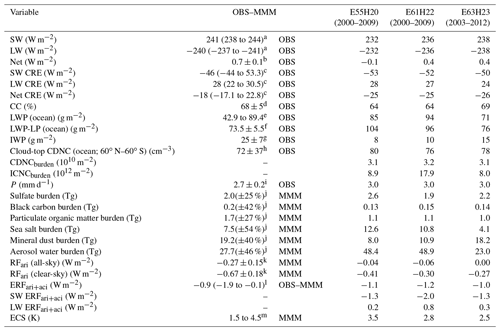
a Central values from Loeb et al. (2018), range from Stevens and
Schwartz (2012). b Loeb et al. (2018) and Johnson et al. (2016). c Central values from Loeb et al. (2018); the range takes into account values
from Loeb et al. (2009) and Matus and L'Ecuyer (2017). d Stubenrauch et
al. (2013). e Platnick et al. (2015, 2017), ATSR2-AATSR v2.0 (Stengel
et al., 2017a; Poulsen et al., 2017), Elsaesser et al. (2017). f Elsaesser et al. (2017). g Li et al. (2012). h Bennartz and Rausch (2017). i Central value from Adler et al. (2018),
uncertainty from
Adler et al. (2012). j Taken from Table 10 of Textor et al. (2006). k Taken from Table 3 of Myhre et al. (2013). l Boucher et al. (2013). m Collins et al. (2013),
Knutti et al. (2017).
The tuning is done with short 1-year simulations with a climatology for SST and sea ice. When a set of parameters has been found, one or more 10-year simulations are done to minimize the uncertainty in TOA net radiative imbalance. For E61H22 the default parameter values are used (Neubauer et al., 2014). For E55H20 it was necessary to retune the model with the tuning strategy described above, as the tuning in Zhang et al. (2012) was undertaken for nudged simulations, and we performed free simulations to compare the three ECHAM–HAM model versions. The largest differences in tuning between the three model versions are in the tuning parameters for the autoconversion of cloud droplets to rain and entrainment for shallow convection. The latter was adopted from the base model ECHAM6.3 (see Mauritsen et al., 2019, for a discussion of the impact of the change in this tuning parameter on climate sensitivity). In E63H23 stratiform rain formation by autoconversion will be faster than in the other two model versions. This is due to the larger value of the respective tuning parameter leading to reduced LWP, CC, and SW CRE and a more positive TOA net radiative imbalance in E63H23 (Lohmann and Ferrachat, 2010). The larger value of the tuning parameter for entrainment for shallow convection in E61H22 and the even larger value in E63H23 have the opposite effect: increased LWP, CC, and SW CRE and a more negative TOA net radiative imbalance (Mauritsen et al., 2012). For E63H23 there is a compensation by changing both tuning parameters; the most pronounced net effect is a reduced LWP compared to the other two model versions (we hypothesize that LWP is reduced since entrainment for shallow convection mainly affects low, thin clouds, whereas the autoconversion rate affects all liquid clouds; since the reflectivity of clouds depends nonlinearly on their thickness, an increase in thin low clouds can compensate for the SW CRE change with a decrease in thicker clouds but lead to a lower global mean LWP).
2.4 Observational products
We list the products and the respective references for the observational products used in the model evaluation. From Moderate-resolution Imaging Spectroradiometer (MODIS Aqua) collection 6.1 (Platnick et al., 2015, 2017) and from the ESA Cloud Climate Change Initiative (CCI) Advanced Very-High-Resolution Radiometer (AVHRR-PM) v3.0 (prototype; Stengel et al., 2017a, b), histograms of cloud-top pressure vs. cloud optical depth and CC are taken. Histograms of cloud-top pressure vs. cloud optical depth were also taken from the International Satellite Cloud Climatology Project (ISCCP; Rossow and Schiffer, 1999; Pincus et al., 2012; Zhang et al., 2012) D1 data. Cloud–Aerosol Lidar and Infrared Pathfinder Satellite Observations (CALIPSO) data for CC are from the GCM-Oriented CALIPSO Cloud Product (GOCCP) dataset (Chepfer et al., 2010). Cloud radiative effect data are from the Clouds and the Earth's Radiant Energy System (CERES) Energy Balanced and Filled (EBAF) TOA edition 4.0 data product (Loeb et al., 2018). Precipitation data are from the Global Precipitation Climatology Product (GPCP) 2.3 (Adler et al., 2018). Cloud-top CDNCs are from the climatology of Bennartz and Rausch (2017). LWP data are from the Multi-Sensor Advanced Climatology of LWP (MAC-LWP; Elsaesser et al., 2017), which is an updated version of the University of Wisconsin LWP climatology, and from MODIS. IWP is from satellite observations compiled by Li et al. (2012).
3.1 Global mean comparison to observations
Table 3 includes global mean values of radiation, cloud, and aerosol-related variables of the PD simulations of E55H20, E61H22, and E63H23 compared to observations (OBS) or multi-model mean (MMM) values when observations are not available. The global mean values of the radiative fluxes shown in Table 3 are tuning targets (see Sect. 2.6) and therefore cannot be used directly for model evaluation. For E63H23 the SW and LW TOA fluxes, as well as the SW and LW TOA CRE, are within the range of the observations. The net TOA flux of E63H23 is also close to the observations (additional tuning could bring it closer to the observed value but was not attempted given the large uncertainty in, e.g., SW and LW TOA fluxes). The SW, LW, and net TOA fluxes of E61H22 and E55H20 are outside the range of observations. This reflects the change in the tuning targets and strategy in E63H23 and the availability of better observations. The net CRE of E63H23 (and also E55H20 and E61H22) is outside the observed range. It was not possible to find parameter settings that bring the net CRE within the range of observations without bringing one or more of the other radiative fluxes outside the range of observations. This is a first indication of a structural problem in ECHAM–HAM, which could be related to how ice crystals nucleate in (warming) cirrus clouds or an underestimation of (cooling) stratocumulus. This will be further discussed in the evaluation. The CC, P, and cloud-top cloud droplet number concentration (CDNC) of all three model versions agree fairly well with observations (for cloud-top CDNC of the model simulations we selected CDNC over ocean only of the topmost layer of clouds with a cloud-top temperature >273.15 K). For LWP a climatology based on microwave sensors (Elsaesser et al., 2017) provides reliable observations as long as the ratio of LWP to LWP+rainwater path is large (>0.8 is used here), i.e., in regions with relatively low precipitation. The values of LWP only in this low precipitation region (LWP-LP) are also shown in Table 3. Whereas the mean values over the global oceans for LWP and LWP-LP of E61H20 and E55H20 are higher than observed, E63H23 shows values within the observational range (71 and 76 g m−2, respectively). This is due to the more physically based activation scheme in E63H23 and improvements in ECHAM6.3 like energy conservation in the physics part and improvements in the cloud cover scheme for marine stratocumulus clouds, which allow for an increase in the tuning parameter for autoconversion (see Table 2). Similarly, the global mean value of IWP in E63H23 with 15 g m−2 is only slightly below the observational range (18–32 g m−2), whereas in E61H22 and E55H20 IWP is considerably smaller (10 and 8 g m−2, respectively). This is because in the accretion of ice crystals by snow, the sticking efficiency follows Seifert and Beheng (2006) in E63H23, whereas in E55H20 and E61H22 it followed Levkov et al. (2012). The Seifert and Beheng (2006) sticking efficiency leads to a less efficient removal of ice crystals by snow. Furthermore, the changed sticking efficiency allows for a reduction of the stratiform snow formation rate by aggregation compared to earlier model versions (see Table 2), which also increases IWP. The aerosol mass burdens of the five prognostic aerosol species in ECHAM–HAM are within the range of AeroCom models (Textor et al., 2006), except for particulate organic matter (POM). This may be related to the simplistic treatment of secondary organic aerosol (SOA) in all three model versions in the experiments for this study. Details on the evaluation of E63H23 with respect to atmospheric aerosol are given in Tegen et al. (2019).
3.2 Zonal mean comparison to observations
Although the global mean values are tuning targets (see Sect. 2.6), biases in net CRE and IWP in the ECHAM–HAM versions, which could not be brought in agreement with observations via tuning, were identified in the previous section. Zonal mean values of observable variables can nevertheless be used for model evaluation because tuning targets the global mean quantities. Figure 1 shows zonal mean distributions of several quantities for the three model versions and observations. The zonal distribution of SW CRE and LWP-LP of E63H23 agrees relatively well with observations, whereas in E61H22 and E55H20 the magnitude of both quantities is overestimated in midlatitudes. The cloud cover distribution of E63H23 also agrees well with observations, whereas E61H22 and E55H20 show an underestimation by up to 10 percentage points in the subtropics. Biases in cloud-top CDNC are more complex, and retrievals of cloud-top CDNC are only possible for specific clouds (e.g., horizontally homogeneous, unobscured, optically thick clouds) and rely on assumptions, such as liquid water content increasing with altitude like in an adiabatically rising cloud parcel (or at least like a constant fraction of this liquid water content), CDNCs being constant throughout the cloud, and further assumptions that together make cloud-top CDNC retrievals uncertain (Grosvenor et al., 2018). We therefore expect larger differences between observations and models for cloud-top CDNC than for other variables. E55H20 agrees well with MODIS observations in the tropics but overestimates cloud-top CDNC in the subtropics on both hemispheres and midlatitudes in the Southern Hemisphere. E61H22 overestimates cloud-top CDNC in the tropics and subtropics but underestimates it at midlatitudes in the Northern Hemisphere. E63H23 also overestimates cloud-top CDNC in the subtropics, but less than E61H22, and also in the tropics. The liquid phase of clouds is therefore better represented in E63H23 than in the previous model versions. IWP is underestimated in all three model versions. E63H23 has the smallest bias, followed by E61H22, and E55H20 shows the largest deviation from observed zonal mean IWP. The underestimation is particularly large in the tropics, which is likely connected to the parameterization of convection in ECHAM (and ECHAM–HAM). ECHAM has a low precipitation bias over land in the tropics (Mauritsen et al., 2012; Stevens et al., 2013). Gasparini et al. (2018) found indications that the level of detrainment from deep convection is too low in altitude in ECHAM–HAM. They lowered the tuning parameter for deep convective entrainment ϵd to 0.00006, whereas all three ECHAM–HAM versions used here have to use a larger value for this parameter (Table 2) as they use a cirrus scheme in which cirrus clouds can only nucleate homogeneously, which may lead to an overestimation of ICNC and an underestimation of IWP by tuning of radiative fluxes (see Sect. 3.3). For LW CRE (and precipitation and AOD, not shown) all three model version differences are within the range of different observational products. In the tropics E55H20 has a rather strong LW CRE, whereas E63H23 and E61H22 have a rather weak LW CRE, but all are within the range of observations.
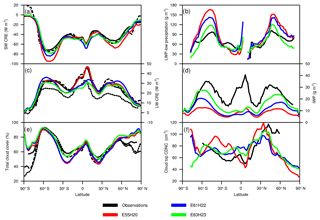
Figure 1Comparison of zonal annual mean values of E55H20, E61H22 and E63H23 to observations, (a) SW CRE, (b) LWP-LP over oceans, (c) LW CRE, (d) IWP, (e) total cloud cover, and (f) cloud-top CDNC of clouds between 268 and 300 K over oceans. Observations of IWP are from Li et al. (2012), LWP-LP over oceans from Elsaesser et al. (2017), cloud-top CDNC over oceans from Bennartz and Rausch (2017). The solid SW and LW CRE lines are from CERES (Loeb et al., 2018), the dashed ones from ERBE (Barkstrom, 1984), and the dotted one for LW CRE is from TOVS satellite data (Susskind et al., 1997). Total cloud cover is from CALIPSO GOCCP (solid line; Chepfer et al., 2010), AVHRR-PM (dashed line; Stengel et al., 2017b), and MODIS collection 6.1 (dotted line; Platnick et al., 2015, 2017).
3.3 Regional comparison to observations
The comparison of CRE of the different model versions with CERES CRE reveals several biases in the representation of clouds. We therefore start by identifying biases in CRE and then use observations for other quantities to identify the causes of the model biases. In Fig. 2 the differences in SW, LW, and net TOA CRE of all model versions to CERES observations are shown. In all three model versions the (negative) SW CRE is too weak in the marine stratocumulus regions west of the continents (the average bias in the wider stratocumulus regions is 1.1, 8.1, and 7.0 W m−2 in E63H23, E61H22, and E55H20, respectively). In addition, the SW CRE is too weak in some land areas in E63H23 and E61H22, in the Southern Ocean in E63H23, and in the tropical oceans in E55H20 (3.3 W m−2 average bias over ocean between 15∘ N and 15∘ S, excluding wider stratocumulus regions). These biases are compensated for by a stronger SW CRE over large parts of the oceans and middle- and high-latitude land areas in the Northern Hemisphere. The bias in stratocumulus regions is smaller in E63H23 than in the older model versions and so are the compensating negative biases. This is due to improvements in ECHAM6.3 like improvements in the cloud cover scheme for marine stratocumulus clouds. The (positive) LW CRE is too weak in the tropics in E63H23 and E61H22 (−7.7 and −4.8 W m−2 average bias, respectively, between 20∘ N and 20∘ S, excluding wider stratocumulus regions) and too strong in the tropics (in particular over land) in E55H20 (1.2 W m−2). Together with the biases in the tropical SW CRE this points to problems with the parameterization of deep convective clouds, detrained condensate, or the representation of anvils from detrained condensate in all three model versions. In all three model versions the LW CRE in midlatitudes is too weak (except over land in the Northern Hemisphere). At high latitudes it is stronger than in the CERES data in all model versions (but the uncertainty of CERES CRE is also larger at high latitudes; Loeb et al., 2018). Only a few of the biases in SW and LW CRE compensate, and therefore the biases in net CRE are as large as or larger than in the SW (LW) CRE. In the net CRE the positive biases in stratocumulus regions and in the Southern Ocean in E63H23 and E61H22, over land in E61H22, and in the tropical oceans in E55H20 are compensated for in the global mean by negative biases in all other regions. The negative biases are caused by adjusting cloud parameters to bring the global mean values in agreement with observations. Therefore, if the biases in stratocumulus regions and the Southern Ocean (and the tropics) could be reduced, the negative biases in SW and net CRE would also be smaller.
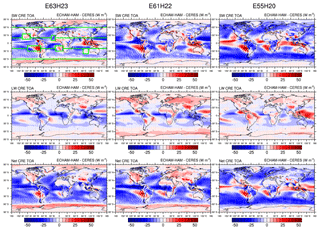
Figure 2Comparison of annual mean SW, LW, and net CRE of E55H20, E61H22, and E63H23 to CERES 4.0 (Loeb et al., 2018) observations. CERES data are for 2005–2015, model data are from the PD simulations. In the top left panel the regions used for cloud-top pressure vs. cloud optical depth histograms are shown by green lines.
In Fig. 3 the cloud cover of the CALIPSO GOCCP product and all three model versions is shown. The hatched areas in Fig. 3 are the regions where the cloud cover of CALIPSO GOCCP, MODIS collection 6.1, and ESA Cloud CCI (AVHRR-PM) differs by more than five percentage points. We therefore use only the areas not marked by hatching in Fig. 3 for the model evaluation. Since the COSP CALIPSO simulator is not implemented in E55H20, the direct model output is shown for all model versions (see Fig. S8 for COSP CALIPSO simulator output of cloud cover for E61H22 and E63H23). The cloud cover of all three model versions agrees fairly well with the observations. The largest biases are in stratocumulus regions west of the continents (−10, −18, and −22 percentage points in E63H23, E61H22, and E55H20, respectively, in the wider stratocumulus regions), where the models underestimate the cloud cover. Over land in the Northern Hemisphere poleward of about 45∘ N the models overestimate cloud cover, and in the Indonesian warm pool region the cloud cover is biased high in the three model versions. The underestimation of cloud cover in stratocumulus regions is less severe in E63H23 than in the other two model versions (the cloud cover scheme in ECHAM6.3 was improved to better represent cloud cover in these regions).
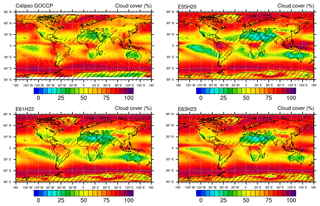
Figure 3Comparison of annual mean cloud cover of E55H20, E61H22, and E63H23 to CALIPSO GOCCP observations. Areas where the cloud cover of CALIPSO GOCCP, MODIS collection 6.1, and AVHRR-PM differ by more than five percentage points are hatched. CALIPSO GOCCP data are for 2006–2010, model data are from the PD simulations (direct model output is used without a simulator).
Figure 4 shows LWP from the MAC-LWP climatology (Elsaesser et al., 2017) and the three model versions. The retrieval of LWP has biases from both visible and near-infrared sensors as well as microwave sensors (Seethala and Horváth, 2010; Lebsock and Su, 2014). Visible and near-infrared sensors such as MODIS have problems when the solar zenith angle is large and detecting pixels of clouds at low altitudes (Lebsock and Su, 2014). Microwave sensors such as AMSR-E may retrieve LWP in cloud-free scenes, and the split between LWP and rainwater path is difficult (Lebsock and Su, 2014). Elsaesser et al. (2017) corrected the retrieval bias of LWP of microwave-sensor-based products in cloud-free scenes. And they recommend using regions with low precipitation (LWP∕(LWP+rainwater path) >0.8) for model evaluation. The regions where precipitation could influence the LWP retrieval are therefore hatched in Fig. 4. This leaves the stratocumulus regions west of the continents and the storm tracks over ocean in the Northern and Southern Hemisphere as the most reliable areas for the evaluation of LWP. All three model regions show a fairly good agreement of LWP in the stratocumulus regions except west of South America and southwest Africa, where all model versions tend to underestimate LWP. In the storm tracks over ocean in the Northern and Southern Hemisphere, on the other hand, E61H22 and even more E55H20 overestimate LWP. E63H23 instead shows a rather good agreement of LWP in the storm tracks compared to observations. This is likely the result of different model tuning in E63H23 (see Sect. 2.3), which was possible due to a more realistic geographic pattern of cloud cover and SW CRE in E63H23.
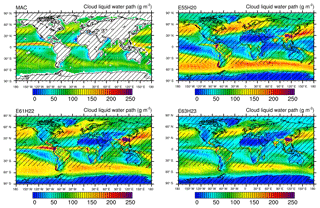
Figure 4Comparison of annual mean LWP of E55H20, E61H22, and E63H23 to MAC-LWP observations. Areas where precipitation could influence the LWP retrieval (LWP∕(LWP+rainwater path) ≤0.8) are hatched. MAC data are for 2003–2012, model data are from the PD simulations.
To further characterize the simulation of liquid clouds in the ECHAM–HAM model versions we also compare cloud-top CDNC of warm (cloud top warmer than 0 ∘C) liquid clouds to the cloud-top CDNC from Bennartz and Rausch (2017), which is based on MODIS Aqua data (Fig. 5). The hatched area marks regions where the relative uncertainty in the observations is larger than 75 %. The general geographical distribution and magnitude of cloud-top CDNC of all model versions agree with the observations, although there are certain areas where model biases are apparent. E61H22 has lower cloud-top CDNCs in midlatitude ocean regions than E63H23; in E55H20 they are higher than in the other two model versions. In E55H20 the higher cloud-top CDNCs can be explained by reduced entrainment of deep convection (see Table 2) compared to the other model versions, which leads to higher relative humidity in the upper tropical troposphere that subsequently leads to increased aerosol nucleation, more Aitken mode particles, and increased CCN concentrations. E63H23 uses the Abdul-Razzak and Ghan (2000) activation scheme and the Long et al. (2011) sea salt emission parameterization (temperature dependent; Sofiev et al., 2011), which lead to higher cloud-top CDNCs than in E61H22 (which uses the Lin and Leaitch, 1997, activation scheme and the Guelle et al., 2001, sea salt emission parameterization) despite the lower LWP in E63H23. Furthermore, in subtropical regions where the cloud cover and LWP are low (see Figs. 3 and 4), cloud-top CDNCs are higher in all three model versions than in the observations. In these regions, shallow trade-wind cumulus clouds occur frequently (Medeiros and Stevens, 2011), and in all model versions shallow convection is triggered frequently (see Fig. S2). The weighted average of stratiform CDNC and detrained CDNC (see Sect. 2.1.3) may overestimate the CDNC of shallow cumulus clouds. The use of a two-moment cloud microphysics scheme for convective clouds (e.g., Lohmann, 2008) so that CDNC in convective clouds can be reduced by collision–coalescence, or a different way to account for detrained CDNC, could help to alleviate this model bias. All three model versions also underestimate cloud-top CDNC at high latitudes. As retrievals from visible and near-infrared sensors often have biases at large zenith angles (see above) this may be a problem with the observations.
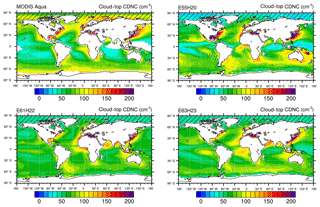
Figure 5Comparison of annual mean cloud-top CDNC of E55H20, E61H22, and E63H23 to MODIS observations from Bennartz and Rausch (2017). Areas where the relative uncertainty in the observations is larger than 75 % are hatched. The MODIS data are for 2003–2015, model data are from the PD simulations.
In Fig. 6 the IWP of all three model versions and IWP satellite observations compiled by Li et al. (2012) are shown. Li et al. (2012) used three different CALIPSO plus CloudSat ice water products and two different methods to remove the contribution of convective clouds and precipitation from the products. Figure 6 displays the compiled mean IWP of the datasets of Li et al. (2012), and areas where the relative standard deviation of the different datasets is larger than 75 % are hatched. The regional distribution of the occurrence of IWP of all three model versions agrees in general quite well with the observations, although it is biased low in all ECHAM–HAM model versions. This could already be seen in the respective global mean and zonal mean values (see Sect. 3.1 and 3.2). Similar to what was found in the analysis of zonal mean IWP the underestimation is largest in the tropics (see Sect. 3.2).
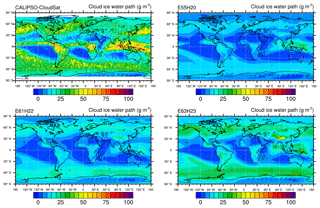
Figure 6Comparison of annual mean IWP of E55H20, E61H22, and E63H23 to CALIPSO–CloudSat observations from Li et al. (2012). Areas where the relative standard deviation of the different datasets compiled in Li et al. (2012) is larger than 75 % are hatched. The CALIPSO–CloudSat data cover the years 2006–2010, model data are from the PD simulations.
Cloud ice mass vertical profiles can be obtained from CALIPSO plus CloudSat observations. The global mean vertical profile of ice water content (IWC) is shown in Fig. 7 for all three model versions and the compiled mean IWC from Li et al. (2012). IWC is underestimated above 700 hPa in all model versions. In E63H23 the maximum of IWC is at the same pressure level as in the observations, at about 350 hPa, whereas in E61H22 and E55H20 the maximum of IWC is at higher altitudes at about 300 to 250 hPa. This can be explained by changes in ICNC and subsequent changes in precipitation formation and ice crystal sedimentation. ICNC changed between the model versions because the way detrained ice crystals are added to existing stratiform clouds has changed since E61H22. The shape of the ice crystals has been made consistent in all modules since E61H22, and a bug in E61H22 was removed in E63H23, which led to homogeneous freezing of dry aerosol particles independent of the availability of water vapor below −35 ∘C (the latter improvement was most important as it doubled the ICNC burden in E61H22 compared to the other two model versions; see Table 3). Below 700 hPa the three model versions are close to the observed IWC, with E63H23 showing an overestimation of IWC and E55H20 an underestimation.
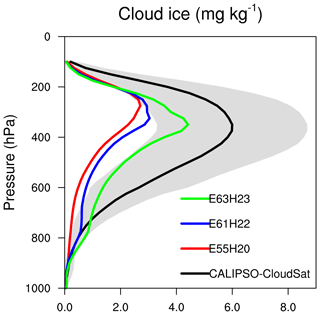
Figure 7Comparison of global annual mean IWC as a function of pressure of E55H20, E61H22, and E63H23 to CALIPSO–CloudSat observations from Li et al. (2012). Gray shading indicates the uncertainty in the CALIPSO–CloudSat observations. The CALIPSO–CloudSat data cover the years 2006–2010, model data are from the PD simulations.
The regions where IWP is underestimated in the three model versions correspond to the regions where the three model versions underestimated LW CRE in Fig. 2 (in particular in the tropics). There are also regions where LW CRE is overestimated (see Fig. 2) in the three model versions, although IWP is underestimated (see Fig. 6). This is an indication that ICNC is too large in the three model versions (the vertical profile of IWC agrees fairly well with observations, although the IWC magnitude is underestimated in all three model versions). As IWP is larger in E63H23 than in E61H22 and E55H20 but the overestimation in LW CRE is smaller in E63H23 than in E61H22 and E55H20, this is an indication that ICNC and the size of the ice crystals are closer to reality in E63H23 than in E61H22 and E55H20. The overestimation of LW CRE in E61H22 around 60∘ N and 60∘ S can be explained by the high ICNC in E61H22 (see Table 3) caused by the ICNC bug mentioned above.
Next to E61H22 there is also a bias of net CRE south of 60∘ S in E63H23 (see Fig. 2). This is not due to ICNC that is too high, as LW CRE of E63H23 agrees well with CERES observations in this region. In E63H23 there is an underestimation of SW CRE south of 60∘ S. Cloud cover and IWP of E63H23 agree well with observations in this region. LWP is slightly underestimated, but cloud-top CDNC is strongly underestimated. Either there is a problem with the satellite retrievals at these high southern latitudes or E63H23 is missing liquid clouds in this region. In E61H22 and E55H20 this possible bias south of 60∘ S is hidden by the overestimation in LWP, which leads to a stronger SW CRE.
In Fig. 8 the total precipitation of all model versions and GPCP2.3 (Adler et al., 2018) is shown. Areas where the relative uncertainty of the GPCP2.3 data is larger than 75 % are hatched. Despite the biases in the representation of clouds in the three model versions identified above, the geographical distribution and magnitude of the annual mean precipitation of all model versions agree well with the observations. Only in the intertropical convergence zone (ITCZ) and South Pacific convergence zone (SPCZ) do the areas and magnitude of precipitation differ from the observations, corresponding to differences in cloud cover and IWP (Figs. 3 and 6, respectively). Cloud cover, IWP, and precipitation are low in the central Pacific and central Atlantic ITCZ but relatively large in the ITCZ west of Central America, east of South America, over the Philippines, and west of Southeast Asia. In the SPCZ cloud cover, IWP, and precipitation are relatively large compared to the respective observations. ECHAM underestimates tropical precipitation over land and overestimates tropical precipitation over ocean (Mauritsen et al., 2012; Stevens et al., 2013). This bias can also be seen in Fig. 8 for all ECHAM–HAM versions. Since ECHAM and ECHAM–HAM use the same parameterizations for convective clouds, this bias is very likely inherited from the base model ECHAM.
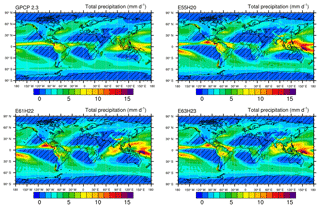
Figure 8Comparison of annual mean precipitation (stratiform + convective) of E55H20, E61H22, and E63H23 to GPCP2.3 observations. Areas where the relative uncertainty of the GPCP2.3 data is larger than 75 % are hatched. The GPCP2.3 data are for 1979–2017, model data are from the PD simulations.
In Fig. 9 histograms of cloud-top pressure vs. cloud optical depth of ECHAM–HAM are compared to ISCCP, AVHRR-PM, and MODIS observations. The COSP simulator (Bodas-Salcedo et al., 2011) was not implemented in E55H20 so we only compare E61H22 and E63H23 to the observations. We applied the COSP–ISCCP simulator for E61H22 and E63H23 for comparison to ISCCP and AVHRR-PM. The COSP–MODIS simulator is only implemented in E63H23 so we compare only E63H23 to MODIS. The histograms were produced for four regions (shown in Fig. 2): wider stratocumulus regions, midlatitudes, tropics, and 60∘ N to 60∘ S. The five marine stratocumulus regions are west of North and South America, west of northern and southern Africa, and west of Australia. The marine stratocumulus regions are extended to the west to approximately cover the regions where the three model versions underestimate SW CRE (see Fig. 2). The midlatitude regions are 60 to 20∘ N and 20 to 60∘ S, excluding the areas covered by the wider stratocumulus regions. The tropics are between 20∘ N and 20∘ S, excluding the areas covered by the wider stratocumulus regions. The region 60∘ N to 60∘ S is the sum of the wider stratocumulus, midlatitudes, and tropics; 60∘ N to 60∘ S was chosen because retrievals from visible and near-infrared sensors often have biases at large zenith angles (see above). Several of the biases described above are also seen in the histograms in Fig. 9. In the region 60∘ N to 60∘ S E63H23 and E61H22 simulate too many optically thick clouds and too few optically thin clouds at low and mid-levels compared to the three satellite datasets. Nam et al. (2012) found a similar bias in several fifth-phase Coupled Model Intercomparison Project (CMIP5) models, as did Stevens et al. (2013) in ECHAM6.1 (“too few, too bright”). This bias can also be seen in the midlatitudes and in the tropics. In the wider stratocumulus region, on the other hand, the occurrence of low-level optically thick clouds of E61H22 and E63H23 agrees rather well with those of the three observational datasets. In the wider stratocumulus regions the optically thin low-level (and mid-level) clouds are missing. This agrees with the analysis of SW CRE in that the underestimation in the stratocumulus regions is compensated for by a stronger SW CRE (clouds being optically thicker) in other regions (by model tuning). Therefore, if the bias in stratocumulus regions could be reduced, the biases in SW CRE and cloud optical depth in other regions could also be reduced. In the midlatitudes the optical depth of low-, mid-, and high-level clouds is larger in E61H22 than in E63H23, ISCCP, and AVHRR-PM. This is related to the stronger compensation by tuning for the lack of clouds in stratocumulus regions (the removal of LWP by autoconversion is slower in E61H22 than in E63H23; see Table 2) and to the ICNC bug in E61H22 mentioned above. In the midlatitudes and the tropics there is also a lack of high-level clouds with optical depth between 1.3 and 23 in E63H23 and E61H22. This lack of cirrus clouds corresponds to the underestimation of IWP and LW CRE. Gasparini et al. (2018) evaluated cirrus clouds in a version of E61H22, which included a cirrus cloud scheme that accounts for a competition in cirrus cloud formation by homogeneous nucleation of solution droplets, heterogeneous freezing of ice-nucleating particles, and water vapor deposition on preexisting ice crystals (Kuebbeler et al., 2014). With this cirrus scheme E61H22 could be tuned such that the global mean IWP agrees with the observations compiled by Li et al. (2012). Similarly, Lohmann and Neubauer (2018) made an experiment in which cirrus clouds could only form by heterogeneous freezing of ice-nucleating particles in E63H23. In their experiment this caused the global mean IWP to agree with the observations compiled by Li et al. (2012). These studies and our analysis indicate that the IWP bias in the three model versions occurs because cirrus clouds can only nucleate homogeneously, and therefore ICNC in cirrus clouds and hence their optical properties are misrepresented.
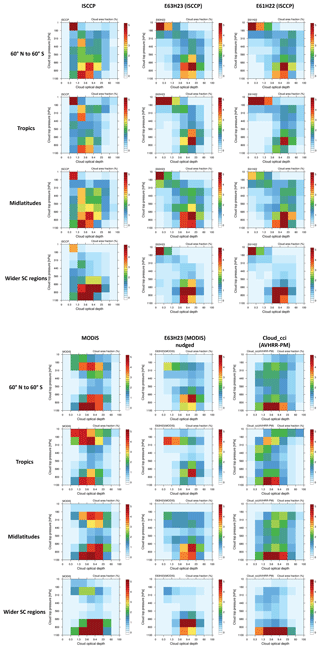
Figure 9Histograms of cloud-top pressure vs. cloud optical depth of E61H22 and E63H23 compared to ISCCP, MODIS, and AVHRR-PM observations for different regions. The definition of the four regions shown is described in the text and the regions are shown in Fig. 2. The ISCCP data are for 2000–2008, MODIS data are for 2003–2012, AVHRR-PM data are for 2003–2012, and the model data are from the PD simulations.
3.4 Summary of model evaluation
Figure 10 shows a Taylor diagram (Taylor, 2001) comparing SW and LW CRE, cloud cover, LWP-LP, cloud-top CDNC, IWP, and precipitation of the three model versions to the respective observations. The standardized deviations of LWP-LP had to be scaled by a factor of 1∕4 so they could fit on the scale. For all variables the root mean square error (RMSE) (solid circles in the diagram in Fig. 10) is smaller than or equal to the RMSE in E63H23 compared to E61H22 and E55H20 (note the scaling for LWP-LP). The changes in the geographical pattern between the three model versions are rather small. E63H23 has somewhat higher correlations except for LW CRE, IWP, and precipitation, for which E55H20 has higher correlations than E63H23 and E61H22. E55H20 has higher correlations of LW CRE, IWP, and precipitation because the ratio of the peaks in these variables in the tropics compared to midlatitudes is better represented in E55H20 (see Fig. 1). Overall, E63H23 is an improvement over earlier model versions.
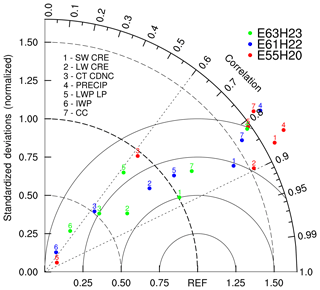
Figure 10Taylor diagram for comparison of SW and LW CRE, cloud cover, LWP-LP, cloud-top CDNC, IWP, and precipitation of E55H20, E61H22, and E63H23 to observations as REF. The standardized deviations of LWP-LP are scaled by a factor of 1∕4 to fit on the diagram. Only areas that are not hatched in Figs. 3–6 were used to create the Taylor diagram. Observations are the same as in Figs. 2–6 and 8. The correlation coefficient is shown as an angle and quantifies the similarity in pattern between modeled and observed annual mean fields. The standard deviation of the modeled fields (normalized by the standard deviation of the observed fields) is shown as the radial distance from the origin. The RMSE is shown as solid black circles and is the distance from the point marked by REF (the closer a model is to REF, the better its skill in reproducing the observations). For E63H23 and the observations for precipitation and LWP-LP, an average over the time period 2003 to 2012 was used. The following time periods were used: for cloud-top CDNC the time period 2003 to 2015, for IWP the time periods in Li et al. (2012), for SW CRE and LW CRE the time period July 2005 to June 2015, for cloud cover the time period June 2006 to December 2010, and for E55H20 and E63H23 the time period 2000 to 2009. Tests with E63H23 showed a negligible impact of the different time periods for the data in the Taylor diagram.
Several biases in the representation of clouds in the three ECHAM–HAM model versions could be identified. The common problem of GCMs in their representation of convective and boundary layer clouds is also present in the three ECHAM–HAM model versions. Stratocumulus clouds are underestimated in all three model versions. Shallow convective clouds are underestimated in E61H22 and E55H20. In E63H23 the cloud cover and LWP in regions where shallow convective clouds are common agree well with observations, but the cloud-top CDNCs are overestimated, leading to an overestimation of SW CRE in these regions. Deep convective clouds over the Atlantic and Pacific oceans form too close to the continents (see Figs. 3, 6 and 8) in E63H23 and ECHAM (Stevens et al., 2013). For the tropical Atlantic this is a common bias in GCMs with coarse horizontal resolution (Siongco et al., 2014). Siongco et al. (2017) discuss different ways this bias in the tropical Atlantic precipitation could be reduced in ECHAM6. IWP is underestimated in all three model versions, in particular in the tropics, whereas LW CRE and the vertical profile of cloud ice agree rather well with observations. This indicates that ICNC may be overestimated in all three model versions (since LW CRE depends on the cloud temperature (∼ cloud altitude) and cloud optical depth: ∝ ICNC, IWP). As E63H23 has the smallest bias in IWP, the bias in ICNC should also be smaller than in the previous versions of ECHAM–HAM. Previous studies (Gasparini et al., 2018; Lohmann and Neubauer, 2018) showed that this overestimation of ICNC is (at least partly) due to missing processes in the formation of cirrus clouds (heterogeneous freezing of ice-nucleating particles and/or water vapor deposition on preexisting ice crystals). These studies also showed that including these processes can reduce the underestimation of IWP in ECHAM–HAM. South of 60∘ S LWP and cloud-top CDNC of E63H23 could be underestimated, although there could also be problems with the satellite retrievals at these high latitudes. In the previous model versions this possible bias was hidden by the overestimation of LWP.
3.5 Simulation of ERFari+aci, RFari, and ECS
In Fig. 11 global maps of SW and LW ERFari+aci for E63H23, E61H22, and E55H20 are shown. An important difference exists in the setup of E55H20 and the two other model versions. E55H20 uses AeroCom I aerosol emission data and the years 1750 and 2000 for preindustrial and present-day aerosol emissions. E63H23 and E61H22, on the other hand, use AeroCom II aerosol emissions and the years 1850 and 2008 for preindustrial and present-day aerosol emissions. The stronger SW ERFari+aci in the east of North America and Europe and the weaker SW ERFari+aci in South and East Asia in E55H20 compared to the two other model versions are therefore predominantly the result of the different representative emission years and inventories (see Supplement Fig. S3). We keep these emission years as they were used as the default in previous studies (e.g., Zhang et al., 2012; Neubauer et al., 2014).
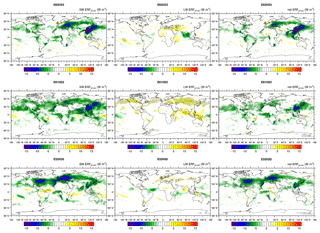
Figure 11Global maps of SW, LW, and net ERFari+aci of E55H20, E61H22, and E63H23 from 20-year free simulations with present-day minus preindustrial aerosol emissions (PDaer − PIaer). Hatching indicates statistically significant differences at the 95 % significance level. The false discovery rate is controlled following Wilks (2016).
The treatment of surface albedo over land, ocean, and sea ice has changed substantially from ECHAM5 to ECHAM6 (see Stevens et al., 2013), which has an impact on SW ERFari+aci (Stier et al., 2013). Because of the differences in the setup and surface albedo treatment of E55H20 we focus the comparison of ERFari+aci on differences between E61H22 and E63H23. ERFari+aci is stronger over land and weaker over oceans in E63H23 compared to E61H22 (Fig. S4). In the global mean 50 % (−0.5 W m−2) of ERFari+aci originates over land in E63H23 and 50 % (−0.5 W m−2) over ocean. This is in contrast to E61H22, wherein 27 % (−0.3 W m−2) of ERFari+aci originates over land and 73 % (−0.9 W m−2) over ocean. E63H23 uses the Köhler-theory-based Abdul-Razzak and Ghan (2000) activation scheme, while E61H22 applies the empirical Lin and Leaitch (1997) activation scheme, which depends only on the number of aerosol particles and updraft velocities. A sensitivity simulation with the Lin and Leaitch (1997) activation scheme applied in E63H23 shows an ERFari+aci of 0.4 W m−2 over land, explaining about half of the difference in ERFari+aci over land between E61H22 and E63H23. The increase in ERFari+aci in E63H23 over land may also be related to the higher rate of autoconversion in E63H23 (Table 2). While Lohmann and Ferrachat (2010) found no strong dependence of ERFari+aci (or a small decrease) on the autoconversion tuning parameters in the global mean, the ratio of autoconversion to the total rain formation rate has a strong regional dependence (see, e.g., Sant et al., 2015), which could lead to regional differences in ERFari+aci.
It is interesting to note that although biases in the simulation of clouds in stratocumulus regions are reduced in E63H23, there seems to be no increase in ERFari+aci in these regions compared to E61H22. Over the remote oceans, the largest differences in ERFari+aci between E63H23 and E61H22 occur between 15 and 45∘ N, where E61H22 simulates a strong ERFari+aci in trade-wind cumulus clouds (Zhang et al., 2016). ERFari+aci in these shallow convective clouds regions is weaker in E63H23, although more clouds are simulated in E63H23 in these regions. LWP and cloud cover in E63H23 are closer to observations in these regions (Figs. 3 and 4), and cloud-top CDNCs are rather too high in E63H23 (Fig. 5). A smaller LWP could lead to a weaker ERFari+aci (Lohmann and Ferrachat, 2010). To better understand the differences over oceans between E63H23 and E61H22, we also compare E63H23 with a simulation with E63H23 wherein CDNCmin was lowered from 40 to 10 cm−3 (E63H23-10CC) as this simulation has a higher LWP (due to retuning with a smaller γr=2.8; not shown). Although the smaller CDNCmin of 10 cm−3 leads to a stronger ERFari+aci everywhere (Hoose et al., 2009; −1.7 W m−2 in the global mean; Table S1), this simulation still provides useful information. In the Northern Hemisphere Pacific there is an increase in ERFari+aci in E63H23-10CC compared to E63H23 (Fig. S5). This may be due to the larger LWP or the change in CDNCmin itself. In the Northern Hemisphere Atlantic, however, ERFari+aci does not increase. The weaker ERFari+aci in the Northern Hemisphere Pacific in E63H23 could therefore be due to the smaller LWP in this simulation, while the smaller ERFari+aci in the Northern Hemisphere Atlantic is due to a different reason. The sensitivity simulation with the Lin and Leaitch (1997) activation scheme applied in E63H23 shows a negative ERFari+aci between 15 and 45∘ N in the Atlantic (Fig. S5). Therefore, the stronger ERFari+aci in E61H22 over oceans can be partly explained by the different activation scheme. Another reason for the stronger ERFari+aci in E61H22 over oceans between 15 and 45∘ N is that different sea salt parameterizations are used in E61H22 and E63H23. Tegen et al. (2019) show that the Long et al. (2011) sea salt parameterization (temperature dependent; Sofiev et al., 2011) used in E63H23 leads to higher aerosol number concentrations over ocean compared to the Guelle et al. (2001) sea salt parameterization used in E61H22, improving the agreement with measured sea salt surface concentrations and particle size distributions at different marine sites (see also the comparison of sea salt parameterizations in Zieger et al., 2017). The higher natural background aerosol concentrations due to the higher sea salt aerosol number concentrations in E63H23 also explain why ERFari+aci is less negative in E63H23 between 15 and 45∘ N over oceans than in E61H22 (Fig. S5). From sensitivity simulations with the Lin and Leaitch (1997) activation scheme or the Guelle et al. (2001) sea salt parameterization applied in E63H23 (Table S1) we conclude that the largest part of the change in SW ERFari+aci is actually from changes in the base model ECHAM6.3.
Most of the differences between the model versions discussed above are differences in SW ERFari+aci. There is one important difference in LW ERFari+aci between the model versions. LW ERFari+aci is more than twice as large in E61H22 as in E55H20 and E63H23 (Table 3). The stronger LW ERFari+aci in E61H22 occurs in Northern Hemisphere midlatitudes and in the tropics (Fig. 12). In Northern Hemisphere midlatitudes LW CRE is also larger in E61H22 due to the ICNC bug (see Fig. 2; ICNC itself is also higher in E61H22; see Table 3). The strong LW ERFari+aci in E61H22 is therefore likely an artifact that was removed in the latest model version.
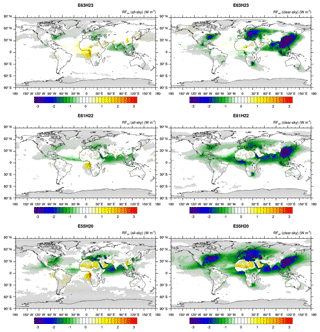
Figure 12 Global maps of all-sky and clear-sky net RFari of E55H20, E61H22, and E63H23 from 20-year free simulations with present-day minus preindustrial aerosol emissions (PDaer − PIaer). Hatching indicates statistically significant differences at the 99 % significance level. The false discovery rate is controlled following Wilks (2016).
Tegen et al. (2019) found an improved aerosol representation in biomass burning regions when GFAS biomass burning emissions, multiplied by a scaling factor of 3.4 as recommended by Kaiser et al. (2012), replace the default ACCMIP biomass burning emissions. Therefore, we performed an E63H23 simulation with GFAS biomass burning emissions multiplied by 3.4 (E63H23-GFAS34). E63H23-GFAS34 has a weaker ERFari+aci (−0.9 W m−2) than E63H23 (−1.0 W m−2) because the preindustrial aerosol burden is higher in E63H23-GFAS34, and ERFari+aci is sensitive to preindustrial aerosol concentrations (Carslaw et al., 2013). Also, the present-day aerosol burdens in E63H23-GFAS34 agree better with the mean aerosol burden of the AeroCom models (Textor et al., 2006) than in E63H23 (see Tables 3 and S1).
We would like to point out that our simulations include interactions between sulfate and mineral dust. On the one hand, (anthropogenic and natural) gaseous sulfate may coat mineral dust particles; this leads to a transfer of dust from insoluble modes to soluble modes in the models, which increases the wet deposition of dust (and leads to decreased present-day mineral dust burdens; see Table S2). On the other hand, mineral dust particles provide surfaces onto which (anthropogenic and natural) gaseous sulfate may condensate, leading to a dampening of the nucleation of new particles. Similar interactions between sulfate and mineral dust have been found by Fan et al. (2004) (using the Geophysical Fluid Dynamics Laboratory (GFDL) global chemical transport model; Mahlman and Moxim, 1978), Bauer and Koch (2005), and Bauer et al. (2007) (using the Goddard Institute for Space Studies (GISS) climate model, modelE; Schmidt et al., 2006; Hansen et al., 2005). The forcing from these interactions between sulfate and mineral dust is included in our estimates for ERFari+aci and RFari (these interactions will make ERFari+aci and RFari less negative, but they are difficult to quantify).
RFari is shown in Fig. 12 for all-sky and clear-sky conditions for E63H23, E61H22, and E55H20 (since RFari is computed by double calls to the radiation scheme, many values in Fig. 12 are statistically significant). RFari is strong in the east of North America, Europe, South Asia, East Asia, and the tropical Atlantic and Indian oceans. The differences in the strength of RFari between E55H20, E63H23, and E61H22 in these regions are predominantly due to different emission years (and a different emission dataset) used in E55H20, as described above for ERFari+aci. Differences in aerosol water uptake can explain the stronger RFari over land in E63H23 than in E61H22. Absorbing aerosol above clouds leads to a positive RFari. This can be seen in all three model versions in the all-sky RFari fluxes west of Africa (in particular in the Southern Hemisphere) and to a lesser extent also west of South America. The significant positive RFari in the Saharan region and the Arabian Peninsula in E55H20 is due to a coding error in E55H20 (the refractive index of POM was used for sulfate aerosol), which was fixed in later model versions. The small positive RFari in the Saharan region, the Arabian Peninsula, and Pakistan in E61H22 and E63H23 is due to a decrease in dust load, which is caused by interaction with sulfate aerosol as described above (also present in E55H20 but shadowed by the coding error). RFari is weaker over ocean in E63H23 than in E61H22 and E55H20. One reason is that the dust burden is larger in E63H23 than in the other model versions and also the decrease in dust burden is larger in E63H23, leading to a positive RFari that compensates for the negative RFari from the increase in anthropogenic aerosol. But there are also differences in aerosol water uptake (aerosol water increases less over oceans in E63H23 than in the other two model versions).
The equilibrium climate sensitivity (ECS) is strongest in E55H20 (3.5 K), weaker in E61H22 (2.8 K), and weakest in E63H23 (2.5 K) (Fig. 13). The corresponding ECS values in the base model versions are: ECHAM5: 3.4 K (Randall et al., 2007; their Table 8.2), ECHAM6.1: 2.8 K (Block and Mauritsen, 2013; Meraner et al., 2013) and ECHAM6.3: 2.8 K (Mauritsen et al., 2019); i.e., changes in ECS between the ECHAM–HAM model versions are driven substantially by changes in the ECHAM base model versions. Note that the ECS value for ECHAM6.3 is from a simulation with abruptly quadrupled CO2 concentrations, in contrast to the CO2 doubling used in the computation of ECS in this study, and that ECHAM has a strong state dependency for ECS (see the discussion in Mauritsen et al., 2019, and references therein). For E61H22 and E63H23 we computed the cloud feedback parameter using the cloud radiative kernel method of Zelinka et al. (2016) (Fig. 14; in E55H20 the COSP–ISCCP simulator is not implemented, and therefore the cloud feedback parameter could not be computed). In addition, we computed ECS and cloud feedback for the E63H23-GFAS34 and E63H23-10CC simulations. E63H23-GFAS34 and E63H23 have very similar ECS and cloud feedback. In E63H23-10CC, on the other hand, ECS is stronger and the cloud feedback is more positive than in E63H23 (leading to more warming in agreement with the stronger ECS). The optical depth feedback of low clouds is more positive between 40∘ N and 40∘ S in E63H23-10CC. The optical depth feedback of non-low clouds is less negative in the tropics and in midlatitudes (not shown). This could be an indication of a weaker cloud phase feedback. As there are fewer but larger cloud droplets in E63H23-10CC than in E63H23 (Fig. S7), the cloud droplets have a shorter lifetime and this decreases differences between ice clouds and liquid water clouds. A similar less negative cloud optical depth feedback of non-low clouds (weaker cloud phase feedback) occurs in E61H22 (CDNCs are higher and the representation of supercooled liquid in mixed-phase clouds is improved in E63H23 compared to E61H22; see Fig. S6). Furthermore, in E61H22 the cloud amount feedback of low clouds is also more positive than in E63H23. This is because in E63H23 in regions of low cloud cover where shallow convective clouds are simulated, the cloud amount feedback is negative, whereas in E61H22 it is positive. This seems to be related to the stronger entrainment rate for shallow convection in E63H23 (Mauritsen et al., 2012, 2019). Mauritsen et al. (2019) describe the increase in entrainment rate for shallow convection in ECHAM6.3 as a measure to reduce ECS in ECHAM6.3. When more of the water vapor remains in the boundary layer as in E63H23, the increased water vapor in the warmer climate can lead to increased cloud cover. The overall more positive cloud feedback in E61H22 than in E63H23 agrees with the stronger ECS in E61H22.
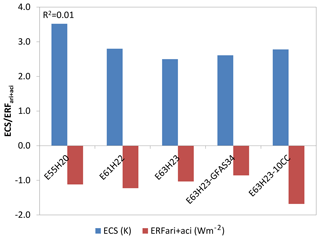
Figure 13Global mean ERFari+aci and ECS of E55H20, E61H22, E63H23, E63H23-GFAS34, and E63H23-10CC. The coefficient of determination between ERFari+aci and ECS is also displayed.
The largest differences between E61H22 and E63H23 in terms of ERFari+aci are therefore due to a more realistic simulation of cloud water, the removal of a bug in ICNC, the new activation scheme, and the new sea salt emission parameterization in E63H23, whereas for ECS they are due to a more realistic simulation of cloud water and to model tuning.
3.6 Impact of changes and improvements in E63H23
The liquid phase of clouds is better represented in E63H23 than in the previous model versions because the low bias in cloud cover in the subtropics is reduced and the zonal distribution of LWP agrees with observations. This also leads to a better agreement of the SW CRE with observations in E63H23. Important reasons for these improvements are the change in the fractional cloud cover scheme for marine stratocumulus clouds, the removal of an inconsistency that had led to either 0 or 1 cloud cover in ECHAM6.3, and subsequent changes in model tuning (Mauritsen et al., 2019). Furthermore E63H23 uses the Abdul-Razzak and Ghan (2000) activation scheme and the Long et al. (2011) sea salt emission parameterization (temperature dependent; Sofiev et al., 2011), which leads to higher CDNCs when LWP is large. The Abdul-Razzak and Ghan (2000) activation scheme is more physically realistic than the empirical Lin and Leaitch (1997) activation scheme used in the previous model versions as it is Köhler theory based and therefore takes into account the size of the aerosol particles and their chemical composition. Although the Abdul-Razzak and Ghan (2000) activation scheme has limitations under certain conditions (the assumption that the aerosol particles are in equilibrium with its environment is not valid in all conditions; Phinney et al., 2003) and does not account for preexisting cloud droplets during cloud droplet activation (Barahona et al., 2010), it certainly helps to improve the representation of cloud droplets in E63H23. The performance of the new Long et al. (2011) (temperature dependent; Sofiev et al., 2011) and the old Guelle et al. (2001) sea salt parameterizations in E63H23 was analyzed by Tegen et al. (2019). The new temperature dependence leads to increased sea salt emissions where the sea surface temperature is warmer than 20 ∘C and a decrease at colder temperatures. The new sea salt parameterization performs better, in particular with respect to number concentrations, than the previous sea salt parameterization compared to measurements on research cruises and at research stations. This is another indication that CDNCs are more realistic in E63H23 than in the previous model versions. The higher CDNCs in E63H23 allowed us to increase the tuning parameter for the autoconversion of cloud droplets to rain. Together these changes led to a better representation of the liquid phase of clouds in E63H23 and to a reduction of the SW component of ERFari+aci in E63H23 compared to E61H22 (because CCN concentrations from natural background aerosol are higher in E63H23).
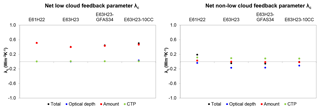
Figure 14Components of the net global mean cloud feedback parameter of E61H22 and E63H23 for low (cloud-top pressure (CTP) >680 hPa) and non-low (CTP <680 hPa) clouds.
Also the ice phase of clouds has improved in E63H23 compared to previous model versions. The low bias in IWP is reduced in E63H23 and the global mean vertical IWC is within the observational range (Fig. 7). This is because the Seifert and Beheng (2006) sticking efficiency used in E63H23 leads to a less efficient removal of ice crystals by snow. A subsequent reduction in the tuning parameter for stratiform snow formation by aggregation further increases IWP in E63H23. Only a few laboratory studies for sticking efficiency have been conducted, and even fewer theories for sticking efficiency have been developed (Phillips et al., 2015). We find that the simple formulation of Seifert and Beheng (2006) for sticking efficiency for the accretion of ice crystals by snow improves the simulation of cloud ice in E63H23. Furthermore, the altitude of the global mean maximum IWC agrees well with observations in E63H23, whereas in E61H22 and E55H20 it is at higher altitudes than observed. This can be explained by the changes in ICNC described in Sect. 2.1.5, such as the use of a consistent ice crystal shape (hexagonal plates), removal of an ICNC bug, or the changed treatment of detrained ice crystals. The subsequent changes in precipitation formation and ice crystal sedimentation can then lead to a different vertical distribution of cloud ice. In E61H22 the global ICNC burden is considerably higher than in the other two model versions because of an inconsistency between cloud droplet activation, condensation, vertical transport of CDNC, and homogeneous freezing of cloud droplets in cirrus clouds, which led to homogeneous freezing of aerosol particles even when the water vapor pressure was too low for homogeneous nucleation. The higher ICNCs are also responsible for the LW component of ERFari+aci in E61H22 being more than twice as large as in the other two model versions. The good agreement of the global mean vertical distribution of IWC and LW CRE with observations in E63H23 is an indication that ICNC and the size of ice crystals are closer to reality in E63H23 than in the previous model versions. In a future version of the model we want to also include the competition for water vapor between homogeneous freezing of solution droplets, heterogeneous freezing of ice-nucleating particles, and preexisting ice crystals in cirrus cloud formation as has been done by Kuebbeler et al. (2014) and Gasparini et al. (2018), which should further improve the simulation of ICNC.
While the global mean values of RFari are quite similar between E63H23 and the previous model versions, there are regional differences. These are caused by the removal of inconsistencies in the model code and for E63H23 also by the new emission parameterization for mineral dust, which uses new satellite-based data for dust sources; this increases the confidence in the simulation of RFari in E63H23.
The weaker ECS in E63H23 compared to E61H22 can be linked to changes in cloud feedbacks. There are indications for a stronger cloud phase feedback in non-low clouds due to increased CDNC and changes in cloud water in E63H23. A stronger (cooling) cloud phase feedback will lead to less warming in the future. Similarly, the less positive cloud amount feedback of low clouds (related to model tuning in ECHAM6.3) in E63H23 contributes to the weaker ECS in E63H23 compared to E61H22.
The changes and improvements in E63H23 (including changes in the base model version ECHAM6.3) have therefore not only improved the representation of clouds in E63H23 compared to previous model versions, but they have also had an impact on ERFari+aci and ECS, decreasing both.
Clouds in the current (E623H23) and previous (E55H20 and E61H22) versions of the ECHAM–HAM global aerosol–climate model were evaluated using a suite of global observational datasets for clouds and precipitation. Improvements in E63H23 compared to previous model versions for cloud water include the following:
-
a more physically based activation scheme (Abdul-Razzak and Ghan, 2000);
-
changes in the treatment of CDNC detrained from convective clouds (as described in Sect. 2.1.5); and
-
an increase in low clouds (Mauritsen et al., 2019),
-
all of which together lead to a more realistic LWP globally.
For cloud ice the improvements include the following:
-
different sticking efficiency for the accretion of ice crystals by snow (Seifert and Beheng, 2006);
-
consistent ice crystal shapes throughout the model (Lohmann and Neubauer, 2018);
-
changes in mixed-phase freezing (as described in Sect. 2.1.5); and
-
the removal of an inconsistency in ICNC in cirrus clouds,
-
all of which together lead to a more realistic IWP globally.
The sum of the changes leads to improved cloud radiative effects. Although the representation of shallow convective clouds has improved in E63H23, stratocumulus clouds are still underrepresented. The comparison of the different model versions showed that the misrepresentation of certain cloud types can lead to compensating biases in other clouds via model tuning. Therefore, if the bias in stratocumulus clouds in E63H23 can be reduced, this could also improve the representation of other cloud types. Reasons for the bias in stratocumulus clouds identified by Neubauer et al. (2014) in E61H22 were, e.g., turbulent mixing that is too strong at cloud top, the shallow convection scheme triggering too often, and/or a lack of vertical resolution. Future work will focus on addressing these difficult issues. Deep convective clouds over the Atlantic and Pacific oceans form too close to the continents, which leads to biases in the geographical distribution of precipitation in E63H23 and ECHAM (while tropical land precipitation is underestimated). While the geographical (except for deep convective clouds) and vertical distributions of cloud ice agree well with observations in E63H23, IWP remains biased low. The combination of observations of IWP, LW CRE, and the vertical distribution of cloud ice indicate that ICNC may be overestimated in ECHAM–HAM. Previous studies with ECHAM–HAM showed that the bias in ICNC and IWP can be reduced when heterogeneous freezing of ice-nucleating particles and/or water vapor deposition on preexisting ice crystals are accounted for in cirrus clouds.
Estimates of ERFari+aci and ECS of E55H20, E61H22, and E63H23 were compared since the representation of clouds is important for both ERFari+aci and ECS. The largest differences between E61H22 and E63H23 in terms of SW ERFari+aci are mainly due to
-
the more realistic simulation of cloud water,
-
but also the new activation scheme in E63H23 and
-
the new sea salt emission parameterization,
which lead to a weaker SW ERFari+aci in E63H23. In terms of LW ERFari+aci the difference is mainly due to
-
the removal of an inconsistency in ICNC in cirrus clouds leading to a weaker LW ERFari+aci in E63H23.
Since there are reductions in both SW and LW ERFari+aci the net ERFari+aci is only slightly weaker in E63H23 (−1.0 W m−2). A sensitivity simulation in which CDNCmin was lowered to 10 cm−3 leads to a stronger ERFari+aci everywhere (−1.7 W m−2), showing that the necessary usage of CDNCmin (Lohmann and Neubauer, 2018) has a strong impact on ERFari+aci. Another sensitivity simulation with increased biomass burning emissions (E63H23-GFAS34) indicates that ERFari+aci in E63H23 would be weaker (−0.9 W m−2) if the representation of biomass burning aerosol could be improved.
ECS is weaker in E63H23 (2.5 K) than in E61H22 (2.8 K) (and E55H20; 3.5 K). The decrease compared to E61H22 is due to the following:
-
changes in the entrainment rate for shallow convection adopted from the base model ECHAM6.3 (which leads to a less positive feedback of cloud amount of low clouds in some regions) and
-
a stronger cloud phase feedback.
Although the differences in both ERFari+aci and ECS between E63H23 and E61H22 can be explained by changes in the representation of clouds, it is not the same changes in the clouds that affect ERFari+aci, which also affect ECS and vice versa. Therefore, many aspects of clouds in GCMs will need to be improved to increase the confidence in computations of ERFari+aci and ECS.
The ECHAM-HAMMOZ model is made freely available to the scientific community under the HAMMOZ Software License Agreement, which defines the conditions under which the model can be used. More information can be found at the HAMMOZ website (https://redmine.hammoz.ethz.ch/projects/hammoz, last access: 13 August 2019).
Scripts can be found at https://doi.org/10.5281/zenodo.2553891 (Neubauer et al., 2019a).
Data can be found at https://doi.org/10.5281/zenodo.2541936 (Neubauer et al., 2019b). ESA cloud CCI data can be downloaded from http://www.esa-cloud-cci.org/?q=data_download (Poulsen et al., 2017; Stengel et al., 2017b). MODIS products are available for download from Level 1 and the Atmosphere Archive and Distribution System (LAADS) at https://ladsweb.modaps.eosdis.nasa.gov/search/ (Platnick, 2017). ISCCP histogram data and the CALIPSO-GOCCP product can be obtained from http://climserv.ipsl.polytechnique.fr/cfmip-obs/ (Zhang et al., 2012; Pincus et al., 2012). Cloud-top CDNC can be downloaded from https://doi.org/10.15695/vudata.ees.1 (Bennartz and Rausch, 2016). MAC-LWP data are available at the Goddard Earth Sciences Data and Information Services Center (GES DISC; current hosting: http://disc.sci.gsfc.nasa.gov, Elsaesser et al., 2016). CERES satellite data can be obtained from the NASA Langley Research Center Atmospheric Science Data Center at https://ceres.larc.nasa.gov/order_data.php (last access: 12 February 2018). The IWP satellite data from Li et al. (2012) were obtained from the authors. GPCP Precipitation data provided by the NOAA/OAR/ESRL PSD, Boulder, Colorado, USA, from their Web site at https://www.esrl.noaa.gov/psd/ (last access: 16 September 2017).
The supplement related to this article is available online at: https://doi.org/10.5194/gmd-12-3609-2019-supplement.
DN designed the evaluation methodology with comments from the coauthors. DN performed the experiments and the analysis of the data. CS and SF provided the support needed to run the ECHAM–HAM model versions. All coauthors were involved in the development of the ECHAM-HAMMOZ model. DN wrote the paper with comments from coauthors.
The authors declare that they have no conflict of interest.
The ECHAM-HAMMOZ model is developed by a consortium composed of ETH Zurich, the Max-Planck-Institut für Meteorologie, Forschungszentrum Jülich, the University of Oxford, the Finnish Meteorological Institute, and the Leibniz Institute for Tropospheric Research; it is managed by the Center for Climate Systems Modeling (C2SM) at ETH Zurich. This study has received partial funding from the Center for Climate System Modelling (C2SM) at ETH Zurich. The computing time for this work was supported by a grant from the Swiss National Supercomputing Centre (CSCS) under project ID s652 and from ETH Zurich. The CERES satellite data were obtained from the NASA Langley Research Center Atmospheric Science Data Center. We would like to thank Sebastian Rast and the anonymous reviewers for useful comments and suggestions.
This research has been supported by the Swiss National Science Foundation (grant no. 200021_160177), the European Union's Seventh Framework Programme (FP7/2007-2013) project BACCHUS (grant no. 603445), the European Research Council project RECAP (grant no. 724602), and the Academy of Finland (grant nos. 308292 and 307331).
This paper was edited by Samuel Remy and reviewed by two anonymous referees.
Abdul-Razzak, H. and Ghan, S. J.: A parameterization of aerosol activation 2. Multiple aerosol types, J. Geophys. Res., 105, 6837–6844, 2000.
Adler, R. F., Gu, G., and Huffman, G. J.: Estimating Climatological Bias Errors for the Global Precipitation Climatology Project (GPCP), J. Appl. Meteorol. Clim., 51, 84–99, 2012.
Adler, R. F., Sapiano, M. R. P., Huffman, G. J., Wang, J.-J., Gu, G., Bolvin, D., Chiu, L., Schneider, U., Becker, A., Nelkin, E., Xie, P., Ferraro, R., and Shin, D.-B.: The Global Precipitation Climatology Project (GPCP) Monthly Analysis (New Version 2.3) and a Review of 2017 Global Precipitation, Atmosphere, 9, 138, https://doi.org/10.3390/atmos9040138, 2018.
Barahona, D., West, R. E. L., Stier, P., Romakkaniemi, S., Kokkola, H., and Nenes, A.: Comprehensively accounting for the effect of giant CCN in cloud activation parameterizations, Atmos. Chem. Phys., 10, 2467–2473, https://doi.org/10.5194/acp-10-2467-2010, 2010.
Barkstrom, B. R.: The Earth Radiation Budget Experiment (ERBE), B. Am. Meteorol. Soc., 65, 1170–1185, https://doi.org/10.1175/1520-0477(1984)065<1170:TERBE>2.0.CO;2, 1984.
Bauer, S. E. and Koch, D.: Impact of heterogeneous sulfate formation at mineral dust surfaces on aerosol loads and radiative forcing in the Goddard Institute for Space Studies general circulation model, J. Geophys. Res., 110, D17202, https://doi.org/10.1029/2005JD005870, 2005.
Bauer, S. E., Mishchenko, M. I., Lacis, A. A., Zhang, S., Perlwitz, J., and Metzger, S. M.: Do sulfate and nitrate coatings on mineral dust have important effects on radiative properties and climate modeling?, J. Geophys. Res., 112, D06307, https://doi.org/10.1029/2005JD006977, 2007.
Bennartz, R. and Rausch, J.: Cloud Droplet Number ConcentrationClimatology, https://doi.org/10.15695/vudata.ees.1, 2016.
Bennartz, R. and Rausch, J.: Global and regional estimates of warm cloud droplet number concentration based on 13 years of AQUA-MODIS observations, Atmos. Chem. Phys., 17, 9815–9836, https://doi.org/10.5194/acp-17-9815-2017, 2017.
Bergeron, T.: On the physics of cloud and precipitation. Proceedings of the Fifth Assembly of the International Union of Geodesy and Geophysics, Vol. 2, International Union of Geodesy and Geophysics, 156–178, 1935.
Block, K. and Mauritsen, T.: Forcing and feedback in the MPI-ESM-LR coupled model under abruptly quadrupled CO2, J. Adv. Model. Earth Sy., 5, 676–691, https://doi.org/10.1002/jame.20041, 2013.
Bodas-Salcedo, A., Webb, M. J., Bony, S., Chepfer, H., Dufresne, J.-L., Klein, S. A., Zhang, Y., Marchand, R., Haynes, J. M., Pincus, R., and John, V. O.: COSP: Satellite simulation software for model assessment, B. Am. Meteorol. Soc., 2011, 1023–1043, https://doi.org/10.1175/2011BAMS2856.1, 2011.
Boucher, O., Randall, D., Artaxo, P., Bretherton, C., Feingold, G., Forster, P., Kerminen, V.-M., Kondo, Y., Liao, H., Lohmann, U., Rasch, P., Satheesh, S. K., Sherwood, S., Stevens, B., and Zhang, X. Y.: Clouds and Aerosols. In: Climate Change 2013: The Physical Science Basis, Contribution of Working Group I to the Fifth Assessment Report of the Intergovernmental Panel on Climate Change, edited by: Stocker, T. F., Qin, D., Plattner, G.-K., Tignor, M., Allen, S. K., Boschung, J., Nauels, A., Xia, Y., Bex, V., and Midgley, P. M., Cambridge University Press, Cambridge, United Kingdom and New York, NY, USA, 2013.
Boudala, F. S., Isaac, G. A., Fu, Q., and Cober, S. G.: Parameterization of effective ice particle size for high-latitude clouds, Int. J. Climatol., 22, 1267–1284, https://doi.org/10.1002/joc.774, 2002.
Brinkop, S. and Roeckner, E.: Sensitivity of a general circulation model to parameterizations of cloud-turbulence interactions in the atmospheric boundary layer, Tellus, 47A, 197–220, 1995.
Carslaw, K. S., Lee, L. A., Reddington, C. L., Pringle, K. J., Rap, A., Forster, P. M., Mann, G. W., Spracklen, D. V., Woodhouse, M. T., Regayre, L. A., and Pierce, J. R.: Large contribution of natural aerosols to uncertainty in indirect forcing, Nature, 503, 67–71, https://doi.org/10.1038/nature12674, 2013.
Chepfer, H., Bony, S., Winker, D., Cesana, G., Dufresne, J. L., Minnis, P., Stubenrauch, C. J., and Zeng, S.: The GCM Oriented CALIPSO Cloud Product (CALIPSO-GOCCP). J. Geophys. Res., 115, D00H16, https://doi.org/10.1029/2009JD012251, 2010.
Collins, M., Knutti, R., Arblaster, J., Dufresne, J.-L., Fichefet, T., Friedlingstein, P., Gao, X., Gutowski, W. J., Johns, T., Krinner, G., Shongwe, M., Tebaldi, C., Weaver, A. J., and Wehner, M.: Long-term Climate Change: Projections, Commitments and Irreversibility, in: Climate Change 2013: The Physical Science Basis. Contribution of Working Group I to the Fifth Assessment Report of the Intergovernmental Panel on Climate Change, edited by: Stocker, T. F., Qin, D., Plattner, G.-K., Tignor, M., Allen, S. K., Boschung, J., Nauels, A., Xia, Y., Bex, V., and Midgley, P. M., Cambridge University Press, Cambridge, United Kingdom and New York, NY, USA, 2013.
Croft, B., Lohmann, U., Martin, R. V., Stier, P., Wurzler, S., Feichter, J., Posselt, R., and Ferrachat, S.: Aerosol size-dependent below-cloud scavenging by rain and snow in the ECHAM5-HAM, Atmos. Chem. Phys., 9, 4653–4675, https://doi.org/10.5194/acp-9-4653-2009, 2009.
Croft, B., Lohmann, U., Martin, R. V., Stier, P., Wurzler, S., Feichter, J., Hoose, C., Heikkilä, U., van Donkelaar, A., and Ferrachat, S.: Influences of in-cloud aerosol scavenging parameterizations on aerosol concentrations and wet deposition in ECHAM5-HAM, Atmos. Chem. Phys., 10, 1511–1543, https://doi.org/10.5194/acp-10-1511-2010, 2010.
Elsaesser, G., O'Dell, C., Lebsock, M., and Teixeira, J.: Multisensor Advanced Climatology Mean Liquid Water Path Diurnal Cycle L3 Monthly 1 degree x 1 degree V1, Greenbelt, MD, USA, Goddard Earth Sciences Data and Information Services Center (GES DISC), https://doi.org/10.5067/MEASURES/MACLWPD, 2016.
Elsaesser, G. S., O'Dell, C. W., Lebsock, M. D., Bennartz, R., Greenwald, T. J., and Wentz, F. J.: The Multisensor Advanced Climatology of Liquid Water Path (MAC-LWP), J. Climate, 30, 10193–10210, https://doi.org/10.1175/JCLI-D-16-0902.1, 2017.
Fan, S.-M., Horowitz, L. W., Levy II, H., and Moxim, W. J.: Impact of air pollution on wet deposition of mineral dust aerosols, Geophys. Res. Lett., 31, L02104, https://doi.org/10.1029/2003GL018501, 2004.
Feichter, J., Kjellstrom, E., Rodhe, H., Dentener, F., Lelieveld, J., and Roelofs, G. J.: Simulation of the tropospheric sulfur cycle in a global climate model, Atmos. Environ., 30, 1693–1707, 1996.
Findeisen, W.: Die kolloidmeteorologischen Vorgänge bei der Niederschlagsbildung (Colloidal meteorological processes in the formation of precipitation), Meteorol. Z., 55, 121–133, 1938.
Gasparini, B., Meyer, A., Neubauer, D., Münch, S., and Lohmann, U.: Cirrus Cloud Properties as Seen by the CALIPSO Satellite and ECHAM-HAM Global Climate Model, J. Climate, 31, 1983–2003, https://doi.org/10.1175/JCLI-D-16-0608.1, 2018.
Grosvenor, D. P., Sourdeval, O., Zuidema, P., Ackerman, A. S., Alexandrov, M. D., Bennartz, R., Boers, R., Cairns, B., Chiu, C., Christensen, M., Deneke, H., Diamond, M., Feingold, G., Fridlind, A., Hünerbein, A., Knist, C., Kollias, P., Marshak, A., McCoy, D., Merk, D., Painemal, D., Rausch, J., Rosenfeld, D., Russchenberg, H., Seifert, P., Sinclair, K., Stier, P., van Diedenhoven, B., Wendisch, M., Werner, F., Wood, R., Zhang, Z., and Quaas, J.: Remote sensing of droplet number concentration in warm clouds: A review of the current state of knowledge and perspectives, Rev. Geophys., 56, 409–453, https://doi.org/10.1029/2017RG000593, 2018.
Guelle, W., Schulz, M., Balkanski, Y., and Dentener, F.: Influence of the source formulation on modelling the atmospheric global distribution of sea salt aerosol, J. Geophys. Res., 106, 27509–27524, 2001.
Hansen, J., Sato, M., Ruedy, R., Nazarenko, L., Lacis, A., Schmidt,G. A., Russell, G., Aleinov, I., Bauer, M., Bauer, S., Bell, N.,Cairns, B., Canuto, V., Chandler, M., Cheng, Y., Del Genio, A., Faluvegi, G., Fleming, E., Friend, A., Hall, T., Jackman, C., Kel-ley, M., Kiang, N., Koch, D., Lean, J., Lerner, J., Lo, K., Menon, S., Miller, R., Minnis, P., Novakov, T., Oinas, V., Perlwitz, Ja.,Perlwitz, Ju., Rind, D., Romanou, A., Shindell, D., Stone, P., Sun,S., Tausnev, N., Thresher, D., Wielicki, B., Wong, T., Yao, M., and Zhang, S.: Efficacy of climate forcings, J. Geophys. Res., 110, D18104, https://doi.org/10.1029/2005JD005776, 2005.
Heinold, B., Tegen, I., Schepanski, K., and Banks, J. R.: New developments in the representation of Saharan dust sources in the aerosol–climate model ECHAM6-HAM2, Geosci. Model Dev., 9, 765–777, https://doi.org/10.5194/gmd-9-765-2016, 2016.
Hoesly, R. M., Smith, S. J., Feng, L., Klimont, Z., Janssens-Maenhout, G., Pitkanen, T., Seibert, J. J., Vu, L., Andres, R. J., Bolt, R. M., Bond, T. C., Dawidowski, L., Kholod, N., Kurokawa, J.-I., Li, M., Liu, L., Lu, Z., Moura, M. C. P., O'Rourke, P. R., and Zhang, Q.: Historical (1750–2014) anthropogenic emissions of reactive gases and aerosols from the Community Emissions Data System (CEDS), Geosci. Model Dev., 11, 369–408, https://doi.org/10.5194/gmd-11-369-2018, 2018.
Hoose, C., Kristjansson, J. E., Iversen, T., Kirkevag, A., Seland, O., and Gettelman, A.: Constraining cloud droplet number concentration in GCMs suppresses the aerosol indirect effect, Geophys. Res. Lett., 36, L12807, https://doi.org/10.1029/2009gl038568, 2009.
Horowitz, L. W., Walters, S., Mauzerall, D. L., Emmons, L. K., Rasch, P. J., Granier, C., Tie, X., Lamarque, J.-F., Schultz, M. G., Tyndall, G. S., Orlando, J. J., and Brasseur, G. P.: A global simulation of tropospheric ozone and related tracers: Description and evaluation of MOZART, version 2, J. Geophys. Res., 108, 4784, https://doi.org/10.1029/2002JD002853, 2003.
Hourdin, F., Mauritsen, T., Gettelman, A., Golaz, J.-C., Balaji, V., Duan, Q., Folini, D., Ji, D., Klocke, D., Qian, Y., Rauser, F., Rio, C., Tomassini, L., Watanabe, M., and Williamson, D.: The Art and Science of Climate Model Tuning, B. Am. Meteorol. Soc., 98, 589–602, https://doi.org/10.1175/BAMS-D-15-00135.1, 2017.
Inness, A., Baier, F., Benedetti, A., Bouarar, I., Chabrillat, S., Clark, H., Clerbaux, C., Coheur, P., Engelen, R. J., Errera, Q., Flemming, J., George, M., Granier, C., Hadji-Lazaro, J., Huijnen, V., Hurtmans, D., Jones, L., Kaiser, J. W., Kapsomenakis, J., Lefever, K., Leitão, J., Razinger, M., Richter, A., Schultz, M. G., Simmons, A. J., Suttie, M., Stein, O., Thépaut, J.-N., Thouret, V., Vrekoussis, M., Zerefos, C., and the MACC team: The MACC reanalysis: an 8 yr data set of atmospheric composition, Atmos. Chem. Phys., 13, 4073–4109, https://doi.org/10.5194/acp-13-4073-2013, 2013.
Johnson, G. C., Lyman, J. M., and Loeb, N. G.: Correspondence: Improving estimates of Earth's energy imbalance, Nat. Clim. Change, 6, 639–640, 2016.
Joos, H., Spichtinger, P., and Lohmann, U.: Influence of a future climate on the microphysical and optical properties of orographic cirrus clouds in ECHAM5, J. Geophys. Res., 115, D19129, https://doi.org/10.1029/2010JD013824, 2010.
Kaiser, J. W., Heil, A., Andreae, M. O., Benedetti, A., Chubarova, N., Jones, L., Morcrette, J.-J., Razinger, M., Schultz, M. G., Suttie, M., and van der Werf, G. R.: Biomass burning emissions estimated with a global fire assimilation system based on observed fire radiative power, Biogeosciences, 9, 527–554, https://doi.org/10.5194/bg-9-527-2012, 2012.
Kärcher, B., and Lohmann, U.: A Parameterization of cirrus cloud formation: Homogeneous freezing including effects of aerosol size, J. Geophys. Res., 107, 4698, https://doi.org/10.1029/2001JD001429, 2002.
Kazil, J. and Lovejoy, E. R.: A semi-analytical method for calculating rates of new sulfate aerosol formation from the gas phase, Atmos. Chem. Phys., 7, 3447–3459, https://doi.org/10.5194/acp-7-3447-2007, 2007.
Kazil, J., Stier, P., Zhang, K., Quaas, J., Kinne, S., O'Donnell, D., Rast, S., Esch, M., Ferrachat, S., Lohmann, U., and Feichter, J.: Aerosol nucleation and its role for clouds and Earth's radiative forcing in the aerosol-climate model ECHAM5-HAM, Atmos. Chem. Phys., 10, 10733–10752, https://doi.org/10.5194/acp-10-10733-2010, 2010.
Khairoutdinov, M. and Kogan, Y.: A New Cloud Physics Parameterization in a Large-Eddy Simulation Model of Marine Stratocumulus, Mon. Weather Rev., 128, 229, https://doi.org/10.1175/1520-0493(2000)128<0229:ANCPPI>2.0.CO;2, 2000.
Kipling, Z., Stier, P., Labbouz, L., and Wagner, T.: Dynamic subgrid heterogeneity of convective cloud in a global model: description and evaluation of the Convective Cloud Field Model (CCFM) in ECHAM6–HAM2, Atmos. Chem. Phys., 17, 327–342, https://doi.org/10.5194/acp-17-327-2017, 2017.
Knutti, R., Rugenstein, M. A. A., and Hegerl, G. C.: Beyond equilibrium climate sensitivity, Nat. Geosci., 10, 727–736, https://doi.org/10.1038/ngeo3017, 2017.
Kokkola, H., Kühn, T., Laakso, A., Bergman, T., Lehtinen, K. E. J., Mielonen, T., Arola, A., Stadtler, S., Korhonen, H., Ferrachat, S., Lohmann, U., Neubauer, D., Tegen, I., Siegenthaler-Le Drian, C., Schultz, M. G., Bey, I., Stier, P., Daskalakis, N., Heald, C. L., and Romakkaniemi, S.: SALSA2.0: The sectional aerosol module of the aerosol–chemistry–climate model ECHAM6.3.0-HAM2.3-MOZ1.0, Geosci. Model Dev., 11, 3833–3863, https://doi.org/10.5194/gmd-11-3833-2018, 2018.
Korolev, A.: Limitations of the Wegener–Bergeron–Findeisen mechanism in the evolution of mixed-phase clouds, J. Atmos. Sci., 64, 3372–3375, https://doi.org/10.1175/JAS4035.1, 2007.
Kuang, C., McMurry, P. H., McCormick, A. V., and Eisele, F. L.: Dependence of nucleation rates on sulfuric acid vapor concentration in diverse atmospheric locations, J. Geophys. Res., 113, D10209, https://doi.org/10.1029/2007JD009253, 2008.
Kuebbeler, M., Lohmann, U., Hendricks, J., and Kärcher, B.: Dust ice nuclei effects on cirrus clouds, Atmos. Chem. Phys., 14, 3027–3046, https://doi.org/10.5194/acp-14-3027-2014, 2014.
Kulmala, M., Lehtinen, K. E. J., and Laaksonen, A.: Cluster activation theory as an explanation of the linear dependence between formation rate of 3 nm particles and sulphuric acid concentration, Atmos. Chem. Phys., 6, 787–793, https://doi.org/10.5194/acp-6-787-2006, 2006.
Labbouz, L., Kipling, Z., Stier, P., and Protat, A.: How Well Can We Represent the Spectrum of Convective Clouds in a Climate Model? Comparisons between Internal Parameterization Variables and Radar Observations, J. Atmos. Sci., 75, 1509–1524 https://doi.org/10.1175/JAS-D-17-0191.s1, 2018.
Lebsock, M. and Su, H.: Application of active spaceborne remote sensing for understanding biases between passive cloud water path retrievals, J. Geophys. Res., 119, 8962–8979, 2014.
Levkov, L., Rockel, B., Kapitza, H., and Raschke, E.: 3D mesoscale numerical studies of cirrus and stratus clouds by their time and space evolution, Beitr. Phys. Atmosph., 65, 35–58, 1992.
Li, J.-L. F., Waliser, D. E., Chen, W.-T., Guan, B., Kubar, T., Stephens, G., Ma, H.-Y., Deng, M., Donner, L., Seman, C., and Horowitz, L.: An observationally based evaluation of cloud ice water in CMIP3 and CMIP5 GCMs and contemporary reanalyses using contemporary satellite data, J. Geophys. Res., 117, D16105, https://doi.org/10.1029/2012JD017640, 2012.
Lin, H. and Leaitch, W. R.: Development of an in-cloud aerosol activation parameterization for climate modelling, in: WMO Workshop on Measurement of Cloud Properties for Forecasts of Weather, Air Quality and Climate, Geneva, Switzerland, World Meteorology Organization, 328–355, 1997.
Loeb, N. G., Wielicki, B. A., Doelling, D. R., Smith, G. L., Keyes, D. F., Kato, S., Manalo-Smith, N., and Wong, T.: Toward Optimal Closure of the Earth's Top-of-Atmosphere Radiation Budget, J. Climate, 22, 748–766, 2009.
Loeb, N. G., Doelling, D. R., Wang, H. L., Su, W. Y., Nguyen, C., Corbett, J. G., Liang, L. S., Mitrescu, C., Rose, F. G., and Kato, S.: Clouds and the Earth's Radiant Energy System (CERES) Energy Balanced and Filled (EBAF) Top-of-Atmosphere (TOA) Edition-4.0 Data Product, J. Climate, 31, 895–918, 2018.
Lohmann, U.: Global anthropogenic aerosol effects on convective clouds in ECHAM5-HAM, Atmos. Chem. Phys., 8, 2115–2131, https://doi.org/10.5194/acp-8-2115-2008, 2008.
Lohmann, U. and Diehl, K.: Sensitivity studies of the importance of dust ice nuclei for the indirect aerosol effect on stratiform mixed phase clouds, J. Atmos. Sci., 63, 968–982, 2006.
Lohmann, U. and Ferrachat, S.: Impact of parametric uncertainties on the present-day climate and on the anthropogenic aerosol effect, Atmos. Chem. Phys., 10, 11373–11383, https://doi.org/10.5194/acp-10-11373-2010, 2010.
Lohmann, U. and Hoose, C.: Sensitivity studies of different aerosol indirect effects in mixed-phase clouds, Atmos. Chem. Phys., 9, 8917–8934, https://doi.org/10.5194/acp-9-8917-2009, 2009.
Lohmann, U. and Neubauer, D.: The importance of mixed-phase and ice clouds for climate sensitivity in the global aerosol–climate model ECHAM6-HAM2, Atmos. Chem. Phys., 18, 8807–8828, https://doi.org/10.5194/acp-18-8807-2018, 2018.
Lohmann, U. and Roeckner, E.: Design and performance of a new cloud microphysics scheme developed for the ECHAM general circulation model, Clim. Dynam., 12, 557–572, 1996.
Lohmann, U., Stier, P., Hoose, C., Ferrachat, S., Kloster, S., Roeckner, E., and Zhang, J.: Cloud microphysics and aerosol indirect effects in the global climate model ECHAM5-HAM, Atmos. Chem. Phys., 7, 3425–3446, https://doi.org/10.5194/acp-7-3425-2007, 2007.
Lohmann, U., Spichtinger, P., Jess, S., Peter, T., and Smit, H.: Cirrus cloud formation and ice supersaturated regions in a global climate model, Environ. Res. Lett., 3, 045022, https://doi.org/10.1088/1748-9326/3/4/045022, 2008.
Long, M. S., Keene, W. C., Kieber, D. J., Erickson, D. J., and Maring, H.: A sea-state based source function for size- and composition-resolved marine aerosol production, Atmos. Chem. Phys., 11, 1203–1216, https://doi.org/10.5194/acp-11-1203-2011, 2011.
Mahlman, J. D. and Moxim, W. J.: Tracer simulation in a global general circulation model: Results from a mid-latitude instaneous source experiment, J. Atmos. Sci., 35, 1340–1374, 1978.
Matus, A. V. and L'Ecuyer, T. S.: The role of cloud phase in Earth's radiation budget, J. Geophys. Res., 122, 2559–2578, 2017.
Mauritsen, T., Stevens, B., Roeckner, E., Crueger, T., Esch, M., Giorgetta, M., Haak,H.,Jungclaus, J., Klocke,D., Matei, D., Mikolajewicz, U., Notz, D., Pincus, R., Schmidt, H., and Tomassini, L.: Tuning the climate of a global model, J. Adv. Model. Earth Sy., 4, M00A01, https://doi.org/10.1029/2012MS000154, 2012.
Mauritsen, T., Bader, J., Becker, T., Behrens, J., Bittner, M., Brokopf, R., Brovkin, V., Claussen, M., Crueger, T., Esch, M., Fast, I., Fiedler, S., Fläschner, D., Gayler, V., Giorgetta, M., Goll, D. S., Haak, H., Hagemann, S., Hedemann, C., Hohenegger, C., Ilyina, T., Jahns, T., Jimenez de la Cuesta Otero, D., Jungclaus, J., Kleinen, T., Kloster, S., Kracher, D., Kinne, S., Kleberg, D., Lasslop, G., Kornblueh, L., Marotzke, J., Matei, D., Meraner, K., Mikolajewicz, U., Modali, K., Möbis, B., Müller, W. A., Nabel, J. E. M. S., Nam, C. C. W., Notz, D., Nyawira, S.-S., Paulsen, H., Peters, K., Pincus, R., Pohlmann, H., Pongratz, J., Popp, M., Raddatz, T., Rast, S., Redler, R., Reick, C. H., Rohrschneider, T., Schemann, V., Schmidt, H., Schnur, R., Schulzweida, U., Six, K. D., Stein, L., Stemmler, I., Stevens, B., von Storch, J.-S., Tian, F., Voigt, A., de Vrese, P., Wieners, K.-H., Wilkenskjeld, S., Winkler, A., and Roeckner, E.: Developments in the MPI-M Earth System Model version 1.2 (MPI-ESM 1.2) and its response to increasing CO2, J. Adv. Model. Earth Sy., 11, 998–1038, https://doi.org/10.1029/2018MS001400, 2019.
Medeiros, B. and Stevens, B: Revealing differences in GCM representations of low clouds, Clim. Dynam., 36, 385–399, https://doi.org/10.1007/s00382-009-0694-5, 2011.
Meraner, K., Mauritsen, T., and Voigt, A.: Robust increase in equilibrium climate sensitivity under global warming, Geophys. Res. Lett., 40, 5944–5948, https://doi.org/10.1002/2013GL058118, 2013.
Miller, D. J., Zhang, Z., Ackerman, A. S., Platnick, S., and Baum, B. A.: The impact of cloud vertical profile on liquid water path retrieval based on the bispectral method: A theoretical study based on large-eddy simulations of shallow marine boundary layer clouds, J. Geophys. Res.-Atmos., 121, 998–1038, https://doi.org/10.1002/2015JD024322, 2016.
Myhre, G., Samset, B. H., Schulz, M., Balkanski, Y., Bauer, S., Berntsen, T. K., Bian, H., Bellouin, N., Chin, M., Diehl, T., Easter, R. C., Feichter, J., Ghan, S. J., Hauglustaine, D., Iversen, T., Kinne, S., Kirkevåg, A., Lamarque, J.-F., Lin, G., Liu, X., Lund, M. T., Luo, G., Ma, X., van Noije, T., Penner, J. E., Rasch, P. J., Ruiz, A., Seland, Ø., Skeie, R. B., Stier, P., Takemura, T., Tsigaridis, K., Wang, P., Wang, Z., Xu, L., Yu, H., Yu, F., Yoon, J.-H., Zhang, K., Zhang, H., and Zhou, C.: Radiative forcing of the direct aerosol effect from AeroCom Phase II simulations, Atmos. Chem. Phys., 13, 1853–1877, https://doi.org/10.5194/acp-13-1853-2013, 2013.
Nam, C., Bony, S., Dufresne, J.-L., and Chepfer, H.: The “too few, too bright” tropical low-cloud problem in CMIP5 models, Geophys. Res. Lett.,39, L21801, https://doi.org/10.1029/2012GL053421, 2012.
Neubauer, D., Lohmann, U., Hoose, C., and Frontoso, M. G.: Impact of the representation of marine stratocumulus clouds on the anthropogenic aerosol effect, Atmos. Chem. Phys., 14, 11997–12022, https://doi.org/10.5194/acp-14-11997-2014, 2014.
Neubauer, D., Ferrachat, S., Siegenthaler-Le Drian, C., Stier, P., Partridge, D. G., Tegen, I., Bey, I., Stanelle, T., Kokkola, H., and Lohmann, U.: Scripts for the publication “The global aerosol-climate model ECHAM6.3-HAM2.3 – Part 2: Cloud evaluation, aerosol radiative forcing and climate sensitivity” (Version 1.1.0), Zenodo, https://doi.org/10.5281/zenodo.2553891, 2019a.
Neubauer, D., Ferrachat, S., Siegenthaler-Le Drian, C., Stier, P., Partridge, D. G., Tegen, I., Bey, I., Stanelle, T., Kokkola, H., and Lohmann, U.: Data for the publication “The global aerosol-climate model ECHAM6.3-HAM2.3 – Part 2: Cloud evaluation, aerosol radiative forcing and climate sensitivity” (Version 1.1.0), Zenodo, https://doi.org/10.5281/zenodo.2541936, 2019b.
Nordeng, T. E.: Extended versions of the convective parameterization scheme at ECMWF and their impact on the mean and transient activity of the model in the tropics, Technical Memorandum 206, ECMWF, Reading, UK, 1994.
Petters, M. D. and Kreidenweis, S. M.: A single parameter representation of hygroscopic growth and cloud condensation nucleus activity, Atmos. Chem. Phys., 7, 1961–1971, https://doi.org/10.5194/acp-7-1961-2007, 2007.
Phillips, V. T. J., Formenton, M., Bansemer, A., Kudzotsa, I., and Lienert, B.: A scheme of sticking efficiency for collisions of snow and graupel with ice crystals: Theory and comparison with observations, J. Atmos. Sci., 72, 4885–4902, https://doi.org/10.1175/JAS-D-14-0096.1, 2015.
Phinney, L. A., Lohmann, U., and Leaitch, W. R.: Limitations of using an equilibrium approximation in an aerosol activation Parameterization, J. Geophys. Res., 108, 4371, https://doi.org/10.1029/2002JD002391, 2003.
Pincus, R., Platnick, S., Ackerman, S. A., Hemler, R. S., and Patrick Hofmann, R. J.: Reconciling Simulated and Observed Views of Clouds: MODIS, ISCCP, and the Limits of Instrument Simulators, J. Climate, 25, 4699–4720, https://doi.org/10.1175/JCLI-D-11-00267.1, 2012.
Pincus, R. and Stevens, B.: Paths to accuracy for radiation parameterizations in atmospheric models, J. Adv. Model. Earth Sy., 5, 225–223, 2013.
Platnick, S.: MODIS Atmosphere L3 Monthly Product. NASA MODIS Adaptive Processing System, Goddard Space Flight Center, USA, https://doi.org/10.5067/MODIS/MOD08_M3.061, 2017.
Platnick, S., King, M. D., Meyer, K. G., Wind, G., Amarasinghe, N., Marchant, B., Arnold, G. T., Zhang, Z. B., Hubanks, P. A., Ridgway, B., and Riedi, J.: MODIS Cloud Optical Properties: User Guide for the Collection 6 Level-2 MOD06/MYD06 Product and Associated Level-3 Datasets, NASA, https://modis-images.gsfc.nasa.gov/_docs/C6MOD06OPUserGuide.pdf, 2015.
Platnick, S., Meyer, K. G., King, M. D., Wind, G., Amarasinghe, N., Marchant, B., Arnold, G. T., Zhang, Z., Hubanks, P. A., Holz, R. E., Yang, P., Ridgway, W. L., and Riedi, J.: The MODIS Cloud Optical and Microphysical Products: Collection 6 Updates and Examples From Terra and Aqua, IEEE T. Geosci. Remote, 55, 502–525, 2017.
Poulsen, C., McGarragh, G., Thomas, G., Christensen, M., Povey, A., Grainger, D., Proud, S., and Hollmann, R.: ESA Cloud Climate Change Initiative (ESA Cloud_cci) data: Cloud_cci ATSR2-AATSR L3C/L3U/L2 CLD_PRODUCTS v2.0, Deutscher Wetterdienst (DWD) and Rutherford Appleton Laboratory (Dataset Producer), https://doi.org/10.5676/DWD/ESA_Cloud_cci/ATSR2-AATSR/V002, 2017.
Randall, D. A., Wood, R. A., Bony, S., Colman, R., Fichefet, T., Fyfe, J., Kattsov, V., Pitman, A., Shukla, J., Srinivasan, J., Stouffer, R. J., Sumi, A., and Taylor, K. E.: Climate Models and Their Evaluation, in: Climate Change 2007: The Physical Science Basis. Contribution of Working Group I to the Fourth Assessment Report of the Intergovernmental Panel on Climate Change, edited by: Solomon, S., Qin, D., Manning, M., Chen, Z., Marquis, M., Averyt, K. B., Tignor, M., and Miller, H. L., Cambridge University Press, Cambridge, United Kingdom and New York, NY, USA, 2007.
Reick, C. H., Raddatz, T., Brovkin, V., and Gayler, V.: Representation of natural and anthropogenic land cover change in MPIESM, J. Adv. Model. Earth Sy., 5, 459–482, 2013.
Roeckner, E., Baeuml, G., Bonaventura, L., Brokopf, R., Esch, M., Giorgetta, M., Hagemann, S., Kirchner, I., Kornblueh, L., Manzini, E., Rhodin, A., Schlese, U., Schulzweida, U., and Tompkins, A.: The atmospheric general circulation model ECHAM5 – Part I: Model description, Tech. Rep. 349, Max-Planck-Institut für Meteorologie, Hamburg, Germany, 2003.
Rossow, W. B. and Schiffer, R. A.: Advances in understanding clouds from ISCCP, B. Am. Meteorol. Soc., 80, 2261–2287, https://doi.org/10.1175/1520-0477(1999)080<2261:AIUCFI>2.0.CO;2, 1999.
Rotstayn, L. D.: A physically based scheme for the treatment of stratiform precipitation in large-scale models. I: Description and evaluation of the microphysical processes, Q. J. Roy. Meteor. Soc., 123, 1227–1282, 1997.
Sant, V., Posselt, R., and Lohmann, U.: Prognostic precipitation with three liquid water classes in the ECHAM5–HAM GCM, Atmos. Chem. Phys., 15, 8717–8738, https://doi.org/10.5194/acp-15-8717-2015, 2015.
Schmidt, G. A., Ruedy, R., Hansen, J. E., Aleinov, I., Bell, N., Bauer, M., Bauer, S., Cairns, B., Canuto, V., Cheng, Y., Del Genio, A., Faluvegi, G., Friend, A. D., Hall, T. M., Hu, Y., Kelley, M., Kiang, N. Y., Koch, D., Lacis, A. A., Lerner, J., Lo, K. K., Miller, R. L., Nazarenko, L., Oinas, V., Perlwitz, J., Perlwitz, J., Rind, D., Romanou, A., Russell, G. L., Sato, M., Shindell, D. T., Stone, P. H., Sun, S., Tausnev, N., Thresher, D., and Yao, M.: Present-Day Atmospheric Simulations Using GISS ModelE: Comparison to In Situ, Satellite, and Reanalysis Data, J. Climate, 19, 153–192, https://doi.org/10.1175/JCLI3612.1, 2006.
Seethala, C. and Horvath, A.: Global assessment of AMSRE and MODIS cloud liquid water path retrievals in warm oceanic clouds, J. Geophys. Res., 115, D13202, https://doi.org/10.1029/2009JD012662, 2010.
Seifert, A. and Beheng, K.: A two-moment cloud microphysics parameterization for mixed-phase clouds. Part 1: Model description, Meteorol. Atmos. Phys., 92, 45, https://doi.org/10.1007/s00703-005-0112-4, 2006.
Siongco, A. C., Hohenegger, C., and Stevens, B.: The Atlantic ITCZ bias in CMIP5 models, Clim. Dynam., 45, 1169–1180, https://doi.org/10.1007/s00382-014-2366-3, 2014.
Siongco, A. C.,Hohenegger, C., and Stevens, B.: Sensitivity of the summertime tropical Atlantic precipitation distribution to convective parameterization and model resolution in ECHAM6, J. Geophys. Res.-Atmos., 122, 2579–2594, https://doi.org/10.1002/2016JD026093, 2017.
Sofiev, M., Soares, J., Prank, M., deLeeuw, G., and Kukkonen, J.: A regional to global model of emission and transport of sea salt particles in the atmosphere, J. Geophys. Res.-Atmos., 116, 4122–4141, https://doi.org/10.1029/2010JD014713, 2011.
Stengel, M., Stapelberg, S., Sus, O., Schlundt, C., Poulsen, C., Thomas, G., Christensen, M., Carbajal Henken, C., Preusker, R., Fischer, J., Devasthale, A., Willén, U., Karlsson, K.-G., McGarragh, G. R., Proud, S., Povey, A. C., Grainger, R. G., Meirink, J. F., Feofilov, A., Bennartz, R., Bojanowski, J. S., and Hollmann, R.: Cloud property datasets retrieved from AVHRR, MODIS, AATSR and MERIS in the framework of the Cloud_cci project, Earth Syst. Sci. Data, 9, 881–904, https://doi.org/10.5194/essd-9-881-2017, 2017a.
Stengel, M., Sus, O., Stapelberg, S., Schlundt, C., Poulsen, C., and Hollmann, R.: ESA Cloud Climate Change Initiative (ESA Cloud_cci) data, Cloud_cci AVHRR-PM L3C/L3U CLD_PRODUCTS v2.0, Deutscher Wetterdienst (DWD), https://doi.org/10.5676/DWD/ESA_Cloud_cci/AVHRR-PM/V002, 2017b.
Stevens, B. and Schwartz, S. E.: Observing and modeling Earth's energy flows, Surv. Geophys., 33, 779–816, 2012.
Stevens, B., Giorgetta, M. A., Esch, M., Mauritsen, T., Crueger, T., Rast, S., Salzmann, M., Schmidt, H., Bader, J., Block, K., Brokopf, R., Fast, I., Kinne, S., Kornblueh, L., Lohmann, U., Pincus, R., Reichler, T., and Roeckner, E.: The atmospheric component of the MPI-M Earth System Model: ECHAM6, J. Adv. Model. Earth Sy., 5, 146–172, https://doi.org/10.1002/jame.20015., 2013.
Stier, P.: Limitations of passive remote sensing to constrain global cloud condensation nuclei, Atmos. Chem. Phys., 16, 6595–6607, https://doi.org/10.5194/acp-16-6595-2016, 2016.
Stier, P., Feichter, J., Kinne, S., Kloster, S., Vignati, E., Wilson, J., Ganzeveld, L., Tegen, I., Werner, M., Balkanski, Y., Schulz, M., Boucher, O., Minikin, A., and Petzold, A.: The aerosol-climate model ECHAM5-HAM, Atmos. Chem. Phys., 5, 1125–1156, https://doi.org/10.5194/acp-5-1125-2005, 2005.
Stier, P., Schutgens, N. A. J., Bellouin, N., Bian, H., Boucher, O., Chin, M., Ghan, S., Huneeus, N., Kinne, S., Lin, G., Ma, X., Myhre, G., Penner, J. E., Randles, C. A., Samset, B., Schulz, M., Takemura, T., Yu, F., Yu, H., and Zhou, C.: Host model uncertainties in aerosol radiative forcing estimates: results from the AeroCom Prescribed intercomparison study, Atmos. Chem. Phys., 13, 3245–3270, https://doi.org/10.5194/acp-13-3245-2013, 2013.
Stubenrauch, C. J., Rossow, W. B., Kinne, S., Ackerman, S., Cesana, G., Chepfer, H., Girolamo, L. D., Getzewich, B., Guignard, A., Heidinger, A., Maddux, B. C., Menzel, W. P., Minnis, P., Pearl, C., Platnick, S., Poulsen, C., Riedi, J., Sun-Mack, S., Walther, A., Winker, D., Zeng, S., and Zhao, G.: Assessment of Global Cloud Datasets from Satellites: Project and Database Initiated by the GEWEX Radiation Panel, B. Am. Meteorol. Soc., 94, 1031–1049, 2013.
Sundqvist, H., Berge, E., and Kristjansson, J. E.: Condensation and Cloud Parameterization Studies with a Mesoscale Numerical Weather Prediction Model, Mon. Weather Rev., 117, 1641–1657, 1989.
Susskind, J., Piraino, P., Rokke, L., Iredell, T., and Mehta, A.: Characteristics of the TOVS Pathfinder Path A dataset, B. Am. Meteorol. Soc., 78, 1449–1472, 1997.
Taylor, K.: Summarizing multiple aspects of model performance in a single diagram, J. Geophys. Res., 106, 7183–7192, 2001.
Taylor, K. E., Williamson, D., and Zwiers, F.: The sea surface temperature and sea ice concentration boundary conditions for AMIP II simulations, PCMDI Report 60, Program for Climate Model Diagnosis and Intercomparison, Lawrence Livermore National Laboratory, 25 pp., available at: https://pcmdi.llnl.gov/report/pdf/60.pdf?id=86 (last access: 14 August 2019), 2000.
Tegen, I., Harrison, S. P., Kohfeld, K., Prentice, I. C., Coe, M. and Heimann, M.: Impact of vegetation and preferential source areas on global dust aerosol: Results from a model study, J. Geophys. Res., 107, 4567–4597, 2002.
Tegen, I., Neubauer, D., Ferrachat, S., Siegenthaler-Le Drian, C., Bey, I., Schutgens, N., Stier, P., Watson-Parris, D., Stanelle, T., Schmidt, H., Rast, S., Kokkola, H., Schultz, M., Schroeder, S., Daskalakis, N., Barthel, S., Heinold, B., and Lohmann, U.: The global aerosol–climate model ECHAM6.3–HAM2.3 – Part 1: Aerosol evaluation, Geosci. Model Dev., 12, 1643–1677, https://doi.org/10.5194/gmd-12-1643-2019, 2019.
Textor, C., Schulz, M., Guibert, S., Kinne, S., Balkanski, Y., Bauer, S., Berntsen, T., Berglen, T., Boucher, O., Chin, M., Dentener, F., Diehl, T., Easter, R., Feichter, H., Fillmore, D., Ghan, S., Ginoux, P., Gong, S., Grini, A., Hendricks, J., Horowitz, L., Huang, P., Isaksen, I., Iversen, I., Kloster, S., Koch, D., Kirkevåg, A., Kristjansson, J. E., Krol, M., Lauer, A., Lamarque, J. F., Liu, X., Montanaro, V., Myhre, G., Penner, J., Pitari, G., Reddy, S., Seland, Ø., Stier, P., Takemura, T., and Tie, X.: Analysis and quantification of the diversities of aerosol life cycles within AeroCom, Atmos. Chem. Phys., 6, 1777–1813, https://doi.org/10.5194/acp-6-1777-2006, 2006.
Tiedtke, M.: A comprehensive mass flux scheme for cumulus parameterization in large-scale models. Mon. Weather Rev., 117, 1779–1800, 1989.
Vial, J., Bony, S., Dufresne, J.-L., and Roehrig, R.: Coupling between lower-tropospheric convective mixing and low-level clouds: Physical mechanisms and dependence on convection scheme, J. Adv. Model. Earth Sy., 8, 1892–1911, https://doi.org/10.1002/2016MS000740, 2016.
Voigt, A., Bony, S., Dufresne, J.-L., and Stevens, B.: The radiative impact of clouds on the shift of the Intertropical Convergence Zone, Geophys. Res. Lett., 41, 4308–4315, https://doi.org/10.1002/2014GL060354, 2014.
Wagner, T. M. and Graf, H. F.: An Ensemble Cumulus Convection Parameterization with Explicit Cloud Treatment, J. Atmos. Sci., 67, 3854–3869 https://doi.org/10.1175/2010jas3485.1, 2010.
Wegener, A.: Thermodynamik der Atmosphäre, Johann Ambrosius Barth, 331 pp., 1911.
West, R. E. L., Stier, P., Jones, A., Johnson, C. E., Mann, G. W., Bellouin, N., Partridge, D. G., and Kipling, Z.: The importance of vertical velocity variability for estimates of the indirect aerosol effects, Atmos. Chem. Phys., 14, 6369–6393, https://doi.org/10.5194/acp-14-6369-2014, 2014.
Wilks, D. S.: The Stippling Shows Statistically Significant Grid Points: How Research Results are Routinely Overstated and Overinterpreted, and What to Do about It, B. Am. Meteorol. Soc., 97, 2263–2273, https://doi.org/10.1175/BAMS-D-15-00267.1, 2016.
Wood, R.: Stratocumulus clouds, Mon. Weather Rev., 140, 2373–2423, https://doi.org/10.1175/MWR-D-11-00121.1, 2012.
Zhang, K., O'Donnell, D., Kazil, J., Stier, P., Kinne, S., Lohmann, U., Ferrachat, S., Croft, B., Quaas, J., Wan, H., Rast, S., and Feichter, J.: The global aerosol-climate model ECHAM-HAM, version 2: sensitivity to improvements in process representations, Atmos. Chem. Phys., 12, 8911–8949, https://doi.org/10.5194/acp-12-8911-2012, 2012.
Zhang, S., Wang, M., Ghan, S. J., Ding, A., Wang, H., Zhang, K., Neubauer, D., Lohmann, U., Ferrachat, S., Takeamura, T., Gettelman, A., Morrison, H., Lee, Y., Shindell, D. T., Partridge, D. G., Stier, P., Kipling, Z., and Fu, C.: On the characteristics of aerosol indirect effect based on dynamic regimes in global climate models, Atmos. Chem. Phys., 16, 2765–2783, https://doi.org/10.5194/acp-16-2765-2016, 2016.
Zelinka, M. D., Zhou, C., and Klein, S. A.: Insights from a refined decomposition of cloud feedbacks, Geophys. Res. Lett., 43, 9259–9269, 2016.
Zhang, Y., Xie, S., Covey, C., Lucas, D. D., Gleckler, P., Klein,S. A., Tannahill, J., Doutriaux, C., and Klein, R.: Regional as-sessment of the parameter-dependent performance of CAM4 insimulating tropical clouds, Geophys. Res. Lett., 39, L14708, https://doi.org/10.1029/2012GL052184, 2012.
Zieger, P., Väisänen, O., Corbin, J. C., Partridge, D. G., Bastel-berger, S., Mousavi-Fard, M., Rosati, B., Gysel, M., Krieger, U. K., Leck, C., Nenes, A., Riipinen, I., Virtanen, A., and Salter, M. E.: Revising the hygroscopicity of inorganic sea salt particles, Nat. Commun., 8, 15883, https://doi.org/10.1038/ncomms15883, 2017.