the Creative Commons Attribution 4.0 License.
the Creative Commons Attribution 4.0 License.
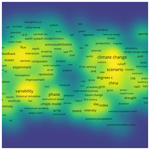
Baseline Climate Variables for Earth System Modelling
Martin Juckes
Karl E. Taylor
Fabrizio Antonio
David Brayshaw
Carlo Buontempo
Jian Cao
Paul J. Durack
Michio Kawamiya
Hyungjun Kim
Tomas Lovato
Chloe Mackallah
Matthew Mizielinski
Alessandra Nuzzo
Martina Stockhause
Daniele Visioni
Jeremy Walton
Briony Turner
Eleanor O'Rourke
Beth Dingley
The Baseline Climate Variables for Earth System Modelling (ESM-BCVs) are defined as a list of 135 variables which have high utility for the evaluation and exploitation of climate simulations. The list reflects the most frequently used elements of the Coupled Model Intercomparison Project Phase 6 (CMIP6) archive. Successive phases of CMIP have supported strong results in science and substantially influence international climate policy formulation. This paper responds to both interest in exploiting CMIP data standards in a broader range of climate modelling activities and a need to achieve greater clarity about the significance and intention of variables in the CMIP Data Request. As Earth system modelling archives grow in scale and complexity, there are emerging problems associated with weak standardisation at the variable collection level. That is, there are good standards covering how specific variables should be archived, but this paper fills a gap in the standardisation of which variables should be archived. The ESM-BCV list is intended as a resource for ESM intercomparison projects (MIPs) developing requests to enable greater consistency among MIPs and as a reference for modelling centres to enhance consistency within MIPs. Provisional planning for the CMIP7 Data Request exploits the ESM-BCVs as a core element. The baseline variable list includes 98 variables which have modest or minor data volume footprints and could be generated systematically when simulations are produced and archived for exploitation by the World Climate Research Programme (WCRP) community. A further 35 variables are classed as “high volume” and are only suitable for production when the resource implications are justified.
- Article
(2525 KB) - Full-text XML
- BibTeX
- EndNote
1.1 Context and motivation
With the publication of the Baseline Climate Variables for Earth System Modelling (hereafter ESM-BCVs; see the end of Sect. 4 for a discussion of the name), we aim to address the growing need for climate model data archives in order to have more consistency between projects and generations of models. We exploit substantial resources and knowledge that have been developed through the Coupled Model Intercomparison Project (CMIP; see Meehl et al., 1997). CMIP was established to collect data from models that could represent some aspects of the atmospheric, oceanic, land, and cryospheric components of the climate system and has grown over successive phases (Meehl et al., 2000, 2007; Taylor et al., 2012; Eyring et al., 2016) to provide both better representation of those processes and more complete coverage of the Earth system, including chemical, biogeochemical, and ecosystem processes. CMIP has also expanded from the initial focus on model evaluation to become “a central element of national and international assessments of climate change” (Eyring et al., 2016).
The CMIP community has led the way in developing climate model archives as a community resource with a range of users which extends far beyond the modelling centres responsible for developing models and delivering data products. The content of the archive is guided by the CMIP Data Request (CMIPDR; see Fig. 1). The latest iteration of this request for CMIP6 (Juckes et al., 2020) contained over 2000 variables, a significant increase from the 970 variables requested for CMIP5 (PCMDI, 2013). The CMIP6 Data Request (CMIP6DR) collated data requirements from dozens of international science projects to create a database of climate variables indexed against priorities, objectives, and experimental configurations. CMIP6DR was seen by many as being too extensive, and the mechanisms provided to enable data producers to filter the request down to an appropriate level were not able to compensate for this. A lack of clarity about priorities detracted from the consistency of the archive content (Sect. 1.3 below). The ESM-BCVs will provide a clear focus to enable greater consistency both within CMIP and between CMIP and other model intercomparison activities. This is, however, as the name suggests, only a baseline, and further variables will generally be needed in many cases. This caveat notwithstanding, the majority of users are interested in a modest subset of the 2000+ variables.
1.2 Expanding the scope and impact of Earth system modelling
The scientific scope of the climate models used to analyse the impact of humanity on the global climate is continually expanding (e.g. Flato, 2011), and the community is now experimenting with kilometre resolution models (e.g. Hohenegger et al., 2023) and explicit modelling of human behavioural response to climate (e.g. Tan et al., 2023). A review of this diverse and growing literature is beyond the scope of the current paper, but it is clear that preservation of clarity and interoperability of existing and future data products will be a challenge for this wide-ranging community. As the range of modelling activities has expanded, a diverse range of models and model configurations has emerged to target different areas of climate science, resulting in a multiverse of models (Fig. 2).
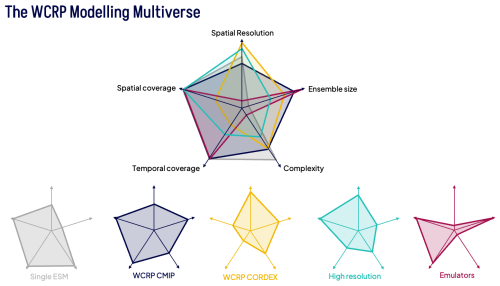
Figure 2The modelling multiverse. This is the phase space covered by each climate modelling endeavour within the WCRP. Each type of model or modelling project has a different ability to model over different spatial resolutions, spatial coverages, temporal coverages, model complexities, and ensemble sizes. Each model type or modelling project is exemplified using a different colour. The elements of the radar charts are (1) spatial resolution, the ability to resolve fine-scale spatial features; (2) ensemble size, the ability to resolve details of internal variability; (3) complexity, the ability to resolve a wide range of physical and bio-geological climate processes; (4) temporal coverage, the ability to cover centennial timescales; and (5) spatial coverage, the ability to cover the complete globe.
The exchange of interoperable climate model output across multiple model intercomparison projects (MIPs) is now a mainstay of climate science and climate assessment, feeding into the development of policies on climate change mitigation and adaptation. Scientific work supported by CMIP has become the foundation for Intergovernmental Panel on Climate Change (IPCC) assessment reports which are alerting humanity to the risks of catastrophic climate change (Touzé-Peiffer et al., 2020; Intergovernmental Panel on Climate Change, 2023), driving international commitments to decarbonisation of the economy (Paris Agreement, United Nations, 2015; Guterres, 2023).
With the growth in the scale and complexity of the models and the intercomparison projects that investigate their behaviour, there is growing interest in multi-variable multi-model analyses. There is an emerging requirement for consistent provision of variable collections across simulations generated by the entire World Climate Research Programme (WCRP) multiverse of models. For robust simulation and analysis of the climate system on centennial timescales, multi-model ensembles are required. Through multiple phases of CMIP, an open and evolving community approach to creating intercomparisons which span multiple MIPs and all the elements of the WCRP multiverse has been established. We refer to the collection of simulations generated through these activities as a multiverse ensemble (MVE).
The success of MVEs in creating value which is greater than the sum of the parts has led to a growing ecosystem of MIPs and other community activities coordinating the specification of science goals, experimental configurations, and data requirements for MVEs. Data requirements now must serve not only climate researchers, but also a diverse community of stakeholders that rely on climate model output. Textual analysis of the 5152 Web of Science publications1 that, on 24 August 2023, referenced CMIP6 shows two main clusters, one associated with model and climate system analysis and experiments and the other associated with impacts, adaptations, and scenarios (Fig. 3). This analysis shows clearly how scenario and impact clusters have acquired equal significance, in terms of quantity of publications, with the climate science research field.
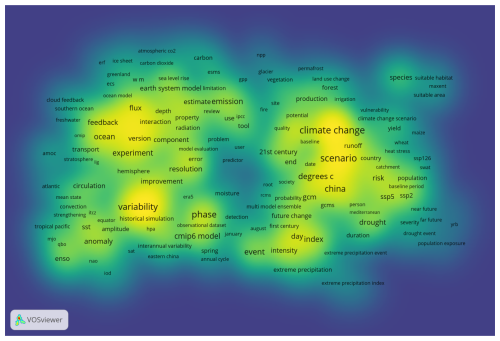
Figure 3Word cloud of CMIP6 science. It is generated by analysis of the titles and abstracts of 5152 Web of Science articles. Words are grouped according to closeness, which is defined as the frequency with which they appear in the same papers. Yellow indicates clustering of more commonly used words. Generated by VOSViewer.
1.3 Objectives of the Baseline Climate Variables for Earth System Modelling list
As the name suggests, the list presented here is intended to define a baseline set of climate variables which can be produced by ESM activities and which are of widespread interest. By including a rather limited subset of commonly analysed variables, we expect that modelling groups will easily be able to routinely provide all variables and that data centres will be able to accommodate the generated data volumes. For indirect users such as the climate and climate impact research communities, the variables in the baseline set will facilitate consistent and efficient comparison of simulations across multiple intercomparison projects, both within and between existing and future CMIP eras, by enhancing standardisation at the variable collection level (see Fig. 4 and the discussion in Sect. 1.4 for the motivation behind this objective).
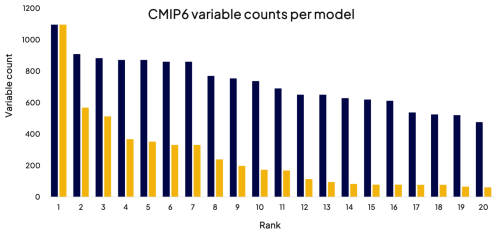
Figure 4Variable provision in CMIP6. The number of variables (y axis) published for the historical simulation by each model (as represented in the DKRZ Earth System Grid Federation (ESGF) index node for August 2023) is shown in the blue columns against the model rank, where models are ranked in order of decreasing variable count. Also shown, in orange, is the number of variables which are included by all models up to the given rank. For comparison, the total number of variables requested by all MIPs from the CMIP6 historical simulation was 2301, with 1484 of those assigned priority one by one or more MIPs.
Use of the term “Earth system modelling” in describing the list is meant to convey that these variables should be of interest from a wide range of models used in studying the climate of the Earth system. This includes, for example, not only models which have a detailed representation of interactions between the physical climate and the biosphere, but also simpler models which play a role in advancing understanding of critical elements of the Earth system.
Although the list serves as a baseline, it is not expected to be sufficient for addressing many of the specific science questions that are the focus of MIPs. Invariably, additional variables will be of value and, in some cases, essential in interpreting and understanding simulation results. There may also be some model intercomparison experiments that focus on a single aspect of the Earth system where many of the baseline variables will be irrelevant or of little interest. As a trivial example, in the case of an atmospheric model run with prescribed sea surface conditions, all the baseline ocean variables, except sea surface temperature and sea ice fraction, will be irrelevant. On the other hand, none of the variables characterising bio-geochemical cycles and atmospheric chemistry appears in the baseline list, even though they would be essential in understanding those aspects of the Earth system.
Even if the list cannot meet all the requirements of MIPs, it can be considered the minimal suite of variables to be archived from simulations meant to serve a broad range of WCRP stakeholders. For the climate and climate impact research communities, the variables in the baseline list will enable consistent and efficient comparison of simulations across multiple intercomparison projects, both within and between existing and future CMIP eras. The baseline list of variables may also nurture development of evaluation tools once there is an expectation that a consistent set of climate variables will be made available from many MIP experiments.
The ESM-BCVs will also provide a basis for comparison with parameter lists widely used in different communities, such as variables used for exchange of meteorological observations in the GRIB2 protocol, the Essential Climate Variables (ECVs: World Meteorological Organisation, 2022a, b), or the Global Climate Indicators (GCIs) (https://gcos.wmo.int/en/global-climate-indicators, last access: 13 March 2025) concept in climate services.
1.4 Variable output by model
The CMIP6 archive contains a comprehensive range of data products, with 723 models contributing to the “all-forcing simulation of the recent past (historical)”4, but users looking for data to support multi-variable analysis can run into problems because of a lack of consistency in the selection of the variables which are available for each model. Thus, although there are 25 models providing 390 or more variables for the historical simulation (see Eyring et al., 2016), the number of variables which those models have in common only goes up to 57 (see Fig. 4)5. This lack of consistency can force analysts to be selective about the models included in any analysis and lead to a lack of interoperability between derived products. If, for instance, a drought indicator is based on a cluster of models “A” which have a full range of precipitation, runoff, and evapotranspiration variables at a monthly frequency and the growing season indicator is based on a cluster of models “B” which have daily precipitation, cloud cover, and temperature variables including daily extremes, the differences between clusters A and B may hamper the combined use of the two products. If set A is defined by models which have, for the historical, ssp126, and ssp245 experiments, variables Amon.pr, Lmon.mrro, Lmon.evspsblveg, and Lmon.evspsblsoi in the CMIP6 naming conventions and set B is defined by models which have day.pr, day.tasmin, day.tasmax, and day.clt for the same experiments, then set A has 34 models from 20 institutions, set B has 27 models from 19 institutions, and the intersection is 20 models from 14 institutions. Publication of CMIP6 data is ongoing and details may evolve, but the patterns of inconsistency seen here represent a snapshot of the data landscape which confronts users dealing with the archive now.
1.5 Stakeholder groups
CMIP, and hence the CMIPDR, has an extensive community of stakeholders. Table 1 lists the main stakeholder groups. Some of these (darker shading) have a direct interest in the specific variables which are requested, archived, and disseminated. Others (lighter shading) are more concerned with derived products and messages and with the level of reliability and trust which can be associated with those products and messages.
The existence of a set of baseline variables which is available consistently from virtually all the models and experiments is of particular importance to this second group because they often use derived products which depend on multiple variables from multiple models and experiments.
The 2022 CMIP6 Community Survey (O'Rourke, 2023) received over 300 responses. There was very clear appreciation for the coordination effort and the principles behind CMIP6DR, but many respondents did suggest that there were too many variables assigned priority “1” and that this placed a burden on the modelling centres6. These responses reflected the discussion at the conference held by the WCRP Working Group on Coupled Modeling (2019) in Barcelona at which a community intention to reduce the number of variables at priority 1 from around 50 % to a significantly smaller number emerged, with a suggestion to start with those prioritised by AR6 WG1 (see Juckes, 2020).
The 2022 CMIP6 community survey also received many responses highlighting a need for additional variables like increased temporal resolution, more ocean variables, variables relevant to extremes, and those variables required to support the CORDEX (Gutowski et al., 2016) regional downscaling community and its downstream users. These requirements for additional variables are not addressed by the baseline list.
2.1 Launch and scoping workshops
The consultation process was launched in April 2022 by the CMIP International Project Office (IPO) with a request for feedback on the proposed process, an invitation to scoping meetings, and a target of establishing “a baseline set of variables for exchange of climate model data” (see Appendix D). The announcement was sent to the modelling centres, data request leads, and MIP chairs and circulated by the World Climate Research Programme. The responses, 32 in all, were received from respondents across Asia, Europe, and North America whose CMIP6 involvement included being data request leads, modelling centre leads, MIP chairs, and users of CMIP data, for scientific and climate impact modelling as well as climate services provision. The findings from this survey were discussed at two scoping workshops held on 12 and 17 May 2022. The focus of the workshops was on finalising the processes of defining the variable list, creating an author team for this paper, and creating an outline of the paper structure.
The scoping workshop report includes directions for authors to focus on clarifying the purpose and function of the list and identifying the requirements of user groups.
There was also concern about the selection criteria. There is clear agreement on the need for a baseline list and recognition of the utility of such a list for many user communities, with a high level of support adopted for the process of expert elicitation. Some contributors argued for a process based on defining specific variable selection criteria which could be applied consistently to every variable in the list, but there were no specific proposals for such criteria. Instead, in line with the established approach in CMIP6DR, the process adopted was to ask experts to consider the list against the agreed-upon objectives (see Sect. 1.2 above).
2.2 Shortlisting from the CMIP6 request
The initial shortlist of baseline variables was arrived at based on the CMIP6 archive's model output statistics, which gauge the willingness of modelling groups to report each variable and the user demand for each variable reported. The resulting shortlist of variables was then edited and augmented based on community input.
Selection of an initial shortlist of variables was based on the variables requested for CMIP6 but excluding all but priority-1 variables. Three scores were calculated, ranking the variables according to the number of models contributing, the volume of data downloaded, and the number of files downloaded. The shortlist provided a starting point for the consultation and expert discussion.
The formal steps taken were as follows:
-
Extract the list of 1206 variables assigned default priority 1 in CMIP6, out of a total of 2062.
-
For each variable, assign three ranking scores r1,r2, and r3:
- a.
r1 is ranked according to the volume of data downloaded across the entire CMIP6 archive and retrieved from the ESGF dashboard (Fiore et al., 2021)7.
- b.
r2 is ranked according to the number of files downloaded across the entire CMIP6 archive and retrieved from the ESGF dashboard.
- c.
r3 is ranked according to the number of models that provided the variable for the CMIP6 historical experiment.
- a.
-
Rather than weighting the criteria, we take the minimum rank rmin = min().
-
Define the shortlist as the first 125 variables ordered by rmin, together with their supporting fixed fields (which are necessary for correct interpretation of the data, e.g. grid cell area or volume).
For details of the variables which were included in the shortlist, see Appendices A and B.
2.3 Community survey and analysis
Following the creation of a shortlist, a community survey was designed to elicit expert feedback on the initial list. The survey was targeted at those providing access to and/or utilising the outputs of climate models within the commercial, public, and voluntary sectors as well as academia. The survey was circulated to the CMIP mailing lists for modelling centres, data requests, and MIP chairs by the WCRP and the author team and was promoted through CMIP social media channels. It was open to respondents for a period of just over 6 weeks between 23 August and 8 October 2022. Of the 44 responses received, the majority identified as climate data users and 12 identified as climate model data providers. The shortlisted variables were reviewed in detail by 29 respondents: these respondents were invited to review a selection of variables relevant to their expertise or data usage. Sixteen respondents reviewed five or fewer variables, and the remainder reviewed a larger selection. A scoring methodology was provided to ensure review consistency. A full summary report of the survey responses has now been published (O'Rourke and Turner, 2022; see also the survey announcement in Appendix D).
2.4 Shortlist revision and consequences
In two further author team meetings in late 2022, the results of the survey were discussed and analysed in depth in order to consider potential additions and deletions of some shortlisted variables.
In early 2024, checks of the ESGF dashboard revealed a previously undetected error in reporting the download statistics that were relied on in arriving at the initial shortlist of variables. Data transfers associated with unsuccessful requests for partial file downloads over very-low-capacity networks had been misreported in log files as successful, exaggerating the user demand for some variables. The team at the CMCC Foundation (Euro-Mediterranean Center on Climate Change) responsible for the dashboard were able to provide corrected download statistics based on a reanalysis of the log files. The corrected download reports were used to reassess variables in the ESM-BCV list agreed on in 2022, resulting in four variables being removed and four different ones being added (see Appendix B and Tables B1 and B2 for the details of the individual variables).
Further discussions by authors and a final meeting in June 2024 led to a review of the criteria for fixed model configuration fields (they were retained if more than 12 models had provided the variable for at least one experiment).
The variable list presented here will be a baseline set of variables for global model intercomparison, evaluation, and exploitation projects and programmes. This is intended as a starting point for more comprehensive lists tailored to specific applications. Many of the variable definitions in the list are used in modelling activities across the whole scope of the WCRP, either through MIPs associated with CMIP (particularly in the Climate and Cryosphere (CliC) and Climate and Ocean Variability, Predictability and Change (CLIVAR) core projects) or output from activities such as CORDEX (the Regional Information for Society (RIfS) and Global Energy and Water Exchanges (GEWEX) core projects) and the Chemistry-Climate Model Initiative (part of the Atmospheric Processes and their Role in Climate (APARC) core project), which are shadowing CMIP data protocols: the ESM-BCV list will support progress towards greater consistency and interoperability in data outputs from this extensive range of activities.
3.1 Form of the list
The baseline variable list should also provide a model for clarity and interoperability. This scope of this paper covers the selection and definition of the physical quantities along with their spatio-temporal sampling structures.
Some variables are categorised as “high volume” and should be considered optional when resource constraints apply. These variables have a particularly high value for many users but are likely to be too resource-intensive for many climate simulations. They are included so that they can benefit from the visibility afforded by the baseline list, but they are not expected to be systematically produced to the same extent as the other variables in the list.
This paper is concerned with the scientific definition of baseline variables with a simple semantic structure. Each entry is identified by a short name (combined from the CMIP6 CMOR table and a variable short name), title, description, standard name, and unit, a format that has evolved since CMIP3 (WGCM Climate Simulation Panel, 2007). Syntax rules for the list entries are given in Table 2. The identifier will be considered a registration identifier and is not expected to be used in CMIP7 era products. New naming conventions are under discussion (Karl E. Taylor, personal communication, 2024).
We have not been able to eliminate redundancy from the list: for instance, there is redundant information in variables on 8 atmospheric levels and the same variables on 19 levels. The evidence from CMIP6 usage statistics is that both variations are very frequently used.
Table 2Syntax rules for items in the baseline variable list.
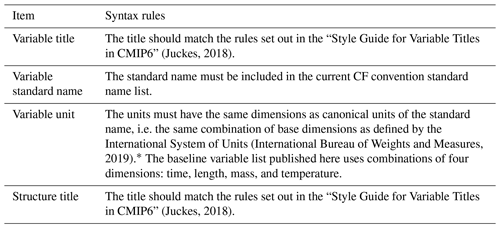
* The University Corporation for Atmospheric Research (UCAR) Udunits package (https://www.unidata.ucar.edu/software/udunits/, last access: 13 March 2025) can be used to check the consistency of the unit dimensionality.
3.2 Role from the modeller perspective
The list of baseline variables will, in the first instance, aid the model development process as a set of diagnostics for which known good output is created by the model. For instance, this set can be used in regression tests when evaluating new model versions in order to detect significant changes in output.
The greater the overlap between what is output by the model and the baseline list, the greater the contribution the model will be able to make in intercomparison exercises and the more widely the variables produced by the model will be used. Thus, producing and publishing as many of the listed baseline variables as possible should be an aspiration in the development and use of the model.
From the model developer's perspective, transparency in the process of creating the baseline variable list is important, because this clarifies the purpose of the list. The value of having a list and using it should be well-understood. It is not expected that all models will be able to generate all variables, but the exclusion of specialised variables from the list will ensure that most models can produce most variables. The process for maintaining and extending the list should be equally transparent. If a modelling group is unable to provide a variable (especially one in the baseline list), they should be encouraged to provide a reason using – for example – one of those listed in Table 3. The process for providing feedback should be lightweight and transparent.
3.3 Role from the infrastructure provider perspective
Data infrastructure such as the Earth System Grid Federation (ESGF, https://esgf.llnl.gov/, last access: 13 March 2025 and Petrie et al., 2021), the Climate and Forecast Conventions (CF, https://cfconventions.org/, last access: 13 March 2025), and the CMIPDR, along with secondary data portals, cloud platforms, and collaborations (e.g. the Copernicus Climate Change Service C3S, https://climate.copernicus.eu/, last access: 13 March 2025), and PANGEO, https://pangeo.io/ (last access: 13 March 2025) and the underlying physical infrastructure, staff, and curation systems provided by host institutions, disseminates climate datasets created by a variety of international modelling centres, building on the data standards set by the community. This standardisation ensures that user analysis can be performed across the multi-model ensemble and facilitates the scaling of data processing systems to provide and work with volumes at the magnitudes involved in CMIP. For automated data processing options, standard compliance is essential (but see the above comments on incomplete compliance). For example, ESGF aims to enhance its compute services as part of its future architecture plans (Kershaw et al., 2020). Secondary data evaluation or analysis packages such as ESMValtool (Weigel et al., 2021) and the Program for Climate Model Diagnosis and Intercomparison (PCMDI) (Lee et al., 2022, 2024) also rely on these data standards. The CMIP approach is founded on the CF metadata standards for NetCDF data. The CMIP project has built on these with the Data Reference Syntax (DRS; Taylor et al., 2018) defining file naming and data structure conventions and the Controlled Vocabularies (Durack et al., 2024) defining the terms within these components.
A baseline variable list with common variable definitions will furthermore enable portals and indexers to support cross-project data discovery and data analysis. The unique identification of the baseline variables and a consequent versioning and maintenance of the list will ensure traceability of the variable usage in the future. The I-ADOPT Framework ontology (Magagna et al., 2023) provides a standard for this, which is implemented by the NERC vocabulary server providing e.g. the CF standard names (http://vocab.nerc.ac.uk/collection/P07/current/, last access: 14 March 2025).
The quality of the data and metadata is of vital importance. There are certain metadata which must have correct values for the data to be ingestible by applications such as ESMValTool (Weigel et al., 2021).
Reliable and maintained software tools for creating standard compliant datasets are available for the modelling centres, but a range of issues associated with implementation workflows has led to incomplete compliance in CMIP archives. A scan of files from the CMIP archive (Petrie et al., 2024) revealed a wide range of technical errors. Some of these are related to mistakes in the specification of the cell methods, which might be obviated by improved documentation – particularly for those cell methods which are used by the baseline variables. It should also be noted however that most of the errors would have little impact on the use of the majority of software applications. Experience shows that time-consuming or resource-intensive data quality checks applied before data publication can reduce the amount of time and energy that has to be invested in correcting issues and replacing datasets. The CMIP6 requirements specified compliance with the CF conventions and correct implementation of metadata in CVs and the data request (the latter can be verified with the PrePARE tool; Mauzey et al., 2024). More detailed data checks such as the World Data Center for Climate quality control approach for CMIP5 (Stockhause et al., 2012) or for the C3S Climate Data Store (CDS; Buontempo et al., 2022) include range, outlier, and time axis checks alongside CF compliance.
Underlying archive services which host ESGF and other climate data infrastructures will also benefit from greater consistency between different intercomparison projects. Stability of data specifications and data structures will allow archives to develop and maintain systems that exploit these structures with the confidence that they will persist and be relevant for the duration of the data exploitation cycle.
3.4 Role from the data user perspective
Data users of CMIP are a diverse community that includes climate modellers, scientists from a wide range of disciplines, and private-sector product developers, and it is therefore hard to define who a “typical” user is. Historically, climate scientists represented the most important component of the user landscape. They used the data to understand processes and study the future evolution of the climate and its potential impact on the natural system and human activities. There is no obvious boundary between climate impact scientists and downstream exploitation of CMIP data for climate services (either public or private). CMIP data represent an important source of quantitative information for a large variety of actors and researchers operating well beyond the baseline remit of the climate science community. These users come from academia and industry, working in areas which could possibly be called the climate adaptation and climate service community. A key need of this community, however, is access to high-quality quantitative climate projection data, particularly focusing on ECVs (e.g. wind speeds, insolation, precipitation, and surface air temperature) mostly close to the surface. These correspond to a very small subset (∼ 10) of the many variables CMIP makes available, but the existence of high-frequency and high-resolution climate data would enable much deeper integration of climate model output with downstream impact models (which often describe highly complex responses to a given set of meteorological time series input). An example of this lies in energy systems research and applications (Craig et al., 2022; Dubus et al., 2022): the models used to inform electricity system planning typically operate on hourly time steps (as many of the fundamental design constraints relate to this timescale), and thus, for effective coupling, hourly gridded climate data (e.g. relating to wind resources at individual sites and time steps) become essential. It would be extremely beneficial for the application community to ensure both widespread output of a small but comprehensive set of essential surface climate variables at the highest feasible sub-daily frequency and very strict observance of data and metadata standards for them. The contrast between this and previous CMIP archives would be considerable: in the current archives any analyst who wishes to look at more than a few essential surface climate variables must make a choice between having heterogeneous diagnostics with different multi-model ensembles for each variable, limiting the number of models involved, and making extreme compromises regarding the data frequency provided (e.g. daily rather than sub-daily). Neither of these is ideal. By establishing a clear and realistic baseline, we hope to ensure that there is a greater level of consistency in the data collections, allowing more robust multi-variable analysis and enabling much stronger linking of raw output from climate models to downstream impact models, thus facilitating the translation of climate risk into meaningful and applicable information for end-users and society as a whole.
The goal is to achieve 90 % of the models providing 90 % of the low-volume and configuration baseline variables across major intercomparison programmes such as CMIP7. In fact, in the last CMIP6 exercise, only 29 % of the models provided 29 % of the priority-1 variables, 90 % of the models provided 8 % or more, and only two models8 provided 50 % or more.
The ESM-BCV list, after shortlisting and revision, contains 132 variables listed in Appendix A (Tables A1, A2, and A3). In the final list there are 121 time series and 14 fixed fields. Of the time-varying fields, 35 are classified as high volume (see Fig. 6 and Table C2 for the illustrative data volumes that determined the categorisation). The high-volume category includes sub-daily data, daily data on 19 pressure levels (see Appendix E for details), and monthly data on ocean model levels. The remaining 86 lower-volume time-varying fields and the 14 fixed field variables should be considered top priority for most WCRP MIP climate simulations, although it is recognised that, in the short term at least, it may not be possible to provide 100 % of them in all cases. More details are given in Fig. 5 and Table C1.
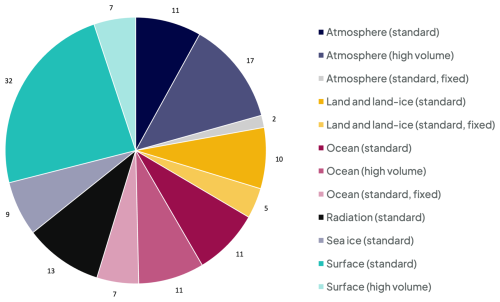
Figure 5ESM baseline climate variable categories and distribution of ESM-BCVs across a range of categories (using the data listed in Table C1 in Appendix C). A variable is considered “high volume” (dark shading) if 10 000 years of simulations generate more than 1500 GB of data from a 1° model with 60 atmospheric levels and 500 oceanic levels archived (assuming single-precision data storage without compression).
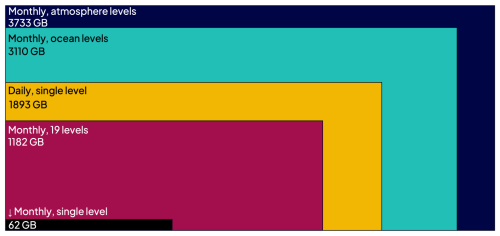
Figure 6Example data volumes expressed in gigabytes per 10 000 years of simulation for a notional 1° resolution model with 60 atmospheric levels and 50 oceanic levels (see Table C2 in Appendix C for details). Each rectangle area (both visible and obscured) represents the nominal volume for a specific output category. Single-precision data storage without compression is considered here.
The shortlisting was based on four criteria: limiting consideration to CMIP6 priority-1 variables, the number of files downloaded, the volume of data downloaded, and the number of models for which a variable was provided.
Although all four criteria were formally included in the shortlisting process, they had differing impacts:
-
Limiting consideration to CMIP6 priority-1 variables prevented only one variable from making the shortlist (monthly Temperature of Soil, Lmon.tsl).
-
The criteria based on the number of files downloaded added one variable which would have otherwise not been included (daily Total Cloud Cover Percentage, day.clt).
-
The shortlist of low-frequency variables (monthly and lower frequencies) would have been unaffected had we only considered the number of contributing models.
-
If download volumes were used as the only criterion, the resulting list of higher-frequency variables (daily and higher) would have been the same when considering all four criteria (apart from day.clt).
Thus, for the fixed and monthly mean fields, the shortlist was largely based on the model participation statistic, and for the high-frequency fields it was based on the volume of data downloaded. This process resulted in a shortlist of 147 variables.
During the subsequent community consultation, 27 variables were removed from the shortlist (see Table B1) and 15 were added (see Table B2), resulting in the 135 variables listed in Appendix A.
We can support the reasonableness of the ESM-BCV list by pointing out that it is not dissimilar to past lists of CMIP high-priority standard output. Modelling groups participating in MIPs have been producing many of these variables for over 2 decades. It is informative to compare the ESM-BCV list with the 118 high-priority variables specified for CMIP3 (WGCM Climate Simulation Panel, 2007). Some variables in the CMIP3 list were dropped prior to CMIP6 because they were designed to monitor model limitations which are no longer relevant (e.g. imposed ocean “flux corrections” that are no longer needed). Eliminating such variables, we find that 80 % of the variables remaining are also included in the BCV list. This indicates that, although list development followed different procedures in the past, there is a high degree of continuity in the perceived value of these variables.
The process of consultation in defining the shortlist and agreeing to subsequent revisions has helped to spread awareness of the scope and impact of the CMIP variable metadata and has driven new engagement in the process. There was strong support for the utility of the list (80 % of the survey respondents rated the usefulness at four or five out of five). There was also support for the process albeit with caveats raised about the possible bias towards past requirements rather than future needs (O'Rourke et al., 2023). The author discussions leading to finalisation of the list went beyond evaluation of the community consultation. The name of the list, which started as “Baseline Climate Variables”, changed twice, firstly to “Baseline Climate Variables for Earth System Models” in order to avoid any appearance of detracting from the Global Climate Observing System (GCOS) Essential Climate Variable work (World Meteorological Organisation, 2023) by clearly emphasising the focus here on model data and then to “Baseline Climate Variables for Earth System Modelling” in order to avoid the potentially restrictive interpretation of Earth system models only being those with a comprehensive range of interactions between the biosphere and physical climate components.
4.1 Provenance
The CMIP6 variables derive from many sources. Many variables were inherited from CMIP5 standard output (PCMDI, 2013). Revisions and extensions to CMIP6 came from Griffies et al. (2016) for Omon variables, van den Hurk (2016) for land surface variables, Notz et al. (2016) for sea ice variables, Gerber and Manzini (2016) for daily atmospheric fields, Haarsma et al. (2016) and Ruane et al. (2016) for 6hrPlev.hurs, and Jones et al. (2016) for the carbon cycle and terrestrial biosphere.
4.2 Limitations, extensions, and revisions
As noted in the Introduction, the ESM-BCV list is deliberately limited in scope so that it can be implemented across a wide range of modelling activities without incurring unreasonable costs. The need for additional variables in many important use cases was discussed, such as the need for more variables to close the carbon budget, accurately reflect the ocean heat content, or monitor ocean currents. Coverage of these use cases is deliberately omitted here and is being picked up in the CMIP AR7 Fast Track Data Request9. The latter request contains, in the version 1.0 release, over 1800 variables which are associated with specified scientific or climate impact use cases.
It has also been noted that the use of model levels for ocean variables results in high-volume datasets which can be difficult for some users to exploit because of the complexity of the vertical coordinates used in the models. Discussions about a potential shift to an agreed-upon set of layers in a standard coordinate system are taking place within the ocean theme of the CMIP AR7 Fast Track Data Request. Some overlapping spatial dimensions (P8 and P19 atmospheric levels) and temporal frequencies (3 h and 1 h) are still part of the list to enable a certain flexibility (e.g. for MIP proposal) and to either retain or avoid such redundancies in the compilation of a specific experiment request.
Revision of the list, i.e. changing the variables included in the baseline rather than constructing a larger list which builds on the baseline, as is being done for the CMIP AR7 Fast Track Data Request, has also been discussed. It is clear that revision will be needed to accommodate changes in scientific focus, but this need for revision needs to be balanced against the need for stability associated with the central aim of enhancing interoperability between distinct activities and distinct phases of CMIP.
The set of 132 ESM-BCVs presented here provides a reference collection of variables for MIPs which will facilitate greater consistency in data requests. By identifying variables which have high utility in many applications, the ESM-BCV list will also enable modelling centres to develop, standardise, and rationalise workflows.
The baseline list presents a standardised set which should be within reach of any modelling centre aspiring to generate data for community evaluation and exploitation. There will always be circumstances in which variables need to be omitted, especially the high-volume subset of 35 variables, but we expect this baseline set to lead to enhanced consistency in the expanding WCRP climate projection archive.
The ESM-BCV list should be seen as a snapshot of variables currently considered by modelling groups and users to be of general high value. Its similarities to earlier CMIP lists of high-priority variables attests to its likely continued relevance long into the future, but the expectation is that reassessment of community priorities will result in modifications to the list.
The baseline variable list has grown out of CMIP6DR (Juckes et al., 2020) and is dominated by variables already present in earlier requests (PCMDI, 2013). It has been shaped by feedback about the problems caused for users by inconsistencies in the CMIP6 archive and for providers by late finalisations of requests (see Fig. 3 for more details). The baseline variable list will reduce the workload for data providers, service providers, and users by providing a reusable and reliable basic set of variables. For users in the climate services and other communities outside the research community, the baseline variables will promote greater consistency and transparency in the derived products used by these communities, which typically depend on multiple variables and multiple climate models.
Although the baseline set includes a little over 7 % of the variables found in CMIP6DR, the consultation process revealed that most climate service users tend to use an even smaller subset of the variables. A more detailed analysis of the needs of the user and stakeholder landscape is required and may call for further differentiation of the baseline variable portfolio.
There has been considerable interest in the creation and sharing of standard indices of climate variability (e.g. Klein Tank et al., 2009). The level of standardisation of definitions of these indices is not sufficiently advanced to support reliable direct collection through the data request. The underlying challenge providing a central reference for these indices will, however, be picked up by the CMIP7 Rapid Evaluation Framework (REF) project10.
The ESM-BCV list is intended to address issues associated with the rapid expansion and relatively weak prioritisation of CMIP6DR (around 50 % of the variables were classified as top priority, which is more than most of the models provided). The list provides a starting point for any model workflows which are intended to support community multi-ESM ensembles.
The list falls well short of the scope needed to support scientific analysis or detailed climate impact assessment. In either of those cases, additional variables will need to be defined for MVEs which target specific science goals or climate impact work. For instance, work on dynamical processes in the atmosphere will require high-resolution models and specialised atmospheric variables to capture details of those processes. Work on the terrestrial biosphere will typically use lower-resolution models and a broad range of land surface variables. Work on climate impacts will require a range of surface and near-surface variables archived at sufficient frequency to support analysis of the impacts on social, economic, and biological systems. For CMIP7, the baseline list will form the core of the data request and be complemented by a set of topic-themed papers to be developed through a process which is based on that described here for the baseline variables11.
We have not made a detailed comparison of the ESM-BCVs and the GCOS Essential Climate Variables in this paper. This work is part of a wider set of changes to the way that climate model data standards are supported within WCRP. Care needs to be taken when comparing model output with observations: for instance, the cloud cover variables used to compare models with each other are not directly comparable with observations.
There are 135 ESM-BCVs. Of these, 35 are flagged as high volume and 14 are fixed model configuration fields. They are listed in Tables A1, A2, and A3 below.
Table A1ESM-BCVs: 14 fixed model configuration fields. These ESM-BCVs are listed under 10 different structures. For masked fields, the nature of the unmasked points is indicated in brackets. For example, “Masked (land)” implies that only land points are included. All are global fields. The “Model configuration” fields have no temporal dimension. Area means and sums are taken over grid cells, and time means are taken over the sampling period, e.g. a day or a calendar month. The frequency in column 2 indicates the frequency of stored data points, which may be time means or instantaneous values. The abbreviation is “f” for fixed.
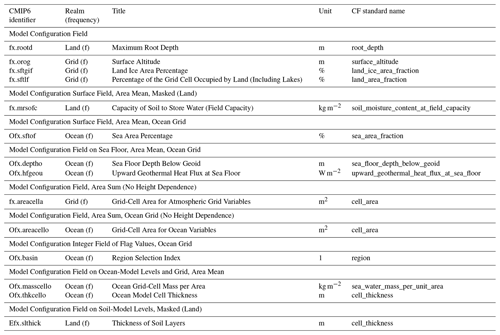
Table A2ESM-BCVs: 86 low-volume variables. These ESM-BCVs are listed under 17 different structures. For masked fields, the nature of the unmasked points is indicated in brackets. For example, “Masked (Land)” implies that only land points are included. All of them are global fields. Area means and sums are taken over grid cells, and time means are taken over the sampling period, e.g. a day or a calendar month. The frequency in column 2 indicates the frequency of stored data points, which may be time means or instantaneous values. The abbreviations are “m” for monthly and “d” for daily.
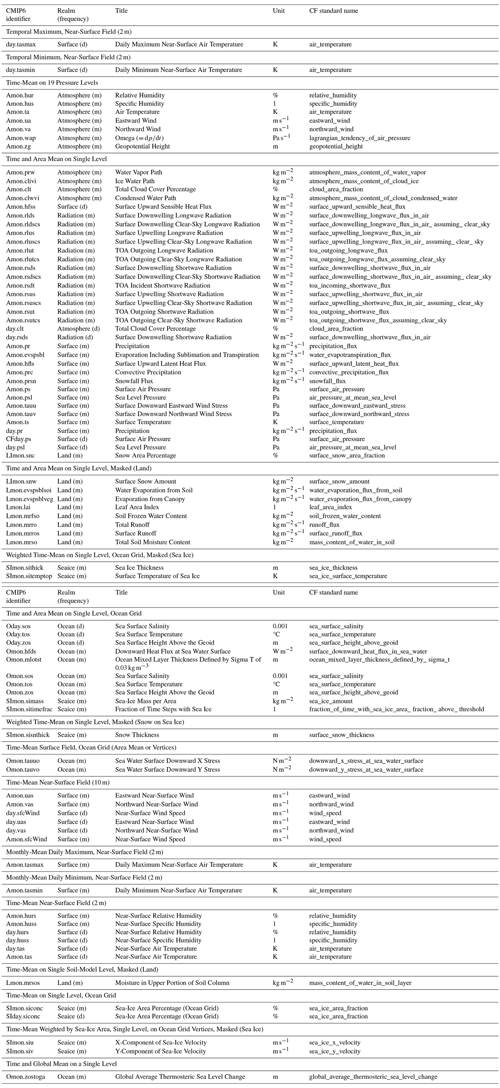
Table A3Baseline climate high-volume list of 35 variables. The abbreviations for frequency are as for Table A1, extended to include the abbreviations “3” and “6” for 3-hourly and 6-hourly, respectively.
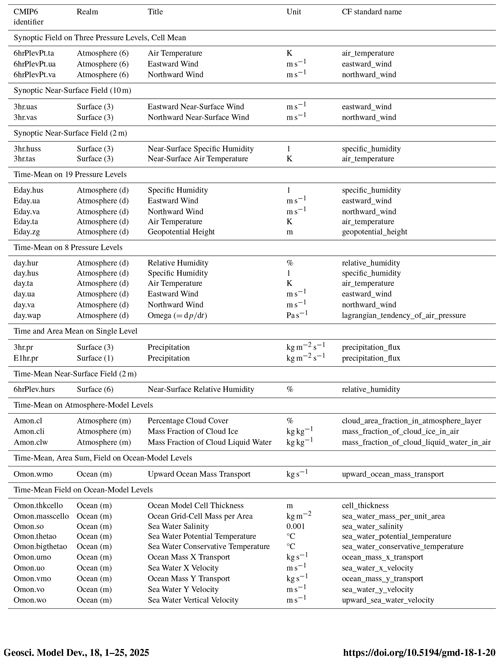
Table B1Variables were included in the shortlist and removed in the revision process. The reasons were as follows: S – specialist variables of use for a limited range of applications; D – duplicate or near duplicate of another variable in the list; E – included in the shortlist as a result of a clerical error as these variables did not meet shortlisting criteria. O – these variables were included following an initial decision to include all fixed variables, but as they have extremely low usage, only being published for 12 or fewer models, they were subsequently removed. X – low usage in corrected download statistics.
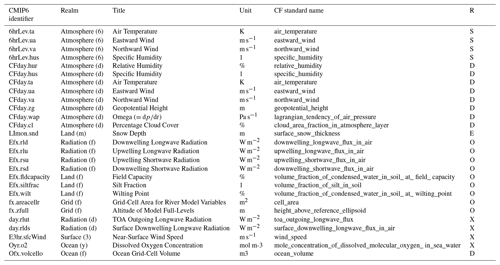
Table C1The counts of baseline climate variables in different categories. For an explanation of the high-volume category, see Table C2.
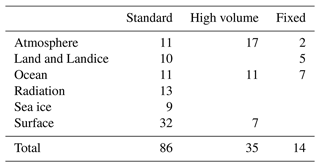
Table C2Example data volumes based on a 1° resolution model with 60 atmospheric levels and 50 oceanic levels (https://wcrp-cmip.org/cmip7-data-request-harmonised-thematic-variables/, last access: 4 December 2024). Single-precision data storage without compression. A variable is considered high volume (italic) if 10 000 years of simulations generate more than 1500 GB of data.
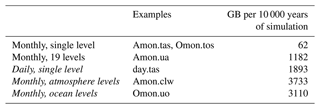
You are invited to participate in a DATA REQUEST exercise on variable prioritisation (13 April 2022).
Greetings from the newly established CMIP International Project Office. As part of the CMIP community, you are invited to participate in a DATA REQUEST exercise on variable prioritisation. We are helping the WGCM Infrastructure Panel (WIP) to implement this activity.
If you would like to participate in this activity, please complete the form below (https://forms.office.com/r/qCNtTfywqN, last access: 14 March 2025) before 11:00 UTC on 21 April 2022. This will enable you to
-
express interest in attending an online workshop in May,
-
express interest in being a paper author or reviewer, and
-
contribute your thoughts on the methodological approach (the questions are based on reviewing this list of parameters, indicating how you feel about the number prioritised, the methodology proposed, any additional quantitative criteria you feel should be taken into account in the shortlisting, any science- or impact-based prioritisation issues for consideration, and any thoughts you have on alternative methodological approaches to prioritisation).
If you have any questions about this or would like to reach out to the new CMIP IPO about anything else, please do contact myself or the CMIP IPO director Eleanor O'Rourke (eleanor.orourke@ext.esa.int).
Form introduction
CMIP DATA REQUEST variable prioritisation: event registration, input, and author expression of interest (EoI)
CMIP has expanded and now has a substantial range of communities, all with their own specialised requirements. The WIP is aware that there are too many variables being listed as top priority and that conflicts are emerging between what the data centres and data users (including intermediary platforms such as C3S) would consider highest priority.
The Data Request function of the WIP wishes to address the immediate challenge of establishing an agreed-upon variable prioritisation methodology from the CMIP modelling community and some means of giving authority to “priority = 1” statements, which was a community intention discussed at WGCM 2019 in Barcelona. It is envisaged that these prioritised variables will form a baseline set of variables for exchange of climate model data, following FAIR (findability, accessibility, interoperability, and reusability) data and open-science principles. The intention is to publish these as a Geoscientific Model Development (GMD) paper.
The CMIP community is therefore invited to provide input to, and consider self-nomination for authorship of, a paper setting out an appropriate methodology for prioritising variables that could be considered a baseline set for exchange of climate model data in any intercomparison project, in accordance with FAIR data and open-science principles. There are three sections to this survey: it will take you 5–10 min to complete and longer if you wish to provide detailed responses:
-
Sect. 1. Your details (required).
-
Sect. 2. Workshop preference and EoI for paper roles (author or reviewer) (required).
-
Sect. 3. Your thoughts on the methodological approach (optional) – these will be used to underpin workshop discussions.
This participation form has been developed by the CMIP IPO hosted by the ESA Climate Office in consultation with the WCRP WGCM Infrastructure Panel. This workstream is being led by Martin Juckes (UKRI-STFC), working alongside Charlotte Pascoe (NCAS/CEDA) and Alison Parent (CEDA). If you have any problems completing this form or accessing the links, please contact Briony Turner at briony.turner@ext.esa.int.
This participation form has been issued by the CMIP IPO to the modelling centre leads, data request leads, and MIP chairs and can be shared more widely if you are aware of others who might wish to contribute to this activity.
Please note that this Registration & Author Expression of Interest form expired at 18:00 UTC on 26 April 2022. However, you can still share your thoughts on the methodological approach and indicate which workshop you would like to attend by 18:00 UTC on 6 May 2022.
This activity is supported by the CMIP IPO and is made possible by funding from IS-ENES3 as part of the European Union's Horizon 2020 research and innovation programme under grant no. 82408.
The pressure levels defined in the CMIP6 Data Request and brought into the ESM-BCV list are given below:
-
19 pressure levels (plev19) – 100 000, 92 500, 85 000, 70 000, 60 000, 50 000, 40 000, 30 000, 25 000, 20 000, 15 000, 10 000, 7000, 5000, 3000, 2000, 1000, 500, and 100 Pa; and
-
8 pressure levels (plev8) – 100 000, 85 000, 70 000, 50 000, 25 000, 10 000, 5000, and 1000 Pa.
The usage and the range of levels may be modified in CMIP7 following detailed discussion of scientific requirements, led by the atmosphere theme of the CMIP AR7 Fast Track Data Request (see https://wcrp-cmip.org/cmip7/cmip7-data-request/public-consultation/, last access: 4 December 2024).
The python script used to harvest information from the ESGF for Fig. 4 is available in Juckes (2025a) (https://doi.org/10.5281/zenodo.15190399). The prioritisation data are available as an Excel workbook in Juckes (2025b) (https://doi.org/10.5281/zenodo.14701274).
Conceptualisation: MJ. Funding acquisition: not applicable. Methodology: MJ, BT, and EO. Project administration: BT, EO, and BD. Writing and original draft preparation: all. Revision and verification of ESGF download statistics: FA and AN. Writing, review, and editing: all.
At least one of the (co-)authors is a member of the editorial board of Geoscientific Model Development. The peer-review process was guided by an independent editor, and the authors also have no other competing interests to declare.
Publisher’s note: Copernicus Publications remains neutral with regard to jurisdictional claims made in the text, published maps, institutional affiliations, or any other geographical representation in this paper. While Copernicus Publications makes every effort to include appropriate place names, the final responsibility lies with the authors.
The work developing the baseline variables was coordinated and led by Martin Juckes (UKRI-STFC). Its implementation was supported by the CMIP International Project Office hosted by the European Space Agency. All the figures have been commissioned by the CMIP International Project Office and are under a Creative Commons Attribution 4.0 International licence. The ESGF download statistics are provided by the Euro-Mediterranean Center on Climate Change of the CMCC Foundation. Thanks go to John Dunne for chairing the discussions leading to the final consensus on the published list. We gratefully acknowledge the valuable feedback provided in reviews by Claire Macintosh and Young Ho Kim and in comments by Anne Marie Treguier, Isla Simpson, Alistair Adcroft, Baylor Fox-Kemper, Nathan Gillett, Christopher Danek, Gaëlle Rigoudy, and Gavin A. Schmidt.
The work developing the baseline variables has been made possible by funding from IS-ENES3 as part of the European Union's Horizon 2020 research and innovation programme under grant no. 824084. The work of Paul J. Durack and Karl E. Taylor was performed under the auspices of the U.S. Department of Energy by Lawrence Livermore National Laboratory (LLNL) under contract no. DE-AC52-07NA27344. It is a contribution to the science portfolio of the U.S. Department of Energy, Office of Science, Earth and Environmental Systems Sciences Division, Regional and Global Model Analysis Program (LLNL release no. LLNL-JNRL-853910). Hyungjun Kim was supported by a National Research Foundation of Korea (NRF) grant funded by the South Korean government (MSIT) (grant no. 2021H1D3A2A03097768). Martin Juckes was supported by UK Research and Innovation (grant no. NE/Y001729/1).
This paper was edited by Tatiana Egorova and reviewed by Claire Macintosh and Young Ho Kim.
Buontempo, C., Burgess, S. N., Dee, D., Pinty, B., Thépaut, J.-N., Rixen, M., Almond, S., Armstrong, D., Brookshaw, A., López-Alos, A., Bell, B., Bergeron, C., Cagnazzo, C., Comyn-Platt, E., Damasio-Da-Costa, E., Guillory, A., Hersbach, H., Horányi, A., Nicolas, J., Obregon, A., Ramos, E. P., Raoult, B., Muñoz-Sabater, J., Simmons, A., Soci, C., Suttie, M., Vamborg, F., Varndell, J., Vermoote, S., Yang, X., and Garcés de Marcilla, J.: The Copernicus Climate Change Service: Climate Science in Action, B. Am. Meteorol. Soc., 103, E2669–E2687, https://doi.org/10.1175/BAMS-D-21-0315.1, 2022.
Craig, M. T., Wohland, J., Stoop, L. P., Kies, A., Pickering, B., Bloomfield, H. C., Browell, J., De Felice, M., Dent, C. J., Deroubaix, A.,nFrischmuth, F., Gonzalez, P. L., Grochowicz, A., Gruber, K., Härtel, P., Kittel, M., Kotzur, L., Labuhn, I., Lundquist, J. K., Pflugradt, N., van der Wiel, K., Zeyringer, M., and Brayshaw, D. J.: Overcoming the disconnect between energy system and climate modeling, Joule, 6, 1405–1417, https://doi.org/10.1016/j.joule.2022.05.010, 2022.
Dubus, L., Brayshaw, D. J., Huertas-Hernando, D., Radu, D., Sharp, J., Zappa, W., and Stoop, L. P.: Towards a future-proof climate database for European energy system studies, Environ. Res. Lett., 17, 121001, https://doi.org/10.1088/1748-9326/aca1d3, 2022.
Durack, P. J., Taylor, K. E., Mizielinski, M., Doutriaux, C., Nadeau, D., and Juckes, M.: WCRP-CMIP/CMIP6_CVs: 6.2.58.68, Zenodo [code], https://doi.org/10.5281/zenodo.12197151, 2024.
Eyring, V., Bony, S., Meehl, G. A., Senior, C. A., Stevens, B., Stouffer, R. J., and Taylor, K. E.: Overview of the Coupled Model Intercomparison Project Phase 6 (CMIP6) experimental design and organization, Geosci. Model Dev., 9, 1937–1958, https://doi.org/10.5194/gmd-9-1937-2016, 2016.
Fiore, S., Nassisi, P., Nuzzo, A., Mirto, M., Cinquini, L., Williams, D., and Aloisio, G.: A climate change community gateway for data usage & data archive metrics across the earth system grid federation, CEUR workshop proceedings, Vol. 2975, CEUR-WS, https://iris.unitn.it/handle/11572/329932 (last access: 14 March 2025), 2021.
Flato, G. M.: Earth system models: An overview, WIREs Climate Change, 2, 783–800, https://doi.org/10.1002/wcc.148, 2011.
Griffies, S. M., Danabasoglu, G., Durack, P. J., Adcroft, A. J., Balaji, V., Böning, C. W., Chassignet, E. P., Curchitser, E., Deshayes, J., Drange, H., Fox-Kemper, B., Gleckler, P. J., Gregory, J. M., Haak, H., Hallberg, R. W., Heimbach, P., Hewitt, H. T., Holland, D. M., Ilyina, T., Jungclaus, J. H., Komuro, Y., Krasting, J. P., Large, W. G., Marsland, S. J., Masina, S., McDougall, T. J., Nurser, A. J. G., Orr, J. C., Pirani, A., Qiao, F., Stouffer, R. J., Taylor, K. E., Treguier, A. M., Tsujino, H., Uotila, P., Valdivieso, M., Wang, Q., Winton, M., and Yeager, S. G.: OMIP contribution to CMIP6: experimental and diagnostic protocol for the physical component of the Ocean Model Intercomparison Project, Geosci. Model Dev., 9, 3231–3296, https://doi.org/10.5194/gmd-9-3231-2016, 2016.
Gerber, E. P. and Manzini, E.: The Dynamics and Variability Model Intercomparison Project (DynVarMIP) for CMIP6: assessing the stratosphere–troposphere system, Geosci. Model Dev., 9, 3413–3425, https://doi.org/10.5194/gmd-9-3413-2016, 2016.
Guterres, A.: Planet Hurtling towards Hell of Global Heating, Secretary-General Warns Austrian World Summit, Urging Immediate Emissions Cuts, Fair Climate Funding, UN Press Release, https://press.un.org/en/2023/sgsm21799.doc.htm (last access: 14 March 2025), 2023.
Gutowski Jr., W. J., Giorgi, F., Timbal, B., Frigon, A., Jacob, D., Kang, H.-S., Raghavan, K., Lee, B., Lennard, C., Nikulin, G., O'Rourke, E., Rixen, M., Solman, S., Stephenson, T., and Tangang, F.: WCRP COordinated Regional Downscaling EXperiment (CORDEX): a diagnostic MIP for CMIP6, Geosci. Model Dev., 9, 4087–4095, https://doi.org/10.5194/gmd-9-4087-2016, 2016.
Haarsma, R. J., Roberts, M. J., Vidale, P. L., Senior, C. A., Bellucci, A., Bao, Q., Chang, P., Corti, S., Fučkar, N. S., Guemas, V., von Hardenberg, J., Hazeleger, W., Kodama, C., Koenigk, T., Leung, L. R., Lu, J., Luo, J.-J., Mao, J., Mizielinski, M. S., Mizuta, R., Nobre, P., Satoh, M., Scoccimarro, E., Semmler, T., Small, J., and von Storch, J.-S.: High Resolution Model Intercomparison Project (HighResMIP v1.0) for CMIP6, Geosci. Model Dev., 9, 4185–4208, https://doi.org/10.5194/gmd-9-4185-2016, 2016.
Hohenegger, C., Korn, P., Linardakis, L., Redler, R., Schnur, R., Adamidis, P., Bao, J., Bastin, S., Behravesh, M., Bergemann, M., Biercamp, J., Bockelmann, H., Brokopf, R., Brüggemann, N., Casaroli, L., Chegini, F., Datseris, G., Esch, M., George, G., Giorgetta, M., Gutjahr, O., Haak, H., Hanke, M., Ilyina, T., Jahns, T., Jungclaus, J., Kern, M., Klocke, D., Kluft, L., Kölling, T., Kornblueh, L., Kosukhin, S., Kroll, C., Lee, J., Mauritsen, T., Mehlmann, C., Mieslinger, T., Naumann, A. K., Paccini, L., Peinado, A., Praturi, D. S., Putrasahan, D., Rast, S., Riddick, T., Roeber, N., Schmidt, H., Schulzweida, U., Schütte, F., Segura, H., Shevchenko, R., Singh, V., Specht, M., Stephan, C. C., von Storch, J.-S., Vogel, R., Wengel, C., Winkler, M., Ziemen, F., Marotzke, J., and Stevens, B.: ICON-Sapphire: simulating the components of the Earth system and their interactions at kilometer and subkilometer scales, Geosci. Model Dev., 16, 779–811, https://doi.org/10.5194/gmd-16-779-2023, 2023.
International Bureau of Weights and Measures: The International System of Units, 9th edn., https://www.bipm.org/documents/20126/41483022/SI-Brochure-9.pdf (last access: 1 November 2024), 2019.
IPCC: Summary for Policymakers, in: Climate Change 2023: Synthesis Report.Contribution of Working Groups I, II and III to the Sixth Assessment Report of the Intergovernmental Panel on Climate Change, edited by: Core Writing Team, Lee, H., and Romero, J., IPCC, Geneva, Switzerland, 34 pp., https://doi.org/10.59327/IPCC/AR6-9789291691647.001, 2023.
Jones, C. D., Arora, V., Friedlingstein, P., Bopp, L., Brovkin, V., Dunne, J., Graven, H., Hoffman, F., Ilyina, T., John, J. G., Jung, M., Kawamiya, M., Koven, C., Pongratz, J., Raddatz, T., Randerson, J. T., and Zaehle, S.: C4MIP – The Coupled Climate–Carbon Cycle Model Intercomparison Project: experimental protocol for CMIP6, Geosci. Model Dev., 9, 2853–2880, https://doi.org/10.5194/gmd-9-2853-2016, 2016.
Juckes, M.: Style Guide for Variable Titles in CMIP6 (0.01), Zenodo, https://doi.org/10.5281/zenodo.2480853, 2018.
Juckes, M.: CMIP Data Request Schema 2.0, Zenodo, https://doi.org/10.5281/zenodo.4287148, 2020.
Juckes, M.: Code to review the ESGF CMIP6 index, Zenodo [code], https://doi.org/10.5281/zenodo.15190399, 2025a.
Juckes, M.: WCRP Baseline Variables – MIP Prioritisation raw data (v1.4), Zenodo [data set], https://doi.org/10.5281/zenodo.14701274, 2025b.
Juckes, M., Taylor, K. E., Durack, P. J., Lawrence, B., Mizielinski, M. S., Pamment, A., Peterschmitt, J.-Y., Rixen, M., and Sénési, S.: The CMIP6 Data Request (DREQ, version 01.00.31), Geosci. Model Dev., 13, 201–224, https://doi.org/10.5194/gmd-13-201-2020, 2020.
Kershaw, P., Abdulla, G., Ames, S., and Evans, B.: ESGF Future Architecture Report, Zenodo, https://doi.org/10.5281/zenodo.3928223, 2020.
Klein Tank, A., Zwiers, F., and Zhang, X.: Guidelines on Analysis of Extremes in a Changing Climate in Support of Informed Decisions for Adaptation, World Meteorological Organization, https://www.ecad.eu/documents/WCDMP_72_TD_1500_en_1.pdf (last access: 14 March 2025), 2009.
Lee, J., Gleckler, P., Ordonez, A., Ahn, M.-S., Ullrich, P., Vo, T., Boutte, J., Doutriaux, C., Durack, P., Shaheen, Z., Muryanto, L., Painter, J., and Krasting, J.: PCMDI/pcmdi_metrics: PMP Version 2.5.1 (v2.5.1), Zenodo [code], https://doi.org/10.5281/zenodo.7231033, 2022.
Lee, J., Gleckler, P. J., Ahn, M.-S., Ordonez, A., Ullrich, P. A., Sperber, K. R., Taylor, K. E., Planton, Y. Y., Guilyardi, E., Durack, P., Bonfils, C., Zelinka, M. D., Chao, L.-W., Dong, B., Doutriaux, C., Zhang, C., Vo, T., Boutte, J., Wehner, M. F., Pendergrass, A. G., Kim, D., Xue, Z., Wittenberg, A. T., and Krasting, J.: Systematic and objective evaluation of Earth system models: PCMDI Metrics Package (PMP) version 3, Geosci. Model Dev., 17, 3919–3948, https://doi.org/10.5194/gmd-17-3919-2024, 2024.
Magagna, B., Schinder, S., Stoica, M., Moncoiffe, G., Devaraju, A., and Pamment, A.: I-ADOPT Framework ontology, https://w3id.org/iadopt/ont/1.0.3 (last access: 1 November 2024), 2023.
Mauzey, C., Doutriaux, C., Nadeau, D., Taylor, K. E., Durack, P. J., Betts, E., Cofino, A. S., Florek, P., Hogan, E., Kettleborough, J., Nicholls, Z., Ogochi, K., Rodríguez González, J. M., Seddon, J., Wachsmann, F., and Weigel, T.: The Climate Model Output Rewriter (CMOR) (Version 3.9.0), Zenodo [code], https://doi.org/10.5281/zenodo.592733, 2024.
Meehl, G. A., Boer, G. J., Covey, C., Latif, M., and Stouffer, R. J.: Intercomparison makes for a better climate model, Eos Transactions American Geophysical Union, 78, 445, https://doi.org/10.1029/97EO00276, 1997.
Meehl, G. A., Boer, G. J., Covey, C., Latif, M., and Stouffer, R. J.: The Coupled Model Intercomparison Project (CMIP), B. Am. Meteorol. Soc., 81, 313–318, 2000.
Meehl, G. A., Covey, C., Taylor, K. E., Delworth, T., Stouffer, R. J., Latif, M., McAvaney, B., and Mitchell, J. F. B.: The WCRP CMIP3 Multimodel Dataset: A New Era in Climate Change Research, B. Am. Meteorol. Soc., 88, 1383–1394, 2007.
Notz, D., Jahn, A., Holland, M., Hunke, E., Massonnet, F., Stroeve, J., Tremblay, B., and Vancoppenolle, M.: The CMIP6 Sea-Ice Model Intercomparison Project (SIMIP): understanding sea ice through climate-model simulations, Geosci. Model Dev., 9, 3427–3446, https://doi.org/10.5194/gmd-9-3427-2016, 2016.
O'Rourke, E.: CMIP6 Community Survey Results, Zenodo, https://doi.org/10.5281/zenodo.8113057, 2023.
O'Rourke, E. and Turner, B. (Eds.): Priority variables for evaluation and exploitation of WCRP climate simulations workshop report, Top priority variables community workshop, online, 12 and 17 May 2022, https://doi.org/10.59555/TUOC4428, 2022.
O'Rourke, E., Turner, B., and Dingley, B. Baseline variables for climate model intercomparison and evaluation – Community survey summary report, Zenodo, https://doi.org/10.5281/zenodo.8248691, 2023.
Petrie, R., Denvil, S., Ames, S., Levavasseur, G., Fiore, S., Allen, C., Antonio, F., Berger, K., Bretonnière, P.-A., Cinquini, L., Dart, E., Dwarakanath, P., Druken, K., Evans, B., Franchistéguy, L., Gardoll, S., Gerbier, E., Greenslade, M., Hassell, D., Iwi, A., Juckes, M., Kindermann, S., Lacinski, L., Mirto, M., Nasser, A. B., Nassisi, P., Nienhouse, E., Nikonov, S., Nuzzo, A., Richards, C., Ridzwan, S., Rixen, M., Serradell, K., Snow, K., Stephens, A., Stockhause, M., Vahlenkamp, H., and Wagner, R.: Coordinating an operational data distribution network for CMIP6 data, Geosci. Model Dev., 14, 629–644, https://doi.org/10.5194/gmd-14-629-2021, 2021.
Petrie, R., Eggleton, F., and Juckes, M.: CF Compliance errors in the CMIP6 archive, Zenodo [data set], https://doi.org/10.5281/zenodo.12820690, 2024.
Program for Climate Model Diagnosis and Intercomparison (PCMDI): Standard Output, Lawrence Livermore National Laboratory, https://pcmdi.llnl.gov/mips/cmip5/docs/standard_output.xls (last access: 3 July 2024), 2013.
Ruane, A. C., Teichmann, C., Arnell, N. W., Carter, T. R., Ebi, K. L., Frieler, K., Goodess, C. M., Hewitson, B., Horton, R., Kovats, R. S., Lotze, H. K., Mearns, L. O., Navarra, A., Ojima, D. S., Riahi, K., Rosenzweig, C., Themessl, M., and Vincent, K.: The Vulnerability, Impacts, Adaptation and Climate Services Advisory Board (VIACS AB v1.0) contribution to CMIP6, Geosci. Model Dev., 9, 3493–3515, https://doi.org/10.5194/gmd-9-3493-2016, 2016.
Stockhause, M., Höck, H., Toussaint, F., and Lautenschlager, M.: Quality assessment concept of the World Data Center for Climate and its application to CMIP5 data, Geosci. Model Dev., 5, 1023–1032, https://doi.org/10.5194/gmd-5-1023-2012, 2012.
Tan, J., Duan, Q., Xiao, C., He, C., and Yan, X.: A brief review of the coupled human-Earth system modeling: Current state and challenges, The Anthropocene Review, 10, 664–684, https://doi.org/10.1177/20530196221149121, 2023.
Taylor, K. E., Stouffer, R. J., and Meehl, G. A.: An Overview of CMIP5 and the Experiment Design, B. Am. Meteorol. Soc., 93, 485–498, 2012.
Taylor, K. E., Juckes, M., Balaji, V., Cinquini, L., Denvil, S., Durack, P. J., Elkington, M., Guilyardi, E., Kharin, S., Lautenschlager, M., Lawrence, B., Nadeau, D., and Stockhause, M.: CMIP6 Model Output Metadata Requirements, Data Reference Syntax (DRS) and Controlled Vocabularies (CVs), Zenodo, https://doi.org/10.5281/zenodo.12768887, 2018.
Touzé-Peiffer, L., Barberousse, A., and Le Treut, H.: The Coupled Model Intercomparison Project: History, uses, and structural effects on climate research, WIREs Clim. Change, 11, e648, https://doi.org/10.1002/wcc.648, 2020.
United Nations: Paris Agreement, United Nations Treaty Collection, Chapter XXVII 7. d, adopted 2015-12-12 and inforce 2016-11-04, United Nations, Treaty Series, Vol. 3156, p. 79, https://treaties.un.org/doc/Treaties/2016/02/20160215%2006-03%20PM/Ch_XXVII-7-d.pdf (last access: 6 March 2025), 2015.
van den Hurk, B., Kim, H., Krinner, G., Seneviratne, S. I., Derksen, C., Oki, T., Douville, H., Colin, J., Ducharne, A., Cheruy, F., Viovy, N., Puma, M. J., Wada, Y., Li, W., Jia, B., Alessandri, A., Lawrence, D. M., Weedon, G. P., Ellis, R., Hagemann, S., Mao, J., Flanner, M. G., Zampieri, M., Materia, S., Law, R. M., and Sheffield, J.: LS3MIP (v1.0) contribution to CMIP6: the Land Surface, Snow and Soil moisture Model Intercomparison Project – aims, setup and expected outcome, Geosci. Model Dev., 9, 2809–2832, https://doi.org/10.5194/gmd-9-2809-2016, 2016.
WCRP Working Group on Coupled Modeling: Report of the 22nd session of the WCRP Working Group on Coupled Modeling (WGCM), vol. 14 of WCRP Report, World Climate Research Programme (WCRP), Geneva, Switzerland, https://www.wcrp-climate.org/WCRP-publications/2019/WCRP-Report-No14-2019-WGCM22.pdf (last access: 6 March 2025), 2019.
Weigel, K., Bock, L., Gier, B. K., Lauer, A., Righi, M., Schlund, M., Adeniyi, K., Andela, B., Arnone, E., Berg, P., Caron, L.-P., Cionni, I., Corti, S., Drost, N., Hunter, A., Lledó, L., Mohr, C. W., Paçal, A., Pérez-Zanón, N., Predoi, V., Sandstad, M., Sillmann, J., Sterl, A., Vegas-Regidor, J., von Hardenberg, J., and Eyring, V.: Earth System Model Evaluation Tool (ESMValTool) v2.0 – diagnostics for extreme events, regional and impact evaluation, and analysis of Earth system models in CMIP, Geosci. Model Dev., 14, 3159–3184, https://doi.org/10.5194/gmd-14-3159-2021, 2021.
WGCM Climate Simulation Panel: IPCC Standard Output from Coupled Ocean-Atmosphere GCMs, Program for Climate Model Diagnosis and Intercomparison, https://pcmdi.llnl.gov/mips/cmip3/variableList.html (last access: 5 December 2024), 2007.
World Meteorological Organisation: The 2022 GCOS Implementation Plan, WMO GCOS 244, World Meteorological Organisation, https://library.wmo.int/idurl/4/58104 (last access: 5 December 2024), 2022a.
World Meteorological Organisation: GCOS-22: The 2022 GCOS ECVs Requirements, WMO GCOS-245, World Meteorological Organisation, https://library.wmo.int/idurl/4/58111 (last access: 5 December 2024), 2022b.
World Meteorological Organisation: Manual on Codes – International Codes, Volume I.2, Annex II to the WMO Technical Regulations, World Meteorological Organisation, https://library.wmo.int/idurl/4/35625 (last access: 5 December 2024), 2023.
The analysis is based on titles and abstracts of 5152 papers identified from Web of Science that either cite Eyring et al. (2016) or mention CMIP6 in the title or abstract. The clustering is based on terms which occur in at least 100 papers.
GRIB (General Regularly distributed Information in Binary form) is the WMO standard for operational exchange of meteorological data (World Meteorological Organisation, 2023).
This discussion is based on information from the Earth System Grid Federation (ESGF) index, https://esgf.llnl.gov/ (last access: 24 August 2023).
The all-forcing experiment of the recent past (historical) in CMIP is designed to enable the evaluation of model simulations against present climate and observed climate change.
Data publication for CMIP6 is still ongoing, but the pattern of gaps in the archive persists as data volumes expand.
The prioritisation of variables in the CMIP6 Data Request was always conditional on an objective such as support for a specific MIP. For example, a variable might be priority 1 for SIMIP (Seaice MIP) but of no interest for LUMIP (Land Use MIP).
http://esgf-ui.cmcc.it/esgf-dashboard-ui/ (last access: 13 March 2025). The download statistics are from the server log files which record successful responses to requests received over HTTP, including requests from scripts and from browsers. Some usage is not monitored, such as multiple users accessing a shared processing space. Open access has been prioritised at the expense of comprehensive usage information, but the majority of users are still accessing data via the mechanisms which do get tracked.
These figures are for August 2023. Figures taken in March 2022 were very similar, with 28 % of the models providing 28 % or more of the priority-1 variables and 90 % of the models providing 7.8 % or more. Two models provided more than 50 %.
The process for extending the list was launched by the CMIP panel decision “G1 [Gateway 1] DR Strategic Approach” (https://airtable.com/shrIAHOuVw8ktdoe1, last access: 14 March 2025, items 9 and 10, approved 24 July 2023) and announced in December 2023 (https://wcrp-cmip.org/cmip7-data-request-harmonised-thematic-variables/, last access: 14 March 2025).
The figure for the number of ocean levels here is based on what was submitted to the CMIP6 archive. Some modelling centres submitted data at a lower resolution than the full model grid.
Launched November 2024: https://wcrp-cmip.org/event/ref-project-launch/ (last access: 13 December 2024).
- Abstract
- Introduction
- Process and methodology
- The form and role of the baseline list
- Results
- Conclusion
- Appendix A: The baseline climate variables
- Appendix B: Variables removed from and added to the shortlist
- Appendix C: Summary tables
- Appendix D: Invitation to participate
- Appendix E: Pressure levels for atmospheric variables
- Code and data availability
- Author contributions
- Competing interests
- Disclaimer
- Acknowledgements
- Financial support
- Review statement
- References
- Abstract
- Introduction
- Process and methodology
- The form and role of the baseline list
- Results
- Conclusion
- Appendix A: The baseline climate variables
- Appendix B: Variables removed from and added to the shortlist
- Appendix C: Summary tables
- Appendix D: Invitation to participate
- Appendix E: Pressure levels for atmospheric variables
- Code and data availability
- Author contributions
- Competing interests
- Disclaimer
- Acknowledgements
- Financial support
- Review statement
- References