the Creative Commons Attribution 4.0 License.
the Creative Commons Attribution 4.0 License.
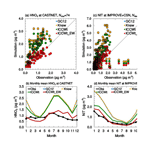
Revised treatment of wet scavenging processes dramatically improves GEOS-Chem 12.0.0 simulations of surface nitric acid, nitrate, and ammonium over the United States
Fangqun Yu
James Schwab
The widely used community model GEOS-Chem 12.0.0 and previous versions have been recognized to significantly overestimate the concentrations of gaseous nitric acid, aerosol nitrate, and aerosol ammonium over the United States. The concentrations of nitric acid are also significantly overpredicted in most global models participating in a recent model intercomparison study. In this study, we show that most or all of this overestimation issue appears to be associated with wet scavenging processes. The replacement of constant in-cloud condensation water (ICCW) assumed in GEOS-Chem standard versions with one varying with location and time from the assimilated meteorology significantly reduces mass loadings of nitrate and ammonium during the wintertime, while the employment of an empirical washout rate for nitric acid significantly decreases mass concentrations of nitric acid and ammonium during the summertime. Compared to the standard version, GEOS-Chem with updated ICCW and washout rate significantly reduces the simulated annual mean mass concentrations of nitric acid, nitrate, and ammonium at surface monitoring network sites in the US from 2.04 to 1.03, 1.89 to 0.88, and 1.09 to 0.68 µg m−3, respectively, in much better agreement with corresponding observed values of 0.83, 0.70, and 0.60 µg m−3, respectively. In addition, the agreement of model-simulated seasonal variations of corresponding species with measurements is also improved. The updated wet scavenging scheme improves the skill of the model in predicting nitric acid, nitrate, and ammonium concentrations, which are important species for air quality and climate.
- Article
(2384 KB) - Full-text XML
-
Supplement
(769 KB) - BibTeX
- EndNote
Nitrate and ammonium are important secondary inorganic aerosols in the atmosphere, contributing significantly to total aerosol mass over most polluted regions (Bian et al., 2017) and to aerosol direct radiative forcing over urban and agriculture regions (Bauer et al., 2007; Myhre et al., 2013). The amount of nitrate and ammonium also regulates the concentration of gaseous ammonia, which often plays an important role in the formation of new particles (Kirkby et al., 2011; Yu et al., 2018). In addition, nitrate and ammonium help newly formed particles grow to larger sizes suitable for cloud condensation nuclei (Yu and Luo, 2009) and can thus impact aerosol indirect radiative forcing (Twomey, 1977).
Nitric acid, nitrate, and ammonium concentrations are often overestimated by atmospheric models (Pye et al., 2009; Walker et al., 2012; Bian et al., 2017; Zakoura and Pandis, 2018), including the widely used community model GEOS-Chem (e.g., Zhang et al., 2012; Heald et al., 2012). Zhang et al. (2012) studied nitrogen deposition over the US with GEOS-Chem and found that both nitric acid and nitrate concentrations are overestimated, especially in wintertime. They suggested that this is the result of excessive nitric acid formation via the nighttime chemistry of heterogeneous N2O5 hydrolysis. However, Heald et al. (2012) found that the overestimate of heterogeneous N2O5 hydrolysis does not fully account for the nitrate bias and suggested that the positive nitrate bias is likely linked with an overestimate of nitric acid concentrations. Heald et al. (2012) investigated other possible causes for the overestimation of nitric acid concentrations arising from uncertainties in daytime formation and dry deposition, and they concluded that none of these uncertainties could fully account for the reduction in nitric acid required to correct the nitrate bias. Based on comparisons of simulated nitrate and ammonium aerosol from nine AEROCOM models with ground station and aircraft measurements, Bian et al. (2017) concluded that most models overestimate the surface nitric acid volume mixing ratio by a factor of up to 3.9 over North America, and the overestimation cannot be simply attributed to model uncertainties. Backes et al. (2016) suggested that uncertainties in the temporal profiles of ammonia emissions could also contribute significantly to the bias of nitrate concentrations. However, the impact of ammonia mostly happened during summertime. Zakoura and Pandis (2018) found a significant decrease in nitrate concentration when they enhanced their model resolution from 36 km × 36 km to 4 km × 4 km in the PMCAMx model. However, similar results are not found in global models with much coarser grids than regional models. All these studies indicate that the overestimation of nitric acid, nitrate, and ammonium mass concentrations in current atmospheric chemistry models remains to be resolved.
In this study, we proposed an improved treatment of wet scavenging in GEOS-Chem by considering cloud condensation water variability and empirical washout rate, which together significantly improve the estimates of nitric acid, nitrate, and ammonium over the US. GEOS-Chem is a global 3-D model of atmospheric chemistry driven by meteorological input from the Goddard Earth Observing System (GEOS) of the NASA Global Modeling and Assimilation Office and includes state-of-the-art routines to deal with emissions, transport, and other key chemical and physical processes for atmospheric trace gases and aerosols (Keller et al., 2014; Fontoukis and Nenes, 2007; Martin et al., 2003; Bey et al., 2001). The improved wet scavenging in GEOS-Chem is described in Sect. 2. The comparison of model results with surface observations and the changes of the three species over the US are presented in Sect. 3. Section 4 is the summary and discussion.
Wet scavenging is the main removal pathway for many atmospheric air pollutants. Two mechanisms are involved in wet scavenging: rainout (in-cloud scavenging) and washout (below-cloud scavenging). GEOS-Chem treats wet scavenging associated with stratiform and convective precipitation separately. The wet deposition scheme in GEOS-Chem is described by Jacob et al. (2000) and Liu et al. (2001) for water-soluble aerosols and by Amos et al. (2012) for gases. Scavenging of aerosol by snow and cold–mixed precipitation is described by Wang et al. (2011, 2014). The first-order rainout parameterization is based on Giorgi and Chameides (1986).
2.1 Impact of in-cloud condensed water (ICCW)
For stratiform precipitation, in the most recently released GEOS-Chem version 12.0.0 (GC12), rainout water-soluble species are parameterized according to Jacob et al. (2000) and Liu at al. (2001) as
where F is the fraction of a water-soluble tracer in the grid box scavenged by rainout, Δt (s) is the model integration time step, and k (s−1) is the first-order rainout loss rate (Giorgi and Chameides, 1986), which represents the conversion of cloud water to precipitation water. ICCW (g m−3) represents the condensed water content (liquid) within the precipitating cloud (i.e., in cloud). Pr (g m−3 s−1) is the rate of new precipitation formation (rain only) in the corresponding grid box.
The rainout loss rate (k) represents how fast cloud condensation water can be removed from the atmosphere and is thus critical for rainout scavenging. k is defined in Jacob et al. (2000) and coded in GC12 (called kGC12 thereafter) as
where kmin (s−1) is the minimum value of rainout loss rate derived from the stochastic collection equation, which indicates that in 1 h at least ∼0.36 cloud droplets are lost to autoconversion–accretion (Beheng and Doms, 1986). In GC12, kmin is set to 0.36 h s−1.
It should be noted that Pr in Eq. (2) is a grid-box mean value, while ICCW is an in-cloud value. To be physically consistent, we suggest a new expression of k (knew) that replaces grid-box mean Pr with the corresponding in-cloud value Pr∕fc:
where fc is the grid-box mean cloud fraction. As we will show later, Eq. (3) gives k values in much better agreement with those derived from cloud model simulations and observations.
To calculate F, GC12 uses Pr from the Modern-Era Retrospective analysis for Research and Applications version 2 (MERRA2) meteorological fields. For ICCW in Eqs. (1)–(3), Jacob et al. (2000) used a constant value of 1.5 g m−3 and Wang et al. (2011) changed it to 1 g m−3. In GC12, the default value of ICCW is 1 g m−3. However, ICCW in the atmosphere varies with time and location. Here we suggest using time- and location-dependent ICCW (named ICCWt), which can be derived from MERRA2 meteorological fields as
where CW is grid-box mean cloud water content, while Pr⋅Δt represents rainwater content produced during the time step Δt. In a previous study, Croft et al. (2016) used cloud liquid and ice water content to replace the fixed ICCW. However, as shown in Eq. (6) in the MERRA2 file specification (Bosilovich et al., 2016), cloud water is the residual condensation water after precipitation and is low when precipitation is occurring. Because the fraction of soluble species rained out should equal the fraction of total condensed water (or ICCW in our case) converted to rainwater, we think that ICCW in Eq. (3) should include rainwater (i.e., Eq. 4).
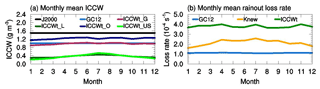
Figure 1(a) Monthly variations of ICCW averaged over the lower troposphere layers of the whole globe (maroon), global land areas (olive), global oceans (navy), and the continental US (green) from MERRA2, along with constant ICCW values assumed in J2000 (black) and GC12 (blue). (b) Monthly variations of the rainout loss rate averaged in the lower troposphere layers of the continental US based on Eq. (2) (i.e, GC12) and Eq. (3) with constant ICCW of 1 g m−3, as well as Eq. (3) with MERRA2 ICCW (Eq. 4).
Figure 1a shows seasonal variations of ICCWt (Eq. 4) averaged throughout the lower troposphere (0–3 km) of the whole globe (ICCWt_G), over all land surface (ICCWt_L), over the oceans (ICCWt_O), and over the continental US (ICCWt_US). For comparison, the constant values of ICCW assumed in Jacob et al. (2000) (ICCWJ2000) and GC12 (ICCWGC12) are also shown. The monthly mean values of ICCWt_G, ICCWt_L, ICCWt_O, and ICCWt_US vary within the ranges of 0.90–1.03, 0.30–0.45, 1.15–1.26, and 0.21–0.53 g m−3, respectively. This figure shows that ICCWt_G is close to the assumed ICCW value of 1 g m−3 used in GC12. As can be seen from Fig. 1a, ICCWt_O is greater than 1 g m−3, but ICCWt_L is much less than the constant value of 1 g m−3 assumed in GC12. The mean ICCW over the continental US (bright green line) is close to ICCWt_L (olive line) and is ∼5 times less than the assumed value in GC12 during the wintertime and ∼2 times less during the summertime. As we will show later, the constant ICCW of 1 g m−3 assumed in GC12 leads to significant underestimation of rainout over the continental US, especially during the wintertime.
Figure 1b shows seasonal variations of mean kGC12, knew, and knew_ICCWt in the lower troposphere (0–3 km) of the continental US. Referring to Eq. (2), the figure shows that kGC12 is dominated by kmin (which is physically unsound) and thus shows negligible seasonal variation. Conversely, knew is low in the wintertime and high in the summertime. knew_ICCWt is 2.3 times higher than knew during January and 1.6 times higher than knew during July. Both knew and knew_ICCWt are within the range of rainout loss rates (10−4–10−3 s−1) indicated by cloud model simulations and estimates based on observations (Giorgi and Chameides, 1986).
From Eqs. (1), (3), and (4), we can get the updated parameterization for rainout loss fraction at each location and time step:
2.2 Impact of empirical washout rate on nitric acid wet scavenging
Still considering the case of stratiform precipitation in GOES-Chem, the fraction of aerosols and HNO3 within a grid box that is scavenged by washout over a time step is parameterized as (Wang et al., 2011; Liu et al., 2001; Jacob et al., 2000)
where fr is the horizontal areal fraction of the grid box experiencing precipitation and ftop is the value of fr in the layer overhead (ftop=0 at the top of the precipitating column). kwash is a washout rate, Λ is washout scavenging coefficient, and b is an exponential coefficient. In the original GEOS-Chem, Λ=1 cm−1 and b=1 for both aerosols and nitric acid (Liu et al., 2001; Jacob et al., 2000).
It has been well recognized that, for aerosols, Λ and b depend on particle size (Wang et al., 2010; Feng, 2007; Andronache et al., 2006; Henzing et al., 2006; Laakso et al., 2003). Feng (2007) suggested values of b=0.62, 0.61, and 0.8 for particles in nucleation (diameter 1–40 nm), accumulation (40 nm–2.5 µm), and coarse mode (>2.5 µm), respectively. Many studies indicate that there are large difference between existing theoretical and observed size-resolved washout rates (Wang et al., 2010; Andronache et al., 2006; Henzing et al., 2006; Laakso et al., 2003). For particles within the diameter range of 0.01–2 µm, size-resolved washout rates derived from analytical formulas are 1 to 2 orders of magnitude smaller than those derived from field measurements (e.g., Wang et al., 2010). This large difference could result from turbulent flow fluctuations (Andronache et al., 2006; Khain and Pinsky, 1997), vertical diffusion process (Zhang et al., 2004), and droplet–particle collection mechanisms (Park et al., 2005).
In GC12, Λ and b for aerosols are parameterized as a function of particle size modes (Wang et al., 2011), following Feng (2007). For nitric acid, GC12 keeps Λ=1 cm−1 and b=1, unchanged from the original GEOS-Chem parameters. In this study, we employ the size-dependent aerosol washout parameterization derived from 6 years of field measurements over forests in southern Finland (Laakso et al., 2003; Wang et al., 2010). We further estimate nitric acid washout scavenging coefficients by referring to field measurements for particles of 10 nm (Laakso et al., 2003) and the theoretical dependence of scavenging coefficients on particle sizes for particles <10 nm (Henzing et al., 2006). The collection efficiency of particles smaller than 10 nm by rain droplets is dominated by Brownian diffusion, and in this regard we can treat nitric acid as a single molecule (or particle) with a diameter of 0.5 nm. Through this approach, we derive the empirical Kwash value for nitric acid to be s−1 when the rain rate is 1 mm h−1. This empirical value is about 2 orders of magnitude larger than the corresponding Kwash value in GC12 (0.1 h s−1). For the dependence of Kwash on rain rate, we adopt the b value of 0.62 for nucleation-mode particles (diameter 1–40 nm) (Feng, 2007) for nitric acid. With this empirical b value of 0.62 and empirical Kwash of s−1, we derive an empirical Λ value for nitric acid of 2. It should be noted that the unit of empirical Λ is not reciprocal centimeters (cm−1) when b is not unity. In our parameterization (Eq. 8), Λ=2 and Pr should be in the unit of centimeters per second (cm s−1). Washout rates for water-soluble aerosols use the empirical values from Laakso et al. (2003), while washout rates for water-insoluble aerosols still use the values from Feng (2007). No change is made to washout by snow, which is based on the approach described in Wang et al. (2011).
For convective precipitation, scavenging in convective updrafts is coupled with convective transport (e.g., Liu et al., 2001). Furthermore, MERRA2 meteorological fields do not provide convective cloud fraction and cloud water content. Therefore, the updated wet scavenging method discussed above for stratiform precipitation cannot be directly applied to convective precipitation rainout scavenging in GEOS-Chem. However, the empirical values for water-soluble aerosol and nitric acid washout are also applied to convective washout in the present study as case 4.
To study the impacts of various updates to the wet scavenging as described in Sect. 2 on model-simulated nitric acid, nitrate, and ammonium mass concentrations, we run GEOS-Chem for four cases: (1) standard GC12 parameterizations for rainout and washout, called GC12; (2) the same as in case GC12 except knew in Eq. (3) is used, and this case is called “Knew”; (3) the same as the Knew case except ICCWt from MERRA2 (Eq. 4) is used, and this case is called ICCWt; (4) the same as case ICCWt except empirical washout rates for nitric acid and water-soluble aerosols are used, and this case is called ICCWt_EW. For each case, we carry out simulations from December 2010 to December 2011, with the first month as spin-up. The model horizontal resolution is and vertically there are 47 layers. The present analysis focuses on the continental United States. We compared simulated nitric acid with in situ observations at Clean Air Status and Trends Network (CASTNET) sites, as well as simulated nitrate and ammonium with in situ observations at Interagency Monitoring of Protected Visual Environments (IMPROVE) and Chemical Speciation Network (CSN) sites. For 2011, there were 74 sites with available nitric acid observations from CASTNET. For the same year, IMPROVE had 120 sites with available nitrate and ammonium observations, while CSN had 94 sites with available nitrate observations and 63 sites with available ammonium observations.
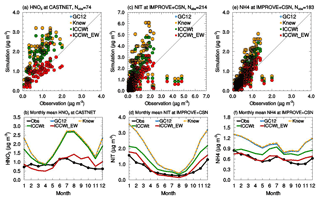
Figure 2(a) Scatter plot of observed and simulated annual mean HNO3 at CASTNET sites and (b) variations of monthly mean for the year 2011 showing the comparison between nitric acid mass concentrations observed at CASTNET sites (black) and simulated by the GC12 (blue), Knew (yellow dash), ICCWt (green), and ICCWt_EW (red) cases. Panels (c) and (d) are the same as panels (a) and (b) but for nitrate at IMPROVE+CSN sites. Panels (e) and (f) are the same as panels (a) and (b) but for ammonium at IMPROVE+CSN sites. It is worth noting that the differences between G12 (blue) and Knew (yellow dash) are small.
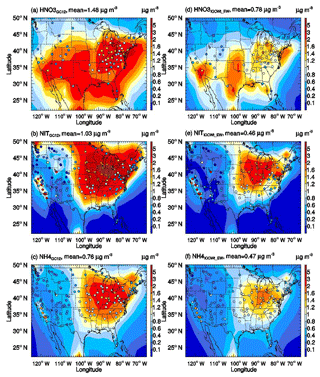
Figure 3Horizontal distributions of surface-layer nitric acid, nitrate, and ammonium simulated by the GC12 case (a–c) and the ICCWt_EW case (d–f). Filled circles are annual mean surface mass concentrations observed at CASTNET, IMPROVE, and CSN for corresponding species.
Table 1Observed annual mean surface concentrations of HNO3, nitrate, and ammonium at CASTNET, IMPROVE, and CSN sites. Annual mean surface concentrations (Mean), normalized mean bias (NMB), and correlation coefficient (r) between observed and simulated annual mean values for the three species for the GC12, Knew, ICCWt, and ICCWt_EW cases.
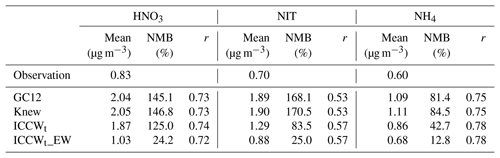
The effects of different modifications to the GC12 wet scavenging parameterization on model-simulated nitric acid, nitrate, and ammonium mass concentrations are shown in Figs. 2–3 and Table 1. Most of the changes in the mass concentrations of the three species over the US are caused by changes in cloud condensation variability and/or empirical washout rate. The impact of the new rainout loss rate (knew) is relatively small because of the canceling effect of k in the denominator and also in the exponent in Eq. (1). As shown in Fig. 2a–b and Table 1, all cases except ICCWt_EW overestimate nitric acid at CASTNET sites by a factor of 2–3 in both wintertime and summertime. Consideration of cloud condensation water variability slightly reduces nitric acid in January and December but has a negligible effect during other months. The inclusion of the empirical washout rate reduces the normalized mean bias (NMB) of nitric acid from 125 % to 24 % (Table 1). Figure 2c and d show the impacts of improved wet scavenging on nitrate. It is clear that GC12 significantly overestimates the nitrate concentration at most sites, especially during the wintertime, in agreement with previous studies (Heald et al., 2012; Walker et al., 2012). Replacing constant ICCW with variable ICCWt reduces the NMB of nitrate from 170 % to 84 %. ICCW has a significant impact on reducing the nitrate mass concentration during the wintertime and a smaller impact during the summertime. The wintertime bias of nitrate was reduced from 2 to 0.7 µg m−3. The change in washout rate from a theoretical value to an empirical formula results in an additional 59 % reduction of NMB for nitrate and impacts the nitrate mass concentration significantly in both winter and summer. For ammonium, NMB is reduced from 85 % to 43 % after considering rainout with variable cloud condensation water. Similar to nitrate, the impact of ICCW is large during the wintertime and smaller during the summertime. After considering empirical washout, the NMB of ammonium is reduced to 13 %. While the update in the wet scavenging parameterization significantly improves the agreement of the model-simulated mass concentrations of nitric acid, nitrate, and ammonium over the US with those observed, it does not affect the correlation coefficients of annual mean values (Table 1), which are dominated by spatial distributions (Fig. 3).
Figure 3 shows the horizontal distributions of surface-layer nitric acid, nitrate, and ammonium mass concentrations over the US for case GC12 (panels a–c) and case ICCWt_EW (panels d–f). For comparison, annual mean mass concentrations observed at CASTNET, IMPROVE, and CSN sites are shown in filled cycles. The spatial pattern of the simulated concentrations of the three species for the ICCW_EW case is close to those for the GC12 case. High concentrations of nitric acid are mainly located in the northeastern, southern, and western US, with values up to 2–4 µg m−3 based on GC12 (Fig. 3a) and 1–2 µg m−3 based on ICCWt_EW (Fig. 3d). The horizontal distribution of nitrate is different from that of nitric acid. Nitrate is mainly located in the Ohio Valley region and the northeastern US, with values up to 4–5 µg m−3 based on GC12 (Fig. 3b) and 1–3 µg m−3 based on ICCWt_EW (Fig. 3e). Ammonium shows a similar horizontal distribution to that of nitrate, but its value is ∼50 % lower than the nitrate concentration. For the whole continental US domain, the annual mean nitric acid, nitrate, and ammonium concentrations in the model surface layer are reduced from 1.48 to 0.78, 1.03 to 0.46, and 0.76 to 0.47 µg m−3, respectively. The percentage changes for nitric acid, nitrate, and ammonium concentrations averaged within the domain are −47 %, −55 %, and −38 %, respectively. The improved wet scavenging treatment had significant impacts on nitric acid, nitrate, and ammonium modeling over the US. As can be seen from Fig. 3a–f (and also Fig. 2 and Table 2), simulated nitric acid, nitrate, and ammonium mass concentrations over the US based on the updated wet scavenging parameterization (i.e., ICCWt_EW) are in much better agreement with in situ measurements.
We present an improved wet scavenging parameterization for use in GEOS-Chem by considering cloud condensation water variability and an empirical washout rate. The updated parameterization significantly reduces the overestimation of simulated annual mean mass concentrations of nitric acid, nitrate, and ammonium at CASTNET, IMPROVE, and CSN sites in the US from 2.04 to 1.03 µg m−3 (observation: 0.83), 1.89 to 0.88 µg m−3 (observation: 0.70), and 1.09 to 0.68 µg m−3 (observation: 0.60), respectively. In addition, the agreement of model-simulated seasonal variations of corresponding species with measurements is also improved. The updated wet scavenging scheme provides a partial solution to the persistent problem of nitric acid and nitrate overestimation in the widely used community model GEOS-Chem (e.g., Heald et al., 2012) and thus improves the skill of the model in predicting nitric acid, nitrate, and ammonium concentrations. It should be noted that in the present study the cloud condensation water variability is considered only for stratiform cloud rainout. Convective cloud removal is important (especially for tropical regions) and is necessary to be studied as well, calling for the output of convective cloud fraction and cloud water content fields in future Global Modeling and Assimilation Office (GMAO) reanalysis products.
The empirical washout rate suggested in the present work will also help to resolve the significant overprediction of nitric acid by most of the nine global models participating in the Aerosol Comparisons between Observations and Models (AEROCOM) phase III study (Bian et al., 2017). Due to the large difference in nitric acid washout rate based on theoretical and field studies and the importance of this rate, further research is needed to better understand the underlying reasons and reduce the difference. For the time being, we recommend the empirical values to be used in models. The revised rainout scheme presented in this study can be applied to other atmospheric chemistry models assuming constant cloud condensation water. The changes in nitrate and ammonium mass concentrations not only impact particle growth but also influence ammonia concentrations, which are important for aerosol nucleation (Kirkby et al., 2011; Yu et al., 2018), via the equilibrium of sulfate–nitrate–ammonium. The updated scheme presented in this study has potential implications to new particle formation, particle growth, aerosol size, cloud condensation nuclei (CCN) number concentration, and associated radiative forcing, which will be the subjects of future research.
In this study, we only evaluated the impacts of the updated wet scavenging parameterization on nitric acid, nitrate, and ammonium concentrations at the surface level over the US. The updated wet scavenging parameterization can also impact other soluble tracers. For example, as shown in the Supplement (Table S1 and Fig. S1), applying ICCW and an empirical washout rate can reduce the simulated annual mean mass concentrations of sulfate at IMPROVE and CSN sites in the US from 1.56 to 1.18 µg m−3 (observation: 1.30). The NMB is changed from 20 % to −9 %. The impacts of the updated wet scavenging parameterization on the concentrations of all major aerosols over the whole globe should be carefully assessed against relevant measurements in future studies. In addition, the impact of the updated treatment of wet scavenging on aerosol vertical profiles and mass loading should be investigated. A previous study by Liu et al. (2001) indicates that Pb-210 is a good tracer for testing wet deposition in GEOS-Chem. It will be helpful to carry out Pb-210 simulations to further evaluate the updated wet scavenging parameterization.
The code of GEOS-Chem 12.0.0 is available through the GEOS-Chem description web page at http://wiki.seas.harvard.edu/geos-chem/index.php/GEOS-Chem_12 (last access: December 2018; The International GEOS-Chem User Community, 2018). All measurement data are publicly available.
The supplement related to this article is available online at: https://doi.org/10.5194/gmd-12-3439-2019-supplement.
GL and FY proposed and implemented the revised wet scavenging scheme and validated model simulations with surface observations. JS provided useful suggestions to improve this work. All authors contributed to the writing and editing of the paper.
The authors declare that they have no conflict of interest.
We would like to acknowledge the Interagency Monitoring of Protected Visual Environments (IMPROVE), Chemical Speciation Network (CSN), and Clean Air Status and Trends Network (CASTNET) for the on-site measurement data. GEOS-Chem is a community model maintained by the GEOS-Chem Support Team at Harvard University.
This research has been supported by NYSERDA (grant no. 100416), NASA (grant no. NNX13AK20G), and the NSF (grant no. 1550816).
This paper was edited by Samuel Remy and reviewed by two anonymous referees.
Amos, H. M., Jacob, D. J., Holmes, C. D., Fisher, J. A., Wang, Q., Yantosca, R. M., Corbitt, E. S., Galarneau, E., Rutter, A. P., Gustin, M. S., Steffen, A., Schauer, J. J., Graydon, J. A., Louis, V. L. St., Talbot, R. W., Edgerton, E. S., Zhang, Y., and Sunderland, E. M.: Gas-particle partitioning of atmospheric Hg(II) and its effect on global mercury deposition, Atmos. Chem. Phys., 12, 591–603, https://doi.org/10.5194/acp-12-591-2012, 2012.
Andronache, C., Grönholm, T., Laakso, L., Phillips, V., and Venäläinen, A.: Scavenging of ultrafine particles by rainfall at a boreal site: observations and model estimations, Atmos. Chem. Phys., 6, 4739–4754, https://doi.org/10.5194/acp-6-4739-2006, 2006.
Backes, A., Aulinger, A., Bieser, J., Matthias, V., and Quante, M.: Ammonia emissions in Europe, part I: development of a dynamical ammonia emission inventory. Atmos. Environ. 131, 55–66, 2016.
Bauer, S. E., Koch, D., Unger, N., Metzger, S. M., Shindell, D. T., and Streets, D. G.: Nitrate aerosols today and in 2030: a global simulation including aerosols and tropospheric ozone, Atmos. Chem. Phys., 7, 5043–5059, https://doi.org/10.5194/acp-7-5043-2007, 2007.
Beheng, K. D. and Doms, G.: A general formulation of collection rates of cloud and raindrops using the kinetic equation and comparison with parameterizations, Beitr. Phys. Atmos., 59, 66–84, 1986.
Bey, I., Jacob, D. J., Yantosca, R. M., Logan, J. A., Field, B., Fiore, A. M., Li, Q., Liu, H., Mickley, L. J., and Schultz, M.: Global modeling of tropospheric chemistry with assimilated meteorology: Model description and evaluation, J. Geophys. Res., 106, 23073–23096, https://doi.org/10.1029/2001JD000807, 2001.
Bian, H., Chin, M., Hauglustaine, D. A., Schulz, M., Myhre, G., Bauer, S. E., Lund, M. T., Karydis, V. A., Kucsera, T. L., Pan, X., Pozzer, A., Skeie, R. B., Steenrod, S. D., Sudo, K., Tsigaridis, K., Tsimpidi, A. P., and Tsyro, S. G.: Investigation of global particulate nitrate from the AeroCom phase III experiment, Atmos. Chem. Phys., 17, 12911–12940, https://doi.org/10.5194/acp-17-12911-2017, 2017.
Bosilovich, M. G., Lucchesi, R., and Suarez, M.: MERRA-2: File Specification. GMAO Office Note No. 9 (Version 1.1), 73 pp., available at: https://gmao.gsfc.nasa.gov/pubs/docs/Bosilovich785.pdf (last access: January 2019), 2016.
Croft, B., Martin, R. V., Leaitch, W. R., Tunved, P., Breider, T. J., D'Andrea, S. D., and Pierce, J. R.: Processes controlling the annual cycle of Arctic aerosol number and size distributions, Atmos. Chem. Phys., 16, 3665–3682, https://doi.org/10.5194/acp-16-3665-2016, 2016.
Feng, J.: A 3-mode parameterization of below-cloud scavenging of aerosols for use in atmospheric dispersion models, Atmos. Environ., 41, 6808–6822, 2007.
Fountoukis, C. and Nenes, A.: ISORROPIA II: a computationally efficient thermodynamic equilibrium model for K+–Ca2+–Mg2+––Na+–––Cl−–H2O aerosols, Atmos. Chem. Phys., 7, 4639–4659, https://doi.org/10.5194/acp-7-4639-2007, 2007.
Giorgi, F. and Chameides, W. L.: Rainout lifetimes of highly soluble aerosols and gases as inferred from simulations with a general circulation model, J. Geophys. Res., 91, 14367–14376, https://doi.org/10.1029/JD091iD13p14367, 1986.
Heald, C. L., Collett Jr., J. L., Lee, T., Benedict, K. B., Schwandner, F. M., Li, Y., Clarisse, L., Hurtmans, D. R., Van Damme, M., Clerbaux, C., Coheur, P.-F., Philip, S., Martin, R. V., and Pye, H. O. T.: Atmospheric ammonia and particulate inorganic nitrogen over the United States, Atmos. Chem. Phys., 12, 10295–10312, https://doi.org/10.5194/acp-12-10295-2012, 2012.
Henzing, J. S., Olivié, D. J. L., and van Velthoven, P. F. J.: A parameterization of size resolved below cloud scavenging of aerosols by rain, Atmos. Chem. Phys., 6, 3363–3375, https://doi.org/10.5194/acp-6-3363-2006, 2006.
Jacob, D. J., Liu, H., Mari, C., and Yantosca, B. M., Harvard wet deposition scheme for GMI, available at: http://acmg.seas.harvard.edu/geos/wiki_docs/deposition/wetdep.jacob_etal_2000.pdf (last access: January 2019), 2000.
Keller, C. A., Long, M. S., Yantosca, R. M., Da Silva, A. M., Pawson, S., and Jacob, D. J.: HEMCO v1.0: a versatile, ESMF-compliant component for calculating emissions in atmospheric models, Geosci. Model Dev., 7, 1409–1417, https://doi.org/10.5194/gmd-7-1409-2014, 2014.
Khain, A. P. and Pinsky, M. B., Turbulence effects on the collision kernel, II: Increase of the swept volume of colliding drops, Q. J. Roy. Meteor. Soc., 123, 1543–1560, 1997.
Kirkby, J., Curtius, J., Almeida, J., Dunne, E., Duplissy, J., Ehrhart, S., Franchin, A., Gagné, S., Ickes, L., Kürten, A., Kupc, A., Metzger, A., Riccobono, L. Rondo, S. Schobesberger, G. Tsagkogeorgas, D. Wimmer, A. Amorim, F., Bianchi, F., Breitenlechner, M., David, A., Dommen, J., Downard, A., Ehn, M., Flagan, R. C., Haider, S., Hansel, A., Hauser, D., Jud, W., Junninen, H., Kreissl, F., Kvashin, A., Laaksonen, A., Lehtipalo, K., Lima, J., Lovejoy, E. R., Makhmutov, V., Mathot, S., Mikkilä, J., Minginette, P., Mogo, S., Nieminen, T., Onnela, A., Pereira, P., Petäjä, T., Schnitzhofer, R., Seinfeld, J. H., Sipilä, M., Stozhkov, Y., Stratmann, F., Tomé, A., Vanhanen, J., Viisanen, Y., Vrtala, A., Wagner, P. E., Walther, H., Weingartner, E., Wex, H., Winkler, P. M., Carslaw, K. S., Worsnop, D. R., Baltensperger, U., and Kulmala, M.: Role of sulphuric acid, ammonia and galactic cosmic rays in atmospheric aerosol nucleation, Nature, 476, 429–433, 2011.
Laakso, L., Grönholm, T., Rannik, U., Kosmale, M., Fiedler, V., Vehkamäki, H., and Kulmala, M.: Ultrafine particle scavenging coefficients calculated from 6 years field measurements, Atmos. Environ., 37, 3605–3613, 2003.
Liu, H. Y., Jacob, D. J., Bey, I., and Yantosca, R. M.: Constraints from Pb-210 and Be-7 on wet deposition and transport in a global three-dimensional chemical tracer model driven by assimilated meteorological fields, J. Geophys. Res.-Atmos., 106, 12109–12128, 2001.
Martin, R. V., Jacob, D. J., Yantosca, R. M., Chin, M., and Ginoux, P., Global and regional decreases in tropospheric oxidants from photochemical effects of aerosols, J. Geophys. Res., 108, 4097, https://doi.org/10.1029/2002JD002622, 2003.
Myhre, G., Samset, B. H., Schulz, M., Balkanski, Y., Bauer, S., Berntsen, T. K., Bian, H., Bellouin, N., Chin, M., Diehl, T., Easter, R. C., Feichter, J., Ghan, S. J., Hauglustaine, D., Iversen, T., Kinne, S., Kirkevåg, A., Lamarque, J.-F., Lin, G., Liu, X., Lund, M. T., Luo, G., Ma, X., van Noije, T., Penner, J. E., Rasch, P. J., Ruiz, A., Seland, Ø., Skeie, R. B., Stier, P., Takemura, T., Tsigaridis, K., Wang, P., Wang, Z., Xu, L., Yu, H., Yu, F., Yoon, J.-H., Zhang, K., Zhang, H., and Zhou, C.: Radiative forcing of the direct aerosol effect from AeroCom Phase II simulations, Atmos. Chem. Phys., 13, 1853–1877, https://doi.org/10.5194/acp-13-1853-2013, 2013.
Park, S. H., Jung, C. H., Jung, K. R., Lee, B. K., and Lee, K. W.: Wet scrubbing of polydisperse aerosols by freely falling droplets, Aerosol Sci., 36, 1444–1458, 2005.
Pye, H. O. T., Liao, H., Wu, S., Mickley, L. J., Jacob, D. J., Henze, D. K., and Seinfeld, J. H.: Effect of changes in climate and emissions on future sulfate-nitrate-ammonium aerosol levels in the United States, J. Geophys. Res., 114, D01205, https://doi.org/10.1029/2008JD010701, 2009.
The International GEOS-Chem User Community: geoschem/geos-chem: GEOS-Chem 12.0.0 release (Version 12.0.0), Zenodo, https://doi.org/10.5281/zenodo.1343547, 2018.
Twomey, S.: The influence of pollution on the shortwave albedo of clouds, J. Atmos. Sci., 34, 1149–1152, 1977.
Walker, J. M., Philip, S., Martin, R. V., and Seinfeld, J. H.: Simulation of nitrate, sulfate, and ammonium aerosols over the United States, Atmos. Chem. Phys., 12, 11213–11227, https://doi.org/10.5194/acp-12-11213-2012, 2012.
Wang, Q., Jacob, D. J., Fisher, J. A., Mao, J., Leibensperger, E. M., Carouge, C. C., Le Sager, P., Kondo, Y., Jimenez, J. L., Cubison, M. J., and Doherty, S. J.: Sources of carbonaceous aerosols and deposited black carbon in the Arctic in winter-spring: implications for radiative forcing, Atmos. Chem. Phys., 11, 12453–12473, https://doi.org/10.5194/acp-11-12453-2011, 2011.
Wang, Q., Jacob, D. J., Spackman, J. R., Perring, A. E., Schwarz, J. P., Moteki, N., Marais, E. A., Ge, C., Wang, J., and Barrett, S. R. H.: Global budget and radiative forcing of black carbon aerosol: constraints from pole-to-pole (HIPPO) observations across the Pacific, J. Geophys. Res., 119, 195–206, 2014.
Wang, X., Zhang, L., and Moran, M. D.: Uncertainty assessment of current size-resolved parameterizations for below-cloud particle scavenging by rain, Atmos. Chem. Phys., 10, 5685–5705, https://doi.org/10.5194/acp-10-5685-2010, 2010.
Yu, F. and Luo, G.: Simulation of particle size distribution with a global aerosol model: contribution of nucleation to aerosol and CCN number concentrations, Atmos. Chem. Phys., 9, 7691–7710, https://doi.org/10.5194/acp-9-7691-2009, 2009.
Yu, F., Nadykto, A. B., Herb, J., Luo, G., Nazarenko, K. M., and Uvarova, L. A.: ternary ion-mediated nucleation (TIMN): kinetic-based model and comparison with CLOUD measurements, Atmos. Chem. Phys., 18, 17451–17474, https://doi.org/10.5194/acp-18-17451-2018, 2018.
Zakoura, M. and Pandis, S. N.: Overprediction of aerosol nitrate by chemical transport models: The role of grid resolution, Atmos. Environ. 187, 390–400, 2018.
Zhang, L., Michelangeli, D. V., and Taylor, P. A., Numerical studies of aerosol scavenging in low-level, warm stratiform clouds and precipitation, Atmos. Environ., 38, 4653–4665, 2004.
Zhang, L., Jacob, D. J., Knipping, E. M., Kumar, N., Munger, J. W., Carouge, C. C., van Donkelaar, A., Wang, Y. X., and Chen, D.: Nitrogen deposition to the United States: distribution, sources, and processes, Atmos. Chem. Phys., 12, 4539–4554, https://doi.org/10.5194/acp-12-4539-2012, 2012.