the Creative Commons Attribution 4.0 License.
the Creative Commons Attribution 4.0 License.
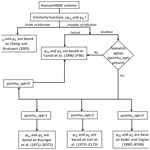
An updated parameterization of the unstable atmospheric surface layer in the Weather Research and Forecasting (WRF) modeling system
Prabhakar Namdev
Maithili Sharan
Piyush Srivastava
Saroj Kanta Mishra
The accurate parameterization of atmospheric surface layer processes is crucial for weather forecasts using numerical weather prediction models. Here, an attempt has been made to improve the surface layer parameterization in the Weather Research and Forecasting model version 4.2.2 (WRFv4.2.2) by implementing similarity functions proposed by Kader and Yaglom (1990) to make it consistent in producing the transfer coefficient for momentum observed over the tropical region (Srivastava and Sharan, 2015). The surface layer module in WRFv4.2.2 is modified in such a way that it contains the commonly used similarity functions for momentum (φm) and heat (φh) under convective conditions instead of the existing single functional form. The updated module has various alternatives of φm and φh, which can be controlled by a flag introduced in the input file. The impacts of utilizing different functional forms have been evaluated using the bulk flux algorithm as well as real-case simulations with the WRFv4.2.2 model. The model-simulated variables have been evaluated with observational data from a flux tower at Ranchi (23.412° N, 85.440° E), India, and the ERA5-Land reanalysis dataset. The transfer coefficient for momentum simulated using the implemented scheme is found to agree well with its observed non-monotonic behavior in convective conditions (Srivastava and Sharan, 2021). The study suggests that the updated surface layer scheme performs well in simulating the surface transfer coefficients and could be potentially utilized for the parameterization of surface fluxes under convective conditions in the WRF model.
- Article
(12354 KB) - Full-text XML
-
Supplement
(3608 KB) - BibTeX
- EndNote
Inadequate representation of near-surface turbulent processes adds significant uncertainty in both climate projections and seasonal weather forecasts obtained from atmospheric models (Bourassa et al., 2013). Most of the numerical weather prediction and general circulation models utilize Monin–Obukhov similarity theory (MOST; Monin and Obukhov, 1954) to parameterize surface turbulent fluxes. To estimate these fluxes and near-surface atmospheric variables, the theory utilizes similarity functions of momentum (φm) and heat (φh) often prescribed as functions of ζ (stability parameter). However, the exact functional forms for these functions have not been provided by MOST; rather it suggests some asymptotic predictions under near-neutral to very stable and unstable conditions. Over the years, researchers have developed many functional forms for these functions based on the different experiments, conducted over different locations and having separate expressions for stable and unstable stratifications (Webb, 1970; Businger et al., 1971; Carl et al., 1973; Dyer, 1974; Hicks, 1976; Holtslag and De Bruin, 1988; Brutsaert, 1992; de Bruin, 1999; Wilson, 2001; Cheng and Brutsaert, 2005; Grachev et al., 2007; Gryanik et al., 2020; Srivastava et al., 2020).
In most of the atmospheric models, the commonly used similarity functions under convective conditions are those proposed by Businger (1966) and Arch J. Dyer (1965, unpublished work; see Businger, 1988) and referred to as Businger–Dyer (BD) functions. However, these functional forms are unable to follow the classical free convection limit. The study by Rao et al. (1996) suggests that MOST using Businger relations is unable to define a transfer coefficient for momentum (CD) consistent with its observed behavior, specifically at low-wind convective conditions, indicating that MOST needs to be modified in the (nearly) windless free convection limits. As a result, a revised scaling of heat flux for weakly forced convection in the atmosphere has been proposed by Rao and Narasimha (2006). Later, the issues of using BD functions in the surface layer scheme based on the fifth-generation Pennsylvania State University – National Centre for Atmospheric Research Mesoscale Model (MM5) of a regional-scale model (Weather Research and Forecasting; WRF) have been reported in a study by Jiménez et al. (2012). They implemented the new scheme (referred to as revised MM5 scheme; Jiménez et al., 2012) in the WRF modeling system and replaced the BD functions by those proposed by Fairall et al. (1996) (F96) under convective conditions. F96 functions are the combination of BD functions and the functions suggested by Carl et al. (1973), and they are valid for the entire range of atmospheric instability. Note that the most recent version of the WRF model still utilizes F96 functions under convective conditions.
Srivastava and Sharan (2015) analyzed the observed behavior of CD over an Indian land surface and suggested that the observed CD shows non-monotonic behavior with −ζ, unlike the behavior of predicted CD from the MOST-based parameterization with commonly used φm and φh (Businger et al., 1971; Carl et al., 1973; Fairall et al., 1996). Later, a theoretical study by Srivastava and Sharan (2021) revealed that the three-sublayer model based on Kader and Yaglom (1990) is able to predict CD consistent with its observed non-monotonic behavior. Note that the three-sublayer model has not yet been installed and evaluated in the WRF modeling framework. However, it is already operational in the surface layer scheme (Community Land Model; CLM) of the National Centre for Atmospheric Research Community Atmosphere Model version 5 (NCAR-CAM5) and the regional climate model (RegCM).
The study by Srivastava and Sharan (2021) also analyzed the possible uncertainties associated with the use of different functional forms of φm and φh under convective conditions. To quantify the impacts of different functional forms, they classified available φm and φh in four classes based on the exponents appearing in the expressions of φm and φh as (1) functional forms having the exponents of φm and φh of and , respectively (Businger et al., 1971; Hogstrom, 1996); (2) functional forms having the exponent of φm and φh of (Carl et al., 1973); (3) functional forms having the exponent of φm and φh of and , respectively, in near-neutral conditions while in very unstable conditions (Fairall et al., 1996; Grachev et al., 2000; Fairall et al., 2003); and (4) functional forms having the exponent of φm and φh of and , respectively, in near-neutral conditions but for φm and for φh in strongly unstable conditions (Kader and Yaglom, 1990; Zeng et al., 1998). This study concludes that utilizing different functional forms of similarity functions in the bulk flux algorithm results in a large deviation in the values of estimated fluxes. The detailed descriptions of different functional forms for φm and φh considered in different classes are given in Appendix A. We wish to highlight that all available functional forms for φm and φh under convective conditions fall in one of the classes stated above.
The revised MM5 surface layer scheme of the WRF model version 4.2.2 (WRFv4.2.2) employed φm and φh based on Fairall et al. (1996), which belong to class 3. As a result, this scheme is not appropriate for producing CD consistent with its observed behavior, specifically over the Indian land surface, as stated above. Recently Namdev et al. (2023a) argue that the performance of numerical weather prediction (NWP) models varies a lot over different seasons and surface types depending upon the functional behavior of φm and φh. Thus, to enhance the potential applicability of the WRF modeling framework, this study attempted to incorporate all the commonly used similarity functions under convective conditions along with the Kader and Yaglom (1990) (KY90) functions as well as existing functional forms in the revised MM5 surface layer scheme of WRFv4.2.2. A namelist flag has been introduced in the WRF model to choose between various φm and φh in the modified scheme. The modified surface layer scheme proposed in this study has been evaluated using offline simulations with a bulk flux algorithm as well as the real-case simulations with WRFv4.2.2 during the pre-monsoon season (March–April–May) of 2009 over a domain centered around the location of the flux tower installed at Ranchi (23.412° N, 85.440° E), India.
2.1 Surface flux computation in the WRF modeling system
The Monin–Obukhov similarity theory serves as the foundation for the surface layer parameterization (revised MM5 scheme) in the WRF model, and the surface turbulent fluxes are calculated based on the bulk approach using bulk transfer coefficients for momentum (CD) and heat (CH) (Namdev et al., 2024; Srivastava et al., 2021; Srivastava and Sharan, 2021). Following MOST they are formulated as follows:
in which k is the von Karmann constant; z0 and zh are the roughness lengths for momentum and heat, respectively; ψm and ψh are the integrated similarity functions for momentum and heat, respectively; and L is the Obukhov length scale.
Their determination based on MOST using integrated forms of the similarity functions is explained in Appendix B. In the following, the default similarity functions used in the WRF model are explained and other functions are introduced in Sect. 2.2.
The default version of the revised MM5 scheme in the WRF model utilizes similarity functions suggested by Cheng and Brutsaert (2005) under stable atmospheric conditions (ζ>0), which are developed using the CASES-99 dataset. The integrated forms of functions proposed by Cheng and Brutsaert (2005) are
where d=1.1, c=5.3, b=2.5, and d=6.1.
On the other hand, the similarity functions for an unstable atmospheric surface layer (ζ<0) are those proposed by Fairall et al. (1996; F96). The corresponding integrated functional forms ψm and ψh are defined as
where and denote the integrated functional forms based on Businger et al. (1971) and Carl et al. (1973), respectively. The expressions for and are
in which and
with . The values of the constants βm and βh are taken as 10 and 34 based on Grachev et al. (2000).
2.2 Implementation of different similarity functions
In this section, we briefly describe the implementation of different similarity functions for unstable stratification in the surface layer parameterization of WRFv4.2.2. Note that two sets of functional forms, namely those suggested by Carl et al. (1973) and the three-sublayer model proposed by Kader and Yaglom (1990) for convective conditions, have not been included and tested in the surface layer scheme of the WRF modeling framework.
2.2.1 Functions by Businger et al. (1971) (BD71)
Similarity functions suggested by Businger et al. (1971) are based on the KANSAS dataset (Izumi, 1971). These functions do not satisfy the classical free convection limit as predicted by MOST. They are already implemented in the old version of the MM5 surface layer scheme (Grell et al., 1994) in the WRF model. The integrated functional forms (ψm and ψh) for φm and φh stated in Eqs. (A1) and (A2) (Appendix A) are given in Eqs. (6) and (7).
2.2.2 Functions by Carl et al. (1973) (CL73)
Carl et al. (1973) proposed an expression of similarity functions φm and φh valid for the stability range . The expressions for φm and φh are given in Eqs. (A3) and (A4) (Appendix A). The similarity functions proposed by Carl et al. (1973) have not been analyzed in the surface layer scheme of the WRF model. The integrated forms (ψm and ψh) of similarity functions φm and φh are given by Eq. (8).
2.2.3 Functions by Kader and Yaglom (1990) (KY90)
Kader and Yaglom (1990) introduced a three-sublayer model for convective conditions. The three sublayers are categorized based on ζ values as (1) the dynamic sublayer which corresponds to near-neutral conditions, (2) the dynamic convective sublayer which corresponds to moderately unstable conditions, and (3) the free convective conditions. The present study utilized φm and φh expressions given in Eqs. (A9) and (A10) (Appendix A) that are being used in the surface layer scheme (CLM4.0; Zeng et al., 1998) of the NCAR-CAM5 model. The corresponding integrated forms for φm and φh are
where .
Note that all the functions stated above have been newly installed in the revised MM5 surface layer scheme of WRFv4.2.2 and can be used in place of F96 functions already employed in the model. Here, we have introduced a new surface layer module where different options for φm and φh can be controlled using an appropriate value of the namelist parameter (psimhu_opt). The parameter psimhu_opt is added under the physics section of the namelist file. The variable psimhu_opt can have values 0, 1, 2, and 3 for different options for functions F96 (default), BD71, CL73, and KY90, respectively. A brief structure and different choices for psimhu_opt based on newly installed and default functional forms of φm and φh in the default and modified revised MM5 scheme are shown in Fig. 1.
2.3 Characteristics of default and newly installed similarity functions
The expressions of φm and φh for different functional forms utilized in this study are stated in Appendix A. Figure S1 in the Supplement shows the variation in different (a) φm and (b) φh under moderately to strongly unstable conditions. It is evident from Fig. S1 that all the different functional forms provide similar values of φm and φh in near-neutral to moderately unstable conditions (up to approximately). However, at higher instabilities one can expect noticeable differences between different functional forms of φm and φh. Note that the functional forms for φm corresponding to BD71 and CL73 decrease continuously with increasing instability; however, φm corresponding to KY90 functional forms shows decreasing behavior in near-neutral to moderately unstable conditions and attains a minimum at , and, as the instability further increases, it starts increasing with −ζ (Fig. S1a). This implies that φm based on class 4 functions shows non-monotonic behavior which contradicts the classical MOST prediction. On the other hand, in the case of φh, all the functional forms provide continuously decreasing behavior of φh from near-neutral to moderately unstable conditions (Fig. S1b).
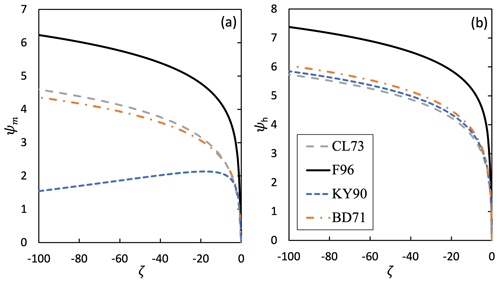
Figure 2Integrated similarity functions ψm,h(ζ) for momentum and heat for default (F96; black line) and newly installed (BD71, CL73, and KY90; orange, gray, and blue lines, respectively) functions for unstable atmospheric surface layer.
Figure 2 illustrates the variation in default (F96) and newly installed integrated similarity functions ψm and ψh (BD71, CL73, and KY90) with respect to −ζ. One can see from Fig. 2a that ψm corresponding to F96, BD71, and CL73 functional forms increases continuously with −ζ in moderately to strongly unstable conditions. However, a non-monotonic behavior has been found for ψm corresponding to the KY90 functions implying it first increases with −ζ and reaches a maximum at and then starts decreasing as instability further grows. On the other hand, ψh corresponding to all the considered functional forms increases continuously in near-neutral to strongly unstable conditions. However, the rate of increase is slightly higher for F96 in comparison to the other three functions (BD71, CL73, and KY90), whose results are very similar to each other (Fig. 2b).
2.4 Observational data for model evaluation
For the evaluation of different simulations corresponding to newly installed similarity functions, observational data derived from the micrometeorological tower installed at Ranchi (India) have been utilized (Srivastava and Sharan, 2019; Srivastava et al., 2020, 2021). The dataset (Ranchi data) is derived from an instrument mounted on a 32 m tall tower at the Birla Institute of Technology Mesra in Ranchi, India (Dwivedi et al., 2015), with an average elevation of 609 m above sea level in a tropical region. The site has a few buildings in between the east and northwest, agricultural land in between the northwest and west, and a residential area and dense trees in between the southeast and east. The site also has a relatively flat area in between southeast and west which is free from any obstacle (Srivastava and Sharan, 2015). A fast response sensor (CSAT3 sonic anemometer) at a height of 10 m with an average elevation 609 m above sea level provides the temperature and the three components of wind at a 10 Hz frequency. The eddy covariance technique (Stull, 1988) is used to estimate heat and momentum fluxes at 1 h time resolution; however the hourly temperature at 2 m is determined by averaging temperature observations available at a temporal scale of 1 min from the slow response sensors located at logarithmic heights on the same tower. We have utilized hourly data for considered variables. Apart from this we have also utilized the ERA5-Land reanalysis dataset available at 0.10° × 0.10° spatial resolution to evaluate the spatial distribution of the model-simulated near-surface atmospheric variables. For consistency, we have regridded the model output to the same grid resolution of the reanalysis and observed datasets.
To analyze the impacts of newly installed similarity functions together with the existing functional forms in the surface layer scheme of WRFv4.2.2, the performance of the default and newly installed similarity functions is investigated in two steps. The first one is independent of the WRF model. Namely, we apply Eq. (B8) (Appendix B) to iteratively determine CD and CH as a function of ζ by prescribing the bulk Richardson number (RiB) and surface roughness parameters for momentum (z0) and heat (zh). The value of ζ is estimated by calculating the root of least magnitude of Eq. (B8) for a given value of RiB. Once ζ is calculated and then utilizing it in Eqs. (B9) and (B10), the values of CD and CH can be estimated. We call this in the following the offline simulation. For the computation, z is taken as 10 m and RiB is in the range . The offline simulations are carried out over three different surface types by considering surface roughness (z0) to be 0.01 m (smooth surface), 0.1 m (transition surface), and 1 m (rough surface) to analyze the impact of the roughness of the underlying surface on the simulation of ζ, CD, and CH.
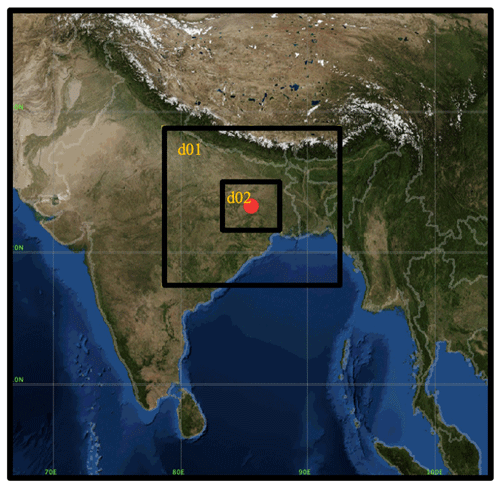
Figure 3Spatial distribution of domain used for the simulations using the WRF model. The spatial resolution for domains d01 and d02 is 6×6 and 2×2 km2, respectively. The domain d02 covers an area of 446×392 km2 around the center point.
The second step is to apply all the parameterizations of the similarity functions in the WRF model version 4.2.2 over an Indian land site whose output is compared then with the observations during the pre-monsoon (March–April–May; MAM) season of the year 2009. The simulations have been conducted over a nested domain centered around the location of a micrometeorological tower installed at Ranchi (23.412° N, 85.44° E), India (Fig. 3). Domain d01 (6×6 km2) consists of 233 east–west and 210 north–south grid points, and domain d02 (2×2 km2) consists of 223 east–west and 196 north–south grid points which cover a 1398×1260 and a 446×392 km2 spatial area around the center point, respectively. Each domain was configured with 50 vertical eta levels from the surface to the top of the atmosphere. We kept five vertical levels below 100 m height. Initial and boundary conditions were taken from an ERA5 global atmospheric reanalysis dataset at a resolution of 0.25° × 0.25°, and boundary conditions were forced every 6 h. For land use and land cover (LU/LC) information, we have used a dataset from MODIS (Moderate Resolution Imaging Spectroradiometer; Friedl et al., 2002). Various physical parameterizations utilized in the simulations are listed in Appendix C. In this study, four sets of simulations were carried out, as given in Table 1.
Note that the revised MM5 surface layer scheme has lower limits on the values of u∗ (>0.001 m s−1) and U (>0.1 m s−1) that allow nocturnal values of u∗ at night and control RiB values to be inordinately high, respectively (Jiménez et al., 2012). However, the stability parameter ζ or RiB is not restricted in the revised MM5 surface layer scheme, which gives complete freedom to the WRF model to show its sensitivity to the tested similarity functions (Jiménez et al., 2012). Moreover, some of the large-eddy simulation (LES) studies reported in the literature suggest that the friction velocity cannot be zero when the mean wind drops to zero; i.e., there should be a minimum friction velocity that is proportional to the w∗ (Schumann, 1988). For this purpose, the existing version of the revised MM5 scheme sets 0.001 m s−1 as the minimum value of u∗ based on the recommendations by Jiménez et al. (2012). Thus, to avoid the complexity that arises when mean wind drops to zero, the updated revised MM5 scheme proposed in the present study also utilizes a minimum value of u∗ (>0.001 m s−1) as suggested by Jiménez et al. (2012) in the existing version of the revised MM5 scheme. Moreover, the scheme uses constant values of z0, while the values of zh are calculated from the expression suggested by Brutsaert (1982).
The whole simulation period is divided into segments of 4 d with 24 h overlapping time between different segments to ensure continuity. The model is initialized at 00:00 UTC of the first day of each simulation and runs for 96 h. In order to avoid the potential spin-up problems at the beginning of the simulation, we discard the first day of each simulation as spin-up time and consider the last 3 d for the analysis (Jiménez et al., 2010, 2012).
For the evaluation of the real-case simulations, different statistical parameters such as mean absolute error (MAE), root mean square error (RMSE), mean bias (MB), index of agreement (IOA), different measures of correlation coefficient (CC), mean bias percent (bias %), and standard deviation of the model-predicted output normalized by that of the observations are used. A brief description of the performance indicators for validation utilized in the present study is given in Appendix C.
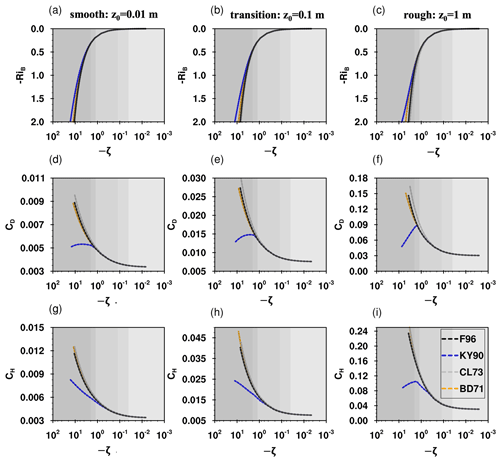
Figure 4Variation in ζ with RiB (a–c), CD (d–f), and CH (g–i) with ζ calculated from the bulk flux algorithm (offline simulation) for different functional forms of ψm and ψh corresponding to BD71, CL73, KY90, and F96 forms for smooth (z0=0.01 m; a, d, g), transition (z0=0.1 m; b, e, h), and rough (z0=1.0 m; c, f, i) surfaces. The background color corresponds to different sublayers in convective conditions (Kader and Yaglom, 1990), from the dynamic sublayer (; light gray) to the free convective sublayer (; dark gray).
4.1 Offline simulations
To analyze the functional dependence of ζ, CD, and CH on the utilized forms of similarity functions, the offline simulations independent of the WRF model have been conducted utilizing newly installed functions (BD71, CL73, and KY90) together with F96 functions existing in the default version of the surface layer scheme of the WRF model for three different roughness lengths for momentum (z0), which are representative of smooth (z0=0.01 m), transition (z0=0.1 m), and rough ( z0=1.0 m) surfaces. The results for ζ (Fig. 4a, b, and c) with RiB, CD (Fig. 4d, e, and f), and CH (Fig. 4g, h, and i) with ζ across various surface types and sublayers have been analyzed. The different sublayers associated with convective stratification include dynamic (DNS), dynamic and dynamic convective transition (DNS-DCS), dynamic convective (DCS), dynamic convective and free convective transition (DCS-FCS), and free convective (FCS) (Srivastava and Sharan, 2021). Note that the sublayers DNS () and DNS-DCS transition () correspond to weakly to moderately unstable conditions, while sublayers DCS (), DCS-FCS (), and FCS () belong to moderately to strongly convective conditions (Srivastava and Sharan, 2015).
It is found that the simulated values of ζ at smaller values of RiB (i.e., in DNS to DCS) from different forms of similarity functions are almost identical to the F96 functional forms (Fig. 4a–c). Moreover, results from the BD71, CL73, and F96 functions are even similar at higher instabilities (i.e., the whole range of ζ values), while they differ strongly from values obtained using the KY90 functions (Fig. 4a–c). Notably, BD71, CL73, and F96 functional forms predict relatively smaller absolute values of ζ for a given value of RiB. However, KY90 functions are found to produce a relatively larger magnitude of ζ for a given value of RiB. This behavior is found to be consistent for all ratios (Fig. 4a–c) representative of smooth, transition, and rough surfaces. A relatively larger magnitude of ζ for a given value of RiB and the smaller values of ψm and ψh (Fig. 2) in KY90 functional forms imply that the momentum and heat fluxes predicted using KY90 functions will be smaller than those anticipated in BD71, CL73, and F96 functional forms.
Figure 4d–f show the variation in CD with ζ estimated using BD71, CL73, KY90, and F96 functional forms over different surfaces. Notice that the CD values calculated from BD71, CL73, and F96 forms of functions are relatively higher than those produced by KY90 functional forms and continue to rise as instability progresses from DCS to FCS. It is important to highlight that CD estimated using KY90 functions shows a non-monotonic behavior, which is consistent with the observed behavior of CD over the Indian region reported in the literature (Srivastava and Sharan, 2019, 2021). Note that this non-monotonic behavior is consistent for all three cases of different roughness lengths (Fig. 4d–f).
On the other hand, across all three surfaces, one can see that the values of CH estimated from all four functional forms increase with increasing instability (Fig. 4g–i), while the rate of increase of CH in KY90 functions is relatively slower. Moreover, BD71, CL73, and F96 functions predict almost similar values over all three types of surfaces. Noticeably, CH estimated using KY90 functions also exhibits non-monotonic behavior with ζ over rough surfaces, which contradicts the predictions of the other three functional forms. In addition, it is important to note that CD and CH predicted by KY90 functional forms are found to be bounded by twice their near-neutral values, while the other functional forms predict continuously increasing values of CD and CH with increasing instability.
Note that the error caused by different values of z0 can be so large that the stability dependence of using different forms of similarity functions is less important in the computation of CD and CH. As a result, three different values of z0 have been chosen, similar to a recent study by Srivastava and Sharan (2021), which are representative of smooth (z0=0.01 m), transition (z0=0.1 m), and rough (z0=1.0 m) surfaces to account for the impacts of using different z0 on the estimation of CD and CH from different functional forms of similarity functions in offline simulations.
Moreover, Fig. 4 depicts the offline simulations with equal values of z0 and zh, while in the revised MM5 surface layer scheme available in the WRF model, the values of z0 and zh are not the same. Thus, we have also attempted to discuss the results from the offline simulations with different values of zh, assuming z0=0.1 m. Figure S2 shows the variation in ζ with RiB, CD, and CH with ζ calculated from the bulk flux algorithm using similarity functions corresponding to BD71, CL73, KY90, and F96 with different values of zh while z0 is fixed. The values of zh are taken such that the ratio assumes 0.1, 1, 2, 3, and 4. Figure S2 clearly shows that the estimated values of ζ are similar in near-neutral to moderately unstable conditions for all values of zh; however, relatively smaller values have been found as the ratio increases for each form of similarity function. Since the computation of CD does not involve the values of zh (Eq. B9), the estimated values of CD for each form of similarity function are found to be approximately the same for different values of zh. However, in the case of CH, differences are clearly visible if one uses different values of zh. The estimated CH using various similarity functions behaves similarly for different values of zh, while the magnitude decreases as the ratio increases.
Hence, it is evident that the BD71, CL73, and F96 functional forms predict values of ζ, CD, and CH that are almost the same over all three different surface types. However, using KY90 functions compared to other commonly used φm and φh, one can expect a significant reduction in the estimated values of transfer coefficients in moderately to strongly unstable stratification.
4.2 Results of the WRF model using different sets of integrated similarity functions
In this section, observational and reanalysis datasets have been used to analyze the simulations performed with WRFv4.2.2 utilizing newly installed and default φm and φh. The model-simulated output has been extracted at the location of the flux tower and compared against the observations derived from the flux tower installed at Ranchi (23.412° N, 85.440° E), India. The mean spatial patterns of certain variables averaged over daytime (04:00–12:00 UTC) have been compared against the ERA5-Land reanalysis dataset. Further, to assess the effects of newly installed functions under free convective conditions, the mean spatial patterns of considered variables averaged across strongly convective conditions (hours in which over most of the domain) have been analyzed against respective hours of ERA5-Land reanalysis data. Bilinear interpolation has been used to interpolate the model output to the same grid resolution as the ERA5-Land data in order to allow a consistent comparison.
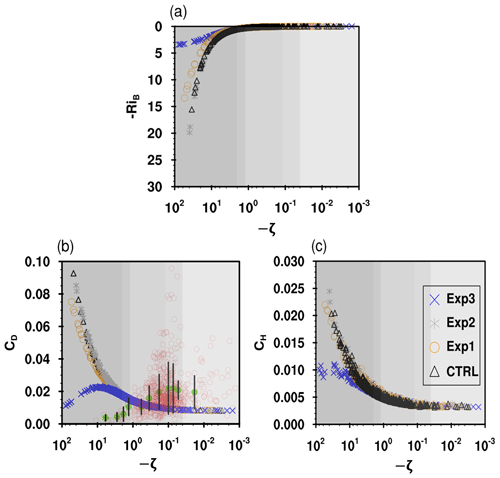
Figure 5Variation in model-simulated ζ with RiB (a), CD (b), and CH (c) with ζ from different experiments using different ψm and ψh corresponding to F96 (CTRL), BD71 (Exp1), CL73 (Exp2), and KY90 (Exp3) under convective conditions. The red circles in (b) denote the observed CD with ζ at the location of the flux tower. The mean values of observed CD in each sublayer are shown with solid green circles along with standard deviations in the form of error bars. Depending upon the data availability, two or three bins of equal width are chosen in each sublayer. The background color corresponds to different sublayers in convective conditions (Kader and Yaglom, 1990), from the dynamic sublayer (; light gray) to the free convective sublayer (; dark gray).
4.2.1 Evaluation against observations derived from the flux tower installed at Ranchi (India)
Figure 5 depicts the variation in (a) ζ with RiB, (b) CD, and (c) CH at the first model level with ζ from different experiments (Exp1, Exp2, and Exp3) and the CTRL simulation. Although the absolute values of the parameters differ from each other due to the different prescribed roughnesses, the variation in ζ with RiB, CD, and CH with ζ is very similar to the offline results. Note that, at the moment, due to the inaccessibility of long-term data on detailed surface properties such as vegetation structure needed to quantify the roughness length, we do not have access to the precise values of z0 and zh at the Ranchi station. Moreover, the values of z0 and zh are not directly involved in the estimation of CD, CH, and the surface fluxes from the observational data, while they are important in computing these variables using the MOST framework. Thus, the default value of z0 is used in the revised MM5 surface layer scheme available in the WRF model, which is found to be approximately in the range 0.1–0.2 m at the Ranchi station. We wish to highlight that the z0 used in the WRF model simulations at the Ranchi station is nearly similar to the case of z0=0.1 m presented in Fig. 4, and the offline simulations also indicate that the behavior of the estimated CD and CH with ζ remains almost the same for different values of z0 with slightly varying magnitudes. Thus, one can interpret the results of CD and CH shown in Figs. 4 and 5 from the offline simulations and the WRF model, respectively, and can compare the WRF-model-simulated CD with the observed one at the Ranchi station. Although the model simulations and observed data may have a different z0, the comparison of model-simulated variables with the Ranchi data allows for an impression of the structural behavior of model results as a function of stratification compared with measurements.
It is clear from Fig. 5 that the values of simulated variables are found to be almost identical in DNS to DCS sublayers for all the experiments. Moreover, in FCS, the results obtained from Exp1, Exp2, and the CTRL simulation are found to be nearly similar; however, relatively strong differences have been found in results from Exp3 (Fig. 5a, b, and c). Simulated ζ for a given RiB in Exp2 and the CTRL simulation are similar and found to be relatively smaller in magnitude than Exp1 and Exp3 in FCS. However, the absolute values of ζ in Exp3 (KY90 functions) are relatively higher in FCS than in all other experiments.
Figure 5b shows the variation in simulated CD with ζ from different experiments. Purple circles denote the variation in observed CD with ζ at the location of the flux tower (Fig. 5b). It is found that the observed CD increases as the instability increases from DNS to DCS and has the maximum value in the DCS (at approx.) and then starts to decrease as instability grows further from DCS to FCS. It is evident that CD simulated using φm and φh based on class 4 functions (Exp3) exhibits non-monotonic behavior (Fig. 5b), which is consistent with the observed behavior of CD (Srivastava and Sharan, 2015, 2021). The magnitude of CD predicted in Exp3 is significantly smaller than that simulated from other experiments as well as the CTRL simulation, specifically in FCS. This may be due to the large differences between the KY90 functional forms of ψm and ψh and other forms of functions. On the other hand, CD simulated using φm and φh based on the first three classes (Exp1, Exp2, and CTRL simulation) increases continuously as instability grows from DNS to FCS (Fig. 5b).
Table 2Comparison statistics for (m2 s−2), sensible heat flux (SHF) (W m−2), U10 (m s−1), and T2 m (K) simulated using different experiments together with the CTRL simulation with respect to observations derived from the flux tower at Ranchi (India) for the MAM season. The mean absolute error (MAE), root mean square error (RMSE), mean bias (MB), index of agreement (IOA), and correlation coefficient (CC) are shown. The highest (lowest) values of CC and IOA (MAE, RMSE, and MB) between different experiments are denoted by bold entries.
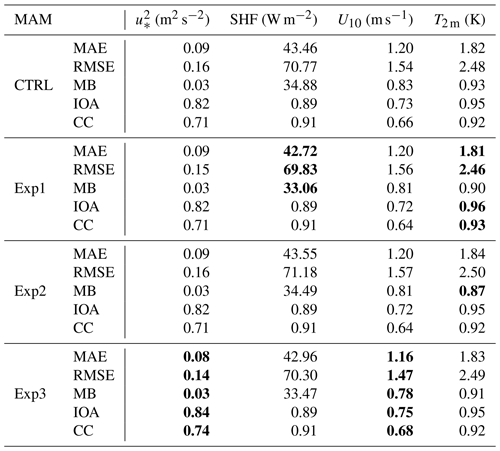
However, it is found that CD predicted from the original forms of class 4 functions (Exp3) shows a large disagreement with its observed behavior, as the predicted CD starts decreasing at ζ lying in FCS, which is different from that observed, i.e., ζ lying in DCS. As a result, the study also highlighted the necessity of fine-tuning the original KY90 functional forms and evaluating their performance in the WRF model with additional observational datasets from various land sites and seasons.
Note that Srivastava and Sharan (2021) tuned the original forms of class 4 functions by enforcing the matching of the point at which both observed and model-predicted CD attain their maximum value. However, more studies in terms of predicting the observed variation in the non-dimensional vertical gradients of mean wind speed and temperature with ζ are essential to further tune the original KY90 functions for the Indian region using observed data from various locations under different seasons.
Further, we would like to point out that currently no observational datasets are available which show a better agreement with the KY90 functions over the Indian region. However, it is desirable to further validate these functional forms over the Indian region once such observational datasets become available.
We wish to highlight that utilizing KY90 (Exp3) functions in the revised MM5 scheme of the WRF model makes it consistent in predicting CD with its observed non-monotonic behavior over the Indian region.
The variation in simulated CH with ζ from different experiments is shown in Fig. 5c. CH simulated from Exp1–3 as well as the CTRL simulation shows continuously increasing behavior with ζ. The magnitude of simulated CH from the CTRL simulation and Exp1–2 is relatively higher than that of Exp3 in FCS beyond (approximately). It is also evident that at higher instabilities, even CH shows non-monotonic behavior with ζ (Fig. 5c). We wish to point out that a relatively larger scatter has been found in the values of CH than CD. The WRF model utilizes constant values for z0, while zh is calculated using expression suggested by Brutsaert (1982). The relatively large scatter in the values of CH simulated from the WRF model can be due to the parameterization of the ratio of momentum and scalar roughness lengths in the model.
Note that the transfer coefficients CD and CH shown in Fig. 5 are at the reference height corresponding to the lowest model grid level, which is ∼12 m in the present study. However, we have also analyzed the behavior of CD and CH at 10 m height with ζ and found that they behave similarly to those presented in Fig. 5.
The analysis presented here indicates that the KY90 functions in the revised MM5 surface layer scheme are found to be appropriate in producing non-monotonic behavior of CD consistent with its observed nature. However, all other functional forms of φm and φh produce CD, which increases continuously with ζ from DNS to FCS.
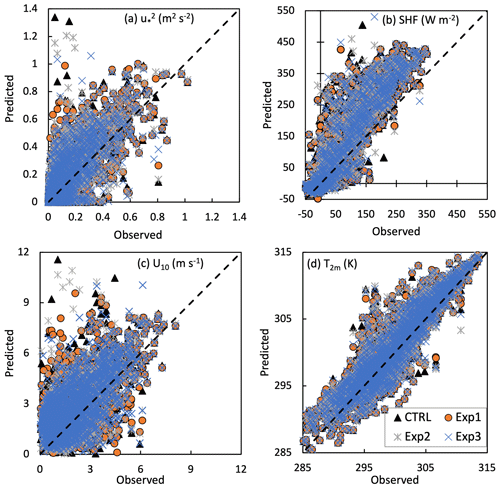
Figure 6Scatter plot of model-simulated (representative of momentum flux) (a), SHF (sensible heat flux) (b), U10 (wind speed at 10 m height) (c), and T2 m (temperature at 2 m height) (d) vs. observed values at the location of the flux tower at Ranchi (23.412° N, 85.440° E), India, during the pre-monsoon season (MAM).
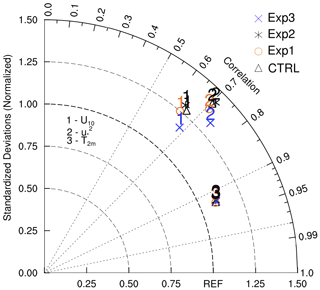
Figure 7Taylor diagram showing the correlation coefficient and normalized standard deviations for U10, , and T2 m from different experiments together with the CTRL simulation with respect to observations derived from the flux tower installed at Ranchi (23.412° N, 85.440° E), India.
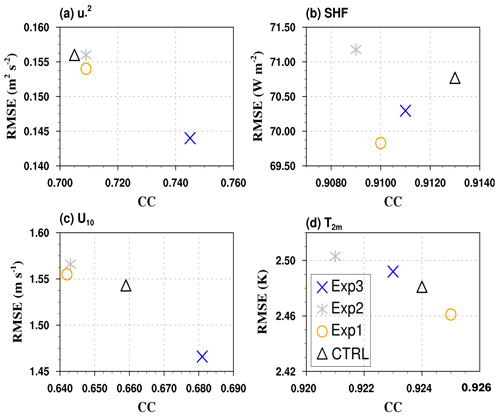
Figure 8Scatter plot between correlation coefficient (CC) and root mean square error (RMSE) for (a), SHF (b), U10 (c), and T2 m (d) simulated by various experiments (Exp1–3) together with the CTRL simulation for the pre-monsoon season (MAM) at the location of the flux tower at Ranchi (23.412° N, 85.440° E), India.
To quantify the uncertainties involved in the simulated surface fluxes and certain near-surface variables using KY90 (Exp3) as well as other functional forms (Exp1–2 and CTRL simulation), model simulations have been compared against the observations. Figure 6 compares the model-simulated (a) (m2 s−2) (representative of momentum flux), (b) SHF (W m−2) (sensible heat flux), (c) U10 (m s−1) (10 m wind speed), and (d) T2 m (K) (2 m temperature) with the observed data obtained from the flux tower at Ranchi (23.412° N, 85.440° E), India. The model output was extracted at a single grid point closest to the flux tower to allow a consistent comparison. In Fig. 7, a Taylor diagram is displayed along with the normalized standard deviations and correlations of considered variables. Figure 8 shows the scatter plot between CC and RMSE for considered variables simulated using different experiments. In the case of , Exp1 and Exp2 are found to be comparable to the CTRL simulation, while Exp3 considerably improved the simulation of (Figs. 6a, 7 and 8). Exp3 reduced MAE (RMSE) from 0.09 (0.16) m2 s−2 to 0.08 (0.14) m2 s−2 (Table 2; Figs. 7 and 8) and improved the CC (0.74) and IOA (0.84) for (Table 2). A Q–Q plot is shown in Fig. S3a suggesting that Exp3 (KY90 functions) is found to be slightly better than all other experiments and the CTRL simulation for . For SHF, all the experiments are comparable to the CTRL simulation; however, Exp3 shows less scatter than other experiments (Fig. 6a).
In the case of U10, Exp3 shows less scatter and appears to be closer to the observations than other experiments (Fig. 6c). Exp3 noticeably improved the simulation of U10 by reducing MAE (RMSE) from 1.20 (1.54) m s−2 to 1.16 (1.47) m s−2 and MB up to 5 % (Figs. 6c, and 7; Table 2). It considerably improved the CC (IOA) for U10 from 0.66 (0.73) to 0.68 (0.75) (Fig. 7 and Table 2). A Q–Q plot (Fig. S3b) reveals that Exp3 is found to be better than all other experiments and the CTRL simulation for U10. Thus, the KY90 functions in the surface layer scheme of the WRF model considerably improve the model in simulating U10 (Figs. 6c, 7, 8, and S2b) at the location of the flux tower. Further, in the case of T2 m, Figs. 7 and 8 show that all the experiments are found to be comparable with the CTRL simulation.
Note that earlier studies, especially the ones done in the GEWEX Atmospheric Boundary Layer Study (GABLS) model intercomparison projects, have studied the impacts of the similarity functions on the modeled profiles and fluxes (though mostly for stable conditions). However, they learned that applying different stability functions in the surface and boundary layer parameterizations may trigger unnatural kinks in the model-simulated wind speed and temperature profiles. Here, we have analyzed the profiles of U10 and T2 m simulated from the WRF model using different similarity functions in the surface layer scheme for the occurrence of unnatural kinks in their values. One can see that the U10 predicted from the CTRL simulation, as well as different experiments corresponding to different similarity functions at certain hours, goes higher than that of its observed maximum value (approx. 8 m s−1) (Fig. S4). These relatively higher magnitudes may be linked with some localized weather phenomenon characterized by rapid changes in weather including strong wind, lightning, and thunderstorms and are justifiable. However, the simulated T2 m from different similarity functions are found to be in line with the observed values across the whole simulation period (Fig. S5). This suggests that the values of U10 and T2 m predicted from the WRF model are found to be in a justifiable range and no unnatural kinks have been found.
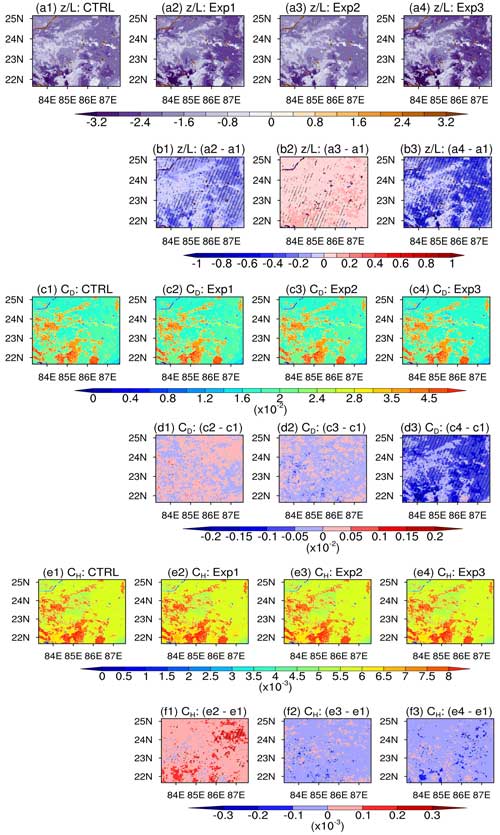
Figure 9Mean spatial distribution of model-simulated ζ (a), CD (c), and CH (e) from different experiments and their differences (b, d, f) with respect to the CTRL simulation averaged during daytime for the whole simulation period. Hatched regions show significant differences at 95 % confidence level in experiments with respect to the CTRL simulation.
4.2.2 Evaluation of mean spatial distribution of simulated variables against ERA5-Land reanalysis data during daytime
In this section, the mean spatial distribution of simulated variables from different experiments, as well as the CTRL simulation averaged during daytime (04:00–12:00 UTC) for the entire simulation period, is compared with the ERA5-Land reanalysis data. Figure 9 depicts the mean spatial patterns of simulated (a1–a4), CD (c1–c4), and CH (e1–a4) from the CTRL simulation and other experiments, as well as their differences with respect to the CTRL simulation. It is found that the absolute value of ζ simulated in Exp3 (KY90 functions) is relatively smaller than the CTRL simulation (Fig. 9b3) across the whole domain, which is consistent with Fig. 5a, and offline simulations presented in Fig. 4a–c. This could be because the magnitude of KY90 functions (φm and φh) (Fig. S1) is relatively smaller than the functions employed in the default scheme (CTRL simulation).
On the other hand, Exp1 also provides slightly smaller absolute values of ζ (Fig. 9b1), while Exp2 is almost comparable to the CTRL simulation (Fig. 9b2). Model-simulated CD is found to be relatively smaller in Exp3 than the CTRL simulation (Fig. 9d3), while Exp1 and Exp2 provide comparable values of CD to the CTRL simulation (Fig. 9d1–2). In the case of CH, the simulated values from different experiments are comparable to the CTRL simulation over the whole study domain (Fig. 9f1–3). Note that simulated CH is found to be comparable in all the experiments, while one can see slight differences in CD in Exp3 compared to all other experiments, which may be related to the fact that only φm functions are involved in the computation of CD (Eq. 1) and the differences between φm corresponding to Exp3 are relatively more than φh, as are the differences in CD. The hatched regions in Fig. 9 show that the differences between simulated variables from different experiments with respect to the CTRL simulation are statistically significant at 95 % confidence level.
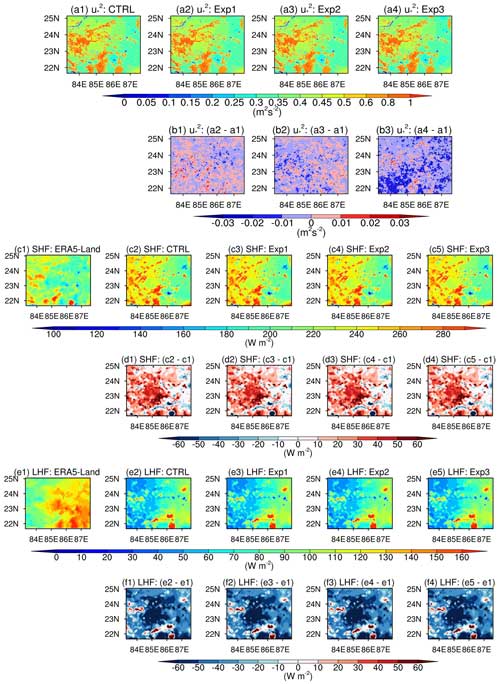
Figure 10Mean spatial distribution of simulated (a) from different experiments and their differences (b) with respect to the CTRL simulation. SHF (c) and LHF (e) from ERA5-Land reanalysis and simulated using various experiments and their differences (d, f) with respect to ERA5-Land data averaged during daytime for the whole simulation period are shown. Hatched regions show significant differences at 95 % confidence level in experiments with respect to the CTRL simulation.
The slight differences in CD in Exp3 are reflected further in the simulated m2 s−2 (a measure of momentum flux) (Fig. 10b3). A slight reduction has been found in simulated in Exp3 compared to the CTRL simulation over some parts of the domain (Fig. 10b3), while in Exp1 and Exp2 values are comparable with the CTRL simulation (Fig. 10b1–2). In the case of SHF and latent heat flux (LHF), the mean spatial distribution from all the experiments is found to be consistent with the ERA5-Land reanalysis data, and the magnitude of differences between model simulation and ERA5-Land data is comparable for all the experiments (Table S1 in the Supplement).
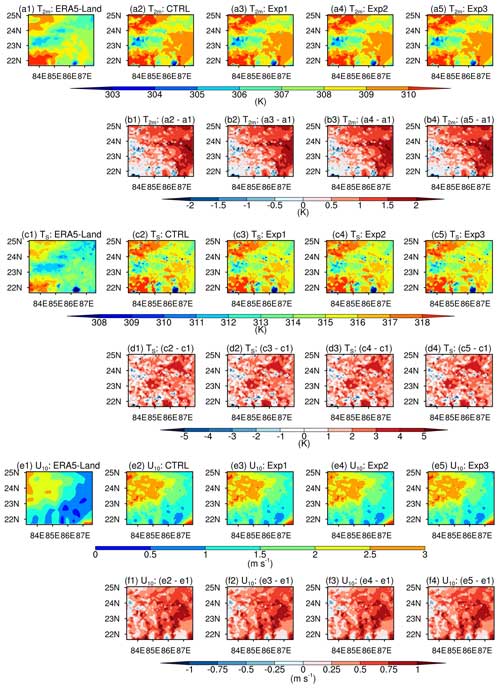
Figure 11Mean spatial distribution of T2 m (upper panels), TS (middle panels), and U10 (lower panels) from ERA5-Land reanalysis (a1, c1, e1, respectively) and different experiments (a2–a5, c2–c5, e2–e5, respectively) and their differences with respect to ERA5-Land reanalysis (b1–b4, d1–d4, f1–f4, respectively) averaged during daytime for the whole simulation period.
For T2 m (upper panels of Fig. 11), TS (middle panels of Fig. 11), and U10 (lower panels of Fig. 11), the mean spatial distribution from different experiments and the CTRL simulation agreed well with slightly varying magnitude with the ERA5-Land reanalysis data. One can see a warm bias up to 2 K (3 K) for T2 m (TS) simulated from different experiments and the CTRL simulation over most of the domain. For T2 m, bias, RMSE, and pattern correlation coefficient (PCC) between different experiments together with the CTRL simulation and ERA5-Land reanalysis data are found to be comparable (Table S1). However, Exp3 slightly improved the PCC from 0.50 to 0.51 for TS (Table S1). Further, in the case of U10, all the simulations exhibit an overprediction over the whole domain (lower panels of Fig. 11: f1–f4), and Exp3 is found to be slightly better than all other experiments as well as the CTRL simulation as it reduced bias % (RMSE) from 32.28 (0.54) m s−2 to 32.06 (0.53) m s−2 and improved the PCC from 0.89 to 0.91 (Table S1).
4.2.3 Evaluation of newly installed functions during strongly unstable conditions with respect to ERA5-Land reanalysis data
This section describes the impacts of utilizing different similarity functions (φm and φh) on simulated variables during highly convective regimes (i.e., ) with respect to the ERA5-Land reanalysis dataset. Since the functional forms of ψm and ψh are almost identical in near-neutral to moderately unstable conditions, however, in strongly unstable conditions, the differences between different functional forms are more pronounced. Thus, the corresponding differences in the simulated values of considered variables are expected to be more pronounced during highly convective regimes. For this purpose, the model output has been extracted for those hours in daytime which show ζ smaller than −10 over most of the domain and compared with the respective hours of ERA5-Land reanalysis data.
Figure S6 depicts the mean spatial distribution of ζ (a1–4), CD (c1–4), and CH (e1–4) as well as their deviations from the CTRL simulation. Notice that the magnitudes of differences for all variables (ζ, CD, and CH) in this case are found to be larger than the case of mean spatial patterns averaged during the whole daytime (Sect. 6.2.2). It is evident from Fig. S6b3 that Exp3 produces large absolute values of ζ and smaller values of CD and CH (Fig. S6b3, d3, and f3) than all other experiments and the CTRL simulation, while Exp1 and Exp2 are found to be comparable to the CTRL simulation for both CD and CH (Fig. S6d1–2 and f1–2).
Table 3Comparison statistics for T2 m (K), TS (K), and U10 (m s−1) simulated using different experiments together with the CTRL simulation with respect to ERA5-Land reanalysis data averaged during strongly unstable stratification (hours during daytime in which ζ is smaller than −10) for the whole simulation period. The mean bias percent (bias %), pattern correlation coefficient (PCC), and root mean square error (RMSE) are shown. The lowest (highest) values of bias and RMSE (PCC) between different experiments are denoted by bold entries.
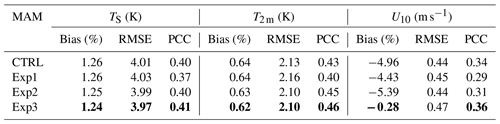
The model simulations for T2 m and TS do not capture the spatial patterns well in comparison to ERA5-Land data (Figs. S7a1–5 and S8a1–5). All experiments, as well as the CTRL simulation, exhibit an overprediction across the whole domain (Figs. S7b1–4 and S8b1–4). We wish to highlight that the differences between various experiments and the CTRL simulation are seen up to 0.5 K for T2 m (Fig. S7c1–3) as well as TS (Fig. S8c1–3), which is slightly higher than the case of mean spatial patterns averaged over the whole daytime (upper and middle panels of Fig. 11). For T2 m, it is evident from Fig. S7 and Table 3 that Exp3 noticeably reduced the bias % (RMSE) from 0.64 (2.13) K to 0.62 (2.10) K and improved the PCC from 0.43 to 0.46 (approximately 6 %). In the case of TS as well, Exp3 slightly improved the PCC and reduced the bias % (RMSE) from 1.25 (4.01) K to 1.24 (3.97) K (Table 3 and Fig. 12).
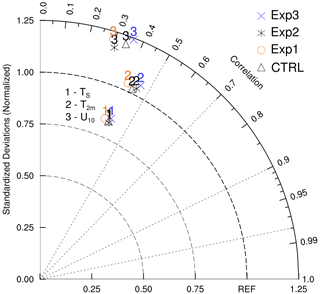
Figure 12Taylor diagram showing the correlation coefficient and normalized standard deviations for TS (K), T2 m (K), and U10 (m s−1) from different experiments together with the CTRL simulation with respect to the ERA5-Land reanalysis dataset averaged during strongly convective conditions (hours during daytime in which ζ is smaller than −10) for the whole simulation period.
For U10, the mean spatial patterns simulated using different experiments agreed well with the ERA5-Land reanalysis data (Fig. S9a1–5), and the magnitude of biases is found to be up to 1 m s−1. Exp3 outperformed all other experiments and the CTRL simulation by lowering the bias % from −4.96 to −0.28 m s−1 and improved the PCC from 0.34 to 0.36 with comparable RMSE values (Figs. S9 and 12; Table 3).
The results presented so far suggest that the changes corresponding to different functional forms of similarity functions in the surface layer parameterization of the WRF model are more pronounced in convective conditions during daytime hours. For the number of grid points over the study domain that are being affected by the changed similarity functions, no fixed pattern was found; however, the changes depend on the considered variable and similarity functions. Furthermore, we observe that the changes are more pronounced in grids that experience strong instability during the daytime.
In the present study, the revised MM5 surface layer scheme of the WRFv4.2.2 model has been modified to incorporate φm and φh as suggested by Kader and Yaglom (1990) to make it consistent in producing the transfer coefficient for momentum (CD) in line with its observed behavior. The revised MM5 scheme is modified in such a way that it contains all commonly used φm and φh under convective conditions instead of a single functional form. Various alternatives of φm and φh in the modified scheme can be controlled by a flag (psimhu_opt) that has been introduced in the physics section of the namelist file. The impacts of utilizing different functional forms of φm and φh in the proposed scheme have been evaluated using offline simulations (with a bulk flux algorithm) as well as real-case simulations with the WRFv4.2.2 model. The model-simulated surface turbulent fluxes and certain near-surface variables have been compared with observational data from a flux tower at Ranchi (23.412° N, 85.440° E), India, and the spatial patterns have been evaluated with the ERA5-Land reanalysis dataset.
Offline simulations indicate that at nearly neutral to moderately unstable conditions, ζ simulated using various functional forms of φm and φh is comparable, and as the instability grows (free convective conditions), the differences between different experiments become more pronounced. This might be connected to the corresponding variations between different functional forms of similarity functions in the respective regimes. Similarly, for simulated CD, Exp3 (KY90 functions) demonstrates non-monotonic behavior with −ζ across all three surface types (representing smooth, transition, and rough surfaces), which is consistent with its observed behavior. However, all other experiments and the CTRL simulation indicate continuously increasing CD with −ζ from near-neutral to free convective conditions over all three surface types, which is inconsistent with its observed behavior over the study domain. The non-monotonic behavior of CD in Exp3 (KY90 functions) may be associated with the analogous non-monotonic behavior of the corresponding ψm in the respective regime.
In real-case simulations, the model-simulated ζ, CD, and CH are found to be consistent with the offline simulations. One can see that the variation in CD in Exp3 (KY90 functions) with −ζ is non-monotonic, as reported in offline simulations and consistent with its observed behavior. This indicates that the KY90 functions in the surface layer scheme of the WRF model make it compatible in producing CD consistent with its observed behavior over the Indian region. As compared with the observations over Ranchi (India), the simulations using KY90 (Exp3) functions are found to perform better for most of the considered variables compared to all other experiments. Further, in the mean spatial distribution averaged during daytime (04:00–12:00 UTC) over the entire simulation period, the significant increase in absolute value of ζ from Exp3 resulted in a noticeable reduction in the values of CD and CH, which further impacted the simulated values of TS, T2 m, and U10. When compared with the ERA5-Land reanalysis data, the spatial patterns for T2 m, TS, and U10 from Exp3 (KY90 functions) provided more consistent results. A reduction has been found in bias (%) and RMSE values for T2 m, TS, and U10. Moreover, in the case of highly convective regime (), Exp3 (KY90 functions) slightly improved the performance of the model by reducing the bias (%) and RMSE for T2 m, TS, and U10 and increasing the correlation to some extent.
Thus, it is concluded that the similarity functions proposed by Kader and Yaglom (1990) (KY90 functions; Exp3) are found to be more appropriate for use in the WRF model as they can simulate CD that is consistent with its observed behavior and improve the simulation for most of the considered variables over the study domain. However, due to the limited spatial coverage of the domain considered in this study and the limited availability of observational data, KY90 functional forms need to be further evaluated in the WRF modeling framework utilizing observations from other sites. The modified surface layer scheme proposed in this study could enhance the potential applicability of the WRF modeling framework for the community in investigating the role of different functional forms of similarity functions under convective conditions for selected events and case studies such as extreme weather events, heat waves during summer, cyclonic storms, and fog predictions using the WRF model.
Here, the detailed description of commonly used functions (φm and φh) in numerical models under convective conditions is provided.
Based on Businger (1966) and Arch J. Dyer (1965, unpublished work; see Businger, 1988, for details) the expressions for φm and φh are as follows:
in which γm=15, γh=9, and Prt=0.74 is the turbulent Prandtl number. Note that in the case of Dyer (1974) the values are and Prt=1.0. These functions are commonly known as Businger–Dyer (BD) similarity functions, and they do not satisfy the classical free convection limit (Srivastava et al., 2021).
The similarity functions proposed by Carl et al. (1973) under convective conditions are applicable for the range . The expressions for φm and φh suggested by Carl et al. (1973) are
in which . However, based on various studies reported in the literature βm and βh can take different values. For example, Delage and Girard (1992) proposed ; on the other hand, Fairall et al. (1996) suggested .
Fairall et al. (1996, 2003) proposed an interpolation function applicable for the entire range of atmospheric instability, which was based on BD functions and functions suggested by Carl et al. (1973). This interpolation function does not have the gradient form (φm and φh), as they have interpolated the integrated forms of the functions. We wish to highlight that the revised MM5 surface layer scheme of the Weather Research and Forecasting model version 4.2.2 utilized the interpolation functions suggested by Fairall et al. (1996).
Kader and Yaglom (1990) proposed a three-sublayer model under convective conditions. The dynamic sublayer corresponds to near-neutral conditions in which φm=1 and φh=Prt. Further, in the dynamic convective sublayer, mechanical energy is in the x direction, while buoyancy-induced energy is in the z direction. Thus, in this sublayer, the functional forms for similarity functions, as determined by dimensional analysis, are
in which Au and AT are constants.
Moreover, in the free convective sublayer, buoyancy dominates the mechanical production of energy, and the pressure redistribution term feeds the buoyant energy in the vertical direction into the horizontal direction (Kader and Yaglom, 1990). Thus, in this case, the dimensional analysis suggests
in which Bu and BT are constants.
Thus, under unstable conditions, φm exhibits a non-monotonic behavior with respect to −ζ, as the three-sublayer theory suggested that for sufficiently large values of −ζ, φm varies as the power of ζ, in contrast to the case of the free convection limit, where both φm and φh follow the power law. In the literature, various expressions for φm and φh are available based on the Kader and Yaglom (1990) three-sublayer model. However, the present study employs φm and φh based on the expressions implemented in the surface layer scheme (CLM4.0) of the NCAR-CAM5 (Zeng et al., 1998) model. The expressions for φm and φh utilized in this study are as follows:
and
Srivastava and Sharan (2021) classified these commonly used similarity functions stated above into four different classes based on the exponents appearing in the expressions of φm and φh. The classification is as follows.
Class 1. This class consists of functions having the exponents and for φm and φh (as in Eqs. A1 and A2), respectively, from near-neutral to strongly unstable conditions. φm and φh proposed by Businger et al. (1971) and Hogstrom (1996) are the examples of class 1 functions.
Class 2. In this class, the similarity functions (φm and φh) having the exponent for φm and φh for the entire range from near-neutral to moderately unstable conditions (as in Eqs. A3 and A4), respectively, are included. The functional forms suggested by Carl et al. (1973) are the example of class 2 functions.
Class 3. φm and φh having the exponents and , respectively, in near-neutral conditions but in strongly unstable conditions are included in this class. φm and φh based on Fairall et al. (1996), Grachev et al. (2000), and Fairall et al. (2003) are some examples of class 3 functions.
Class 4. Functional forms of φm and φh having the exponents and , respectively, in near-neutral conditions but for φm and for φh in strongly unstable conditions are classified in this class (as in Eqs. A9 and A10). The three-sublayer model for φm and φh suggested by Kader and Yaglom (1990) (Zeng et al., 1998) is one of the examples of functions in this class.
This section consists of a brief description of the computation of surface turbulent fluxes in the revised MM5 surface layer scheme. In a homogeneous surface layer, the dimensionless wind and temperature gradients are defined as
where L denotes the Obukhov length scale and U is the wind speed at height z; k represents the von Karman constant, and its value is taken as 0.4. Integrating Eqs. (B1) and (B2) with respect to z leads to
in which ψm and ψh denote the integrated form of similarity functions φm and φh. The roughness lengths for momentum and heat are denoted by z0 and zh, respectively. The ground and surface air potential temperatures are denoted by θg and θa, respectively. is the stability parameter and is defined as
ψm and ψh can be calculated from the following expression (e.g., Panofsky and Dutton, 1984):
The bulk Richardson number (RiB) is given by
Substituting the values of U and (θa−θg) from Eqs. (B3) and (B4) in Eq. (B7), one gets
Note that Eq. (B8) is a transcendental equation, and for a given value of RiB, the corresponding ζ value can be calculated using any iterative method.
The bulk transfer coefficients for momentum (CD) and heat (CH) are defined as
Once we get CD and CH, then the momentum (τ) and sensible heat (H) fluxes are calculated using the following expressions:
In this section, the details of various physical parameterizations utilized in the real-case simulations using the WRFv4.2.2 model and the different statistical indicators used for model evaluation are explained.
The real-case simulations with the WRFv4.2.2 model utilized the Purdue Lin microphysics scheme (Lin et al., 1983), the YSU (Hong et al., 2006) planetary boundary layer (PBL) scheme, the Kain–Fritsch (Kain, 2004) cumulus scheme, the Dudhia (Dudhia, 1989) shortwave scheme, the rapid radiative transfer model (RRTM) (Mlawer et al., 1997) longwave scheme, the Noah-MP land surface model (Niu et al., 2011), and the revised MM5 surface layer scheme (Jiménez et al., 2012).
In the present study, different statistical indicators have been used for the model evaluation with respect to observations and reanalysis datasets. Statistical parameters such as mean absolute error (MAE), root mean square error (RMSE), mean bias (MB), index of agreement (IOA), and correlation coefficient (CC) are defined as follows:
-
-
-
-
-
in which pi and oi represent the predicted and observed time series, respectively, while and are the predicted and observed means for a considered variable, respectively;
-
Taylor diagrams exhibit how well patterns match each other in terms of their correlation, ratio of their variances, and root mean square differences (Taylor, 2001);
-
Q–Q plots are a graphical technique used to compare the overall distribution of predicted and observed values for a variable (Venkatram, 1999).
The error or deviation between observed and simulated values is measured by MAE, RMSE, and MB. On the other hand, IOA is used to assess the trend relationship or how closely the magnitudes and signs of the observed values are related to the projected values (Schluenzen and Sokhi, 2008). In order to evaluate the spatial patterns with the ERA5-Land reanalysis dataset, statistical metrics such as mean bias (%), RMSE, and pattern correlation coefficient (PCC) have been used.
The Weather Research and Forecasting model version 4.2.2 (WRFv4.2.2) is an open-source model and can be downloaded from https://github.com/wrf- model/WRF/releases/tag/v4.2.2 (Skamarock et al., 2019). The model output at the location of the flux tower at Ranchi (23.412° N, 85.440° E), India, is openly available at https://doi.org/10.5281/zenodo.10435513 (Namdev et al., 2023b). The raw observational data derived from the flux tower at Ranchi utilized in the present study can be obtained from the Indian National Centre for Ocean Information Service upon request (https://odis.incois.gov.in/portal/datainfo/ctczdata.jsp, ESSO, 2024; Dwivedi et al., 2015). Hourly ERA5-Land reanalysis data utilized in this study can be found on the official website at https://doi.org/10.24381/cds.e2161bac (Muñoz Sabater, 2019).
The supplement related to this article is available online at: https://doi.org/10.5194/gmd-17-8093-2024-supplement.
All authors contributed to the design of the study, analysis, and writing of the manuscript. PN carried out the computations as well as the analysis of the model output.
The contact author has declared that none of the authors has any competing interests.
Publisher's note: Copernicus Publications remains neutral with regard to jurisdictional claims made in the text, published maps, institutional affiliations, or any other geographical representation in this paper. While Copernicus Publications makes every effort to include appropriate place names, the final responsibility lies with the authors.
We would like to thank Manoj Kumar for providing observational data at Ranchi. The authors acknowledge the use of the NCAR-NCL and ERA5-Land reanalysis dataset for this study. The use of the supercomputing facility (HPC) provided by IIT Delhi is gratefully acknowledged. We wish to thank the reviewers for their helpful comments and suggestions which have significantly enhanced the quality of this paper.
This research is partially supported under the INSA Senior Scientist Programme, the DST Centre of Excellence for Climate Information at IIT Delhi, and the YES Foundation. Piyush Srivastava has been supported by the DST Inspire Faculty Fellowship.
This paper was edited by Nicola Bodini and reviewed by Christof Lüpkes and one anonymous referee.
Bourassa, M., Gille, S., Bitz, C., Carlson, D., Cerovecki, I., Clayson, C., Cronin, M., Drennan, W., Fairall, C., Hoffman, R., Magnusdottir, G., Pinker, R., Renfrew, I., Serreze, M., Speer, K., Talley, L. and Wick, G.: High-Latitude Ocean and Sea Ice Surface Fluxes: Challenges for Climate Research, B. Am. Meteorol. Soc., 94, 403–423, https://doi.org/10.1175/BAMS-D-11-00244.1, 2013.
Brutsaert, W.: Evaporation into the Atmosphere: Theory, History, and Applications. Springer, Dordrecht, 299, https://doi.org/10.1007/978-94-017-1497-6, 1982.
Brutsaert, W.: Stability Correction Functions for the Mean Wind Speed and Temperature in the Unstable Surface Layer, Geophys. Res. Lett., 19, 469–472, https://doi.org/10.1029/92GL00084, 1992.
Businger, J. A.: Transfer of heat and momentum in the atmospheric boundary layer, Proceedings of the Symposium on the Arctic Heat Budget and Atmospheric Circulation, edited by: Fletcher, J. O., The Rand Corporation, 305–332, 1966.
Businger, J. A.: A Note on the Businger-Dyer Profiles: (Research Note), in: Topics in Micrometeorology, A Festschrift for Arch Dyer, 145–151, Springer, Dordrecht, the Netherlands, 1988.
Businger, J. A., Wyngaard, J. C., Izumi, Y., and Bradley, E. F.: Flux-Profile Relationships in the Atmospheric Surface Layer in the Atmospheric Surface Layer, J. Atmos. Sci., 28, 181–189, https://doi.org/10.1175/1520-0469(1971)028<0181:FPRITA>2.0.CO;2, 1971.
Carl, D. M., Tarbell, T. C., and Panofsky, H. A.: Profiles of Wind and Temperature from Towers over Homogeneous Terrain, J. Atmos. Sci., 30, 788–794, https://doi.org/10.1175/1520-0469(1973)030<0788:POWATF>2.0.CO;2, 1973.
Cheng, Y. and Brutsaert, W.: Flux-Profile Relationships for Wind Speed and Temperature in the Stable Atmospheric Boundary Layer, Bound.-Lay. Meteorol., 114, 519–538, https://doi.org/10.1007/s10546-004-1425-4, 2005.
de Bruin, H. A. R.: A Note on Businger's Derivation of Nondimensional Wind and Temperature Profiles under Unstable Conditions, J. Appl. Meteor. Climatol., 38, 626–628, https://doi.org/10.1175/1520-0450(1999)038<0626:ANOBSD>2.0.CO;2, 1999.
Delage, Y. and Girard, C.: Stability functions correct at the free convection limit and consistent for for both the surface and Ekman layers, Bound.-Lay. Meteorol., 58, 19–31, https://doi.org/10.1007/BF00120749, 1992.
Dudhia, J.: Numerical Study of Convection Observed during the Winter Monsoon Experiment Using a Mesoscale Two-Dimensional Model, J. Atmos. Sci., 46, 3077–3107, https://doi.org/10.1175/1520-0469(1989)046<3077:NSOCOD>2.0.CO;2, 1989.
Dwivedi, A. K., Chandra, S., Kumar, M., Kumar, S., and Kumar, N. V. P. K.: Spectral Analysis of Wind and Temperature Components during Lightning in Pre-Monsoon Season over Ranchi, Meteorol. Atmos. Phys., 127, 95–105, https://doi.org/10.1007/s00703-014-0346-0, 2015.
Dyer, A. J.: A Review of Flux-Profile Relationships, Bound.-Lay. Meteorol., 7, 363–372, https://doi.org/10.1007/BF00240838, 1974.
ESSO: CTCZ – Data Availability, ESSO – Indian National Centre for Ocean Information Services [data set], https://odis.incois.gov.in/portal/datainfo/ctczdata.jsp, last access: 6 November 2024.
Fairall, C. W., Bradley, E. F., Rogers, D. P., Edson, J. B., and Young, G. S.: Bulk Parameterization of Air-Sea Fluxes for Tropical Ocean global Atmosphere Coupled-Ocean Atmosphere Response Experiment, J. Geophys. Res., 101, 3747–3764, https://doi.org/10.1029/95JC03205, 1996.
Fairall, C. W., Bradley, E. F., Hare, J. E., Grachev, A. A., and Edson, J. B.: Bulk Parameterization of Air–Sea Fluxes: Updates and Verification for the COARE Algorithm, J. Climate, 16, 571–591, https://doi.org/10.1175/1520-0442(2003)016<0571:BPOASF>2.0.CO;2, 2003.
Friedl, M. A., McIver, D. K., Hodges, J. C. F., Zhang, X. Y., Muchoney, D., Strahler, A. H., Woodcock, C. E., Gopal, S., Schneider, A., Cooper, A., Baccini, A., Gao, F., and Schaaf, C.: Global Land Cover Mapping from MODIS: Algorithms and Early Results, Remote Sens. Environ. 83, 287–302, https://doi.org/10.1016/S0034-4257(02)00078-0, 2002.
Grachev, A. A., Fairall, C. W., and Bradley, E. F.: Convective Profile Constants Revisited, Bound.-Lay. Meteorol., 94, 495–515, https://doi.org/10.1023/A:1002452529672, 2000.
Grachev, A. A., Andreas, E. L., Fairall, C. W., Guest, P. S., and Persson, P. O. G.: SHEBA Flux-Profile Relationships in the Stable Atmospheric Boundary Layer, Bound.-Lay. Meteorol., 124, 315–33, https://doi.org/10.1007/s10546-007-9177-6, 2007.
Grell, G. A., Dudhia, J., and Stauffer, D.: A description of the fifth-generation Penn State/NCAR Mesoscale Model (MM5) (No. NCAR/TN-398+STR), University Corporation for Atmospheric Research, https://doi.org/10.5065/D60Z716B, 1994.
Gryanik, V., Lüpkes, C., Grachev, A., and Sidorenko, D.: New Modified and Extended Stability Functions for the Stable Boundary Layer based on SHEBA and Parametrizations of Bulk Transfer Coefficients for Climate Models, J. Atmos. Sci., 77, 26872716, https://doi.org/10.1175/JAS-D-19-0255.1, 2020.
Hicks, B. B.: Wind Profile Relationships from the “Wangara” Experiment, Q. J. Roy. Meteor. Soc., 102, 535–551, https://doi.org/10.1002/qj.49710243304, 1976.
Hogstrom, U.: Review of Some Basic Characteristics of the Atmospheric Surface Layer, Bound.-Lay. Meteorol., 78, 215–246, https://doi.org/10.1007/BF00120937, 1996.
Holtslag, A. A. M. and De Bruin, H. A. R.: Applied Modeling of the Night-time Surface Energy Balance over Land, J. Appl. Meteor. Climatol., 27, 689–704, https://doi.org/10.1175/1520-0450(1988)027<0689:AMOTNS>2.0.CO;2, 1988.
Hong, S., Noh, Y., and Dudhia, J.: A New Vertical Diffusion Package with an Explicit Treatment of Entrainment Processes, Mon. Weather Rev., 134, 2318–2341, https://doi.org/10.1175/MWR3199.1, 2006.
Izumi, Y.: Kansas 1968 Field Program Data Report. Bedford, MA, Air Force Cambridge Research Papers, No. 379, 79 pp., 1971.
Jiménez, P. A., González-Rouco, J. F., García-Bustamante, E., Navarro, J., Montávez, J. P., de Arellano, J. V., Dudhia, J., and Muñoz-Roldan, A.: Surface Wind Regionalization over Complex Terrain: Evaluation and Analysis of a High-Resolution WRF Simulation, J. Appl. Meteor. Climatol., 49, 268–287, https://doi.org/10.1175/2009JAMC2175.1, 2010.
Jiménez, P. A., Dudhia, J., González-Rouco, J. F., Navarro, J., Montávez, J. P., and García-Bustamante, E.: A Revised Scheme for the WRF Surface Layer Formulation, Mon. Weather Rev., 140, 898–918, https://doi.org/10.1175/MWR-D-11-00056.1, 2012.
Kader, B. A. and Yaglom, A. M.: Mean Fields and Fluctuation Moments in Unstably Stratified Turbulent Boundary Layers, J. Fluid Mech., 212, 637–662, https://doi.org/10.1017/S0022112090002129, 1990.
Kain, J.: The Kain–Fritsch Convective Parameterization: An Update, J. Appl. Meteorol. Climatol., 43, 170–181, https://doi.org/10.1175/15200450(2004)043<0170:TKCPAU>2.0.CO;2, 2004.
Lin, Y., Farley, R., and Orville, H.: Bulk Parameterization of the Snow Field in a Cloud Model, J. Appl. Meteorol. Climatol., 22, 1065–1092, https://doi.org/10.1175/1520-0450(1983)022<1065:BPOTSF>2.0.CO;2, 1983.
Mlawer, E. J., Taubman, S. J., Brown, P. D., Iacono, M. J., and Clough, S. A.: Radiative transfer for inhomogeneous atmospheres: RRTM, a validated correlated-k model for the longwave, J. Geophys. Res., 102, 16663–16682, https://doi.org/10.1029/97JD00237, 1997.
Monin, A. S. and Obukhov, A. M.: Basic Laws of Turbulent Mixing in the Surface Layer of the Atmosphere, Tr. Akad. Nauk SSSR Geophiz. Inst., 24, 163–187, 1954.
Muñoz Sabater, J.: ERA5-Land hourly data from 1950 to present, Copernicus Climate Change Service (C3S) Climate Data Store (CDS) [data set], https://doi.org/10.24381/cds.e2161bac, 2019.
Namdev, P., Sharan, M., and Mishra, S. K.: Impact of the similarity functions of surface layer parametrization in a climate model over the Indian region, Q. J. Roy. Meteor. Soc., 149, 152–170, https://doi.org/10.1002/qj.4400, 2023a.
Namdev, P., Sharan, M., Srivastava, P., and Mishra, S. K.: Dataset for the article titled “An Updated Parameterization of the Unstable Atmospheric Surface Layer in WRF Modeling System”, Zenodo [data set], https://doi.org/10.5281/zenodo.10435513, 2023b.
Namdev, P., Srivastava, P., Sharan, M., and Mishra, S. K.: An Update to WRF Surface Layer Parameterization over an Indian Region, Dynam. Atmos. Ocean, 105, 101414, https://doi.org/10.1016/j.dynatmoce.2023.101414, 2024.
Niu, G. Y., Yang, Z. L., Mitchell, K. E., Chen, F., Ek, M. B., Barlage, M., Kumar, A., Manning, K., Niyogi, D., Rosero, E., Tewari, M., and Xia, Y.: The community Noah land surface model with multiparameterization options (Noah-MP): 1. Model description and evaluation with local-scale measurements, J. Geophys. Res.-Atmos., 116, D12109, https://doi.org/10.1029/2010JD015139, 2011.
Panofsky, H. and Dutton, J.: Atmospheric Turbulence, John Wiley & Sons, New York, 397 pp., 1984.
Rao, K. G. and Narasimha, R.: Heat-Flux Scaling for Weakly Forced Turbulent Convection in the Atmosphere, J. Fluid Mech., 547, 115–135, https://doi.org/10.1017/S0022112005007251, 2006.
Rao, K. G., Narasimha, R., and Prabhu, A.: Estimation of Drag Coefficient at Low Wind Speeds over the Monsoon Trough Land Region during MONTBLEX-90, Geophys. Res. Lett., 23, 2617–2620, https://doi.org/10.1029/96GL02368, 1996.
Schluenzen, K. H. and Sokhi, R. S.: Overview of tools and methods for meteorological and air pollution mesoscale model evaluation and user training, Joint report by WMO and COST 728, WMO/TD-No. 1457, Geneva, Switzerland, November 2008.
Schumann, U.: Minimum friction velocity and heat transfer in the rough surface layer of a convective boundary layer, Bound.-Lay. Meteorol., 44, 311–326, https://doi.org/10.1007/BF00123019, 1988.
Skamarock, W. C., Klemp, J. B., Dudhia, J., Gill, D. O., Liu, Z., Berner, J., Wang, W., Powers, J. G., Duda, M. G., Barker, D. M., and Huang, X.-Y.: A Description of the Advanced Research WRF Version 4, NCAR Tech. Note NCAR/TN-556+STR, 145 pp., https://github.com/wrf- model/WRF/releases/tag/v4.2.2 (last access: 12 November 2024), 2019.
Srivastava, P. and Sharan, M.: Characteristics of the Drag Coefficient over a Tropical Environment in Convective Conditions, J. Atmos. Sci., 72, 4903–4913, https://doi.org/10.1175/JAS-D-14-0383.1, 2015.
Srivastava, P. and Sharan, M.: Analysis of Dual Nature of Heat Flux Predicted by Monin-Obukhov Similarity Theory: An Impact of Empirical Forms of Stability Correction Functions, J. Geophys. Res.-Atmos., 124, 3627–3646, https://doi.org/10.1029/2018JD029740, 2019.
Srivastava, P. and Sharan, M.: Uncertainty in the Parameterization of Surface Fluxes under Unstable Conditions, J. Atmos. Sci., 78, 2237–2247, https://doi.org/10.1175/JAS-D-20-0350.1, 2021.
Srivastava, P., Sharan, M., Kumar, M., and Dhuria, A. K.: On Stability Correction Functions over the Indian Region under Stable Conditions, Meteorol. Appl., 27, e1880, https://doi.org/10.1002/met.1880, 2020.
Srivastava, P., Sharan, M., and Kumar, M.: A Note on Surface Layer Parameterizations in the Weather Research and Forecast Model, Dynam. Atmos. Ocean, 96, 101259, https://doi.org/10.1016/j.dynatmoce.2021.101259, 2021.
Stull, R. B.: An Introduction to Boundary Layer Meteorology, Kluwer Academic Publishers, Dordrecht, the Netherlands, 13, 670 pp., https://doi.org/10.1007/978-94-009-3027-8, 1988.
Taylor, K. E.: Summarizing multiple aspects of model performance in a single diagram, J. Geophys. Res., 106, 7183–7192, https://doi.org/10.1029/2000JD900719, 2001.
Venkatram, A.: Applying a framework for evaluating the performance of air quality models, in: Proceedings of the sixth International Conference on Harmonisation within Atmospheric Dispersion modeling for Regulatory Applications, 11–14 October 1999, Rouen, France, 11–14, 1999.
Webb, E. K.: Profile Relationships: The Log-linear Range, and Extension to Strong Stability, Q. J. Roy. Meteor. Soc., 96, 67–90, https://doi.org/10.1002/qj.49709640708, 1970.
Wilson, D. K.: An Alternative Function for the Wind and Temperature Gradients in Unstable Surface Layers, Bound.-Lay. Meteorol., 99, 151–158, https://doi.org/10.1023/A:1018718707419, 2001.
Zeng, X., Zhao, M., and Dickinson, R. E.: Intercomparison of Bulk Aerodynamic Algorithms for the Computation of Sea Surface Fluxes Using TOGA COARE and TAO Data, J. Climate, 11, 2628–2644, https://doi.org/10.1175/1520-0442(1998)011<2628:IOBAAF>2.0.CO;2, 1998.