the Creative Commons Attribution 4.0 License.
the Creative Commons Attribution 4.0 License.
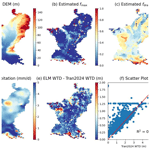
Subsurface hydrological controls on the short-term effects of hurricanes on nitrate–nitrogen runoff loading: a case study of Hurricane Ida using the Energy Exascale Earth System Model (E3SM) Land Model (v2.1)
Hoang Viet Tran
L. Ruby Leung
When the nutrient level in the soil surpasses vegetation demand, nutrient losses due to surface runoff and subsurface leaching are the major reasons for the deterioration of water quality. The lower Mississippi River basin (LMRB) is one of the sub-basins that deliver the highest nitrogen loads to the Gulf of Mexico. Potential changes in episodic events induced by hurricanes may exacerbate water quality issue in the future. However, uncertainties in modeling the hydrologic response to hurricanes may limit the modeling of nutrient losses during such events. Using a machine learning approach, we calibrated the land component of the Energy Exascale Earth System Model (E3SM), or ELM, version 2.1, based on the water table depth (WTD) of a calibrated 3D subsurface hydrology model. While the overall performance of the calibrated ELM is satisfactory, some discrepancies in WTD remain in slope areas with low precipitation due to the missing lateral flow process in ELM. Simulations including biogeochemistry performed using ELM with and without model calibration showed important influences of soil hydrology, precipitation intensity, and runoff parameterization on the magnitude of nitrogen runoff loss and the leaching pathway. Despite such sensitivities, both ELM simulations produced reduced WTD and increased runoff and accelerated nitrate–nitrogen runoff loading during Hurricane Ida in August 2021, consistent with the observations. With observations suggesting more pronounced effects of Hurricane Ida on nitrogen runoff than the simulations, we identified factors for model improvement to provide a useful tool for studying hurricane-induced nutrient losses in the LMRB region.
- Article
(3695 KB) - Full-text XML
- BibTeX
- EndNote
Tropical cyclones are projected to be more intense and potentially make more frequent landfall in some coastal regions in the future due to global warming (Knutson et al., 2020; Pérez-Alarcón et al., 2023; Balaguru et al., 2023). Hurricanes can cause widespread, acute disturbances for coastal aquatic and terrestrial ecosystems (Valiela et al., 1998). Besides catastrophic flooding, enhanced nutrient input coupled with increased runoff were often observed as a result of the heavy precipitation associated with landfalling hurricanes in coastal regions. For instance, 5 d after Hurricane Katrina made landfall in August 2005, the mean bay-wide nitrate concentration increased by 5.2-fold over the pre-hurricane levels in Biscayne Bay, Florida (Zhang et al., 2009). In a forested watershed draining into Chesapeake Bay, Hurricane Irene in August 2011 caused an increase in total nitrogen on the rising limb of the storm compared to the baseflow levels (Vidon et al., 2018). High discharge due to Hurricane Irene in 2011 also resulted in high nutrient loading to Newark Bay in northern New Jersey (Nie et al., 2023). The loss of vegetation, attributed to Hurricane Hugo, led to a 108 %–154 % increase in exported nutrients primarily due to increased outflow during the hurricane (Wilson et al., 2006). In coastal North Carolina, nutrient loadings coincided with the increases in freshwater discharge associated with recent tropical storms (Paerl et al., 2020). Additionally, a model simulation suggests that immediate surges of heavy precipitation associated with hurricanes accelerate nitrogen export more than the long-term average (Sun et al., 2022).
Besides the episodic influence of hurricanes in coastal regions, riverine nitrogen (N) loading from agricultural lands upstream can lead to significant soil fertility depletion and degradation of water quality in downstream aquatic ecosystems (Li et al., 2022). Excessive nutrient loads can contribute to eutrophication, leading to adverse effects on aquatic ecosystems and water quality (Carpenter et al., 1998). For instance, excessive nutrient loading from cropland in the Mississippi River basin is a significant contributing factor to the formation of the hypoxic zone in the northern Gulf of Mexico (Ritter and Chitikela, 2020). Assessing the immediate and long-term impact of hurricanes on water quality in the affected ecosystems is challenging due to logistical constraints associated with sampling during these events (Filippino et al., 2017). A thorough understanding of the mechanisms governing nutrient export from agricultural watersheds will be crucial in managing nutrient pollution, especially in light of the expected hydrological modifications due to a shifting climate (Speir et al., 2021).
Earth system models have the capability of simulating the coupled carbon and nitrogen cycles and river nitrogen (Nevison et al., 2016). However, there remains a research gap regarding the Earth system model's capability of accurately predicting the impact of hurricanes on nitrogen river loading, particularly considering the spatial heterogeneity and temporal variability of precipitation patterns associated with hurricanes. Understanding the driving mechanism behind excessive riverine N loading during hurricanes, i.e., through surface runoff or groundwater flow, is also lacking. Addressing these gaps is critical for improving our understanding of nutrient transport dynamics and enhancing the capabilities of Earth system models in regions affected by storms. This study investigates the short-time effect of hurricanes on nitrogen loading in runoff, with an emphasis on how such an effect is influenced by soil hydrology and its representations in Earth system models. Using Hurricane Ida as an example, we simulate its impact on nitrate–nitrogen runoff loading in the lower Mississippi River basin (LMRB) using the Energy Exascale Earth System Model (E3SM) Land Model (Golaz et al., 2019). We will first describe the model and calibration of the runoff parameterizations using a machine learning approach. The model is used to assess the transient effects of Hurricane Ida on hydrological and nitrogen river loading in the LMRB, which extends into the Gulf of Mexico. We will not delve into the full dynamics of nitrogen cycling within the stream due to the limitation of the model, as addressing the limitation is beyond the scope of this study. Comparison of model simulations with and without calibration provides insights into the sensitivity of the hydrologic response and nutrient losses to soil hydrology and its representations in models to inform future development needs.
2.1 Study area
The LMRB, with almost 4×106 ha of irrigated cropland spanning six southern US states, plays a crucial role in the economic landscape. The LMRB is characterized by a humid subtropical climate and significant soil and precipitation variations (Reba and Massey, 2020). For example, the LMRB experiences varying annual average rainfall ranging from approximately 1143 mm in the north to about 1524 mm in the southern coastal region (Nelson et al., 2022). Cropland is the dominant land cover type in the LMRB. Agriculture relies heavily on the Mississippi River valley alluvial aquifer to provide over 90 % of the irrigation water because a majority of precipitation falls during the winter and spring (Reba and Massey, 2020). Furthermore, within the last 20 years, the LMRB has been subjected to cyclical flooding events and declines in groundwater levels due to extreme climate events, leading to the degradation of surface water quality during flooding (Ouyang et al., 2020). The strongest hurricane to hit the LMRB on record is Hurricane Ida, which formed on 26 August 2021 and made landfall on 29 August 2021 (Fig. 1). Ida had a weak post-landfall decay rate, retaining hurricane intensity even 12 h after landfall, potentially due to high soil moisture content ahead of Ida that provided a source of atmospheric moisture and latent energy to fuel the storm (Zhu et al., 2022).
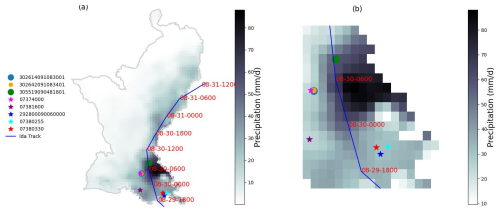
Figure 1(a) Average precipitation rate in August 2021 in the lower Mississippi River basin and the locations of observation stations along the path of Hurricane Ida (blue line). The red numbers along the path represent the timing of the 6-hourly locations of Ida along its track. Dots are water table stations, and stars are stream water quality stations. Note that stations 302614091083001, 302642091083401, and 07374000 are in close proximity to each other as shown in (b), a zoomed-in view of the southeastern subregion in (a). Please note that the date format in this figure is month-day-hour.
2.2 Data from measurements and the model simulation
In the LMRB, hydrologic data (streamflow, groundwater level) are provided by the U.S. Geological Survey (USGS) National Water Information System (NWIS), while water quality data are obtained from the Water Quality Portal (WQP). The WQP currently houses data from the USGS, Environmental Protection Agency (EPA), and U.S. Department of Agriculture (USDA).
Groundwater levels are from monitoring wells including stations 302614091083001 and 302642091083401 in East Baton Rouge Parish and station 305519090481801 in St. Helena Parish, Louisiana. Water quality monitoring locations include station 07374000, which is associated with a stream in West Baton Rouge Parish, Louisiana; 07381600, which is associated with a stream in St. Mary Parish, Louisiana; 292800090060000, which is associated with an impoundment in Jefferson Parish, Louisiana; 07380255, which is associated with a stream in Jefferson Parish, Louisiana; and 07380330, which is associated with an estuary in Lafourche Parish, Louisiana. The locations of these monitoring stations are shown in Fig. 1.
We also make use of groundwater level simulated by an integrated surface–subsurface hydrologic model from our previous effort to investigate the impacts of land cover change on the hydrologic response to Hurricane Ida in the LMRB (Tran et al., 2024). The integrated surface–subsurface hydrologic model, ELM–ParFlow, couples the Energy Exascale Earth System Model (E3SM) Land Model (ELM) and the 3D subsurface hydrology model ParFlow (Fang et al., 2022). ParFlow integrates 3D subsurface flow with overland flow using physics-based equations (Kollet and Maxwell, 2006; Maxwell, 2013; Maxwell and Miller, 2005). ELM–ParFlow was developed to address the subsurface lateral flow, or the movement of water through soils and bedrock on hillslopes, which is often missing in Earth system models that adopt 1D land surface models. The study conducted by Tran et al. (2024) employed ELM–ParFlow to investigate the relative influence of the changes in surface runoff versus evapotranspiration due to land cover change on streamflow in inland areas during hurricane events. Changes in soil hydrology due to land cover change, as examined by Tran et al. (2024), or due to model representations of soil hydrology, as to be investigated below, can lead to significant alterations in soil water, with important implications for soil biogeochemistry and nitrogen river loading.
2.3 Energy Exascale Earth System Model (E3SM) Land Model (ELM) (v2.1)
Derived from CLM4.5 (Community Land Model; Oleson et al., 2013), ELM has been enhanced with additional features, specifically addressing soil hydrology and biogeochemistry, as described in Golaz et al. (2019) and Burrows et al. (2020). Operating at the grid-cell level, ELM delineates the land surface into multiple soil layers and plant functional types. Relevant hydrological processes in ELM for this study include changes in surface water, canopy water, soil water, and snow water through interception, throughfall, canopy drip, snow accumulation and melt, infiltration, evapotranspiration, runoff, redistribution of water within the soil column, and groundwater discharge and recharge. Similar to other global land surface and Earth system models, soil hydrology in ELM is simulated through 1D columns, with no interaction between grid cells. The runoff generation in ELM is based on the simple TOPMODEL-based runoff parameterization (Niu et al., 2005).
The biogeochemical configuration of ELM, or ELM-BGC, is designed to simulate various biogeochemical processes (Burrows et al., 2020). The model simulates active plant phenology and incorporates nutrient controls on vegetation photosynthesis and includes multiple prognostic pools for carbon, nitrogen, and phosphorus within vegetation, litter, and soil organic matter. Two representations of terrestrial carbon–nitrogen–phosphorus coupling are incorporated into the model: the conceptual convergent trophic cascade (CTC) approach (Yang et al., 2016; Duarte et al., 2017) and the mechanistic equilibrium chemistry approximation (ECA) approach (Tang, 2015; Medvigy et al., 2019). Details of the approaches can be found in Burrows et al. (2020) and the citations therein. The CTC representation is the default option in the model, which is used in this study.
In ELM-BGC, mineral nitrogen transformations include competition among plant uptake for growth, nitrogen mineralization, microbial immobilization (nitrogen taken up by soil organisms, limited by the availability of mineral nitrogen), and denitrification and nitrification (Oleson et al., 2013). Mineral nitrogen that remained in the soil is subject to loss due to leaching from land to rivers and oceans. The leaching is assumed to act only on nitrate–nitrogen pools. Total nitrogen leaching includes soil nitrogen loss by surface runoff and leaching by subsurface drainage, which is represented by the equation below in a general form:
where FN is the soil nitrogen runoff or leaching, Q is the surface runoff or subsurface drainage, Nsminn is the soil mineral nitrogen, and WSsoil is the water storage in soil. The subsurface nitrogen leaching is limited on each time step to not exceed that in the soil.
2.4 ELM calibration
Because groundwater depths can significantly influence soil nutrient concentrations in various ecosystems (Hefting et al., 2004; Miao et al., 2013; Jasinski et al., 2022; Zhang et al., 2022), we first calibrated ELM based on the (ground)water table depth (WTD) simulated by ELM–ParFlow (Fang et al., 2022; Tran et al., 2024) in which lateral hydrological flow was simulated explicitly by the 3D subsurface flow and overland flow. The ELM–ParFlow simulation at 90 m grid resolution was reported in Tran et al. (2024; hence referred to as Tran2024 hereafter) and corresponds to the simulation with current land cover which has been evaluated using observed streamflow data. To capture the ELM grid-level groundwater table dynamics due to both vertical and lateral hydrological flow processes represented in Tran2024 using ELM–ParFlow, we calibrated the parameter values of the ELM parameterizations of surface and subsurface runoff as shown in the equations below.
where Rover is the surface runoff, qliq is the flux of water reaching the soil surface from the top, fmax is the maximum saturation fraction at a given grid cell, fover is a decay factor, and z∇ is the groundwater table depth.
where Rdrain is the subsurface runoff, qdrain,max is the maximum drainage rate, and fdrai is the decay factor. For a given z∇, the larger fdrai results in lower Rdrain and vice versa. By default, fmax ranges from 0.23 to 0.58 and fdrai=2.5.
To match the (ground)water table depth (WTD) in Tran2024, the total runoff is adjusted by estimating the maximum saturation fraction, fmax, and the decay factor, fdrai, for each ELM grid cell. The parameter estimation can be achieved by running multiple ELM simulations with varied fmax and fdrai parameter values to train an emulator which can then be used to find the optimal parameter values. While this could be an effective method for closely matching the observed WTD values from Tran2024, it needs a substantial number of simulations to produce the training data, which can be computationally expensive. Instead, we explored an alternative approach using machine learning, in particular, neural networks, where atmospheric forcing, topography, and the grid-level average of the WTD of Tran2024 are the predictors and fmax and fdrai are the targets. The initial training dataset of WTD was from an ELM simulation in which the values of fmax and fdrai are randomly assigned to each grid, assuming uniform distributions of fmax within the range of 0 to 1 and fdrai within the range of to 100. To estimate fmax and fdrai given the grid-level temporal average of the WTD of Tran2024 at each ELM grid, the following procedures are taken to iteratively improve the parameter estimation by updating the training dataset at each iteration:
-
Construct a neural network using the initial training dataset of the ELM WTD, atmospheric forcing, and topography as the predictors to train the model to predict fmax and fdrai corresponding to the ELM WTD as the targets. The root mean square error (RMSE) between the predicted and randomly prescribed values of fmax and fdrai within the simulation domain serves as the loss function in this training process.
-
Use the trained neural network model by replacing the ELM WTD with the Tran2024 WTD as the predictors to predict new fmax and fdrai values and use the predicted fmax and fdrai to update the ELM WTD by running ELM with the new fmax and fdrai.
-
Combine the updated ELM WTD values with the atmospheric forcing and topography as predictors and the new fmax and fdrain predicted in step (2) as targets. Merge this combined dataset with the previous dataset in steps (1) and (2) and retrain the neural network model using the updated dataset. Combining the improved data from the previous iterations can increase the model's training dataset size, enabling the model to learn and adapt to the more complex patterns to better represent the underlying relationship in the data.
-
Continuously refine the neural network model by repeating steps (2) and (3) iteratively until the predicted fmax and fdrai converge to a point where the correlation between the ELM WTD and Tran2024 WTD cannot be significantly improved from the previous iteration. When the iterations steps exceed four, only the newest five datasets are included to refine the model.
The neural network model includes two hidden layers with 128 neurons each and an output layer with 2 neurons. The ReLU (rectified linear unit) activation function is used to introduce nonlinearity. A dropout layer with a dropout rate of 25 % is inserted to mitigate overfitting by randomly deactivating neurons during training. The Adam (adaptive moment estimation) optimizer with a learning rate of is used for optimization; 80 % of the dataset is used as training data and processed in batches with a size of 128 over 3000 epochs.
2.5 ELM-BGC simulations
The atmospheric forcing, including precipitation, air temperature, shortwave and longwave radiation, wind speed, specific humidity, and atmospheric pressure, used to drive the ELM simulations is from the North American Land Data Assimilation System (NLDAS) project at grid spacing. The resolution of the ELM simulation domain is also set to . The ELM hydrology simulation was driven by forcing data spanning from 1980 to 2022. The forcing from the year 1980 was used repeatedly for the 600-year spin-up of ELM-BGC for the default model. Transient simulation of ELM-BGC was then conducted from 1980 to 2020. The result at the end of 2019 was used as an initial condition for the model comparison between the default and calibrated ELM. Land cover information was derived from the Land-Use Harmonization (LUH2) project (Hurtt et al., 2021).
In this study, we only consider nitrate–nitrogen loading in runoff due to natural terrestrial N inputs during Hurricane Ida, and crop management (e.g., fertilization and irrigation) is not considered. The impacted regions from Hurricane Ida will be examined. The selection criteria of the impacted regions include grids with accumulated precipitation exceeding 17.3 mm d−1 in the eastern part of the domain from the time of Ida's landfall until the end of August.
3.1 Calibration of ELM parameter values
When running the uncalibrated ELM, the simulated WTD ranged between 3 and 5 m, with an R2 value of −1.66 when compared to Tran2024, indicating poor performance in simulating the spatial distribution of WTD. Using the procedure described in Sect. 2.4, a satisfactory match between the WTD from the ELM simulation and Tran2024 is achieved within 10 iterations of parameter estimation using the machine learning and ELM simulations. Figure 2 shows a comparison of the fmax and fdrai used to perform the ELM simulation before the last iteration and those estimated using machine learning during the last iteration to match the WTD from the ELM simulation with the WTD from Tran2024, with R2 values of 0.87 and 0.93, respectively. The machine learning model has decent performance considering the heterogeneity in topography and precipitation (Fig. 3a and d) within the simulated domain.
In a majority of the domain, the estimated fmax values are nearly 0 (Fig. 3b) and fdrai (Fig. 3c) follows the pattern of the elevation (Fig. 3a). In the midwestern part of the domain, high elevation and precipitation lead to large fmax and low fdrai values and consequently large runoff based on Eqs. (2) and (3) in those grid cells. In the western slope area with low precipitation (indicated by the red colored area in Fig. 3d), the ELM WTD is deeper than that in Tran2024 (Fig. 3e). This occurs even when fmax is approximately 0 and fdrai is high, favoring nearly no runoff. This result suggests that these areas receive water from wetter areas at high elevations through lateral flow represented by ELM–ParFlow in Tran2024, which cannot be represented in the 1D ELM through simple calibration of the parameters related to the runoff parameterizations. The overpredictions of the WTD in other areas (Fig. 3e and f) are primarily due to the same reason.
3.2 Observation from the measurements
To examine the impact of Hurricane Ida on the soil hydrology and nitrogen in the LMRB region, we first analyze observations from measurements that are available within the region. There are significant differences in the observed WTD at stations 302614091083001 and 302642091083401, although they are in close proximity to each other. However, Hurricane Ida influenced the observed water table depth at all selected monitoring stations (Fig. 4a), albeit with a weak signal. The river stage increased by more than 3 m after Ida made landfall at stations 07374000, 07380330, and 07380255 (Fig. 4b). The nearshore stations 07381600 and 292800090060000 experienced less impact from Ida at the river stage. Water quality was also affected, with an increase in nitrogen concentration observed after Ida's landfall at both the inland station (07374000) and the nearshore station (07381600) (Fig. 4c). The diurnal variation in the nitrogen concentration at station 07381600 disappeared during Ida, indicating a direct impact from the elevated loss of nitrogen due to inland runoff. The rise in total N runoff at station 07374000 intensified following the landfall of Hurricane Ida (Fig. 4d) but gradually diminished as Ida progressed northeastward. The rise in total N runoff at 07381600 is weak. An increase in chlorophyll fluorescence was also observed during Ida near the estuary (Fig. 4e), which peaked at station 2928000090060000 shortly after Ida formed on 26 August and peaked at station 07380255 during Ida's landfall on 29 August. Overall, observations revealed that Hurricane Ida reduced the water table depth and increased the river stage, nitrogen concentration in the stream, nitrogen runoff loading, and chlorophyll fluorescence in the estuary.
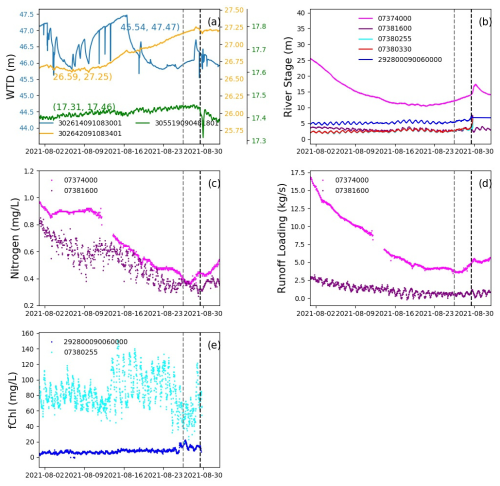
Figure 4Observed (a) water table depth, (b) river stage, (c) nitrogen concentration, (d) nitrogen runoff loading, and (e) chlorophyll fluorescence (fChl) at selected station locations shown in Fig. 1. The numbers in the parentheses in (a) are the minimum and maximum values of WTD at each location in August 2021. The colors match station colors in Fig. 1 for convenience. The three y axes in (a) display the range of WTD at each station, with a corresponding legend color. The vertical dashed grey line represents the time Hurricane Ida formed. The vertical dashed black line represents the time Hurricane Ida made landfall. Please note that the date format in this figure is year-month-day.
3.3 Hurricane impact on modeled nitrogen loading in runoff
We examined water and nitrogen runoff loading related to Hurricane Ida simulated by ELM with the default and calibrated parameters (fmax and fdrai) in the area affected by Ida. After Ida formed, lower temperatures happened concurrently with abundant precipitation (Fig. 5a and b). The WTD from the calibrated model shows more pronounced response to changes in precipitation than the WTD from the model with default parameter values (Fig. 5c). By the end of August, after Hurricane Ida's landfall, WTD is reduced by 0.05 and 0.31 m for the default model and calibrated model, respectively (Fig. 5c). As crop irrigation from groundwater pumping is not considered in the modeling, the simulated WTD shown in Fig. 5c is much shallower compared to the observations at the USGS stations (Fig. 4a) within the domain. Consistent with the rising water table, there is a notable increase in topsoil moisture evident in both models induced by the precipitation 2 d before and following the landfall of Hurricane Ida (Fig. 5d).
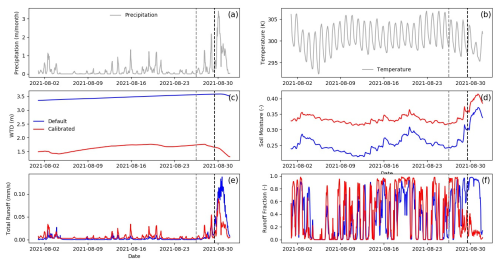
Figure 5Model inputs and comparison of simulations with default and calibrated parameters in August 2021 averaged within the Ida-affected area, including (a) precipitation, (b) air temperature, (c) water table depth (WTD), (d) average soil moisture of the topsoil layers, (e) total runoff, and (f) fraction of surface runoff in total runoff. The vertical dashed grey line represents the time Hurricane Ida formed. The vertical dashed black line represents the time Hurricane Ida made landfall. Please note that the date format in this figure is year-month-day.
During the whole period of August, the calibrated model has a shallower water table and higher soil moisture compared to the default model, which results in higher total runoff consistently in August except after Ida's landfall (Fig. 5e). The two models noticeably respond differently to the heavy rainfall produced by Ida – the default model produced a larger increase in soil moisture and higher runoff, while the calibrated model produced a larger response in WTD and a more muted response in soil moisture and runoff. These differences are also reflected in a change in the surface runoff ratio before and after Ida's landfall (Fig. 5f). The calibrated model generally shows a higher surface runoff ratio than that of the default model in August until 28 August with the arrival of the first heavy rainfall event related to Ida; this ratio drastically drops in the calibrated model, while it remains about the same in the default model. Combining the changes in the total runoff and the surface runoff ratio suggest that the much smaller total runoff in the calibrated model compared to the default model shortly before and after Ida's landfall is mainly due to a much smaller surface runoff response to heavy rainfall in the calibrated model. Notably, the calibrated model has much smaller fmax values compared to the default model, which limit the surface runoff response to heavy rainfall, while for smaller rain events, the calibrated model can still produce more surface runoff than the default model despite the smaller fmax values due to its shallower groundwater table.
Differences in the soil hydrology response between the calibrated and default models may result in differences in the nitrogen response to Hurricane Ida. Corresponding to the increase in soil moisture (Fig. 5d) and the total runoff generation (Fig. 5e) 2 d before Ida's landfall, the cumulative nitrogen (N) loss increased sharply on 28 August, followed by smaller increases as rainfall continued in the next few days (Fig. 6a). The larger increase in cumulative N loss in the default model is consistent with its higher total runoff compared to the calibrated model (Fig. 5e). Overall, nitrogen loss due to surface runoff constitutes a dominant portion of the total nitrogen loss in both models (Fig. 6b) in majority of the time in August, especially between 28–30 August under the influence of rainfall associated with Ida. Although the surface runoff ratio drops significantly in the calibrated model after 28 August, the fraction of surface N loss does not drop until after 30 August, indicating a delayed N loss response relative to the runoff changes.
During low-precipitation periods, nitrogen loss due to surface runoff constitutes a more dominant portion of total nitrogen leaching in the default model compared to the calibrated model (Fig. 6b) because of more concentrated surface soil mineral nitrogen due to drier soil in the default model. The total nitrogen leaching in August in the calibrated model is 80 % of that in the default model, largely because the calibrated model has smaller N loss during Ida's heavy rainfall events as limited by the smaller surface runoff response. Nitrogen leaching during Ida accounts for 38 % and 31 % of total nitrogen leaching in August for the default model and calibrated model, respectively.
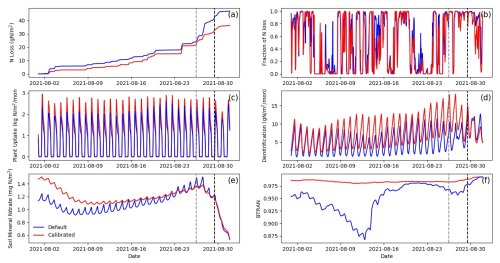
Figure 6Comparison between the default (blue) and calibrated (red) model results in August 2021 averaged within the Ida-affected area: (a) cumulative sum of total nitrogen loss; (b) fraction of surface runoff; (c) total plant nitrogen uptake; (d) total denitrification flux; (e) soil mineral nitrogen; and (f) soil water stress factor, BTRAN (non-stressed when BTRAN=1). The vertical dashed grey line represents the time Hurricane Ida formed. The vertical dashed black line represents the time Hurricane Ida made landfall.
Compared to the calibrated model, plant nitrogen uptake (Fig. 6c) and denitrification (occurs only in the anoxic fraction of soils) (Fig. 6d) in the default model are limited more by soil water, as reflected by the drier soil in the default model (Fig. 5d), resulting in a higher accumulation rate of nitrate in the soil (Fig. 6e), even though the default model simulates more runoff N loss (Fig. 6b). Denitrification declines more rapidly in the calibrated model than in the default model during Ida due to increased soil saturation. This results in a reduction in the anoxic fraction of soil, leading to a faster decrease in denitrification rates. As the cumulative nitrogen lost to runoff exhibits a notable increase 2 d after Ida formed on 26 August, the soil mineral nitrate–nitrogen drops sharply after 26 August (Fig. 6e).
The total nitrogen leaching (Fig. 6a) does not strongly correlate with precipitation (Fig. 5a) or runoff (Fig. 5e). There is a high leaching spike on 24 August in the calibrated model 2 d before Ida formed (Fig. 5e). On the previous day (23 August), the air temperature reached a maximum in August after a relatively dry period (Fig. 5b and d). Compared to the calibrated model prior to Ida forming, the dry stress (Figs. 5d and 6f) simulated by the default model caused a relatively faster increase in soil mineral nitrate–nitrogen (Fig. 6e) mainly due to lower plant nitrogen uptake and denitrification under stress (Fig. 6c).
A notable increase in nitrogen leaching loss from the simulations was observed on 24 August (Fig. 6a). A spatial examination of the variables for the calibrated model on 23 and 24 August revealed a shift in abundant precipitation towards the southeastern region near the Gulf Coast on 24 August (Fig. 7a and b). The increased runoff on 24 August (Fig. 7e), triggered by heavy precipitation, mobilized the accumulated nitrate (Fig. 7d) in the previously dry soil (Fig. 7c). This led to concentrated leaching in that area (Fig. 7f), explaining the spike in total nitrogen loss on 24 August shown in Fig. 6a. Although this event occurred before Hurricane Ida, these findings underscore the significance of considering preceding environmental conditions in understanding the hurricane impact on nitrogen leaching loss.
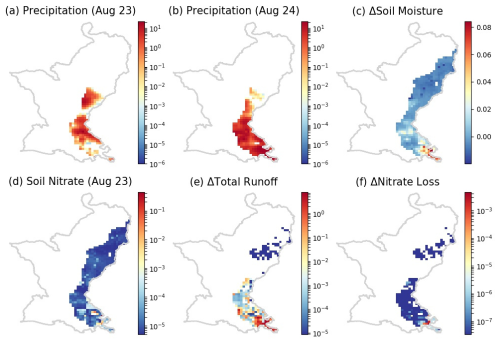
Figure 7Comparison of variables between 23 and 24 August for the calibrated model: (a) precipitation (mm d−1) on 23 August, (b) precipitation (mm d−1) on 24 August, (c) positive increase in soil moisture (m3 m−3), (d) soil nitrate–nitrogen (g N m−2) on 23 August, (e) positive increase in runoff (mm d−1), and (f) positive increase in nitrate–nitrogen leaching loss () from 23 August.
To understand the driving mechanism of nitrogen leaching loss under different soil water conditions, we selected a nearshore grid in the subdomain affected by Ida from the two simulations which have different levels of soil water as an example. At this selected grid, unlike the conditions averaged over the Ida-affected area discussed in Figs. 5 and 6, the calibrated model happens to have drier soil (Fig. 8b) compared to the default model (Fig. 8a). As a result of the smaller rate of denitrification and plant uptake as a soil nitrate–nitrogen sink and the reduced source from nitrogen fixation due to water stress, there is more soil nitrate accumulation in the calibrated model (Fig. 8d). Soil water affects not only the competition for nitrogen between the plant and soil microbes but also the vertical transport of soil mineral nitrogen. Compared to the default model, drier soil from the calibrated model due to a larger fmax at this grid favors higher surface runoff before Hurricane Ida formed. Drier soil also causes a slightly warmer temperature. It is not shown because the contrast is not visually discernible. This warmer temperature can partially offset the deceleration of soil organic matter decomposition caused by dry soil conditions. After Ida-induced precipitation in the area, infiltration pushed the accumulated nitrogen further down to the deeper soil layers (Fig. 8d). More nitrogen from the calibrated model leached through the pathway of subsurface runoff (Fig. 8f) during Ida, even though the subsurface runoff is far less than that of the default model.
4.1 Potential applications of the iterative parameterization approach
The iterative parameterization approach presented in this study demonstrates a promising method for improving subsurface hydrological simulations and can be easily extended to other watersheds. The use of a surrogate model to estimate model parameters reduces computational costs while maintaining accuracy, allowing for efficient iterative refinement of the simulation results. This approach can be particularly beneficial for watersheds with complex hydrogeological characteristics, where traditional calibration methods may be computationally prohibitive or require extensive datasets.
Successful application of this method relies on prior knowledge of the most sensitive and important parameters to include in the parameterization process. Without a clear understanding of which parameters have the greatest impact on model predictions, the iterative approach may not effectively reduce uncertainty or improve simulation accuracy. Additionally, identifying key parameters can help to avoid over-parameterization, where the inclusion of too many parameters can lead to overfitting and degradation of predictive performance.
4.2 Importance of soil hydrology on nitrogen leaching
By conducting two simulations with ELM using default and calibrated parameters that influence surface and subsurface runoff processes, our results revealed that soil hydrology can have a large impact on nitrate–nitrogen riverine loading through surface and subsurface runoff when there is a significant concentration of nitrogen in soil water and sufficient recharge through rainfall or irrigation (Meisinger and Delgado, 2002). The dynamics of nitrogen riverine loading is linked to the movement of water through the soil profile. Adequate soil moisture levels promote microbial decomposition of organic matter and subsequent release of nitrogen into the soil. Different parameterizations of subsurface and surface runoff can have a significant impact on the nitrogen dynamics in the soil and consequently loss through the runoff.
The interplay between soil moisture dynamics and nitrogen transport is critical for understanding riverine nitrogen loading, especially in the context of short-term impacts from hurricane events when soil moisture can experience high-frequency variability. When surface runoff dominates, nitrogen can be rapidly transported to surface water, leading to spikes in the riverine level. On the other hand, nitrogen is slowly leached through the soil profile and into groundwater systems before eventually reaching rivers, showing a delayed response compared to the surface runoff loss. Both model simulations in this study suggest dominant nitrogen loss through surface runoff, and the response to hurricanes in WTD and nitrogen runoff loading is approximately consistent with the observations. This suggests ELM can provide some valuable insights into the mechanisms driving nitrogen runoff loading during hurricanes, such as the significant role of surface runoff and changes in water table depth.
While the focus of this study is primarily analyzing the response of nitrogen runoff loading during Hurricane Ida using ELM, it is also important to consider the pre-existing conditions of soil water for water quality management. This is particularly crucial for nearshore areas which are dry prior to hurricane landfall, as the potential harm to water quality can be particularly acute. By understanding both aspects (prior to and during the hurricane), we can better anticipate and mitigate the adverse effects of a hurricane in the vulnerable regions.
4.3 Model limitations
Although the models successfully captured the groundwater table response to Hurricane Ida's landfall, consistent with observations from the monitoring wells that show a rising water table, our findings revealed a faster diminishing of nitrogen runoff after Ida's landfall on 29 August in the area affected by Hurricane Ida. The model indicated significantly higher nitrogen leaching before Ida's landfall, earlier than the water quality observations at monitoring stations. The early nitrogen runoff was attributed to the abundant precipitation in the southern LMRB in late August 2021 before and after Hurricane Ida formed on 26 August, leading to elevated soil moisture levels prior to Ida's landfall. This precipitation mobilized nitrogen in the previously dry and warm soil, as indicated by the model results, leaving a lower soil mineral concentration for leaching after Ida's landfall. Apart from the heterogeneity in precipitation both in time and space, the discrepancy in WTD and nitrogen leaching between the simulations and observations largely stem from the omission of crop management factors in the model. As one of the largest agricultural crop-producing areas in the US (Tiwari et al., 2023), the LMRB experiences significant agricultural activities that contribute to nitrogen dynamics. Over 90 % of the groundwater used for irrigation in the LMRB comes from the Mississippi River valley alluvial aquifer (Reba and Massey, 2020). The absence of accounting for these agricultural practices could be a factor influencing the observation–model inconsistencies in nitrogen leaching patterns, as evidenced by the results between our two model simulations with distinct WTDs.
Another important overlooked process in the model is the lateral transport of nitrogen by advection and diffusion induced by hydrologic connectivity and a strong nitrogen gradient between neighboring grid cells. The timing of hydrologic connectivity and nutrient gradients may affect a range of downslope nutrient transport and biogeochemical transformation along the topographic sequence (Stieglitz et al., 2003; Kelly et al., 2021).
Note that the current version of ELM does not include an in-stream nitrogen model, which is under active development. Consequently, while our study successfully examines the impact of hurricanes on runoff nitrogen loading, it does not fully capture the complete dynamics of nitrogen in stream before and after the hurricane, and hence it cannot be used to explain the observed N loading dynamics before Ida. Future improvements to ELM, including the integration of an in-stream N model, will enable more detailed and accurate simulations of nitrogen and runoff loading dynamics in rivers.
In conclusion, we calibrated two parameters associated with surface and subsurface runoff in ELM using the water table depth (WTD) obtained from a previous 3D hydrology simulation in the lower Mississippi River basin (LMRB). We then compared the nitrogen runoff leaching results from the calibrated ELM with those from the default ELM. Our analysis of the WTD in the calibrated model and the 3D model revealed that despite model calibration to match the ELM WTD with that simulated by the 3D model, neglecting lateral flow in ELM can still result in noticeable differences between the WTD in the two models, particularly in slope areas with limited precipitation. The calibrated ELM was able to simulate the increased nitrate–nitrogen runoff leaching during Hurricane Ida, as evidenced by water quality and hydrologic observations within the affected region. However, the timing of peak leaching and the leaching pathways can be influenced by factors such as soil moisture, soil temperature, precipitation, and lateral transport. Thus differences between the calibrated and default models as well as differences between the models and observations (e.g., WTD, crop management) can result in differences between the observed and simulated nitrogen response to Hurricane Ida. Even though the model captures the N runoff loading signal in the area affected by Hurricane Ida, the current lack of lateral transport of nitrogen within the soil and in the river in ELM hinders the realistic prediction of nitrogen runoff loading in response to hurricanes.
The code is available at https://doi.org/10.5281/zenodo.11372002 (Fang, 2024b). The neural network model script, data used to train the model, and the observation data used in this study are available at https://doi.org/10.5281/zenodo.10927512 (Fang, 2024a).
YF designed the study, conducted the experiments and analysis, and drafted the manuscript. HVT provided the water table depth data from the ELM–ParFlow simulation, contributed to the analysis with machine learning, and edited the manuscript. LRL contributed to the result interpretation and discussion and edited the manuscript.
The contact author has declared that none of the authors has any competing interests.
Publisher's note: Copernicus Publications remains neutral with regard to jurisdictional claims made in the text, published maps, institutional affiliations, or any other geographical representation in this paper. While Copernicus Publications makes every effort to include appropriate place names, the final responsibility lies with the authors.
This study was supported by the U.S. Department of Energy Advanced Scientific Computing Research (ASCR) program through the Multiphysics Simulations and Knowledge discovery through AI/ML technologies (MuSiKAL) project. The simulations presented in this article were performed on computational resources managed and supported by the Department of Energy (DOE) Office of Biological and Environmental Research (BER) and housed in the Pacific Northwest National Laboratory (PNNL) Computational Science Facility. The PNNL is operated for DOE by the Battelle Memorial Institute (contract no. DE-AC05-76RL01830).
This research has been supported by the DOE Advanced Scientific Computing Research program (grant no. 78667).
This paper was edited by Hans Verbeeck and reviewed by two anonymous referees.
Balaguru, K., Xu, W. W., Chang, C. C., Leung, L. R., Judi, D. R., Hagos, S. M., Wehner, M. F., Kossin, J. P., and Ting, M. F.: Increased US coastal hurricane risk under climate change, Sci. Adv., 9, eadf0259, https://doi.org/10.1126/sciadv.adf0259, 2023.
Burrows, S. M., Maltrud, M., Yang, X., Zhu, Q., Jeffery, N., Shi, X., Ricciuto, D., Wang, S., Bisht, G., Tang, J., Wolfe, J., Harrop, B. E., Singh, B., Brent, L., Baldwin, S., Zhou, T., Cameron-Smith, P., Keen, N., Collier, N., Xu, M., Hunke, E. C., Elliott, S. M., Turner, A. K., Li, H., Wang, H., Golaz, J. C., Bond-Lamberty, B., Hoffman, F. M., Riley, W. J., Thornton, P. E., Calvin, K., and Leung, L. R.: The DOE E3SM v1.1 Biogeochemistry Configuration: Description and Simulated Ecosystem-Climate Responses to Historical Changes in Forcing, J. Adv. Model. Earth Sy., 12, e2019MS001766, https://doi.org/10.1029/2019MS001766, 2020.
Carpenter, S. R., Bolgrien, D., Lathrop, R. C., Stow, C. A., Reed, T., and Wilson, M. A.: Ecological and economic analysis of lake eutrophication by nonpoint pollution, Aust. J. Ecol., 23, 68–79, https://doi.org/10.1111/j.1442-9993.1998.tb00706.x, 1998.
Duarte, H. F., Raczka, B. M., Ricciuto, D. M., Lin, J. C., Koven, C. D., Thornton, P. E., Bowling, D. R., Lai, C.-T., Bible, K. J., and Ehleringer, J. R.: Evaluating the Community Land Model (CLM4.5) at a coniferous forest site in northwestern United States using flux and carbon-isotope measurements, Biogeosciences, 14, 4315–4340, https://doi.org/10.5194/bg-14-4315-2017, 2017.
Fang, Y.: Data used to study the short-term effects of hurricane Ida on nitrate–nitrogen runoff loading using E3SM land model, Zenodo [data set], https://doi.org/10.5281/zenodo.10927512, 2024a.
Fang, Y.: E3SM land model used to study the short-term effects of hurricanes on nitrate–nitrogen runoff loading, Zenodo [code], https://doi.org/10.5281/zenodo.11372002, 2024b.
Fang, Y., Leung, L. R., Koven, C. D., Bisht, G., Detto, M., Cheng, Y., McDowell, N., Muller-Landau, H., Wright, S. J., and Chambers, J. Q.: Modeling the topographic influence on aboveground biomass using a coupled model of hillslope hydrology and ecosystem dynamics, Geosci. Model Dev., 15, 7879–7901, https://doi.org/10.5194/gmd-15-7879-2022, 2022.
Kollet, S. J. and Maxwell, R. M.: Integrated surface-groundwater flow modeling: A free-surface overland flow boundary condition in a parallel groundwater flow model, Adv. Water Resour., 29, 945–958, https://doi.org/10.1016/j.advwatres.2005.08.006, 2006.
Filippino, K. C., Egerton, T. A., Hunley, W. S., and Mulholland, M. R.: The Influence of Storms on Water Quality and Phytoplankton Dynamics in the Tidal James River, Estuar. Coast., 40, 80–94, https://doi.org/10.1007/s12237-016-0145-6, 2017.
Golaz, J. C., Caldwell, P. M., Van Roekel, L. P., Petersen, M. R., Tang, Q., Wolfe, J. D., Abeshu, G., Anantharaj, V., Asay-Davis, X. S., Bader, D. C., Baldwin, S. A., Bisht, G., Bogenschutz, P. A., Branstetter, M., Brunke, M. A., Brus, S. R., Burrows, S. M., Cameron-Smith, P. J., Donahue, A. S., Deakin, M., Easter, R. C., Evans, K. J., Feng, Y., Flanner, M., Foucar, J. G., Fyke, J. G., Griffin, B. M., Hannay, C., Harrop, B. E., Hoffman, M. J., Hunke, E. C., Jacob, R. L., Jacobsen, D. W., Jeffery, N., Jones, P. W., Keen, N. D., Klein, S. A., Larson, V. E., Leung, L. R., Li, H. Y., Lin, W. Y., Lipscomb, W. H., Ma, P. L., Mahajan, S., Maltrud, M. E., Mametjanov, A., McClean, J. L., McCoy, R. B., Neale, R. B., Price, S. F., Qian, Y., Rasch, P. J., Eyre, J. E. J. R., Riley, W. J., Ringler, T. D., Roberts, A. F., Roesler, E. L., Salinger, A. G., Shaheen, Z., Shi, X. Y., Singh, B., Tang, J. Y., Taylor, M. A., Thornton, P. E., Turner, A. K., Veneziani, M., Wan, H., Wang, H. L., Wang, S. L., Williams, D. N., Wolfram, P. J., Worley, P. H., Xie, S. C., Yang, Y., Yoon, J. H., Zelinka, M. D., Zender, C. S., Zeng, X. B., Zhang, C. Z., Zhang, K., Zhang, Y., Zheng, X., Zhou, T., and Zhu, Q.: The DOE E3SM Coupled Model Version 1: Overview and Evaluation at Standard Resolution, J. Adv. Model. Earth Sy., 11, 2089–2129, https://doi.org/10.1029/2018ms001603, 2019.
Hefting, M., Clément, J. C., Dowrick, D., Cosandey, A. C., Bernal, S., Cimpian, C., Tatur, A., Burt, T. P., and Pinay, G.: Water table elevation controls on soil nitrogen cycling in riparian wetlands along a European climatic gradient, Biogeochemistry, 67, 113–134, https://doi.org/10.1023/B:Biog.0000015320.69868.33, 2004.
Hurtt, G. C., Chini, L., Sahajpal, R., Frolking, S., Bodirsky, B. L., Calvin, K., Doelman, J. C., Fisk, J., Fujimori, S., Klein Goldewijk, K., Hasegawa, T., Havlik, P., Heinimann, A., Humpenöder, F., Jungclaus, J., Kaplan, J. O., Kennedy, J., Krisztin, T., Lawrence, D., Lawrence, P., Ma, L., Mertz, O., Pongratz, J., Popp, A., Poulter, B., Riahi, K., Shevliakova, E., Stehfest, E., Thornton, P., Tubiello, F. N., van Vuuren, D. P., and Zhang, X.: Harmonization of global land use change and management for the period 850–2100 (LUH2) for CMIP6, Geosci. Model Dev., 13, 5425–5464, https://doi.org/10.5194/gmd-13-5425-2020, 2020.
Jasinski, B. L., Hewitt, R. E., Mauritz, M., Miller, S. N., Schuur, E. A. G., Taylor, M. A., Walker, X. J., and Mack, M. C.: Plant foliar nutrient response to active layer and water table depth in warming permafrost soils, J. Ecol., 110, 1201–1216, https://doi.org/10.1111/1365-2745.13864, 2022.
Kelly, T. B., Knapp, A. N., Landry, M. R., Selph, K. E., Shropshire, T. A., Thomas, R. K., and Stukel, M. R.: Lateral advection supports nitrogen export in the oligotrophic open-ocean Gulf of Mexico, Nat. Commun., 12, 3325, https://doi.org/10.1038/s41467-021-23678-9, 2021.
Knutson, T., Camargo, S. J., Chan, J. C. L., Emanuel, K., Ho, C., Kossin, J., Mohapatra, M., Satoh, M., Sugi, M., Walsh, K., and Wu, L.: Tropical cyclones and climate change assessment: Part II: projected response to anthropogenic warming, B. Am. Meteorol. Soc., 101, E303–E322, https://doi.org/10.1175/BAMS-D-18-0194.1, 2020.
Li, T. Y., Zhang, Y., He, B. H., Wu, X. Y., and Du, Y. N.: Nitrate loss by runoff in response to rainfall amount category and different combinations of fertilization and cultivation in sloping croplands, Agr. Water Manage., 273, 107916, https://doi.org/10.1016/j.agwat.2022.107916, 2022.
Maxwell, R. M.: A terrain-following grid transform and preconditioner for parallel, large-scale, integrated hydrologic modeling, Adv. Water Resour., 53, 10917, https://doi.org/10.1016/j.advwatres.2012.10.001, 2013.
Maxwell, R. M. and Miller, N. L.: Development of a coupled land surface and groundwater model, J. Hydrometeorol., 6, 233–247, https://doi.org/10.1175/Jhm422.1, 2005.
Medvigy, D., Wang, G. S., Zhu, Q., Riley, W. J., Trierweiler, A. M., Waring, B. G., Xu, X. T., and Powers, J. S.: Observed variation in soil properties can drive large variation in modelled forest functioning and composition during tropical forest secondary succession, New Phytol., 223, 1820–1833, https://doi.org/10.1111/nph.15848, 2019.
Meisinger, J. J. and Delgado, J. A.: Principles for managing nitrogen leaching, J. Soil Water Conserv., 57, 485–498, 2002.
Miao, G. F., Noormets, A., Domec, J. C., Trettin, C. C., McNulty, S. G., Sun, G., and King, J. S.: The effect of water table fluctuation on soil respiration in a lower coastal plain forested wetland in the southeastern US, J. Geophys. Res.-Biogeo., 118, 1748–1762, https://doi.org/10.1002/2013jg002354, 2013.
Nelson, A. M., Ashwell, N. E. Q., Delhom, C. D., and Gholson, D. M.: Leveraging Big Data to Preserve the Mississippi River Valley Alluvial Aquifer: A Blueprint for the National Center for Alluvial Aquifer Research, Land, 11, 1925, https://doi.org/10.3390/land11111925, 2022.
Nevison, C., Hess, P., Riddick, S., and Ward, D.: Denitrification, leaching, and river nitrogen export in the Community Earth System Model, J. Adv. Model. Earth Sy., 8, 272–291, https://doi.org/10.1002/2015ms000573, 2016.
Nie, J., Mirza, S., Viteritto, M., Li, Y. Y., Witherell, B. B., Deng, Y., Yoo, S., and Feng, H.: Estimation of nutrient (N and P) fluxes into Newark Bay, USA, Mar. Pollut. Bull., 190, 114832, https://doi.org/10.1016/j.marpolbul.2023.114832, 2023.
Niu, G. Y., Yang, Z. L., Dickinson, R. E., and Gulden, L. E.: A simple TOPMODEL-based runoff parameterization (SIMTOP) for use in global climate models, J. Geophys. Res.-Atmos., 110, D21106, https://doi.org/10.1029/2005jd006111, 2005.
Oleson, K., Lawrence, D. M., Bonan, G. B., Drewniak, B., Huang, M., Koven, C. D., Levis, S., Li, F., Riley, W. J., Subin, Z. M. Swenson, S. C., Thornton, P. E., Bozbiyik, A., Fisher, R., Heald, C. L., Kluzek, E., Lamarque, J., Lawrence, P. J., Leung, L. R., Lipscomb, W., Muszala, S., Ricciuto, D. M., Sacks, W., Sun, Y., Tang, J., and Yang, Z.-L.: Technical description of version 4.5 of the Community Land Model (CLM) (No. NCAR/TN-503+STR) Research, National Center for Atmospheric Rep., Boulder, Colorado, https://doi.org/10.5065/D6RR1W7M, 420 pp., 2013.
Ouyang, Y., Zhang, J., Feng, G., Wan, Y., and Leininger, T. D.: A century of precipitation trends in forest lands of the Lower Mississippi River Alluvial Valley, Sci. Rep., 10, 12802, https://doi.org/10.1038/s41598-020-69508-8, 2020.
Paerl, H. W., Hall, N. S., Hounshell, A. G., Rossignol, K. L., Barnard, M. A., Luettich, R. A., Rudolph, J. C., Osburn, C. L., Bales, J., and Harding, L. W.: Recent increases of rainfall and flooding from tropical cyclones (TCs) in North Carolina (USA): implications for organic matter and nutrient cycling in coastal watersheds, Biogeochemistry, 150, 197–216, https://doi.org/10.1007/s10533-020-00693-4, 2020.
Pérez-Alarcón, A., Fernández-Alvarez, J. C., and Coll-Hidalgo, P.: Global Increase of the Intensity of Tropical Cyclones under Global Warming Based on their Maximum Potential Intensity and CMIP6 Models, Environ. Process., 10, 36, https://doi.org/10.1007/s40710-023-00649-4, 2023.
Reba, M. L. and Massey, J. H.: Surface Irrigation in the Lower Mississippi River Basin: Trends and Innovations, T. ASABE, 63, 1305–1314, https://doi.org/10.13031/trans.13970, 2020.
Ritter, W. F. and Chitikela, S. R.: The Mississippi River Basin Nitrogen Problem: Past History and Future Challenges to Solve It, Watershed Management, 2020, 109–123, 2020.
Speir, S. L., Tank, J. L., Bieroza, M., Mahl, U. H., and Royer, T. V.: Storm size and hydrologic modification influence nitrate mobilization and transport in agricultural watersheds, Biogeochemistry, 156, 319–334, https://doi.org/10.1007/s10533-021-00847-y, 2021.
Stieglitz, M., Shaman, J., McNamara, J., Engel, V., Shanley, J., and Kling, G. W.: An approach to understanding hydrologic connectivity on the hillslope and the implications for nutrient transport, Global Biogeochem. Cy., 17, 1105, https://doi.org/10.1029/2003gb002041, 2003.
Sun, C. L., Cui, L., Zhou, B. Q., Wang, X., Guo, L. P., and Liu, W.: Visualizing the spatial distribution and alteration of metabolites in continuously cropped Salvia miltiorrhiza Bge using MALDI-MSI, J. Pharm. Anal., 12, 719–724, https://doi.org/10.1016/j.jpha.2021.09.011, 2022.
Tang, J. Y.: On the relationships between the Michaelis–Menten kinetics, reverse Michaelis–Menten kinetics, equilibrium chemistry approximation kinetics, and quadratic kinetics, Geosci. Model Dev., 8, 3823–3835, https://doi.org/10.5194/gmd-8-3823-2015, 2015.
Tiwari, P., Poudel, K. P., Yang, J., Silva, B., Yang, Y., and McConnell, M.: Marginal agricultural land identification in the Lower Mississippi Alluvial Valley based on remote sensing and machine learning model, Int. J. Appl. Earth Obs., 125, 103568, https://doi.org/10.1016/j.jag.2023.103568, 2023.
Tran, H., Fang, Y., Tan, Z., Zhou, T., and Leung, L. R.: Quantifying the impacts of land cover change on the hydrologic response to Hurricane Ida in the Lower Mississippi River Basin, J. Hydrometeorol., 25, 899–914, https://doi.org/10.1175/JHM-D-23-0094.1, 2024.
Valiela, I., Peckol, P., D'Avanzo, C., Kremer, J., Hersh, D., Foreman, K., Lajtha, K., Seely, B., Geyer, W. R., Isaji, T., and Crawford, R.: Ecological effects of major storms on coastal watersheds and coastal waters: Hurricane Bob on Cape Cod, J. Coastal Res., 14, 218–238, 1998.
Vidon, P., Karwan, D. L., Andres, A. S., Inamdar, S., Kaushal, S., Morrison, J., Mullaney, J., Ross, D. S., Schroth, A. W., Shanley, J. B., and Yoon, B.: In the path of the Hurricane: impact of Hurricane Irene and Tropical Storm Lee on watershed hydrology and biogeochemistry from North Carolina to Maine, USA, Biogeochemistry, 141, 351–364, https://doi.org/10.1007/s10533-018-0423-4, 2018.
Wilson, L., Amatya, D., Callahan, T., and Trettin, C.: Hurricane impact on stream flow and nutrient exports for a first-order forested watershed of the lower coastal plain, South Carolina, in: Proceedings of the Second Interagency Conference on Research in the Watersheds, Coweeta Hydrologic Laboratory Otto, NC, USA, 16–18 May, 169–179, 2006.
Yang, X. J., Thornton, P. E., Ricciuto, D. M., and Hoffman, F. M.: Phosphorus feedbacks constraining tropical ecosystem responses to changes in atmospheric CO2 and climate, Geophys. Res. Lett., 43, 7205–7214, https://doi.org/10.1002/2016gl069241, 2016.
Zhang, B., Zeng, F. J., Gao, X. P., Shareef, M., Zhang, Z. H., Yu, Q., Gao, Y. J., Li, C. J., Yin, H., Lu, Y., Huang, C. B., and Tang, G. L.: Groundwater depth alters soil nutrient concentrations in different environments in an arid desert, Front. Env. Sci.-Switz., 10, 939382, https://doi.org/10.3389/fenvs.2022.939382, 2022.
Zhang, J. Z., Kelble, C. R., Fischer, C. J., and Moore, L.: Hurricane Katrina induced nutrient runoff from an agricultural area to coastal waters in Biscayne Bay, Florida, Estuar. Coast. Shelf S., 84, 209–218, https://doi.org/10.1016/j.ecss.2009.06.026, 2009.
Zhu, Y. J., Collins, J. M., Klotzbach, P. J., and Schreck, C. J.: Hurricane Ida (2021): Rapid Intensification Followed by Slow Inland Decay, B. Am. Meteorol. Soc., 103, E2354–E2369, https://doi.org/10.1175/Bams-D-21-0240.1, 2022.