the Creative Commons Attribution 3.0 License.
the Creative Commons Attribution 3.0 License.
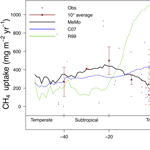
Soil Methanotrophy Model (MeMo v1.0): a process-based model to quantify global uptake of atmospheric methane by soil
Fabiola Murguia-Flores
Sandra Arndt
Anita L. Ganesan
Guillermo Murray-Tortarolo
Edward R. C. Hornibrook
Soil bacteria known as methanotrophs are the sole biological sink for atmospheric methane (CH4), a potent greenhouse gas that is responsible for ∼ 20 % of the human-driven increase in radiative forcing since pre-industrial times. Soil methanotrophy is controlled by a plethora of factors, including temperature, soil texture, moisture and nitrogen content, resulting in spatially and temporally heterogeneous rates of soil methanotrophy. As a consequence, the exact magnitude of the global soil sink, as well as its temporal and spatial variability, remains poorly constrained. We developed a process-based model (Methanotrophy Model; MeMo v1.0) to simulate and quantify the uptake of atmospheric CH4 by soils at the global scale. MeMo builds on previous models by Ridgwell et al. (1999) and Curry (2007) by introducing several advances, including (1) a general analytical solution of the one-dimensional diffusion–reaction equation in porous media, (2) a refined representation of nitrogen inhibition on soil methanotrophy, (3) updated factors governing the influence of soil moisture and temperature on CH4 oxidation rates and (4) the ability to evaluate the impact of autochthonous soil CH4 sources on uptake of atmospheric CH4. We show that the improved structural and parametric representation of key drivers of soil methanotrophy in MeMo results in a better fit to observational data. A global simulation of soil methanotrophy for the period 1990–2009 using MeMo yielded an average annual sink of 33.5 ± 0.6 Tg CH4 yr−1. Warm and semi-arid regions (tropical deciduous forest and open shrubland) had the highest CH4 uptake rates of 602 and 518 mg CH4 m−2 yr−1, respectively. In these regions, favourable annual soil moisture content (∼ 20 % saturation) and low seasonal temperature variations (variations < ∼ 6 ∘C) provided optimal conditions for soil methanotrophy and soil–atmosphere gas exchange. In contrast to previous model analyses, but in agreement with recent observational data, MeMo predicted low fluxes in wet tropical regions because of refinements in formulation of the influence of excess soil moisture on methanotrophy. Tundra and mixed forest had the lowest simulated CH4 uptake rates of 176 and 182 mg CH4 m−2 yr−1, respectively, due to their marked seasonality driven by temperature. Global soil uptake of atmospheric CH4 was decreased by 4 % by the effect of nitrogen inputs to the system; however, the direct addition of fertilizers attenuated the flux by 72 % in regions with high agricultural intensity (i.e. China, India and Europe) and by 4–10 % in agriculture areas receiving low rates of N input (e.g. South America). Globally, nitrogen inputs reduced soil uptake of atmospheric CH4 by 1.38 Tg yr−1, which is 2–5 times smaller than reported previously. In addition to improved characterization of the contemporary soil sink for atmospheric CH4, MeMo provides an opportunity to quantify more accurately the relative importance of soil methanotrophy in the global CH4 cycle in the past and its capacity to contribute to reduction of atmospheric CH4 levels under future global change scenarios.
Please read the corrigendum first before continuing.
-
Notice on corrigendum
The requested paper has a corresponding corrigendum published. Please read the corrigendum first before downloading the article.
-
Article
(7130 KB)
- Corrigendum
-
Supplement
(20612 KB)
-
The requested paper has a corresponding corrigendum published. Please read the corrigendum first before downloading the article.
- Article
(7130 KB) - Full-text XML
- Corrigendum
-
Supplement
(20612 KB) - BibTeX
- EndNote
Methane (CH4) is the most abundant organic trace gas in the atmosphere and responsible for approximately 20 % of the human-driven increase in radiative forcing since pre-industrial times (Myhre et al., 1998; Ciais et al., 2013). Anthropogenic activities during the last 200 years have increased the concentration of CH4 in the atmosphere from pre-industrial era levels of approximately 710 parts per billion (ppb) to the current mixing ratio of approximately 1800 ppb (Etheridge et al., 1998; Kirschke et al., 2013). The atmospheric lifetime of CH4 is 9.1 ± 0.9 years (Prather et al., 2012) and most CH4 is consumed in the troposphere via oxidation by OH radicals, which represents ∼ 90 % of the global CH4 sink (Prather et al., 2012; Ciais et al., 2013). Soil bacteria known as methanotrophs consume ∼ 9 to 10 % of atmospheric CH4 and a further ∼ 1 % is oxidized by reaction with chlorine radicals from sea salt in the marine boundary layer (Allan et al., 2007; Ciais et al., 2013).
Soil methanotrophy is the only biological sink for CH4 and its rate is highly dependent on environmental conditions. The total global soil sink is similar in size to global emissions of CH4 from rice paddies (Kirschke et al., 2013), and consequently, year-to-year changes in factors that impact rates of soil CH4 oxidation may contribute to variability in the interannual growth rate of atmospheric CH4. Moreover, soil methanotrophy consumes up to 90 % of CH4 produced via methanogenesis in persistently or periodically wet soil and thus factors that impact soil uptake of atmospheric CH4 may reduce the capacity of soil methanotrophs to attenuate emission of soil-produced CH4 (Oremland and Culbertson, 1992; Singh et al., 2010).
The rate of methanotrophy in soil is controlled by several environmental factors including temperature, soil texture, moisture and nitrogen (N) content (Czepiel et al., 1995; Le Mer and Roger, 2001; Wang et al., 2005). The influence of these factors on rates of CH4 oxidation has been widely studied both at the ecosystem level and under laboratory conditions. Positive correlations have been consistently reported between temperature and rates of CH4 oxidation in soil (Castro et al., 1995; Butterbach-Bahl and Papen, 2002; Rosenkranz et al., 2006; Luo et al., 2013). Atypically low and high soil moisture levels both have a negative impact on rates of atmospheric CH4 consumption. A soil moisture content of ∼ 20 % appears to yield optimum rates of CH4 uptake in different ecosystems, including tropical forests, short-grass steppe and tundra (Adamsen and King, 1993; Mosier et al., 2002; Burke et al., 1999; Castro et al., 1995; Epstein et al., 1998; Klemedtsson and Klemedtsson, 1997; McLain and Ahmann, 2007; West et al., 1999). Soil texture impacts the ability of soil to retain water and influences diffusion of atmospheric CH4 and O2 into soil because of its control on pore size and connectivity. Thus, sandy soil generally exhibits higher rates of CH4 uptake than silt-rich soil followed by clayey soil (Born et al., 1990; Dörr et al., 1993). The influence of N input from atmospheric deposition and fertilizer application is more complex; however, the majority of studies report inhibition of soil methanotrophy with increased addition of N (Aronson and Helliker, 2010; Bodelier and Laanbroek, 2004; Fang et al., 2014).
There is a large interannual variability and uncertainty in the accounting of the global CH4 budget, particularly for processes that consume CH4 (Kirschke et al., 2013). Our understanding of the main drivers of CH4 uptake in soils and how those factors respond to climate change is incomplete. Estimates of the soil CH4 sink based upon field data (Dutaur and Verchot, 2007) show high variability globally and within different ecosystems. Numerical models provide an efficient means to deal with the spatial and temporal heterogeneity and to evaluate mechanistic understanding of physical and biological processes that influence soil methanotrophy. Ultimately, models enable derivation of regional and global estimates of soil uptake of atmospheric CH4 and provide the ability to predict the response of soil methanotrophy to past and future global change. In addition, they provide a platform of interdisciplinary knowledge synthesis, help identify the most important parameters and environmental controls, and can thus inform future field and laboratory research.
Several detailed biogeochemical models have been developed to quantify consumption of atmospheric CH4 by soil. Saggar et al. (2007) produced a modified version (the denitrification–decomposition model for New Zealand – NZ-DNDC) of DNDC (Li et al., 2000) to evaluate local impacts of changes in climate, soil properties, fertilizer management and grazing regimes on soil methanotrophy. Sabrekov et al. (2016) developed a process-based model of soil CH4 uptake that also incorporates rhizosphere methanotrophy. Oh et al. (2016) developed a model (XHAM) that explicitly simulates high-affinity methanotrophy and active microbial biomass dynamics. These models are driven by high-resolution local data sets, which presents challenges for conducting global simulations of soil methanotrophy because of limited availability of input data necessary to drive the models (e.g. global rhizosphere depth, specific soil management, specific metabolic data and enzyme concentrations).
Previous global models included Potter et al. (1996) (hereafter referred to as the “P96” model), which estimates terrestrial uptake of CH4 by calculating diffusive flux of atmospheric CH4 into soil using a modified version of Fick's first law. Ridgwell et al. (1999) (hereafter referred to as the “R99” model) improved the P96 model by explicitly accounting for microbial CH4 oxidation in soil. The R99 model quantifies CH4 oxidation rates as a function of soil temperature, moisture and N content. The latter parameter was estimated using agricultural land area as a proxy for fertilizer application. Solution of the resulting one-dimensional diffusion–reaction equation was approximated semi-numerically assuming steady-state conditions. Curry (2007) (hereafter referred to as the “C07” model) employed a steady-state analytical solution of the one-dimensional diffusion–reaction equation and introduced a scalar modifier to account for the regulation of CH4 oxidation rates by soil moisture and the impact of temperature below 0 ∘C. The C07 model continued to use the R99 agricultural land area approximation to evaluate the effect of N loading on CH4 uptake. The C07 model has been employed as a reference model for the Global Carbon Project (Saunois et al., 2016) and has been used to estimate global CH4 uptake in dynamic global vegetation models, such as the Lund–Potsdam–Jena model (LPJ-WHyMe; Wania et al., 2010; Spahni et al., 2011).
The model of Zhuang et al. (2013) (hereafter referred to as model “Z13”) employs the same steady-state diffusion–reaction equation for CH4 as previous models; however, Z13 solves the steady-state diffusion–reaction equation for CH4 numerically using multiple soil layers. Additionally, parameterization of microbial activity in model Z13 is based upon redox potential, ecosystem-specific inputs for Q10 and optimum soil moisture, and maximum rates of CH4 consumption instead of a base rate for CH4 oxidation. Consequently, model Z13 operates within the Terrestrial Ecosystem Model (TEM) that provides the necessary driving data because global data sets for many of these parameters are not available. If external data were available, model Z13 presumably could be operated independently of the TEM in a manner similar to the P96, R99 and C07 models. However, such a stand-alone application (i.e. decoupled from TEM) would require a new implementation or presumably significant modifications to the code.
We have chosen to focus on refining the R99 and C07 models because availability of new observational and experimental data presents an opportunity to re-evaluate global simulations of soil methanotrophy based upon an enhanced version of these models. For example, new global data sets quantifying N deposition and N input via fertilizers now enable better representation of this inhibitory effect on soil uptake of atmospheric CH4 (Lamarque et al., 2013). In addition, a new global inventory of CH4 uptake rates in soil (Dutaur and Verchot, 2007) provides a means to better compare and validate model simulations.
Here, we present an updated process-based model to quantity the global sink for atmospheric CH4 by soil (hereafter referred to as “MeMo”: soil Methanotrophy Model). MeMo is based on a general analytical solution of the one-dimensional diffusion–reaction equation, which makes obsolete the a priori assumption of complete CH4 consumption in the model domain applied in the C07 model. The refinement now also provides the opportunity to account for CH4 flux from below (i.e. due to CH4 production in soil, if present) and to set a minimum methane concentration threshold at which methanotrophy can occur in the soil column. In addition, MeMo revisits and improves R99 and C07 model formulations to incorporate advances in the mechanistic understanding of soil methanotrophy that have resulted from availability of new data. Finally, MeMo utilizes for the first time data for atmospheric N deposition and N input from fertilizers to explore more accurately the effect of land use and land-use changes on the global CH4 sink. We present a comprehensive description of the new model, a comparison of MeMo with the R99 and C07 models, and a critical discussion of model formulations and assumptions based on observational data. We then provide an assessment of global and regional soil uptake and variability across ecosystem types and seasons.
The following sections provide a detailed description of MeMo in the context of existing global soil CH4 uptake. Table 1 provides a summary of all terms, names and units used in the model description section, while Table 2 contains a short summary of the four global CH4 uptake models based on the P96 family.
2.1 Conservation equation
The general, one-dimensional mass conservation equation for CH4 in soil is given by
where denotes the flux of CH4 and ΣR is the sum of all production and consumption processes that affect CH4 concentrations in soil. The flux in the soil is generally controlled by diffusion. Consequently, the P96 model assumes that global uptake of atmospheric CH4 by soil is diffusion limited and thus describes the soil CH4 sink as a purely diffusive process (i.e. . However, CH4 is consumed by microbial activity in the soil and the simplified diffusion model may thus underestimate total uptake of CH4. Consequently, R99 extended the diffusion model by explicitly accounting for microbial oxidation of CH4 through a first-order rate expression. The resulting diffusion–reaction equation forms the basis of the R99 model, the C07 model and MeMo:
where is the CH4 diffusion coefficient and kd the first-order rate constant for microbial CH4 oxidation. Under steady-state conditions (i.e. ), soil CH4 uptake is controlled by the balance between diffusion of CH4 into soil and the rate of microbial CH4 oxidation. Hence, accurate characterization of and kd is essential for a robust quantification of CH4 uptake by soil.
2.2 Solution of the reaction–transport equation
The R99 model solved Eq. (2) semi-numerically by (i) assuming steady state, (ii) numerically approximating the diffusion term similar to the approach applied in the P96 model (Table 2, Eq. 11) and (iii) assigning CH4 oxidation exclusively to a distinct soil layer of thickness ϵ at depth zd=6 cm (Table 2, Eq. 12). However, CH4 consumption can occur throughout a soil profile, and thus Eq. (12) (Table 2) may either overestimate or underestimate the CH4 sink.
In the C07 model, Eq. (2) was solved analytically, providing a more accurate and mathematically robust estimate of CH4 uptake (Table 2, Eq. 13). Assuming steady-state conditions and constant and kd throughout the soil profile, integration of Eq. (2) provides a general solution for determining CH4 concentration at depth z in soil:
where A and B are integration constants that can be determined by setting upper and lower boundary conditions for the soil profile. The concentration of CH4 at the soil–atmosphere interface is defined by the atmospheric concentration of CH4 (, and thus a Dirichlet boundary (i.e. fixed concentration) is applied at the upper boundary. Conditions at the lower boundary are more challenging to ascribe because the soil depth at which atmospheric CH4 is completely consumed is not known a priori.
2.2.1 Negligible CH4 flux through the lower boundary (C07 solution)
The C07 model circumvents the problem by applying a homogenous Neumann (no-flux) condition at the lower model boundary: .
The application of this boundary condition allows derivation of the integration constants and B=0, which simplifies Eq. (3) to
The diffusive uptake of atmospheric CH4 at the soil–atmosphere interface can then be calculated using the derivative of Eq. (4) at z=0:
This formulation of soil uptake of CH4 is the simplest analytical solution to Eq. (2). It represents an improvement from the semi-numerical representation used in the R99 model and enables complete consumption of CH4 to be accounted for within the soil; however, the homogeneous Neumann boundary condition applied here is only an approximation, which is not generally valid. The simulation will not be influenced if the Neumann boundary is infinitely far from the consumption depth of CH4, and thus the corresponding Neumann boundary condition can be neglected. However, if this is not the case, it will result in simulation error.
2.2.2 Complete consumption of CH4 at an a priori unknown depth L (MeMo solution)
Therefore, we adopted an approach similar to the C07 model but one that is generally valid. We assume that methanotrophy consumes atmospheric CH4 in the soil until CH4 reaches a threshold (CH4(L)= CH4 min) that can be imposed based on biological limits (CH4 min = 100 ppb) or when CH4 is fully depleted (CH4 min = 0). The integration constants in Eq. (3) thus become
In addition to the concentration condition CH4 (L) = CH4 min, a flux condition also is imposed on the lower boundary in order to determine depth L: , where denotes a potential CH4 flux across the lower boundary that can be specified (i.e. CH4 (L) = CH4 min) or set equal to zero (i.e. CH4 (L) =0). The unknown depth L is then calculated by substituting the derivative of Eq. (3) into the expression for the lower boundary condition:
Rearranging Eq. (8) and finding its root allows for the determination of the initially unknown depth L where CH4(L) = CH4 min:
Once L is known, total CH4 uptake can be calculated from
where A and B are defined by Eqs. (6) and (7). When L tends to infinity, Eq. (10) is equivalent to the C07 model solution; however, Eq. (10) also allows for (i) complete consumption of CH4 within the soil interval, (ii) influx of CH4 from beneath the soil profile (e.g. from thawing permafrost or production of CH4 in oxygen-depleted microsites in soil) and (iii) a minimum CH4 concentration at which methanotrophy can occur in the soil column.
Figure 1 illustrates CH4 soil profiles and the penetration depth of CH4 into soil, L, for different kd values, and (diffusivity in free air) (Table 1). It is expected that L will vary spatially depending on local kd, and soil properties.
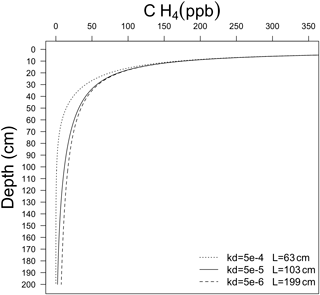
Figure 1Computational solution of Eq. (9) for different values of kd. Parameter L is defined as the depth where CH4 min = 0, assuming complete removal of CH4 in soil pore spaces.
MeMo is based on the more general solution (Eq. 10) and uses local methanotrophy rates (kd) and diffusion coefficients ( based upon soil conditions to determine CH4 penetration depths (L). Additionally, Eq. (9) allows one to set a minimum CH4 concentration if this parameter is known. Here, we assume a minimum of 0 or complete consumption. We assume no in situ production of CH4 or upward CH4 flux from below (i.e. ) because of a scarcity of field data for model validation. However, a flux from below can be employed in MeMo to enable a more comprehensive quantification of soil CH4 uptake that also potentially accounts for consumption of upward-migrating CH4 and autochthonous CH4 produced in oxygen-depleted microsites of finely textured soil.
2.3 Parameters
The rate of CH4 uptake by soil is controlled by the balance between gaseous diffusion of atmospheric CH4 into soil and the rate of CH4 oxidation by methanotrophic bacteria as described by Eqs. (14) and (20), respectively. Thus, and kd are key parameters, and accurate characterization of their values is essential for robust quantification of the soil CH4 sink.
2.3.1 Soil CH4 diffusivity,
Similar to the R99 and C07 models, in MeMo is determined from the diffusivity of CH4 in free air (; Table 1) adjusted for the influence of temperature (GT) and soil structure (Gsoil):
The gaseous diffusion coefficient of CH4 in soil increases linearly with temperature T (∘C) (Potter et al., 1996) according to the relationship
The soil structure factor (Gsoil) accounts for the effects of pore size, connectivity and tortuosity on gaseous diffusion and is determined according to the parameterization of Moldrup et al. (1996, 2013):
where Φ is total pore volume (cm3 cm−3), Φair is air-filled porosity (cm3 cm−3), and b is a scalar that accounts for soil structure. Total pore volume is defined as a function of bulk density ρ (g cm−3) and average particle density d (Table 1) (Brady et al., 1999):
The scalar b in Eq. (16) is calculated as a function of soil clay content (fclay; %) as proposed by Saxton et al. (1986):
Air-filled porosity (Φair) is determined from the difference between total pore volume and soil water content θ (%):
2.3.2 Rate constant for CH4 oxidation, kd
The CH4 oxidation rate (kd) is defined as the base oxidation rate constant (k0) for an uncultivated moist soil at 0 ∘C scaled by three factors to account for the influence of soil moisture (rSM), soil temperature (rT) and nitrogen content (rN):
The R99 and C07 models used a similar equation to estimate kd but without the rN parameter, opting instead to employ intensity of agricultural activity as a proxy to account for the inhibitory effects of N deposition on soil methanotrophy. Moreover, the C07 model excluded rN from the kd formulation and used a N deposition term to modify total CH4 uptake flux (Table 2, Eq. 13), which results in a larger N inhibition effect. The approach employed in MeMo is to use N deposition data directly to modify kd.
2.3.3 Base oxidation rate constant, k0
The base oxidation rate constant (k0) is a key parameter that exerts significant control on kd and thus the estimated CH4 uptake flux. For example, a 10-fold change in k0 (and thus kd) leads to a 3-fold decrease in the depth L at which CH4 is fully depleted from soil pores (Fig. 1) and a ∼ 3-fold increase in total uptake of CH4 (Fig. 2).
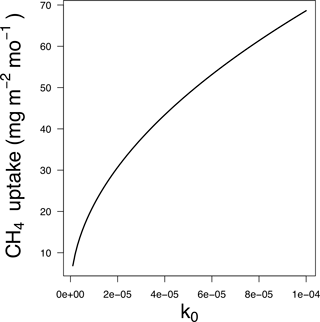
Figure 2Total CH4 uptake for different values of k0 (s−1), assuming a constant value of and no modification by soil temperature, moisture or nitrogen deposition.
Rate constants can be defined either on the basis of theoretical considerations or through site-specific field and laboratory observations. Rates of soil microbial processes, such as CH4 oxidation, are controlled by microbial biomass dynamics and community structure, and thus a complex array of environmental factors, including temperature, substrate (CH4) concentration, land use, moisture, pH and soil type (Ho et al., 2013). The influence of these environmental factors on microbial CH4 oxidation rates is not well characterized, and thus all factors are not explicitly represented in models. Consequently, apparent rate constants implicitly account for some environmental factors via fitting field observations or laboratory experiments, resulting in parameter values that may be more environment- and model-specific. A possible limitation of such an approach is reduced transferability and predictive capacity in other environments or from a regional to global scale. For example, Ridgwell et al. (1996) derived a single global estimate of s−1 by fitting Eq. (12) to 13 measured values of , and soil temperature from four different studies. In contrast, Curry (2007) estimated a global k0 of s−1 based upon fitting Eq. (13) to a 5-year time series of and soil temperature, moisture and CH4 flux measurements from a single site in Colorado (Mosier et al., 1996). The order of magnitude difference in k0 between the R99 and C07 models illustrates the potential model-specific nature of parameter values derived from experimental and observational data, as well as the limits and challenges for transferability. Soil methanotrophy is not unique in this regard, and parameterization of microbially mediated processes remains a common problem more generally in modelling approaches (e.g. Arndt et al., 2013; Bradley et al., 2016).
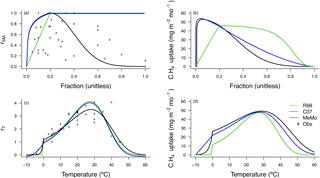
Figure 3CH4 uptake response factors (a, c) and uptake fluxes (b, d) as a function of soil moisture (rSM) and temperature (rT). Observations (shown as crosses) (rSM, File 1 in the Supplement, Table S1; rT, File 1 in the Supplement, Table S2), MeMo (black line), C07 (blue line) and R99 (green line).
Parameterization of k0 in MeMo has been refined using time-series data recently published by Luo et al. (2013), which consist of daily soil CH4 uptake rates and temperature and soil moisture data from three contrasting environments: temperate forest (Höglwald, Germany), tropical rainforest (Bellenden Ker, Australia) and steppe (Inner Mongolia, China). The data sets were used to explore potential variations in apparent k0 values in different environments, including comparison with k0 values from the R99 and C07 models; however, the uncertainty of this value could not be characterized due to a dearth of available observational data. Data from each site were interpolated according to Eq. (10) to derive an apparent k0 value for each biome. The k0 values for temperate forest and steppe are similar to the k0 value employed in the C07 model; however, the apparent k0 for tropical forest is approximately 3 times smaller than the C07 model k0 value. The three newly derived k0 values were employed in MeMo for their respective biomes and the k0 value from the C07 model ( s−1) was used for all other regions for which no biome-specific k0 values exist (Table 3). Similar k0 values of s−1 for temperate forest, steppe and short- grass steppe indicate that this magnitude of k0 is appropriate for many ecosystems. Yet, apart from the tropical wet forest, the data clearly indicate additional controls and the use of s−1 will thus prevent an overestimation of simulated fluxes. Nevertheless, further research is required to better characterize this key parameter.
2.3.4 Soil moisture factor, rSM
Both low and high soil moisture levels can negatively impact soil uptake of atmospheric CH4 (Schnell and King, 1996; von Fischer et al., 2009). Scarcity of soil water generally inhibits soil microbial activity while excessive moisture attenuates gas diffusion, limiting entry of atmospheric CH4 and O2 into soil (Burke et al., 1999; McLain et al., 2002; McLain and Ahmann, 2007; West et al., 1999).
The R99 and C07 models incorporated parameters to address the limiting effects of low soil moisture levels on CH4 uptake fluxes. The R99 model applied a soil moisture factor adopted from Potter et al. (1986) where rSM was calculated as a proportional ratio of precipitation (P) plus soil moisture (SM) divided by potential evapotranspiration (ET; Table 4, Eq. 21). It was assumed that rSM decreases linearly when (P + SM) ∕ ET is less than 1. The C07 model modified the response of soil methanotrophy to moisture using an empirical water stress parameterization and soil water potential based on findings from Clapp and Hornberger (1978) (Table 4, Eq. 22). A consequence of that approach is that rSM decreases logarithmically to zero at an absolute soil water potential of w<0.2 MPa (Fig. 3).
In MeMo, soil moisture (%) is used to calculate rSM and a formulation similar to the C07 model is used for low soil moisture values. A threshold of < 20 % soil moisture is applied because that value corresponds to optimum conditions for CH4 oxidation in soil (Castro et al., 1995; Whalen and Reeburgh, 1996) and because inclusion of a water stress parameter better captures CH4 uptake flux in dry ecosystems (Fig. 3; Curry, 2007).
Establishing parameters to quantify the impact of excess moisture on soil methanotrophy has proven more challenging. The C07 model relied upon soil pore space characteristics in factor Gsoil (Eq. 16) to account for decreased gas diffusion and limitation of kd at high soil moisture content. However, attenuation of gas diffusion is only one impact of high soil water content and it is necessary also to account for the inhibitory effects of excessive moisture on kd (Boeckx and Van Cleemput, 1996; van den Pol-van Dasselaar et al., 1998; Visvanathan et al., 1999). Soil moisture content > 20 % reduces CH4 uptake due to a restricted diffusion of CH4 and supply of O2. The R99 and C07 models assume that microbial CH4 oxidation remains active at a soil moisture content of 80 %, an assumption that contradicts field investigations, which show that CH4 uptake decreases rapidly at soil moisture levels > 50 % (van den Pol-van Dasselaar et al., 1998). Thus, the soil moisture factor employed in MeMo also accounts for limitation of microbial CH4 oxidation at a soil moisture content > 20 % after which rates of CH4 uptake begin to decrease (Adamsen and King, 1993; Visvanathan et al., 1999). The rSM factor used in MeMo was determined by fitting a Gaussian function to laboratory experimental data (Table 4, Eq. 23; Fig. 3a), following the approach of Del Grosso et al. (2000). The mean rSM and standard deviation determined using this approach were 0.2 ± 0.2.
A soil moisture factor (rSM) was calculated for each set of observational data from independent field sites (File 1 in the Supplement, Table S1) based upon an optimum rate of CH4 uptake occurring at a soil moisture content of 20 % (rSM=1). The remaining rSM values were computed as a linear ratio of the CH4 uptake rate at 20 % water content. Figure 3b illustrates the pattern of response in methanotrophy rates to changes in soil moisture content in the R99, C07 models and MeMo, and the net effect on CH4 uptake fluxes across a range of absolute soil moisture levels used to force parameter rSM. The CH4 uptake fluxes were calculated by varying soil moisture content while holding constant all other environmental parameters (temperature, and Ndep). The R99 and C07 models both predict greater CH4 uptake fluxes than MeMo at soil moisture contents > 20 % with the R99 model yielding the highest flux rates; however, the C07 model and MeMo yield similar CH4 uptake rates for much of the soil moisture range. Reduction of CH4 uptake flux at high soil moisture levels due to attenuation of gas diffusion cannot be managed solely through the term Gsoil (i.e. reduction in free pore space). MeMo also accounts for inhibition of microbial CH4 oxidation rates at elevated soil moisture content, predicting lower CH4 uptake flux as a result of more realistic rSM values determined from the Gaussian response observed in field data from three different global biomes (Luo et al., 2013).
2.3.5 Temperature factor, rT
Temperature exerts an important influence on rates of microbial processes, and consequently, all models parameterize for the effects of temperature on soil methanotrophy. The R99 model employs a Q10 function derived from experimental data with a Q10 factor of 2 change over the temperature interval 0 to 15 ∘C. The model assumes that bacterial methanotrophy ceases at temperatures < 0 ∘C (Table 5, Eq. 24). The C07 model adopts the same Q10 factor as R99 for temperatures > 0 ∘C but employs a different response below 0 ∘C. Soil water generally does not freeze at a surface temperature of 0 ∘C, and observations from cold regions provide ample evidence for the presence of methanotrophic activity at temperatures < 0 ∘C (Vecherskaya et al., 2013). The C07 model allows for a parabolic decrease of methanotrophy rates from 0 to −10 ∘C (Table 5, Eq. 25) based upon observations of CH4 uptake in soil at subzero temperatures (Del Grosso et al., 2000).
Parameterization of a temperature factor (rT) is revisited in MeMo based upon availability of new experimental data for soil from different biomes (File 1 in the Supplement, Table S2). A Q10 factor having a value of 1.95 was determined for the temperature interval 0 to 15 ∘C by curve fitting and minimizing linear errors (r2=0.75, ; Table 5, Eq. 26). The factor rT was determined by using the observed CH4 uptake flux at 10 ∘C at each site as the base of the Q10 function (Fig. 3c). An exponential decrease in CH4 uptake flux was assigned to the temperature range 0 to −5 ∘C as recommend by Castro et al. (1995) and Del Grosso et al. (2000). Moreover, the amount of frozen soil increases exponentially with decreasing temperatures (Low et al., 1968), and consequently, CH4 uptake also should decline exponentially.
The pattern of change in the rT factor and CH4 uptake flux for the temperature range −10 to 60 ∘C is shown in Fig. 3d. The CH4 uptake fluxes shown were calculated by varying temperature while holding other environmental factors constant (i.e. soil moisture, N deposition or agricultural land use, and . All models exhibit an optimum in CH4 uptake at 25 ∘C characterized by a maximum rT and CH4 oxidation rate. The key differences between models are the behaviour of rT at temperatures below 0 ∘C and the amplitude of response curves. The R99 model assumes that methanotrophic activity ceases at 0 ∘C, and consequently, CH4 uptake rates decrease sharply at that temperature. In contrast, the C07 and MeMo models both allow for methanotrophy at temperatures < 0 ∘C. In general, the exponential decrease of rT employed in MeMo more closely resembles natural patterns of soil methanotrophy at subzero temperatures than the parabolic decline employed in the C07 model consistent with observations reported by Castro et al. (1999) and Del Grosso et al. (2000). Although our parameterization yields a fit similar to C07 to the limited observations available at temperatures < 0 ∘C, the rT used in MeMo provides a simpler solution because it does not require multiple conditions to be met. In contrast, the C07 parameterization increases parabolically at temperatures < −10 ∘C, which requires an additional condition to be incorporated into the model to prevent increased rates of CH4 uptake at very low temperatures. Soil CH4 uptake fluxes predicted by the C07 model are greater than those calculated using MeMo because of the different parameterization at temperatures < 0 ∘C. Finally, the amplitude of the temperature response curve is greater and similar in the C07 and MeMo models compared to the R99 model, in particular, at temperatures > 25 ∘C as a result of differences in the formulation and solution for CH4 uptake flux (Fig. 3d).
2.3.6 Nitrogen deposition factor, rN
The effect of nitrogen (N) deposition on CH4 uptake is not as well constrained as the effects of temperature and soil moisture. In general, field observations have shown that CH4 consumption rates, and thus uptake fluxes, decrease with N additions (Aronson and Helliker, 2010; Butterbach-Bahl and Papen, 2002; Steinkamp et al., 2001). Different processes have been suggested to explain this negative effect. Firstly, methanotrophs and ammonia oxidizers are capable of switching substrates (although the latter microorganisms typically consume N compounds preferentially if available), and therefore the presence of N compounds reduces CH4 consumption (Bradford et al., 2001; Gulledge and Schimel, 1998; Phillips et al., 2001; Wang and Ineson, 2003; Whalen, 2000). In addition, intermediate and end products from methanotrophic ammonia oxidation (i.e. hydroxylamine and nitrite) can be toxic to methanotrophic bacteria (Bronson and Mosier, 1994; MacDonald et al., 1996; Sitaula et al., 2000). Finally, large amounts of mineral fertilizers (i.e. ammonium salts) can induce osmotic stress in methanotrophs inhibiting CH4 consumption (Whalen, 2000). However, other studies suggest a positive effect of N fertilization on CH4 oxidation rates. One of the mechanisms invoked to explain the positive effect is a stimulation of nitrifying bacteria to consume CH4 by increased inputs of N due to an improvement in living conditions (Cai and Mosier, 2000; De Visscher and Cleemput, 2003; Rigler and Zechmeister-Boltenstern, 1999). The positive effect of N addition on CH4 oxidation rates has been observed primarily under experimental conditions and also greatly depends on the local microbial community structure. Therefore, we assumed that N has an inhibitory effect on uptake of atmospheric CH4 in all scenarios.
The C07 and R99 models both account for the negative effect of N inputs on CH4 uptake fluxes via the factor rN. In the R99 model, rN directly affects kd, while in the C07 model, rN directly modifies the uptake flux. Both models parameterize the negative effect of N inputs on CH4 oxidation rates as a function of agricultural intensity (as a fraction of area) as a proxy for fertilizer application (Table 6, Eq. 27). However, the mathematical description of rN used by the R99 and C07 models does not account for the enhanced N deposition by anthropogenic activity or direct N input via fertilizers because its global distribution was not well known at the time of model development. Here, we suggest a mathematical description of rN that accounts for all anthropogenic N input sources: fossil fuel combustion, biomass burning and fertilizer application (Lamarque, 2013; Nishina et al., 2017).
The computation of rN in MeMo is a function of (i) the inhibitory effect on CH4 uptake and (ii) the distribution and amount of N input in soil (Zhuang et al., 2013). We estimated the percent reduction of CH4 uptake per mole of N added based on field and laboratory observations (File 1 in the Supplement, Table S3). We determined an average inhibition α of 0.33 % mol N−1 based on the mean uptake reduction per mole of N added. The N response function rN was governed by Eq. (29):
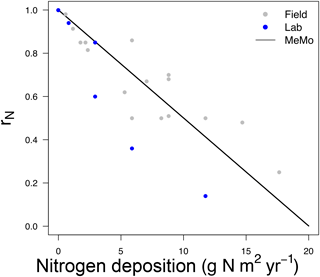
Figure 4CH4 uptake response as a function of nitrogen deposition and fertilizer application factor rN. The linear fit (black line) is based on observations from field (long-term) and laboratory measurements (gray and blue dots; File 1 in the Supplement, Table S3).
In the cases where entry of N into soil is limited by bulk density ρ, 90 % of N compounds tend to remain at depths cm before exponentially decreasing in concentration with depth (Schnell and King, 1994). Thus, Nsoil was calculated as N input (kg N ha−1 yr−1) divided by ρ at z=5 cm:
Figure 4 shows the change in rN in relation to N input rate and the form of Eq. (29).
MeMo was implemented in R (version 3.0.1) and simulations were carried out with a spatial resolution of 1∘ × 1∘ and a monthly temporal resolution for the period between 1990 and 2009. The model code, a simple model case study for the year 2000 and output for 1990–2009 are available as the Supplement to this paper. To enable model–model comparisons and assess the combined effect of all refinements introduced in MeMo on the global CH4 uptake flux estimate, the R99 and C07 models also were implemented in R at identical spatial and temporal resolutions and forced using the same driving data.
3.1 Forcing data
MeMo and the C07 and R99 models were forced using global monthly observations of soil moisture, temperature, atmospheric CH4 concentration, N deposition, soil bulk density and clay content for the period 1990–2009. Information about data sources and maps of the forcing data are provided in File 3 in the Supplement.
Satellite observations of soil moisture at a spatial resolution of 1 × 1∘ and a monthly temporal resolution are available for the period 1990–2009 from Dorigo et al. (2011); however, the data set contains gaps in some regions (e.g. in areas of high-density vegetation). The use of MeMo as a predictive tool to estimate the past and future global CH4 soil sink relies strongly on the use of soil moisture from standard climate models, such as output from land surface models or dynamic global vegetation models (DGVMs). Therefore, gaps in the Dorigo et al. (2011) data set were filled using soil moisture data from an ensemble of nine DGVMs (TRENDY; Sitch et al., 2015). The R99 model parameterizes the effect of soil moisture on CH4 uptake fluxes as a function of precipitation and evaporation, and therefore R99 was forced using monthly data sets of precipitation (CRU3.1; Harris et al., 2014) and evapotranspiration (TRENDY; Sitch et al., 2015). Temperature forcing is constrained by global data sets for surface temperature as a proxy for soil temperature (CRU3.1; Harris et al., 2014). Monthly mean global atmospheric CH4 concentrations multiplied by the latitudinal atmospheric CH4 gradient were calculated from Rigby et al. (2008). The N deposition data were obtained from an atmospheric chemical transport model embedded in an Earth system model (Lamarque et al., 2013), and the N input via fertilizers was obtained from Nishina et al. (2017). Because the R99 and C07 models express the influence of N on CH4 uptake fluxes as a function of fraction agricultural area (see Sect. 2.3.6), R99 and C07 were forced using annual global gridded land-use change data from Hurtt et al. (2011). Finally, global gridded observations for bulk density and clay content were taken from Shangguan et al. (2014).
Areas that had less than 0.5 % average annual soil moisture content were masked (e.g. Sahara) because it was assumed CH4 uptake is negligible under such conditions. If the areas were left unmasked, then MeMo would overestimate CH4 uptake across the regions due to high temporal variability in the driving data (e.g. a month with no moisture followed by a month with > 20 %). Irregular short-lived precipitation events in deserts led to unreliable estimates of soil uptake of atmospheric CH4 because such areas are unlikely to host well-established communities of methanotrophic bacteria capable of responding rapidly to short-term increases in soil moisture.
The following sections critically evaluate MeMo estimates of the global CH4 sink (Sect. 4.1) as well as the regional distribution of CH4 uptake and its main drivers (Sect. 4.2) in the context of available field data and published model predictions.
4.1 Global CH4 uptake by soils
MeMo predicts an average annual global flux of 33.5 ± 0.6 Tg CH4 yr−1 for the period 1990 to 2009. Uncertainty in this flux was calculated as the standard deviation of annual global CH4 uptake. The estimated global uptake compares well with estimates from terrestrial ecosystem models, DGVMs and global atmospheric inversions (Table 7). Zhuang et al. (2013) determined a similar average global uptake flux of 34 ± 2 Tg CH4 yr−1 during the 21st century using a process-based model included in the TEM while Spahni et al. (2011) estimated an uptake flux of 38.9 Tg CH4 yr−1 using the LPJ-WHyMe DGVM. Hein et al. (1997) predicted a similar flux through atmospheric inversions but with a greater level of uncertainty (30 ± 15 Tg CH4 yr−1). Upscaling of field measurements of soil methanotrophy rates from 120 different studies spanning a wide range of ecosystems yielded an uptake flux of 36 ± 23 Tg CH4 yr−1 (Dutaur and Verchot, 2007). The large uncertainty associated with the mean flux results from differences in data representation for ecosystems and a tendency for sampling to be conducted seasonally rather than annually. In contrast, flux estimates based upon extrapolation of long-term records of CH4 uptake in a smaller number of soil types resulted in an estimated flux of 28.7 Tg CH4 yr−1 (Dörr et al., 1993). Similarly, global extrapolation of measurements made solely on northern European soils yielded a sink strength of 29 Tg CH4 yr−1 (Smith et al., 2000).
The average annual soil sink for atmospheric CH4 estimated by MeMo (33.5 ± 0.6 Tg CH4 yr−1) is greater than global uptake predicted using the P96 and C07 models (20 ± 3 and 29.3 ± 0.6 Tg CH4 yr−1, respectively). The R99 model predicts a global sink of 38.1 ± 1.1 Tg CH4 yr−1, which compares more favourably with the MeMo estimate. The observed differences in mean global soil uptake of atmospheric CH4 estimated using the R99, C07 and MeMo models forced with identical data are attributed primarily to three factors: (i) their respective mathematical solutions of reaction–transport equations (Sect. 2.2), (ii) differences in parameterization of k0 (Sect. 2.3.3) and (iii) differences in formulation of rN (Sect. 2.3.6). The R99 model predicts soil uptake that is 12 and 24 % greater, respectively, than fluxes estimated using MeMo and the C07 model. These differences are due to the R99 model applying a k0 that is 1 order of magnitude greater than k0 values used in the C07 model and MeMo. The amplifying effect of the large k0 is partially offset by the semi-numerical approximation (Eq. 12) employed in the R99 model, which results in the final global CH4 uptake flux being of similar magnitude to the MeMo and the C07 model estimates. Finally, the low uptake predicted by the C07 model is a consequence of the parameterization of the nitrogen inhibition effect (rN) and its direct modification of the CH4 flux rather than the CH4 oxidation activity (kd) (Sect. 2.3.3). Nitrogen inhibition was responsible for a global reduction in CH4 uptake of 1.4 Tg yr−1 in MeMo compared to 7.3 and 2.3 Tg yr−1 in the C07 and R99 models, respectively.
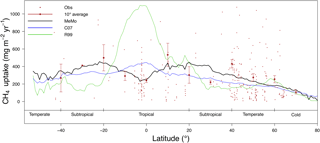
Figure 5Latitudinal distribution of the soil uptake predicted by the R99 (green line), C07 (blue line) and MeMo (black line) models. Measurements of CH4 uptake (small brown dots; Dutaur and Verchot, 2007) and a 10∘ running mean of direct observations (large brown dots for average with bars representing 1 standard deviation error).
4.2 Regional CH4 uptake by soils
The latitudinal distribution of soil uptake rates of atmospheric CH4 predicted using the R99 and C07 models, and MeMo is shown in Fig. 5 accompanied by direct measurements of CH4 oxidation rates from Dutaur and Verchot (2007) and a 10∘ running average. We chose to validate MeMo and previous models against regionally averaged observations to conduct the comparison at scales resolved by global models such as MeMo. This model is not intended to represent fine-scale site-specific attributes of soil but rather broad regional soil characteristics and CH4 uptake fluxes.
The latitudinal distribution of observations reveals a scarcity of direct measurements of soil methanotrophy from sites in the Southern Hemisphere. Additionally, the frequency of measurements generally is low and rarely encompasses a full 12-month period, which creates challenges for verifying model estimates of annual CH4 uptake fluxes. Observations at specific latitudes typically exhibit a wide range of values, which are reflected in the large standard error bars calculated for the 10∘ running means (Fig. 5). Nevertheless, the averages of direct observations calculated for each 10∘ latitude interval show a distinct bimodal pattern with the lowest soil CH4 uptake fluxes in the tropics and at high latitudes. Maximum rates of CH4 uptake occur between 10 to 20∘ latitude in both hemispheres (Fig. 5). MeMo simulates a similar bimodal latitudinal distribution of CH4 uptake fluxes with an RMSE that is 16.8 mg CH4 m−2 yr−1 lower than other models when fitted to 10∘ latitudinal averages of observational data. In contrast, the C07 and R99 models both predict a latitudinal distribution of soil methanotrophy that has CH4 uptake maxima in equatorial regions and lower rates of CH4 oxidation at midlatitudes (∼ 40∘ N and 20 to 40∘ S), resulting in higher RMSEs of 28.6 and 72.1 mg CH4 m−2 yr−1, respectively, when fitted to the 10∘ latitude-averaged data. The R99 model significantly overestimates CH4 uptake fluxes in the tropics (20∘ N to 20∘ S) and underestimates CH4 oxidation in the subtropics (20 to 40∘ N and S), resulting in large differences for these regions relative to the MeMo simulations (Fig. 6e). The C07 model predicts a latitudinal pattern of simulated CH4 fluxes that is similar to R99, however, with much lower uptake fluxes in the tropics and no pronounced minima in the subtropics. Consequently, the RMSE of the fit to observational data is much lower and regional differences relative to MeMo generally are smaller, ranging from 30 % in the tropics to 20 % in the subtropics (Fig. 6d).
The regional differences between MeMo and the R99 and C07 models result from differences in the parameterization of factors that govern CH4 oxidation rates in the models: k0, rSM, rT and rN. The lower k0 assigned to tropical wet forest (see Sect. 2.3.3) accounts for the reduction in CH4 uptake by tropical soil in MeMo. The strong agreement between MeMo simulation results and CH4 uptake measurements presented in Fig. 5 suggests that the empirically derived lower k0 value more accurately reflects soil CH4 oxidation rates in the tropics. However, we note the possibility that additional factors, or unexpected combinations of current factors, may influence rates of atmospheric CH4 uptake in the tropics in ways that are not explicitly represented in the models.
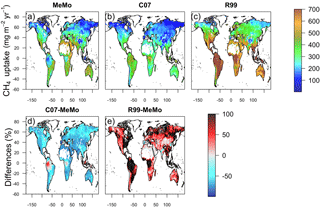
Figure 6Annual mean CH4 uptake by soil predicted using models (a) MeMo, (b) C07 and (c) R99 for the period 1990–2009. Differences between models expressed in percent are shown in (d) C07 minus MeMo and (e) R99 minus MeMo.
The influence of different environmental factors on soil CH4 uptake was assessed by calculating the global CH4 uptake flux while varying each factor (temperature, soil moisture and nitrogen input) independently and keeping other factors constant (Figs. 7–9). Comparison of rSM values reveals large differences across models in tropical wet regions (Fig. 7), which explains the contrasting predictions of CH4 uptake by MeMo (213 mg CH4 m−2 yr−1) versus the R99 (689 mg CH4 m−2 yr−1) and C07 (329 mg CH4 m−2 yr−1) models. Formulation of rSM in MeMo (Sect. 2.3.4) accounts for limitation of methanotrophic oxidation rates when soil moisture levels are at > 20 % water content, a feature that is absent in the R99 and C07 models. In addition, the R99 model implements a linear decrease of rSM for soil moisture conditions < 20 %, which results in a 60 to 80 % reduction in CH4 oxidation rates in the subtopics. The absence of this condition in models MeMo and C07 explains the significant differences in CH4 uptake fluxes in subtropical regions (Figs. 5 and 6).
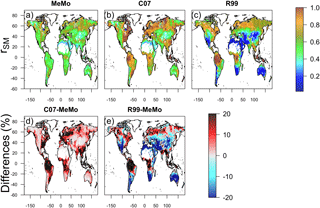
Figure 7Soil moisture response (rSM) of CH4 oxidation simulated by models (a) MeMo, (b) C07 and (c) R99. Differences in model response expressed in percent are shown in (d) C07 minus MeMo and (e) R99 minus MeMo.
Formulations of rT are similar in the three models (Sect. 2.3.5), and consequently, gridded maps of simulated rT values exhibit broadly similar global patterns in which high rT values are present at warm low latitudes and low rT values are predicted at cold high latitudes. Notably, MeMo generally simulates rT values that are approximately 20 % lower than those predicted by the C07 and R99 models (Fig. 8) because of the revised formulation of the Q10 value. MeMo and the C07 model simulate higher rT values than R99 at high latitudes because of differences in parameterization of rT at temperatures near 0 ∘C.
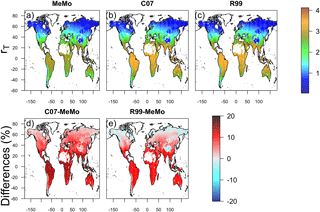
Figure 8Temperature response (rT) of soil methanotrophy simulated by models (a) MeMo, (b) C07 and (c) R99. Differences in model response expressed in percent are shown in (d) C07 minus MeMo and (e) R99 minus MeMo.
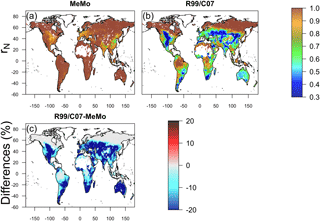
Figure 9Response of soil methanotrophy to nitrogen effect (rN) simulated by models (a) MeMo, (b) R99 and C07. The responses for the R99 and C07 models are both shown in panel (b) because they have the same formulation. The difference in model response between the R99/C07 models minus MeMo expressed in percent is shown in panel (c).
Inhibition of soil methanotrophy due to N (rN) differs significantly between the three models. Nitrogen inhibition of CH4 oxidation rates is lower in MeMo compared to the R99 and C07 models, in particular, at midlatitudes (Fig. 9). The R99 and C07 models formulate rN as a function of agricultural intensity in contrast to MeMo, which uses modelled N deposition and N input via fertilizers. The difference in approach results in an rN factor that is up to 20 % higher in MeMo across most regions with the exception of high latitude areas (Fig. 9).
In regions of intense agricultural activity and high N deposition (∼ 150 kg N ha−1), such as Europe, the midwestern US, China and India, MeMo predicts a reduction in CH4 uptake rates of up to 60 % on average, which is consistent with R99 and C07 models. However, inhibition of methanotrophy simulated by MeMo in areas experiencing low rates of N deposition is much smaller than R99 or C07. The key limitation of the N effect approach adopted in the R99 and C07 models is the generalization of N inhibitory effects across different agricultural areas, crops and types of land management, which results in a homogeneous and excessive attenuation of CH4 oxidation rates. In contrast, the MeMo rN parameterization employs a more conservative rN factor and a realistic regional distribution, which is based upon observational data that are consistent with recent studies reporting that high rates of N deposition (10 kg N ha−1 yr−1) can reduce soil uptake of atmospheric CH4 by ∼ 8.6 % (Fang et al., 2014; Zhang et al., 2008). Direct application of fertilizers at more extreme rates (> 300 kg N ha−1 yr−1) can entirely eliminate uptake of atmospheric CH4 by agricultural soil (Veldkamp et al., 2001). Nevertheless, the importance of accurate characterization of the attenuating effects of N addition on soil methanotrophy highlights the need for additional efforts to verify and refine parameterization of this key factor.
4.3 Temporal and spatial variability of soil CH4 uptake
Field observations of soil uptake of atmospheric CH4 are generally sparse both spatially and temporally. Consequently, our quantitative understanding of CH4 uptake fluxes across different ecosystems and seasons is limited. Models provide a means to quantitatively explore spatial and temporal patterns of soil methanotrophy on scales that cannot be readily captured by field-based observations. Therefore, once tested and validated (see Sect. 4.2), MeMo was used to quantitatively assess the variability of soil CH4 uptake in different climate zones and ecosystems on seasonal timescales.
4.3.1 Regional variability
The relative contribution of soil in each climatic zone to global uptake of atmospheric CH4 as predicted by MeMo is summarized in Table 8. Soil in the Northern Hemisphere is estimated to account for approximately two-thirds (65 %) of the total global sink for atmospheric CH4 because of the uneven distribution of landmasses between the Northern and Southern hemispheres. Notably, terrestrial areas in the northern subtropical and temperate zones collectively account for ∼ 45 % of the global soil sink for atmospheric CH4. The southern tropical zone contributes a further ∼ 19 % to soil uptake of CH4. The southern subtropical and northern tropical zones are estimated to contribute almost equally (∼ 14 %) to total CH4 uptake (Table 8). The smallest proportion of soil CH4 oxidation occurs in the southern temperate (0.6 %) and northern polar (5 %) zones due to a combination of small land area and low rates of CH4 uptake. Model predictions of CH4 uptake by climatic zone provide insights into the relative importance of each region in the global CH4 cycle but additionally begin to facilitate analysis of potential responses of the soil CH4 sink within each zone to global change both due to climate and land management.
Table 9MeMo CH4 uptake estimates by ecosystem type from Ramankutty and Foley (1999) land cover classification.
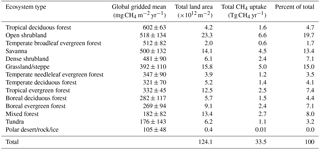
Further analysis of soil CH4 uptake by ecosystem types (Table 9) shows that the highest gridded mean rates of CH4 oxidation are associated with tropical deciduous forests (602 mg CH4 m−2 yr−1). The relatively low soil moisture content during the dry season (File 2 in the Supplement, Fig. S3) and the consistently high mean annual temperature (File 2 in the Supplement, Fig. S7) in such ecosystems promote high rates of soil methanotrophy. Furthermore, the soil typically possesses a low clay content (File 2 in the Supplement, Fig. S2), which results in higher porosity that enhances gas diffusion and promotes higher rates of CH4 oxidation. In comparison, rates of CH4 uptake by soil in open and dense shrubland, temperate evergreen forest and savanna ecosystems (Table 9) are ∼ 100 mg CH4 m−2 yr−1 lower but still highly significant globally.
Dense and open shrubland are characterized by constant climatic conditions (temperate and relatively low soil moisture; File 2 in the Supplement: Figs. S7 and S3, respectively) throughout the year, which, in combination with a soil texture that typically is sandy, results in high annual CH4 uptake rates (Tate et al., 2007). In contrast, high annual rates of CH4 uptake in temperate evergreen forests result from elevated rates of soil methanotrophy during summer months (Sect. 2.3.4), indicating that temperature is a key driver of CH4 oxidation in such ecosystems (Borken et al., 2006; Ueyama et al., 2015; Wang and Ineson, 2003). Savannas share many climatic conditions with tropical deciduous forests but also commonly experience wildfire during the dry season. Both ecosystem types though are characterized by a marked seasonality driven by the presence or absence of precipitation in combination with a consistent high mean annual temperature (File 2 in the Supplement, Figs. S7 and S3), which collectively support high rates of CH4 uptake by soil.
Tundra, taiga, polar desert and other ecosystem types that are common at high latitudes (File 2 in the Supplement, Fig. S10) are characterized by the lowest mean annual rates of soil methanotrophy (< 180 mg CH4 m−2 yr−1) because of low temperatures throughout most of the year. MeMo also predicts low rates of CH4 uptake in tropical humid forest (332 mg CH4 m−2 yr−1) due to low rates of bacterial CH4 oxidation and the negative impact of high soil moisture levels on gas diffusion (see Sect. 2.3.5). The CH4 uptake rates estimated by MeMo are consistent with field observations by Dasselar et al. (1998) and Luo et al. (2013), which indicate that excess soil moisture strongly attenuates CH4 uptake rates across a range of ecosystem types.
Finally, the global significance of each ecosystem type as a CH4 sink depends strongly on spatial extent as well as CH4 oxidation rates. Open shrubland (19.7 %), grassland and steppe (15.0 %), and savanna (13.4 %) are the most important ecosystem types contributing to the global CH4 soil sink (∼ 48 % collectively; Table 9) in MeMo because of high mean rates of CH4 uptake (392 to 518 mg CH4 m−2 yr−1) in combination with a large areal extent globally (14×1012 to 23×1012 m2). This finding is similar to the estimate reported by Potter et al. (1996) that warm and relatively dry ecosystems, such as semi-arid steppe, tropical savanna, tropical seasonal forest and chaparral, account for 40 % of soil uptake of atmospheric CH4 globally. Moreover, Luo et al. (2013) reported the highest annual CH4 uptake rates in dry savanna as part of a long-term field investigation of soil methanotrophy in several ecosystem types. Singh et al. (1997) also observed CH4 uptake rates that were higher in savanna than in temperate forest. Although both model simulations and available field observations suggest these ecosystems are important global sinks for atmospheric CH4, there is presently a dearth of field measurements for warm and dry environments relative to temperate ecosystems.
4.3.2 Seasonal variability
Global annual uptake of atmospheric CH4 by soil exhibits a marked seasonality that reflects the dominance of the Northern Hemisphere in the soil sink. The highest simulated CH4 uptake fluxes occur during June, July and August (JJA) (10.3 Tg CH4) followed by September, October and November (SON) (10.1 Tg CH4), March, April and May (MAM) (6.8 Tg CH4), and finally, December, January and February (DJF) (6.3 Tg CH4) (Fig. 10).
Methane uptake in the cold and temperate regions of the Northern Hemisphere generally is characterized by the largest seasonality, exhibiting an amplitude of 30 mg CH4 m−2 month−1. In these regions, modelled uptake of CH4 by soil is controlled strongly by temperature, and consequently, ecosystems common at these latitudes (e.g. boreal, needle leaf, temperate deciduous, mixed forest, polar deserts/rock/ice and tundra) show pronounced seasonal trends (Fig. 11), which also are evident in field measurements (e.g. Priemé and Christensen, 1997) and emphasized in local mechanistic models (e.g. Oh et al., 2016). These finding suggest that the soil CH4 sink in such ecosystems may be more sensitive to future change as a result of global warming.
In contrast, soil methanotrophy in temperate regions in the Southern Hemisphere is characterized by a weaker seasonality having an amplitude of 17 mg CH4 m−2 month−1 due to the prevalence of grassland and steppe, which contrasts with a dominance of forest in the Northern Hemisphere. Seasonality of soil CH4 uptake fluxes is even more muted in tropical and subtropical environments (< 10 mg CH4 m−2 month−1) because of favourable and stable environmental conditions. Tropical deciduous forest and tropical evergreen forest, which are common in these climate zones, are characterized by relatively constant CH4 uptake fluxes throughout the year (Fig. 11); however, MeMo predicts greater seasonality (20 mg CH4 m−2 month−1) of CH4 uptake by soil in drier subtropical ecosystems, such as open shrubland, savanna and grasslands (Fig. 11) because of seasonality in soil moisture.
Notably, northern temperate forest in summer (JJA) was the ecosystem and time period possessing the highest average monthly CH4 uptake fluxes (76.7 mg CH4 m−2 month−1) simulated by MeMo. During the rest of the year, the largest soil sink for atmospheric CH4 occurred in the Southern Hemisphere in the tropical deciduous forest of central Africa (DJF, 69.5 mg CH4 m−2 month−1; MAM, 73.5 mg CH4 m−2 month−1; SON, 75.5 mg CH4 m−2 month−1). This finding is significant because field observations of soil methanotrophy in northern temperate forest during summer are the measurements most commonly extrapolated to an annual basis, which may lead to a possible overestimation of global CH4 uptake fluxes.
4.4 Model limitations and scope of applicability
Several aspects of MeMo can be developed further, pending availability of new field data to improve estimation of global soil uptake of atmospheric CH4. Firstly, the base oxidation rate of bacterial methanotrophy at 0 ∘C (k0) is a critical parameter necessary for accurate estimation of CH4 uptake rates. There is presently a general dearth of published k0 values for soil methanotrophy, and moreover, ecosystem coverage is incomplete. Additionally, our parameterization for kd accounts for methanotrophic activity in a one-dimensional soil matrix; however, other studies have separated CH4 uptake in soil from methanotrophy in the rhizosphere to improve estimates of total CH4 uptake (e.g. Sabrekov et al., 2016). This refinement has been modelled for local conditions but insufficient data about rhizosphere CH4 oxidation rates prevent inclusion in MeMo and extension to a global scale. Secondly, the Q10 response of soil methanotrophy has been determined to date in only a small subset of ecosystems in which soils function as a sink for atmospheric CH4. The majority of Q10 values have been determined for bacterial oxidation of CH4 under laboratory conditions and there is considerable variability in values across different ecosystems. Thirdly, additional field observations of CH4 uptake by soil are needed, in particular, long-term measurements at individual sites that capture seasonality and interannual variability and from regions that presently have minimal or no representation (i.e. the Southern Hemisphere, semi-arid ecosystems) in the current pool of observations. Fourthly, additional observations and characterization of the effects of N deposition on soil methanotrophy are needed. The measurements ideally should be conducted in situ using N input rates that are appropriate for different environments and land-use practices. MeMo can be used to guide new field and laboratory experiments to address the lack of parameterization data, in particular, k0 and Q10 values for soil methanotrophy in different ecosystem and latitudes, and long-term in situ studies of N inhibition on CH4 uptake by soil. It also can be used to compare results from short- and long-term investigations of CH4 uptake in field and laboratory experiments.
MeMo is also parameterized to accommodate input of CH4 from below (i.e. subsurface methanogenesis or upward migration of deeply sourced CH4); however, rigorous validation of that aspect of the model will require additional field observations, including better characterization of conditions under which CH4 is produced in finely textured soils and deep sub-horizons. The presence, or periodic input, of high concentrations of CH4 (e.g. from permafrost melting) may impact competition for oxygen and niche space between low-affinity CH4-oxidizing bacteria and the high-affinity methanotrophs responsible for uptake of atmospheric CH4. Refinement and validation of the capacity for MeMo to account for upward-migrating or autochthonous CH4 will enable the model to be used to estimate CH4 flux from intermittently wet environments, which may currently fall outside the scope of process-based wetland models.
The process-based nature of MeMo and the breadth of conditions for which it has been validated provide scope for using the model to quantify CH4 uptake in soil in a broad range of scenarios. For example, MeMo could be used to determine global uptake of CH4 by soil in the past during glacial or former interglacial periods. It may also be used to assess potential uptake rates of atmospheric CH4 in future climate scenarios and further elevated tropospheric CH4 mixing ratios. Additionally, MeMo can be used to evaluate the impact of different proposed policies and mitigation strategies for managing the atmospheric burden and growth rate of CH4 because of its capacity to evaluate different future scenarios based upon parameterization of key drivers that impact rates of CH4 uptake by soil globally.
We developed a processed-based model to simulate uptake of atmospheric CH4 by soil, which was refined using newly reported experimental data and the introduction of recent insights into physical and biological mechanisms that drive soil methanotrophy. We modified the general analytical solution proposed by Ridgwell et al. (1999) and Curry (2007) to account for a maximum depth of CH4 uptake and to quantify upward migration and consumption of CH4 produced in situ. Representation of the effects of N deposition and input via fertilizers, soil moisture and temperature on methanotrophy were improved based upon newly available data and recent advances in characterization of these processes. Finally, we proposed utilization of a different base oxidation rate k0 for methanotrophy in different regions because its value changes in relation to environmental conditions.
MeMo simulations produced a closer fit to observational data than two previous soil methanotrophy models (Ridgwell et al., 1999; Curry, 2007). MeMo and observational data show a similar bimodal latitudinal distribution of atmospheric CH4 uptake by soil with the lowest fluxes at the Equator and high latitudes, and largest uptake fluxes at midlatitudes. Previous models simulated a dissimilar pattern with large uptake fluxes in equatorial regions, a difference that results primarily from improved representation of the soil moisture effect in MeMo.
MeMo simulations supported by observational data indicate that warm and semi-arid regions are the most efficient soil sink for atmospheric CH4. In these regions, tropical deciduous forest and dense open shrubland are characterized by relatively low soil moisture and constant temperature during the year, which are key factors that promote high rates of CH4 uptake by soil. In contrast, cold regions possessed the lowest CH4 uptake rates, in particular, tundra and boreal forest, which have a marked seasonality driven by temperature, making soil methanotrophy in such areas potentially sensitive to future global climate change. The warm and wet tropical evergreen forest biome has CH4 uptake rates that are ∼ 50 % less than warm and semi-arid regions because excess soil moisture impacts soil–atmosphere gas exchange, resulting in a smaller k0 (1.6 s−1). The extensive area of shrubland, grassland, steppe and savanna globally yields a high total uptake of CH4; however, there is presently a dearth of experimental data for these biomes and additional field observations are required to strengthen validation of MeMo simulations for these globally extensive areas.
MeMo simulations indicate that global soil uptake of atmospheric CH4 is reduced 4 % on average and by as much as 60 % in regions that receive high rates of atmospheric N deposition and N input from fertilizers. Globally, N deposition and input via fertilizers attenuates the soil sink for atmospheric CH4 by 1.38 Tg yr−1, which is 2–5 times less than previously reported values because of the refined representation of the nitrogen inhibition on soil CH4 oxidation in MeMo.
The accuracy of quantifying the modern soil sink for atmospheric CH4 is improved using MeMo. In addition, the model can be used to explore changes in the relative importance of soil methanotrophy in the global CH4 cycle in the past and the capacity of the soil sink to consume atmospheric CH4 under future global change scenarios.
MeMo was implemented in R (version 3.0.1). The model code and model output for 1990–2009 are available as the Supplement to this paper. In addition, we also provide a postprocessed driving data set to run an example model case study for the year 2000. All forcing data used in this study are available from the following sources:
-
temperature from CRU3.1, Harris et al. (2014):
https://crudata.uea.ac.uk/cru/data/hrg/; -
vegetation mask from Ramankutty and Foley (1999):
https://nelson.wisc.edu/sage/data-and-models/global-potential-vegetation/index.php; -
soil moisture from Dorigo et al. (2011) (satellite):
http://www.esa-soilmoisture-cci.org; -
soil moisture from TRENDY: (Sitch et al., 2015):
http://www-lscedods.cea.fr/invsat/RECCAP/; -
nitrogen deposition from Lamarque et al. (2013):
-
N input via fertilizers from Nischina et al. (2017):
https://www.earth-syst-sci-data.net/9/149/2017/; -
clay content and bulk density from Shangguan et al. (2014):
http://globalchange.bnu.edu.cn.
The supplement related to this article is available online at: https://doi.org/10.5194/gmd-11-2009-2018-supplement.
FMF and SA developed and modified the model. FMF and GMT created the code. FMF ran the simulations, analysed the data and created all figures. All authors contributed to the interpretation of the results and preparation of the paper.
The authors declare that they have no conflict of interest.
CONACyT Mexico is thanked for providing PhD funding support to
Fabiola Murguia-Flores. Sandra Arndt acknowledges funding from the European
Union's Horizon 2020 research and innovation programme under the Marie
Sklodowska-Curie grant agreement no. 643052 745 (C-CASCADES project).
Anita L. Ganesan is funded by a UK Natural Environment Research Council
Independent Research Fellowship NE/L010992/1. Guillermo Murray-Tortarolo
thanks the Universidad Nacional Autonoma de Mexico for providing funding
during his postdoctoral studies and CONACyT for funding during the “Catedra
CONACyT”. We thank Guangjuan Luo and Klaus Butterbach-Bahl for providing
methane uptake data from their long-term observational sites. The TRENDY
modelling compendium is gratefully acknowledged for providing soil moisture
data.
Edited by: Tomomichi Kato
Reviewed by: three anonymous referees
Adamsen, A. P. S. and King, G. M.: Methane Consumption in Temperate and Subarctic Forest Soils: Rates, Vertical Zonation, and Responses to Water and Nitrogen, Appl. Environ, Microbiol., 59, 485–490, 1993.
Allan, W., Struthers, H., and Lowe, D. C.: Methane carbon isotope effects caused by atomic chlorine in the marine boundary layer: Global model results compared with Southern Hemisphere measurements, J. Geophys. Res.-Atmos., 112, D04306, https://doi.org/10.1029/2006JD007369, 2007.
Arndt, S., Jørgensen, B. B., LaRowe, D. E., Middelburg, J. J., Pancost, R. D., and Regnier, P.: Quantifying the degradation of organic matter in marine sediments: A review and synthesis, Earth-Sci. Rev., 123, 53–86, https://doi.org/10.1016/j.earscirev.2013.02.008, 2013.
Aronson, E. L. and Helliker, B. R.: Methane flux in non-wetland soils in response to nitrogen addition: a meta-analysis, Ecology, 91, 3242–3251, https://doi.org/10.1890/09-2185.1, 2010.
Bodelier, P. L. E. and Laanbroek, H. J.: Nitrogen as a regulatory factor of methane oxidation in soils and sediments, FEMS Microbiol. Ecol., 47, 265–277, https://doi.org/10.1016/S0168-6496(03)00304-0, 2004.
Boeckx, P. and Van Cleemput, O.: Methane Oxidation in a Neutral Landfill Cover Soil: Influence of Moisture Content, Temperature, and Nitrogen-Turnover, J. Environ. Qual., 25, 178, https://doi.org/10.2134/jeq1996.00472425002500010023x, 1996.
Borken, W., Davidson, E. A., Savage, K., Sundquist, E. T., and Steudler, P.: Effect of summer throughfall exclusion, summer drought, and winter snow cover on methane fluxes in a temperate forest soil, Soil Biol. Biochem., 38, 1388–1395, https://doi.org/10.1016/j.soilbio.2005.10.011, 2006.
Born, M., Dörr, H., and Levin, I.: Methane consumption in aerated soils of the temperate zone, Tellus B, 42, 2–8, https://doi.org/10.1034/j.1600-0889.1990.00002.x, 1990.
Bradford, M. A., Ineson, P., Wookey, P. A., and Lappin-Scott, H. M.: The effects of acid nitrogen and acid sulphur deposition on CH4 oxidation in a forest soil: a laboratory study, Soil Biol. Biochem., 33, 1695–1702, https://doi.org/10.1016/S0038-0717(01)00091-8, 2001.
Bradley, J. A., Anesio, A. M., and Arndt, S.: Bridging the divide: a model-data approach to Polar and Alpine microbiology, FEMS Microbiol. Ecol., 92, fiw015, https://doi.org/10.1093/femsec/fiw015, 2016.
Brady, N. C., Weil, R. R., and Weil, R.: The Nature and Properties of Soils, Prentice-Hall, Upper Sadler River, 881 pp., 1999.
Bronson, K. F. and Mosier, A. R.: Suppression of methane oxidation in aerobic soil by nitrogen fertilizers, nitrification inhibitors, and urease inhibitors, Biol. Fertil. Soils, 17, 263–268, https://doi.org/10.1007/BF00383979, 1994.
Burke, R. A., Meyer, J. L., Cruse, J. M., Birkhead, K. M., and Paul, M. J.: Soil-atmosphere exchange of methane in adjacent cultivated and floodplain forest soils, J. Geophys. Res.-Atmos., 104, 8161–8171, https://doi.org/10.1029/1999JD900015, 1999.
Butterbach-Bahl, K. and Papen, H.: Four years continuous record of CH4-exchange between the atmosphere and untreated and limed soil of a N-saturated spruce and beech forest ecosystem in Germany, Plant Soil, 240, 77–90, https://doi.org/10.1023/A:1015856617553, 2002.
Cai, Z. C. and Mosier, A. R.: Effect of NH4Cl addition on methane oxidation by paddy soils, Soil Biol. Biochem., 32, 1537–1545, https://doi.org/10.1016/S0038-0717(00)00065-1, 2000.
Castro, M. S., Steudler, P. A., Melillo, J. M., Aber, J. D., and Bowden, R. D.: Factors controlling atmospheric methane consumption by temperate forest soils, Global Biogeochem. Cy., 9, 1–10, https://doi.org/10.1029/94GB02651, 1995.
Ciais, P., Sabine, C., Bala, G., Bopp, L., Brovkin, V., Canadell, J., Chhabra, A., DeFries, R., Galloway, J., Heimann, M., Jones, C., Le Quéré, C., Myneni, R. B., Piao, S., and Thornton, P.: Carbon and Other Biogeochemical Cycles, in: Climate Change 2013: The Physical Science Basis. Contribution of Working Group I to the Fifth Assessment Report of the Intergovernmental Panel on Climate Change, edited by: Stocker, T. F., Qin, D., Plattner, G.-K., Tignor, M., Allen, S. K., Boschung, J., Nauels, A., Xia, Y., Bex, V., and Midgley, P. M., Cambridge University Press, Cambridge, UK, New York, NY, USA, 2013.
Clapp, R. B. and Hornberger, G. M.: Empirical equations for some soil hydraulic properties, Water Resour. Res., 14, 601–604, https://doi.org/10.1029/WR014i004p00601, 1978.
Curry, C. L.: Modeling the soil consumption of atmospheric methane at the global scale, Global Biogeochem. Cy., 21, GB4012, https://doi.org/10.1029/2006GB002818, 2007.
Czepiel, P. M., Crill, P. M., and Harriss, R. C.: Environmental factors influencing the variability of methane oxidation in temperate zone soils, J. Geophys. Res.-Atmos., 100, 9359–9364, https://doi.org/10.1029/95JD00542, 1995.
Del Grosso, S. J., Parton, W. J., Mosier, A. R., Ojima, D. S., Potter, C. S., Borken, W., Brumme, R., Butterbach-Bahl, K., Crill, P. M., Dobbie, K., and Smith, K. A.: General CH4 oxidation model and comparisons of CH4 Oxidation in natural and managed systems, Global Biogeochem. Cy., 14, 999–1019, https://doi.org/10.1029/1999GB001226, 2000.
De Visscher, A. and Cleemput, O. V.: Induction of enhanced CH4 oxidation in soils: NH inhibition patterns, Soil Biol. Biochem., 35, 907–913, https://doi.org/10.1016/S0038-0717(03)00122-6, 2003.
Dorigo, W. A., Wagner, W., Hohensinn, R., Hahn, S., Paulik, C., Xaver, A., Gruber, A., Drusch, M., Mecklenburg, S., van Oevelen, P., Robock, A., and Jackson, T.: The International Soil Moisture Network: a data hosting facility for global in situ soil moisture measurements, Hydrol. Earth Syst. Sci., 15, 1675–1698, https://doi.org/10.5194/hess-15-1675-2011, 2011.
Dörr, H., Katruff, L., and Levin, I.: Proceedings of the NATO advanced research workshop Soil texture parameterization of the methane uptake in aerated soils, Chemosphere, 26, 697–713, https://doi.org/10.1016/0045-6535(93)90454-D, 1993.
Dutaur, L. and Verchot, L. V.: A global inventory of the soil CH4 sink, Global Biogeochem. Cy., 21, GB4013, https://doi.org/10.1029/2006GB002734, 2007.
Epstein, H. E., Burke, I. C., Mosier, A. R., Hutchinson, G. L.: Plant functional type effects on trace gas fluxes in the shortgrass steppe, in: Plant-Induced Soil Changes: Processes and Feedbacks, Developments in Biogeochemistry, edited by: Breemen, N. V., Springer Netherlands, 145–168, 1998.
Etheridge, D. M., Steele, L. P., Francey, R. J., and Langenfelds, R. L.: Atmospheric methane between 1000 A.D. and present: Evidence of anthropogenic emissions and climatic variability, J. Geophys. Res.-Atmos., 103, 15979–15993, https://doi.org/10.1029/98JD00923, 1998.
Fang, H., Cheng, S., Yu, G., Cooch, J., Wang, Y., Xu, M., Li, L., Dang, X., and Li, Y.: Low-level nitrogen deposition significantly inhibits methane uptake from an alpine meadow soil on the Qinghai–Tibetan Plateau, Geoderma, 213, 444–452, https://doi.org/10.1016/j.geoderma.2013.08.006, 2014.
Gulledge, J. and Schimel, J. P.: Low-Concentration Kinetics of Atmospheric CH4 Oxidation in Soil and Mechanism of NH Inhibition, Appl. Environ. Microbiol., 64, 4291–4298, 1998.
Harris, I., Jones, P. D., Osborn, T. J., and Lister, D. H.: Updated high-resolution grids of monthly climatic observations – the CRU TS3.10 Dataset, Int., J. Climatol., 34, 623–642, https://doi.org/10.1002/joc.3711, 2014.
Hein, R., Crutzen, P. J., and Heimann, M.: An inverse modelling approach to investigate the global atmospheric methane cycle, Global Biogeochem. Cy., 11, 43–76, https://doi.org/10.1029/96GB03043, 1997.
Ho, A., Kerckhof, F.-M., Luke, C., Reim, A., Krause, S., Boon, N., and Bodelier, P. L. E.: Conceptualizing functional traits and ecological characteristics of methane-oxidizing bacteria as life strategies, Environ. Microbiol. Rep., 5, 335–345, https://doi.org/10.1111/j.1758-2229.2012.00370.x, 2013.
Hurtt, G. C., Chini, L. P., Frolking, S., Betts, R. A., Feddema, J., Fischer, G., Fisk, J. P., Hibbard, K., Houghton, R. A., Janetos, A., Jones, C. D., Kindermann, G., Kinoshita, T., Goldewijk, K. K., Riahi, K., Shevliakova, E., Smith, S., Stehfest, E., Thomson, A., Thornton, P., Vuuren, D. P., and van Wang, Y. P.: Harmonization of land-use scenarios for the period 1500–2100: 600 years of global gridded annual land-use transitions, wood harvest, and resulting secondary lands, Clim. Change, 109, 117–161, https://doi.org/10.1007/s10584-011-0153-2, 2011.
Kirschke, S., Bousquet, P., Ciais, P., Saunois, M., Canadell, J. G., Dlugokencky, E. J., Bergamaschi, P., Bergmann, D., Blake, D. R., Bruhwiler, L., Cameron-Smith, P., Castaldi, S., Chevallier, F., Feng, L., Fraser, A., Heimann, M., Hodson, E. L., Houweling, S., Josse, B., Fraser, P. J., Krummel, P. B., Lamarque, J.-F., Langenfelds, R. L., Le Quéré, C., Naik, V., O'Doherty, S., Palmer, P. I., Pison, I., Plummer, D., Poulter, B., Prinn, R. G., Rigby, M., Ringeval, B., Santini, M., Schmidt, M., Shindell, D. T., Simpson, I. J., Spahni, R., Steele, L. P., Strode, S. A., Sudo, K., Szopa, S., van der Werf, G. R., Voulgarakis, A., van Weele, M., Weiss, R. F., Williams, J. E., and Zeng, G.: Three decades of global methane sources and sinks, Nat. Geosci., 6, 813–823, https://doi.org/10.1038/ngeo1955, 2013.
Klemedtsson, Å. K. and Klemedtsson, L.: Methane uptake in Swedish forest soil in relation to liming and extra N-deposition, Biol. Fertil. Soils., 25, 296–301, https://doi.org/10.1007/s003740050318, 1997.
Lamarque, J.-F., Dentener, F., McConnell, J., Ro, C.-U., Shaw, M., Vet, R., Bergmann, D., Cameron-Smith, P., Dalsoren, S., Doherty, R., Faluvegi, G., Ghan, S. J., Josse, B., Lee, Y. H., MacKenzie, I. A., Plummer, D., Shindell, D. T., Skeie, R. B., Stevenson, D. S., Strode, S., Zeng, G., Curran, M., Dahl-Jensen, D., Das, S., Fritzsche, D., and Nolan, M.: Multi-model mean nitrogen and sulfur deposition from the Atmospheric Chemistry and Climate Model Intercomparison Project (ACCMIP): evaluation of historical and projected future changes, Atmos. Chem. Phys., 13, 7997–8018, https://doi.org/10.5194/acp-13-7997-2013, 2013.
Le Mer, J. and Roger, P.: Production, oxidation, emission and consumption of methane by soils: A review, Eur. J. Soil Biol., 37, 25–50, https://doi.org/10.1016/S1164-5563(01)01067-6, 2001.
Li, C., Aber, J., Stange, F., Butterbach-Bahl, K., and Papen, H.: A process-oriented model of N2O and NO emissions from forest soils: 1. Model development, J. Geophys. Res.-Atmos., 105, 4369–4384, https://doi.org/10.1029/1999JD900949, 2000.
Low, P. F., Hoekstra, P., and Anderson, D. M.: Some Thermodynamic Relationships for Soils at or Below the Freezing Point: 2. Effects of Temperature and Pressure on Unfrozen Soil Water, Water Resour. Res., 4, 541–544, https://doi.org/10.1029/WR004i003p00541, 1968.
Luo, G. J., Kiese, R., Wolf, B., and Butterbach-Bahl, K.: Effects of soil temperature and moisture on methane uptake and nitrous oxide emissions across three different ecosystem types, Biogeosciences, 10, 3205–3219, https://doi.org/10.5194/bg-10-3205-2013, 2013.
MacDonald, J. A., Skiba, U., Sheppard, L. J., Hargreaves, K. J., Smith, K. A., and Fowler, D.: Soil environmental variables affecting the flux of methane from a range of forest, moorland and agricultural soils, Biogeochemistry, 34, 113–132, https://doi.org/10.1007/BF00000898, 1996.
McLain, J. E. T. and Ahmann, D. M.: Increased moisture and methanogenesis contribute to reduced methane oxidation in elevated CO2 soils, Biol. Fertil. Soils, 44, 623–631, https://doi.org/10.1007/s00374-007-0246-2, 2007.
Mclain, J. E. T., Kepler, T. B., and Ahmann, D. M.: Belowground factors mediating changes in methane consumption in a forest soil under elevated CO2, Global Biogeochem. Cy., 16, 1050, https://doi.org/10.1029/2001GB001439, 2002.
Moldrup, P., Kruse, C. W., Rolston, D. E., and Yamaguchi, T.: Modelling diffusion and reaction in soils: III. Predicting gas diffusivity from the Campbell soil-water retention model, Soil Sci., 161, 366–375, 1996.
Moldrup, P., Deepagoda, T. K. K. C., Hamamoto, S., Komatsu, T., Kawamoto, K., Rolston, D. E., and de Jonge, L. W.: Structure-Dependent Water-Induced Linear Reduction Model for Predicting Gas Diffusivity and Tortuosity in Repacked and Intact Soil, Vadose Zone J., 12, 1–11, https://doi.org/10.2136/vzj2013.01.0026, 2013.
Mosier, A. R., Parton, W. J., Valentine, D. W., Ojima, D. S., Schimel, D. S., and Delgado, J. A.: CH4 and N2O fluxes in the Colorado shortgrass steppe: 1. Impact of landscape and nitrogen addition, Global Biogeochem. Cy., 10, 387–399, https://doi.org/10.1029/96GB01454, 1996.
Mosier, A. R., Morgan, J. A., King, J. Y., LeCain, D., and Milchunas, D. G.: Soil-atmosphere exchange of CH4, CO2, NOx, and N2O in the Colorado shortgrass steppe under elevated CO2, Plant Soil, 240, 201–211, https://doi.org/10.1023/A:1015783801324, 2002.
Myhre, G., Highwood, E. J., Shine, K. P., and Stordal, F.: New estimates of radiative forcing due to well mixed greenhouse gases, Geophys. Res. Lett., 25, 2715–2718, https://doi.org/10.1029/98GL01908, 1998.
Nishina, K., Ito, A., Hanasaki, N., and Hayashi, S.: Reconstruction of spatially detailed global map of NH and NO application in synthetic nitrogen fertilizer, Earth Syst. Sci. Data, 9, 149–162, https://doi.org/10.5194/essd-9-149-2017, 2017.
Oh, Y., Stackhouse, B., Lau, M. C. Y., Xu, X., Trugman, A. T., Moch, J., Onstott, T. C., Jørgensen, C. J., D'Imperio, L., Elberling, B., Emmerton, C. A., St. Louis, V. L., and Medvigy, D.: A scalable model for methane consumption in arctic mineral soils, Geophys. Res. Lett., 43, 5143–5150, https://doi.org/10.1002/2016GL069049, 2016.
Oremland, R. S. and Culbertson, C. W.: Importance of methane-oxidizing bacteria in the methane budget as revealed by the use of a specific inhibitor, Nature, 356, 421–423, https://doi.org/10.1038/356421a0, 1992.
Phillips, R. L., Whalen, S. C., Schlesinger, W. H.: Response of soil methanotrophic activity to carbon dioxide enrichment in a North Carolina coniferous forest, Soil Biol. Biochem., 33, 793–800, https://doi.org/10.1016/S0038-0717(00)00227-3, 2001.
Potter, C. S., Davidson, E. A., and Verchot, L. V.: Estimation of global biogeochemical controls and seasonality in soil methane consumption, Chemosphere, 32, 2219–2246, https://doi.org/10.1016/0045-6535(96)00119-1, 1996.
Prather, M. J., Holmes, C. D., and Hsu, J.: Reactive greenhouse gas scenarios: Systematic exploration of uncertainties and the role of atmospheric chemistry, Geophys. Res. Lett., 39, L09803, https://doi.org/10.1029/2012GL051440, 2012.
Priemé, A. and Christensen, S.: Seasonal and spatial variation of methane oxidation in a Danish spruce forest, Soil Biol. Biochem., 29, 1165–1172, https://doi.org/10.1016/S0038-0717(97)00038-2, 1997.
Ramankutty, N. and Foley, J. A.: Estimating historical changes in global land cover: Croplands from 1700 to 1992, Global Biogeochem. Cy., 13, 997–1027, https://doi.org/10.1029/1999GB900046, 1999.
Ridgwell, A. J., Marshall, S. J., and Gregson, K.: Consumption of atmospheric methane by soils: A process-based model, Global Biogeochem. Cy., 13, 59–70, https://doi.org/10.1029/1998GB900004, 1999.
Rigby, M., Prinn, R. G., Fraser, P. J., Simmonds, P. G., Langenfelds, R. L., Huang, J., Cunnold, D. M., Steele, L. P., Krummel, P. B., Weiss, R. F., O'Doherty, S., Salameh, P. K., Wang, H. J., Harth, C. M., Mühle, J., and Porter, L. W.: Renewed growth of atmospheric methane, Geophys. Res. Lett., 35, L22805, https://doi.org/10.1029/2008GL036037, 2008.
Rigler, E. and Zechmeister-Boltenstern, S.: Oxidation of ethylene and methane in forest soils–effect of CO2 and mineral nitrogen, Geoderma, 90, 147–159, https://doi.org/10.1016/S0016-7061(98)00099-8, 1999.
Rosenkranz, P., Brüggemann, N., Papen, H., Xu, Z., Horváth, L., and Butterbach-Bahl, K.: Soil N and C trace gas fluxes and microbial soil N turnover in a sessile oak (Quercus petraea (Matt.) Liebl.) forest in Hungary, Plant Soil, 286, 301–322, https://doi.org/10.1007/s11104-006-9045-z, 2006.
Sabrekov, A. F., Glagolev, M. V., Alekseychik, P. K., Smolentsev, B. A., Terentieva, I. E., Krivenok, L. A., and Maksyutov, S. S.: A process-based model of methane consumption by upland soils, Environ. Res. Lett., 11, 075001, https://doi.org/10.1088/1748-9326/11/7/075001, 2016.
Saggar, S., Hedley, C. B., Giltrap, D. L., and Lambie, S. M.: Measured and modelled estimates of nitrous oxide emission and methane consumption from a sheep-grazed pasture, Agric. Ecosyst. Environ, 122, 357–365, https://doi.org/10.1016/j.agee.2007.02.006, 2007.
Saunois, M., Bousquet, P., Poulter, B., Peregon, A., Ciais, P., Canadell, J. G., Dlugokencky, E. J., Etiope, G., Bastviken, D., Houweling, S., Janssens-Maenhout, G., Tubiello, F. N., Castaldi, S., Jackson, R. B., Alexe, M., Arora, V. K., Beerling, D. J., Bergamaschi, P., Blake, D. R., Brailsford, G., Brovkin, V., Bruhwiler, L., Crevoisier, C., Crill, P., Covey, K., Curry, C., Frankenberg, C., Gedney, N., Höglund-Isaksson, L., Ishizawa, M., Ito, A., Joos, F., Kim, H.-S., Kleinen, T., Krummel, P., Lamarque, J.-F., Langenfelds, R., Locatelli, R., Machida, T., Maksyutov, S., McDonald, K. C., Marshall, J., Melton, J. R., Morino, I., Naik, V., O'Doherty, S., Parmentier, F.-J. W., Patra, P. K., Peng, C., Peng, S., Peters, G. P., Pison, I., Prigent, C., Prinn, R., Ramonet, M., Riley, W. J., Saito, M., Santini, M., Schroeder, R., Simpson, I. J., Spahni, R., Steele, P., Takizawa, A., Thornton, B. F., Tian, H., Tohjima, Y., Viovy, N., Voulgarakis, A., van Weele, M., van der Werf, G. R., Weiss, R., Wiedinmyer, C., Wilton, D. J., Wiltshire, A., Worthy, D., Wunch, D., Xu, X., Yoshida, Y., Zhang, B., Zhang, Z., and Zhu, Q.: The global methane budget 2000–2012, Earth Syst. Sci. Data, 8, 697–751, https://doi.org/10.5194/essd-8-697-2016, 2016.
Saxton, K. E., Rawls, W. J., Romberger, J. S., and Papendick, R. I.: Estimating Generalized Soil-water Characteristics from Texture, Soil Sci. Soc. Am. J., 50, 1031, https://doi.org/10.2136/sssaj1986.03615995005000040039x, 1986.
Schnell, S. and King, G. M.: Mechanistic Analysis of Ammonium Inhibition of Atmospheric Methane Consumption in Forest Soils, Appl. Environ. Microbiol., 60, 3514–3521, 1994.
Schnell, S. and King, G. M.: Responses of Methanotrophic Activity in Soils and Cultures to Water Stress, Appl. Environ. Microbiol., 62, 3203–3209, 1996.
Shangguan, W., Dai, Y., Duan, Q., Liu, B., and Yuan, H.: A global soil data set for earth system modelling, J. Adv. Model. Earth Syst., 6, 249–263, https://doi.org/10.1002/2013MS000293, 2014.
Singh, B. K., Bardgett, R. D., Smith, P., and Reay, D. S.: Microorganisms and climate change: terrestrial feedbacks and mitigation options, Nat. Rev. Microbiol., 8, 779–790, https://doi.org/10.1038/nrmicro2439, 2010.
Singh, J. S., Singh, S., Raghubanshi, A. S., Singh, S., Kashyap, A. K., and Reddy, V. S.: Effect of soil nitrogen, carbon and moisture on methane uptake by dry tropical forest soils, Plant Soil, 196, 115–121, https://doi.org/10.1023/A:1004233208325, 1997.
Sitaula, B. K., Hansen, S., Sitaula, J. I. B., and Bakken, L. R.: Methane oxidation potentials and fluxes in agricultural soil: Effects of fertilisation and soil compaction, Biogeochemistry, 48, 323–339, https://doi.org/10.1023/A:1006262404600, 2000.
Sitch, S., Friedlingstein, P., Gruber, N., Jones, S. D., Murray-Tortarolo, G., Ahlström, A., Doney, S. C., Graven, H., Heinze, C., Huntingford, C., Levis, S., Levy, P. E., Lomas, M., Poulter, B., Viovy, N., Zaehle, S., Zeng, N., Arneth, A., Bonan, G., Bopp, L., Canadell, J. G., Chevallier, F., Ciais, P., Ellis, R., Gloor, M., Peylin, P., Piao, S. L., Le Quéré, C., Smith, B., Zhu, Z., and Myneni, R.: Recent trends and drivers of regional sources and sinks of carbon dioxide, Biogeosciences, 12, 653–679, https://doi.org/10.5194/bg-12-653-2015, 2015.
Smith, K. A., Dobbie, K. E., Ball, B. C., Bakken, L. R., Sitaula, B. K., Hansen, S., Brumme, R., Borken, W., Christensen, S., Priemé, A., Fowler, D., Macdonald, J. A., Skiba, U., Klemedtsson, L., Kasimir-Klemedtsson, A., Degórska, A., and Orlanski, P.: Oxidation of atmospheric methane in Northern European soils, comparison with other ecosystems, and uncertainties in the global terrestrial sink, Global Change Biol., 6, 791–803, https://doi.org/10.1046/j.1365-2486.2000.00356.x, 2000.
Spahni, R., Wania, R., Neef, L., van Weele, M., Pison, I., Bousquet, P., Frankenberg, C., Foster, P. N., Joos, F., Prentice, I. C., and van Velthoven, P.: Constraining global methane emissions and uptake by ecosystems, Biogeosciences, 8, 1643–1665, https://doi.org/10.5194/bg-8-1643-2011, 2011.
Steinkamp, R., Butterbach-Bahl, K., and Papen, H.: Methane oxidation by soils of an N limited and N fertilized spruce forest in the Black Forest, Germany, Soil Biol. Biochem., 33, 145–153, https://doi.org/10.1016/S0038-0717(00)00124-3, 2001.
Tate, K. R., Ross, D. J., Saggar, S., Hedley, C. B., Dando, J., Singh, B. K., and Lambie, S. M.: Methane uptake in soils from Pinus radiata plantations, a reverting shrubland and adjacent pastures: Effects of land-use change, and soil texture, water and mineral nitrogen, Soil Biol. Biochem., 39, 1437–1449, https://doi.org/10.1016/j.soilbio.2007.01.005, 2007.
Ueyama, M., Takeuchi, R., Takahashi, Y., Ide, R., Ataka, M., Kosugi, Y., Takahashi, K., and Saigusa, N.: Methane uptake in a temperate forest soil using continuous closed-chamber measurements, Agric. For. Meteorol., 213, 1–9, https://doi.org/10.1016/j.agrformet.2015.05.004, 2015.
van den Pol-van Dasselaar, P., van Beusichem, M. L., and Oenema, O.: Effects of soil moisture content and temperature on methane uptake by grasslands on sandy soils, Plant Soil, 204, 213–222, https://doi.org/10.1023/A:1004371309361, 1998.
Vecherskaya, M. S., Galchenko, D. V. F., Sokolova, E. N., and Samarkin, V. A.: Activity and species composition of aerobic methanotrophic communities in tundra soils, Curr. Microbiol., 27, 181–184, https://doi.org/10.1007/BF01576018, 2013.
Veldkamp, E., Weitz, A. M., and Keller, M.: Management effects on methane fluxes in humid tropical pasture soils, Soil Biol. Biochem., 33, 1493–1499, https://doi.org/10.1016/S0038-0717(01)00060-8, 2001.
Visvanathan, C., Pokhrel, D., Cheimchaisri, W., Hettiaratchi, J. P. A., and Wu, J. S.: Methanotrophic activities in tropical landfill cover soils: effects of temperature, moisture content and methane concentration, Waste Manag. Res., 17, 313–323, https://doi.org/10.1034/j.1399-3070.1999.00052.x, 1999.
von Fischer, J. C., Butters, G., Duchateau, P. C., Thelwell, R. J., and Siller, R.: In situ measures of methanotroph activity in upland soils: A reaction-diffusion model and field observation of water stress, J. Geophys. Res.-Biogeo., 114, G01015, https://doi.org/10.1029/2008JG000731, 2009.
Wang, Y., Xue, M., Zheng, X., Ji, B., Du, R., and Wang, Y.: Effects of environmental factors on N2O emission from and CH4 uptake by the typical grasslands in the Inner Mongolia, Chemosphere, 58, 205–215, https://doi.org/10.1016/j.chemosphere.2004.04.043, 2005.
Wang, Z.-P. and Ineson, P.: Methane oxidation in a temperate coniferous forest soil: effects of inorganic N, Soil Biol. Biochem., 35, 427–433, https://doi.org/10.1016/S0038-0717(02)00294-8, 2003.
Wania, R., Ross, I., and Prentice, I. C.: Implementation and evaluation of a new methane model within a dynamic global vegetation model: LPJ-WHyMe v1.3.1, Geosci. Model Dev., 3, 565–584, https://doi.org/10.5194/gmd-3-565-2010, 2010.
West, A. E., Brooks, P. D., Fisk, M. C., Smith, L. K., Holland, E. A., Iii, C. H. J., Babcock, S., Lai, R. S., and Schmidt, S. K.: Landscape patterns of CH4 fluxes in an alpine tundra ecosystem, Biogeochemistry, 45, 243–264, https://doi.org/10.1023/A:1006130911046, 1999.
Whalen, S. C.: Influence of N and non-N salts on atmospheric methane oxidation by upland boreal forest and tundra soils, Biol. Fertil. Soils, 31, 279–287, https://doi.org/10.1007/s003740050657, 2000.
Whalen, S. C. and Reeburgh, W. S.: Moisture and temperature sensitivity of CH4 oxidation in boreal soils, Soil Biol. Biochem., 28, 1271–1281, https://doi.org/10.1016/S0038-0717(96)00139-3, 1996.
Zhang, W., Mo, J., Zhou, G., Gundersen, P., Fang, Y., Lu, X., Zhang, T., and Dong, S.: Methane uptake responses to nitrogen deposition in three tropical forests in southern China, J. Geophys. Res.-Atmos., 113, D11116, https://doi.org/10.1029/2007JD009195, 2008.
Zhuang, Q., Chen, M., Xu, K., Tang, J., Saikawa, E., Lu, Y., Melillo, J. M., Prinn, R. G., and McGuire, A. D.: Response of global soil consumption of atmospheric methane to changes in atmospheric climate and nitrogen deposition, Global Biogeochem. Cy., 27, 650–663, https://doi.org/10.1002/gbc.20057, 2013.
The requested paper has a corresponding corrigendum published. Please read the corrigendum first before downloading the article.
- Article
(7130 KB) - Full-text XML
- Corrigendum
-
Supplement
(20612 KB) - BibTeX
- EndNote