the Creative Commons Attribution 4.0 License.
the Creative Commons Attribution 4.0 License.
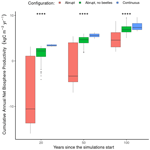
Simulating Ips typographus L. outbreak dynamics and their influence on carbon balance estimates with ORCHIDEE r8627
Guillaume Marie
Hervé Jactel
Gunnar Petter
Maxime Cailleret
Matthew J. McGrath
Vladislav Bastrikov
Josefine Ghattas
Bertrand Guenet
Anne Sofie Lansø
Kim Naudts
Aude Valade
Chao Yue
New (a)biotic conditions resulting from climate change are expected to change disturbance dynamics, such as windthrow, forest fires, droughts, and insect outbreaks, and their interactions. These unprecedented natural disturbance dynamics might alter the capability of forest ecosystems to buffer atmospheric CO2 increases, potentially leading forests to transform from sinks into sources of CO2. This study aims to enhance the ORCHIDEE land surface model to study the impacts of climate change on the dynamics of the bark beetle, Ips typographus, and subsequent effects on forest functioning. The Ips typographus outbreak model is inspired by previous work from Temperli et al. (2013) for the LandClim landscape model. The new implementation of this model in ORCHIDEE r8627 accounts for key differences between ORCHIDEE and LandClim: (1) the coarser spatial resolution of ORCHIDEE; (2) the higher temporal resolution of ORCHIDEE; and (3) the pre-existing process representation of windthrow, drought, and forest structure in ORCHIDEE. Simulation experiments demonstrated the capability of ORCHIDEE to simulate a variety of post-disturbance forest dynamics observed in empirical studies. Through an array of simulation experiments across various climatic conditions and windthrow intensities, the model was tested for its sensitivity to climate, initial disturbance, and selected parameter values. The results of these tests indicated that with a single set of parameters, ORCHIDEE outputs spanned the range of observed dynamics. Additional tests highlighted the substantial impact of incorporating Ips typographus outbreaks on carbon dynamics. Notably, the study revealed that modeling abrupt mortality events as opposed to a continuous mortality framework provides new insights into the short-term carbon sequestration potential of forests under disturbance regimes by showing that the continuous mortality framework tends to overestimate the carbon sink capacity of forests in the 20- to 50-year range in ecosystems under high disturbance pressure compared to scenarios with abrupt mortality events. This model enhancement underscores the critical need to include disturbance dynamics in land surface models to refine predictions of forest carbon dynamics in a changing climate.
- Article
(1773 KB) - Full-text XML
-
Supplement
(1297 KB) - BibTeX
- EndNote
Future climate will likely bring new abiotic constraints through the co-occurrence of multiple connected hazards, e.g., “hotter droughts”, which are droughts combined with not only heat waves (Allen et al., 2015; Zscheischler et al., 2018), but also new biotic conditions from interacting natural and anthropogenic disturbances, such as insect outbreaks following windthrow or forest fires (Seidl et al., 2017). Unprecedented natural disturbance dynamics might alter biogeochemical cycles – specifically the capability of forest ecosystems to buffer the CO2 increase in the atmosphere (Hicke et al., 2012; Seidl et al., 2014) and the risk that forests are transformed from sinks into sources of CO2 (Kurz et al., 2008a). The magnitude of such alteration, however, remains uncertain principally due to the lack of impact studies that include disturbance regime shifts on a global scale (Seidl et al., 2011).
Land surface models are used to study the relationships between climate change and the biogeochemical cycles of carbon, water, and nitrogen (Ciais et al., 2005; Cox et al., 2000; Friedlingstein et al., 2006; Luyssaert et al., 2018; Zaehle and Dalmonech, 2011). Many of these models use background mortality to obtain an equilibrium in their biomass pools. This classic approach towards forest dynamics, which assumes steady-state conditions over long periods of time, may not be suitable for assessing the impacts of disturbances on shorter timescales under a fast-changing climate. This could be considered a shortcoming in land surface models because disturbances can have significant impacts on ecosystem services, such as water regulation, carbon sequestration, and biodiversity (Quillet et al., 2010). Mechanistic approaches that account for a variety of mortality causes, such as age, size, competition, climate, and disturbances, are now being considered and tested to simulate forest dynamics more accurately (Migliavacca et al., 2021). For example, the land surface model ORCHIDEE accounts for mortality from interspecific competition for light in addition to background mortality (Naudts et al., 2015). Implementing a more mechanistic view on mortality is thought to be essential for improving our understanding of the impacts of climate change on forest dynamics and the provision of ecosystem services.
Land surface models also face the challenge of better describing mortality, particularly when it comes to ecosystem responses to “cascading disturbances”, where legacy effects from one disturbance affect the next (Buma, 2015; Zscheischler et al., 2018). Biotic disturbances, such as bark beetle outbreaks, strongly depend on previous disturbances as their infestation capabilities are higher when tree vitality is low, for example, following drought or storm events (Seidl et al., 2018). This illustrates how interactions between biotic and abiotic disturbances can have substantial effects on ecosystem dynamics and must be accounted for in land surface models to improve our understanding of the impacts of climate change on forest dynamics (Seidl et al., 2011; Temperli et al., 2013). While progress has been made towards including abrupt mortality from individual disturbance types such as wildfire (Lasslop et al., 2014; Migliavacca et al., 2013; Yue et al., 2014), windthrow (Chen et al., 2018), and drought (Yao et al., 2022), the interaction of biotic and abiotic disturbances remains both a knowledge and modeling gap (Kautz et al., 2018).
Bark beetle infestations are increasingly recognized as disturbance events of regional to global importance (Bentz et al., 2010; Kurz et al., 2008a; Seidl et al., 2018). Notably, a bark beetle outbreak ravaged over 90 % of Engelmann spruce trees across approximately 325 000 ha in the Canadian and American Rocky Mountains between 2005 and 2017 (Andrus et al., 2020). In Europe, the bark beetle Ips typographus has been involved in up to 8 % of total tree mortality due to natural disturbances from 1850 to 2000 (Hlásny et al., 2021a). A recent increase in beetle activity, particularly following mild winters (Andrus et al., 2020; Kurz et al., 2008b), windthrow (Mezei et al., 2017), and droughts (Nardi et al., 2023) has been well documented (Hlásny et al., 2021a; Pasztor et al., 2014), underscoring the need to integrate bark beetle dynamics into land surface modeling.
Past studies used a variety of approaches to model the impacts of bark beetles on forests. While some models treated bark beetle outbreaks as background mortality (Luyssaert et al., 2018; Naudts et al., 2016), others dynamically modeled these outbreaks within ecosystems (Jönsson et al., 2012; Seidl and Rammer, 2016; Temperli et al., 2013). Studies with prescribed beetle outbreaks tend to focus on the direct effects of the outbreak on forest conditions and carbon fluxes but are likely to overlook more complex feedback processes, such as interactions with other disturbances and longer-term impacts. Conversely, dynamic modeling of beetle outbreaks, provides a more comprehensive view by incorporating the life cycle of bark beetles and tree defense mechanisms and ensuing alterations in forest composition and functionality.
Simulation experiments for Ips typographus outbreaks using the LPJ-GUESS vegetation model highlighted regional variations in outbreak frequencies, pinpointing climate change as a key exacerbating factor (Jönsson et al., 2012). Simulation experiments with the iLand landscape model suggested that almost 65 % of Ips typographus outbreaks are aggravated by other environmental drivers (Seidl and Rammer, 2016). A 4 °C temperature increase could result in a 265 % increase in disturbed area and a 1800 % growth in the average patch size of the disturbance (Seidl and Rammer, 2016). Disturbance interactions were 10 times more sensitive to temperature changes, boosting the disturbance regime's climate sensitivity. The results of these studies justify the inclusion of interacting disturbances in land surface models, such as ORCHIDEE, which are used in future climate predictions and impact studies (Boucher et al., 2020).
The objectives of this study are to (1) develop and implement a spatially implicit outbreak model for Ips typographus in the land surface model ORCHIDEE inspired by the work from Temperli et al. (2013) and (2) use simulation experiments to characterize the behavior of this newly added model functionality.
2.1 The land surface model ORCHIDEE
ORCHIDEE is the land surface model of the IPSL (Institut Pierre-Simon Laplace) Earth system model (Boucher et al., 2020; Krinner et al., 2005). ORCHIDEE can, however, also be run uncoupled as a standalone land surface model forced by temperature, humidity, pressure, precipitation, and wind fields. Unlike the coupled setup, which needs to run on the global scale, the standalone configuration can cover any area ranging from a single grid point to the global domain. In this study, ORCHIDEE was run as a standalone land surface model.
ORCHIDEE does not enforce any particular spatial resolution. The spatial resolution is an implicit user setting that is determined by the resolution of the climate forcing (or the resolution of the atmospheric model in a coupled configuration). ORCHIDEE can run on any temporal resolution. This apparent flexibility is somewhat restricted as processes are formalized at given time steps: half-hourly (e.g., photosynthesis and energy budget), daily (i.e., net primary production), and annual (i.e., vegetation demographic processes). With the current model architecture, meaningful simulations should have a temporal resolution of 1 min to 1 h for the calculation of energy balance, water balance, and photosynthesis.
ORCHIDEE utilizes metaclasses to discretize the global diversity in vegetation. The model includes 13 metaclasses by default, including one class for bare soil, eight classes for various combinations of leaf-type and climate zones of forests, two classes for grasslands, and two classes for croplands. Each metaclass can be further subdivided into an unlimited number of plant functional types (PFTs). The current default setting of ORCHIDEE distinguishes 15 PFTs where the metaclass of C3 grasslands have been separated into boreal, temperate, and tropical C3 grassland PFTs.
At the beginning of a simulation, each forest PFT in ORCHIDEE contains a monospecific forest stand that is structured by a user-defined but fixed number of diameter classes (three by default). Throughout the simulation, the boundaries of the diameter classes are adjusted to accommodate changes in the stand structure, while the number of classes remains constant. Flexible class boundaries provide a computationally efficient approach to simulate different forest structures. For instance, an even-aged forest is simulated using a small diameter range between the smallest and largest trees, resulting in all trees belonging to the same stratum. Conversely, an uneven-aged forest is simulated by applying a wide range between diameter classes so that different classes represent different canopy strata.
The model uses allometric relationships to link tree height and crown diameter to stem diameter. Individual tree canopies are not explicitly represented; instead, a canopy structure model based on simple geometric forms (Haverd et al., 2012) has been included in ORCHIDEE (Naudts et al., 2015). Diameter classes represent trees with different mean diameter and height, which informs the user about the social position of trees within the canopy. Intra-stand competition is based on the basal area of individual trees, which accounts for the fact that trees with a higher basal area occupy dominant positions in the canopy and are therefore more likely to intercept light and thus contribute more to stand-level photosynthesis and biomass growth compared to suppressed trees (Deleuze et al., 2004). If recruitment occurs, diameter classes evolve into cohorts. However, in the absence of recruitment, all diameter classes contain trees of the same age.
Individual tree mortality from self-thinning, wind storms, and forest management is explicitly simulated. Other sources of mortality are implicitly accounted for through a so-called constant background mortality rate. Furthermore, age classes (four by default) can be used after land cover change, forest management, and disturbance events to explicitly simulate the regrowth of the forest. Following a land cover change, biomass and soil carbon pools (but not soil water columns) are either merged or split to represent the various outcomes of a land cover change. The ability of ORCHIDEE to simulate dynamic canopy structures (Chen et al., 2016; Naudts et al., 2015; Ryder et al., 2016), a feature essential to simulate both the biogeochemical and the biophysical effects of natural and anthropogenic disturbances, is exploited in other parts of the model, i.e., precipitation interception, transpiration, energy budget calculations, radiation scheme, and calculation of the absorbed light for photosynthesis.
Since revision 7791, mortality from Ips typographus outbreaks is explicitly accounted for and thus conceptually excluded from the so-called environmental background mortality. Subsequently, changes in canopy structure resulting from growth, forest management, land cover changes, wind storms, and Ips typographus outbreaks are accounted for in the calculations of the carbon, water, and energy exchanges between the land surface and the atmosphere. For details on the functionality of the ORCHIDEE model that is not of direct relevance for this study, e.g., the energy budget calculations, soil hydrology, snow phenology, albedo, roughness, photosynthesis, respiration, phenology, carbon and nitrogen allocation, land cover changes, product use, and nitrogen cycle, readers are referred to Krinner et al. (2005), Zaehle and Friend (2010), Naudts et al. (2015), Vuichard et al. (2019).
2.2 Origin of the bark beetle (Ips typographus) model – the LandClim legacy
Although mortality from windthrow (Chen et al., 2018) and forest management (Luyssaert et al., 2018; Naudts et al., 2016) was already accounted for in ORCHIDEE prior to r8627, insect outbreaks and their interaction with other disturbances were not. The LandClim model (Schumacher, 2004) and, more specifically, the Ips typographus model developed by Temperli et al. (2013) have been used as a basis to develop the Ips typographus model in ORCHIDEE r8627.
LandClim is a spatially explicit stochastic landscape model in which forest dynamics are simulated at a yearly time step for 10–100 km2 landscapes consisting of 25 m × 25 m patches. Within a patch recruitment, growth, mortality, and competition among age cohorts of different tree species are simulated with a gap model (Bugmann, 1996) in response to monthly mean temperature, climatic drought, and light availability. LandClim, for which a detailed description can be found in Schumacher (2004), Temperli et al. (2013), includes the functionality to simulate the decadal dynamics and consequences of Ips typographus outbreaks at the landscape scale (Temperli et al., 2013). In the LandClim approach, the extent, occurrence and severity of beetle-induced tree mortality are driven by the landscape susceptibility, beetle pressure, and infested tree biomass. While the LandClim beetle model was designed and structured to be generally applicable for Northern Hemisphere climate-sensitive bark beetle–host systems, it was parameterized to represent disturbances by the Ips typographus in Norway spruce (Picea abies Karst.; Temperli et al., 2013).
As LandClim and ORCHIDEE are developed for different purposes, their temporal and spatial scales differ. These differences in model resolution justify developing a new model while following the principles embedded in the LandClim approach. LandClim assesses bark beetle damage at 25 m × 25 m patches, and, to do so, it uses information from other nearby patches as well as landscape characteristics, such as slope, aspect, and altitude. The susceptibility of a landscape to bark beetle infestations is calculated using multiple factors, such as drought-induced tree resistance, age of the oldest spruce cohort, proportion of spruce in the patch's basal area, and spruce biomass damaged by windthrow. These drivers are presented as sigmoidal relationships ranging from 0 to 1 (denoting none to maximum susceptibility, respectively) that are combined in a susceptibility index for each Norway spruce cohort in a patch. Bark beetle pressure is quantified as the potential number of beetles that can infest a patch, and its calculation considers, among others, previous beetle activity, maximum possible spruce biomass that beetles could kill, and temperature-dependent bark beetle phenology. Finally, the susceptibility index and beetle pressure are used to estimate the total infested tree biomass and total biomass killed by bark beetles for each cohort within a patch.
In ORCHIDEE, however, the simulation unit is about 6 orders of magnitude larger, i.e., 25 km × 25 km. Hence, a single grid cell in ORCHIDEE exceeds the size of an entire landscape in LandClim. Where landscape characteristics in LandClim can be represented by statistical distributions, the same characteristics in ORCHIDEE are represented by single values. These differences between LandClim and ORCHIDEE imply that the original bark beetle model cannot be implemented in ORCHIDEE without substantial adjustments; the model at the ORCHIDEE grid cell should work without requiring spatial information and statistical distributions of landscape characteristics because those are not available in ORCHIDEE.
2.3 Bark beetle outbreak development stages
Bark beetle outbreak development stages (Fig. 1) are useful to understand the dynamics of an outbreak (Edburg et al., 2012; Hlásny et al., 2021a; Wermelinger, 2004). Nonetheless, the outbreak model in ORCHIDEE r8627 simulates the dynamic of the Ips typographus outbreak as a continuous process. Hence, endemic, epidemic, buildup and post-epidemic stages are not explicitly simulated. In this study, outbreak development stages were only introduced to structure the model description. If needed, these stages could be distinguished while post-processing the simulation results if (arbitrary) thresholds are set for specific variables such as DRbeetles.
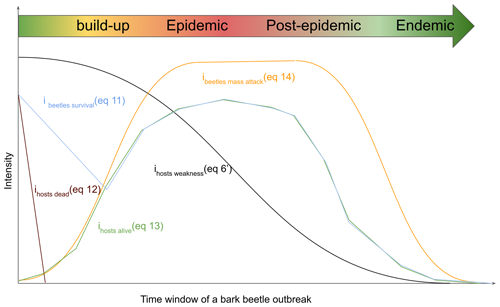
Figure 1Dynamic interplay of the different host and beetle characteristics during a bark beetle (Ips typographus) outbreak. The time window spans four outbreak development stages: buildup, epidemic, post-epidemic, and endemic. The curves represent key characteristics, showing the growth in beetle population and subsequent decline in host population. Ihosts dead characterizes the presence of defenseless uprooted or cut spruce trees, ihosts alive,characterizes living spruce trees that could become hosts for the bark beetles, ihosts susceptibility susceptibility of spruce trees to bark beetle attacks, ibeetles mass attack quantifies the capability of the bark beetles to mass attack, and ibeetles survival characterizes the survival of bark beetles. Host and bark beetle characteristics are detailed in the subsequent text. When the density of the host trees is declining due to an increased host mortality from the bark beetle outbreak itself, the competition between trees for light and nutrients declines as well. As a consequence, the host susceptibility decreases, which in ORCHIDEE is the main pathway for an outbreak to move back to the endemic phase. After 1 year, the wood from a storm is not fresh enough for bark beetles to breed in, so ihost dead goes to zero. In ORCHIDEE, the bark beetle population needs to be capable of mass attacking living trees within a year to make the transition from the buildup to the epidemic phase.
2.4 Bark beetle (Ips typographus) damage in ORCHIDEE
The biomass of trees killed by bark beetles in 1 year and one grid cell (Bbeetles kill) is calculated as the product of the biomass of trees attacked by bark beetles (Bbeetles attacked) by the probability of a successful attack (Psuccess, a) averaged over the number of spruce age classes and weighted by their actual fraction (). The approach assumes that a successful beetle colonization always results in the death of the attacked tree, which is a simplification of reality (Schlyter et al., 1989).
During the endemic stage, Bbeetles attacked and Bbeetles kill are at their lowest values, and the damage from bark beetles has little impact on the structure and function of the forest. Losses from Bbeetles kill can be considered contributors to the background mortality.
The biomass of trees attacked by bark beetles (Bbeetles attacked) is the outcome of bark beetles that successfully overcame the tree defenses and succeeded in boring holes in the bark in order to reach the sapwood. Bbeetles attacked is calculated at the grid cell by multiplying the actual stand biomass of spruce (Btotal) and the probability that bark beetles attack spruce trees in the grid cell (Pattacked).
Pattacked represents the ability of the bark beetles to spread and to locate new suitable spruce trees as hosts for breeding. Pattacked is calculated by the product of two indexes (all indexes in this study are denoted i and are analogue to the susceptibility indexes from Temperli et al., 2013): (1) the beetle pressure index (ibeetles pressure), which is a proxy for the bark beetle population, and (2) the stand attractiveness index (ihosts attractiveness) is related to its health and reflects the ability of the forest to resist an external stressor such as bark beetle attacks.
2.5 Host attractiveness
The stand attractiveness index (ihosts attractiveness) represents how interesting a stand is for a new bark beetle colony. When ihosts attractiveness tends to 0, the stand is constituted mainly by healthy trees, which are less attractive for beetles, whereas an ihosts attractiveness value approaching 1 represents a highly stressed spruce stand suitable for colonization by bark beetles. Factors that contribute to the stress of a forest are nitrogen limitation, limited carbohydrate reserves, and monospecific spruce forest. Trees experiencing extended periods of environmental stress are expected to have lower carbon and nitrogen reserves available for defense compounds, making them vulnerable to bark beetle attacks even at relatively low beetle population densities (Raffa et al., 2008). Nonetheless, reserve pools in ORCHIDEE r8627 have not yet been evaluated, so, instead, proxies were used, such as long-term drought (PWSmax) and relative density index (ird, spruce), which were already simulated in ORCHIDEE r8627.
where ihosts competition and ihosts defense both represent proxies for the reduction in the nitrogen and carbohydrate reserve due to strong competition for light and soil resources and consecutive years that are drier than average. For this study, the maximum drought intensity during the last 3 years (PWSmax) is considered as a proxy for spruce stand healthiness:
where PWSy, spruce is the average daily plant water stress index over the growing season for the spruce stand and is equal to 0 when plants are highly stressed and equal to 1 when plants are not stressed. PWSlimit is the plant water stress below which the healthiness of the stand will strongly be affected. The number of age class (a) within the stand is equal to 3 in this study. In addition to drought, an overstocked forest may also decrease the overall healthiness of a spruce stand (ihosts competition).
In ORCHIDEE, the relative density index (ird) is used to quantify the competition between trees at the stand level. At an ird of 1, the forest is expected to be at its maximum density given the carrying capacity of the site, implying the highest level of competition between trees. ird limit represents the limit at which the bark beetle outbreak starts to decline because of a lack of suitable host trees. The severity of bark-beetle-caused tree mortality decreases when we increase the spatial resolution from the stand to the landscape scale. At the landscape scale, which can cover areas up to 2500 km2, the duration of mortality may be longer and the severity lower because beetles disperse across the landscape and cause mortality at different times. This distinction is important for interpreting model results, particularly when considering parameters like ird limit in the ORCHIDEE model. The parameter ird limit describes the proportion of trees surviving after an outbreak and should therefore be adjusted for the spatial scale of a grid cell in ORCHIDEE. In a model setup, where a grid cell represents a single stand (∼1 ha), ird limit should be close to 0, indicating that nearly all trees may be killed. However, in a simulation with grid cells representing 2500 km2, not all trees will be killed, which is reflected in setting ird limit to 0.4.
The value of ird spruce is computed as follows:
where Dspruce, a is the current tree density of an age class and Fspruce, a is the fraction of spruce in the grid cell that resides in this age class. Dmax represents the maximum stand density of a stand given its diameter. In ORCHIDEE, Dmax is calculated based on the quadratic mean diameter (cm) and two species specific parameters, α and β:
The index ihosts share (used in Eq. 4) takes into account that in a mixed tree species landscape, even a few non-host trees may chemically hinder bark beetles in finding their host trees (Zhang and Schlyter, 2004), explaining why insect pests, including Ips typographus outbreaks, often cause more damage in pure compared to mixed stands (Nardi et al., 2023). ORCHIDEE r8627 does not simulate multi-species stands but does account for landscape-level heterogeneity of forests with different plant functional types. The bark beetle model in ORCHIDEE assumes that within a grid cell, the fraction of spruce over other tree species is a proxy for the degree of mixture:
where
2.6 Implicit representation of bark beetle populations
The bark beetle pressure index (ibeetles pressure) is now formulated based on two components: (1) the bark beetle breeding index of the current year (ibeetles generation) and (2) an index of the loss of tree biomass in the previous year due to a bark beetle infestation (ibeetles activity). The index ibeetles activity is thus a proxy for the previous year's bark beetle activity. The expression accounts for the legacy effect of bark beetle activities by averaging activities over the current and previous years. In this approach, the susceptibility index (ibeetles survival) serves as an indicator for increased bark beetle survival, which could result from favorable conditions for beetle demography (see the next section).
The model calculates ibeetles generation from a logistic function, which depends on the number of generations a bark beetle population can sustain within a single year:
where Sgeneration and Glimit are tuning parameters for the logistic function, DDeff represents the sum of effective temperature for bark beetle reproduction in °C d−1, and DDref denotes the thermal sum of degree-days for one bark beetle generation in °C d−1. Saturation of ibeetles generation represents the lack of an available breeding substrate when many generations develop over a short period.
DDeff is calculated from 1 January until the diapause of the first generation. In ORCHIDEE, a diapause is triggered when the day length exceeds 14.5 h (e.g., 27 April for France). Each day before the diapause with a daily average temperature around the bark above 8.3 °C (Tmin) and below 38.4 °C (Tmax) is accounted for in the summation of DDeff (Eq. 10). This approach simulates the phenology of bark beetles, which tend to breed earlier when winter and spring are warmer, thus allowing for multiple generations in the same year (Hlásny et al., 2021a).
where i is a day and ndiapause is the number of days between the 1 January and the day of the diapause. Topt (30.3 °C) is the optimal bark temperature for beetle development, and Tmin (8.3 °C) is the temperature below which the beetles development stops. Tbark, i is the average daily bark temperature. Tbark, i is calculated as the daily average air temperature with 2 °C subtracted. All parameter values are taken from Temperli et al. (2013).
The bark beetle activity of the previous year (ibeetles activity) is calculated as follows:
where ibeetles activity denotes the biomass of the stand damaged by bark beetles in the previous year, Btotal is the total biomass of the stand, and Sactivity and actlimit are parameters that drive the intensity of this negative feedback.
During the buildup stage, the population of bark beetles can either return to its endemic stage if tree defense mechanisms are preventing bark beetles from successfully attacking healthy trees or evolve into an epidemic stage (Fig. 1) if the tree defense mechanisms fail. During the post-epidemic stage, the forest is still subject to higher mortality than usual, but signs of recovery appear (Hlásny et al., 2021a). Recovery may help the forest ecosystem to return to its original state or switch to a new state (different species, change in the forest structure) depending on the intensity and the frequency of the disturbance (Van Meerbeek et al., 2021).
2.7 Bark beetle survival
The capability of the bark beetles to survive the winter in between two breeding seasons is critical in simulating epidemic outbreaks. During regular winters, winter mortality for bark beetles is around 40 % for the adults and 100 % for the juveniles (Jönsson et al., 2012). In our scheme, this mortality rate is implicitly accounted for in the calculation of the bark beetle survival index (ibeetles survival). A lack of data linking bark beetle survival to anomalous winter temperatures justifies the implicit approach and prevented including this information as a modulator of ibeetles survival. The latter explains why winter temperatures do not appear in Eq. (11). Instead, the model simulates the survival as a function of the abundance of suitable tree hosts, which decreases the competition for shelter and food:
The availability of wood necromass from trees that died recently, particularly following windstorms, plays a critical role in bark beetle survival and proliferation. In the year following a windstorm, uprooted and broken trees may offer an ideal breeding substrate for bark beetles, facilitating their population growth.
In Temperli et al. (2013), an empirical correlation between windthrow events and bark beetle susceptibility was established. ORCHIDEE enhances realism by considering the actual suitable hosts (living or recently dead trees) as the primary driver of bark beetle survival. To avoid overestimating bark beetle population growth, max has been introduced. Any addition of dead trees beyond max is considered ineffective in affecting the bark beetle population. This ensures that an excess of breeding substrate does not artificially inflate beetle numbers.
This relationship is quantitatively represented in ORCHIDEE through the dead host index, ihosts dead, which is driven by the availability of recent dead trees. The formulation of ihosts dead is as follows:
Here, Nwood represents the quantity of woody necromass from the current year, Bwood is the total living woody biomass in the stand, and max is the threshold of the ratio signifying the maximum level. This index captures the immediate increase in dead trees suitable for bark beetle breeding following a windthrow event. However, it takes about a year for dead wood to lose its freshness and suitability for bark beetle breeding. This is accounted for by excluding woody necromass that is older than 1 year from the ihosts dead calculation.
max can also be considered a parameter that depends on the spatial scale of the simulation. The mortality rate of trees (DRwindthrow) that will trigger an outbreak is very different across spatial scales. Where a relatively high share of dead wood is needed to trigger an outbreak on the patch scale, a much lower share of dead wood suffices on the landscape scale to trigger a widespread bark beetle outbreak. Therefore, these parameters must be set according to the spatial resolution of the simulation experiment.
The index ihosts alive denotes the survival of bark beetles, which is facilitated by the abundance of suitable trees that reduces the competition among bark beetles for breeding substrates and therefore increases their survival.
The number of suitable tree hosts, ihosts alive, is driven by two factors: (1) the abundance of weak trees, which can be more easily infected by bark beetles. ORCHIDEE does not explicitly represent weak trees, but tree health is thought to decrease with an increasing density given the stand diameter. The index for host suitability is thus calculated by making use of the relative density index (ird spruce).
Equation (6a′) is close to Eq. (6a), but the parameter Ssusceptibility has been reduced by a factor of 2 in order to reflect that ihosts susceptibility is more sensitive to ird spruce than ihosts competition. The second factor is (2) ihosts mass attack, which represents the ability of bark beetles to attack healthy trees when the number of bark beetles is large enough. This index only depends on the size of the bark beetle population (ibeetles pressure; see Eq. 8).
where Shosts mass attack and BPlimit are parameters. Smass attack controls the steepness of the relationship, while BPlimit is the bark beetle pressure index at which the population is moving from endemic to epidemic stage where mass attacks are possible.
The epidemic stage corresponds to the capability of bark beetles to mass attack healthy trees and overrule tree defenses (Biedermann et al., 2019). At this point in the outbreak, all trees are potential targets irrespective of their health. Three causes have been suggested to explain the end of the epidemic phase: (1) the most likely cause is a high interspecific competition among beetles for the tree host when the density is decreasing (decreasing ihosts alive) (Komonen et al., 2011; Pineau et al., 2017), (2) a series of very cold years will decrease their ability to reproduce (decreasing ibeetles generation), and (3) a rarely demonstrated increasing population of beetle predators (Berryman, 2002). In ORCHIDEE r8627, the first two causes are represented, but the final one, i.e., the predators, are not.
2.8 Tree mortality from bark beetle infestation
When bark beetles attack a tree, the success of their attack will likely depend on the capability of the tree to defend itself from the attack. Trees defend themselves against beetle attacks by producing secondary metabolites (Huang et al., 2020). The high carbon and nitrogen costs of these compounds limit their production to periods with environmental conditions favorable for growth (Lieutier, 2002). The probability of a successful bark beetle attack is driven by the size of the bark beetle population (ibeetles pressure) and the health of each tree. ORCHIDEE, however, is not simulating individual trees but rather diameter classes within an age class. An index of tree health for each age class (ihosts health, a) was calculated as follows:
A tree rarely dies solely from bark beetle damage (except during mass attacks) as female beetles often carry blue stain fungi, which colonize the phloem and sapwood, blocking the water-conducting vessels of the tree (Ballard et al., 1982). This results in tree death from carbon starvation or desiccation. As ORCHIDEE r8627 does not simulate the effects of changes in sapwood conductivity on photosynthesis and the resultant probability of tree mortality, the index of weakened trees (ihosts health, a) makes use of two proxies similarly to Eqs. (5) and (6) but simplified to be calculated only for one age class at a time:
Contrary to Eq. (5a), PWS is the plant water stress from the current year.
To assess the bark beetle damage rate (DRbeetles), Bbeetles kill has to be divided by Btotal.
2.9 Flow of the calculations
The equations presented above contain feedback loops which are visualized in Fig. 2. In ORCHIDEE, these feedback loops are accounted for in subsequent time steps rather than the same time step.
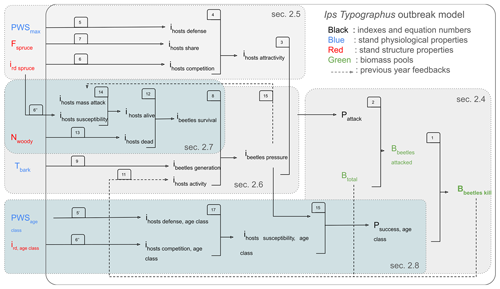
Figure 2Order of the calculations and feedback in the Ips typographus outbreak model of ORCHIDEE. The numbers correspond to the equation numbers in this study. The dotted line boxes represent the five main concepts of the outbreak model described in Sect. 2.4, 2.5, 2.6, 2.7, and 2.8. The variable name definitions are listed in Table 1.
3.1 Model configuration
Given the large-scale nature of the ORCHIDEE, a sensitivity experiment of the bark beetle outbreak functionality was carried out rather than focusing the model evaluation on matching observed damage volumes at specific case studies. Focusing on model sensitivity for a range of environmental conditions is thought to reduce the risk of overfitting the model to specific site conditions (Abramowitz et al., 2008).
ORCHIDEE r8627, including the bark beetle model, was run at the location of eight FLUXNET sites selected to simulate a credible temperature and precipitation gradient for spruce (see below). For each location, the half-hourly meteorological data from the flux tower were gap-filled and reformatted so that they could be used as climate forcing by ORCHIDEE. Boundary conditions for ORCHIDEE, such as soil texture, pH, and soil color were retrieved from the US Department of Agriculture map for the corresponding grid cell. The observed land cover and land use for the grid cell were ignored and set to pure spruce because this study did not investigate the effect of species mixture in the simulation experiments. The resolution of the grid cell chosen for this analysis is 2500 km2. Although this corresponds to a high resolution for large-scale simulations with ORCHIDEE, it is a coarse resolution for studying bark beetle outbreaks.
The climate forcings were looped over as much as needed to bring the carbon, nitrogen, and water pools to equilibrium during a 340-year-long spin-up. Following the spin-up, a 100-year simulation was run starting with a windthrow event on the first day of the first year. The results presented in this study come from the 100-year-long simulations. Given the focus on even-aged monospecific spruce forests in regions where spruce growth is not constrained by precipitation, variables such as ihosts share and ihosts defense were omitted from this study. Note that ORCHIDEE does not account for possible acclimation e.g., temporal changes in bark beetle behavior or bark beetle resistance to external stressors such as winter temperature.
3.2 Selection of locations
Bark beetle populations are known to be sensitive to temperature as they are more likely to survive a mild winter (Lombardero et al., 2000) and tend to breed earlier when winter and spring are warmer than usual, allowing for multiple generations in the same year (Hlásny et al., 2021a; see also Eq. 10 in Sect. 2.6). In order to assess the temperature effect of the bark beetle outbreak model in ORCHIDEE, eight locations in Europe were selected (Table 2) which represent the range of climatic conditions within the distribution area of Norway spruce (Picea abies H. Karst L.), the main host plant for Ips typographus, the bark beetle species under investigation.
Table 2Climate characteristics of the eight locations used in the simulation experiments. The acronyms refer to the site names used in the FLUXNET database (Pastorello et al., 2020).
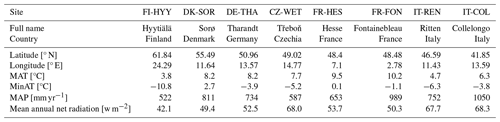
For these eight locations, half-hourly weather data from the FLUXNET database (Pastorello et al., 2020) were used to drive ORCHIDEE. Some of these locations (FON, SOR, HES, COL, WET) are in reality not covered by spruce, but all sites are, however, located within the distribution of Norway spruce. In this study, locations were selected to use the observed weather data to simulate a credible temperature and rainfall gradient for spruce. HES location is no longer part of the FLUXNET network, but the previous data are still available and relevant for this analysis.
3.3 Sensitivity to model parameters
The sensitivity assessment evaluates the responsiveness of four key variables (ihosts susceptibility, ibeetles mass attack, ibeetles generation, and ibeetles activity) of the Ips typographus outbreak model implemented in ORCHIDEE. The assessment aims to demonstrate the ability of ORCHIDEE to simulate diverse dynamics of bark beetle infestations. The selection of ihosts susceptibility, ibeetles activity, ibeetles mass attack, and ibeetles generation was based on two criteria: (1) their substantial influence on the dynamics of the Ips typographus outbreak noted during model development and (2) their independence from direct measurable data, rendering them less suitable for evaluation through literature review.
For each of the four variables, three distinct values were assigned to two parameters labeled “shape” and “limit”. The shape parameter determines the shape of the logistic relationship, with three values tested: (a) shape = −1.0, yielding a linear relationship; (b) , resulting in a logistic curve; and (c) shape = −500.0, turning the logistic relationship into a step function. For the logistic curve, the exact shape value between −30.0 and −5.0 is chosen according to each index under study: (1) , (2) , (3) , and (4) Sgeneration=5.0. For Smass attack and Sactivity, higher values were chosen because the slope of the logistic curve has significant impact in order to trigger an outbreak.
The second parameter called limit determines the threshold, derived from expert insights, at which the logistic relationship will reach its midpoint value of 0.5 (ird susceptibility, BPlimit, Actlimit, or Glimit). For instance, ird susceptibility is set to 0.55, indicating ihosts susceptibility midpoint sensitivity (Eq. 6′). Setting BPlimit to 0.12 results in an ibeetles mass attack midpoint when ibeetles pressure is 0.12, selected for its proximity to scenarios where ihosts dead equals 1.0 (Eq. 14). The variable actlimit was positioned at 0.06, signifying the ibeetles activity midpoint at DRbeetles=6 % from the preceding year, exceeding endemic levels yet not reaching epidemic outbreaks (Eq. 10). Lastly, Glimit is fixed at 1.0, denoting the midpoint for ibeetles generation upon completing one generation annually, underpinning the rarity of bark beetle outbreaks with fewer than one generation per year (Eq. 9). Starting from these reference values, a restrictive simulation was run in which the limit parameter values were reduced by 50 %. Likewise, a permissive simulation was run to test 50 % higher values for limit.
The sensitivity analysis of the model parameters explores 36 (product of three shapes, three limits, and four equations) combinations of parameter values named a set, but the full design of the experiment is 83=512 sets (eight parameters, with three values for each). This deliberate choice was made because of the computation time cost of a single run. In order to reduce the number of runs from 512 to 36, we had to make simplifications: (1) one equation at the time is studied, reducing the number of sets necessary to realize the sensitivity analysis to nine, and (2) every other parameters from the remaining equation is set to default value; e.g., limit values are set to their reference values, and shape values are set to their a priori assumption (Table 4). The major drawback of this approach is that interaction effects between equations cannot be investigated in the study. Nonetheless, this sensitivity analysis aims to document model behavior, rather than seek precise parameter values which can be achieved with the main effect of each equation only (see Sect. 3.4).
The simulations were run for the THA site, where they were repeated for two prescribed windthrow events with a different intensity, i.e., a DRwindthrow of 0.1 % and 10 %. The effect of the parameters with a negligible windthrow event, i.e., killing only 0.1 % of the trees, was tested to confirm that the selected parameters did make ORCHIDEE simulate a bark beetle outbreak in the absence of windthrow (score 5 in Sect. 3.4).
3.4 Parameter tuning and credibility score
The results of the sensitivity experiment were used to select key model parameters. Selecting the values for the Shape and Limit parameters (see Sect. 3.3) used in the calculation of the variables ihosts susceptibility, ibeetles mass attack, ibeetles generation, and ibeetles activity has been carried out in order to reproduce the observed dynamics of bark beetle outbreaks. Observed dynamics were compiled through a literature search for peer-reviewed papers that reported quantitative characteristics of bark beetle outbreaks (Table 3). Five characteristics could be documented and used to calculate the score:
-
the delay between the windthrow event and the start of the bark beetle outbreak (score 1);
-
the length of the bark beetle outbreak, defined by the number of years required for a bark beetle population to go back to its endemic level (score 2);
-
the cumulative number of trees per unit area, killed by the bark beetles at the end of an outbreak (score 3);
-
the average tree mortality rate (DRbeetles) during an endemic stage (score 4);
-
no outbreak triggered when DRwindthrow=0.1 %, which is a mandatory characteristic in order to avoid outbreak for no specific event (score 5).
Based on Table S1 in the Supplement and the reference range in Table 3, scores are calculated for each parameter set, and values are either 0 or 1. The credibility score (CS) is the sum of four scores multiplied by a fifth score. The CS is computed as follows: CS = (score 1 + score 2 + score 3 + score 4) × score 5. Only parameter sets that achieve a CS of 4 are selected. If multiple parameter values are possible for a given equation, the most frequently selected value is preferred.
Table 3Literature-based summary of characteristics of large-scale bark beetle outbreaks. Due to data scarcity, the characteristics combine outbreak dynamics of different bark beetle species, different host species, and different locations. The reference range is used to calculate the credibility score (CS) of each set of parameters (see Table S1).
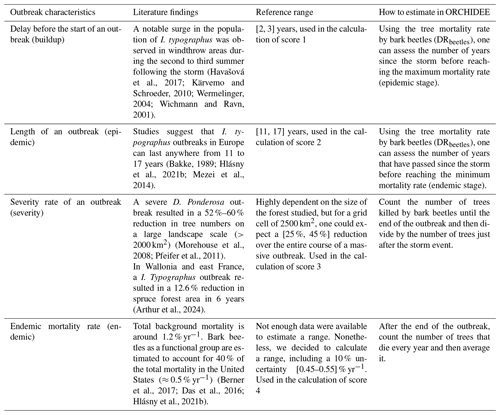
3.5 Sensitivity to climate and windthrow
In this simulation experiment, the influx of fresh dead tree hosts (Nwood) used for bark beetle breeding was controlled by modifying the maximum damage rate of a windthrow event (DRwindthrow) in ORCHIDEE. Seven DRwindthrow values were simulated (i.e., 0.1 %, 5 %, 7.5 %, 10 %, 15 %, 20 %, and 35 %). Given the monotonic nature of the relationships between DRwindthrow and ihosts dead (Eq. 12), each event triggers a proportional increase in the dead host availability (ihosts dead) scaling between 0 and 1 (Fig. 3). Through its equations, ORCHIDEE assumes that for damage rates above 20 %, the variable ihosts dead (Nwood) will always be equal to 1.0. The index ird spruce, however, may further decrease with increasing windthrow damage, which makes the 35 % damage rate still interesting to investigate. Although the simulations were run for all DRwindthrow, only four windthrow damage rates, including a windstorm resulting in a 35 % damage rate (Fig. 3), were presented to enhance the readability of the Results section.
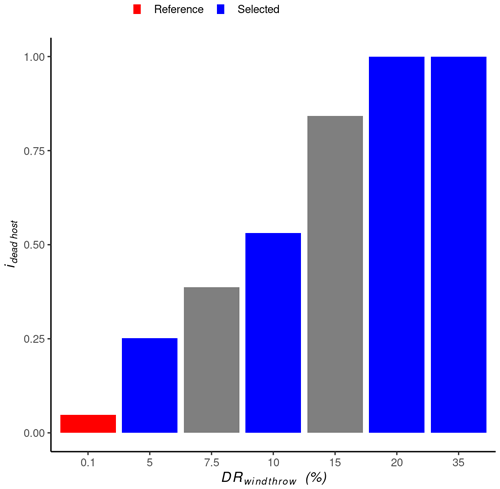
Figure 3Relationship between windthrow damage rate (DRwindthrow) and the dead host index (ihosts dead). For each site, a DRwindthrow=0.1 % was used as the reference simulation because an endemic bark beetle population is expected following such a low-intensity windthrow event. The four DRwindthrow values shown in blue were selected for the subsequent presentation of the results because they cover the entire range for ihosts dead.
The main driver of the number of generations a bark beetle population can achieve in 1 year is the number of days with a temperature higher than 8.3 °C during winter time (Temperli et al., 2013), which is the reason why temperature is so important for bark beetle reproduction. By taking REN, THA, WET, and HES, the number of bark beetle generations ranged from 0.8 to 3.5 (Fig. 4), which is similar to the number of generations observed across Europe (Faccoli and Stergulc, 2006; Jönsson et al., 2009, 2011). Limiting the analysis to only four sites simplifies the presentation without affecting the range under investigation.
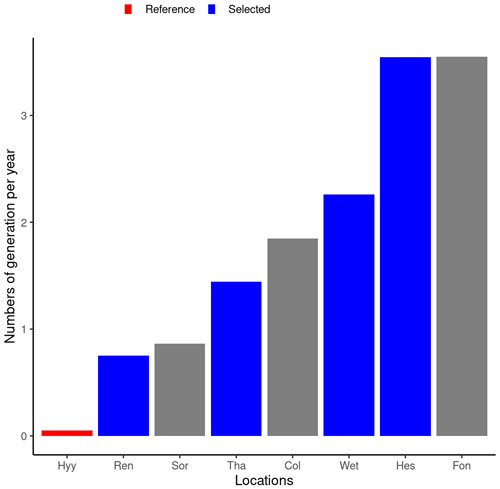
Figure 4Average number of bark beetle generations during the 5 years following the wind storm for eight locations along a climate gradient. The HYY location in Finland was selected as the reference for the REN, THA, WET, and HES locations. Only results from the reference and four selected locations (shown in blue) are shown to enhance readability.
For the climate gradient, the simulation for HYY served as a reference since the number of generations is lower than 1, for which no outbreak should happen under any circumstances. Under present climate conditions, an outbreak in HYY should be considered an undesirable model result. Likewise, a DRwindthrow=0.1 % is considered too low to trigger an outbreak and was therefore used as the reference for the wind damage rate tests.
The experiment consisted of 40 simulations, i.e., right sites (including the reference) multiplied by five wind damage rates (including the reference). Although the simulations were also run for SOR, COL, and FON, their results were found to be too similar to the results of selected sites to present them as well. Hence, the Results section presents only 25 out of the 40 simulations. Three output variables were assessed: bark beetle damage rate (DRbeetles), total biomass (Btotal), and net primary production (NPP). Total biomass was investigated over 100 years, whereas DRbeetles and NPP were assessed for the first 20 years following a windthrow.
3.6 Continuous vs. abrupt mortality
Where most land surface models use a fixed turnover time to simulate continuous mortality (Pugh et al., 2017; Thurner et al., 2017), ecological reality is better described by abrupt mortality events. An idealized simulation experiment was used to qualify the impact of abrupt mortality on net biome productivity by changing from a framework in which mortality is approximated by a constant background mortality to a framework in which mortality occurs in abrupt, discrete events. The impact of a change in the mortality framework was assessed with an idealized simulation experiment that compared three configurations of ORCHIDEE: (1) a configuration that simulates mortality as a continuous process, labeled “the continuous configuration”, which corresponds to previous versions of ORCHIDEE; (2) a configuration capable of simulating abrupt mortality from windthrow and subsequent bark beetle outbreaks, labeled “the abrupt configuration”; and (3) a configuration in which windthrow is activated but a bark beetle outbreak is implicitly accounted for in the background mortality. This third configuration enabled attributing the impact to windthrow. The effect of simulating abrupt mortality was evaluated over 20-, 50-, and 100-year time horizons.
The impact of changing the mortality framework from continuous to abrupt was quantified on the basis of 120 simulations (eight locations multiplied by seven windthrow damage rates and two configurations, with added eight sites multiplied by one configuration) of 100 years each.
The simulations with abrupt mortality were run first. Subsequently, the number of trees killed was quantified and used as a reference value for the continuous mortality setup. This approach resulted in the same quantities of dead trees at the end of the simulation for both frameworks, which then differed only in the timing of the simulated mortality. This precaution is necessary to avoid comparing two different mortality regimes where the result would mainly be explained by the intensity of the mortality rather than by its underlying mechanisms.
Changes in forest functioning were evaluated through the temporal evolution of accumulated net biome productivity (NBP) over a 100-year time frame. NBP is defined as the regional net carbon accumulation after considering losses of carbon from fire, harvest, and other episodic disturbances. In ORCHIDEE, NBP is calculated following the definition by Chapin et al. (2006) as the carbon remaining in the biomass, litter, and soil after accounting for photosynthesis and respiration because fire, harvest, leaching, and volatile emissions were not accounted for in this simulation experiment.
4.1 Sensitivity to model parameter sets
The impact of spruce stand competition (ihosts susceptibility) on outbreak dynamics was examined by adjusting the parameters Ssusceptibility and ird susceptibility in Eq. (6a′). When Ssusceptibility resulted in a linear relationship (), no peak in bark beetle damage occurred for the three tested values of ird susceptibility (permissive, reference, and restrictive) at a 10 % windthrow damage rate (Fig. 5h). However, employing a step function ) led to either sporadic peaks of bark beetle damage with a permissive ird susceptibility or a 2-year outbreak with a maximum damage rate of 60 % and a restrictive ird susceptibility (Fig. 5h), neither of which aligns with the observations summarized in Table 3.
The outcome closest to observations from Table 3 was obtained with a logistic relationship (), where ird susceptibility determined the duration of the outbreak: 11, 16, and 25 years for restrictive, reference, and permissive parameter values, respectively (Fig. 5h). Either the restrictive or the reference parameter value could be utilized since a range of 11–16 years aligns with the observations (Table 3). To examine the occurrence of improbable outbreaks, sensitivity tests were repeated for a 0.1 % windthrow damage rate. None of the nine parameter combinations triggered an outbreak (Fig. 5g), suggesting that improbable outbreaks due to the calculation of ihosts susceptibility are unlikely.
From the calculation of the credibility score, only one set obtains a score of 4 (, ird susceptibility=0.55; Table S1). The concerning parameter value was selected and is reported in Table 4.
The effect of the capability of bark beetle to mass attack (ibeetles mass attack) when the population exceeds a threshold was evaluated by varying Smass attack and BPlimit (Eq. 14). Linear relationships () resulted in similar outbreak dynamic for all BPlimit values, with the model settling on a constant endemic damage following an outbreak, though higher than observed (Table 3; Fig. 5f). Introducing a logistic or step function slightly altered outbreak dynamics except when assuming a step function for the restrictive value, which prevented an outbreak. Repeating sensitivity tests for a 0.1 % windthrow damage rate showed that assuming linear or logistic relationships could trigger an outbreak (Fig. 5e), indicating that improbable outbreaks may arise from the calculation of ihosts mass attack.
From the calculation of the credibility score, three sets obtain a score of 4, but only set 4.6 was chosen because of its intermediate position compared to sets 4.9 and 4.5 (Table S1). The concerning parameter values (, BPlimit=0.06) were selected and are reported in Table 4.
The impact of bark beetle activities from the previous year (ibeetles activity) on outbreak dynamics was investigated by varying Sactivity and actlimit (Eq. 10). Linear or logistic relationships resulted in excessively long outbreaks (>30 years) compared to observations (Table 3; Fig. 5b), whereas assuming a step function relationship simulated a decline in the outbreak after 14 years. Sensitivity tests repeated for a 0.1 % windthrow damage rate showed that assuming a linear relationship could trigger an improbable outbreak (Fig. 5a) through the calculation of ibeetles activity.
From the calculation of the credibility score, only one set obtains a score of 4 (, actlimit=0.12; Table S1). The concerning parameter value was selected and are reported in Table 4.
To explore the effect of the numbers of generation (ibeetles generation) on the outbreak dynamics, Sgeneration and Glimit from Eq. (9) were varied. The bark beetle damage rate was more sensitive to Glimit than Sgeneration, but only a linear relationship with the reference Glimit=1.0 yielded an intermediate outbreak intensity consistent with the continental climate at the test location (i.e., THA; Fig. 5d). Other combinations resulted in either too strong of a peak or no peak during the outbreak. Repeating sensitivity tests for a 0.1 % windthrow damage rate showed that none of the nine parameter combinations triggered an outbreak (Fig. 5c), indicating that improbable outbreaks from the calculation of ibeetles generation are unlikely.
From the calculation of the credibility score, three sets obtain a score of 4, but only set 1.4 was chosen because of its intermediate position compared to sets 1.1 and 1.5 (Table S1). The concerning parameter values (Sgeneration=1.0, Glimit=1.0) were selected and are reported in Table 4.
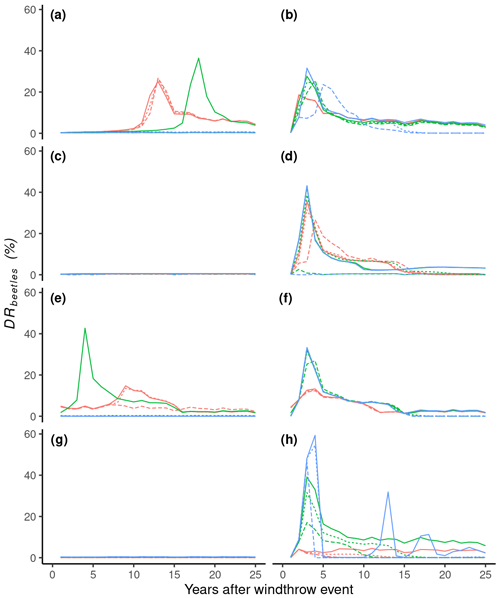
Figure 5Simulation results from the sensitivity experiment at the THA site. Eight parameters from four equations were evaluated. Each equation represents an index from the bark beetle outbreak model (ihosts susceptibility, ihosts mass attack, ibeetles activity, and ibeetles generation). Each index is represented by a logistic function defined by a shape parameter (Shape) and a limit parameter (Limit). Three values were chosen for each parameter, resulting in nine pairs of parameters for each index. Colored lines represent the shape parameter varying from linear (Shape = −1.0, red; logistic , green) to step function, where Shape = −500.0 (blue). The line type represents three different values for the Limit parameter, where references (dashed line) are values of ird susceptibility, BPlimit, actlimit, and Glimit (given in Table 4), whereas permissive (full line) and restrictive (dashed–dotted) represent a 50 % decrease or increase, respectively.
4.2 Model tuning
By comparing the outcomes of the sensitivity tests (Sect. 4.1) to a compilation of observations (Table 3), a first estimate for several parameters was proposed (Table 4). Details about the other values used in the sensitivity test can be found in Table S1.
4.3 Impact of climate and windthrow on bark beetle damage
In ORCHIDEE, the warmest sites, HES and WET, experienced significant bark beetle outbreaks across a wide spectrum of windthrow mortality rates, whereas colder sites like REN and THA saw outbreaks only in response to the most severe windthrow events (Fig. 6b, c). A greater average number of bark beetle generations in the years following windthrow events led to higher bark beetle damage rates at the peak of outbreaks. For instance, at a 35 % windthrow mortality rate, HES reached a maximum bark beetle damage rate of 50 %, whereas REN's maximum was 22 % (Fig. 6a, b).
Interestingly, high tree mortality rates from windthrow could also lead to delays and a lower maximum DRbeetles (Fig. 6). For instance, at the HES site, 10 %, 20 %, and 35 % windthrow damage rates triggered maximum DRbeetles values of 50 %, 43 %, and 37 %, respectively (Fig. 6a). Conversely, low DRwindthrow, such as that of 5 % at WET, delayed the peak of bark beetle outbreaks by 9 years (Fig. 6d). Additionally, the model simulated a post-epidemic stage during which the outbreak damage rate remained relatively low (<10 %) and lasted between 3 and 10 years (Fig. 6). Overall, the simulated outbreaks lasted between 11 and 20 years, which is consistent with field observations (Table 3).
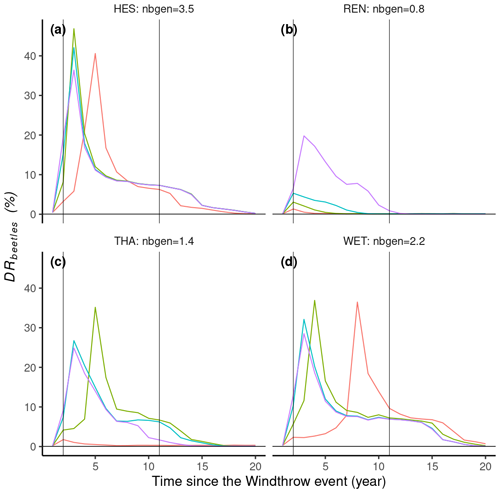
Figure 6Simulation results of 16 simulations (four locations with four windthrow damage rate values, DRwindthrow). Lines represent the annual bark beetle damage rate as a fraction of the total biomass (DRbeetles). Nbgen is the average number of bark beetle generations during 5 years after the windthrow event. DRwindthrow represents the percentage of biomass loss by a windthrow event at the start of the simulation.
At the coldest site, HYY, ORCHIDEE simulated only a small number of bark beetle generations, preventing outbreaks from occurring. This observation validates the initial parameter tuning (Table 4), indicating that it is robust enough to prevent improbable outbreaks, such as the model triggering outbreaks in sites where bark beetles cannot reproduce.
4.4 Impact of climate and windthrow on stand biomass and net primary production
All locations experienced a 10- to 20-year decrease in total biomass until at most 9 kg C m−2, at which time the outbreak ended (Fig. 7a, b, c, d). The model can simulate significant epidemic events even if the initial trigger, such as the windthrow event in our study, is not particularly intense. Once the bark beetles can mass attack living trees, the bark beetle population (ibeetles pressure) will increase and kill more and more trees until so many trees are killed that the stand density of the remaining living trees drops below the threshold of . In ORCHIDEE, an ird limit=0.4 for spruce forest corresponds to a biomass of around 9 kg C m−2, which in ORCHIDEE is too low to maintain an epidemic population of bark beetles at the 2500 km2 grid cell. Interestingly, for the climate observed at REN, where the number of generations is approximately one, the bark beetle population can only become an epidemic following an intense windthrow event with a 35 % damage rate (Fig. 7).
Throughout the outbreak period, there was a notable decrease in net primary production (NPP) (Fig. 7). This decrease is primarily attributed to a sharp decline in the leaf area index (not shown). Following the epidemic phase, the leaf area recovers. Following the outbreak, the reduction in stand tree density due to bark beetle damage decreases autotrophic respiration (not shown), and the sparser canopy allows for more light to reach the forest floor, where it fosters recruitment (not shown), resulting in a higher NPP or forest growth (Fig. 7). Consequently, carbon use efficiency tends to be higher in sparsely populated stands compared to densely populated ones.
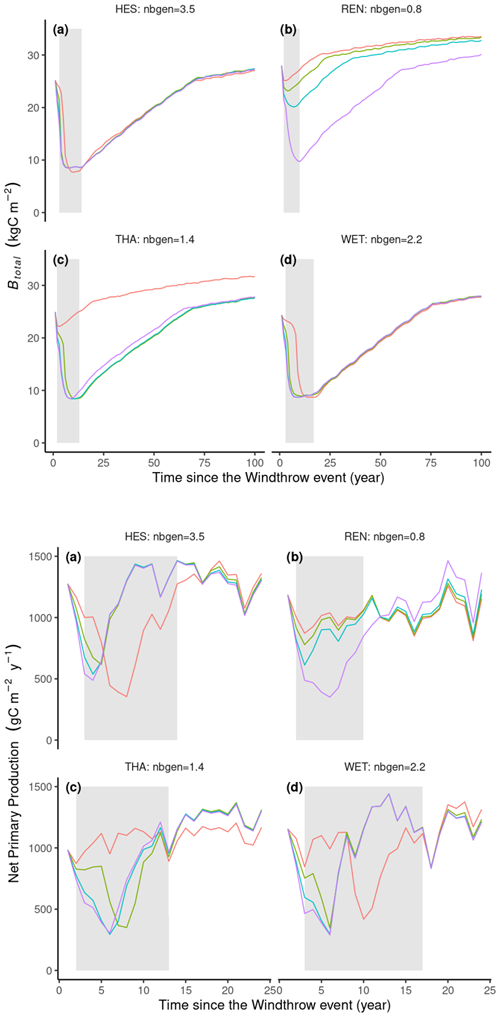
Figure 7Simulation results of 16 simulations (four sites with four windthrow mortality rate values). Lines represent the annual average net primary production (NPP) in g C m−2 yr−1 or total stand biomass (Btotal) in kg C m−2. Nbgen is the average number of bark beetle generations during the 5 years after the windthrow event. DRwindthrow represents the percentage of biomass loss by a windthrow event at the start of the simulation. Grey areas represent the epidemic phase.
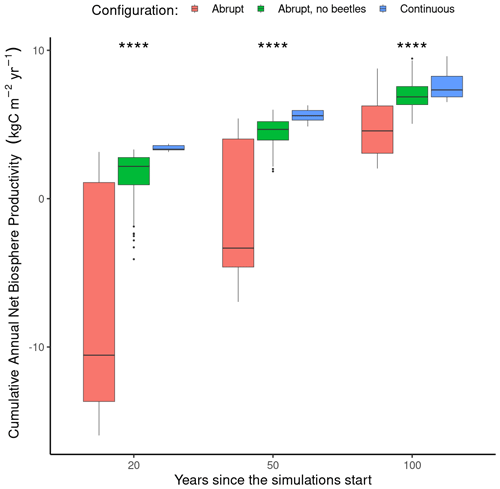
Figure 8Difference in cumulative net biome production at three discrete time horizons (i.e., 20, 50, and 100 years) between a fixed continuous mortality rate (blue, n=8), abrupt tree mortality from a windstorm and the subsequent bark beetle outbreak (red, n=56), and abrupt mortality from a windstorm not followed by a bark beetles outbreak (green, n=56). Note that in the continuous mortality configuration, the mortality rate was adjusted to obtain a similar number of trees killed after 100 years as in the abrupt mortality configuration. The variation in each box plot arises due to different locations and prescribed storm intensities. Each box plot displays the median value (thick horizontal line), the quartile range (box border), and the 95 % confidence interval (vertical line). A Wilcoxon test between the three configurations at each time horizon showed significant differences (p value <0.0001) denoted by the four stars.
4.5 Continuous vs. abrupt mortality
The total accumulated net biome production (NBP) was evaluated using the ORCHIDEE model across three different time frames: 20, 50, and 100 years. At the 20-year mark, the average accumulated NBP notably differed between mortality configurations that are continuous, abrupt, and abrupt without a bark beetle outbreak (“no-beetle”): , and 3.39±0.74 kg C m−2 yr−1 for the abrupt, no-beetle, and continuous mortality configurations, respectively. These differences were statistically significant (Wilcoxon, p value <0.0001), indicating a substantial initial reduction in NBP with the abrupt configurations as ecosystems behaved as carbon sources, whereas under the continuous configuration, they acted as carbon sinks (Fig. 8). The variability in NBP demonstrated the broad temperature gradient in Europe and indicated that despite many locations potentially acting as sources under the abrupt configuration, some may transition to carbon sinks within the first 20 years following a disturbance.
Moving to the 50-year horizon, the difference between the three frameworks decreased, with net biome productions of , 4.43±0.15, and 5.61±0.18 kg C m−2 yr−1 for the abrupt, no-beetle, and continuous mortality configurations, respectively. The difference in sink strength remained statistically significant (Wilcoxon; p value <0.001), with the NBP in the abrupt configuration approaching carbon neutrality, while without the consecutive bark beetles outbreak, the ecosystems already became a carbon sink. The climate conditions had a lasting effect on the responses, with the abrupt configuration showing a greater range in responses compared to the continuous one.
At the 100-year mark, the average cumulative NBP values for the abrupt and continuous configurations approached each other with values of 4.85±0.26, 7.09±0.17, and 7.73±0.40 kg C m−2 yr−1, respectively (Fig. 8) but were still significantly different (Wilcoxon, p value <0.001). ORCHIDEE simulated a return to a carbon sink (indicated by positive cumulative NBP values) suggesting a long-term recovery and potential return to pre-disturbance productivity levels within a century following the windthrow and beetle outbreak event. The continuous configuration displayed a consistently higher median value, suggesting weaker impact of tree mortality dynamics on the long-term carbon cycle.
5.1 Simulating the dynamics of bark beetle outbreaks and their interaction with windthrow
Our Ips typographus outbreak model has demonstrated its capability to simulate a broad range of disturbance dynamics. The variation in the outbreak dynamics and the response of the outbreak to its main drivers (Figs. 5 and 6) give confidence in the ability of ORCHIDEE to simulate various outbreak scenarios observed across the temperate and boreal zones under changing climate conditions.
Windthrow events have a significant ecological impact because such disturbances offer fresh breeding substrates, which in turn increase bark beetle populations (Lausch et al., 2011). Our model results align with these findings, indicating that windthrows causing damage of 5 % or more may trigger beetle outbreaks (Fig. 6). Additionally, a strong increase in bark beetle populations has been observed following a windthrow event (Wermelinger, 2004), a pattern reflected in the ORCHIDEE simulations. The model simulates a buildup stage spanning 1 to 9 years, where bark beetle numbers increase prior to peaking, with the duration influenced by the severity of the windthrow and the prevailing climate (Fig. 6).
Temperature is another critical factor affecting bark beetle life cycles. Intra- and interannual variation in temperature impact bark beetles, with warmer conditions fostering multiple generations per year, whereas cooler, damp climates slow breeding and survival rates (Benz et al., 2010). In line with these findings, the temperature dependence of the ORCHIDEE simulations show that cold winters at locations such as SOR and REN reduced bark beetle activity compared to warmer locations like THA and WET (Fig. 6). Lieutier et al. (2002) documented that if the population is large enough, bark beetles can mass attack healthy trees. Our model incorporates this dynamic, which is illustrated by epidemic stages where living trees become viable hosts, which then exacerbates the growth of the beetle population (Fig. 1).
The aftermath of a windthrow and subsequent bark beetle outbreak also affects the forest carbon and nitrogen cycles. This impact is observed in the form of snags, which are standing dead trees that undergo decomposition. Snags can temporarily disrupt the link between soil and ecosystem carbon and nitrogen dynamics (Rhoades, 2019; Custer et al., 2020). While in ORCHIDEE the decay of fallen logs does not account for snags yet, the model suggests a recovery period ranging from 5 to 15 years, contingent upon the intensity of the bark beetle outbreak (Fig. 7). As snags create gaps in the canopy, conditions favorable to natural forest regeneration emerge (Jonášová and Prach, 2004).
5.2 Emerging properties from interacting disturbances
While this study did not precisely quantify the impact of simulating abrupt mortality rather than approaching mortality as a continuous process, it demonstrated that the impact of abrupt mortality varies across location and time; i.e., ecosystem functions, such as carbon storage, are affected by natural disturbances like Ips typographus outbreaks, having significant impacts on short- to mid-term carbon balance estimates (Fig. 8). The simulation experiments also highlighted that the legacy effects of disturbances can endure for decades even for a simplified representation of forest ecosystems such as ORCHIDEE, where the recovery might be too fast due to the absence of snags (Senf et al., 2017).
The ability to simulate resistance (i.e., staying essentially unchanged despite the presence of disturbances; Grimm and Wissel, 1997) as an emerging property is evident from Figs. 6 and 7 for REN, where no bark beetle outbreaks were observed following a medium windthrow event (5 %–20 %). However, in all simulated locations that could not resist a bark beetle outbreak, the forest was resilient (i.e., returning to the reference state or dynamic after a temporary disturbance; Grimm and Wissel, 1997), and ecosystem functions were restored to the level from before the windthrow. The elasticity (the speed of return to the reference state or dynamic after a temporary disturbance; Grimm and Wissel, 1997) of the carbon sink capacity ranged from 7 to 14 years. This elasticity is in line with the little observational evidence of ecosystem shifts due to natural disturbances in forests (Millar and Stephenson, 2015). Finally, after the disturbance and the recovery of vegetation structure, the ecosystems simulated by ORCHIDEE showed persistence (i.e., continue along their initial developmental path; Grimm and Wissel, 1997).
5.3 Are cascading disturbances important for carbon balance estimates?
The enhanced complexity introduced in the ORCHIDEE model by incorporating abrupt mortality events as opposed to a continuous mortality prompts the question of whether this model refinement yields new insights into carbon balance estimates. Our century-long analysis demonstrated that the net biome production, a the metric for carbon sequestration, ultimately converges between the continuous and abrupt mortality frameworks (Fig. 8). This suggests that irrespective of the nature of the mortality events, the forest ecosystem goes through a recovery phase, marked by increased growth that compensates for the growth deficits during the disturbance.
However, our experiment has not taken into account the frequency of disturbances. Given the profound influence of disturbance legacies on carbon dynamics, a recurrence interval shorter than the recovery time of the forest might result in a tipping point. Such a scenario could diminish the carbon sequestration potential of the forest beyond the 100-year time frame and, in extreme cases, may even lead to ecosystem collapse, an outcome not explored in the current simulations nor documented in the recent literature (Millar and Stephenson, 2015).
In the mid-term, spanning 20 to 50 years, the widely used continuous mortality model appears to inflate the carbon sink capabilities of forests when juxtaposed with abrupt mortality scenarios. Since policy frameworks, including the Green Deal for Europe (2023) and the Paris Agreement (UNFCCC, https://unfccc.int/process/the-kyoto-protocol, last access: March 2023), were imposed upon these medium-term predictions, they would benefit from adopting model simulations that integrate abrupt mortality events to avoid an overestimation of carbon sink capacities of forest. Furthermore, the accuracy of carbon balance estimates strongly depends on the initial state of the forest in the model. Forest conditions markedly affect carbon uptake rates. Thus, incorporating an abrupt mortality framework into the ORCHIDEE model could substantially refine and strengthen the predictive power of our carbon balance assessments across short-, medium-, and long-term scales.
However, it is important to note that the global-scale NBP might not be significantly impacted by abrupt disturbances compared to continuous ones. This is because the scale is so vast that abrupt mortality events are constantly occurring somewhere on the planet, which smooths out the effects illustrated in Fig. 8.
5.4 Shortcomings of the bark beetle outbreak model
The bark beetle outbreak model developed in this study builds upon the strengths of the previously established LandClim model, though it also inherited some of its limitations. One notable shortcoming is the model for bark beetle phenology, which is an empirical model that makes use of accumulated degree-days. Since the conception of the phenology model a decade ago, Europe's climate has undergone substantial changes, primarily manifested in warmer winters and springs (Copernicus, 2024). Because of these changes, chances have increased that two or even more bark beetle generations occur within a calendar year (Hlásny et al., 2021a). These changes call for an update of the beetle's phenology model to align with these more recent observations (Ogris et al., 2019).
A second limitation is that our study, ORCHIDEE, has been parameterized to simulate only Ips typographus in Europe. In order to change the beetles and tree host interactions e.g., pine bark beetle in North America (Dendroctonus monticolae Hopkins), the sensitivity of indexes must be revised; for example, the pine beetle does not breed on the dead wood falling from windthrow but is very sensitive to drought events (Preisler et al., 2012). The indexes ihosts defense and ihosts dead as well as the phenology model will need to be revised.
Another issue is the model's consideration of drought. As outlined in the “Methods and material” section, drought is treated as an exacerbating factor rather than a primary trigger, as is the case for windthrow. This understanding was accurate for Ips typographus a decade ago (Temperli et al., 2013); however, emerging evidence increasingly suggests that drought events may indeed trigger bark beetle outbreaks across Europe (Nardi et al., 2023; Netherer et al., 2015). Consequently, this extreme drought as a trigger should be incorporated in a future revision of ORCHIDEE's Ips typographus outbreak model.
This study simulated the one-way interaction between windthrow and Ips typographus outbreaks in unmanaged forests. Future research will incorporate additional interactions, such as the interplay between droughts, storms, and bark beetles; storms, bark beetles, and fires; and forest management, storms, and bark beetles.
The bark beetle outbreak model could also be enhanced by simulating: (a) standing dead trees (or snags), which would help account for differences in wood decomposition between snags and logs (Angers et al., 2012; Storaunet et al., 2005); (b) migration of bark beetles to neighboring locations, which becomes significant to account for in a model that operates at spatial resolutions below approximately 10 km; and (c) an up-to-date beetle phenology model which accounts for the recent change in their behavior induced by climate change.
This research provided an initial qualitative assessment of a new model feature. However, the application of the model necessitates an evaluation of the simulations against observations of cascading disturbances on the regional scale, which is the topic of an ongoing study.
Our approach enables improving the realism of the Ips typographus model in ORCHIDEE without reducing its generality (Levins, 1966). The integration of a bark beetle outbreak model in interaction with other natural disturbances such as windthrow into the ORCHIDEE land surface model has resulted in a broader range of disturbance dynamics and has demonstrated the importance of simulating various disturbance interaction scenarios under different climatic conditions. Incorporating abrupt mortality events instead of a fixed continuous mortality calculation provided new insights into carbon balance estimates. The study showed that the continuous mortality framework, which is commonly used in the land surface modeling community, tends to overestimate the carbon sink capacity of forests in the 20- to 50-year range in the region/area under the same disturbance pressure compared to scenarios with abrupt mortality events.
Apart from these advances, the study revealed possible shortcomings in the bark beetle outbreak model, including the need to update the beetle's phenology model to reflect recent climate changes and the need to consider extreme drought as a trigger for bark beetle outbreaks in line with emerging evidence. Looking ahead, future work will further develop the capability of ORCHIDEE to simulate interacting disturbances such as the interplay between extreme droughts, storms, and bark beetles and between storms, bark beetles, and fires.
The final step will be a quantitative evaluation based on observed data (Marini et al., 2017) in order to assess the capability of ORCHIDEE to simulate complex interaction between multiple sources of tree mortality affecting the carbon balance on a large scale.
R script and data are available at https://doi.org/10.5281/zenodo.12806280 (Marie, 2024). ORCHIDEE rev 8627 code is also available from https://doi.org/10.14768/08ca9318-b663-40e3-90e6-6baee0475ba0 (IPSL Data Catalog, 2024).
The Fluxnet climate forcing data are available at https://fluxnet.org/data/download-data/ (Pastorello et al., 2020).
The simulation results used in this study are available at https://doi.org/10.5281/zenodo.12806280 (Marie, 2024).
The supplement related to this article is available online at: https://doi.org/10.5194/gmd-17-8023-2024-supplement.
GM and SL designed the experiments, and GM conducted them. Following discussions with HJ, GP, and MC, GM developed the bark beetle model code and performed the simulations. JJ coupled the wind damage and bark beetle models with each other. GM, JJ, VB, JG, BG, ASL, MJM, KN, AV, CY, and SL contributed to the development, parameterization and evaluation of the ORCHIDEE revision used in this study. GM, JJ, and SL prepared the paper with contributions from all co-authors.
The contact author has declared that none of the authors has any competing interests.
Publisher's note: Copernicus Publications remains neutral with regard to jurisdictional claims made in the text, published maps, institutional affiliations, or any other geographical representation in this paper. While Copernicus Publications makes every effort to include appropriate place names, the final responsibility lies with the authors.
This work was performed using high-performance computing (HPC) resources from GENCI-TGCC (grant no. 2022-06328). The Open AI textual AI GPT4 (https://chat.openai.com/, last access: March 2024) was used for language editing at an early stage of manuscript preparation.
Guillaume Marie was funded by MSCF (CLIMPRO; grant no. 895455) and ADEME (DIPROG; grant no. 2003C0002). Sebastiaan Luyssaert and Kim Naudts were funded by the EU Horizon 2020 HoliSoils program (grant no. SEP-210673589) and the Horizon Europe INFORMA program (grant no. 101060309). Jina Jeong and Bertrand Guenet were funded by the EU Horizon 2020 HoliSoils program (grant no. SEP-210673589). Gunnar Petter was funded by the Swiss National Science Foundation (grant no. SNF 163250). Anne Sofie Lansø was funded by the EU Horizon 2020 Crescendo program (grant no. 641816). Chao Yue was funded by the National Science Foundation of China (grant nos. U20A2090 and 41971132). Matthew J. McGrath was supported by the European Commission Horizon 2020 framework program (VERIFY; grant no. 776810) and the European Union’s Horizon 2020 research and innovation program (CoCO2; grant no. 958927). Aude Valade was funded by the Agropolis Fondation (grant no. 2101-048).
This paper was edited by Sam Rabin and reviewed by two anonymous referees.
Abramowitz, G., Leuning, R., Clark, M., and Pitman, A.: Evaluating the Performance of Land Surface Models, J. Climate, 21, 5468–5481, https://doi.org/10.1175/2008JCLI2378.1, 2008.
Allen, C. D., Breshears, D. D., and McDowell, N. G.: On underestimation of global vulnerability to tree mortality and forest die-off from hotter drought in the Anthropocene, Ecosphere, 6, art129, https://doi.org/10.1890/ES15-00203.1, 2015.
Andrus, R. A., Hart, S. J., and Veblen, T. T.: Forest recovery following synchronous outbreaks of spruce and western balsam bark beetle is slowed by ungulate browsing, Ecology, 101, e02998, https://doi.org/10.1002/ecy.2998, 2020.
Angers, V. A., Drapeau, P., and Bergeron, Y.: Mineralization rates and factors influencing snag decay in four North American boreal tree species, Can. J. For. Res., 42, 157–166, https://doi.org/10.1139/x11-167, 2012.
Arthur, G., Jonathan, L., Juliette, C., Latte, N., Piedallu, C., and Claessens, H.: Spatial and remote sensing monitoring shows the end of the bark beetle outbreak on Belgian and north-eastern France Norway spruce (Picea abies) stands, Environ. Monit. Assess., 196, 226, https://doi.org/10.1007/s10661-024-12372-0, 2024.
Bakke, A.: The recent Ips typographus outbreak in Norway – experiences from a control program, Ecography, 12, 515–519, https://doi.org/10.1111/j.1600-0587.1989.tb00930.x, 1989.
Ballard, R. G., Walsh, M. A., and Cole, W. E.: Blue-stain fungi in xylem of lodgepole pine: a light-microscope study on extent of hyphal distribution, Can. J. Bot., 60, 2334–2341, https://doi.org/10.1139/b82-285, 1982.
Bentz, B. J., Régnière, J., Fettig, C. J., Hansen, E. M., Hayes, J. L., Hicke, J. A., Kelsey, R. G., Negrón, J. F., and Seybold, S. J.: Climate Change and Bark Beetles of the Western United States and Canada: Direct and Indirect Effects, BioScience, 60, 602–613, https://doi.org/10.1525/bio.2010.60.8.6, 2010.
Berner, L. T., Law, B. E., Meddens, A. J. H., and Hicke, J. A.: Tree mortality from fires, bark beetles, and timber harvest during a hot and dry decade in the western United States (2003–2012), Environ. Res. Lett., 12, 065005, https://doi.org/10.1088/1748-9326/aa6f94, 2017.
Berryman, A. A.: Population Cycles: The Case for Trophic Interactions, Oxford University Press, 207 pp., 2002.
Biedermann, P. H. W., Müller, J., Grégoire, J.-C., Gruppe, A., Hagge, J., Hammerbacher, A., Hofstetter, R. W., Kandasamy, D., Kolarik, M., Kostovcik, M., Krokene, P., Sallé, A., Six, D. L., Turrini, T., Vanderpool, D., Wingfield, M. J., and Bässler, C.: Bark Beetle Population Dynamics in the Anthropocene: Challenges and Solutions, Trends Ecol. Evol., 34, 914–924, https://doi.org/10.1016/j.tree.2019.06.002, 2019.
Boucher, O., Servonnat, J., Albright, A. L., Aumont, O., Balkanski, Y., Bastrikov, V., Bekki, S., Bonnet, R., Bony, S., Bopp, L., Braconnot, P., Brockmann, P., Cadule, P., Caubel, A., Cheruy, F., Codron, F., Cozic, A., Cugnet, D., D'Andrea, F., Davini, P., Lavergne, C. de, Denvil, S., Deshayes, J., Devilliers, M., Ducharne, A., Dufresne, J.-L., Dupont, E., Éthé, C., Fairhead, L., Falletti, L., Flavoni, S., Foujols, M.-A., Gardoll, S., Gastineau, G., Ghattas, J., Grandpeix, J.-Y., Guenet, B., Guez, L., E., Guilyardi, E., Guimberteau, M., Hauglustaine, D., Hourdin, F., Idelkadi, A., Joussaume, S., Kageyama, M., Khodri, M., Krinner, G., Lebas, N., Levavasseur, G., Lévy, C., Li, L., Lott, F., Lurton, T., Luyssaert, S., Madec, G., Madeleine, J.-B., Maignan, F., Marchand, M., Marti, O., Mellul, L., Meurdesoif, Y., Mignot, J., Musat, I., Ottlé, C., Peylin, P., Planton, Y., Polcher, J., Rio, C., Rochetin, N., Rousset, C., Sepulchre, P., Sima, A., Swingedouw, D., Thiéblemont, R., Traore, A. K., Vancoppenolle, M., Vial, J., Vialard, J., Viovy, N., and Vuichard, N.: Presentation and Evaluation of the IPSL-CM6A-LR Climate Model, J. Adv. Model. Earth Sy., 12, e2019MS002010, https://doi.org/10.1029/2019MS002010, 2020.
Bugmann, H. K. M.: A Simplified Forest Model to Study Species Composition Along Climate Gradients, Ecology, 77, 2055–2074, https://doi.org/10.2307/2265700, 1996.
Buma, B.: Disturbance interactions: characterization, prediction, and the potential for cascading effects, Ecosphere, 6, art70, https://doi.org/10.1890/ES15-00058.1, 2015.
Chapin, F. S., Woodwell, G. M., Randerson, J. T., Rastetter, E. B., Lovett, G. M., Baldocchi, D. D., Clark, D. A., Harmon, M. E., Schimel, D. S., Valentini, R., Wirth, C., Aber, J. D., Cole, J. J., Goulden, M. L., Harden, J. W., Heimann, M., Howarth, R. W., Matson, P. A., McGuire, A. D., Melillo, J. M., Mooney, H. A., Neff, J. C., Houghton, R. A., Pace, M. L., Ryan, M. G., Running, S. W., Sala, O. E., Schlesinger, W. H., and Schulze, E.-D.: Reconciling Carbon-cycle Concepts, Terminology, and Methods. Ecosystems 9, 1041–1050, https://doi.org/10.1007/s10021-005-0105-7, 2006.
Chen, Y., Ryder, J., Bastrikov, V., McGrath, M. J., Naudts, K., Otto, J., Ottlé, C., Peylin, P., Polcher, J., Valade, A., Black, A., Elbers, J. A., Moors, E., Foken, T., van Gorsel, E., Haverd, V., Heinesch, B., Tiedemann, F., Knohl, A., Launiainen, S., Loustau, D., Ogée, J., Vessala, T., and Luyssaert, S.: Evaluating the performance of land surface model ORCHIDEE-CAN v1.0 on water and energy flux estimation with a single- and multi-layer energy budget scheme, Geosci. Model Dev., 9, 2951–2972, https://doi.org/10.5194/gmd-9-2951-2016, 2016.
Chen, Y.-Y., Gardiner, B., Pasztor, F., Blennow, K., Ryder, J., Valade, A., Naudts, K., Otto, J., McGrath, M. J., Planque, C., and Luyssaert, S.: Simulating damage for wind storms in the land surface model ORCHIDEE-CAN (revision 4262), Geosci. Model Dev., 11, 771–791, https://doi.org/10.5194/gmd-11-771-2018, 2018.
Ciais, P., Reichstein, M., Viovy, N., Granier, A., Ogée, J., Allard, V., Aubinet, M., Buchmann, N., Bernhofer, C., Carrara, A., Chevallier, F., De Noblet, N., Friend, A. D., Friedlingstein, P., Grünwald, T., Heinesch, B., Keronen, P., Knohl, A., Krinner, G., Loustau, D., Manca, G., Matteucci, G., Miglietta, F., Ourcival, J. M., Papale, D., Pilegaard, K., Rambal, S., Seufert, G., Soussana, J. F., Sanz, M. J., Schulze, E. D., Vesala, T., and Valentini, R.: Europe-wide reduction in primary productivity caused by the heat and drought in 2003, Nature, 437, 529–533, https://doi.org/10.1038/nature03972, 2005.
Copernicus: European State of the Climate, https://climate.copernicus.eu/ESOTC, last access: 25 March 2024.
Cox, P. M., Betts, R. A., Jones, C. D., Spall, S. A., and Totterdell, I. J.: Acceleration of global warming due to carbon-cycle feedbacks in a coupled climate model, Nature, 408, 184–187, https://doi.org/10.1038/35041539, 2000.
Custer, G. F., van Diepen, L. T. A., and Stump, W. L.: Structural and Functional Dynamics of Soil Microbes following Spruce Beetle Infestation, Appl. Environ. Microbiol., 86, e01984-19, https://doi.org/10.1128/AEM.01984-19, 2020.
Das, A. J., Stephenson, N. L., and Davis, K. P.: Why do trees die? Characterizing the drivers of background tree mortality, Ecology, 97, 2616–2627, https://doi.org/10.1002/ecy.1497, 2016.
Deleuze, C., Pain, O., Dhôte, J.-F., and Hervé, J.-C.: A flexible radial increment model for individual trees in pure even-aged stands, Ann. For. Sci., 61, 327–335, https://doi.org/10.1051/forest:2004026, 2004.
Edburg, S. L., Hicke, J. A., Brooks, P. D., Pendall, E. G., Ewers, B. E., Norton, U., Gochis, D., Gutmann, E. D., and Meddens, A. J.: Cascading impacts of bark beetle-caused tree mortality on coupled biogeophysical and biogeochemical processes, Front. Ecol. Environ., 10, 416–424, https://doi.org/10.1890/110173, 2012.
Faccoli, M. and Stergulc, F.: A practical method for predicting the short-time trend of bivoltine populations of Ips typographus (L.) (Col., Scolytidae), J. Appl. Entomol., 130, 61–66, https://doi.org/10.1111/j.1439-0418.2005.01019.x, 2006.
Friedlingstein, P., Cox, P., Betts, R., Bopp, L., Bloh, W. von, Brovkin, V., Cadule, P., Doney, S., Eby, M., Fung, I., Bala, G., John, J., Jones, C., Joos, F., Kato, T., Kawamiya, M., Knorr, W., Lindsay, K., Matthews, H. D., Raddatz, T., Rayner, P., Reick, C., Roeckner, E., Schnitzler, K.-G., Schnur, R., Strassmann, K., Weaver, A. J., Yoshikawa, C., and Zeng, N.: Climate–Carbon Cycle Feedback Analysis: Results from the C4MIP Model Intercomparison, J. Climate, 19, 3337–3353, https://doi.org/10.1175/JCLI3800.1, 2006.
Grimm, V. and Wissel, C.: Babel, or the ecological stability discussions: an inventory and analysis of terminology and a guide for avoiding confusion, Oecologia, 109, 323–334, https://doi.org/10.1007/s004420050090, 1997.
Havašová, M., Ferenčík, J., and Jakuš, R.: Interactions between windthrow, bark beetles and forest management in the Tatra national parks, For. Ecol. Manag., 391, 349–361, https://doi.org/10.1016/j.foreco.2017.01.009, 2017.
Haverd, V., Lovell, J. L., Cuntz, M., Jupp, D. L. B., Newnham, G. J., and Sea, W.: The Canopy Semi-analytic Pgap And Radiative Transfer (CanSPART) model: Formulation and application, Agric. For. Meteorol., 160, 14–35, https://doi.org/10.1016/j.agrformet.2012.01.018, 2012.
Hicke, J. A., Allen, C. D., Desai, A. R., Dietze, M. C., Hall, R. J., Hogg, E. H., Kashian, D. M., Moore, D., Raffa, K. F., Sturrock, R. N., and Vogelmann, J.: Effects of biotic disturbances on forest carbon cycling in the United States and Canada, Glob. Change Biol., 18, 7–34, https://doi.org/10.1111/j.1365-2486.2011.02543.x, 2012.
Hlásny, T., König, L., Krokene, P., Lindner, M., Montagné-Huck, C., Müller, J., Qin, H., Raffa, K. F., Schelhaas, M.-J., Svoboda, M., Viiri, H., and Seidl, R.: Bark Beetle Outbreaks in Europe: State of Knowledge and Ways Forward for Management, Curr. For. Rep., 7, 138–165, https://doi.org/10.1007/s40725-021-00142-x, 2021a.
Hlásny, T., Zimová, S., Merganičová, K., Štěpánek, P., Modlinger, R., and Turčáni, M.: Devastating outbreak of bark beetles in the Czech Republic: Drivers, impacts, and management implications, For. Ecol. Manag., 490, 119075, https://doi.org/10.1016/j.foreco.2021.119075, 2021b.
Huang, J., Kautz, M., Trowbridge, A. M., Hammerbacher, A., Raffa, K. F., Adams, H. D., Goodsman, D. W., Xu, C., Meddens, A. J. H., Kandasamy, D., Gershenzon, J., Seidl, R., and Hartmann, H.: Tree defence and bark beetles in a drying world: carbon partitioning, functioning and modelling, New Phytol., 225, 26–36, https://doi.org/10.1111/nph.16173, 2020.
IPSL Data Catalog: ORCHIDEE_Bark_beetles_outbreak_gmd_2024, IPSL Data Catalog [code], https://doi.org/10.14768/08ca9318-b663-40e3-90e6-6baee0475ba0, 2024.
Jonášová, M. and Prach, K.: Central-European mountain spruce (Picea abies (L.) Karst.) forests: regeneration of tree species after a bark beetle outbreak, Ecol. Eng., 23, 15–27, 2004. https://doi.org/10.1016/j.ecoleng.2004.06.010
Jönsson, A. M., Appelberg, G., Harding, S., and Bärring, L.: Spatio-temporal impact of climate change on the activity and voltinism of the spruce bark beetle, Ips typographus, Glob. Change Biol., 15, 486–499, https://doi.org/10.1111/j.1365-2486.2008.01742.x, 2009.
Jönsson, A. M., Harding, S., Krokene, P., Lange, H., Lindelöw, Å., Økland, B., Ravn, H. P., and Schroeder, L. M.: Modelling the potential impact of global warming on Ips typographus voltinism and reproductive diapause, Clim. Change, 109, 695–718, https://doi.org/10.1007/s10584-011-0038-4, 2011.
Jönsson, A. M., Schroeder, L. M., Lagergren, F., Anderbrant, O., and Smith, B.: Guess the impact of Ips typographus – An ecosystem modelling approach for simulating spruce bark beetle outbreaks, Agric. For. Meteorol., 166–167, 188–200, https://doi.org/10.1016/j.agrformet.2012.07.012, 2012.
Kärvemo, S. and Schroeder, L. M.: A comparison of outbreak dynamics of the spruce bark beetle in Sweden and the mountain pine beetle in Canada (Curculionidae: Scolytinae) [En jämförelse av utbrottsdynamiken mellan granbarkborre i Sverige och contortabast-borre i Kanada (Curculionidae: Scolytinae)], Entomologisk Tidskrift, 131, 215–224, 2010.
Kautz, M., Anthoni, P., Meddens, A. J. H., Pugh, T. A. M., and Arneth, A.: Simulating the recent impacts of multiple biotic disturbances on forest carbon cycling across the United States, Glob. Change Biol., 24, 2079–2092, https://doi.org/10.1111/gcb.13974, 2018.
Komonen, A., Schroeder, L. M., and Weslien, J.: Ips typographus population development after a severe storm in a nature reserve in southern Sweden, J. Appl. Entomol., 135, 132–141, https://doi.org/10.1111/j.1439-0418.2010.01520.x, 2011.
Krinner, G., Viovy, N., de Noblet-Ducoudré, N., Ogée, J., Polcher, J., Friedlingstein, P., Ciais, P., Sitch, S., and Prentice, I. C.: A dynamic global vegetation model for studies of the coupled atmosphere-biosphere system: DVGM FOR COUPLED CLIMATE STUDIES, Global Biogeochem. Cy., 19, GB1015, https://doi.org/10.1029/2003GB002199, 2005.
Kurz, W. A., Dymond, C. C., Stinson, G., Rampley, G. J., Neilson, E. T., Carroll, A. L., Ebata, T., and Safranyik, L.: Mountain pine beetle and forest carbon feedback to climate change, Nature, 452, 987–990, https://doi.org/10.1038/nature06777, 2008a.
Kurz, W. A., Stinson, G., Rampley, G. J., Dymond, C. C., and Neilson, E. T.: Risk of natural disturbances makes future contribution of Canada's forests to the global carbon cycle highly uncertain, P. Natl. Acad. Sci. USA, 105, 1551–1555, https://doi.org/10.1073/pnas.0708133105, 2008b.
Lasslop, G., Thonicke, K., and Kloster, S.: SPITFIRE within the MPI Earth system model: Model development and evaluation, J. Adv. Model. Earth Sy., 6, 740–755, https://doi.org/10.1002/2013MS000284, 2014.
Lausch, A., Fahse, L., and Heurich, M.: Factors affecting the spatio-temporal dispersion of Ips typographus (L.) in Bavarian Forest National Park: A long-term quantitative landscape-level analysis, For. Ecol. Manag., 261, 233–245, https://doi.org/10.1016/j.foreco.2010.10.012, 2011.
Levins, R.: The Strategy of Model Building in Population Biology, Am. Sci., 54, 421–431, 1966.
Lieutier, F.: Mechanisms of Resistance in Conifers and Bark beetle Attack Strategies, in: Mechanisms and Deployment of Resistance in Trees to Insects, edited by: Wagner, M. R., Clancy, K. M., Lieutier, F., and Paine, T. D., Springer Netherlands, Dordrecht, 31–77, https://doi.org/10.1007/0-306-47596-0_2, 2002.
Lombardero, M. J., Ayres, M. P., Ayres, B. D., and Reeve, J. D.: Cold Tolerance of Four Species of Bark Beetle (Coleoptera: Scolytidae) in North America, Environ. Entomol., 29, 421–432, https://doi.org/10.1603/0046-225X-29.3.421, 2000.
Luyssaert, S., Marie, G., Valade, A., Chen, Y.-Y., Njakou Djomo, S., Ryder, J., Otto, J., Naudts, K., Lansø, A. S., Ghattas, J., and McGrath, M. J.: Trade-offs in using European forests to meet climate objectives, Nature, 562, 259–262, https://doi.org/10.1038/s41586-018-0577-1, 2018.
Marie, G.: Bark Beetle outbreak module dev into ORCHIDEE – simulation results and quantitative evaluation, Zenodo [code and data set], https://doi.org/10.5281/zenodo.12806280, 2024.
Marini, L., Økland, B., Jönsson, A. M., Bentz, B., Carroll, A., Forster, B., Grégoire, J.-C., Hurling, R., Nageleisen, L. M., Netherer, S., Ravn, H. P., Weed, A., and Schroeder, M.: Climate drivers of bark beetle outbreak dynamics in Norway spruce forests, Ecography, 40, 1426–1435, https://doi.org/10.1111/ecog.02769, 2017.
Mezei, P., Grodzki, W., Blaženec, M., and Jakuš, R.: Factors influencing the wind–bark beetles' disturbance system in the course of an Ips typographus outbreak in the Tatra Mountains, For. Ecol. Manag., 312, 67–77, https://doi.org/10.1016/j.foreco.2013.10.020, 2014.
Mezei, P., Jakuš, R., Pennerstorfer, J., Havašová, M., Škvarenina, J., Ferenčík, J., Slivinský, J., Bičárová, S., Bilčík, D., Blaženec, M., and Netherer, S.: Storms, temperature maxima and the Eurasian spruce bark beetle Ips typographus – An infernal trio in Norway spruce forests of the Central European High Tatra Mountains, Agric. For. Meteorol., 242, 85–95, https://doi.org/10.1016/j.agrformet.2017.04.004, 2017.
Migliavacca, M., Dosio, A., Kloster, S., Ward, D. S., Camia, A., Houborg, R., Houston Durrant, T., Khabarov, N., Krasovskii, A. A., San Miguel-Ayanz, J., and Cescatti, A.: Modeling burned area in Europe with the Community Land Model, J. Geophys. Res.-Biogeo., 118, 265–279, https://doi.org/10.1002/jgrg.20026, 2013.
Migliavacca, M., Musavi, T., Mahecha, M. D., Nelson, J. A., Knauer, J., Baldocchi, D. D., Perez-Priego, O., Christiansen, R., Peters, J., Anderson, K., Bahn, M., Black, T. A., Blanken, P. D., Bonal, D., Buchmann, N., Caldararu, S., Carrara, A., Carvalhais, N., Cescatti, A., Chen, J., Cleverly, J., Cremonese, E., Desai, A. R., El-Madany, T. S., Farella, M. M., Fernández-Martínez, M., Filippa, G., Forkel, M., Galvagno, M., Gomarasca, U., Gough, C. M., Göckede, M., Ibrom, A., Ikawa, H., Janssens, I. A., Jung, M., Kattge, J., Keenan, T. F., Knohl, A., Kobayashi, H., Kraemer, G., Law, B. E., Liddell, M. J., Ma, X., Mammarella, I., Martini, D., Macfarlane, C., Matteucci, G., Montagnani, L., Pabon-Moreno, D. E., Panigada, C., Papale, D., Pendall, E., Penuelas, J., Phillips, R. P., Reich, P. B., Rossini, M., Rotenberg, E., Scott, R. L., Stahl, C., Weber, U., Wohlfahrt, G., Wolf, S., Wright, I. J., Yakir, D., Zaehle, S., and Reichstein, M.: The three major axes of terrestrial ecosystem function, Nature, 598, 468–472, https://doi.org/10.1038/s41586-021-03939-9, 2021.
Millar, C. I. and Stephenson, N. L.: Temperate forest health in an era of emerging megadisturbance, Science, 349, 823–826, https://doi.org/10.1126/science.aaa9933, 2015.
Morehouse, K., Johns, T., Kaye, J., and Kaye, M.: Carbon and nitrogen cycling immediately following bark beetle outbreaks in southwestern ponderosa pine forests, For. Ecol. Manag., 255, 2698–2708, https://doi.org/10.1016/j.foreco.2008.01.050, 2008.
Nardi, D., Jactel, H., Pagot, E., Samalens, J.-C., and Marini, L.: Drought and stand susceptibility to attacks by the European spruce bark beetle: A remote sensing approach, Agric. For. Entomol., 25, 119–129, https://doi.org/10.1111/afe.12536, 2023.
Naudts, K., Ryder, J., McGrath, M. J., Otto, J., Chen, Y., Valade, A., Bellasen, V., Berhongaray, G., Bönisch, G., Campioli, M., Ghattas, J., De Groote, T., Haverd, V., Kattge, J., MacBean, N., Maignan, F., Merilä, P., Penuelas, J., Peylin, P., Pinty, B., Pretzsch, H., Schulze, E. D., Solyga, D., Vuichard, N., Yan, Y., and Luyssaert, S.: A vertically discretised canopy description for ORCHIDEE (SVN r2290) and the modifications to the energy, water and carbon fluxes, Geosci. Model Dev., 8, 2035–2065, https://doi.org/10.5194/gmd-8-2035-2015, 2015.
Naudts, K., Chen, Y., McGrath, M. J., Ryder, J., Valade, A., Otto, J., and Luyssaert, S.: Europe's forest management did not mitigate climate warming, Science, 351, 597–600, https://doi.org/10.1126/science.aad7270, 2016.
Netherer, S., Matthews, B., Katzensteiner, K., Blackwell, E., Henschke, P., Hietz, P., Pennerstorfer, J., Rosner, S., Kikuta, S., Schume, H., and Schopf, A.: Do water-limiting conditions predispose Norway spruce to bark beetle attack?, New Phytol., 205, 1128–1141, https://doi.org/10.1111/nph.13166, 2015.
Ogris, N., Ferlan, M., Hauptman, T., Pavlin, R., Kavčič, A., Jurc, M., and de Groot, M.: RITY – A phenology model of Ips typographus as a tool for optimization of its monitoring, Ecol. Model., 410, 108775, https://doi.org/10.1016/j.ecolmodel.2019.108775, 2019.
Pastorello, G., Trotta, C., Canfora, E., Chu, H., Christianson, D., Cheah, Y.-W., Poindexter, C., Chen, J., Elbashandy, A., Humphrey, M., Isaac, P., Polidori, D., Reichstein, M., Ribeca, A., van Ingen, C., Vuichard, N., Zhang, L., Amiro, B., Ammann, C., Arain, M. A., Ardö, J., Arkebauer, T., Arndt, S. K., Arriga, N., Aubinet, M., Aurela, M., Baldocchi, D., Barr, A., Beamesderfer, E., Marchesini, L. B., Bergeron, O., Beringer, J., Bernhofer, C., Berveiller, D., Billesbach, D., Black, T. A., Blanken, P. D., Bohrer, G., Boike, J., Bolstad, P. V., Bonal, D., Bonnefond, J.-M., Bowling, D. R., Bracho, R., Brodeur, J., Brümmer, C., Buchmann, N., Burban, B., Burns, S. P., Buysse, P., Cale, P., Cavagna, M., Cellier, P., Chen, S., Chini, I., Christensen, T. R., Cleverly, J., Collalti, A., Consalvo, C., Cook, B. D., Cook, D., Coursolle, C., Cremonese, E., Curtis, P. S., D'Andrea, E., da Rocha, H., Dai, X., Davis, K. J., Cinti, B. D., Grandcourt, A. de, Ligne, A. D., De Oliveira, R. C., Delpierre, N., Desai, A. R., Di Bella, C. M., Tommasi, P. di, Dolman, H., Domingo, F., Dong, G., Dore, S., Duce, P., Dufrêne, E., Dunn, A., Dušek, J., Eamus, D., Eichelmann, U., ElKhidir, H. A. M., Eugster, W., Ewenz, C. M., Ewers, B., Famulari, D., Fares, S., Feigenwinter, I., Feitz, A., Fensholt, R., Filippa, G., Fischer, M., Frank, J., Galvagno, M., et al.: The FLUXNET2015 dataset and the ONEFlux processing pipeline for eddy covariance data, Sci. Data, 7, 225, https://doi.org/10.1038/s41597-020-0534-3, 2020.
Pasztor, F., Matulla, C., Rammer, W., and Lexer, M. J.: Drivers of the bark beetle disturbance regime in Alpine forests in Austria, For. Ecol. Manag., 318, 349–358, https://doi.org/10.1016/j.foreco.2014.01.044, 2014.
Pfeifer, E. M., Hicke, J. A., and Meddens, A. J. H.: Observations and modeling of aboveground tree carbon stocks and fluxes following a bark beetle outbreak in the western United States, Glob. Change Biol., 17, 339–350, https://doi.org/10.1111/j.1365-2486.2010.02226.x, 2011.
Pineau, X., David, G., Peter, Z., Sallé, A., Baude, M., Lieutier, F., and Jactel, H.: Effect of temperature on the reproductive success, developmental rate and brood characteristics of Ips sexdentatus (Boern.), Agric. For. Entomol., 19, 23–33, https://doi.org/10.1111/afe.12177, 2017.
Preisler, H. K., Hicke, J. A., Ager, A. A., and Hayes, J. L.: Climate and weather influences on spatial temporal patterns of mountain pine beetle populations in Washington and Oregon, Ecology, 93, 2421–2434, https://doi.org/10.1890/11-1412.1, 2012.
Pugh, T. A. M., Jones, C. D., Huntingford, C., Burton, C., Arneth, A., Brovkin, V., Ciais, P., Lomas, M., Robertson, E., and Piao, S. L.: A Large Committed Long-Term Sink of Carbon due to Vegetation Dynamics, Earths Future, 6, 1413–1432, 2017.
Quillet, A., Peng, C., and Garneau, M.: Toward dynamic global vegetation models for simulating vegetation–climate interactions and feedbacks: recent developments, limitations, and future challenges, Environ. Rev., 18, 333–353, https://doi.org/10.1139/A10-016, 2010.
Raffa, K. F., Aukema, B. H., Bentz, B. J., Carroll, A. L., Hicke, J. A., Turner, M. G., and Romme, W. H.: Cross-scale Drivers of Natural Disturbances Prone to Anthropogenic Amplification: The Dynamics of Bark Beetle Eruptions, BioScience, 58, 501–517, https://doi.org/10.1641/B580607, 2008.
Rhoades, C. C.: Soil Nitrogen Leaching in Logged Beetle-Killed Forests and Implications for Riparian Fuel Reduction, J. Environ. Qual., 48, 305–313, https://doi.org/10.2134/jeq2018.04.0169, 2019.
Ryder, J., Polcher, J., Peylin, P., Ottlé, C., Chen, Y., van Gorsel, E., Haverd, V., McGrath, M. J., Naudts, K., Otto, J., Valade, A., and Luyssaert, S.: A multi-layer land surface energy budget model for implicit coupling with global atmospheric simulations, Geosci. Model Dev., 9, 223–245, https://doi.org/10.5194/gmd-9-223-2016, 2016.
Schlyter, F., Birgersson, G., and Leufvén, A.: Inhibition of attraction to aggregation pheromone by verbenone and ipsenol, J. Chem. Ecol., 15, 2263–2277, https://doi.org/10.1007/BF01014114, 1989.
Schumacher, S.: The role of large-scale disturbances and climate for the dynamics of forested landscapes in the European Alps, Doctoral Thesis, ETH Zurich, https://doi.org/10.3929/ethz-a-004818825, 2004.
Seidl, R. and Rammer, W.: Climate change amplifies the interactions between wind and bark beetle disturbances in forest landscapes, Landsc. Ecol., 32, 1485–1498, https://doi.org/10.1007/s10980-016-0396-4, 2016.
Seidl, R., Fernandes, P. M., Fonseca, T. F., Gillet, F., Jönsson, A. M., Merganičová, K., Netherer, S., Arpaci, A., Bontemps, J.-D., Bugmann, H., González-Olabarria, J. R., Lasch, P., Meredieu, C., Moreira, F., Schelhaas, M.-J., and Mohren, F.: Modelling natural disturbances in forest ecosystems: a review, Ecol. Model., 222, 903–924, https://doi.org/10.1016/j.ecolmodel.2010.09.040, 2011.
Seidl, R., Schelhaas, M.-J., Rammer, W., and Verkerk, P. J.: Increasing forest disturbances in Europe and their impact on carbon storage, Nat. Clim. Change, 4, 806–810, https://doi.org/10.1038/nclimate2318, 2014.
Seidl, R., Thom, D., Kautz, M., Martin-Benito, D., Peltoniemi, M., Vacchiano, G., Wild, J., Ascoli, D., Petr, M., Honkaniemi, J., Lexer, M. J., Trotsiuk, V., Mairota, P., Svoboda, M., Fabrika, M., Nagel, T. A., and Reyer, C. P. O.: Forest disturbances under climate change, Nat. Clim. Change, 7, 395–402, https://doi.org/10.1038/nclimate3303, 2017.
Seidl, R., Klonner, G., Rammer, W., Essl, F., Moreno, A., Neumann, M., and Dullinger, S.: Invasive alien pests threaten the carbon stored in Europe's forests, Nat. Commun., 9, 1626, https://doi.org/10.1038/s41467-018-04096-w, 2018.
Senf, C., Pflugmacher, D., Hostert, P., and Seidl, R.: Using Landsat time series for characterizing forest disturbance dynamics in the coupled human and natural systems of Central Europe, ISPRS J. Photogramm. Remote Sens., 130, 453–463, https://doi.org/10.1016/j.isprsjprs.2017.07.004, 2017.
Storaunet, K. O., Rolstad, J., Gjerde, I., and Gundersen, V. S.:Historical logging, productivity, and structural characteristics of boreal coniferous forests in Norway. Silva Fenn., 39, 429–442, 2005.
Temperli, C., Bugmann, H., and Elkin, C.: Cross-scale interactions among bark beetles, climate change, and wind disturbances: a landscape modeling approach, Ecol. Monogr., 83, 383–402, https://doi.org/10.1890/12-1503.1, 2013.
Thurner, M., Beer, C., Ciais, P., Friend, A. D., Ito, A., Kleidon, A., Lomas, M. R., Quegan, S., Rademacher, T. T., Schaphoff, S., Tum, M., Wiltshire, A., and Carvalhais, N.: Evaluation of climate-related carbon turnover processes in global vegetation models for boreal and temperate forests, Glob. Change Biol., 23, 3076–3091, https://doi.org/10.1111/gcb.13660, 2017.
Van Meerbeek, K., Jucker, T., and Svenning, J.-C.: Unifying the concepts of stability and resilience in ecology, J. Ecol., 109, 3114–3132, https://doi.org/10.1111/1365-2745.13651, 2021.
Vuichard, N., Messina, P., Luyssaert, S., Guenet, B., Zaehle, S., Ghattas, J., Bastrikov, V., and Peylin, P.: Accounting for carbon and nitrogen interactions in the global terrestrial ecosystem model ORCHIDEE (trunk version, rev 4999): multi-scale evaluation of gross primary production, Geosci. Model Dev., 12, 4751–4779, https://doi.org/10.5194/gmd-12-4751-2019, 2019.
Wermelinger, B.: Ecology and management of the spruce bark beetle Ips typographus – a review of recent research, For. Ecol. Manag., 202, 67–82, https://doi.org/10.1016/j.foreco.2004.07.018, 2004.
Wichmann, L. and Ravn, H. P.: The spread of Ips typographus (L.) (Coleoptera, Scolytidae) attacks following heavy windthrow in Denmark, analysed using GIS, For. Ecol. Manag., 148, 31–39, https://doi.org/10.1016/S0378-1127(00)00477-1, 2001.
Yao, Y., Joetzjer, E., Ciais, P., Viovy, N., Cresto Aleina, F., Chave, J., Sack, L., Bartlett, M., Meir, P., Fisher, R., and Luyssaert, S.: Forest fluxes and mortality response to drought: model description (ORCHIDEE-CAN-NHA r7236) and evaluation at the Caxiuanã drought experiment, Geosci. Model Dev., 15, 7809–7833, https://doi.org/10.5194/gmd-15-7809-2022, 2022.
Yue, C., Ciais, P., Cadule, P., Thonicke, K., Archibald, S., Poulter, B., Hao, W. M., Hantson, S., Mouillot, F., Friedlingstein, P., Maignan, F., and Viovy, N.: Modelling the role of fires in the terrestrial carbon balance by incorporating SPITFIRE into the global vegetation model ORCHIDEE – Part 1: simulating historical global burned area and fire regimes, Geosci. Model Dev., 7, 2747–2767, https://doi.org/10.5194/gmd-7-2747-2014, 2014.
Zaehle, S. and Dalmonech, D.: Carbon–nitrogen interactions on land at global scales: current understanding in modelling climate biosphere feedbacks, Curr. Opin. Environ. Sustain., 3, 311–320, https://doi.org/10.1016/j.cosust.2011.08.008, 2011.
Zaehle, S. and Friend, A. D.: Carbon and nitrogen cycle dynamics in the O-CN land surface model: 1. Model description, site-scale evaluation, and sensitivity to parameter estimates, Global Biogeochem. Cy., 24, GB1005, https://doi.org/10.1029/2009GB003521, 2010.
Zhang, Q.-H. and Schlyter, F.: Olfactory recognition and behavioural avoidance of angiosperm nonhost volatiles by conifer-inhabiting bark beetles, Agric. For. Entomol., 6, 1–20, https://doi.org/10.1111/j.1461-9555.2004.00202.x, 2004.
Zscheischler, J., Westra, S., van den Hurk, B. J. J. M., Seneviratne, S. I., Ward, P. J., Pitman, A., AghaKouchak, A., Bresch, D. N., Leonard, M., Wahl, T., and Zhang, X.: Future climate risk from compound events, Nat. Clim. Change, 8, 469–477, https://doi.org/10.1038/s41558-018-0156-3, 2018.