the Creative Commons Attribution 4.0 License.
the Creative Commons Attribution 4.0 License.
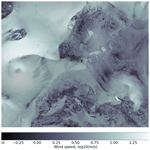
Multi-year simulations at kilometre scale with the Integrated Forecasting System coupled to FESOM2.5 and NEMOv3.4
Xabier Pedruzo-Bagazgoitia
Tobias Becker
Sebastian Milinski
Irina Sandu
Razvan Aguridan
Peter Bechtold
Sebastian Beyer
Jean Bidlot
Souhail Boussetta
Willem Deconinck
Michail Diamantakis
Peter Dueben
Emanuel Dutra
Richard Forbes
Rohit Ghosh
Helge F. Goessling
Ioan Hadade
Jan Hegewald
Thomas Jung
Sarah Keeley
Lukas Kluft
Nikolay Koldunov
Aleksei Koldunov
Tobias Kölling
Josh Kousal
Christian Kühnlein
Pedro Maciel
Kristian Mogensen
Tiago Quintino
Inna Polichtchouk
Balthasar Reuter
Domokos Sármány
Patrick Scholz
Dmitry Sidorenko
Jan Streffing
Birgit Sützl
Daisuke Takasuka
Steffen Tietsche
Mirco Valentini
Benoît Vannière
Nils Wedi
Lorenzo Zampieri
Florian Ziemen
We report on the first multi-year kilometre-scale global coupled simulations using ECMWF's Integrated Forecasting System (IFS) coupled to both the NEMO and FESOM ocean–sea ice models, as part of the H2020 Next Generation Earth Modelling Systems (nextGEMS) project. We focus mainly on an unprecedented IFS-FESOM coupled setup, with an atmospheric resolution of 4.4 km and a spatially varying ocean resolution that reaches locally below 5 km grid spacing. A shorter coupled IFS-FESOM simulation with an atmospheric resolution of 2.8 km has also been performed. A number of shortcomings in the original numerical weather prediction (NWP)-focused model configurations were identified and mitigated over several cycles collaboratively by the modelling centres, academia, and the wider nextGEMS community. The main improvements are (i) better conservation properties of the coupled model system in terms of water and energy budgets, which also benefit ECMWF's operational 9 km IFS-NEMO model; (ii) a realistic top-of-the-atmosphere (TOA) radiation balance throughout the year; (iii) improved intense precipitation characteristics; and (iv) eddy-resolving features in large parts of the mid- and high-latitude oceans (finer than 5 km grid spacing) to resolve mesoscale eddies and sea ice leads. New developments at ECMWF for a better representation of snow and land use, including a dedicated scheme for urban areas, were also tested on multi-year timescales. We provide first examples of significant advances in the realism and thus opportunities of these kilometre-scale simulations, such as a clear imprint of resolved Arctic sea ice leads on atmospheric temperature, impacts of kilometre-scale urban areas on the diurnal temperature cycle in cities, and better propagation and symmetry characteristics of the Madden–Julian Oscillation.
- Article
(20756 KB) - Full-text XML
- BibTeX
- EndNote
Current state-of-the-art climate models with typical spatial resolutions of 50–100 km still rely heavily on parametrizations for under-resolved processes, such as deep convection, the effects of sub-grid orography and gravity waves in the atmosphere, or the effects of mesoscale eddies in the ocean. The emerging new generation of kilometre-scale climate models can explicitly represent and combine several of these energy-redistributing small-scale processes and physical phenomena that were historically approximated or even neglected in coarse-resolution models (Palmer, 2014). The advantage of kilometre-scale models thus lies in their ability to more directly represent phenomena such as tropical cyclones (Judt et al., 2021) or the atmospheric response to small-scale features in the topography, for example, mountains, orography gradients, lakes, urban areas, and cities. The distribution and intensity (and particularly the extremes) of precipitation (Judt and Rios-Berrios, 2021), winds, and potentially also temperature will be different at improved spatial resolution. Importantly, features of deep convection start to be explicitly resolved at kilometre-scale resolutions. This does not only improve the local representation of the diurnal cycle, convective organization, and the propagation of convective storms (Prein et al., 2015; Satoh et al., 2019; Schär et al., 2020) but can also impact the large-scale circulation (Gao et al., 2023). Ultimately, the replacement of parametrizations by explicitly resolved atmospheric dynamics is also expected to narrow the still large uncertainty range of cloud-related feedbacks and thus climate sensitivity (Bony et al., 2015; Stevens et al., 2016).
Kilometre-scale resolutions are also particularly beneficial for the ocean, where mesoscale ocean eddies (Frenger et al., 2013), leads opening up in the sea ice cover, and the response of oceanic heat transport to the presence of narrow canyons (Morrison et al., 2020) can be studied directly. The small scales in the ocean, in particular mesoscale ocean eddies, have large-scale impacts on climate and control the distribution of nutrients, heat uptake, and carbon cycling (Hogg et al., 2015). Eddies also play an important role in the comprehensive response of the climate system to warming (Hewitt et al., 2022; Rackow et al., 2022; Griffies et al., 2015). In addition to the influence of mesoscale ocean features on the predictability of European weather downstream of the Gulf Stream area (Keeley et al., 2012), it has been proposed that higher-resolution simulations can enhance the representation of local heterogeneities in the sea ice cover (Hutter et al., 2022). Via their impact on small-scale ocean features such as eddies, atmospheric storms can impact deep water formation in the Labrador Sea (Gutjahr et al., 2022), an ocean region of global significance because of its role in the meridional overturning circulation of the ocean. Coupled ocean–atmosphere variability patterns such as the El Niño–Southern Oscillation (ENSO), the largest signal of interannual variability on Earth, may also benefit from kilometre-scale resolutions since ENSO-relevant ocean mesoscale features (Wengel et al., 2021) and westerly wind bursts should be better resolved.
High-resolution simulations pose significant challenges in terms of numerical methods, data management, storage, and analysis (Schär et al., 2020). To exploit the potential of kilometre-scale modelling, it is essential to develop scalable models that can run efficiently on large supercomputers and take advantage of the next generation of exascale computing platforms (Bauer et al., 2021; Taylor et al., 2023). Global atmosphere-only climate simulations at kilometre scale were pioneered by the NICAM group (Nonhydrostatic ICosahedral Atmospheric Model) almost 2 decades ago. On sub-seasonal to seasonal timescales, a global aqua-planet configuration at 3.5 km resolution was performed (Tomita et al., 2005), and the Madden–Julian Oscillation (MJO) was realistically reproduced at 7 and 3.5 km resolutions (Miura et al., 2007). In the last decade, the NICAM group, as well as the European Centre for Medium-Range Weather Forecasts (ECMWF), has run simulations on climate timescales at around 10–15 km spatial resolution. In particular, 14 km resolution 30-year AMIP (Kodama et al., 2015) and HighResMIP simulations (Kodama et al., 2021) were performed with NICAM. During Project Athena, the climate and seasonal predictive skill of ECMWF's Integrated Forecasting System was analysed at resolutions up to 10 km based on many 13-month simulations (totalling several decadal simulations), complemented with a 48-year AMIP-style simulation with future time slices at 15 km resolution (Jung et al., 2012). Recently, the NICAM group presented 10-year AMIP simulations at 3.5 km using an updated NICAM version (Takasuka et al., 2024). Other modelling groups around the world have also increased their model resolution towards the kilometre scale, and many participated in the recent DYAMOND intercomparison project (DYnamics of the Atmospheric general circulation Modeled On Non-hydrostatic Domains) with a grid spacing as fine as 2.5 km, simulations running over 40 d, and some of them already coupled to an ocean (Stevens et al., 2019).
While different modelling groups push global atmosphere-only simulations towards unprecedented resolutions (e.g. 220 m resolution in short simulations with NICAM), another scientific frontier has emerged around running kilometre-scale simulations on multi-year timescales, coupled to an equally refined ocean model. Indeed, in recent years, several kilometre-scale simulations have been run on up to monthly and seasonal timescales (Stevens et al., 2019, Wedi et al., 2020) but not many beyond these timescales and not yet with a kilometre-scale ocean (Miyakawa et al., 2017). This is due to the fact that even the most efficient high-resolution coupled models that are currently available require substantial computing resources to run, and the comprehensive and diverse code bases are also challenging to adapt to the latest computing technologies. As a result, the number of simulations and realizations that can be performed is limited, making it difficult to calibrate and optimize the model settings. Coarser-resolution models have been tuned for decades to be relatively reliable on the spatial scales that they can resolve and to match the historical period well for which high-quality observations are available. Nevertheless, this is often achieved by compensating errors, which cannot necessarily be expected to work similarly in a warming climate. These models also have some long-standing biases that can locally be larger than the interannual variability or the climate change signal (Rackow et al., 2019; Palmer and Stevens, 2019). The lack of explicitly simulated small-scale features is one likely source for these long-standing biases in weather and climate models (Schär et al., 2020). Coarser-resolution models also struggle with answering some important climate questions, such as the behaviour of extreme events in a warmer world and the impact of climate changes at the regional scale.
The European H2020 Next Generation Earth Modelling Systems (nextGEMS) project aims to build a new generation of eddy- and storm-resolving global coupled Earth system models to be used for multi-decadal climate projections at kilometre scale. By providing globally consistent information at scales where extreme events and the effects of climate change matter and are felt, global kilometre-scale multi-decadal projections will support the increasing need to provide localized climate information to inform local adaptation measures. The nextGEMS models build upon models that are also operationally used for numerical weather prediction (NWP): ICON, which is jointly developed by DWD and MPI-M (Hohenegger et al., 2023), and the Integrated Forecasting System (IFS) of ECMWF, coupled to the NEMO and FESOM ocean models. The nextGEMS project revolves around a series of hackathons, in which the simulations performed with the two models are examined in detail by an international community of more than 100 participants, followed by new model development iterations or “cycles”. The nextGEMS models have been (re-)designed for scalability and portability across different architectures (Satoh et al., 2019; Schulthess et al., 2019; Müller et al., 2019; Bauer et al., 2020, 2022) and lay the foundation for the Climate Change Adaptation Digital Twin developed in the EU's Destination Earth initiative (DestinE).
The operational NWP system at ECMWF uses an average 9 km grid spacing for the atmosphere coupled to an ocean at 0.25° spatial resolution (NEMO v3.4), which translates to a horizontal grid spacing of about 25 km along the Equator. While many coupled effects such as the atmosphere–ocean interactions during tropical cyclone conditions (Mogensen et al., 2017) can be realistically simulated at this resolution, ocean eddies in the mid-latitudes are still only “permitted” due to their decreasing size with latitude (Hallberg, 2013). This setup is far from our goal to explicitly resolve mesoscale ocean eddies all around the globe (Sein et al., 2017). In this study, we therefore focus mainly on configurations in which kilometre-scale versions of IFS (the main one at 4.4 km grid spacing in the atmosphere and land) are coupled to the FESOM2.5 ocean–sea ice model at about 5 km grid spacing, developed by the Alfred Wegener Institute, Helmholtz Centre for Polar and Marine Research (AWI). These configurations allow us to resolve many essential climate processes directly, for example mesoscale ocean eddies and sea ice leads in large parts of the mid- and high-latitude ocean, atmospheric storms, and certain small-scale features in the topography and land surface. We also test new developments of the IFS carried out in recent years at ECMWF to improve the representation of snow cover, land surface, and cities worldwide.
This paper documents the coupled kilometre-scale model configurations with the Integrated Forecasting System in Sect. 2. The technical and scientific model improvements, carried out along the nextGEMS model development cycles based on feedback by the nextGEMS community, are presented in Sect. 3. A first set of emerging advances stemming from the kilometre-scale character of the simulations is presented in Sect. 4, and more in-depth process studies will be the focus of dedicated future work. The paper closes with a summary and discussion of future steps in Sect. 5.
2.1 The Integrated Forecasting System and its coupling to NEMO and FESOM
The Integrated Forecasting System (IFS) is a spectral-transform atmospheric model with two-time-level semi-implicit, semi-Lagrangian time stepping (Temperton et al., 2001; Hortal, 2002; Diamantakis and Váňa, 2021). It is coupled to other Earth system components (land, waves, ocean, sea ice), and it is used in its version Cy48r1 (https://www.ecmwf.int/en/publications/ifs-documentation, last access: 7 November 2024), which has been used for operational forecasts at ECMWF since July 2023 (and modifications that will be detailed in this study). In its operational configuration (“oper”), the atmospheric component is coupled to the NEMO v3.4 ocean model. The octahedral reduced Gaussian grid (short “octahedral grid”) with a cubic (spectral) truncation (TCo) is used in the IFS (Malardel et al., 2016). The cubic truncation with the TCo grid implies higher effective resolution and better efficiency than the former linear truncation. It acts as a numerical filter without the need for expensive de-aliasing procedures, requires little diffusion, and produces small total mass conservation errors for medium-range forecasts; see Wedi (2014), Wedi et al. (2015), and Malardel et al. (2016), for further discussion. A hybrid, pressure-based vertical coordinate is used, which is a monotonic function of pressure and depends on the surface pressure (Simmons and Strüfing, 1983). The vertical coordinate follows the terrain at the lowest level and relaxes to a pure pressure-level vertical coordinate system in the upper part of the atmosphere. The vertical discretization scheme is a finite-element method using cubic B-spline basis functions (Vivoda et al., 2018; Untch and Hortal, 2004).
The atmosphere component of the IFS has a full range of parametrizations described in detail in ECMWF (2023a, b). The moist convection parametrization, originally described in Tiedtke (1989), is based on the mass-flux approach, and represents deep, shallow, and mid-level convection. For deep convection the mass flux is determined by removing a modified Convective Available Potential Energy (CAPE) over a given timescale (Bechtold et al., 2008, 2014), taking into account an additional dependence on total moisture convergence and a grid resolution dependent scaling factor to reduce the cloud-base mass flux further at grid resolutions higher than 9 km (Becker et al., 2021). The sub-grid cloud and precipitation microphysics scheme is based on Tiedtke (1993) and has since been substantially upgraded with separate prognostic variables for cloud water, cloud ice, rain, snow, and cloud fraction and an improved parametrization of microphysical processes (Forbes et al., 2011; Forbes and Ahlgrimm, 2014). The parametrization of sub-grid turbulent mixing follows the eddy-diffusivity–mass-flux (EDMF) framework, with a K-diffusion turbulence closure and a mass-flux component to represent the non-local eddy fluxes in unstable boundary layers (Siebesma et al., 2007; Köhler et al., 2011). The orographic gravity wave drag is parametrized following Lott and Miller (1997) and Beljaars et al. (2004) and a non-orographic gravity wave drag parametrization is described in Orr et al. (2010). The radiation scheme is described in Hogan and Bozzo (2018, ecRad). Full radiation computations are calculated on a coarser grid every hour, with approximate updates for radiation–surface interactions every time step at the model resolution.
The IFS land model ECLand (Boussetta et al., 2021) runs on the model grid and is fully coupled to the atmosphere through an implicit flux solver. ECLand represents the surface processes that interact with the atmosphere in the form of fluxes. The ECLand version in this work contains, among others, a four-layer soil scheme, a lake model, an urban model, a simple vegetation model, a multi-layer snow scheme, and a vast range of global maps describing the surface characteristics. A wave model component is provided by ecWAM to account for sea-state-dependent processes in the IFS (ECMWF, 2023c). The wave model runs on a reduced lat–long 0.125° grid with 36 frequencies and 36 directions. This means that the distance between latitudes is 0.125°, and the number of points per latitude is reduced polewards in order to keep the actual distance between grid points roughly equal to the spacing between two consecutive latitudes. The frequency discretization is such that ocean waves with periods between 1 and 28 s are represented.
For the purpose of nextGEMS and other related projects such as the DestinE Climate Change Adaptation Digital Twin, where an IFS-NEMO configuration with a ° ocean (NEMO v4) is also applied, the complementary IFS-FESOM model option was developed. We coupled the Finite VolumE Sea ice-Ocean Model, FESOM2 (Danilov et al., 2017; Scholz et al., 2019; Koldunov et al., 2019; Sidorenko et al., 2019), to IFS (see details below). Instead of using a coupler for this task, as for the OpenIFS-FESOM (Streffing et al., 2022), the alternative adopted here is to follow the strategy for IFS-NEMO coupling, where the ocean and IFS models are integrated into a single executable and share a common time-stepping loop (Mogensen et al., 2012). In this sequential coupling approach (akin to the model physics–dynamics and land surface coupling that occurs every model time step), the atmosphere advances for 1 h (length of the coupling interval), and fluxes are passed as upper boundary conditions to the ocean. It then in turn advances for 1 h, up to the same checkpoint. The following atmospheric step then uses updated surface ocean fields as lower boundary conditions for the next coupling interval (Mogensen et al., 2012). Note that there is no need to introduce a lag of one coupling time step because the ocean and atmosphere models run sequentially and not overlapping in parallel. A study into the effect of model lag on flux/state convergence by Marti et al. (2021) found that sequential instead of parallel coupling reduces the error nearly to the fully converged solution.
In the operational IFS, in areas where sea ice is present in the ocean model, currently a sea ice thickness of 1.5 m and no snow cover are assumed for the computation of the conductive heat flux on the atmospheric side. Our initial implementation for the multi-year simulations carried out in nextGEMS does not divert yet from this assumption of the operational configuration, in which the atmosphere only “sees” the sea ice fraction computed by the ocean–sea ice model. There are more consistent options available to couple the simulated sea ice albedo, ice surface temperature, ice, and snow thickness from the ocean models to the atmospheric component (Mogensen et al., 2012), and those will also be considered in future setups.
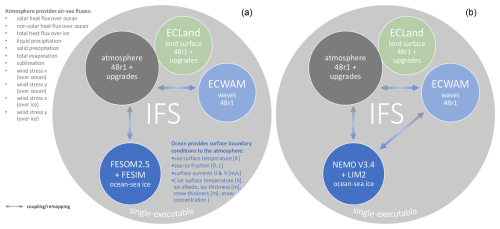
Figure 1Coupling of the Integrated Forecasting System (IFS) components in (a) IFS-FESOM and (b) IFS-NEMO in nextGEMS configurations. Coupling between the unstructured FESOM grid and the Gaussian grid of the atmosphere is via pre-prepared remapping weights in SCRIP format (Jones, 1999). Direct coupling between the surface wave model (ECWAM) and the ocean is only implemented in IFS-NEMO at the moment; in IFS-FESOM, the ocean and waves only interact indirectly via the atmosphere. ECWAM and the atmosphere have their own set of remapping weights for direct coupling, while ECLand and the atmosphere are more closely coupled to each other.
The oceans provide surface boundary conditions to the atmosphere (sea surface temperature, sea ice concentration, zonal and meridional surface currents), while the atmospheric component provides air–sea fluxes to the ocean models (as listed in Fig. 1). The exchange between the different model grids is implemented as a Gaussian distance-weighted interpolation for both directions. Since the implementation accepts any weight files as long as they are provided in SCRIP format (Jones, 1999), future setups will explore other interpolation strategies, such as the use of conservative remapping weights for the air–sea fluxes to ensure better flux conservation. River runoff for the ocean models is taken from climatology; for IFS-FESOM, the runoff from the COREv2 (Large and Yeager, 2009) flux dataset is applied based on Dai et al. (2009).
In order to couple FESOM with IFS, the existing single-executable coupling interface (i.e. the set of Fortran subroutines) between IFS and NEMO (Mogensen et al., 2012) has been extracted and newly implemented directly in the FESOM source code (Rackow et al., 2023c). From the perspective of the atmospheric component, after linking, FESOM and NEMO thus appear to IFS virtually identical in terms of provided fields and functionality in forecast runs with IFS. Clear gaps and differences to the operational configuration with NEMO v3.4 remain in terms of ocean data assimilation capabilities (NEMOVAR), ocean initial condition generation, and missing surface ocean-wave coupling (Fig. 1). However, these differences do not critically impact the multi-year simulations for nextGEMS described in this study or multi-decadal simulations planned for nextGEMS and DestinE.
2.2 Performed nextGEMS runs and cycles
The nextGEMS project relies on several model development cycles, in which the high-resolution models are run and improved based on community feedback from the analysis of successive runs. In an initial set of kilometre-scale coupled simulations (termed “Cycle 1”), the models were integrated for 75 d, starting on 20 January 2020 (Table 1). For Cycle 1, ECMWF's IFS in Cy47r3 (Cy46r1 for IFS-FESOM) was run at 9 km (TCo1279 in Gaussian octahedral grid notation) and 4.4 km (TCo2559) global spatial resolution. The runs at 9 km were performed with the deep convection parametrization, while at 4.4 km, the IFS was run with and without the deep convection parametrization. The underlying ocean models NEMO and FESOM2.1 had been run on an eddy-permitting 0.25° resolution grid in this initial model cycle (ORCA025 for NEMO and a triangulated version of this for FESOM, tORCA025). Based on the analysis by project partners during a hackathon organized in Berlin in October 2021, several key issues were identified both in the runs with IFS and in those runs with ICON (Hohenegger et al., 2023).
As will be detailed below, the IFS has been significantly improved for the longer “Cycle 2” simulations based on IFS Cy47r3 (Pedruzo-Bagazgoitia et al., 2022a; Wieners et al., 2023), where a 2.8 km simulation (TCo3999) has also been performed. For the purpose of nextGEMS Cycle 2 and 3, an ocean grid with up to 5 km resolution (“NG5”) has been introduced for the FESOM model, which is eddy-resolving in most parts of the global ocean (see Appendix B). The NG5 ocean has been spun up for a duration of 5 years in stand-alone mode, with ERA5 atmospheric forcing (Hersbach et al., 2020) until 20 January 2020. In contrast, NEMO performs active data assimilation to estimate ocean initial conditions for 20 January 2020.
Based on feedback from the second hackathon in Vienna in 2022, “Cycle 3” simulations based on IFS Cy48r1 for the third hackathon in Madrid (June 2023) have been further improved. The ocean has been updated to FESOM2.5 (Rackow et al., 2023c) and run coupled for up to 5 years (see Fig. 2 for an example wind speed snapshot at 4.4 km resolution). In Sect. 3, we will detail the series of scientific improvements in the atmosphere, ocean, and land components of IFS-NEMO/FESOM that were performed to address the identified key issues and how these successive steps result in a better representation of the coupled physical system.
Table 1nextGEMS configurations of the IFS and coupled simulations analysed in this study. The Gaussian octahedral grid notations TCo1279, TCo2559, and TCo3999 refer to 9, 4.4, and 2.8 km global atmospheric spatial resolution, respectively. The simulations were performed with constant greenhouse gas forcing from the year 2020 (CO2 = 413.72 ppmv, CH4 = 1914.28 ppbv, N2O = 331.80 ppbv, CFC11 = 857.38 pptv, CFC12 = 497.10 pptv), prognostic ozone, no volcanic aerosols, and the CAMS aerosol climatology (Bozzo et al., 2020).
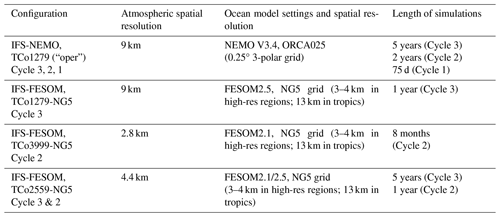
2.3 Technical refactoring for the FESOM2.5 ocean–sea ice model code
Prior to the start of nextGEMS, FESOM had been fully MPI-parallelized only and was shown to scale well on processor counts beyond 100 000 (Koldunov et al., 2019). In order to fully support hybrid MPI-OpenMP parallelization in the single-executable framework with IFS, numerous non-iterative loops in the ocean model code were rewritten with the release of FESOM version 2.5. The FESOM model has also been significantly refactored in other aspects over recent years to support coupling with IFS. In the single executable coupled system, the IFS initializes the MPI communicator (Mogensen et al., 2012) and passes it to the ocean model for initialization of FESOM. In particular, FESOM's main routine has been split into three cleanly defined steps, namely the initialization, time-stepping, and finalization steps. This was a necessary step for the current single-executable coupled model strategy at ECMWF, where the ocean is called and controlled from within the atmospheric model. The single-executable configuration is a necessary condition for coupled data assimilation at ECMWF. The adopted strategy means that some IFS-NEMO developments can be directly applied also to IFS-FESOM configurations. Similar to what is done for the wave and atmosphere components of the IFS, we implemented a fast “memory dump” restart mechanism for FESOM. This has the advantage that the whole coupled model can be quickly restarted as long as the parallel distribution (number of MPI tasks and OpenMP processes) does not change during the simulation.
2.4 Model output and online diagnostics
One of the concerns for the scientific evaluation of multi-year high-resolution simulations is the need to read large volumes of output from the global parallel filesystem. This is required for certain processing tasks, such as the computation of monthly averages in a climate context and regridding to regular meshes, so that the relevant information can be easily analysed and visualized. One way to mitigate this burden is to move these computations closer to where the data are produced and process the data in memory. Many of these computations are currently not possible in the IFS code, so starting in Cycle 3 we used MultIO (Sármány et al., 2024), a set of software libraries that provide, among other functionalities, user-programmable processing pipelines that operate on model output directly. IFS has its own Fortran-based I/O server that is responsible for aggregating geographically distributed three-dimensional information and creating layers of horizontal two-dimensional fields. It passes these pre-aggregated fields directly to MultIO for the on-the-fly computation of temporal means and data regridding.
One of the key benefits of this approach is that with the in-memory computation of, for example, monthly statistics, the requirement of storage space may be reduced significantly. Higher-frequency data may only be required for the computation of these statistics and as such would not need to be written to disk at all. For the nextGEMS runs in this study, however, the decision was taken to make use of MultIO mostly for user-convenience, i.e. to produce post-processed output in addition to the native high-frequency output. The computational overhead associated with this (approximately 15 % in this case) is more than offset by the increased productivity gained from much faster and easier evaluation of high-resolution climate output, particularly in the context of hackathons with a large number of participants. As a result, the MultIO pipelines have been configured to support the following five groups of output:
-
hourly or 6-hourly output (depending on variable) on native octahedral grids;
-
hourly or 6-hourly output (depending on variable), interpolated to regular (coarser) meshes for ease of data analysis (the MultIO configuration uses parts of the functionality of the Meteorological Interpolation and Regridding package (MIR), ECMWF's open-source re-gridding software, to be able to execute this in memory);
-
monthly means for all output variables on native grids;
-
monthly means for all output variables on regular (coarser) meshes, interpolated by MultIO calling MIR;
-
all fields encoded or re-encoded in GRIB by MultIO calling ECCODES, an open-source encoding library.
At the end of each pipeline, all data are streamed to disk, more specifically to the Fields DataBase (FDB; Smart et al., 2017), an indexed domain-specific object store for archival and retrieval – according to a well-defined schema – of meteorological and climate data. This mirrors the operational setup at ECMWF. For the nextGEMS hackathons, all simulations and their GRIB data in the corresponding FDBs have been made available in Jupyter Notebooks (Kluyver et al., 2016) via intake catalogs (https://intake.readthedocs.io/en/latest/, last access: 7 November 2024) using gribscan. The gribscan tools scans GRIB files and creates Zarr-compatible indices (Kölling et al., 2024).
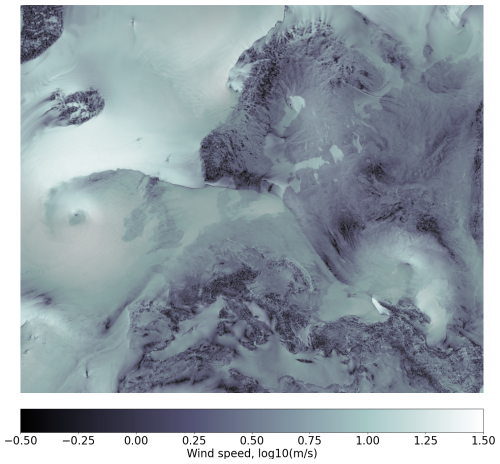
Figure 2Wind speed snapshot over Europe as simulated by the IFS with a 4.4 km spatial resolution in the atmosphere. The wind speed map is overlaid with a map of the zonal wind component in a grey-scale colour map for further shading, which has been made partly transparent. The figure does not explicitly plot land. Nevertheless, the high-resolution simulation clearly exposes the continental land masses and orographic details due to larger surface friction and hence smaller wind speeds (darker areas depict lower wind speeds). The image is a reproduction with Cycle 3 data of the award winning entry by Nikolay Koldunov for 2022's Helmholtz Scientific Imaging Contest, https://helmholtz-imaging.de/about_us/overview/index_eng.html (last access: 7 November 2024).
This section details model developments for the atmosphere (Sect. 3.1); ocean, sea ice, and wave (Sect. 3.2); and land (Sect. 3.3) components of IFS-FESOM and NEMO in the different cycles of nextGEMS. Following a short overview of identified key issues and developments at the beginning of each section, we present how those successive development steps translate to a better representation of the coupled physical system.
3.1 Atmosphere
3.1.1 Key issues and model developments
Water and energy imbalances
At the first nextGEMS hackathon, large water and energy imbalances were identified as key issues in the Cycle 1 simulations, which led to large biases in the top-of-the-atmosphere (TOA) radiation balance. If run for longer than the 75 d of Cycle 1, e.g. multiple years, this would lead to a strong drift in global mean 2 m temperature. Analysis confirmed that most of the energy imbalance in the IFS was related to water non-conservation and that this issue gets worse (i) when spatial resolution is increased, and (ii) when the parametrization of deep convection is switched off (hereafter “Deep Off”). This is because the semi-Lagrangian advection scheme used in the IFS is not conserving the mass of advected tracers, e.g. the water species (see Appendix A). However, while this issue was acknowledged to be detrimental for the accuracy of climate integrations, so far it has been thought that it was small enough to not significantly affect the quality of numerical weather forecasts, which span timescales ranging from a few hours to seasons ahead. To address the problem of water non-conservation in the IFS, a tracer global mass fixer was activated for all prognostic hydrometeors (cloud liquid, ice, rain, and snow) in nextGEMS Cycle 2, as well as water vapour (for more details, see Appendix A, which describes the mass fixer approach). The tracer mass fixer ensures global mass conservation, but it cannot guarantee local mass conservation. However, it estimates where the mass conservation errors are larger and inserts larger corrections in such regions, which is often beneficial for local mass conservation and accuracy (see Diamantakis and Agusti-Panareda, 2017). When adding tracer mass fixers to a simulation, the computational cost increases by a few percentage points (typically less than 5 %). Water and energy conservation in Cycle 1 versus Cycle 2 is discussed in Sect. 3.1.2.
Top-of-the-atmosphere radiation balance
To reduce drift in global mean surface temperature, it is essential that the global top-of-the-atmosphere (TOA) radiation imbalance is small. In the nextGEMS Cycle 2 simulation at 4.4 km resolution coupled to FESOM2.1 (Table 1), the TOA net imbalance, relative to observed fluxes from the CERES-EBAF product (Loeb et al., 2018), had been about +3 W m−2 (positive values indicate downward fluxes), resulting from a +5 W m−2 shortwave imbalance that was partly balanced by a −2 W m−2 longwave imbalance. Because of anthropogenic greenhouse gas emissions, CERES shows a +1 W m−2 imbalance. Due to the larger TOA imbalance, the nextGEMS Cycle 2 simulations warmed too much, by about 1K over the course of 1 year (see Sect. 3.1.3). Thus, addressing the TOA radiation imbalance was a major development focus in preparation for the 5-year integration in nextGEMS Cycle 3.
On top of IFS 48r1, in Cycle 3, we used a combination of model changes targeting a reduced TOA radiation imbalance, mostly affecting cloud amount. Changes that increased the fraction of low clouds are (i) a change restricting the detrainment of mid-level convection to the liquid phase; (ii) a reduction of cloud edge erosion following Fielding et al. (2020); and (iii) a reduction of the cloud inhomogeneity, which increases cloud amount as it reduces the rate of accretion. This change is in line with nextGEMS's kilometre-scale resolutions as cloud inhomogeneity is expected to be smaller at high resolutions. High clouds were increased in areas with strong deep convective activity by (iv) decreasing a threshold that limits the minimum size of ice effective radius, in agreement with observational evidence and (v) changing from cubic to linear interpolation for the departure point interpolation of the semi-Lagrangian advection scheme for all moist species except water vapour. The resulting TOA balance in Cycle 3 is discussed in Sect. 3.1.3.
Representation of intense precipitation and convective cells
Precipitation has many important roles in the climate system. It is not only important for the water cycle over land and ocean, but also provides a source of energy to the atmosphere, as heat is released when water vapour condensates and rain forms, which balances radiative cooling. Precipitation is also often associated with mesoscale or large-scale vertical motion, and the corresponding overturning circulation is crucial for the horizontal and vertical redistribution of moisture and energy within the atmosphere.
In kilometre-scale simulations in which the deep convection parametrization is switched off (e.g. Cycle 2 at 4.4 and 2.8 km resolution), convective cells tend to be too localized and too intense, and they lack organization into larger convective systems (e.g. Crook et al., 2019; Becker et al., 2021). The tropical troposphere also gets too warm and too dry, and these mean biases as well as biases that concern the characteristics of mesoscale organization of convection also affect the larger scales, for instance zonal-mean precipitation and the associated large-scale circulation. For example, with deep convection parametrization off in Cycle 2 (Deep Off), the Intertropical Convergence Zone (ITCZ) often organizes into a continuous and persistent line of deep convection over the Pacific at 5° N (see Fig. D1 in Appendix D), and the zonal-mean precipitation at 5° N is strongly overestimated.
To address these issues, instead of switching the deep convection scheme off completely, we have reduced its activity by reducing the cloud-base mass flux in Cycle 3. The cloud-base mass flux is the key ingredient of the convective closure, and depends on the convective adjustment timescale τ, which ensures a transition to resolved convection at high resolution via an empirical scaling function that depends on the grid spacing (discussed in more detail in Becker et al., 2021). To significantly reduce the activity of the deep convection scheme in Cycle 3, we use the value of the empirical scaling function that is by default used at 700 m resolution (TCo15999) already at 4.4 km resolution (TCo2559), which corresponds to a reduction of the empirical value that determines the cloud-base mass flux by a factor of 6 compared to its value at 9 km resolution. Precipitation characteristics in Cycle 3 vs Cycle 2 are discussed in Sect. 3.1.4.
3.1.2 Improvements of mass and energy conservation in Cycle 2 vs Cycle 1
To address the water non-conservation mentioned in Sect. 3.1.1, tracer mass fixers for all moist species were introduced in Cycle 2. Figure 3 shows that the Cycle 1 simulations with the IFS have an artificial source of water in the atmosphere. This artificial source is responsible for 4.6 % of total precipitation in the 9 km simulation with deep convection parametrization switched on (hereafter “Deep On”), which is also used for ECMWF's operational high-resolution 10 d forecasts, and for 10.7 % at 4.4 km with Deep Off. Further analysis after the hackathon by the modelling teams at ECMWF has shown that about 50 % of the artificial atmospheric water source is created as water vapour. The additional water vapour not only affects the radiation energy budget of the atmosphere but can also cause energy non-conservation when heat is released through condensation. The other 50 % of water is created as cloud liquid, cloud ice, rain, or snow. This is related to the higher-order interpolation in the semi-Lagrangian advection scheme introduced for cloud liquid, cloud ice, rain, and snow in IFS Cycle 47r3, which can result in spurious maxima and minima, including negative values, which are then clipped to remain physical. It turns out that the spurious minima are in excess of the spurious maxima, and by clipping them, the mass of cloud liquid, cloud ice, rain, and snow is effectively increased. When activating global tracer mass fixers, global water non-conservation is essentially eliminated (about 0.1 %) in the Cycle 2 simulations (Fig. 3).
On a global scale, the total energy budget of the atmosphere can be defined as
where T is temperature, and qv, qi, and qs are water vapour, cloud ice, and snow. Together, these terms describe the change in vertically integrated frozen moist static energy over time, while the last term on the left-hand side of the equation is the change in vertically integrated kinetic energy (KE). Sources and sinks of the atmosphere's total energy are Fs and Fq, which are the surface turbulent sensible and latent heat fluxes, and , which are the TOA and surface net radiative shortwave and longwave fluxes, and (Ls0−Lv0)Ps is the energy required to melt snow at the surface. Note that dissipation is not a source or sink of total energy.
Using this equation to calculate the global energy budget imbalance in Fig. 3, the Cycle 1 simulation with 9 km resolution has an atmospheric energy imbalance of 2.0 W m−2, and this imbalance increased to 6.4 W m−2 at 4.4 km resolution with Deep Off. In Cycle 2, the energy budget imbalance due to the mass conservation of water species is substantially smaller, having reduced to less than 1 W m−2. This remaining imbalance can be related to the explicit and semi-implicit dynamics because they are still non-conserving, for example causing an error in surface pressure, as well as the mass fixers. The remaining imbalance could be removed by adding a total energy fixer to the model.
As a result of activating the tracer mass fixers for all moist species, the overestimate of mean precipitation reduces, and the troposphere gets slightly colder and drier. While these changes are dominated on climate timescales by the effects that energy conservation has on global mean temperature, they can have a significant impact on timescales of numerical weather prediction. Indeed, the discussed setup with improved water and energy conservation is part of ECMWF's recent operational IFS upgrade in June 2023 (48r1) because it improves the skill scores of the operational weather forecasts (ECMWF Newsletter 172, 2022).
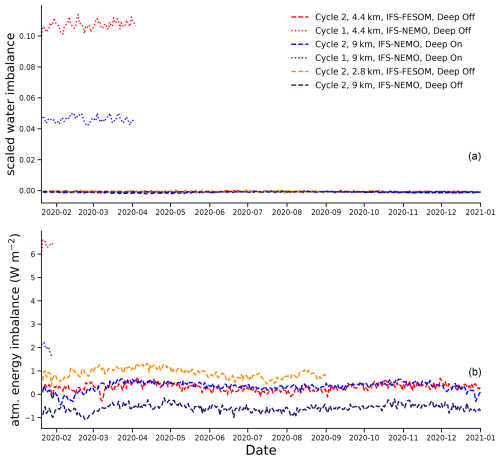
Figure 3(a) Daily mean water non-conservation and (b) daily-mean atmospheric energy imbalance, as a function of lead time for Cycle 1 and Cycle 2 simulations. Water non-conservation is computed as the daily change in globally integrated total water, taking account of surface evaporation and precipitation, as a fraction of the daily precipitation. The atmospheric energy imbalance is calculated with Eq. (1).
3.1.3 Realistic TOA radiation balance and surface temperature evolution in Cycle 3
Due to the model changes detailed in Sect. 3.1.1, the nextGEMS Cycle 3 simulations with the IFS have a TOA radiation imbalance that is within observational uncertainty, with respect to the net, shortwave, and longwave fluxes, at all resolutions (Fig. 4). This is not only true for the annual mean value, but also for the annual cycle of TOA imbalance (figure of 8 shape in Fig. 4). As a result, the global mean surface temperature in the Cycle 3 simulations is in close agreement with the ERA5 reanalysis (Hersbach et al., 2020) and stays in close agreement over the 5 years of coupled simulations (Figs. 5 and C1 in Appendix C). Going from Cycle 2 to Cycle 3, the warming over time is not evident anymore in IFS-FESOM and IFS-NEMO (Fig. 5). Differences in local warming over the Southern Ocean in the two models are further discussed in Sect. 3.2.2.
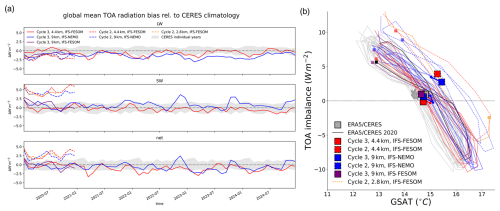
Figure 4Global-mean TOA radiation deviation from the CERES climatology in the 5-year-long nextGEMS simulations and global-mean TOA imbalance as a function of global-mean surface air temperature (GSAT). (a) Grey shading shows the climatological range of individual CERES years. Due to the free-running nature of the nextGEMS simulations, variations within the grey envelope are to be expected even in the absence of any bias. (b) Grey lines show the climatological range of individual CERES years (2001–2020) over ERA5 GSAT data (Hersbach et al., 2020). Thin lines are tracing monthly mean values, with a small square marking the final month for each simulation. Big squares depict annual means (dashed for Cycle 2, solid for Cycle 3), and for multi-year simulations, thick solid lines are tracing annual means for each year, with the big square marking the last simulated annual mean.
Locally, some of the persistent TOA radiation biases in Cycle 2 are also still evident in Cycle 3, for example a positive shortwave bias along coastlines in stratocumulus regions, while other biases, for example associated with deep convective activity over the Maritime Continent, have significantly reduced (not shown).
3.1.4 Improved precipitation characteristics in Cycle 3 vs Cycle 2 and larger-scale impacts
Snapshots of cloudy brightness temperature and precipitation over the Indian Ocean (Fig. 6) illustrate that after 12 d of simulation in Cycle 3, there are biases in the characteristics of precipitating deep convection compared to satellite observations, even after the developments for Cycle 3 (see Sect. 3.1.1) were introduced. The observations show multiple mesoscale convective systems (MCSs), which are associated with strong precipitation intensities and large anvil clouds. Neither the baseline 9 km Cycle 3 simulation nor the 4.4 km simulation manages to represent the MCS as observed. At 9 km, the convective cells are not well defined, with widespread areas of weak precipitation. Indeed, precipitation intensity is underestimated in this setup, with precipitation intensity rarely exceeding 10 mm h−1 (Fig. 7a). Instead of organizing into MCSs, hints of spurious gravity waves initiated from parametrized convective cells can be seen in the precipitation snapshot, emanating in different directions.
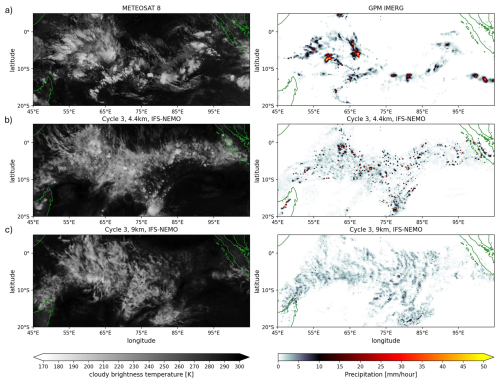
Figure 6Snapshot for 31 January 2020 at 21:00 UTC of infrared brightness temperature (left) and hourly precipitation rate (right) over the Indian Ocean, from (a) observations (Meteosat 8 SEVIRI channel 9 and GPM IMERG) and on forecast day 12 of (b) IFS-NEMO 4.4 km and (c) 9 km (third row) simulations. The simulations use the nextGEMS Cycle 3 setup except that they are run with a satellite image simulator and, for technical reasons, are coupled to NEMO V3.4 (ORCA025) here.
However, at 4.4 km resolution, the deep convection scheme is much less active, as the cloud-base mass flux has been reduced by a factor of 6 compared to its value at 9 km (see Sect. 3.1.1). Compared to the Cycle 2 simulations with Deep Off, the tropical troposphere is colder and more humid. This setup also features more realistic precipitation intensities, and particularly the strong precipitation of more than 10 mm h−1 is close to the satellite retrieval GPM IMERG (Fig. 7a), while the Cycle 2 simulations with Deep Off overestimate and with Deep On underestimate intense precipitation. In contrast, weak precipitation of 0.1 to 1 mm h−1 is most strongly overestimated at 4.4 km resolution in Cycle 3. This is mostly precipitation that stems from the weakly active deep convection scheme. Solutions of how to reduce this drizzle bias are being worked on, for example, through an increase in the rain evaporation rate.
A related issue is that the size of convective cells is too small, as illustrated by the size distribution of connected grid cells with precipitation exceeding 3 mm h−1 (Fig. 7b). The average size of a precipitation cell is rather similar in all simulations and only about half the value of that in GPM IMERG. While GPM IMERG has a substantial number of precipitation cells that exceed a size of 103 grid points, which for example would correspond to a precipitation object of 5°×2°, this size is almost never reached in the IFS simulations. The baseline simulations reach this size more often than the higher-resolution simulations but mainly in association with the spurious gravity waves, not because an MCS would be correctly represented. In summary, the representation of intense precipitation has been improved from Cycle 2 to Cycle 3, but that has not led to more realistic precipitation cell sizes. Even though it is possible that GPM IMERG overestimates precipitation cell size, cloudy brightness temperature shows the same issue (Fig. 6). Work with other models (e.g. ICON, NICAM, SCREAM) has also shown that an underestimation of precipitation cell size is a common issue in global kilometre-scale resolution simulations, in some models even leading to “popcorn” convection, and will require more attention in the future.
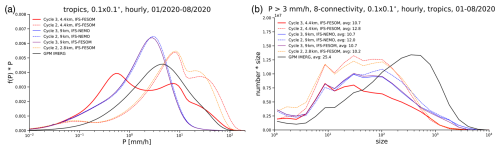
Figure 7(a) Frequency times bin intensity of hourly precipitation intensity in the tropics (30° S–30° N), conservatively interpolated to a 0.1° grid from January to August 2020. Following Berthou et al. (2019), the bins are exponential, meaning that the area under the curve represents the contribution of that intensity range to the mean. (b) Histogram of precipitation cell size times bin size, using a similar approach to that in (a). The precipitation cell size is defined as the number of connected grid cells on a 0.1° grid (also considering diagonal neighbours) where precipitation exceeds 3 mm h−1, counting cells in the whole tropics (30° S–30° N), again from January to August 2020. The average precipitation cell size is given in the legend. The observational estimate is from GPM IMERG.
As already mentioned in Sect. 3.1.1, the characteristics of mesoscale organization of convection also affect the larger scales. For example, in Cycle 2 simulations with Deep Off, the ITCZ often organizes into a continuous and persistent line of deep convection over the Pacific at 5° N (see Fig. D1 in Appendix D), and as a consequence, the zonal-mean precipitation is strongly overestimated. This bias improved significantly from Cycle 2 to Cycle 3, when switching from a setup with no deep convection scheme in Cycle 2 (at 2.8 and 4.4 km resolution) to a setup with reduced cloud-base mass flux in Cycle 3 (at 4.4 km). While the peak of precipitation around 5° N was overestimated by a factor of 2 during individual winter months in the 2.8 and 4.4 km Cycle 2 run (see Fig. D2 in Appendix D), the 4.4 km Cycle 3 run shows a very reduced bias, and the peak at 5° N is thus perfectly aligned with the GPM IMERG observations during September–December (Fig. 8d). The 9 km baseline run did not change significantly from Cycle 2 to Cycle 3, but it also shows some small improvements with regards to the overestimation of the precipitation peak at 5° N.
Comparing the FESOM and NEMO runs, it is striking that all FESOM runs overestimate precipitation in the Southern Hemisphere tropics around 10° S, hinting at a biased large-scale circulation, while NEMO runs show some good agreement with observations. The different seasons (Fig. 8b–d) show an overestimation of precipitation at 10° S only during January–April in the NEMO runs, while FESOM runs overestimate precipitation at 10° S during most of the year. Additionally, the FESOM runs also slightly underestimate precipitation at the Equator (particularly during January–April), hinting at a double ITCZ bias, which is a common issue in coupled simulations at kilometre-scale resolutions during boreal winter, e.g. in ICON (Hohenegger et al., 2023). Compared to ICON and other global coupled kilometre-scale models that contributed to the DYAMOND model intercomparison project (Stevens et al., 2019), the zonal-mean precipitation biases in IFS nextGEMS Cycle 3 are of similar nature to and in part smaller than in the other models.
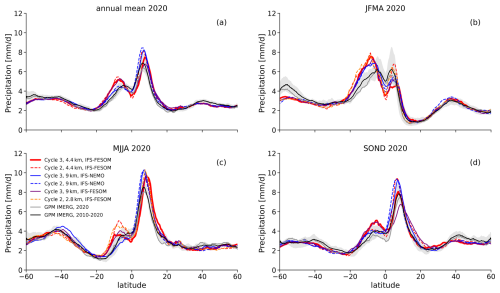
Figure 8Zonal-mean precipitation in nextGEMS Cycle 2 and 3, averaged over (a) the year 2020 and for different 4-month periods in 2020, (b) January–April, (c) May–August, and (d) September–December. Observations are from GPM IMERG for the year 2020 and for the 2010–2020 climatological period, indicating the climatological range of individual years via the grey shading.
3.1.5 Stratospheric Quasi-Biennial Oscillation
The Quasi-Biennial Oscillation (QBO) in the equatorial stratospheric winds is driven by momentum deposited by breaking small-scale convectively generated gravity waves (GWs) and large-scale Kelvin and Rossby-gravity waves (e.g. Baldwin et al., 2001). The QBO can have a downward influence on the troposphere (e.g. Scaife et al., 2022), and it is thus important to simulate it well in seasonal and decadal prediction models. As kilometre-scale models explicitly resolve GWs to a large extent, they have the potential to better simulate the QBO than lower-resolution models (e.g. CMIP), which fully rely on GW parametrizations. However, GW parametrizations are often tuned to get a good QBO in lower-resolution models (Garfinkel et al., 2022; Stockdale et al., 2022), and at higher resolution the resolved GW forcing can be overestimated with less freedom for tuning. For example, whether parametrized deep convection is switched on or off has a large impact on resolved GWs, with fully resolved convection generating more than 2 times stronger GW forcing (Stephan et al., 2019; Polichtchouk et al., 2021) and a QBO period that is – as a result – too fast.
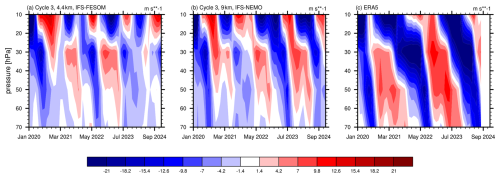
Figure 9Time evolution of monthly-mean zonal winds, averaged over the equatorial band 10° S–10° N, for (a) the 9 km Cycle 3 simulation with IFS-NEMO, (b) the 4.4 km Cycle 3 simulation with IFS-FESOM, and (c) the ERA5 reanalysis for reference.
We find that the QBO is reasonably well simulated in the nextGEMS Cycle 3 simulations at 9 km and even at kilometre-scale (4.4 km) resolution (Fig. 9). The periodicity is reasonable, peaking at around 20 months at 30 hPa for both simulations (calculated by performing fast Fourier transform (FFT) on the monthly time series). This can probably be further improved by tuning the strength of parametrized non-orographic GW drag, which is still on with reduced magnitude in both 9 and 4.4 km simulations, reduced to 70 % and 35 %, respectively, compared to that at 28 km resolution.
In the lower stratosphere below 40 hPa, the amplitude of the QBO, however, is underestimated (compare panels a–b to panel c in Fig. 9), especially for the eastward phase. This deficiency is also observed in many lower-resolution models (Bushell et al., 2022). We hypothesize that the overall reasonable QBO simulation at kilometre-scale resolution might partly be due to the parametrization for deep convection being still “slightly on” in the Cycle 3 simulations with IFS, as detailed in the previous section.
3.2 Ocean, sea ice, and waves
3.2.1 Key issues and model developments
From a model development point of view, one of the main purposes of the nextGEMS Cycle 3 simulations was to set up and test a fully coupled global model that runs over multiple years and still does not show drift in global mean surface temperature and other main climate characteristics, prior to performing the final multi-decadal integrations foreseen in nextGEMS. To improve the general ocean state, an eddy-resolving ocean grid had been introduced already from Cycle 2 onwards. To reduce the drift further (Fig. 5), in particular over the Southern Ocean where the model in Cycle 2 had still shown a strong warming over the ocean with time compared to the ERA5 range for 2020–2021, the FESOM ocean component has been updated to the latest release version 2.5, and coupling between the ocean and atmosphere has been improved.
Warm biases over the ocean
The warming ocean in Cycle 2 leads to an overall warming of the atmosphere as well. The 4.4 km IFS-FESOM simulations in Cycle 2 with 5 km resolution in the ocean had shown a warming over the Southern Ocean in winter and year-round in the tropics. For Cycle 3, the latter has been significantly improved by tuning the TOA balance and using partially active parametrized convection, while the former has been solved by a combination of different factors, namely (i) improvements in the consistency of the heat flux treatment between the atmosphere and ocean/sea ice component; (ii) heat being taken from the ocean in order to melt snow falling into the ocean, which had been overlooked before; (iii) the activation of a climatological runoff/meltwater flux around Antarctica (COREv2, Large and Yeager 2009); and (iv) a general update from FESOM2.1 to FESOM2.5 (Rackow et al., 2023c, https://github.com/FESOM/fesom2/releases/tag/2.5/, last access: 7 November 2024). The resulting more realistic temperature evolution in Cycle 3 is discussed in Sect. 3.2.2.
Ocean currents, eddy variability, and the mixed layer
The eddy-permitting ocean grid in Cycle 1 simulations with IFS-FESOM can impact not just the temperature evolution but also the simulated eddy variability, mean currents, and details of the simulated mixed layer, which all evolve on sub-5-year timescales and are thus relevant to the longer-term performance of a coupled model. An analysis of the resulting simulated ocean state, including mesoscale eddy statistics and the mixed layer, with the final ocean eddy-resolving IFS-FESOM simulations in Cycle 3, is presented in Sect. 3.2.3.
Sea ice performance
In Cycle 1 and 2, the sea ice representation in IFS-FESOM showed prominent deviations from the observed seasonal cycle in the Ocean and Sea Ice Satellite Application Facility (OSI-SAF) dataset. This could mainly be addressed by correcting the shortwave flux over ice with the release of FESOM version 2.5. The resulting sea ice performance in Cycle 3 is discussed in Sect. 3.2.4.
3.2.2 Improved Southern Ocean temperature evolution
As already mentioned in Sect. 3.1.3, IFS-FESOM simulations in Cycle 2 (TCo2559 and NG5 grid in the ocean) had shown a warming over the Southern Ocean in winter and year-round in the tropics. For Cycle 3, the improvement in IFS-FESOM 4.4 km is particularly evident when comparing to the operational 9 km IFS setup with NEMO V3.4. While the Southern Ocean shows a similar magnitude of anomalies in IFS-FESOM TCo2559-NG5 in year 5 compared to the first year, there appears to be an increase in anomalies over time in IFS-NEMO (Fig. 10). This has been confirmed in a second set of IFS-FESOM simulations at TCo399 resolution (28 km) and on the tORCA025 ocean grid (not shown).
3.2.3 Simulated ocean state in terms of currents, eddy variability, and mixed layer
Daily sea surface height (SSH) data are taken from the IFS-FESOM outputs and compared with the AVISO multi satellite altimeter data of daily gridded absolute dynamic topography, representing the observed SSH (Pujol et al., 2016). While ocean eddy variability in the 4.4 km IFS-FESOM Cycle 3 simulation and AVISO can be diagnosed from standard deviation of sea surface height, the structure of (geostrophic) mean currents is diagnosed here from the time-mean SSH.
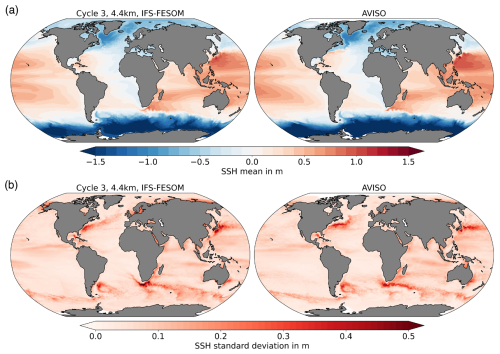
Figure 11Mean ocean currents and eddy variability expressed (a) as the time mean of the daily sea surface height (SSH) and (b) as the standard deviation of daily SSH data. The left column shows data from the Cycle 3 simulation of IFS-FESOM model, while the right column shows AVISO multi-satellite altimeter data. In AVISO, the global mean SSH is removed from each grid point. AVISO data consist of the time period 2017–2021, while 2020–2024 is used for IFS-FESOM.
Both the time mean and variability of SSH show excellent agreement between the simulation and observations from AVISO (Fig. 11). The position of the main gyres and the gradient of SSH are well reproduced, indicating a good performance in terms of position and strength of the main ocean currents. Ocean eddy variability is also very similar to the eddy-resolving NG5 grid that has been introduced for IFS nextGEMS simulations (see Fig. B1 in Appendix B). However, while there are positive indications, the North Atlantic Current as a northward extension of the Gulf Stream still underestimates SSH variability over the north-west corner. Moreover, Agulhas rings forming at the southern tip of Africa seem to follow a too narrow, static path compared to observations.
Mixed-layer depth (MLD) is calculated using a density threshold criterion of 0.03 kg m3 from the 10 m depth value. The in situ MLD climatology dataset produced by de Boyer Montégut et al. (2004) and de Boyer Montégut (2023) is based on about 7.3 million casts/profiles of temperature and salinity measurements made at sea between January 1970 and December 2021. While the qualitative agreement between the 4.4 km IFS-FESOM Cycle 3 simulation and observations is excellent (Fig. 12), IFS-FESOM underestimates MLD across most of the ocean areas, with values not exceeding 0–50 m. The largest biases are in the North Atlantic sector, which aligns with MLD bias results from stand-alone FESOM simulations (Treguier et al., 2023) for a 10–50 km ocean grid. Specifically, FESOM overestimates (deepens) MLD in the Labrador Sea, over the Reykjanes Ridge, and in the Norwegian Sea, while underestimating MLD in the Irminger Sea and the Greenland Sea.
Overall, the distribution of MLD in IFS-FESOM is comparable to the stand-alone lower-resolution FESOM ocean simulations. In coupled models, we could typically expect larger biases than presented here, although the relatively short 5-year period of the Cycle 3 simulation may not be sufficient to fully develop the MLD biases. In particular, IFS-FESOM does not show open-ocean convection in the Southern Ocean's Weddell Sea, which is a common bias in CMIP models.
3.2.4 Integrated sea ice performance metrics
The performance of the nextGEMS Cycle 3 simulations is analysed in terms of the sea ice extent and sea ice edge position (Fig. 13). The integrated ice edge error (IIEE), the absolute extent error (AEE), and the sea ice extent (SIE) metrics are used for comparing the model simulations and daily 2020 remote-sensing sea ice concentration observations from the Ocean and Sea Ice Satellite Application Facility (OSI SAF). Specifically, the recently released Global Sea Ice Concentration climate data record (SMMR/SSMI/SSMIS), release 3 (OSI-450-a; OSI SAF, 2022) is considered in our analysis. The IIEE is a positively defined metric introduced by Goessling et al. (2016), and it is commonly used for evaluating the correctness of the sea ice edge position in Arctic and Antarctic sea ice predictions (Zampieri et al., 2018, 2019). We compute the IIEE by summing the areas where the model overestimates and underestimates the observed sea ice edge, here defined by the 15 % sea ice concentration contour. The SIE is the hemispherically integrated area where the sea ice concentration is larger than 15 %. Finally, the AEE represents the absolute difference in the hemispheric SIE of models and observations, therefore not accounting for errors arising from a different distribution of the ice edge in the two sets.
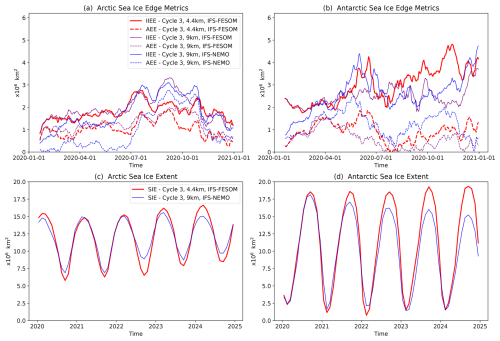
Figure 13(a) Arctic daily integrated ice edge error (IIEE; solid lines) and absolute extent error (AEE; dashed lines) for three different Cycle 3 simulations. Panel (b) is the same as panel (a) but for Antarctic sea ice. The IIEE and AEE metrics are computed by comparing the three model runs against remote-sensing sea ice concentration observations from OSI-SAF. Panels (c) and (d) show the Arctic and Antarctic sea ice extent for two different Cycle 3 simulations from 2020 until the end of 2024.
All model configurations show substantial errors in representing the initial state. In the Arctic, the error grows in the first simulation days in response to the active coupling between the sea ice components and the IFS atmospheric model (Fig. 13a). In the Antarctic, an initial error growth takes place for the IFS-NEMO model configuration, while modest error mitigation is seen for the two IFS-FESOM configurations (Fig. 13b). The latter feature suggests that a coupled setup could be better suited to represent the Antarctic sea ice processes in the FESOM models, at least for this specific instance. Both in the Arctic and Antarctic, the initial error of the IFS-NEMO configuration is substantially lower than that of the IFS-FESOM configurations. This behaviour is expected since NEMO performs active data assimilation, while the sea ice in FESOM is only constrained by the ERA5 atmospheric forcing (Hersbach et al., 2020) imposed during the ocean–sea ice model spinup. In the Antarctic, the initial error differences diminish quickly, and, after a couple of months, the errors of IFS-NEMO and IFS-FESOM are similar. In the Arctic, IFS-NEMO exhibits residual prediction skill over IFS-FESOM in late spring, 4–6 months after the initialization, possibly due to a more accurate description of the Arctic Ocean heat content influenced by the use of proper ocean data assimilation techniques. After the initialization, the pan-hemispheric sea ice model performance is similar for the three configurations, and attributing the error differences to the use of different model resolution or complexity is not obvious, confirming previous findings (e.g. Streffing et al., 2022; Selivanova et al., 2024). Overall, the model errors for the first year of simulations are in line with state-of-the-art seasonal prediction systems (Johnson et al., 2019; Mu et al., 2020, 2022), showing similar features in terms of seasonal error growth.
When considering longer timescales (5-year simulations), model drifts are visible for the IFS-NEMO configuration and, to a lesser extent, for the IFS-FESOM setup. In particular, the NEMO setup appears to progressively lose the winter sea ice cover in the Southern Ocean (Fig. 13d). This behaviour is not compatible with the observed interannual variability of the Antarctic sea ice, and it is likely due to the near-surface temperature warming, which is not affecting the IFS-FESOM setup. Our hypothesis is that the initialization strategy for FESOM and NEMO accounts for some of the discrepancies in the multi-year drift between IFS-NEMO and IFS-FESOM. We found that active data assimilation improved the model performance for the initial months, while an uncoupled ocean spinup might be preferable for minimizing the drift towards the ocean model's equilibrium state during the 5-year coupled simulation. In the Arctic, the sea ice extent tends to increase progressively in both the FESOM and NEMO setups, with an additional dampening of the seasonal cycle observed for NEMO (Fig. 13c). Different multi-year drift regimes between NEMO and FESOM could also be attributed to diverse complexity of the underlying sea ice models. The more sophisticated physical parametrizations of the NEMO V3.4 configuration could respond more to the active coupling with IFS compared to the FESOM setups.
3.2.5 Wind and waves
As written above in Sect. 2, in the IFS there is an active two-way coupling between the atmosphere and ocean waves. Surface wind stress generates ocean surface waves, and in turn those waves modulate the wind stress. The increase in resolution from 4.4 km relative to the 9 km for the IFS-FESOM simulations results in significant increases in wind speed in the storm tracks (∼ 50° S and ∼ 45° N; Fig. 14a), most likely due to the increased ability to resolve the intense winds in the extratropical cyclones. This increased resolution looks to be particularly important for the Southern Ocean, as the 4.4 km simulation is the only one of the three simulations that can achieve winds of realistic intensity in this area. We also note a significant improvement in the trade winds (∼ 15° N) for the 4.4 km IFS-FESOM simulation.
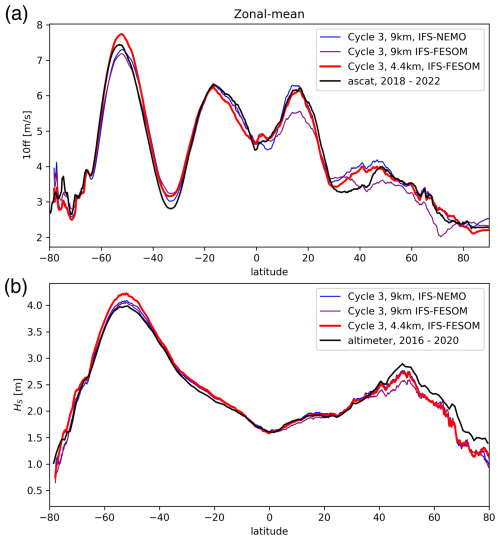
Figure 14Zonal means of 10 m wind speed “10ff” over ocean (a) and significant wave height (b) in nextGEMS Cycle 3. Observations in black are from Copernicus Marine Service for wind speed (“ascat”; scatterometer combined with ERA5), and the ESA-CCI (v3) cross-calibrated altimeter record for wave height (“altimeter”).
The waves in the storm tracks are also significantly larger (Fig. 14b). The increased wind is likely partly responsible for this increase. The second factor likely playing a role here is the change in fetch, i.e. the area of ocean over which the wind is contributing to wave growth. A notable decrease in mean sea ice concentration (more than 10 %) takes place in the 4.4 km simulation (Fig. E1a), thereby freeing up the ocean surface here for wave growth. These changes can be directly seen in the wave field in the according areas (Fig. E1b). These waves then continue to grow with the wind as they propagate into the Southern Ocean, thereby contributing to the larger waves seen in this region. For the NH storm track, this points to an improvement with respect to altimeter observations, but for the Southern Ocean the 4.4 km simulation is now somewhat overestimating the waves.
3.3 Land
Performing simulations at the kilometre scale inherently brings a richer picture in the atmosphere and ocean in terms of small-scale features, as more scales become explicitly resolved. To gain the full benefit of the resolution over land, it is important that the surface information is also at an equivalent or finer resolution. Therefore, work at ECMWF in recent years has been directed to provide the IFS surface model ECLand (Boussetta et al., 2021) with surface global ancillary information of a resolution down to 1 km or finer and to include additional processes that become relevant at those scales. These developments always had the improvement of the operational IFS as a goal and focused, therefore, on timescales from days to a few months. nextGEMS simulations present a timely opportunity to test these changes in parallel before they become operational and to assess their impact when fully coupled on multi-annual timescales. Most of the developments in this section are described in more detail by Boussetta et al. (2021). Here in this section, nextGEMS Cycle 2 and Cycle 3 will refer to IFS CY48r1 (ECMWF, 2023b) and CY49r1 (scheduled for 2024), respectively.
3.3.1 Kilometre-scale surface information
An improved land–water mask was included for nextGEMS Cycle 2. The original source belonging to the Joint Research Centre (JRC) had a nominal resolution of 30 m. The mask was further improved by including glacier data and new land–water and lake fraction masks. In parallel, lake depth data were improved (Boussetta et al., 2021).
Further changes to the land–water mask were tested in nextGEMS Cycle 3. The land use–land cover maps (LU/LC) used before nextGEMS Cycle 3 were based on those from GLCCv1.2 data (Loveland et al., 2000), which is based on observations from the Advanced Very High Resolution Radiometer (AVHRR) covering the period 1992–1993. They had a nominal resolution of about 1 km. In nextGEMS Cycle 3, we used new maps, based on ESA-CCI, which exploit the high resolution of recent remote-sensing products down to 300 m and will pave the way to enable observation-based time-varying LU/LC maps in the future. These maps lead to a more realistic overall increase in low vegetation cover compared to the GLCCv1.2-based maps, at the expense of the high vegetation cover. The new conversion from ESA-CCI to the Biosphere-Atmosphere Transfer Scheme (BATS) vegetation types used by ECLand also reduces the presence of ambiguous vegetation types like “interrupted forest” or “mixed forest”. In addition, work has been done on upgrading the leaf area index (LAI) seasonality and its disaggregation into low- and high-vegetation LAI. This improves, among others, the previously found overestimation of total LAI during March–April–May (MAM) and September–October–November (SON). This revised description of the vegetation will also be used in the next operational IFS cycle (49R1), and an initial implementation and evaluation is presented in Nogueira et al. (2021).
The thermodynamic effects of urban environments emerge at the surface as models refine resolution down to the kilometre scale, and the rural–urban contrast sharpens. To determine where to activate the urban processes at the surface, a global map of urban land cover is used in our nextGEMS Cycle 3 simulations. This map, based on information provided by ECOCLIMAP-SG at an initial 300 m horizontal resolution (McNorton et al., 2023; Faroux et al., 2013), will also be used in the next operational IFS cycle 49R1.
3.3.2 Kilometre-scale surface processes
The presence of the fine spatial information described above opens the path to simulate relevant kilometre-scale processes and interactions. In particular, the representation of snow, 2 m temperature, and urban areas was improved, as explained in the following.
A newly developed multi-layer snow scheme was implemented in IFS CY48r1 and was already used in the nextGEMS Cycle 2 (Arduini et al., 2019), substituting the existing snow bulk-layer scheme. The new scheme dynamically varies the number of snow model layers depending on the snow depth and provides snow temperature, density, liquid water content, and albedo as prognostic variables. In addition, snow and frozen soil parameters were modified for improved river discharge (Zsoter et al., 2022) and permafrost extent (Cao et al., 2022). An additional upgrade in nextGEMS Cycle 3 was a package of changes to ECLand, which will be included in the next operational IFS cycle (49R1). This contains an improved post-processing of 2 m temperature, reducing the warm bias present occasionally under very stable conditions. It also contains a significant upgrade to the representation of the near-surface impact of urban areas. For this purpose, the urban scheme developed in ECLand was activated. This scheme considers the urban environment as an interface connecting the sub-surface soil and the atmosphere above (McNorton et al., 2021, 2023). The urban tile comprises both a canyon and roof fraction. In terms of energy and moisture storage, the uppermost soil layer is not specific to the tile but represents a grid-cell average. This results in a weighted average that accounts for both urban and non-urban environments. The albedo and emissivity values used in radiation exchange computations (McNorton et al., 2021, 2023) are determined based on an assumption of an “infinite canyon”, taking into account “shadowing”. The roughness length for momentum and heat follows the model proposed by Macdonald et al. (1998) and varies according to urban morphology. Simplified assumptions regarding snow clearing and run-off are incorporated based on literature estimates (e.g. Paul and Meyer, 2001). Illustrative examples of urban cover characteristics and the impact of accounting for urbanized areas in Cycle 3 vs Cycle 2 simulations are highlighted in Sect. 4.3.
In this section, we will highlight three examples of notable advances in the Cycle 3 4.4 km nextGEMS simulations that emerge due to the kilometre-scale character of our simulations. Besides successes in the representation of the Madden–Julian Oscillation (MJO), an important variability pattern that is linked to the monsoons, we also provide examples of small-scale air–sea ice interactions in the Arctic and touch on atmospheric impacts due to the new addition of kilometre-scale cities in the IFS. We expect more in-depth process studies as part of ongoing analyses within the nextGEMS community and as part of dedicated future work.
4.1 MJO propagation and spectral characteristics of tropical convection
The MJO is a dominant intraseasonal variability mode in the tropics, characterized by slow eastward propagation of large-scale convective envelopes over the Indo-Pacific Warm Pool (Madden and Julian, 1972). The MJO convection and circulations have profound impacts on weather and climate variability globally (Zhang, 2013); therefore it is important to reproduce the MJO in global circulation models (GCMs) targeting seasonal-to-decadal simulations. Having the MJO well represented in models is indicative of a better tropical or global circulation. Because the reproducibility of the MJO is highly sensitive to the treatment of cumulus convection (e.g. Hannah and Maloney, 2011), many conventional GCMs that adopt cumulus parametrizations, which have uncertainties in the estimation of cumulus mass fluxes and moistening and heating rates, still struggle with simulating important MJO characteristics such as amplitudes, propagation speeds, and occurrence frequencies appropriately (e.g. Ling et al., 2019; Ahn et al., 2020; Chen et al., 2022). This issue might be improved by kilometre-scale simulations as a result of more accurate representation of moist processes, as represented by the first success of an MJO hindcast simulation with NICAM (Miura et al., 2007), but also other physical processes (besides convection) play a role for skilful MJO simulations (Yano and Wedi, 2021).
Figure 15 illustrates the MJO propagation characteristics in the Cycle 3 4.4 km IFS-FESOM simulation in comparison with the observations and the 9 km IFS-NEMO simulation, using the MJO event-based detection method (Suematsu and Miura, 2018; Takasuka and Satoh, 2020). Note that the observational reference is made by the interpolated daily outgoing longwave radiation (OLR) from the NOAA polar-orbiting satellite (Liebmann and Smith, 1996) and ERA-Interim reanalysis (Dee et al., 2011) during the period of 1982–2018. While the 9 km simulation already does a very good job and both the 9 and 4.4 km simulations can reproduce the overall eastward propagation of MJO convection coupled with zonal winds (Fig. 15b and c), the 4.4 km simulation allows improvement even further in terms of amplitudes and propagation speeds. Specifically, MJO convective envelopes in the 4.4 km simulation are continuously organized when they propagate into the Maritime Continent (see OLR anomalies in 100–120° E), and their propagation speeds become slower than in the 9 km simulation and thus closer to those in the observation. We hypothesize that kilometre-scale resolutions and partially resolved convection can better represent convective systems around complex land-sea distributions and topography. Nevertheless, the 4.4 km simulation still retains several biases compared to the observed MJOs, such as much faster propagation and weaker convection amplitudes to the east of 120° E (i.e. the eastern part of the Maritime Continent).
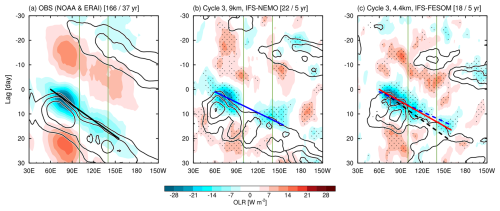
Figure 15Propagation characteristics of MJO convection and circulations composited from (a) observations, (b) IFS 9 km simulation with NEMO, and (c) IFS 4.4 km simulation with FESOM. Time–longitude diagrams of lagged-composite intraseasonal OLR (shading) and 850 hPa westerly wind anomalies (contours) averaged over 10° N–10° S. Contour interval is 0.5 m s−1, with zero contours omitted. Stippling in (b) and (c) denotes statistical significance of OLR anomalies at the 90 % level (all shading in (a) satisfies this significance). The number of detected MJO cases is denoted at the top of the figures together with analysis periods. Green lines indicate the longitudinal range over the Maritime Continent, and black, blue, and red lines indicate the centre of MJO convective envelopes for the observations, for the 9 km simulation, and for the 4.4 km simulation, respectively.
Notwithstanding the intricacies of tropical mesoscale circulations (Stephan et al., 2021), we further compare with linear Fourier analysis the appearance of convectively coupled equatorial wave activities between the observation and 9 and 4.4 km simulations (Fig. 16), following the methodology of Takayabu (1994) and Wheeler and Kiladis (1999). Several previous studies also evaluated the representation of equatorial waves in IFS simulations (Dias et al., 2018; Bengtsson et al., 2019). For the equatorially symmetric components of tropical convection (Fig. 16a–c), the IFS simulations at both resolutions can simulate Kelvin waves separated from the MJO, whereas the amplitudes of equatorial Rossby waves and tropical depression-type disturbances (i.e. westward-propagating systems in several-day periods) are somewhat underestimated, especially in the 4.4 km simulation. Meanwhile, the representation of the equatorially antisymmetric wave modes is significantly improved in the 4.4 km simulation; both n=0 eastward inertia-gravity waves and mixed Rossby-gravity waves can be reproduced with amplitudes as large as in the observation.
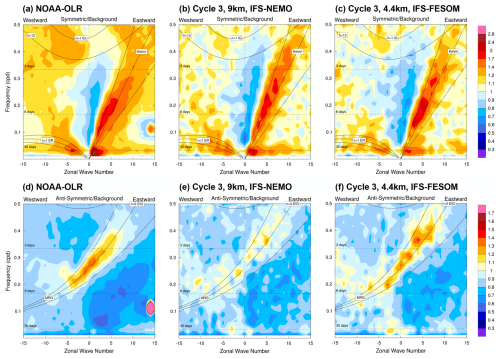
Figure 16Wavenumber–frequency power spectra of equatorially (a–c) symmetric and (d–f) antisymmetric components of tropical convection measured by OLR anomalies in (a, d) observations, (b, e) IFS 9 km simulation with NEMO, and (c, f) IFS 4.4 km simulation with FESOM. Power spectra are summed from 15° S to 15° N and plotted as the ratio of raw to background power. Abbreviations of WIG, TD, ER, MRG, and EIG indicate westward inertia-gravity waves, tropical depressions, equatorial Rossby waves, mixed Rossby-gravity waves, and eastward inertia-gravity waves, respectively. Dispersion curves for corresponding equatorial waves are plotted for equivalent depths h=12, 25, and 50 m. n denotes the number of meridional modes.
4.2 Sea ice imprint on the atmosphere
Leads are narrow open areas in the sea ice cover that typically form after deformation events, such as caused by a persisting Arctic storm over the ice cover. Individual leads can form typical “linear” channels of several kilometres length, while the larger connected lead systems can extend up to hundreds of kilometres (Overland et al., 1995) or even cross the entire Arctic. They are detectable in satellite synthetic-aperture radar images (von Albedyll et al., 2024). Especially in winter, open leads can significantly impact the stability of the atmospheric column and other atmospheric parameters above them. A change in sea ice cover of 1 % can cause near-surface temperature responses around 3.5 K (Lüpkes et al., 2008).
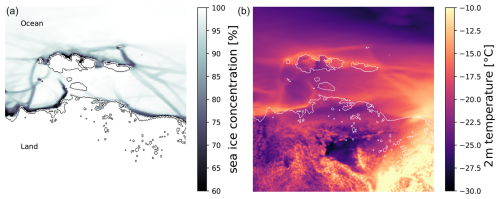
Figure 17Imprint of simulated Arctic sea ice leads on 2 m temperature in the Laptev Sea and East Siberian Sea. (a) Sea ice concentration field and (b) 2 m temperature field. The panels depict 13 February 2020, at 08:00, in the IFS-FESOM Cycle 3 simulation with TCo2559 (4.4 km), coupled to the NG5 ocean (∼ 4–5 km resolution in this area). The black and white contour lines represent the border between land and ocean areas.
At the kilometre-scale resolution employed here, there is first evidence of resolved linear kinematic features in the sea ice cover at a grid spacing of ∼ 4–5 km in our coupled simulations (ECMWF News Item, 2022). With resolutions of 4.4 and 2.8 km, the atmosphere can thus “see” these narrow features in the sea ice cover and simulate a response explicitly. Similar to the effect that mesoscale ocean eddies can have on the atmosphere above them (Frenger et al., 2013), we find that the leads in sea ice can strongly modulate the atmospheric state above them in our simulations. To give an example from the Arctic winter, north of Siberia in the Laptev and East Siberian Sea, due to the relatively warm ocean compared to the atmosphere, 2 m temperature anomalies over sea ice leads can often reach 10–20 K against the surrounding closed sea ice cover background (Fig. 17). While the realism with respect to the size, number, spatial distribution, and orientation of the simulated leads still needs to be quantified (Hutter et al., 2022), the direct simulation of sea ice lead effects within a coupled kilometre-scale climate model is entirely novel and opens up new areas of research. Potential climate impacts of this air–ice–ocean interaction on the atmospheric column, such as Arctic clouds (Saavedra Garfias et al., 2023), will be one focus of our future work.
4.3 Cities and urban heat island effects
Between Cycles 2 and 3, significant improvements have been achieved in representing urban heat island effects around the globe at the kilometre scale (Fig. 18). To give an example, the difference in land surface temperature (LST) between the city of Warsaw and its more rural surroundings during the 5-year clear-sky hours (in the JJA season) depicts a clear urban heat island effect (Fig. 18a), with temperature anomalies compared to the rural areas in exceedance of typically 1 K over any given day and exceeding 2 K around noon. When comparing with observations from the Satellite Application Facility on Land Surface Analysis (LSA SAF) LST product (Trigo et al., 2008), the results in Cycle 3 show a closer fit to the satellite product than was possible in Cycle 2; both the average temperature difference over the day and its temporal variability are better captured (Fig. 18a).
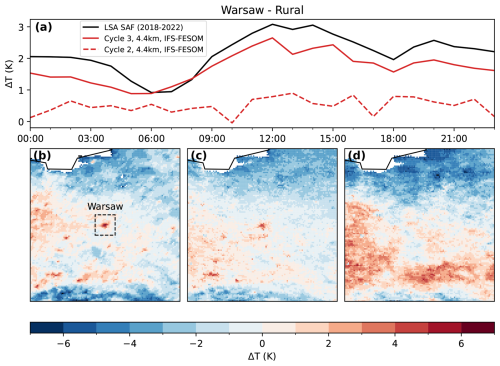
Figure 18Diurnal cycle of land surface temperature (LST) difference between the city of Warsaw and its rural surroundings, for (a) the summer months (JJA) during clear-sky conditions (5-year mean). The IFS 4.4 km simulations are given with red lines (Cycle 2 dashed, Cycle 3 solid), and observations from LSA SAF are given in black. The bottom panels show JJA-mean clear-sky LST anomaly maps at 13:00 local time, with respect to the surrounding rural LST average, for (b) observations from LSA SAF, (c) IFS 4.4 km Cycle 3 with the urban scheme, and (d) IFS 4.4 km in Cycle 2 without the urban scheme and using older land use–land cover maps.
Although the sub-diurnal variability is qualitatively well represented, the Cycle 3 modelled urban–rural contrast is systematically around 0.5 K smaller than in observations. We hypothesize that missing anthropogenic heating as well as an underestimation of the urban heat storage due to urban cover or building height being too low may explain some of the discrepancies. In terms of spatial variability of LST JJA-mean clear-sky anomalies, our Cycle 3 4.4 km IFS simulation (year 2020) matches kilometre-scale details of the LSA SAF dataset (2018–2022) well (compare Fig. 18b and c), while Cycle 2 4.4 km IFS cannot provide this local detail in the absence of updated land use–land cover maps and the urban scheme (Fig. 18d). Note also that the changes in high and low vegetation cover and vegetation types in Cycle 3 impact the areas found to be too warm in Cycle 2 in the south and east of Warsaw positively. These results illustrate clearly that high-resolution surface information as well as an urban scheme will be necessary in the context of the increasing need for local climate information on a city scale and for local projections of direct socio-economic relevance.
In this paper, storm- and eddy-resolving simulations performed with the nextGEMS configurations of the ECMWF Integrated Forecasting System have been described and analysed. While we have also presented eddy-permitting simulations with IFS-NEMO as the ECMWF operational baseline configuration, we have mostly focused on IFS-FESOM runs that feature not only the highest atmospheric resolution (4.4 km and also 2.8 km) but also an eddy-resolving ocean at 5 km. The large-scale performance in terms of the mean state has been presented, such as top-of-the atmosphere radiation balance and surface temperature biases, but also important variability patterns (e.g. MJO and QBO) that can be analysed in 5-year long simulations. The illustrated set of emerging advances in the kilometre-scale nextGEMS simulations are first indications of the added value of kilometre-scale modelling and explicit simulation of smaller scales. We expect to be able to show more of these examples once longer simulations will be available from the multi-decadal production simulations planned in nextGEMS for 2024/2025. In this study it is the first time that the model configuration and quality of the simulations with IFS-FESOM have been described; and it thus represents a significant milestone in terms of both documentation of this novel model capability and the scientific readiness of the coupled modelling system.
A number of model advances delivered in the nextGEMS development cycles improved the realism of the kilometre-scale simulations. For example, activating mass fixers for water vapour, cloud liquid, ice, rain, and snow made global water non-conservation negligible and reduced energy non-conservation to an amount that is acceptable for long climate simulations. Importantly, global water conservation turns out to be beneficial, not only for long climate integrations but also for the quality of ECMWF's medium-range weather forecasts. Work for ECMWF's recent operational IFS upgrade in June 2023 (48r1) showed that the model changes performed to fix the water and energy imbalances reduce the overestimation of mean precipitation at different timescales and improve the skill scores for the recent operational resolution upgrade for medium-range ensemble weather forecasts (ECMWF Newsletter 172, 2022). For example, in 9 km forecasts where we ensured global water conservation, the mean absolute error of precipitation against rain gauge measurements is about 2 %–3 % smaller. This is a great example of model development from the nextGEMS multi-year simulations feeding into the improvement of the operational NWP system at ECMWF.
Variability patterns that could be studied with the 5-year nextGEMS simulations so far are the Madden–Julian Oscillation (MJO) and the Quasi-Biennial Oscillation (QBO) in the equatorial stratospheric winds. The QBO is simulated with reasonable periodicity, which is typically challenging for kilometre-scale models without any active parametrization for deep convection. The remaining deficiencies we explained are likely due to the overly active vertical diffusion parametrization in stable conditions, which will be addressed in an upcoming version of the IFS. The MJO is similarly well represented in both the 9 and 4.4 km simulations. However, the MJO convective envelopes are continuously organized in the 4.4 km simulation when they propagate over the Maritime Continent, which is in better agreement with observations. We think that this is not just an effect of sampling different numbers of MJO events in our simulations and in the observations (simulated 5-year periods at 9 and 4.4 km resolution versus long-term observational period) since the observed MJO for shorter periods of time (e.g. 2011–2015) shows a similar result to the full observational record. The realistic representation of tropical variability and wave activity in the IFS at 9 and 4.4 km is the result of 15 years of sustained efforts in model developments, notably convection, cloud–radiation interaction, and air–sea coupling (Bechtold et al., 2008; Dias et al., 2018). The documented additional improvements in the 4.4 km simulation compared to 9 km may result from reduced cloud-base mass fluxes (i.e. more weight on explicit convection), but further detailed study of this subject is part of our future work.
With our kilometre-scale simulations that resolve mesoscale ocean eddies over large parts of the globe, we can also investigate coupled effects between sea ice leads, open narrow channels in the sea ice cover, and the atmosphere above them for the first time. Leads form during deformation events and can span distances from several to hundreds of kilometres. From limited observations and field campaigns, it is known that sea ice leads can significantly impact the stability and temperature of the atmospheric column, especially in winter. We find that our model can resolve the linear features of the leads and represent explicitly the resulting heating of the atmosphere. This is a novel and promising approach that reveals new aspects of the air–ice–ocean interaction.
The nextGEMS model configurations are also starting points for the Climate Adaptation Digital Twin in DestinE, which aims to provide local climate information, for instance at the scale of cities, globally. The urban heat island effect, which is the phenomenon of higher temperatures in urban areas compared to rural areas, is an aspect of socio-economic importance that will need to be accurately represented by kilometre-scale models in the future. In this study, we have shown that the implementation of an urban scheme in the IFS for nextGEMS Cycle 3 can significantly improve the simulation of land surface temperature (LST) over urban areas around the world, compared to previous model cycles that were missing specific urban tiles. The example of Warsaw illustrates the improvement in both temporal and spatial variability of land surface temperatures when compared to observations. We have also identified some limitations, such as nocturnal LST differences, which may be related to the lack of some anthropogenic heating in the model. Our first results here demonstrate the necessity and benefit of using an urban scheme in kilometre-scale models for future efforts to provide reliable local climate information at the city scale.
While kilometre-scale model resolution is of benefit for the representation of the atmosphere, ocean, sea ice, and land, it is also of importance for our understanding of other components of the climate system that have not been covered in this study yet, such as deep ocean circulation and ice sheet behaviour. For example, ocean heat transport at depth towards the Antarctic ice sheet and ice-shelf cavities is localized in narrow canyons (Morrison et al., 2020). Resolving bathymetric features like this and their potentially far-reaching impacts could be a strength of high-resolution models. Another example is the equilibration of the Antarctic Circumpolar Current, which is a balance of the wind-driven circulation and the opposing eddy-induced circulation cells. While transient ocean eddies can be parametrized to some degree, the effect of standing eddies (or meanders of this current) is beyond what parametrizations can achieve (Bryan et al., 2014). The first studies indicate that explicit simulation of these effects with kilometre-scale ocean models might be warranted to achieve higher confidence in projections of the Southern Ocean and global sea level rise (van Westen and Dijkstra, 2021; Rackow et al., 2022).
We have demonstrated that kilometre-scale modelling, which will soon enable multi-decadal simulations, has become feasible and offers advantages over lower-resolution models. At the scales used in this study, some modified subgrid parametrizations (e.g. deep convection with reduced cloud-base mass flux) are still active for best performance, even though the influence of resolved-scale horizontal and vertical motions increases. The results presented here prove that our seamless model development approach, where numerical weather prediction models are extended for kilometre-scale multi-decadal climate applications, is useful (Randall and Emanuel, 2024) and can benefit the original NWP application as well. As we have shown by running those models for 5 years, the kilometre-scale simulations improve the representation of atmospheric circulation and extreme precipitation but also enhance the coupling between the atmosphere, land, urban areas, ocean, and sea ice. We have revealed novel interactions among these components for the first time that will be further explored in ongoing work. With upcoming multi-decadal simulations from the nextGEMS and DestinE projects we will be able to generate even more statistics on kilometre-scale modelling soon, with an extended set of simulations from several models. These projects aim to provide accurate and globally consistent information on local climate change – at the scales that matter for individual cities or local impact modelling.
The IFS uses a semi-Lagrangian (SL) advection scheme that is stable for long time steps and essential for the efficiency of the overall model. It is also multi-tracer efficient as many fields can be transported with a relatively small overhead: to advect a field (e.g. temperature, wind components, tracers), the upstream departure locations of the model grid points are computed, but these are the same for all fields. The only remaining task is then to find the value of each field by interpolation to the departure location (for details, see Diamantakis and Váňa, 2021). However, despite being accurate and efficient, the transport scheme lacks local and global conservation. In the absence of sources/sinks, the global mass of a tracer should remain constant; however, SL advection changes its global mass slightly. This change depends strongly on the spatial characteristics of the tracer such as smoothness of the field and its geographic location, with larger conservation errors for tracers that have sharp gradients and interact with the orography.
Conservation properties are important for water and energy budgets, especially for high resolutions. A practical solution that restores the global mass conservation of water tracers without altering the efficient and accurate numerical formulation of the IFS is the mass fixer approach. However, simple mass fixers that change each tracer grid point value by the same proportion may result in unwanted biases in some regions. Hence, a more “local” approach is applied in the IFS advection scheme, which was originally developed and tested for atmospheric composition tracers yielding accurate results when compared against observations (Diamantakis and Fleming, 2014; Diamantakis and Agusti-Panareda, 2017). This is a “weighted” approach as the correction of the tracer field at each grid point depends on a weight factor that is proportional to a local error measure. The correction restores global conservation, using local criteria, and it also preserves positive definiteness and monotonicity of the field.
The 5 km nextGEMS ocean grid in this study (termed “NG5”) makes use of the multi-resolution mesh capabilities provided by the FESOM ocean–sea ice model (Fig. B1). From nextGEMS Cycle 2 and the following cycles, FESOM was run with this new eddy-resolving ocean grid with spacing of less than ∼ 5 km (at the poles) and around 13 km in the tropics. This grid, specifically designed by the Alfred Wegener Institute (AWI) to better match the high atmospheric resolution of 4.4 km in the IFS, allows areas of particular interest to be better resolved at higher resolution, such as the western boundary currents or the Southern Ocean. The mesh was created with the JIGSAW-GEO package (Engwirda, 2017).
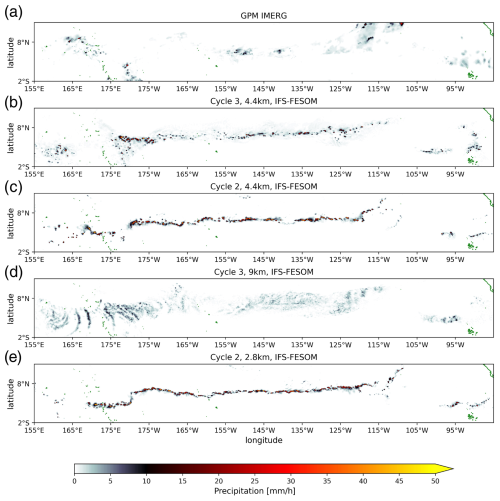
Figure D1Snapshots of precipitation in nextGEMS Cycle 2 and 3 simulations in the tropical Pacific at 5° N, compared to observations from (a) GPM IMERG. The ITCZ often organizes into a continuous and persistent line of deep convection over the Pacific at 5° N in (c, e) Cycle 2 at 4.4 and 2.8 km resolution, with strongly overestimated zonal-mean precipitation along this latitude. In (b) Cycle 3 this has been addressed via a reduced cloud-base mass flux with 4.4 km resolution. The (d) 9 km Cycle 3 simulation uses active deep convection parametrization (Deep On).
The latest version FESOM2.5 including all developments used in nextGEMS Cycle 3 is archived in a Zenodo repository, https://doi.org/10.5281/zenodo.10225420 (Rackow et al., 2023c). The FESOM2.5 model is also available from GitHub (https://github.com/FESOM/fesom2, FESOM2 GitHub, 2024). The ocean coupling interface to the Integrated Forecasting System (IFS) has been extracted for IFS-FESOM and is publicly available as part of the FESOM2.5 code above as well (folder ifs_interface). MultIO, MIR, ECCODES, and FDB are all free software and available at https://github.com/ecmwf (ECMWF GitHub, 2024). The IFS source code is available subject to a licence agreement with ECMWF. ECMWF member-state weather services and approved partners will be granted access. The IFS code without modules for data assimilation is also available for educational and academic purposes via an OpenIFS licence (http://www.ecmwf.int/en/research/projects/openifs, OpenIFS licence, 2024). For easier public access and review, the IFS code modifications from this study and developments detailed in Sect. 3.1.1 for nextGEMS have also been separately archived in a Zenodo repository, https://doi.org/10.5281/zenodo.10223576 (Rackow et al., 2023b). Scripts and data to reproduce the figures and analysis of this paper can be found at https://doi.org/10.5281/zenodo.13987877 (Rackow et al., 2024). GRIB data in FDB were made available to hackathon participants using gribscan (https://doi.org/10.5281/zenodo.10625189, Kölling et al., 2024).
Data for our simulations are openly accessible and can be obtained either from the web (see DOIs below), from ECMWF's MARS archive, or directly from DKRZ's supercomputer Levante after registration (https://luv.dkrz.de/register/, German Climate Computing Center (DKRZ), 2024). The Cycle 2 data for 20 January 2020 to 31 December 2020 of TCo2559-NG5 with deep convection parametrization disabled can be found at https://doi.org/10.21957/1n36-qg55 (Pedruzo-Bagazgoitia et al., 2022a). The Cycle 2 data for TCo1279-ORCA025 (20 January 2020 to 31 December 2021) with deep convection parametrization active can be found at https://doi.org/10.21957/x4vb-3b40 (Pedruzo-Bagazgoitia et al., 2022b). More Cycle 2 output, also for the nextGEMS sister model ICON, can be found at the World Data Center for Climate (WDCC), at https://doi.org/10.26050/WDCC/nextGEMS_cyc2 (Wieners et al., 2023). Cycle 3 data for ICON and IFS can be found at WDCC under https://doi.org/10.26050/WDCC/nextGEMS_cyc3 (Koldunov et al., 2023). Namelist files to reproduce the settings of the ocean, atmosphere, land, and wave model in the Cycle 3 simulations are archived in a Zenodo repository, https://doi.org/10.5281/zenodo.10221652 (Rackow et al., 2023a). Land Surface Analysis Satellite Application Facility (LSA SAF) LST data are available from the LSA SAF data service (EUMETSAT, 2024). Observed SSH AVISO data are taken from http://marine.copernicus.eu/services-portfolio/access-to-products/ (Copernicus Marine Data Store, 2024). The ocean mixed-layer climatology is taken from the SEANOE repository https://doi.org/10.17882/91774 (de Boyer Montégut, 2023).
TR led the writing of the paper and prepared the initial manuscript with TB and XPB. TR, TB, XPB, and IH performed the simulations. TB, XPB, RF, MD, and TR developed the model code changes. The refactoring of the FESOM model has been led by DmS, NK, JS, PS, and JH. Initial implementation of the IFS-FESOM single-executable coupling was the joint work of KM and TR with support from CK. NK created the 5 km nextGEMS FESOM grid NG5 in discussions with TR. IP performed the QBO analysis. TB analysed the precipitation characteristics and performed the TOA tuning. XPB contributed the 5-year temperature time series. SM performed TOA budget analyses. DT performed the MJO analyses. JB and JK contributed the wave model analyses. The city and urban heat island analyses were by XPB and ED. RG performed the SSH analyses. AK performed the mixed-layer analysis. Sea ice performance indices were the work of LZ. TR performed the sea ice lead analysis. HFG provided ocean grid descriptions for coupling weight computations. In the paper, MD and CK discussed the mass fixer approach, and RF discussed the physics parametrizations. DoS added the MultIO section to the paper. TK, LK, and FZ helped with faster data access. PM developed necessary software tools, in particular MIR. All co-authors discussed and contributed to the final document.
The contact author has declared that none of the authors has any competing interests.
Publisher’s note: Copernicus Publications remains neutral with regard to jurisdictional claims made in the text, published maps, institutional affiliations, or any other geographical representation in this paper. While Copernicus Publications makes every effort to include appropriate place names, the final responsibility lies with the authors.
This work used supercomputing resources of the German Climate Computing Centre (Deutsches Klimarechenzentrum, DKRZ) granted by its Scientific Steering Committee (WLA) under project ID 1235. We want to thank DKRZ staff for their continued support in terms of data handling, data hosting, and running of the presented Cycle 3 simulations, in particular Jan Frederik Engels, Hendryk Bockelmann, Fabian Wachsmann, Irina Fast, and Carsten Beyer. We want to thank colleagues at AWI for active discussions and their support towards the upcoming multi-decadal simulations, in particular Suvarchal Kumar Cheedela, Bimochan Niraula, and Sergey Danilov. We also would like to thank all colleagues at ECMWF who are not co-authors but also had a substantial impact on kilometre-scale model development and modelling on climate timescales, e.g. Gabriele Arduini, Gianpaolo Balsamo, Magdalena Alonso Balmaseda, Margarita Choulga, Jasper Denissen, Joe McNorton, Simon Smart, James Hawkes, Philipp Geier, Charles Pelletier, Andreas Mueller, Michael Lange, Olivier Marsden, Sam Hatfield, Matthew Griffith, Shannon Mason, and Mark Fielding. We thank Philippe Lopez for providing the Meteosat-8 observations in Fig. 6. We also want to thank the international nextGEMS hackathon community, including many early-career researchers, who analysed our simulations in detail and helped guide some of the model development efforts. This work was supported by the European Union's Destination Earth Initiative and relates to tasks entrusted by the European Union to the European Centre for Medium-Range Weather Forecasts implementing part of this initiative with funding by the European Union.
This research has been supported by the European Commission Horizon 2020 Framework Programme nextGEMS, H2020 Societal Challenges (grant no. 101003470).
The article processing charges for this open-access publication were covered by the Alfred-Wegener-Institut Helmholtz-Zentrum für Polar- und Meeresforschung.
This paper was edited by Peter Caldwell and reviewed by three anonymous referees.
Ahn, M.-S., Kim, D., Kang, D., Lee, J., Sperber, K. R., Gleckler, P. J., Jiang, X., Ham, Y.-G., and Kim, H.: MJO propagation across the Maritime Continent: Are CMIP6 models better than CMIP5 models?, Geophys. Res. Lett., 47, e2020GL087250, https://doi.org/10.1029/2020GL087250, 2020.
Arduini, G., Balsamo, G., Dutra, E., Day, J. J., Sandu, I., Boussetta, S., and Haiden, T.: Impact of a multi-layer snow scheme on near-surface weather forecasts, J. Adv. Model. Earth Sy., 11, 4687–4710, https://doi.org/10.1029/2019MS001725, 2019.
Baldwin, M. P., Gray, L. J., Dunkerton, T. J., Hamilton, K., Haynes, P. H., Randel, W. J., Holton, J. R., Alexander, M. J., Hirota, I., Horinouchi, T., Jones, D. B. A., Kinnersley, J. S., Marquardt, C., Sato, K., and Takahashi, M.: The quasi-biennial oscillation, Rev. Geophys., 39, 179–229, https://doi.org/10.1029/1999RG000073, 2001.
Bauer, P., Quintino, T., Wedi, N., Bonanni, A., Chrust, M., Deconinck, W., Diamantakis, M., Düben, P., English, S., Flemming, J., Gillies, P., Hadade, I., Hawkes, J., Hawkins, M., Iffrig, O., Kühnlein, C., Lange, M., Lean, P., Marsden, O., Müller, A., Saarinen, S., Sarmany, D., Sleigh, M., Smart, S., Smolarkiewicz, P., Thiemert, D., Tumolo, G., Weihrauch, C., Zanna, C., and Maciel, P.: The ECMWF scalability programme: Progress and plans, European Centre for Medium Range Weather Forecasts, https://doi.org/10.21957/gdit22ulm, 2020.
Bauer, P., Dueben, P. D., Hoefler, T., Quintino, T., Schulthess, T., and Wedi, N. P.: The digital revolution of Earth-system science, Nat. Comput. Sci., 1, 104–113, https://doi.org/10.1038/s43588-021-00023-0, 2021.
Bauer, P., Quintino, T., and Wedi, N.P.: From the Scalability Programme to Destination Earth, ECMWF Newsletter, 171, 15–22, https://doi.org/10.21957/pb2vnp59ks, 2022.
Bechtold, P., Köhler, M., Jung, T., Leutbecher, M., Rodwell, M., Vitart, F., and Balsamo, G.: Advances in predicting atmospheric variability with the ECMWF model: From synoptic to decadal time-scales, Q. J. Roy. Meteor. Soc., 134, 1337–1351, https://doi.org/10.1002/qj.289, 2008.
Bechtold, P., Semane, N., Lopez, P., Chaboureau, J.-P., Beljaars, A., and Bormann, N.: Representing equilibrium and non-equilibrium convection in large-scale models, J. Atmos. Sci., 134, 1337–1351, https://doi.org/10.1175/JAS-D-13-0163.1, 2014.
Becker, T., Bechtold, P., and Sandu, I.: Characteristics of convective precipitation over tropical Africa in storm-resolving global simulations, Q. J. Roy. Meteor. Soc., 147, 4388–4407, https://doi.org/10.1002/qj.4185, 2021.
Beljaars, A. C. M., Brown, A. R., and Wood, N.: A new parametrization of turbulent orographic form drag, Q. J. Roy. Meteor. Soc., 130, 1327–1347, https://doi.org/10.1256/qj.03.73, 2004.
Bengtsson, L, Dias, J., Gehne, M., Bechtold, P., Whitaker, J., Bao, J.-W., Magnusson, L., Michelson, S., Pegion, P., Tulich, S., and Kiladis, G.: Convectively coupled equatorial wave simulations using the ECMWF IFS and the NOAA GFS cumulus convection schemes in the NOAA GFS model, Mon. Weather Rev, 147, 4005–4025, https://doi.org/10.1175/MWR-D-19-0195.1, 2019.
Berthou, S., Rowell, D. P., Kendon, E. J., Roberts, M. J., Stratton, R. A., Crook, J. A., and Wilcox, C: Improved climatological precipitation characteristics over West Africa at convection-permitting scales, Clim. Dynam., 53, 1991–2011, https://doi.org/10.1007/s00382-019-04759-4, 2019.
Bony, S., Stevens, B., Frierson, D. M. W., Jakob, C., Kageyama, M., Pincus, R., Shepherd, T. G., Sherwood, S. C., Siebesma, A. P., Sobel, A. H., Watanabe, M., and Webb, M. J.: Clouds, circulation and climate sensitivity, Nat. Geosci., 8, 261–268, https://doi.org/10.1038/ngeo2398, 2015.
Boussetta, S., Balsamo, G., Arduini, G., Dutra, E., McNorton, J., Choulga, M., Agustí-Panareda, A., Beljaars, A., Wedi, N., Munõz-Sabater, J., de Rosnay, P, Sandu, I., Hadade, I., Carver, G., Mazzetti, C., Prudhomme, C., Yamazaki, D., and Zsoter, E.: ECLand: The ECMWF Land Surface Modelling System, Atmosphere, 12, 723, https://doi.org/10.3390/atmos12060723, 2021.
Bozzo, A., Benedetti, A., Flemming, J., Kipling, Z., and Rémy, S.: An aerosol climatology for global models based on the tropospheric aerosol scheme in the Integrated Forecasting System of ECMWF, Geosci. Model Dev., 13, 1007–1034, https://doi.org/10.5194/gmd-13-1007-2020, 2020.
Bryan, F. O., Gent, P. R., and Tomas, R.: Can Southern Ocean Eddy Effects Be Parameterized in Climate Models?, J. Climate, 27, 411–425, https://doi.org/10.1175/JCLI-D-12-00759.1, 2014.
Bushell, A. C., Anstey, J. A., Butchart, N., Kawatani, Y., Osprey, S. M., Richter, J. H., Serva, F., Braesicke, P., Cagnazzo, C., Chen, C.-C., Chun, H.-.-Y., Garcia, R. R., Gray, L. J., Hamilton, K., Kerzenmacher, T., Kim, Y.-.-H., Lott, F., McLandress, C., Naoe, H., Scinocca, J., Smith, A. K., Stockdale, T. N., Versick, S., Watanabe, S., Yoshida, K., and Yukimoto, S.: Evaluation of the Quasi-Biennial Oscillation in global climate models for the SPARC QBO-initiative, Q. J. Roy. Meteor. Soc., 148, 1459–1489, https://doi.org/10.1002/qj.3765, 2022.
Cao, B., Arduini, G., and Zsoter, E.: Brief communication: Improving ERA5-Land soil temperature in permafrost regions using an optimized multi-layer snow scheme, The Cryosphere, 16, 2701–2708, https://doi.org/10.5194/tc-16-2701-2022, 2022.
Chen, G., Ling, J., Zhang, R., Xiao, Z., and Li, C.: The MJO from CMIP5 to CMIP6: Perspectives from tracking MJO precipitation, Geophys. Res. Lett., 49, e2021GL095241, https://doi.org/10.1029/2021GL095241, 2022.
Copernicus Marine Data Store: Overview of products, http://marine.copernicus.eu/services-portfolio/access-to-products/, last access: 7 November 2024.
Crook, J., Klein, C., Folwell, S., Taylor, C. M., Parker, D. J., Stratton, R., and Stein, T.: Assessment of the representation of West African storm lifecycles in convection-permitting simulations, Earth Space Sci., 6, 818–835, https://doi.org/10.1029/2018EA000491, 2019.
Dai, A., Qian, T., Trenberth, K. E., and Milliman, J. D.: Changes in Continental Freshwater Discharge from 1948 to 2004, J. Climate, 22, 2773–2792, https://doi.org/10.1175/2008JCLI2592.1, 2009.
Danilov, S., Sidorenko, D., Wang, Q., and Jung, T.: The Finite-volumE Sea ice–Ocean Model (FESOM2), Geosci. Model Dev., 10, 765–789, https://doi.org/10.5194/gmd-10-765-2017, 2017.
de Boyer Montégut, C., Madec, G., Fischer, A. S., Lazar, A., and Iudicone, D.: Mixed layer depth over the global ocean: An examination of profile data and a profile-based climatology, J. Geophys. Res.-Oceans, 109 1–20, https://doi.org/10.1029/2004JC002378, 2004.
de Boyer Montégut, C.: Mixed layer depth climatology computed with a density threshold criterion of 0.03kg/m3 from 10 m depth value, SEANOE [data set], https://doi.org/10.17882/91774, 2023.
Dee, D. P., Uppala, S. M., Simmons, A. J., Berrisford, P., Poli, P., Kobayashi, S., Andrae, U., Balmaseda, M. A., Balsamo, G., Bauer, P., Bechtold, P., Beljaars, A. C. M., van de Berg, L., Bidlot, J., Bormann, N., Delsol, C., Dragani, R., Fuentes, M., Geer, A. J., Haimberger, L., Healy, S. B., Hersbach, H., Hólm, E. V., Isaksen, L., Kållberg, P., Köhler, M., Matricardi, M., McNally, A. P., Monge-Sanz, B. M., Morcrette, J.-J., Park, B.-K., Peubey, C., de Rosnay, P., Tavolato, C., Thépaut, J.-N., and Vitart, F.: The ERA-Interim reanalysis: Configuration and performance of the data assimilation system, Q. J. Roy. Meteor. Soc., 137, 553–597, https://doi.org/10.1002/qj.828, 2011.
Diamantakis, M. and Agusti-Panareda, A.: A positive definite tracer mass fixer for high resolution weather and atmospheric composition forecasts, ECMWF Technical Memoranda, 819, https://doi.org/10.21957/qpogzoy, 2017.
Diamantakis, M. and Flemming, J.: Global mass fixer algorithms for conservative tracer transport in the ECMWF model, Geosci. Model Dev., 7, 965–979, https://doi.org/10.5194/gmd-7-965-2014, 2014.
Diamantakis, M. and Váňa, F.: A fast converging and concise algorithm for computing the departure points in semi-Lagrangian weather and climate models, Q. J. Roy. Meteor. Soc., 148, 670–684, https://doi.org/10.1002/qj.4224, 2021.
Dias, J., Gehne, M., Kiladis, G. N., Sakaeda, N., Bechtold, P., and Haiden, T.: Equatorial waves and the skill of NCEP and ECMWF forecast systems, Mon. Weather Rev., 146, 1763–1784, https://doi.org/10.1175/MWR-D-17-0362.1, 2018.
ECMWF: ECMWF IFS Documentation CY48R1 – Part III: Dynamics and Numerical Procedures, IFS Documentation CY48R1, https://doi.org/10.21957/26f0ad3473, 2023a.
ECMWF: ECMWF IFS documentation CY48R1 – Part IV Physical processes, IFS Documentation CY48R1, https://doi.org/10.21957/02054f0fbf, 2023b.
ECMWF: ECMWF IFS Documentation CY48R1 – Part VII: ECMWF Wave Model, IFS Documentation CY48R1, https://doi.org/10.21957/cd1936d846, 2023c.
ECMWF GitHub: Software to work with meteorological data and services, https://github.com/ecmwf, last access: 7 November 2024.
ECMWF News Item: nextGEMS probes km-scale resolutions in the Integrated Forecasting System, https://www.ecmwf.int/en/about/media-centre/news/2022/nextgems-probes-km-scale-resolutions-integrated-forecasting-system/ (last access: 4 January 2024), October 2022.
ECMWF Newsletter 172: Fixing water and energy budget imbalances in the Integrated Forecasting System, https://www.ecmwf.int/en/newsletter/172/ news/fixing-water-and-energy-budget-imbalances-integrated-forecasting-system/ (last access: 4 January 2024), Summer 2022.
Engwirda, D.: JIGSAW-GEO (1.0): locally orthogonal staggered unstructured grid generation for general circulation modelling on the sphere, Geosci. Model Dev., 10, 2117–2140, https://doi.org/10.5194/gmd-10-2117-2017, 2017.
EUMETSAT: Land Surface Analysis Satellite Application Facility (LSA SAF) data service, https://datalsasaf.lsasvcs.ipma.pt/PRODUCTS/MSG/MLST/, last access: 7 November 2024.
Faroux, S., Kaptué Tchuenté, A. T., Roujean, J.-L., Masson, V., Martin, E., and Le Moigne, P.: ECOCLIMAP-II/Europe: a twofold database of ecosystems and surface parameters at 1 km resolution based on satellite information for use in land surface, meteorological and climate models, Geosci. Model Dev., 6, 563–582, https://doi.org/10.5194/gmd-6-563-2013, 2013.
FESOM2 GitHub: The Finite Element Sea Ice-Ocean Model (FESOM2), https://github.com/FESOM/fesom2, last access: 7 November 2024.
Fielding, M. D., Schäfer, S. A. K., Hogan, R. J., and Forbes, R. M.: Parametrizing cloud geometry and its application in a subgrid cloud-edge erosion scheme, Q. J. Roy. Meteor. Soc., 146, 1651–1667, https://doi.org/10.1002/qj.3758, 2020.
Forbes, R. M. and Ahlgrimm, M.: On the Representation of High-Latitude Boundary Layer Mixed-Phase Cloud in the ECMWF Global Model, Mon. Weather Rev., 142, 3425–3445, https://doi.org/10.1175/MWR-D-13-00325.1, 2014.
Forbes, R. M., Tompkins, A. M., and Untch, A.: A new prognostic bulk microphysics scheme for the IFS, ECMWF Technical Memoranda, No. 649, https://doi.org/10.21957/bf6vjvxk, 2011.
Frenger, I., Gruber, N., Knutti, R., and Münnich, M.: Imprint of Southern Ocean eddies on winds, clouds and rainfall, Nat. Geosci., 6, 608–612, https://doi.org/10.1038/ngeo1863, 2013.
Gao, K., Harris, L., Bender, M., Chen, J.-H., Zhou, L., and Knutson, T.: Regulating fine-scale resolved convection in high-resolution models for better hurricane track prediction, Geophys. Res. Lett., 50, e2023GL103329, https://doi.org/10.1029/2023GL103329, 2023.
Garfinkel, C. I., Gerber, E. P., Shamir, O., Rao, J., Jucker, M., White, I., and Paldor, N.: A QBO cookbook: Sensitivity of the quasi-biennial oscillation to resolution, resolved waves, and parameterized gravity waves, J. Adv. Model. Earth Sy., 14, e2021MS002568, https://doi.org/10.1029/2021MS002568, 2022.
German Climate Computing Center (DKRZ): Registration on Levante supercomputer, https://luv.dkrz.de/register/, last access: 7 November 2024.
Goessling, H. F., Tietsche, S., Day, J. J., Hawkins, E., and Jung, T.: Predictability of the Arctic sea ice edge, Geophys. Res. Lett., 43, 1642–1650, https://doi.org/10.1002/2015GL067232, 2016.
Griffies, S. M., Winton, M., Anderson, W. G., Benson, R., Delworth, T. L., Dufour, C. O., Dunne, J. P., Goddard, P., Morrison, A. K., Rosati, A., Wittenberg, A.T., Yin, J., and Zhang, R.: Impacts on Ocean Heat from Transient Mesoscale Eddies in a Hierarchy of Climate Models, J. Climate, 28, 952–977, https://doi.org/10.1175/JCLI-D-14-00353.1, 2015.
Gutjahr, O., Jungclaus, J. H., Brüggemann, N., Haak, H., and Marotzke, J.: Air-sea interactions and water mass transformation during a katabatic storm in the Irminger Sea, J. Geophys. Res.-Oceans, 127, e2021JC018075, https://doi.org/10.1029/2021JC018075, 2022.
Hallberg, R.: Using a resolution function to regulate parameterizations of oceanic mesoscale eddy effects, Ocean Model., 72, 92–103, https://doi.org/10.1016/j.ocemod.2013.08.007, 2013.
Hannah, W. M. and Maloney, E. D.: The role of moisture–convection feedbacks in simulating the Madden–Julian oscillation, J. Climate, 24, 2754–2770, https://doi.org/10.1175/2011JCLI3803.1, 2011.
Hersbach, H., Bell, B., Berrisford, P., Hirahara, S., Horányi, A., Muñoz-Sabater, J., Nicolas, J., Peubey, C., Radu, R., Schepers, D., Simmons, A., Soci, C., Abdalla, S., Abellan, X., Balsamo, G., Bechtold, P., Biavati, G., Bidlot, J., Bonavita, M., De Chiara, G., Dahlgren, P., Dee, D., Diamantakis, M., Dragani, R., Flemming, J., Forbes, R., Fuentes, M., Geer, A., Haimberger, L., Healy, S., Hogan, R. J., Hólm, E., Janisková, M., Keeley, S., Laloyaux, P., Lopez, P., Lupu, C., Radnoti, G., de Rosnay, P., Rozum, I., Vamborg, F., Villaume, S., and Thépaut, J.-N.: The ERA5 global reanalysis, Q. J. Roy. Meteor. Soc., 146, 1999–2049, https://doi.org/10.1002/qj.3803, 2020.
Hewitt, H., Fox-Kemper, B., Pearson, B. Roberts, M., and Klocke, D.: The small scales of the ocean may hold the key to surprises, Nat. Clim. Change, 12, 496–499, https://doi.org/10.1038/s41558-022-01386-6, 2022.
Hogan, R. J. and Bozzo, A.: A flexible and efficient radiation scheme for the ECMWF model, J. Adv. Model. Earth Sy., 10, 1990–2008, https://doi.org/10.1029/2018MS001364, 2018.
Hogg, A. McC., Meredith, M. P., Chambers, D. P., Abrahamsen, E. P., Hughes, C. W., and Morrison, A. K.: Recent trends in the Southern Ocean eddy field, J. Geophys. Res.-Oceans, 120, 57–267, https://doi.org/10.1002/2014JC010470, 2015.
Hohenegger, C., Korn, P., Linardakis, L., Redler, R., Schnur, R., Adamidis, P., Bao, J., Bastin, S., Behravesh, M., Bergemann, M., Biercamp, J., Bockelmann, H., Brokopf, R., Brüggemann, N., Casaroli, L., Chegini, F., Datseris, G., Esch, M., George, G., Giorgetta, M., Gutjahr, O., Haak, H., Hanke, M., Ilyina, T., Jahns, T., Jungclaus, J., Kern, M., Klocke, D., Kluft, L., Kölling, T., Kornblueh, L., Kosukhin, S., Kroll, C., Lee, J., Mauritsen, T., Mehlmann, C., Mieslinger, T., Naumann, A. K., Paccini, L., Peinado, A., Praturi, D. S., Putrasahan, D., Rast, S., Riddick, T., Roeber, N., Schmidt, H., Schulzweida, U., Schütte, F., Segura, H., Shevchenko, R., Singh, V., Specht, M., Stephan, C. C., von Storch, J.-S., Vogel, R., Wengel, C., Winkler, M., Ziemen, F., Marotzke, J., and Stevens, B.: ICON-Sapphire: simulating the components of the Earth system and their interactions at kilometer and subkilometer scales, Geosci. Model Dev., 16, 779–811, https://doi.org/10.5194/gmd-16-779-2023, 2023.
Hortal, M.: The development and testing of a new two-time-level semi-Lagrangian scheme (SETTLS) in the ECMWF forecast model, Q. J. Roy. Meteor. Soc., 128, 1671–1687, https://doi.org/10.1002/qj.200212858314, 2002.
Hutter, N., Bouchat, A., Dupont, F., Dukhovskoy, D., Koldunov, N., Lee, Y. J., Lemieux, J.-F., Lique, C., Losch, M., Maslowski, W., Myers, P. G., Ólason, E., Rampal, P., Rasmussen, T., Talandier, C., Tremblay, B., and Wang, Q.: Sea Ice Rheology Experiment (SIREx): 2. Evaluating linear kinematic features in high-resolution sea ice simulations, J. Geophys. Res.-Oceans, 127, e2021JC017666, https://doi.org/10.1029/2021JC017666, 2022.
Johnson, S. J., Stockdale, T. N., Ferranti, L., Balmaseda, M. A., Molteni, F., Magnusson, L., Tietsche, S., Decremer, D., Weisheimer, A., Balsamo, G., Keeley, S. P. E., Mogensen, K., Zuo, H., and Monge-Sanz, B. M.: SEAS5: the new ECMWF seasonal forecast system, Geosci. Model Dev., 12, 1087–1117, https://doi.org/10.5194/gmd-12-1087-2019, 2019.
Jones, P. W.: First- and second-order conservative remapping schemes for grids in spherical coordinates, Mon. Weather Rev., 127, 2204–2210, https://doi.org/10.1175/1520-0493(1999)127<2204:FASOCR>2.0.CO;2, 1999.
Judt, F. and Rios-Berrios, R: Resolved convection improves the representation of equatorial waves and tropical rainfall variability in a global nonhydrostatic model, Geophys. Res. Lett., 48, e2021GL093265, https://doi.org/10.1029/2021GL093265, 2021.
Judt, F., Klocke, D., Rios-Berrios, R. Vanniere, B., Ziemen, F., Auger, L., Biercamp, J., Bretherton, C., Chen, X., Düben, P., Hohenegger, C., Khairoutdinov, M., Kodama, C., Kornblueh, L., Lin, S.-J., Nakano, M., Neumann, P., Putman, W., Röber, N., Roberts, M., Satoh, M., Shibuya, R., Stevens, B., Vidale, P. L., Wedi, N., and Zhou, L.: Tropical Cyclones in Global Storm-Resolving Models, J. Meteorol. Soc. Jpn. Ser. II, 99, 579–602, https://doi.org/10.2151/jmsj.2021-029, 2021.
Jung, T., Miller, M. J., Palmer, T. N., Towers, P., Wedi, N., Achuthavarier, D., Adams, J. M., Altshuler, E. L., Cash, B. A., Kinter III, J. L., Marx, L., Stan, C., and Hodges, K. I.: High-Resolution Global Climate Simulations with the ECMWF Model in Project Athena: Experimental Design, Model Climate, and Seasonal Forecast Skill, J. Climate, 25, 3155–3172, https://doi.org/10.1175/JCLI-D-11-00265.1, 2012.
Keeley, S. P. E., Sutton, R. T., and Shaffrey, L. C.: The impact of North Atlantic sea surface temperature errors on the simulation of North Atlantic European region climate, Q. J. Roy. Meteor. Soc., 138, 1774–1783, https://doi.org/10.1002/qj.1912, 2012.
Kluyver, T., Ragan-Kelley, B., Pérez, F., Granger, B., Bussonnier, M., Frederic, J., Kelley, K., Hamrick, J., Grout, J., Corlay, S., Ivanov, P., Avila, D., Abdalla, S., Willing, C., and Jupyter development team: Jupyter notebooks – a publishing format for reproducible computational workflows, in: Positioning and Power in Academic Publishing: Players, Agents and Agendas, edited by: Loizides, F. and Schmidt, B., IOS Press, 87–90, https://doi.org/10.3233/978-1-61499-649-1-87, 2016.
Kodama, C., Yamada, Y., Noda, A. T., Kikuchi, K., Kajikawa, Y., Nasuno, T., Tomita, T., Yamaura, T., Takahashi, H. G., Hara, M., Kawatani, Y., Satoh, M., and Sugi, M.: A 20-year climatology of a NICAM AMIP-type simulation, J. Meteorol. Soc. Jpn. Ser. II, 93, 393–424, https://doi.org/10.2151/jmsj.2015-024, 2015.
Kodama, C., Ohno, T., Seiki, T., Yashiro, H., Noda, A. T., Nakano, M., Yamada, Y., Roh, W., Satoh, M., Nitta, T., Goto, D., Miura, H., Nasuno, T., Miyakawa, T., Chen, Y.-W., and Sugi, M.: The Nonhydrostatic ICosahedral Atmospheric Model for CMIP6 HighResMIP simulations (NICAM16-S): experimental design, model description, and impacts of model updates, Geosci. Model Dev., 14, 795–820, https://doi.org/10.5194/gmd-14-795-2021, 2021.
Köhler, M., Ahlgrimm, M. and Beljaars, A.: Unified treatment of dry convective and stratocumulus-topped boundary layers in the ECMWF model, Q. J. Roy. Meteor. Soc., 137, 43–57, https://doi.org/10.1002/qj.713, 2011.
Koldunov, N. V., Aizinger, V., Rakowsky, N., Scholz, P., Sidorenko, D., Danilov, S., and Jung, T.: Scalability and some optimization of the Finite-volumE Sea ice–Ocean Model, Version 2.0 (FESOM2), Geosci. Model Dev., 12, 3991–4012, https://doi.org/10.5194/gmd-12-3991-2019, 2019.
Koldunov, N., Kölling, T., Pedruzo-Bagazgoitia, X., Rackow, T., Redler, R., Sidorenko, D., Wieners, K.-H., Ziemen, F. A.: nextGEMS: output of the model development cycle 3 simulations for ICON and IFS, World Data Center for Climate (WDCC) at DKRZ [data set], https://doi.org/10.26050/WDCC/nextGEMS_cyc3, 2023.
Kölling, T., Kluft, L., and Rackow, T.: gribscan (v0.0.10), Zenodo [code], https://doi.org/10.5281/zenodo.10625189, 2024.
Large, W. G. and Yeager, S. G.: The global climatology of an interannually varying air–sea flux data set, Clim. Dynam., 33, 341–364, https://doi.org/10.1007/s00382-008-0441-3, 2009.
Liebmann, B. and Smith, C. A.: Description of a Complete (Interpolated) Outgoing Longwave Radiation Dataset, B. Am. Meteorol. Soc., 77, 1275–1277, 1996.
Ling, J., Zhao, Y., and Chen, G.: Barrier effect on MJO propagation by the Maritime Continent in the MJO Task Force/GEWEX atmospheric system study models, J. Climate, 32, 5529–5547, https://doi.org/10.1175/JCLI-D-18-0870.1, 2019.
Loeb, N. G., Doelling, D. R., Wang, H., Su, W., Nguyen, C., Corbett, J. G., Liang, L., Mitrescu, C., Rose, F. G., and Kato, S.: Clouds and the Earth's Radiant Energy System (CERES) Energy Balanced and Filled (EBAF) Top-of-Atmosphere (TOA) Edition-4.0 Data Product, J. Climate, 31, 895–918, https://doi.org/10.1175/JCLI-D-17-0208.1, 2018.
Loveland, T. R., Reed, B. C., Brown, J. F., Ohlen, D. O., Zhu, Z., Youing, L., and Merchant, J. W.: Development of a global land cover characteristics database and IGB6 DISCover from the 1 km AVHRR data, Int. J. Remote Sens., 21, 1303–1330, https://doi.org/10.1080/014311600210191, 2000.
Lott, F. and Miller, M. J.: A new subgrid-scale orographic drag parametrization: Its formulation and testing, Q. J. Roy. Meteor. Soc., 123, 101–127, https://doi.org/10.1002/qj.49712353704, 1997.
Lüpkes, C., Vihma, T., Birnbaum, G., and Wacker, U.: Influence of leads in sea ice on the temperature of the atmospheric boundary layer during polar night, Geophys. Res. Lett., 35, L03805, https://doi.org/10.1029/2007GL032461, 2008.
Macdonald, R. W., Griffiths, R. F., and Hall, D. J.: An improved method for the estimation of surface roughness of obstacle arrays, Atmos. Environ., 32, 1857–1864, https://doi.org/10.1016/s1352-2310(97)00403-2, 1998.
Madden, R. A. and Julian, P. R.: Description of global-scale circulation cells in the tropics with a 40–50 day period, J. Atmos. Sci., 29, 1109–1123, https://doi.org/10.1175/1520-0469(1972)029<1109:DOGSCC>2.0.CO;2, 1972.
Malardel, S., Wedi, N., Deconinck, W., Diamantakis, M., Kuehnlein, C., Mozdzynski, G., Hamrud, M., and Smolarkiewicz, P.: A new grid for the IFS, ECMWF Newsletter, 146, 23–28, https://doi.org/10.21957/zwdu9u5i, 2016.
Marti, O., Nguyen, S., Braconnot, P., Valcke, S., Lemarié, F., and Blayo, E.: A Schwarz iterative method to evaluate ocean–atmosphere coupling schemes: implementation and diagnostics in IPSL-CM6-SW-VLR, Geosci. Model Dev., 14, 2959–2975, https://doi.org/10.5194/gmd-14-2959-2021, 2021.
McNorton, J. R., Arduini, G., Bousserez, N., Agustí-Panareda, A., Balsamo, G., Boussetta, S., Choulga, M., Hadade, I., and Hogan, R. J.: An urban scheme for the ECMWF integrated forecasting system: Single-column and global offline application, J. Adv. Model. Earth Sy., 13, e2020MS002375, https://doi.org/10.1029/2020MS002375, 2021.
McNorton, J. R., Agustí-Panareda, A., Arduini, G., Balsamo, G., Bousserez, N., Boussetta, S., Chericoni, M., Choulga, M., Engelen, R., and Guevara, M.: An urban scheme for the ECMWF Integrated forecasting system: Global forecasts and residential CO2 emissions, J. Adv. Model. Earth Sy., 15, e2022MS003286, https://doi.org/10.1029/2022MS003286, 2023.
Miura, H., Satoh, M., Nasuno, T., Noda, A. T., and Oouchi, K.: A Madden-Julian oscillation event realistically simulated by a global cloud-resolving model, Science, 318, 1763–1765, https://doi.org/10.1126/science.1148443, 2007.
Miyakawa, T., Yashiro, H., Suzuki, T., Tatebe, H., and Satoh, M.: A Madden-Julian Oscillation event remotely accelerates ocean upwelling to abruptly terminate the 1997/1998 super El Niño, Geophys. Res. Lett., 44, 9489–9495, https://doi.org/10.1002/2017GL074683, 2017.
Mogensen, K. S., Keeley, S., and Towers, P.: Coupling of the NEMO and IFS models in a single executable, ECMWF Technical Memoranda, 673, https://doi.org/10.21957/rfplwzuol, 2012.
Mogensen, K. S., Magnusson, L., and Bidlot, J.-R.: Tropical cyclone sensitivity to ocean coupling in the ECMWF coupled model, J. Geophys. Res.-Oceans, 122, 4392–4412, https://doi.org/10.1002/2017JC012753, 2017.
Morrison, A. K., Hogg, A. McC., England, M. H., and Spence, P.: Warm Circumpolar Deep Water transport toward Antarctica driven by local dense water export in canyons, Sci. Adv., 6, 85–101, https://doi.org/10.1126/sciadv.aav2516, 2020.
Mu, L., Nerger, L., Tang, Q., Loza, S. N., Sidorenko, D., Wang, Q., Semmler, T., Zampieri, L., Losch, M., and Goessling, H. F.: Toward a data assimilation system for seamless sea ice prediction based on the AWI Climate Model, J. Adv. Model. Earth Sy., 12, e2019MS001937, https://doi.org/10.1029/2019MS001937, 2020.
Mu, L., Nerger, L., Streffing, J., Tang, Q., Niraula, B., Zampieri, L., Loza, S. L., and Goessling, H. F.: Sea-ice forecasts with an upgraded AWI Coupled Prediction System, J. Adv. Model. Earth Sy., 14, e2022MS003176, https://doi.org/10.1029/2022MS003176, 2022.
Müller, A., Deconinck, W., Kühnlein, C., Mengaldo, G., Lange, M., Wedi, N., Bauer, P., Smolarkiewicz, P. K., Diamantakis, M., Lock, S.-J., Hamrud, M., Saarinen, S., Mozdzynski, G., Thiemert, D., Glinton, M., Bénard, P., Voitus, F., Colavolpe, C., Marguinaud, P., Zheng, Y., Van Bever, J., Degrauwe, D., Smet, G., Termonia, P., Nielsen, K. P., Sass, B. H., Poulsen, J. W., Berg, P., Osuna, C., Fuhrer, O., Clement, V., Baldauf, M., Gillard, M., Szmelter, J., O'Brien, E., McKinstry, A., Robinson, O., Shukla, P., Lysaght, M., Kulczewski, M., Ciznicki, M., Piątek, W., Ciesielski, S., Błażewicz, M., Kurowski, K., Procyk, M., Spychala, P., Bosak, B., Piotrowski, Z. P., Wyszogrodzki, A., Raffin, E., Mazauric, C., Guibert, D., Douriez, L., Vigouroux, X., Gray, A., Messmer, P., Macfaden, A. J., and New, N.: The ESCAPE project: Energy-efficient Scalable Algorithms for Weather Prediction at Exascale, Geosci. Model Dev., 12, 4425–4441, https://doi.org/10.5194/gmd-12-4425-2019, 2019.
Nogueira, M., Boussetta, S., Balsamo, G., Albergel, C., Trigo, I. F., Johannsen, F., Miralles, D. G., and Dutra, E.: Upgrading land-cover and vegetation seasonality in the ECMWF coupled system: Verification with FLUXNET sites, METEOSAT satellite land surface temperatures, and ERA5 atmospheric reanalysis, J. Geophys. Res.-Atmos., 126, e2020JD034163, https://doi.org/10.1029/2020JD034163, 2021.
OpenIFS licence: Overview, Objectives, and Further Information, http://www.ecmwf.int/en/research/projects/openifs, last access: 7 November 2024.
Orr, A., Bechtold, P., Scinocca, J. F., Ern, M., and Janiskova, M.: Improved middle atmosphere climate and forecasts in the ECMWF model through a non-orographic gravity wave drag parametrization, J. Climate, 23, 5905–5926, https://doi.org/10.1175/2010JCLI3490.1, 2010.
OSI SAF: Global Sea Ice Concentration Climate Data Record v3.0 – Multimission, EUMETSAT SAF on Ocean and Sea Ice [data set], https://doi.org/10.15770/EUM_SAF_OSI_0013, 2022.
Overland, J. E., Curtin, T. B., and Smith Jr., W. O.: Preface [to special section on Leads and Polynyas], J. Geophys. Res., 100, 4267–4268, https://doi.org/10.1029/95JC00336, 1995.
Palmer, T.: Climate forecasting: Build high-resolution global climate models, Nature, 515, 338–339, https://doi.org/10.1038/515338a, 2014.
Palmer, T. and Stevens, B.: The scientific challenge of understanding and estimating climate change, P. Natl. Acad. Sci. USA, 116, 24390–24395, https://doi.org/10.1073/pnas.1906691116, 2019.
Paul, M. J. and Meyer, J. L.: Streams in the urban landscape, Annu. Rev. Ecol. Syst., 32, 333–365, https://doi.org/10.1146/annurev.ecolsys.32.081501.114040, 2001.
Pedruzo-Bagazgoitia, X., Rackow, T., and Hadade, I.: IFS-FESOM nextGEMS Cycle 2 4.4 km 1-year simulation, ECMWF [data set], https://doi.org/10.21957/1n36-qg55, 2022a.
Pedruzo-Bagazgoitia, X., Rackow, T., and Hadade, I.: IFS-NEMO nextGEMS Cycle 2 9 km baseline 2-year simulation, ECMWF [data set], https://doi.org/10.21957/x4vb-3b40, 2022b.
Polichtchouk, I., Wedi, N., and Kim, Y.-H.: Resolved gravity waves in the tropical stratosphere: Impact of horizontal resolution and deep convection parametrization, Q. J. Roy. Meteor. Soc., 148, 233–251, https://doi.org/10.1002/qj.4202, 2021.
Prein, A. F., Langhans, W., Fosser, G., Ferrone, A., Ban, N., Goergen, K., Keller, M., Tölle, M., Gutjahr, O., Feser, F., Brisson, E., Kollet, S., Schmidli, J., van Lipzig, N. P. M., and Leung, R.: A review on regional convection-permitting climate modeling: Demonstrations, prospects, and challenges, Rev. Geophys., 53, 323–361, https://doi.org/10.1002/2014RG000475, 2015.
Pujol, M.-I., Faugère, Y., Taburet, G., Dupuy, S., Pelloquin, C., Ablain, M., and Picot, N.: DUACS DT2014: the new multi-mission altimeter data set reprocessed over 20 years, Ocean Sci., 12, 1067–1090, https://doi.org/10.5194/os-12-1067-2016, 2016.
Rackow, T., Sein, D. V., Semmler, T., Danilov, S., Koldunov, N. V., Sidorenko, D., Wang, Q., and Jung, T.: Sensitivity of deep ocean biases to horizontal resolution in prototype CMIP6 simulations with AWI-CM1.0, Geosci. Model Dev., 12, 2635–2656, https://doi.org/10.5194/gmd-12-2635-2019, 2019.
Rackow, T., Danilov, S., Goessling, H. F., Hellmer, H. H., Sein, D. V., Semmler, T., Sidorenko, D., and Jung, T.: Delayed Antarctic sea-ice decline in high-resolution climate change simulations, Nat. Commun., 13, 637, https://doi.org/10.1038/s41467-022-28259-y, 2022.
Rackow, T., Pedruzo-Bagazgoitia, X., and Becker, T.: Namelist files and settings for multi-year km-scale nextGEMS Cycle 3 simulations with IFS-FESOM/NEMO, Zenodo [data set], https://doi.org/10.5281/zenodo.10221652, 2023a.
Rackow, T., Becker, T., Forbes, R., and Fielding, M.: Source code changes to the Integrated Forecasting System (IFS) for nextGEMS simulations, Zenodo [code], https://doi.org/10.5281/zenodo.10223577, 2023b.
Rackow, T., Hegewald, J., Koldunov, N. V., Mogensen, K., Scholz, P., Sidorenko, D., and Streffing, J.: FESOM2.5 source code used in nextGEMS Cycle 3 simulations with IFS-FESOM, Zenodo [code], https://doi.org/10.5281/zenodo.10225420, 2023c.
Rackow, T., Kousal, J., Pedruzo-Bagazgoitia, X., and Zampieri, L.: trackow/nextGEMS-paper: Jupyter notebooks to reproduce the main figures of the nextGEMS overview paper, Zenodo [code], https://doi.org/10.5281/zenodo.13987877, 2024.
Randall, D. A. and Emanuel, K: The Weather–Climate Schism, B. Am. Meteorol. Soc., 105, E300–E305, https://doi.org/10.1175/BAMS-D-23-0124.1, 2024.
Saavedra Garfias, P., Kalesse-Los, H., von Albedyll, L., Griesche, H., and Spreen, G.: Asymmetries in cloud microphysical properties ascribed to sea ice leads via water vapour transport in the central Arctic, Atmos. Chem. Phys., 23, 14521–14546, https://doi.org/10.5194/acp-23-14521-2023, 2023.
Sármány, D., Valentini, M., Maciel, P., Geier, P., Smart, S., Aguridan, R., Hawkes, J., and Quintino, T.: MultIO: A Framework for Message-Driven Data Routing For Weather and Climate Simulations, in: Proceedings of the Platform for Advanced Scientific Computing Conference (PASC '24), Association for Computing Machinery, New York, NY, USA, Article 24, 1–12, https://doi.org/10.1145/3659914.3659938, 2024.
Satoh, M., Stevens, B., Judt, F., Khairoutdinov, M., Lin, S.-J., Putman, W. M., and Düben, P.: Global Cloud-Resolving Models, Curr. Clim. Change Rep., 5, 172–184, https://doi.org/10.1007/s40641-019-00131-0, 2019.
Scaife, A. A., Baldwin, M. P., Butler, A. H., Charlton-Perez, A. J., Domeisen, D. I. V., Garfinkel, C. I., Hardiman, S. C., Haynes, P., Karpechko, A. Y., Lim, E.-P., Noguchi, S., Perlwitz, J., Polvani, L., Richter, J. H., Scinocca, J., Sigmond, M., Shepherd, T. G., Son, S.-W., and Thompson, D. W. J.: Long-range prediction and the stratosphere, Atmos. Chem. Phys., 22, 2601–2623, https://doi.org/10.5194/acp-22-2601-2022, 2022.
Schär, C., Fuhrer, O., Arteaga, A., Ban, N., Charpilloz, C., Di Girolamo, S., Hentgen, L., Hoefler, T., Lapillonne, X., Leutwyler, D., Osterried, K., Panosetti, D., Rüdisühli, S., Schlemmer, L., Schulthess, T. C., Sprenger, M., Ubbiali, S., and Wernli, H.: Kilometer-Scale Climate Models: Prospects and Challenges, B. Am. Meteorol. Soc., 101, E567–E587, https://doi.org/10.1175/BAMS-D-18-0167.1, 2020.
Scholz, P., Sidorenko, D., Gurses, O., Danilov, S., Koldunov, N., Wang, Q., Sein, D., Smolentseva, M., Rakowsky, N., and Jung, T.: Assessment of the Finite-volumE Sea ice-Ocean Model (FESOM2.0) – Part 1: Description of selected key model elements and comparison to its predecessor version, Geosci. Model Dev., 12, 4875–4899, https://doi.org/10.5194/gmd-12-4875-2019, 2019.
Schulthess, T. C., Bauer, P., Wedi, N., Fuhrer, O., Hoefler, T., and Schär, C.: Reflecting on the goal and baseline for exascale computing: A roadmap based on weather and climate simulations, Comput. Sci. Eng., 21, 30–41, https://doi.org/10.1109/MCSE.2018.2888788, 2019.
Sein, D. V., Koldunov, N. V., Danilov, S., Wang, Q., Sidorenko, D., Fast, I., Rackow, T., Cabos, W., and Jung, T.: Ocean modeling on a mesh with resolution following the local Rossby radius, J. Adv. Model. Earth Sy., 9, 2601–2614, https://doi.org/10.1002/2017MS001099, 2017.
Selivanova, J., Iovino, D., and Cocetta, F.: Past and future of the Arctic sea ice in High-Resolution Model Intercomparison Project (HighResMIP) climate models, The Cryosphere, 18, 2739–2763, https://doi.org/10.5194/tc-18-2739-2024, 2024.
Sidorenko, D., Goessling, H. F., Koldunov, N. V., Scholz, P., Danilov, S., Barbi, D., Cabos, W., Gurses, O., Harig, S., Hinrichs, C., Juricke, S., Lohmann, G., Losch, M., Mu, L., Rackow, T., Rakowsky, N., Sein, D., Semmler, T., Shi, X., Stepanek, C., Streffing, J., Wang, Q., Wekerle, C., Yang, H., and Jung, T.: Evaluation of FESOM2.0 coupled to ECHAM6.3: Pre-industrial and HighResMIP simulations, J. Adv. Model. Earth Sy., 11, 3794–3815, https://doi.org/10.1029/2019MS001696, 2019.
Siebesma, A. P., Soares, P. M., and Teixeira, J.: A combined eddy-diffusivity mass-flux approach for the convective boundary layer, J. Atmos. Sci., 64, 1230–1248, https://doi.org/10.1175/JAS3888.1, 2007.
Simmons, A. J. and Strüfing, R.: Numerical forecasts of stratospheric warming events using a model with a hybrid vertical coordinate, Q. J. Roy. Meteor. Soc., 109, 81–111, https://doi.org/10.1002/qj.49710945905, 1983.
Smart, S. D., Quintino, T., and Raoult, B.: A Scalable Object Store for Meteorological and Climate Data, in: Proceedings of the Platform for Advanced Scientific Computing Conference (PASC '17), Association for Computing Machinery, New York, NY, USA, Article 13, 1–8, https://doi.org/10.1145/3093172.3093238, 2017.
Stephan, C. C., Strube, C., Klocke, D., Ern, M., Hoffmann, L., Preusse, P., and Schmidt, H.: Gravity waves in global high-resolution simulations with explicit and parameterized convection, J. Geophys. Res.-Atmos., 124, 4446–4459, https://doi.org/10.1029/2018JD030073, 2019.
Stephan, C. C., Žagar, N., and Shepherd, T. G.: Waves and coherent flows in the tropical atmosphere: New opportunities, old challenges, Q. J. Roy. Meteor. Soc., 147, 2597–2624, https://doi.org/10.1002/qj.4109, 2021.
Stevens, B., Sherwood, S. C., Bony, S., and Webb, M. J.: Prospects for narrowing bounds on Earth's equilibrium climate sensitivity, Earth's Future, 4, 512–522, https://doi.org/10.1002/2016EF000376, 2016.
Stevens, B., Satoh, M., Auger, L., Biercamp, J., Bretherton, C.S., Chen, X., Düben, P., Judt, F., Khairoutdinov, M., Klocke, D., Kodama, C., Kornblueh, L., Lin, S.-L., Putman, W., Shibuya, R., Neumann, P., Röber, N., Vannier, B., Vidale, P.-L., Wedi, N., and Zhou, L.: DYAMOND: the DYnamics of the Atmospheric general circulation Modeled On Non-hydrostatic Domains, Prog. Earth Planet. Sci., 6, 61, https://doi.org/10.1186/s40645-019-0304-z, 2019.
Stockdale, T. N., Kim, Y.-H., Anstey, J. A., Palmeiro, F. M., Butchart, N., Scaife, A. A., Andrews, M., Bushell, A. C., Dobrynin, M., Garcia-Serrano, J., Hamilton, K., Kawatani, Y., Lott, F., McLandress, C., Naoe, H., Osprey, S., Pohlmann, H., Scinocca, J., Watanabe, S., Yoshida, K., and Yukimoto, S.: Prediction of the quasi-biennial oscillation with a multi-model ensemble of QBO-resolving models, Q. J. Roy. Meteor. Soc., 148, 1519–1540, https://doi.org/10.1002/qj.3919, 2022.
Streffing, J., Sidorenko, D., Semmler, T., Zampieri, L., Scholz, P., Andrés-Martínez, M., Koldunov, N., Rackow, T., Kjellsson, J., Goessling, H., Athanase, M., Wang, Q., Hegewald, J., Sein, D. V., Mu, L., Fladrich, U., Barbi, D., Gierz, P., Danilov, S., Juricke, S., Lohmann, G., and Jung, T.: AWI-CM3 coupled climate model: description and evaluation experiments for a prototype post-CMIP6 model, Geosci. Model Dev., 15, 6399–6427, https://doi.org/10.5194/gmd-15-6399-2022, 2022.
Suematsu, T. and Miura, H.: Zonal SST Difference as a Potential Environmental Factor Supporting the Longevity of the Madden–Julian Oscillation, J. Climate, 31, 7549–7564, https://doi.org/10.1175/JCLI-D-17-0822.1, 2018.
Takasuka, D. and Satoh, M.: Dynamical Roles of Mixed Rossby–Gravity Waves in Driving Convective Initiation and Propagation of the Madden–Julian Oscillation: General Views, J. Atmos. Sci., 77, 4211–4231, https://doi.org/10.1175/JAS-D-20-0050.1, 2020.
Takasuka, D., Kodama, C., Suematsu, T., Ohno, T., Yamada, Y., Seiki, T., Yashiro, H., Nakano, M., Miura, H., Noda, A. T., Nasuno, T., Miyakawa, T., and Masunaga, R.: How can we improve the seamless representation of climatological statistics and weather toward reliable global K-scale climate simulations?, J. Adv. Model. Earth Sy., 16, e2023MS003701, https://doi.org/10.1029/2023MS003701, 2024.
Takayabu, Y. N.: Large-scale cloud disturbances associated with equatorial waves Part I: Spectral features of the cloud disturbances, J. Meteorol. Soc. Jpn. Ser. II, 72, 433–449, https://doi.org/10.2151/jmsj1965.72.3_433, 1994.
Taylor, M., Caldwell, P. M., Bertagna, L., Clevenger, C., Donahue, A., Foucar, J., Guba, O., Hillman, B., Keen, N., Krishna, J., Norman, M., Sreepathi, S., Terai, C., White, J. B., Salinger, A. G., McCoy, R. B., Leung, L. R., Bader, D. C., and Wu, D.: The Simple Cloud-Resolving E3SM Atmosphere Model Running on the Frontier Exascale System, in: Proceedings of the International Conference for High Performance Computing, Networking, Storage and Analysis (SC '23), Association for Computing Machinery, New York, NY, USA, Article 7, 1–11, https://dl.acm.org/doi/10.1145/3581784.3627044, 2023.
Temperton, C., Hortal, M., and Simmons, A. J.: A two-time-level semi-Lagrangian global spectral model, Q. J. Roy. Meteor. Soc., 127, 111–127, https://doi.org/10.1002/qj.49712757107, 2001.
Tiedtke, M.: A comprehensive mass flux scheme for cumulus parametrization in large-scale models, Mon. Weather Rev., 117, 1779–1800, https://doi.org/10.1175/1520-0493(1989)117<1779:ACMFSF>2.0.CO;2, 1989.
Tiedtke, M.: Representation of clouds in large-scale models, Mon. Weather Rev., 121, 3040–3061, https://doi.org/10.1175/1520-0493(1993)121<3040:ROCILS>2.0.CO;2, 1993.
Tomita, H., Miura, H., Iga, S.-I., Nasuno, T., and Satoh, M.: A global cloud-resolving simulation: Preliminary results from an aqua planet experiment, Geophys. Res. Lett., 32, L08805, https://doi.org/10.1029/2005gl022459, 2005.
Treguier, A. M., de Boyer Montégut, C., Bozec, A., Chassignet, E. P., Fox-Kemper, B., McC. Hogg, A., Iovino, D., Kiss, A. E., Le Sommer, J., Li, Y., Lin, P., Lique, C., Liu, H., Serazin, G., Sidorenko, D., Wang, Q., Xu, X., and Yeager, S.: The mixed-layer depth in the Ocean Model Intercomparison Project (OMIP): impact of resolving mesoscale eddies, Geosci. Model Dev., 16, 3849–3872, https://doi.org/10.5194/gmd-16-3849-2023, 2023.
Trigo, I. F., Monteiro, I. T., Olesen, F., and Kabsch, E.: An assessment of remotely sensed land surface temperature, J. Geophys. Res., 113, 1–12, https://doi.org/10.1029/2008JD010035, 2008.
Untch, A. and Hortal, M.: A finite-element scheme for the vertical discretization of the semi-Lagrangian version of the ECMWF forecast model, Q. J. Roy. Meteor. Soc., 130, 1505–1530, https://doi.org/10.1256/qj.03.173, 2004.
van Westen, R. M. and Dijkstra, H. A.: Ocean eddies strongly affect global mean sea-level projections, Sci. Adv., 7, eabf1674, https://doi.org/10.1126/sciadv.abf1674, 2021.
Vivoda, J., Smolíková, P., and Simarro, J.: Finite Elements Used in the Vertical Discretization of the Fully Compressible Core of the ALADIN System, Mon. Weather Rev., 146, 3293–3310, https://doi.org/10.1175/MWR-D-18-0043.1, 2018.
von Albedyll, L., Hendricks, S., Hutter, N., Murashkin, D., Kaleschke, L., Willmes, S., Thielke, L., Tian-Kunze, X., Spreen, G., and Haas, C.: Lead fractions from SAR-derived sea ice divergence during MOSAiC, The Cryosphere, 18, 1259–1285, https://doi.org/10.5194/tc-18-1259-2024, 2024.
Wedi, N. P.: Increasing horizontal resolution in numerical weather prediction and climate simulations: Illusion or panacea?, Philos. T. Roy. Soc. A, 372, 20130289, https://doi.org/10.1098/rsta.2013.0289, 2014.
Wedi, N. P., Bauer, P., Denoninck, W., Diamantakis, M., Hamrud, M., Kuehnlein, C., Malardel, S., Mogensen, K., Mozdzynski, G., and Smolarkiewicz, P. K.: The modelling infrastructure of the Integrated Forecasting System: Recent advances and future challenges, ECMWF Technical Memoranda, 760, https://doi.org/10.21957/thtpwp67e, 2015.
Wedi, N. P., Polichtchouk, I., Dueben, P., Anantharaj, V.G., Bauer, P., Boussetta, S., Browne, P., Deconinck, W., Gaudin, W., Hadade, I., Hatfield, S., Iffrig, O., Lopez, P., Maciel, P., Mueller, A., Saarinen, S., Sandu, I., Quintino, T., and Vitart, F.: A baseline for global weather and climate simulations at 1 km resolution, J. Adv. Model. Earth Sy., 12, e2020MS002192, https://doi.org/10.1029/2020MS002192, 2020.
Wengel, C., Lee, S.-S., Stuecker, M. F., Timmermann, A., Chu, J.-E., and Schloesser, F.: Future high-resolution El Niño/Southern Oscillation dynamics, Nat. Clim. Change, 11, 758–765, https://doi.org/10.1038/s41558-021-01132-4, 2021.
Wheeler, M. and Kiladis, G. N: Convectively coupled equatorial waves: Analysis of clouds and temperature in the wavenumber–frequency domain, J. Atmos. Sci., 56, 374–399, https://doi.org/10.1175/1520-0469(1999)056<0374:CCEWAO>2.0.CO;2, 1999.
Wieners, K.-H., Ziemen, F. A., Koldunov, N., Pedruzo-Bagazgoitia, X., Rackow, T., Redler, R., Sidorenko, D., and Kölling, T.: nextGEMS: output of the model development cycle 2 simulations for ICON and IFS, World Data Center for Climate (WDCC) at DKRZ [data set], https://doi.org/10.26050/WDCC/nextGEMS_cyc2, 2023.
Yano, J.-I. and Wedi, N. P.: Sensitivities of the Madden–Julian oscillation forecasts to configurations of physics in the ECMWF global model, Atmos. Chem. Phys., 21, 4759–4778, https://doi.org/10.5194/acp-21-4759-2021, 2021.
Zampieri, L., Goessling, H. F., and Jung, T.: Bright prospects for Arctic sea ice prediction on subseasonal time scales, Geophys. Res. Lett., 45, 9731–9738, https://doi.org/10.1029/2018GL079394, 2018.
Zampieri, L., Goessling, H. F., and Jung, T.: Predictability of Antarctic sea ice edge on subseasonal time scales, Geophys. Res. Lett., 46, 9719–9727, https://doi.org/10.1029/2019GL084096, 2019.
Zhang, C.: Madden–Julian oscillation: Bridging weather and climate, B. Am. Meteorol. Soc., 94, 1849–1870, https://doi.org/10.1175/BAMS-D-12-00026.1, 2013.
Zsoter, E., Arduini, G., Prudhomme, C., Stephens, E., and Cloke, H.: Hydrological Impact of the New ECMWF Multi-Layer Snow Scheme, Atmosphere, 13, 727, https://doi.org/10.3390/atmos13050727, 2022.
- Abstract
- Introduction
- Model configurations
- Model developments for multi-year coupled kilometre-scale IFS simulations
- Selected examples of significant advances in kilometre-scale nextGEMS simulations
- Summary and conclusions
- Appendix A: Conservation properties of the IFS advection scheme and mass fixer approach
- Appendix B
- Appendix C
- Appendix D
- Appendix E
- Code availability
- Data availability
- Author contributions
- Competing interests
- Disclaimer
- Acknowledgements
- Financial support
- Review statement
- References
- Abstract
- Introduction
- Model configurations
- Model developments for multi-year coupled kilometre-scale IFS simulations
- Selected examples of significant advances in kilometre-scale nextGEMS simulations
- Summary and conclusions
- Appendix A: Conservation properties of the IFS advection scheme and mass fixer approach
- Appendix B
- Appendix C
- Appendix D
- Appendix E
- Code availability
- Data availability
- Author contributions
- Competing interests
- Disclaimer
- Acknowledgements
- Financial support
- Review statement
- References