the Creative Commons Attribution 4.0 License.
the Creative Commons Attribution 4.0 License.
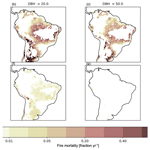
Dynamic ecosystem assembly and escaping the “fire trap” in the tropics: insights from FATES_15.0.0
Jacquelyn K. Shuman
Rosie A. Fisher
Charles Koven
Ryan Knox
Lara Kueppers
Chonggang Xu
Fire is a fundamental part of the Earth system, with impacts on vegetation structure, biomass, and community composition, the latter mediated in part via key fire-tolerance traits, such as bark thickness. Due to anthropogenic climate change and land use pressure, fire regimes are changing across the world, and fire risk has already increased across much of the tropics. Projecting the impacts of these changes at global scales requires that we capture the selective force of fire on vegetation distribution through vegetation functional traits and size structure. We have adapted the fire behavior and effects module, SPITFIRE (SPread and InTensity of FIRE), for use with the Functionally Assembled Terrestrial Ecosystem Simulator (FATES), a size-structured vegetation demographic model. We test how climate, fire regime, and fire-tolerance plant traits interact to determine the biogeography of tropical forests and grasslands. We assign different fire-tolerance strategies based on crown, leaf, and bark characteristics, which are key observed fire-tolerance traits across woody plants. For these simulations, three types of vegetation compete for resources: a fire-vulnerable tree with thin bark, a vulnerable deep crown, and fire-intolerant foliage; a fire-tolerant tree with thick bark, a thin crown, and fire-tolerant foliage; and a fire-promoting C4 grass. We explore the model sensitivity to a critical parameter governing fuel moisture and show that drier fuels promote increased burning, an expansion of area for grass and fire-tolerant trees, and a reduction of area for fire-vulnerable trees. This conversion to lower biomass or grass areas with increased fuel drying results in increased fire-burned area and its effects, which could feed back to local climate variables. Simulated size-based fire mortality for trees less than 20 cm in diameter and those with fire-vulnerable traits is higher than that for larger and/or fire-tolerant trees, in agreement with observations. Fire-disturbed forests demonstrate reasonable productivity and capture observed patterns of aboveground biomass in areas dominated by natural vegetation for the recent historical period but have a large bias in less disturbed areas. Though the model predicts a greater extent of burned fraction than observed in areas with grass dominance, the resulting biogeography of fire-tolerant, thick-bark trees and fire-vulnerable, thin-bark trees corresponds to observations across the tropics. In areas with more than 2500 mm of precipitation, simulated fire frequency and burned area are low, with fire intensities below 150 kW m−1, consistent with observed understory fire behavior across the Amazon. Areas drier than this demonstrate fire intensities consistent with those measured in savannas and grasslands, with high values up to 4000 kW m−1. The results support a positive grass–fire feedback across the region and suggest that forests which have existed without frequent burning may be vulnerable at higher fire intensities, which is of greater concern under intensifying climate and land use pressures. The ability of FATES to capture the connection between fire disturbance and plant fire-tolerance strategies in determining biogeography provides a useful tool for assessing the vulnerability and resilience of these critical carbon storage areas under changing conditions across the tropics.
- Article
(6919 KB) - Full-text XML
-
Supplement
(7020 KB) - BibTeX
- EndNote
Fire is a fundamental component of the Earth system, with a diversity of global fire regimes playing a role in determining vegetation distribution, composition and structure, and carbon storage (Pausas and Keeley, 2014; McLauchlan et al., 2020). Recent decades show changing fire conditions with increases in fire season length (Jolly et al., 2015; Jones et al., 2022), driven largely by hotter and drier conditions (Jain et al., 2022). It is expected that these changes will continue with rising greenhouse gas emissions, leading to further elevation in fire risk (Touma et al., 2021). The projection of fire under changing climate and CO2 conditions is challenging (Hantson et al., 2020) due to the many drivers of fire (climate, fuel properties, land management, anthropogenic activities) that are simultaneously evolving. For tropical forests in particular (Cochrane, 2003; Cochrane et al., 1999; Nepstad et al., 2008; Nobre et al., 2016), these increasing drivers represent an emergence of conditions that have no observable analog in the present day. Thus, while purely data-driven approaches can help inform how combinations of drivers affect fire behavior (Haas et al., 2022), we must rely on (and improve) process-based models to effectively project these rapidly changing fire regimes and their effects.
In many land surface models – the terrestrial components of Earth system models (Blyth et al., 2021) – fire is represented as a function of fuel availability and dryness, climate conditions, and human activity (Rabin et al., 2017). Some models represent fire-induced plant mortality using constant combustion and mortality factors to determine the portion of vegetation burned or killed (Rabin et al., 2017). A small set of land models represent tree mortality from fire as a function of tree size and potentially other vegetation factors. Among six land surface models that consider tree mortality from fire based on tree size, four include bark thickness as determined by tree size as a factor, one considers bark thickness as a factor irrespective of tree size, and one does not consider bark thickness (Rabin et al., 2017). Most use the common land surface model area-averaged representation of each type of plant within a given location, which is not able to capture natural ecosystem heterogeneity or demography and potential feedback between vegetation structure and fire behavior (Fisher et al., 2018). Most land surface models, however, do not resolve both the size distribution of plants and variation in fire-tolerance traits, omissions with potentially important implications. First, smaller trees and grasses are more prone to direct consumption by fire, while larger trees can place more of their branches and leaves clear of flames that are produced by surface fires. Second, tree mortality from fire of a given intensity and duration is also known to be a strong function of bark thickness (Hoffmann et al., 2003, 2012; Hoffmann and Solbrig, 2003). Bark thickness varies as a function of tree size as well as tree type, and both size and bark investment determine the survival of trees or stems during fire (Balch et al., 2008; Hoffmann and Solbrig, 2003; Hoffmann et al., 2012; Pellegrini et al., 2017). Size-dependent mortality gives rise to the concept of the “fire trap”, upon which many aspects of fire ecology are thought to depend (Bond, 2008; Ryan and Williams, 2011; Hoffmann et al., 2020), including bimodal size distributions, and the selection for thick-barked tree species under frequent fire regimes (Pellegrini et al., 2017). The latter implies that in areas where fire is rare, the absence of selection for thick bark will mean that trees are more vulnerable to mortality under a given set of fire conditions. Thus, models that do not differentiate plant types based on their size and tolerance of fire (expressed via bark thickness and canopy characteristics) may not capture these dynamics.
Here we describe the implementation of the process-based fire module, SPITFIRE (SPread and InTensity of FIRE; Thonicke et al., 2010), into the vegetation demographic model, FATES (the Functionally Assembled Terrestrial Ecosystem Simulator; Fisher et al., 2015; Koven et al., 2020), and explore how fire activity and vegetation–fire feedbacks influence ecosystem composition across tropical South America using hypothetical fuel drying scenarios. FATES is one of a class of demographic models presently being implemented in Earth system models (Fisher et al., 2018; Naudts et al., 2015; Chen et al., 2018; Haverd et al., 2018). It captures heterogeneity of plant size by tracking populations of co-occurring plants using a set of “cohorts” that recruit, grow in size, and die through time on a discrete set of “patches” which vary in age since disturbance and collectively track succession following canopy mortality events. The fire module SPITFIRE (SPread and InTensity of FIRE) (Thonicke et al., 2010), which is already used in other land surface schemes (Rabin et al., 2017) and incorporates size-dependent mortality algorithms, is implemented within FATES and modified to facilitate the interaction between the size- and age-structured vegetation. To test the influence of fire on ecosystem assembly, we use FATES to simulate the distribution of forest and grass under multiple fuel drying conditions, evaluating size-dependent mortality and associated fire behavior and effects. The results of these simulations provide insight into the extent to which fire feedbacks regulate ecosystem assembly.
2.1 The integrated vegetation–fire model FATES–SPITFIRE
FATES–SPITFIRE has been integrated into the land models of both the Community Earth System Model (CESM, Danabasoglu et al., 2020) and the Energy Exascale Earth System Model (E3SM, Golaz et al., 2019) (the Community Land Model and E3SM Land Model – CLM and ELM, respectively). This study used FATES within the CLM to develop the climate–fire–vegetation interactions and feedbacks at regional scale. Similar to many land surface models, the default CLM wildfire scheme does not consider size cohorts and evaluates fire impact on vegetation and the carbon cycle as a weighted fraction of the fractional coverage of vegetation within the grid cell with fire altering the biomass and area of each vegetation type. The use of FATES allows for the inclusion of size- and age-structured vegetation and consideration of differential size-dependent mortality and associated fire behavior and effects.
2.1.1 FATES
We use FATES (version: ctsm5.1.dev036-fates_api15.0.0_crown_scorch_damage with git hash version number ff1ae2c2-a3b92952), which has been described most recently by Koven et al. (2020), based on the initial description by Fisher et al. (2015, 2010). Recent applications of FATES include investigation of vegetation dynamics in western US ecosystems in the presence of fire (Buotte et al., 2021). In FATES, patches are used to represent a fraction of potentially vegetated area consisting of all parts of the ecosystem with a similar disturbance history and can therefore be thought of as “time since disturbance” where a given patch can contain cohorts which vary in physical attributes in height and spatial position. FATES allows disturbance through three processes: (1) mortality of canopy trees, (2) fire, and (3) anthropogenic disturbance. With canopy tree mortality, some fraction of crown area of dead trees is used to generate newly disturbed patch area and the remainder stays in the existing patch. This paper includes disturbance mortality due to canopy tree mortality and fire, but not anthropogenic factors. The model code used here for the non-fire elements of this version of FATES is consistent with that documented in Koven et al. (2020).
2.1.2 SPITFIRE
The process-based fire behavior and effects module SPITFIRE (Spread and InTensity of FIRE; Thonicke et al., 2010) is implemented in multiple vegetation models (e.g., Lasslop et al., 2014; Yue et al., 2014; Drüke et al., 2019), with complete technical details for this implementation found in Sect. S3 in the Supplement. In FATES, the SPITFIRE module operates at a daily time step and separately for each patch to allow for sub-grid representation of different litter pools and vegetation characteristics according to the FATES patch structure. SPITFIRE simulates fires through calculation of fire danger, ignition, behavior, and effects for live and dead vegetation fuels. Here we review the structure of the SPITFIRE module and introduce modifications specific to its implementation in FATES.
Ignitions and fire danger
Within FATES–SPITFIRE, anthropogenic ignitions and natural lightning strikes are both potential sources of ignition. Lightning strikes are prescribed by a lightning forcing dataset derived from the NASA LIS/OTD Gridded Climatology (https://ghrc.nsstc.nasa.gov/pub/lis/climatology/, last access: 28 May 2024) as also used in Li et al. (2013), assuming that a percentage of these strikes reach the ground to result in lightning-driven potential ignitions (Ilightning) (). For this study the percentage of cloud-to-ground lightning strikes that have the potential to cause burning is set at 10 % (Latham and Williams, 2001). In this study due to the focus on natural fire–vegetation feedbacks, anthropogenic ignitions (Ianthro) were not used and instead set to zero. When in use, anthropogenic ignitions () are calculated according to Li et al. (2012), with details included in Sect. S3 in the Supplement. Fire duration (Fdur) is calculated as a function of the fire danger index (FDI) with a maximum daily duration of 240 min (Thonicke et al., 2010). FDI, a representation of the effect of meteorological conditions on the likelihood of a fire, is computed daily by using the Nesterov index (NI) per Venevsky et al. (2002), which is a cumulative function of daily temperature (T) and dew point (Dew) that resets to zero when total precipitation exceeds 3.0 mm. See Sect. S3 in the Supplement for further details.
Here a=0.00037 per Venevsky et al. (2002).
Characteristics of fuel
The rate of spread, fire intensity, and fuel combustion are determined based on multiple fuel conditions: fuel loading (w, kg m−2), bulk density (BD) (kg m−3), surface area to volume ratio (SAVfc) (cm−1), moisture (moistfc) (m3 m−3), and moisture of extinction (moistext,fc) (m3 m−3), the moisture content at which fuel no longer burns. Weighted averages across fuel classes (fc) are calculated for each of these variables. Total fuel load (Fpatch) (kg m−2) is the sum of the aboveground coarse woody debris (CWDAG,fc), leaf litter (llitter), and live grass biomass (bl,grass). As in Thonicke et al. (2010), fuels are separated into multiple classes. Dead woody fuels are grouped according to diameter ranges associated with a time lag that defines the time necessary for the loss of initial moisture to attain an equilibrium moisture content (Bradshaw et al., 1984) per the methods of Rothermel (1983) and Fosberg and Deeming (1971). According to this relationship, these dead woody fuels are categorized by their diameter as 1 h for fuels less than 0.6 cm, 10 h for fuels between 0.6 and 2.5 cm, 100 h for those between 2.5 and 7.6 cm, and 1000 h for fuels greater than 7.6 cm (Bradshaw et al., 1984). A fraction of simulated biomass following tree mortality is partitioned to each of these classes as set in the parameter file (fates_frag_cwd_frac), which for this paper uses 0.045, 0.075, 0.21, and 0.67 for the 1, 10, 100, and 1000 h fuels, respectively. Fine and woody fuels accumulate according to litterfall and size-differentiated mortality inputs produced by FATES and temperature- and moisture-sensitive litter decomposition within CLM (Lawrence et al., 2019). The rates of decomposition transfer for fuels were updated for the 1, 10, and 100 h fuels according to Eaton and Lawrence (2006), 1000 h fuels per Chambers et al. (2000), and dead leaves per Thonicke et al. (2010) (Table 1). The impact of 1000 h fuels on mean fuel properties is not considered in rate of spread or fire intensity equations, but they can be combusted during a fire.
Table 1Fuel class characteristics used in the parameter file for this study. Bulk density for dead leaves from Andrews (2018) and for live grass from Snell (1979); other values from Thonicke et al. (2010). 1 h fuel minimum and mid-moisture thresholds as well as the low-moisture coefficient are derived from Peterson and Ryan (1986).
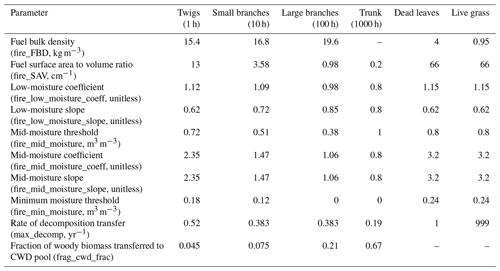
Dead fuel moisture (moistfc) is calculated as
where fc indicates “fuel class” and SAVfc is the fuel class surface area to volume ratio (cm−1), which includes the water and dry fuel by fuel class. The drying ratio represents a parameterizable value used to calculate the relative fuel moisture for a particular fuel type's surface area to volume. Live grass fuel moisture (moistl,grass) is calculated as
where rel_fmfc indicates the relative fuel moisture rate of drying of the fuel classes. Lower drying ratio values are associated with more rapid drying and lower relative moisture (Fig. S1 in the Supplement), which in turn impacts fuel combustion (Fig. S2 in the Supplement). The moisture of extinction, the moisture content (m3 m−3) at which fuel can no longer burn, is calculated as in Peterson and Ryan (1986).
Effective fuel moisture is then the ratio of fuel moisture moistfc to moistext,fc and is used to determine the combustion completeness. Fuel-specific consumption threshold parameters for the 1 h fuels are updated from Thonicke et al. (2010) with modifications to the minimum and mid-moisture thresholds as well as the low-moisture coefficient derived from Peterson and Ryan (1986) to remove a drop in combustion completeness at mid-moisture levels (Table 1, Fig. S2).
Here , , , and are fuel-type-specific parameters, and mmin,fc and midmoist are the fuel-specific thresholds for relative moisture content. Fuel-specific consumption FCfc is summed to calculate the overall FCpatch.
Rate of spread
Once an ignition event occurs, the potential forward rate of spread (ROSf) (m min−1) is calculated as in Thonicke et al. (2010) per the equations of Rothermel (1972):
where Ir is the reaction intensity (kJ m2 min−1) and represents the energy release per unit area of the fire front; xi is the propagation flux ratio and represents the proportion of Ir that heats fuel particles to ignition; θw is a wind factor; ε is the effective heating number and represents the number of particles heated to ignition temperature; Qign is the heat of pre-ignition (kJ kg−1), which is the amount of heat required to ignite a given mass of fuel, and BDpatch is a weighted average of bulk density across the fuel classes in that patch that are available for burning.
Fire intensity and area burned
The surface fire intensity (Isurf) (kW m−1) is then calculated as in Thonicke et al. (2010):
where h (kJ kg−1) is the heat content of fuel set to a default value of 18 000 kJ kg−1 and FCpatch (kg m−2) is the overall fuel consumption from the fire. Fires with a surface intensity below a user-defined minimum energy threshold cannot be sustained and are extinguished. The default value for this threshold is 50 kW m−1 per Peterson and Ryan (1986) and Thonicke et al. (2010). For this study, the minimum energy threshold for sustained burning was set to 25 kW m−1 for sites where the tree canopy cover is less than or equal to a 55 % threshold for savanna (Staver et al., 2011) and 100 kW m−1 for areas above this tree cover threshold based on fire intensity measurements for savanna (Govender et al., 2006) and neotropical forests (Brando et al., 2016).
The total area burned is assumed to be in the shape of an ellipse, with the major axis determined by the forward and backward rates of spread (ROSf and ROSb, respectively).
ROSb is a function of ROSf and wind speed (W).
The major axis to minor axis ratio, or length to breadth ratio (lb) (m), of the ellipse is determined by the wind speed. If W is less than 16.67 m min−1 (i.e., 1 km h−1) then lb=1. Otherwise, lb is calculated for forest areas or grass fuel areas using prior values (Forestry Canada Fire Danger Group, 1992; Wotton et al., 2009) based on a forest to grassland threshold per Staver et al. (2011). Note that there was a typographic error in the lb equation for grasses by the Forestry Canada Fire Danger Group (1992), which was reported and corrected in Wotton et al. (2009) but nonetheless incorporated into the original SPITFIRE code of Thonicke et al. (2010, their Eq. 13); we remove that error and use the Wotton et al. (2009) equation here (Eq. 12). Weffect (m min−1) is the wind adjusted by vegetation fraction with W being the site-level wind boundary condition.
The length of the major axis is calculated for both the front, df (m), and back, db (m), of the fire ellipse using the associated ROS.
Fire size, (Fsize) (m2), is calculated using the methods of Arora and Boer (2005).
The total area burned (Aburn,patch) (m2 km−2) is calculated for fires of size Fsize (m2) for each of the daily successful ignitions () (Ilightning and Ianthro) while accounting for the fire danger conditions (FDI). Ignitions (Ilightning and Ianthro) are input or calculated for the total grid cell area, and we assume that ignitions are equally distributed per unit area across each patch; therefore Ilightning and Ianthro are provided as strikes per km−2 of patch area per day. The Aburn,patch is therefore m2 km−2 per patch area per day.
Fire damage and mortality
As in Thonicke et al. (2010) tree mortality from fire is calculated based on both cambial damage to bark and crown scorch to the canopy. Damage from crown scorch is calculated in relation to scorch height (SH) (m) of a fire:
where F is a PFT-specific parameter based on field studies. In this study F is set to 0.1487 for the fire-vulnerable tree and 0.06 for the fire-tolerant tree as in the tropical broadleaved evergreen and tropical broadleaved rain-green tree PFTs, respectively, from Thonicke et al. (2010).
Within FATES, fire effects on plants are evaluated for each cohort that experiences fire. Assuming a cylindrical crown shape, the proportion of crown scorch (CS) is calculated for each cohort as
where H (m) is the height of the tree cohort (m) and CD (m) is the crown depth length calculated using a PFT-specific crown depth fraction (CDfrac). For this study, the fire-vulnerable tree PFT has a CDfrac of 0.33 and the fire-tolerant tree PFT a CDfrac of 0.1. The probability of tree mortality from crown scorch (pcs) is calculated as
where r is a PFT-specific resistance factor for crown scorch survival and p is a parameter based on defoliation from crown scorch set to a default value of 3.0 (Thonicke et al., 2010). For this study, the resistance factor for crown scorch survival (r) is set to 1 for the fire-vulnerable tree PFT and 0.05 for the fire-tolerant tree PFT.
Cambial damage is based on the residence time of the fire (τf) and the bark thickness of the cohort. Probability of mortality from cambial damage (pτ) is calculated as
where τc is the critical fire residence time (min) based on bark thickness (BT) (centimeter bark per centimeter diameter at breast height – DBH).
The overall probability of mortality (pm) is calculated as
Thus, for each day with a fire, a burned area is calculated for each patch, burned plants are killed and sent to coarse woody debris pools, and unburned plants are added to a new patch. Fire effects, including consumption of ground fuels, damage to vegetation through cambial damage, and crown scorch, are applied to the fraction of each patch that burns, which in turn splits into a newly disturbed patch with area equal to the area that burned. Fire effects on fuels and vegetation thus only occur on the newly burned patch. The newly burned patches resulting from the burned fraction of each patch are given a time-since-disturbance age of zero and are generally fused together and into other recently disturbed patches, following the FATES patch fusion logic (Fisher et al., 2015). The newly burned patch thus retains a fire-impacted vegetation structure of plants that have survived the fire event.
2.1.3 Model experiments
We defined a series of model experiments reflecting trade-offs associated with fire-tolerance strategies in vegetation traits selected for each plant functional type (PFT) and conducted a test of model sensitivity to the parameter governing fuel drying (drying ratio). We then explored how climate–fuel relationships and vegetation traits mediate ecosystem assembly, as well as the impact of vegetation state on fire behavior (Table 1). We completed a set of simulations for South America varying the fuel drying ratio and then compared results to contemporary observations. We then ran a simulation using the intermediate drying ratio parameterization across the tropics.
FATES–SPITFIRE was run as a module within the CLM5 (Lawrence et al., 2019) using air temperature, humidity, wind, air pressure, precipitation, and shortwave and longwave radiation produced by the Global Soil Wetness Project (GSWP) for the period 1994–2013, with forcing data at a 6-hourly time step (disaggregated to 30 min time steps by the native CLM algorithm). The forcing data were part of the third phase of GSWP (GSWP3v1, Dirmeyer et al., 2006; Hyungjun, 2017; http://hydro.iis.u-tokyo.ac.jp/GSWP3/, last access: 28 May 2024) and are based on the 20th Century Reanalysis version 2 from the NCEP model (Compo et al., 2011). To allow for vegetation spin-up, the forcing data were cycled repeatedly for a period of 300 years with the final 10 years used for evaluation. All simulations started from a bare ground condition with fire active from the beginning of the simulation and were conducted under a stable recent historical (2000) CO2 concentration (367 ppm). Anthropogenic land use was not used in this study, and thus these simulations represent a potential vegetation case.
Table 2Parameter values for the two tree PFTs and C4 grass used in the simulations.
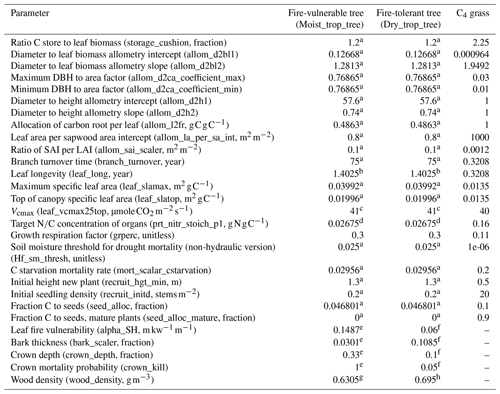
References: a Koven et al. (2020); b Kattge et al. (2011); c Kattge et al. (2009). d Calculated based on Vcmax and SLA per Walker et al. (2014), model 2, their Table 3. e Tropical broadleaved evergreen (Thonicke et al., 2010); f tropical broadleaved rain-green (Thonicke et al., 2010); g mean for species from the Amazon forests (Chave et al., 2006); h mean for species from the South American dry forests (Chave et al., 2006).
Three PFTs were used in all simulations and allowed to establish and compete on all grid cells: a C4 grass and two tropical trees, with one tree PFT utilizing a set of fire-tolerant traits and the other a set of fire-vulnerable traits (Table 2). Specifically, the fire-vulnerable and fire-tolerant trees are distinct for five parameters: leaf fire vulnerability, bark thickness, crown depth, crown mortality probability, and wood density (Table 2). Supplemental seed dispersal from outside the grid cells was disabled in these simulations. Given that coexistence of PFTs is sensitive to the representation of seed rain (Maréchaux and Chave, 2017; Fisher et al., 2010), coexistence is not assured and a PFT may go locally extinct in a given grid cell. The tree PFTs were otherwise parameterized with common traits and allometry from Koven et al. (2020) with updates to the maximum carboxylation at reference temperature (Vcmax) (Kattge et al., 2009), leaf longevity (Kattge et al., 2011), and leaf nitrogen derived from Vcmax and specific leaf area (SLA) per the relationship between these quantities derived by Walker et al. (2014) (model 2 in their Table 3) (Table 2). The tree PFT growth respiration factor (grperc) was adjusted from the CLM5 default of 0.11 to 0.3 for a carbon use efficiency (CUE) with a mean of 50 % (Table 2, Fig. S3 in the Supplement) calculated as the ratio between mean net primary productivity (NPP) and gross primary productivity (GPP). This version of FATES does not use the same maintenance respiration terms as CLM5 and thus gives biased-low CUE when the CLM5 value is used. Distinct tree fire strategies represented with trait trade-offs for crown, leaf, and bark characteristics were parameterized as in Thonicke et al. (2010) using their tropical broadleaved evergreen as the fire-vulnerable strategy and their tropical broadleaved rain-green as the fire-tolerant strategy in this study (Table 2). Wood density uses data from Chave et al. (2006) (their Table 1) with the lower-wood-density fire-vulnerable tree represented by the mean value for species from the Amazon forests and the higher-wood-density fire-tolerant tree represented by the mean value for species from the South American dry forests (Table 2). The fire-vulnerable tree has lower wood density and, as a result, less costly resource allocation than the fire-tolerant tree, resulting in faster height growth and biomass accumulation, but is more likely to experience damage and mortality due to fire. The fire-vulnerable tree has higher leaf fire vulnerability, a thicker crown creating more exposure to flame scorch, a lower accumulation of protective bark, and a higher probability of crown mortality than the fire-tolerant tree. Thus, while FATES does not directly impose a penalty on trees for having thick bark or other fire-tolerant traits, we have imposed a trade-off between wood density and fire tolerance such that in the absence of fire we expect the lower-wood-density fire-vulnerable tree to outcompete the higher-wood-density fire-tolerant tree. In situations with fire, despite their faster growth rate from less costly resource allocation associated with the lower wood density, the fire-vulnerable trees are more likely to have crown mortality due to their higher sensitivity to leaf scorch and crown scorch and to have cambial damage due to their lower bark thickness compared to the fire-tolerant tree.
We estimate grass allometry based on the tiller size and height of Spartina alterniflora, which are well studied to parameterize the allometric relationships in FATES. Grass height allometry is based on observation data from Daehler et al. (1999) and Travis and Grace (2010). The aboveground biomass allometry parameters are estimated based on observed height, tiller diameter, and tissue density (Radabaugh et al., 2017). The leaf allometry parameters are fitted based on observed aboveground biomass in relationship to height and diameter, assuming that ∼ 50 % of aboveground biomass is leaf biomass (Gross et al., 1991). The live fine root biomass to live leaf ratio is set to 1.0 and storage to leaf ratio as 2.25, considering that ∼ 75 % of belowground biomass is rhizome for storage (Schubauer and Hopkinson, 1984). The specific leaf area is estimated from Giurgevich and Dunn (1979). The ratio of tiller diameter to crown area is fitted to observed tiller density (Radabaugh et al., 2017). The Vc,max25 is set to 40 (Giurgevich and Dunn, 1979).
A total of five CLM–FATES simulations were completed with four at the 0.5°×0.5° grid resolution for South America exploring a range of fuel drying ratio parameterizations and one pan-tropical simulation at the 0.9°×1.25° grid resolution applying the intermediate fuel drying parameterization. The fuel drying ratio and geometry determine how fuel moisture content responds to fire-relevant weather conditions (Fig. S1,) and this fuel moisture in turn impacts the effectiveness of combustion (Fig. S2), with smaller or drier fuels experiencing more combustion than larger or wetter fuels. To explore the model sensitivity to this crucial aspect of fire dynamics and allow us to generate potentially variable fire regimes, we modified the parameter for the fuel drying ratio using a value for low fuel drying at 66 000 °C−2 (Thonicke et al., 2010), high fuel drying at 13 000 °C−2 (Lasslop et al., 2014), and medium fuel drying at 25 000 °C−2 (Table 3). In these idealized experiments, we investigated whether fuel drying acts as a significant factor in the biogeography and explored the connection between fuel drying across the same climate conditions to investigate the span of potential responses across the tropics. In the real world, these connections will have a more complex and heterogeneous spatial pattern related to variability in local conditions. The simulations for these hypothetical fuel drying scenarios were compared against a control simulation without fire disturbance and against contemporary observations.
2.1.4 Evaluation data
We evaluated simulated output using data processed and regridded to 0.5°×0.5° resolution available as part of the ILAMB project (Collier et al., 2018). Productivity was evaluated using gross primary productivity (GPP) for the period from 1980–2013 from the GBAF product derived from FluxNet MTE observations (Jung et al., 2010) and leaf area index (LAI) for the period of 2011–2015 generated from the MODIS satellite observations (De Kauwe et al., 2011). Biomass was evaluated against the carbon stock product of Saatchi et al. (2011). Simulated burned area was evaluated against the burned-area product from the Global Fire Emissions Database (GFED4S; Giglio et al., 2013; van der Werf et al., 2017) for the period of 1997–2016, which includes small fires.
3.1 Influence of fuel drying assumptions
The mean burned area across South America displays a variable spatial pattern among the three FATES simulations that differ in the fuel drying parameterization (Fig. 1). The peak burned-area region in both the observations and the model extends from the northeast of Brazil and along the southeastern edge of the Amazon, with high burned areas also to the north of the forest. The three simulations show the high parameter sensitivity of FATES–SPITFIRE, where increased fuel drying leads to simulations with higher burned area than observed. Higher fuel drying parameterizations were associated with an increased spatial extent of burned area, a longer peak fire season with more ignitions, faster forward rate of spread, more intense fires, and higher burned fraction across annual averages for all fires (Table 4) and all months, but with the largest increases from June to October (Fig. 2) and more recurrent burning during those months, shown as values greater than 1.0 (Fig. 2d). Across the South American region, the different parameterizations of fuel drying result in different ecosystem structure and function due to the changes to the fire regime, including lower biomass and tree cover with higher fuel drying (Fig. 3, Table 4).
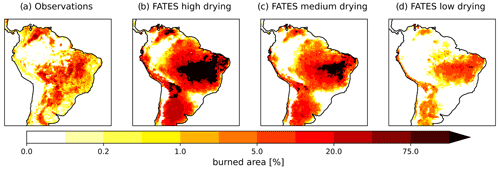
Figure 1Mean annual fraction area burned from (a) observations (van der Werf et al., 2017) and for CLM–FATES for the final 10 years of 300-year simulations with active fire disturbance and a (b) high, (c) medium, or (d) low fuel drying parameterization.
Table 4Mean (standard deviation) across fuel drying ratio assumptions for South America regional simulations for the final 10 years of simulation.
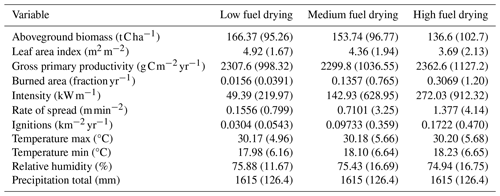
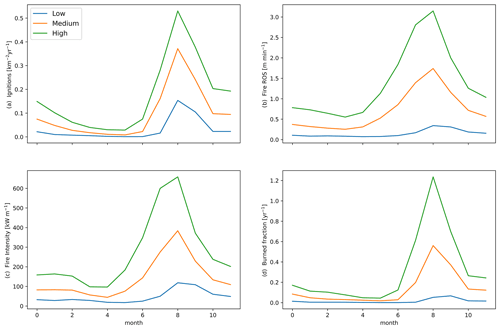
Figure 2Mean seasonal change in (a) fire ignitions, (b) rate of spread (ROS), (c) intensity, and (d) burned fraction for parameterizations with low (blue), medium (orange), or high (green) fuel drying for the final 10 years of 300-year simulations in CLM–FATES across South America.
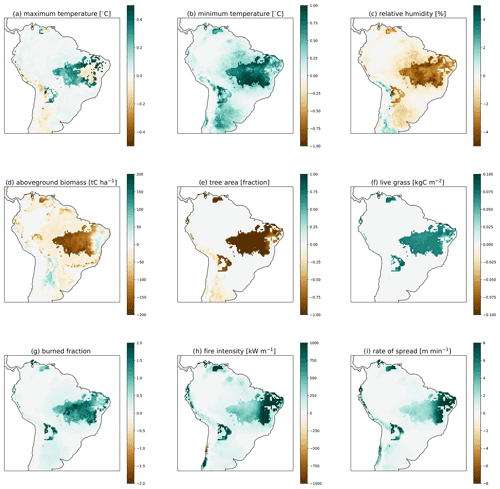
Figure 3Difference between the high and low fuel drying parameterizations for (a) maximum 2 m air temperature, (b) minimum 2 m air temperature, (c) relative humidity, (d) simulated aboveground biomass, (e) tree area, (f) live grass, (g) burned fraction, (h) fire intensity, (i) rate of spread, and (j) ignitions for the final 10 years of 300-year simulations in CLM–FATES.
Though the same atmospheric forcing data were used for all simulations, the resulting vegetation distribution differences under the high (vs. low) fuel drying parameterization led to higher maximum and minimum temperature by as much as 1 °C and lower mean annual relative humidity by up to 4 % (Figs. 3, S4, and S5 in the Supplement). These differences were primarily concentrated in regions with a change in tree cover fraction. Across all simulations, areas which lost biomass were associated with lower relative humidity, more burning, and fire effects (Figs. 3, S4, and S5). Seasonal declines in precipitation and relative humidity coincide with the peak fire season, and the highest rates of burning and fire effects occurred in August (Figs. 2 and S6 in the Supplement). The natural seasonal decline in fuel moisture for dead leaves and live grass coincides with the increase in fire behavior and effects from June to October (Figs. 2 and 4), whereas twigs and small branch fuels did not have large seasonal fluctuations in moisture. Across the region, more intense and larger simulated fires were associated with the presence of live grass fuels but did not have a clear relationship with live grass moisture or amount (Figs. 5 and S7 in the Supplement). Fire intensity decreases as dead fuel moisture increases with precipitation and relative humidity, but dead fuel amount shows mixed relationships across climate variables, without simple linear consequences for fire intensity or burned fraction (Figs. S8 and S9 in the Supplement). Mean aboveground biomass decreased with increased fuel drying, with biomass losses occurring in the drier northeastern regions of South America (Figs. 6 and S10 in the Supplement, Table 4).
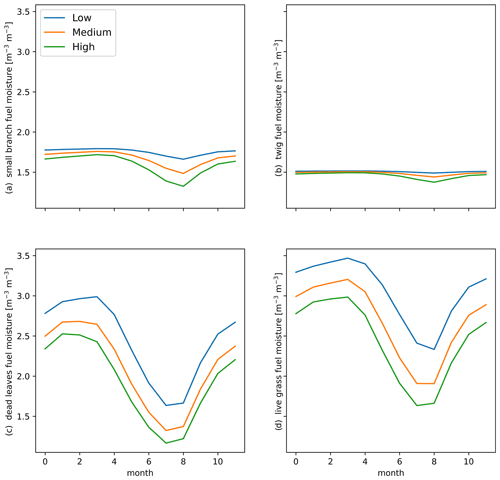
Figure 4Mean seasonal change in fuel moisture (m3 m−3) for (a) small branches, (b) twigs, (c) dead leaves, and (d) live grass fuels for parameterizations with a low (blue), medium (orange), or high (green) fuel drying ratio for the final 10 years of 300-year simulations in CLM–FATES.
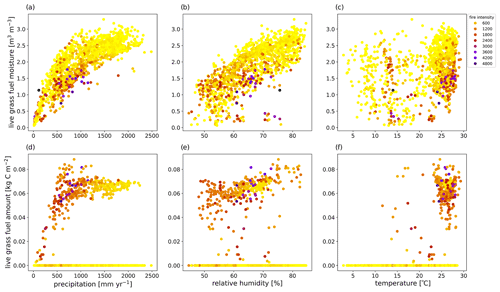
Figure 5Association of fire intensity (colors; kW m−1) for live grass fuel moisture (m3 m−3) with (a) precipitation, (b) relative humidity, and (c) temperature, as well as for live grass fuel amount (kg C m−2) with (d) precipitation, (e) relative humidity, and (f) temperature for fire intensities above 100 kW m−1 from the final 10 years of a 300-year simulation in CLM–FATES across South America using a medium fuel drying parameterization.
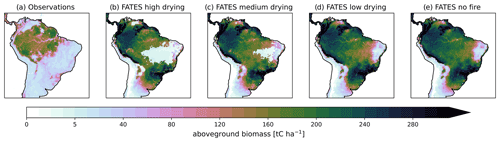
Figure 6Aboveground biomass for (a) observations (Saatchi et al., 2011) and for CLM–FATES using parameterizations with (b) high, (c) medium, or (d) low fuel drying as well as (e) without fire disturbance for the final 10 years of 300-year simulations.
Parameterizations with higher fuel drying resulted in the expansion of grass and fire-tolerant tree PFT distributions as well as their associated biomass and a lower total mean biomass across the region (Fig. 7, Table 4). Comparisons of simulated size-based fire mortality showed that, for all simulations with fire disturbance, the fire-tolerant trees escaped fire mortality through height and fire-resistant traits more effectively than the fire-vulnerable trees, but trees below 20 cm diameter at breast height (DBH) for both PFTs experienced elevated mortality during fire events (Figs. 8, S11, and S12 in the Supplement).
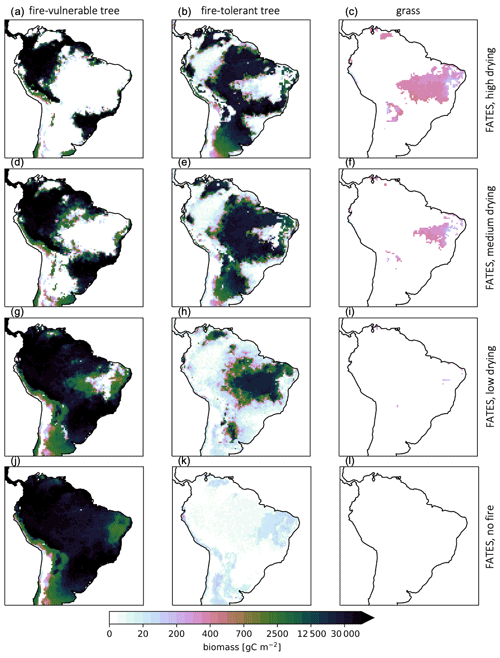
Figure 7Mean PFT biomass (g C m−2) for parameterizations with a high (a–c), medium (d–f), or low (g–i) fuel drying and active fire or no fire (j–l) for the final 10 years of a 300-year CLM–FATES simulation.
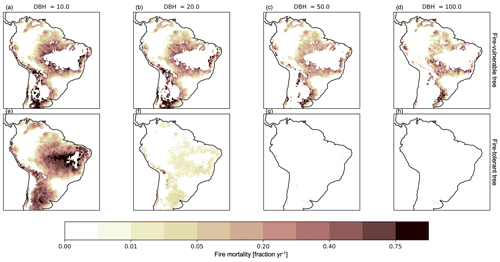
Figure 8Mean annual fraction of tree mortality due to fire effects across tree cohort sizes with diameter at breast height (DBH) of (a, e) 10, (b, f) 20, (c, g) 50, and (d, h) 100 cm from FATES simulations using a medium fuel drying parameterization for the final 10 years of a 300-year simulation. The top row (a–d) is the fire-vulnerable tree PFT and the bottom row (e–h) is the fire-tolerant tree PFT.
3.2 Comparisons against observations
Active fire disturbance across the South American region reduced the biomass density (Figs. 6 and 9). When comparing our simulations of a potential forest state to contemporary observations we find that all simulations, including the no-fire simulation, had a high bias compared to contemporary observations for biomass (Fig. 9), especially in the less disturbed areas of the Amazon and, as expected given the lack of land use in the simulations, in the highly anthropogenically disturbed Atlantic coastal forest region (Fig. 6). Without fire disturbance, the fire-vulnerable tree becomes dominant, driving the grasses to extinction throughout much of the domain, and the fire-tolerant tree to near extinction (Fig. 7). Simulated vegetation productivity (GPP) showed a high bias across grassland-dominated regions and a low bias for forested regions when compared to contemporary GPP data products (Figs. S13 and S14 in the Supplement). Mean GPP () and leaf area index (LAI) across South America were high for all fuel drying parameterizations (Table 4) compared to the mean GPP of 1981.6 and the LAI of 2.68 for observations (Fig. S13). The observed seasonality of fires was captured by the medium fuel drying simulation with agreement on the timing of peak fire season (June to October; Fig. 2). The simulated burned area across the South American region for the medium and high fuel drying parameterizations had areas of repeat annual burns that were not in the observations and extended into the eastern Amazon, beyond that of observations (Fig. 1). Within the forested areas, fires had mean fire intensity values less than 300 kW m−1 (Fig. S15 in the Supplement), which is consistent with the fire intensities observed in these ecosystems (Brando et al., 2016).
3.3 Pan-tropical application
Application of the medium fuel drying parameterization across the tropics for a 1°×1° simulation also showed high biases in simulated biomass for areas of naturally occurring high biomass accumulation across wet areas of Africa and Indonesia (Figs. 10 and 11) with observations 60 % lower than the simulated values. Simulated mean annual burned fraction was high, with areas of repeat burns that extended beyond observed burned areas (Fig. 12). In the simulation, mean annual rainfall (MAR) (mm yr−1) above 2500 mm is associated with closed forest canopies and nearly continuous tree cover, fire intensity is generally below 150 kW m−1, and there is a low frequency and extent of burning (Fig. 13). Across the tropics, simulated mean GPP, LAI, aboveground biomass, and burned fraction were biased high compared to observations (Table 5, Fig. S14). Pan-tropical simulated burned fractions were associated with grass areas for the highest simulated mean annual fire intensities, generally above 400 kW m−1 (Figs. 12 and 13).
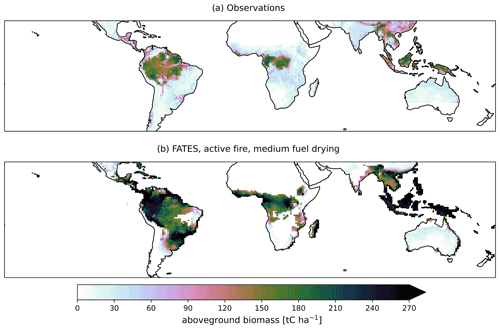
Figure 10Mean aboveground biomass from (a) observations (Saatchi et al., 2011) and (b) CLM–FATES from the final 10 years of a 275-year simulation with active fire disturbance and medium fuel drying parameterization.
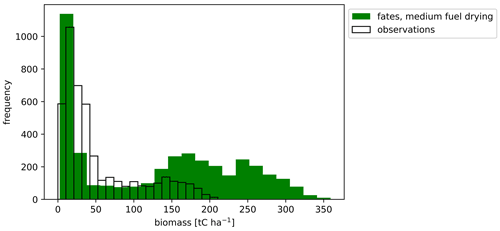
Figure 11Mean aboveground biomass (t C ha−1) for the pan-tropics from observations (Saatchi et al., 2011) (clear) and CLM–FATES (green) for the final 10 years of a 275-year simulation with parameterizations for a medium fuel drying ratio.
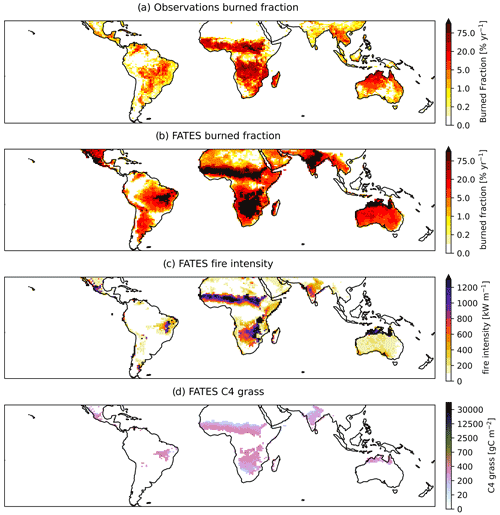
Figure 12Mean burn area (% yr−1) from (a) observations (GFED41s) and CLM–FATES simulation for (b) burned area, (c) fire intensity, and (d) C4 grass biomass from the final 10 years of a 275-year simulation with active fire disturbance and medium fuel drying parameterization.
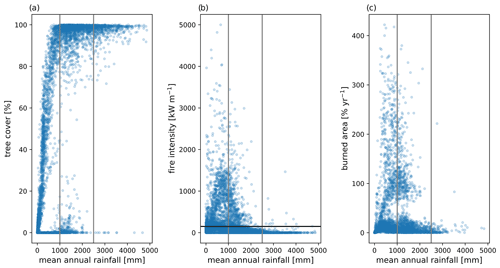
Figure 13Simulated mean (a) tree cover, (b) fire intensity, and (c) burned area as a function of mean annual rainfall (MAR) (mm yr−1) from the final 10 years of a 275-year CLM–FATES simulation across the tropics with active fire disturbance and a medium fuel drying parameterization. Gray vertical lines indicate 1000 and 2500 mm MAR. The horizontal black line in (b) indicates a fire intensity of 150 kW m−1.
Globally, fire disturbance and associated fire behavior and effects are important contributors to shifting ecosystem structure and function (Bowman et al., 2020; McLauchlan et al., 2020). We demonstrate here using differences in fire-related ecological traits and hypothetical fuel drying that size structure and fire-tolerance strategy together determine the susceptibility of trees to fire mortality and the resulting biogeography and accumulation of biomass. Further, the FATES-projected biomass and distribution of simulated fire-tolerant and fire-vulnerable trees and grasses were strongly influenced by fuel drying and associated fire behavior, highlighting the importance of fuel state interacting with fire-tolerance traits to structure savanna and forest biomes.
Small tree cohorts of both types suffered high mortality in fire-prone areas, but fire-tolerant trees were more consistently resilient across fuel parameterizations, including in simulations with drier fuels that resulted in increased fire frequency. The variation in functional strategies was fundamental to capturing shifts in vegetation type and overall biomass accumulation across a gradient of fire disturbance. Fire-tolerant trees and grasses are more competitive under increased fire conditions and, conversely, impeded via resource-related competition dynamics under fire-free conditions.
4.1 Tropical biogeography and associated fire behavior
4.1.1 Vegetation traits and size structure as drivers of fire behavior and effects
Across a wide range of fire intensity and frequency, fire acts as a selective pressure on tree survival and ultimate success. Though all simulated small trees experienced high mortality across the fuel drying parameterizations (Figs. 8, S11, and S12), it was only through trait differences that tree biogeography was determined. The trade-off between wood density and fire tolerance (Table 2) provided a competitive advantage to the fire-vulnerable tree in areas where fire was absent. Simulated biogeography for the medium fuel drying parameterization, where the fire-vulnerable, large-canopied, thin-barked tree was dominant across the Amazon region (Fig. 7), reflects the spatial distribution of thin- versus thick-bark trees documented by Pellegrini et al. (2017). These results are in agreement with studies of bark variation and its association with fire disturbance in the tropics (Staver et al., 2020; Pellegrini et al., 2017; Hoffmann et al., 2009; Brando et al., 2016; Uhl and Kauffman, 1990) in suggesting that fire-driven losses will be higher in fire-vulnerable forests. Though these results use hypothetical scenarios, representation of the spatiotemporal dynamics of competition between PFTs with different fire-tolerance strategies is critically important for prediction of future fire severity impact on vegetation biomass accumulation and composition.
Differences in fire mortality across tree sizes is well documented (Hoffmann et al., 2009; Uhl and Kauffman, 1990; Brando et al., 2016), and our results are consistent with previous studies. Fire effects on trees are generally dependent on the trees' size structure, bark thickness, and canopy characteristics. For studies at the edge of frequently fire-disturbed areas in South America, on the rare occasions that fire enters forests, smaller trees are killed, but larger trees survive (Hoffmann et al., 2009; Higgins et al., 2000; Hoffmann and Solbrig, 2003) based on the larger trees' accumulation of thicker bark and a taller canopy height that escapes flame damage. Among established fire-tolerant trees, once a tree has surpassed the height of the flame zone, mortality is low in fire-disturbed areas (Higgins et al., 2000; Hoffmann et al., 2009). Our results capture the decrease in tree mortality with an increase in size (Figs. 8, S11, and S12). In our model, the simulated fire-tolerant trees were able to maintain a distribution of biomass and basal area across sizes (Figs. S16 and S17 in the Supplement) in the fire-disturbed area, despite experiencing high mortality for plants smaller than 20 cm DBH (Figs. 8, S11, and S12). The fire-vulnerable tree, in contrast, became extinct in these fire-prone areas (Figs. S16 and S17). Field studies on fire-disturbance impacts on vegetation structure and function are limited, with only two main field studies in the dry Amazon forests of Tanguro, Brazil (Brando et al., 2016, 2012), and the Cerrado forests of the IBGE Reserve (Hoffmann et al., 2009). Increased survivability past 20 cm DBH in simulations is consistent with field measurements by Balch et al. (2015) and Brando et al. (2016) for experimental burns in the dry forests of Tanguro.
Fire mortality in FATES results from the combination of bark and canopy effects that vary as trees grow larger, with canopy damage from crown scorch calculated as a function of fire intensity and PFT-specific fire tolerance (Eq. 17). A shift to drier fuels leads to an increase in simulated mean fire intensity to 143 kW m−1 for the medium fuel drying parameterization from 49 kW m−1 for the low fuel drying parameterization (Fig. S15, Table 4). With this shift to drier fuels and subsequent increase in fire intensity, fire-associated tree mortality extends into the Amazon (Figs. 8 and S12), implying that the increase in fire intensity under the medium fuel drying parameterization surpasses the fire characteristics (e.g., intensity, flame height, duration) that these fire-vulnerable trees can survive. Though fire is active from the beginning of the simulations, variation of tree distribution and biomass accumulation among the fuel drying scenarios (Fig. 7) demonstrate that less frequent burning (Fig. 1) and lower annual fire intensity (Fig. S15) associated with wetter fuels and less fuel drying are considerations for tree survival and distribution. Initialization with a potential tree stand structure would need to be evaluated for survival and resilience under similar fuel drying conditions and the associated fire frequency and intensity, as small-stature establishing trees would be expected to show more vulnerability to fire than existing tree stands. The simulated mean fire intensity of 143 kW m−1 for the medium fuel drying parameterization is close to the fire intensity mortality threshold value of 149 kW m−1 derived by De Faria et al. (2021) using data from Staver et al. (2020), demonstrating that bark thickness continues to increase and protect against mortality until reaching this fire intensity mortality threshold. The pattern of increased tree mortality for areas with simulated fire intensities beyond the fire intensity threshold derived by De Faria et al. (2021) suggests broad agreement with the functional relationship from Staver et al. (2020). The Amazon has high diversity among trees classified as fire-vulnerable or fire-tolerant, and within that diversity tree bark thickness varies in space and time due to its connection with demography; these results only capture two broad categories of trees with variable strategies. Simulation results from the low fuel drying parameterization, with a mean fire intensity of 49 kW m−1, still demonstrated mortality across all sizes for the fire-vulnerable tree (Fig. S12), with mortality occurring within the Amazon region, but at a much lower rate compared to simulations using increased fuel drying parameterizations (Figs. 8 and S11). This allows the simulated fire-vulnerable tree to extend its dominance across much of South America through competitive advantage. Across the tropics, fire-tolerant trees vary from one savanna region to another and are not a subset of forest tree species (Bond et al., 2003; Hoffmann et al., 2003). Within Australia, the Myrtaceae family, which includes eucalypts and is characterized as an excellent resprouter even after high-intensity fire, dominates fire-dependent forests across the continent (Crisp et al., 2011; Burrows, 2002) and may actually promote fire with elevated fuels and flammable leaf litter (Lehmann et al., 2011). These continental differences among trees are not accounted for in this current study and suggest that for forested regions outside of South America further parameterization and additional PFTs may be needed to capture the interaction between climate, fire, and vegetation.
Further, this version of FATES does not include the capacity of trees to resprout following fires, which is a key feature of persistence for trees in savanna regions (Gignoux et al., 1997; Hoffmann et al., 2009; Govender et al., 2006; Higgins et al., 2000). In reality, fires often cause loss of the whole aboveground stem, but not mortality for some individual fire-tolerant trees. This “topkill” of the individual tree stem is then followed by resprouting, which can accelerate recovery after fires (Hoffmann et al., 2009; Higgins et al., 2000; Van Wilgen et al., 2004). The actual rate of mortality can therefore be low in established fire-tolerant stands where small trees are able to persist even with repeated topkill by fires (Higgins et al., 2000; Hoffmann, 2000). Additionally, simulated trees in FATES can experience canopy and/or bark cambial damage from fire without mortality, but damaged trees in FATES do not experience a long-term loss of function and quickly return to a pre-fire state with pre-disturbance allometry. Across the tropics, crown damage is an important predictor of mortality (Reis et al., 2022; Arellano et al., 2021), and after light limitation, crown damage was the most important mortality risk indicator (Zuleta et al., 2022). Future work will link fire-related damage to the FATES mechanistic crown damage module (Needham et al., 2022) that imposes limitations on tree regrowth following a damage event as the trees attempt to recover.
Within the closed moist forests of the Amazon, the dense canopy shade prevents grass establishment (Hoffmann et al., 2003; Brando et al., 2020; Cochrane et al., 1999), but along the drier savanna and Cerrado regions trees and grasses coexist (Hoffmann et al., 2003; Higgins et al., 2000). Across the tropics, mean annual rainfall (MAR) acts to moderate tree cover, which limits fire behavior (Staver et al., 2011; Pueyo et al., 2010). Intermediate MAR between 1000 and 2500 mm can be associated with both forest with tree cover greater than 55 % and with savanna with less than 55 % tree cover (Staver et al., 2011; Hirota et al., 2011). Though our simulations capture this range of variability in tree cover for low to intermediate MAR (Fig. 13), we do not capture coexistence between trees and grasses. Grass–tree coexistence is thought to occur during transitional stages where trees cannot escape flame zones before entering larger size classes (Higgins et al., 2000). Slower tree growth rates or consistent disturbance allow grasses to invade and expand, but faster tree growth rates favor forest expansion (Higgins et al., 2000). FATES captures very limited areas of tree–grass coexistence at the edges between the fire-tolerant tree and C4 grass area, as the simulated forest canopy quickly closes and shades out the simulated grasses (Fig. 7). Fire behavior and effects vary depending on interactions among vegetation type, distribution, and surface fuels. For this study, in areas with lower tree cover we use a grass-specific fire spread equation (Eq. 12), where low tree cover and grass areas have a longer burned ellipse than higher tree cover areas (Wotton et al., 2009). The amount of tree cover used for the shift to a longer burned ellipse area warrants further investigation as it may reinforce feedbacks to promote grasses and prevent tree–grass coexistence.
The simulated fire-tolerant tree is resilient under the higher fuel drying parameterizations, maintaining and expanding its area of dominance into the range of the fire-vulnerable tree, while losing range in drier regions to the C4 grass with associated fire increases. The loss of simulated biomass of 17.14 t C ha−1 across the whole domain with a transition from fire-vulnerable trees to fire-tolerant trees and grasses highlights the vulnerability of regional carbon stores with drier fuels (Table 4). The potential for biomass loss associated with increased fire disturbance is in agreement with previous studies (De Faria et al., 2021; Burton et al., 2022; Bond et al., 2005). Notably, areas that are degraded by disturbance have demonstrated colonization by grasses that then facilitate increased fire frequency and expanded grass invasion (Balch et al., 2015; Veldman and Putz, 2011; Silvério et al., 2013; Hoffmann et al., 2012; Veldman et al., 2009). Conversion from forest to grasses has been shown to dramatically increase fine fuel loads compared to forest litter (Silvério et al., 2013; Hoffmann et al., 2012), thereby increasing the potential for more intense fires. Our results demonstrate this increase in fire intensity with the presence of grasses (Figs. 5 and 12). Historically, fire was a major factor in determining the current distribution of grasses (Bond and Midgley, 2012b; Staver et al., 2011; Hirota et al., 2011; Lehmann et al., 2011; Sankaran et al., 2005, 2008; Bucini and Hanan, 2007). Slow tree recovery after fire has also been suggested as a key factor in the spread of grasses (Bond, 2008; Hoffmann et al., 2009), as fires maintain grasses in areas suitable for forests through frequent burning that favors vegetation with underground storage (Bond and Midgley, 2012b; Ratnam et al., 2011; Hoffmann, 2000). At forest–savanna margins trees are able to recruit and grow when they escape the influence of grass fires through local variations in seasonal fire intensity (Higgins et al., 2000; Balch et al., 2015; Govender et al., 2006).
4.1.2 Fire intensity and fuel dynamics
The spatial patterns in simulated fire intensity and burned fraction were defined through interactions between the climate and emergent vegetation and climate, resulting in, broadly, two groups: low-intensity fires below 150 kW m−1 in regions without grass presence and higher-intensity fires (> 500 kW m−1) in regions with grass presence (Figs. 12 and 13). The simulations demonstrated a positive grass–fire feedback where regions with MAR below 2500 mm have frequent and high-intensity large fires that promote grass dominance. Simulated fire intensities for these areas with low to intermediate MAR (less than 2500 mm) (Figs. 12 and 13) are consistent with those measured in savannas in Kruger National Park in Africa (up to 17 905 kW m−1) (Govender et al., 2006), the Northern Territory of Australia (500–18 000 kW m−1) (Williams et al., 2003), the Campos grasslands of Brazil (36 to 319 kW m−1) (Fidelis et al., 2010), and the Cerrado of South America (2842 to 16 394 kW m−1) (Kauffman et al., 1994). Across the tropics with sufficient moisture and in the absence of grazing, grass production during the wet season becomes available as fuel during the dry season capable of supporting frequent fires (Higgins et al., 2000; Bond and Midgley, 2012b; Govender et al., 2006). The seasonal shifts in fuel availability and moisture for fine fuels of live grass and dead leaves demonstrated in this study (Fig. 4) were associated with higher fire intensities and burned fraction (Fig. 5) and are consistent with previous work (Higgins et al., 2000; Balch et al., 2015; Hoffmann et al., 2012; Govender et al., 2006). In contrast, regions with high MAR above 2500 mm generally have low simulated fire intensities, with values generally below 150 kW m−1, and more than 80 % tree cover, characteristics that are consistent with observed understory fires of the Amazon (Fig. 13).
Historically, within the Amazon, fire was previously limited to deforested or agricultural areas (Alencar et al., 2011), as the closed forest canopy creates a moist understory microclimate environment that limits the potential for fire (Brando et al., 2020; Hoffmann et al., 2009). Understory low-intensity fires are documented across portions of the Amazon (Morton et al., 2013; Aragão et al., 2018), but the range and variation in fire intensity of these understory fires are not extensively documented (Staver et al., 2020; Cochrane et al., 1999). This study simulated fire intensities across South America with mean values of 49, 143, and 272 kW m−1 for the hypothetical low, medium, and high fuel drying parameterizations, respectively (Table 4). Simulated fire intensity varied regionally as well as seasonally, but across the Amazon it was consistently lower in all fuel drying parameterizations (Figs. 2 and S15). This places the simulation that used the low fuel drying parameterization within the upper range of intensity values derived for the Amazon by Staver et al. (2020) using data from Cochrane et al. (1999) with an upper limit of around 55 kW m−1 and below the value of 75 kW m−1 reported by Brando et al. (2016) for Tanguro, which is located on the dry edge of the Amazon. These differences between the Amazon and Tanguro suggest that fuel characteristics for the closed-canopy forests of the Amazon are not the same as the open- and sparse-canopy forests in drier regions of South America, such as Tanguro. Characterization of fuels, including their presence as both live and dead fine fuels as well as their decomposition, geometry, and moisture, are key uncertainties for fire models (Hanan et al., 2022). In the simulation, the fuel drying parameterization is the same across South America, and fire behavior and intensity respond to climate, vegetation, and fuel variability. The effects of moist understory microclimate on fuel characteristics as is documented for closed-canopy forests are not captured in this version of FATES, but future versions that include moist understory conditions, such as through the use of a multi-layer canopy (Bonan et al., 2021), may increase fuel moisture and thus lower fire intensity mechanistically with fuel moisture responding to local microclimate conditions rather than a global drying parameterization. The low simulated levels of fire occurrence, burned area, and fire intensity, with energy generally below 150 kW m−1 associated with high MAR above 2500 mm for the tropical simulation (Fig. 13), demonstrate behavior consistent with that of understory forest fires in the Amazon where grasses are excluded and fine surface fuel amounts are limited. Furthermore, low-intensity understory forest fires, such as those observed in the Amazon, are not representative across the tropics, which is characterized by a diversity of pyromes, or regions with similar fire characteristics, such as regions with high-intensity large fires like those found in Australia (Archibald et al., 2013). This version of FATES does not include the potential for a surface fire to become a crown fire, whereby a surface fire ignites canopy fuels, creating a more intense fire, but future work will include the potential for crown fire behavior. Though these results have frequent burning across the tropics (Fig. 12), they do not fully capture the potential for high fire intensities across the diversity of forested areas (Archibald et al., 2013).
4.2 Modeling fire behavior and effects at the Earth system scale
At the Earth system scale there are an increasing number of models which capture fire occurrence and impacts (Hantson et al., 2016), but they vary in the process complexity and aspects of fire that are included (Hantson et al., 2020). The Fire Model Intercomparison Project (FireMIP) is an international initiative aimed at comparing and evaluating existing models against benchmark datasets at the global scale (Hantson et al., 2016; Rabin et al., 2017; Forkel et al., 2019). Many of these models, like FATES, use simplified processes, such as aggregated area burned rather than individual fires and fire spread, due to challenges in representing the complexity of how fire behavior changes from the flame scale to fire event and the coupling and interactions between those scales (Hantson et al., 2020). Similar to the reductions in tree area when including fire seen in this study, a multi-model global assessment of fire-induced tree cover change demonstrated a consistent reduction with the most significant losses in savanna regions with low tree cover and high burned area compared to simulations without fire disturbance (Lasslop et al., 2020). Expanding and encouraging further development in fire-enabled dynamic vegetation models is an important step towards improving our ability to represent future fire behavior and effects in a fully coupled ESM. The inclusion of anthropogenic impacts and their improved characterization within global fire models is an important uncertainty and opportunity for fire-enabled models like FATES (Jones and Tingley, 2022; Teckentrup et al., 2019; Venevsky et al., 2019; Forkel et al., 2019; Chuvieco et al., 2021). With the current representation of anthropogenic impacts, Burton et al. (2022) used the dynamic vegetation model JULES-ES to demonstrate that across South America under various future scenarios with increases in temperature and CO2 the increases in burned area and reductions in biomass and tree area imply that there is the potential for enhanced drying across the region. The representation of vegetation–fire–climate feedbacks is crucial to exploring these types of land–atmosphere interactions.
Our study focuses specifically on the trait trade-off between fire-tolerant and fire-vulnerable trees and their competition with C4 grass in changing conditions of fuel dryness. The FATES–SPITFIRE fire module includes impacts on size structure, fuel and fire characteristics, and fire behavior in a dynamic framework, all of which are critical components to capturing fire behavior and effects in this system (Balch et al., 2015; Brando et al., 2012; Cochrane et al., 1999). The use of generalized PFT parameters is not meant to capture detailed site-level responses, but rather potential biogeography across the region. The dynamic vegetation–fire feedback as displayed through shifts in biogeophysical and biogeochemical properties and fire behavior in response to vegetation shifts (Figs. 3, S4, and S5) highlights the utility of this framework in exploring feedbacks and interactions. Simulated size-structured mortality and fire intensity across the Amazon and South America were representative of observations (Brando et al., 2016; Balch et al., 2015; Hoffmann et al., 2009), suggesting that FATES is capturing the mechanism of size-structured mortality for the region (Fig. 8). Though the results demonstrated a high bias for biomass accumulation in areas of low disturbance, in fire-disturbed areas the importance of these competitive trade-offs was evident through the variable dominance of PFTs across different fuel drying parameterizations (Fig. 7) in relation to increased fire intensity and burned area (Fig. S15). Our results showed increased fire behavior and effects with a transition to grasses that supports an increase in flammable fine fuels and fire intensity and are consistent with field measurements (Hoffmann et al., 2012; Balch et al., 2015; Brando et al., 2012; Higgins et al., 2000; Govender et al., 2006; Williams et al., 2003; Kauffman et al., 1994). Our work is also consistent with that of a process-based model of forest growth and fire effects in demonstrating that the drier parts of the Amazon are vulnerable to grass conversion in response to changing disturbance drivers (De Faria et al., 2021). Additional modeling work has considered the balance of trees and grasses in tropical forest–savanna–grassland transition areas, and all studies agree that fire is an essential factor in simulating the dominance of grasses in fire-prone areas (Scheiter and Higgins, 2009; Bond et al., 2003; Bond and Midgley, 2012b; Baudena et al., 2015; Blanco et al., 2014). Though our study does not examine the dynamic response to climate change, the variable fuel drying parameterizations provide a proxy for fuel response to altered climate (Fig. S1) and suggest that drier fuels support increased dominance of grassland. Conversion of forest to grassland may increase the risk of fire susceptibility in regions globally (Bowman et al., 2020). Further, the association of grasses with higher fire intensities and the high rate of size-related mortality for fire intensities above 150 kW m−1 suggest that a return to a forest state after grass conversion may be challenging for fire-vulnerable trees of the Amazon.
The incorporation of size structure and its interaction with a process-based fire behavior and effects module, as in this study, adds a level of complexity that allows for improved exploration of the impacts of fire and vegetation structure on ecosystem resilience and functioning. Under the same climate forcing, drier fuels promoted increases in fire behavior that allowed grasses to establish and support regular fire occurrence in areas suitable for tree establishment. The results support a positive grass–fire feedback and are in agreement with modeling studies from an array of models of variable complexity (Baudena et al., 2015; Blanco et al., 2014; De Faria et al., 2021; Bond et al., 2005). Previous studies suggest that C4 grasses established and expanded under conditions of fire and low CO2 conditions (Scheiter et al., 2012; Higgins and Scheiter, 2012) and that increasing CO2 potentially favors trees over C4 grasses (Bond et al., 2003; Bond and Midgley, 2012a). Elevated CO2 increases carbon assimilation and plant water-use efficiency (Swann et al., 2016) and could thus favor C3 plants, but drought may offset this by maintaining or increasing flammability (Bowman et al., 2020). FATES is well positioned to explore the interaction between CO2, drought, and flammability through the process-based representation of fire, interaction between aboveground and belowground processes impacting soil moisture, and simulation of leaf-level responses to altered CO2. Further, though there are general structural similarities, the interactions and feedbacks of fire on savanna and grassland systems vary across continents through variation in vegetation traits (Bond et al., 2003; Hoffmann et al., 2003) and belowground site conditions; therefore, vegetation response to altered climate and fire disturbance should not be assumed to be consistent across regions (Scheiter et al., 2013; Buis et al., 2009; Bond et al., 2005; Lehmann et al., 2011).
Fire has a clear role in determining the biogeography of forests, savannas, and grasslands across the tropics. Shifts in vegetation type and structure across the Amazon have implications for the global coupled climate system with impacts on water cycling and climate regulation through altered albedo, increased drying associated with forest degradation, decreased resilience, and carbon sequestration capacity of Amazon forests (Artaxo et al., 2022a, b; Hubau et al., 2020; Lawrence et al., 2022). This work advances our ability to capture dynamic ecosystem assembly and the potential for shifts in vegetation state and structure in response to climate–fire–vegetation feedbacks. The Amazon forest ecosystem is coupled across scales via feedbacks between vegetation and climate – a significant conversion of forest affects regional climate, which then feeds back on forest ecosystems, potentially driving further degradation. Future modeling work that captures changes in climatic conditions at forest edges and within stand microclimate would help to incorporate the influence of forest degradation, which is increasing globally (Brando et al., 2019; Baccini et al., 2017; Silva Junior et al., 2020). Capturing this in a modeling context would allow more detailed exploration of the interaction between land-clearing activities, such as logging or agricultural conversion, and forest degradation and could quantify potential future impacts of degradation on carbon cycling and forest flammability under scenarios of deforestation and altered climate. Representing fire in the context of interactions between the social environment, the physical environment, and the policy sphere is an essential advance for the current generation of fire-enabled land surface models to better inform and support global communities (Shuman et al., 2022).
Because FATES explicitly tracks size-based competition and mortality as well as the feedback between vegetation and fire, it can be used to explore the response of the system to fire under variable conditions. The results presented here demonstrated a long-term response of the system to consistent fire disturbance under stable climate and CO2 conditions without anthropogenic influences. The mechanisms demonstrated in this study provide a foundation for exploring the impacts of each of these factors on vegetation biogeography as well as fire behavior and effects. Results suggest that drier fuels promote a positive grass–fire feedback, increased fire behavior and characteristics, and an overall loss of biomass, as fire-tolerant vegetation with lower biomass accumulation rates has a competitive advantage under increased disturbance. Increased fire intensity and area burned are associated with areas that have less than 2500 mm of annual rainfall, whereas higher-rainfall regions have consistently higher tree cover and low-intensity surface fires characteristic of understory fires observed in the Amazon. Though the simulations capture appropriate size-structured tree mortality due to low-intensity fires, these results highlight the need for the incorporation of crown fire behavior to capture the potential for high-intensity fires observed in regions such as Australia. This study further confirms that vegetation traits associated with fire-tolerance adaptations, size-level interactions, and vegetation–fire feedbacks are important in capturing the response of ecosystems to fire disturbance.
The code is available at https://doi.org/10.5281/zenodo.10652358 (FATES Development Team, 2024). The parameter file, analysis scripts, and output files are archived at https://doi.org/10.15486/ngt/1992487 (Shuman, 2023). GSWP3 data used to force the model are available at https://doi.org/10.20783/DIAS.501 (Hyungjun, 2017; Dirmeyer et al., 2006).
The supplement related to this article is available online at: https://doi.org/10.5194/gmd-17-4643-2024-supplement.
JKS, RAF, CK, and RK developed the code. JKS set up the model, performed simulations, and prepared the figures. CX prepared the C4 grass parameterization. JKS performed the analysis with inputs from RAF, CK, and LK. JKS wrote the paper with contributions from all co-authors.
The contact author has declared that none of the authors has any competing interests.
Publisher's note: Copernicus Publications remains neutral with regard to jurisdictional claims made in the text, published maps, institutional affiliations, or any other geographical representation in this paper. While Copernicus Publications makes every effort to include appropriate place names, the final responsibility lies with the authors.
Jacquelyn K. Shuman, Rosie A. Fisher, Charles Koven, Ryan Knox, Lara Kueppers, and Chonggang Xu were supported as part of the Next-Generation Ecosystem Experiments – Tropics, funded by the US Department of Energy, Office of Science, Office of Biological and Environmental Research. We would like to acknowledge high-performance computing support from Cheyenne (https://doi.org/10.5065/D6RX99HX, Computational and Information Systems Laboratory, 2019) provided by NCAR's Computational and Information Systems Laboratory, sponsored by the National Science Foundation. We want to acknowledge the reviewers for their careful and thorough evaluation of the manuscript and their extensive and helpful comments which helped to improve the final text.
This research has been supported by the Next-Generation Ecosystem Experiments – Tropics, funded by the US Department of Energy, Office of Science, Office of Biological and Environmental Research. Jacquelyn K. Shuman was also supported by the National Center for Atmospheric Research, a major facility sponsored by the National Science Foundation (NSF), under cooperative agreement no. 1852977, and by NASA's FireSense project under the direction of Michael Falkowski. Rosie A. Fisher was also supported by the European Union's Horizon 2020 (H2020) research and innovation program under grant agreement nos. 101003536 (ESM2025 – Earth System Models for the Future) and 821003 (4C, Climate–Carbon Interactions in the Coming Century).
This paper was edited by Hisashi Sato and reviewed by Huilin Huang and Chao Yue.
Alencar, A., Asner, G. P., Knapp, D., and Zarin, D.: Temporal variability of forest fires in eastern Amazonia, Ecol. Appl., 21, 2397–2412, https://doi.org/10.1890/10-1168.1, 2011.
Andrews, P. L.: The Rothermel surface fire spread model and associated developments: A comprehensive explanation, U. S. Department of Agriculture, Forest Service, Rocky Mountain Research Station, Ft. Collins, CO, https://doi.org/10.2737/RMRS-GTR-371, 2018.
Aragão, L. E. O. C., Anderson, L. O., Fonseca, M. G., Rosan, T. M., Vedovato, L. B., Wagner, F. H., Silva, C. V. J., Silva Junior, C. H. L., Arai, E., Aguiar, A. P., Barlow, J., Berenguer, E., Deeter, M. N., Domingues, L. G., Gatti, L., Gloor, M., Malhi, Y., Marengo, J. A., Miller, J. B., Phillips, O. L., and Saatchi, S.: 21st Century drought-related fires counteract the decline of Amazon deforestation carbon emissions, Nat. Commun., 9, 536, https://doi.org/10.1038/s41467-017-02771-y, 2018.
Archibald, S., Lehmann, C. E. R., Gómez-Dans, J. L., and Bradstock, R. A.: Defining pyromes and global syndromes of fire regimes, P. Natl. Acad. Sci. USA, 110, 6442–6447, https://doi.org/10.1073/pnas.1211466110, 2013.
Arellano, G., Zuleta, D., and Davies, S. J.: Tree death and damage: A standardized protocol for frequent surveys in tropical forests, J. Veg. Sci., 32, e12981, https://doi.org/10.1111/jvs.12981, 2021.
Arora, V. K. and Boer, G. J.: Fire as an interactive component of dynamic vegetation models: Fire in Dynamic Vegetation Models, J. Geophys. Res., 110, G02008, https://doi.org/10.1029/2005JG000042, 2005.
Artaxo, P., Hansson, H.-C., Andreae, M. O., Bäck, J., Alves, E. G., Barbosa, H. M. J., Bender, F., Bourtsoukidis, E., Carbone, S., Chi, J., Decesari, S., Després, V. R., Ditas, F., Ezhova, E., Fuzzi, S., Hasselquist, N. J., Heintzenberg, J., Holanda, B. A., Guenther, A., Hakola, H., Heikkinen, L., Kerminen, V.-M., Kontkanen, J., Krejci, R., Kulmala, M., Lavric, J. V., de Leeuw, G., Lehtipalo, K., Machado, L. A. T., McFiggans, G., Franco, M. A. M., Meller, B. B., Morais, F. G., Mohr, C., Morgan, W., Nilsson, M. B., Peichl, M., Petäjä, T., Praß, M., Pöhlker, C., Pöhlker, M. L., Pöschl, U., Von Randow, C., Riipinen, I., Rinne, J., V. Rizzo, L., Rosenfeld, D., Dias, M. A. F. S., Sogacheva, L., Stier, P., Swietlicki, E., Sörgel, M., Tunved, P., Virkkula, A., Wang, J., Weber, B., Yáñez-Serrano, A. M., Zieger, P., Mikhailov, E., Smith, J. N., and Kesselmeier, J.: Tropical and Boreal Forest – Atmosphere Interactions: A Review, Tellus B, 74, 24–163, https://doi.org/10.16993/tellusb.34, 2022a.
Artaxo, P., Hansson, H. C., Machado, L. A. T., and Rizzo, L. V.: Tropical forests are crucial in regulating the climate on Earth, PLOS Clim., 1, e0000054, https://doi.org/10.1371/journal.pclm.0000054, 2022b.
Baccini, A., Walker, W., Carvalho, L., Farina, M., Sulla-Menashe, D., and Houghton, R. A.: Tropical forests are a net carbon source based on aboveground measurements of gain and loss, Science, 358, 230–234, https://doi.org/10.1126/science.aam5962, 2017.
Balch, J. K., Nepstad, D. C., Brando, P. M., Curran, L. M., Portela, O., De Carvalho, O., and Lefebvre, P.: Negative fire feedback in a transitional forest of southeastern Amazonia: Negative Fire Feedback in Southeast Amazonia, Glob. Change Biol., 14, 2276–2287, https://doi.org/10.1111/j.1365-2486.2008.01655.x, 2008.
Balch, J. K., Brando, P. M., Nepstad, D. C., Coe, M. T., Silvério, D., Massad, T. J., Davidson, E. A., Lefebvre, P., Oliveira-Santos, C., Rocha, W., Cury, R. T. S., Parsons, A., and Carvalho, K. S.: The Susceptibility of Southeastern Amazon Forests to Fire: Insights from a Large-Scale Burn Experiment, BioScience, 65, 893–905, https://doi.org/10.1093/biosci/biv106, 2015.
Baudena, M., Dekker, S. C., van Bodegom, P. M., Cuesta, B., Higgins, S. I., Lehsten, V., Reick, C. H., Rietkerk, M., Scheiter, S., Yin, Z., Zavala, M. A., and Brovkin, V.: Forests, savannas, and grasslands: bridging the knowledge gap between ecology and Dynamic Global Vegetation Models, Biogeosciences, 12, 1833–1848, https://doi.org/10.5194/bg-12-1833-2015, 2015.
Blanco, C. C., Scheiter, S., Sosinski, E., Fidelis, A., Anand, M., and Pillar, V. D.: Feedbacks between vegetation and disturbance processes promote long-term persistence of forest–grassland mosaics in south Brazil, Ecol. Model., 291, 224–232, https://doi.org/10.1016/j.ecolmodel.2014.07.024, 2014.
Blyth, E. M., Arora, V. K., Clark, D. B., Dadson, S. J., De Kauwe, M. G., Lawrence, D. M., Melton, J. R., Pongratz, J., Turton, R. H., Yoshimura, K., and Yuan, H.: Advances in Land Surface Modelling, Curr. Clim. Change Rep., 7, 45–71, https://doi.org/10.1007/s40641-021-00171-5, 2021.
Bonan, G. B., Patton, E. G., Finnigan, J. J., Baldocchi, D. D., and Harman, I. N.: Moving beyond the incorrect but useful paradigm: reevaluating big-leaf and multilayer plant canopies to model biosphere-atmosphere fluxes – a review, Agr. Forest Meteorol., 306, 108435, https://doi.org/10.1016/j.agrformet.2021.108435, 2021.
Bond, W. J.: What Limits Trees in C4 Grasslands and Savannas?, Annu. Rev. Ecol. Evol. S., 39, 641–659, https://doi.org/10.1146/annurev.ecolsys.39.110707.173411, 2008.
Bond, W. J. and Midgley, G. F.: Carbon dioxide and the uneasy interactions of trees and savannah grasses, Philos. T. Roy. Soc. B, 367, 601–612, https://doi.org/10.1098/rstb.2011.0182, 2012a.
Bond, W. J. and Midgley, J. J.: Fire and the Angiosperm Revolutions, Int. J. Plant Sci., 173, 569–583, https://doi.org/10.1086/665819, 2012b.
Bond, W. J., Midgley, G. F., and Woodward, F. I.: The importance of low atmospheric CO2 and fire in promoting the spread of grasslands and savannas: FIRE, LOW CO2 and TREES, Glob. Change Biol., 9, 973–982, https://doi.org/10.1046/j.1365-2486.2003.00577.x, 2003.
Bond, W. J., Woodward, F. I., and Midgley, G. F.: The global distribution of ecosystems in a world without fire, New Phytol., 165, 525–538, https://doi.org/10.1111/j.1469-8137.2004.01252.x, 2005.
Bowman, D. M. J. S., Kolden, C. A., Abatzoglou, J. T., Johnston, F. H., van der Werf, G. R., and Flannigan, M.: Vegetation fires in the Anthropocene, Nature Reviews Earth & Environment, 1, 500–515, https://doi.org/10.1038/s43017-020-0085-3, 2020.
Bradshaw, L. S., Deeming, J. E., Burgan, R. E., and Cohen, J. D.: The 1978 National Fire-Danger Rating System: technical documentation. General Technical Report INT-169, U.S. Department of Agriculture, Forest Service, Intermountain Forest and Range Experiment Station, Ogden, UT, 44 pp., https://doi.org/10.2737/INT-GTR-169, 1984.
Brando, P. M., Nepstad, D. C., Balch, J. K., Bolker, B., Christman, M. C., Coe, M., and Putz, F. E.: Fire-induced tree mortality in a neotropical forest: the roles of bark traits, tree size, wood density and fire behavior, Glob. Change Biol., 18, 630–641, https://doi.org/10.1111/j.1365-2486.2011.02533.x, 2012.
Brando, P. M., Oliveria-Santos, C., Rocha, W., Cury, R., and Coe, M. T.: Effects of experimental fuel additions on fire intensity and severity: unexpected carbon resilience of a neotropical forest, Glob. Change Biol., 22, 2516–2525, https://doi.org/10.1111/gcb.13172, 2016.
Brando, P. M., Silvério, D., Maracahipes-Santos, L., Oliveira-Santos, C., Levick, S. R., Coe, M. T., Migliavacca, M., Balch, J. K., Macedo, M. N., Nepstad, D. C., Maracahipes, L., Davidson, E., Asner, G., Kolle, O., and Trumbore, S.: Prolonged tropical forest degradation due to compounding disturbances: Implications for CO2 and H2O fluxes, Glob. Change Biol., 25, 2855–2868, https://doi.org/10.1111/gcb.14659, 2019.
Brando, P. M., Soares-Filho, B., Rodrigues, L., Assunção, A., Morton, D., Tuchschneider, D., Fernandes, E. C. M., Macedo, M. N., Oliveira, U., and Coe, M. T.: The gathering firestorm in southern Amazonia, Sci. Adv., 6, eaay1632, https://doi.org/10.1126/sciadv.aay1632, 2020.
Bucini, G. and Hanan, N. P.: A continental-scale analysis of tree cover in African savannas, Global Ecol. Biogeogr., 16, 593–605, https://doi.org/10.1111/j.1466-8238.2007.00325.x, 2007.
Buis, G. M., Blair, J. M., Burkepile, D. E., Burns, C. E., Chamberlain, A. J., Chapman, P. L., Collins, S. L., Fynn, R. W. S., Govender, N., Kirkman, K. P., Smith, M. D., and Knapp, A. K.: Controls of Aboveground Net Primary Production in Mesic Savanna Grasslands: An Inter-Hemispheric Comparison, Ecosystems, 12, 982–995, https://doi.org/10.1007/s10021-009-9273-1, 2009.
Buotte, P. C., Koven, C. D., Xu, C., Shuman, J. K., Goulden, M. L., Levis, S., Katz, J., Ding, J., Ma, W., Robbins, Z., and Kueppers, L. M.: Capturing functional strategies and compositional dynamics in vegetation demographic models, Biogeosciences, 18, 4473–4490, https://doi.org/10.5194/bg-18-4473-2021, 2021.
Burrows, G. E.: Epicormic strand structure in Angophora, Eucalyptus and Lophostemon (Myrtaceae) – implications for fire resistance and recovery, New Phytol., 153, 111–131, https://doi.org/10.1046/j.0028-646X.2001.00299.x, 2002.
Burton, C., Kelley, D. I., Jones, C. D., Betts, R. A., Cardoso, M., and Anderson, L.: South American fires and their impacts on ecosystems increase with continued emissions, Climate Resilience, 1, e8, https://doi.org/10.1002/cli2.8, 2022.
Chambers, J. Q., Higuchi, N., Schimel, J. P., Ferreira, L. V., and Melack, J. M.: Decomposition and carbon cycling of dead trees in tropical forests of the central Amazon, Oecologia, 122, 380–388, https://doi.org/10.1007/s004420050044, 2000.
Chave, J., Muller-Landau, H. C., Baker, T. R., Easdale, T. A., ter Steege, H., and Webb, C. O.: Regional and Phylogenetic Variation of Wood Density Across 2456 Neotropical Tree Species, Ecol. Appl., 16, 2356–2367, https://doi.org/10.1890/1051-0761(2006)016[2356:RAPVOW]2.0.CO;2, 2006.
Chen, Y.-Y., Gardiner, B., Pasztor, F., Blennow, K., Ryder, J., Valade, A., Naudts, K., Otto, J., McGrath, M. J., Planque, C., and Luyssaert, S.: Simulating damage for wind storms in the land surface model ORCHIDEE-CAN (revision 4262), Geosci. Model Dev., 11, 771–791, https://doi.org/10.5194/gmd-11-771-2018, 2018.
Chuvieco, E., Pettinari, M. L., Koutsias, N., Forkel, M., Hantson, S., and Turco, M.: Human and climate drivers of global biomass burning variability, Sci. Total Environ., 779, 146361, https://doi.org/10.1016/j.scitotenv.2021.146361, 2021.
Cochrane, M. A.: Fire science for rainforests, Nature, 421, 913–919, https://doi.org/10.1038/nature01437, 2003.
Cochrane, M. A., Alencar, A., Schulze, M. D., Souza, C. M., Nepstad, D. C., Lefebvre, P., and Davidson, E. A.: Positive Feedbacks in the Fire Dynamic of Closed Canopy Tropical Forests, Science, 284, 1832–1835, https://doi.org/10.1126/science.284.5421.1832, 1999.
Collier, N., Hoffman, F. M., Lawrence, D. M., Keppel-Aleks, G., Koven, C. D., Riley, W. J., Mu, M., and Randerson, J. T.: The International Land Model Benchmarking (ILAMB) System: Design, Theory, and Implementation, J. Adv. Model. Earth Sy., 10, 2731–2754, https://doi.org/10.1029/2018MS001354, 2018.
Compo, G. P., Whitaker, J. S., Sardeshmukh, P. D., Matsui, N., Allan, R. J., Yin, X., Gleason Jr., B. E., Vose, R. S., Rutledge, G., Bessemoulin, P., Brönnimann, S., Brunet, M., Crouthamel, R. I., Grant, A. N., Groisman, P. Y., Jones, P. D., Kruk, M. C., Kruger, A. C., Marshall, G. J., Maugeri, M., Mok, H. Y., Nordli, Ø., Ross, T. F., Trigo, R. M., Wang, X. L., Woodruff, S. D., and Worley, S. J.: The Twentieth Century Reanalysis Project, Q. J. Roy. Meteor. Soc., 137, 1–28, https://doi.org/10.1002/qj.776, 2011.
Computational and Information Systems Laboratory: Cheyenne: HPE/SGI ICE XA System (NCAR Community Computing), National Center for Atmospheric Research, Boulder, CO, https://doi.org/10.5065/D6RX99HX, 2019.
Crisp, M. D., Burrows, G. E., Cook, L. G., Thornhill, A. H., and Bowman, D. M. J. S.: Flammable biomes dominated by eucalypts originated at the Cretaceous–Palaeogene boundary, Nat. Commun., 2, 193, https://doi.org/10.1038/ncomms1191, 2011.
Daehler, C. C., Anttila, C. K., Ayres, D. R., Strong, D. R., and Bailey, J. P.: Evolution of a new ecotype of Spartina alterniflora (Poaceae) in San Francisco Bay, California, USA, Am. J. Bot., 86, 543–546, https://doi.org/10.2307/2656815, 1999.
Danabasoglu, G., Lamarque, J.-F., Bacmeister, J., Bailey, D. A., DuVivier, A. K., Edwards, J., Emmons, L. K., Fasullo, J., Garcia, R., Gettelman, A., Hannay, C., Holland, M. M., Large, W. G., Lauritzen, P. H., Lawrence, D. M., Lenaerts, J. T. M., Lindsay, K., Lipscomb, W. H., Mills, M. J., Neale, R., Oleson, K. W., Otto-Bliesner, B., Phillips, A. S., Sacks, W., Tilmes, S., Kampenhout, L., Vertenstein, M., Bertini, A., Dennis, J., Deser, C., Fischer, C., Fox-Kemper, B., Kay, J. E., Kinnison, D., Kushner, P. J., Larson, V. E., Long, M. C., Mickelson, S., Moore, J. K., Nienhouse, E., Polvani, L., Rasch, P. J., and Strand, W. G.: The Community Earth System Model Version 2 (CESM2), J. Adv. Model. Earth Sy., 12, e2019MS00191, https://doi.org/10.1029/2019MS001916, 2020.
De Faria, B. L., Staal, A., Silva, C. A., Martin, P. A., Panday, P. K., Dantas, V. L., and Silva, T.: Climate change and deforestation increase the vulnerability of Amazonian forests to post-fire grass invasion, Global Ecol. Biogeogr., 30, 2368–2381, https://doi.org/10.1111/geb.13388, 2021.
De Kauwe, M. G., Disney, M. I., Quaife, T., Lewis, P., and Williams, M.: An assessment of the MODIS collection 5 leaf area index product for a region of mixed coniferous forest, Remote Sens. Environ., 115, 767–780, https://doi.org/10.1016/j.rse.2010.11.004, 2011.
Dirmeyer, P. A., Gao, X., Zhao, M., Guo, Z., Oki, T., and Hanasaki, N.: GSWP-2: Multimodel Analysis and Implications for Our Perception of the Land Surface, B. Am. Meteorol. Soc., 87, 1381–1398, 2006.
Drüke, M., Forkel, M., von Bloh, W., Sakschewski, B., Cardoso, M., Bustamante, M., Kurths, J., and Thonicke, K.: Improving the LPJmL4-SPITFIRE vegetation–fire model for South America using satellite data, Geosci. Model Dev., 12, 5029–5054, https://doi.org/10.5194/gmd-12-5029-2019, 2019.
Eaton, J. M. and Lawrence, D.: Woody debris stocks and fluxes during succession in a dry tropical forest, Forest Ecol. Manag., 232, 46–55, https://doi.org/10.1016/j.foreco.2006.05.038, 2006.
FATES Development Team: The Functionally Assembled Terrestrial Ecosystem Simulator (FATES) (fates-spitfire-ms), Zenodo [code], https://doi.org/10.5281/zenodo.10652358, 2024.
Fidelis, A., Delgado-Cartay, M. D., Blanco, C. C., Müller, S. C., Pillar, V. D., and Pfadenhauer, J.: Fire Intensity and Severity in Brazilian Campos Grasslands, Interciencia, 35, 739–745, 2010.
Fisher, R., McDowell, N., Purves, D., Moorcroft, P., Sitch, S., Cox, P., Huntingford, C., Meir, P., and Woodward, F. I.: Assessing uncertainties in a second-generation dynamic vegetation model caused by ecological scale limitations, New Phytol., 187, 666–681, https://doi.org/10.1111/j.1469-8137.2010.03340.x, 2010.
Fisher, R. A., Muszala, S., Verteinstein, M., Lawrence, P., Xu, C., McDowell, N. G., Knox, R. G., Koven, C., Holm, J., Rogers, B. M., Spessa, A., Lawrence, D., and Bonan, G.: Taking off the training wheels: the properties of a dynamic vegetation model without climate envelopes, CLM4.5(ED), Geosci. Model Dev., 8, 3593–3619, https://doi.org/10.5194/gmd-8-3593-2015, 2015.
Fisher, R. A., Koven, C. D., Anderegg, W. R. L., Christoffersen, B. O., Dietze, M. C., Farrior, C. E., Holm, J. A., Hurtt, G. C., Knox, R. G., Lawrence, P. J., Lichstein, J. W., Longo, M., Matheny, A. M., Medvigy, D., Muller-Landau, H. C., Powell, T. L., Serbin, S. P., Sato, H., Shuman, J. K., Smith, B., Trugman, A. T., Viskari, T., Verbeeck, H., Weng, E., Xu, C., Xu, X., Zhang, T., and Moorcroft, P. R.: Vegetation demographics in Earth System Models: A review of progress and priorities, Glob. Change Biol., 24, 35–54, https://doi.org/10.1111/gcb.13910, 2018.
Forestry Canada Fire Danger Group: Development and structure of the Canadian Forest Fire Behavior Prediction System. For. Can., Ottawa, Ont. Inf. Rep. ST-X-3. 63 pp., 1992.
Forkel, M., Andela, N., Harrison, S. P., Lasslop, G., van Marle, M., Chuvieco, E., Dorigo, W., Forrest, M., Hantson, S., Heil, A., Li, F., Melton, J., Sitch, S., Yue, C., and Arneth, A.: Emergent relationships with respect to burned area in global satellite observations and fire-enabled vegetation models, Biogeosciences, 16, 57–76, https://doi.org/10.5194/bg-16-57-2019, 2019.
Fosberg, M. A. and Deeming, J. E.: Derivation of the 1- and 10-hour timelag fuel moisture calculations for fire-danger rating. Research Note RM-RN-207, USDA Forest Service, Rocky Mountain Forest and Range Experiment Station, Fort Collins, CO, 8 pp., 1971.
Giglio, L., Randerson, J. T., and van der Werf, G. R.: Analysis of daily, monthly, and annual burned area using the fourth-generation global fire emissions database (GFED4): Analysis of Burned Area, J. Geophys. Res.-Biogeo., 118, 317–328, https://doi.org/10.1002/jgrg.20042, 2013.
Gignoux, J., Clobert, J., and Menaut, J.-C.: Alternative fire resistance strategies in savanna trees, Oecologia, 110, 576–583, 1997.
Giurgevich, J. R. and Dunn, E. L.: Seasonal Patterns of CO2 and Water Vapor Exchange of the Tall and Short Height Forms of Spartina aiterniflora Loisel in a Georgia Salt Marsh, Oecologia, 43, 139–156, 1979.
Golaz, J., Caldwell, P. M., Van Roekel, L. P., Petersen, M. R., Tang, Q., Wolfe, J. D., Abeshu, G., Anantharaj, V., Asay-Davis, X. S., Bader, D. C., Baldwin, S. A., Bisht, G., Bogenschutz, P. A., Branstetter, M., Brunke, M. A., Brus, S. R., Burrows, S. M., Cameron-Smith, P. J., Donahue, A. S., Deakin, M., Easter, R. C., Evans, K. J., Feng, Y., Flanner, M., Foucar, J. G., Fyke, J. G., Griffin, B. M., Hannay, C., Harrop, B. E., Hoffman, M. J., Hunke, E. C., Jacob, R. L., Jacobsen, D. W., Jeffery, N., Jones, P. W., Keen, N. D., Klein, S. A., Larson, V. E., Leung, L. R., Li, H., Lin, W., Lipscomb, W. H., Ma, P., Mahajan, S., Maltrud, M. E., Mametjanov, A., McClean, J. L., McCoy, R. B., Neale, R. B., Price, S. F., Qian, Y., Rasch, P. J., Reeves Eyre, J. E. J., Riley, W. J., Ringler, T. D., Roberts, A. F., Roesler, E. L., Salinger, A. G., Shaheen, Z., Shi, X., Singh, B., Tang, J., Taylor, M. A., Thornton, P. E., Turner, A. K., Veneziani, M., Wan, H., Wang, H., Wang, S., Williams, D. N., Wolfram, P. J., Worley, P. H., Xie, S., Yang, Y., Yoon, J., Zelinka, M. D., Zender, C. S., Zeng, X., Zhang, C., Zhang, K., Zhang, Y., Zheng, X., Zhou, T., and Zhu, Q.: The DOE E3SM Coupled Model Version 1: Overview and Evaluation at Standard Resolution, J. Adv. Model. Earth Sy., 11, 2089–2129, https://doi.org/10.1029/2018MS001603, 2019.
Govender, N., Trollope, W. S. W., and Van Wilgen, B. W.: The effect of fire season, fire frequency, rainfall and management on fire intensity in savanna vegetation in South Africa: Fire intensity in savanna, J. Appl. Ecol., 43, 748–758, https://doi.org/10.1111/j.1365-2664.2006.01184.x, 2006.
Gross, M. F., Hardisky, M. A., Wolf, P. L., and Klemas, V.: Relationship between Aboveground and Belowground Biomass of Spartina alterniflora (Smooth Cordgrass), Estuaries, 14, 180, https://doi.org/10.2307/1351692, 1991.
Haas, O., Prentice, I. C., and Harrison, S. P.: Global environmental controls on wildfire burnt area, size, and intensity, Environ. Res. Lett., 17, 065004, https://doi.org/10.1088/1748-9326/ac6a69, 2022.
Hanan, E. J., Kennedy, M. C., Ren, J., Johnson, M. C., and Smith, A. M. S.: Missing Climate Feedbacks in Fire Models: Limitations and Uncertainties in Fuel Loadings and the Role of Decomposition in Fine Fuel Accumulation, J. Adv. Model. Earth Sy., 14, e2021MS002818, https://doi.org/10.1029/2021MS002818, 2022.
Hantson, S., Arneth, A., Harrison, S. P., Kelley, D. I., Prentice, I. C., Rabin, S. S., Archibald, S., Mouillot, F., Arnold, S. R., Artaxo, P., Bachelet, D., Ciais, P., Forrest, M., Friedlingstein, P., Hickler, T., Kaplan, J. O., Kloster, S., Knorr, W., Lasslop, G., Li, F., Mangeon, S., Melton, J. R., Meyn, A., Sitch, S., Spessa, A., van der Werf, G. R., Voulgarakis, A., and Yue, C.: The status and challenge of global fire modelling, Biogeosciences, 13, 3359–3375, https://doi.org/10.5194/bg-13-3359-2016, 2016.
Hantson, S., Kelley, D. I., Arneth, A., Harrison, S. P., Archibald, S., Bachelet, D., Forrest, M., Hickler, T., Lasslop, G., Li, F., Mangeon, S., Melton, J. R., Nieradzik, L., Rabin, S. S., Prentice, I. C., Sheehan, T., Sitch, S., Teckentrup, L., Voulgarakis, A., and Yue, C.: Quantitative assessment of fire and vegetation properties in simulations with fire-enabled vegetation models from the Fire Model Intercomparison Project, Geosci. Model Dev., 13, 3299–3318, https://doi.org/10.5194/gmd-13-3299-2020, 2020.
Haverd, V., Smith, B., Nieradzik, L., Briggs, P. R., Woodgate, W., Trudinger, C. M., Canadell, J. G., and Cuntz, M.: A new version of the CABLE land surface model (Subversion revision r4601) incorporating land use and land cover change, woody vegetation demography, and a novel optimisation-based approach to plant coordination of photosynthesis, Geosci. Model Dev., 11, 2995–3026, https://doi.org/10.5194/gmd-11-2995-2018, 2018.
Higgins, S. I. and Scheiter, S.: Atmospheric CO2 forces abrupt vegetation shifts locally, but not globally, Nature, 488, 209–212, https://doi.org/10.1038/nature11238, 2012.
Higgins, S. I., Bond, W. J., and Trollope, W. S. W.: Fire, resprouting and variability: a recipe for grass-tree coexistence in savanna, J. Ecol., 88, 213–229, https://doi.org/10.1046/j.1365-2745.2000.00435.x, 2000.
Hirota, M., Holmgren, M., Van Nes, E. H., and Scheffer, M.: Global Resilience of Tropical Forest and Savanna to Critical Transitions, Science, 334, 232–235, https://doi.org/10.1126/science.1210657, 2011.
Hoffmann, W. A.: Post-Establishment Seedling Success in the Brazilian Cerrado: A Comparison of Savanna and Forest Species, Biotropica, 32, 62–69, 2000.
Hoffmann, W. A. and Solbrig, O. T.: The role of topkill in the differential response of savanna woody species to fire, Forest Ecol. Manag., 180, 273–286, https://doi.org/10.1016/S0378-1127(02)00566-2, 2003.
Hoffmann, W. A., Orthen, B., and do Nascimento, P. K. V.: Comparative fire ecology of tropical savanna and forest trees: Fire traits of savanna and forest trees, Funct. Ecol., 17, 720–726, https://doi.org/10.1111/j.1365-2435.2003.00796.x, 2003.
Hoffmann, W. A., Adasme, R., Haridasan, M., de Carvalho, M. T., Geiger, E. L., Pereira, M. A. B., Gotsch, S. G., and Franco, A. C.: Tree topkill, not mortality, governs the dynamics of savanna–forest boundaries under frequent fire in central Brazil, Ecology, 90, 1326–1337, https://doi.org/10.1890/08-0741.1, 2009.
Hoffmann, W. A., Geiger, E. L., Gotsch, S. G., Rossatto, D. R., Silva, L. C. R., Lau, O. L., Haridasan, M., and Franco, A. C.: Ecological thresholds at the savanna-forest boundary: how plant traits, resources and fire govern the distribution of tropical biomes, Ecol. Lett., 15, 759–768, https://doi.org/10.1111/j.1461-0248.2012.01789.x, 2012.
Hoffmann, W. A., Sanders, R. W., Just, M. G., Wall, W. A., and Hohmann, M. G.: Better lucky than good: How savanna trees escape the fire trap in a variable world, Ecology, 101, e02895, https://doi.org/10.1002/ecy.2895, 2020.
Hubau, W., Lewis, S. L., Phillips, O. L., Affum-Baffoe, K., Beeckman, H., Cuní-Sanchez, A., Daniels, A. K., Ewango, C. E. N., Fauset, S., Mukinzi, J. M., Sheil, D., Sonké, B., Sullivan, M. J. P., Sunderland, T. C. H., Taedoumg, H., Thomas, S. C., White, L. J. T., Abernethy, K. A., Adu-Bredu, S., Amani, C. A., Baker, T. R., Banin, L. F., Baya, F., Begne, S. K., Bennett, A. C., Bengone, N. N., Benedet, F., Bitariho, R., Bocko, Y. E., Boeckx, P., Boundja, P., Brienen, R. J. W., Brncic, T., Chezeaux, E., Chuyong, G. B., Clark, C. J., Collins, M., Comiskey, J. A., Coomes, D. A., Dargie, G. C., de Haulleville, T., Kamdem, M. N. D., Doucet, J.-L., Esquivel-Muelbert, A., Feldpausch, T. R., Fofanah, A., Foli, E. G., Gilpin, M., Gloor, E., Gonmadje, C., Gourlet-Fleury, S., Hall, J. S., Hamilton, A. C., Harris, D. J., Hart, T. B., Hockemba, M. B. N., Hladik, A., Ifo, S. A., Jeffery, K. J., Jucker, T., Yakusu, E. K., Kearsley, E., Kenfack, D., Koch, A., Leal, M. E., Levesley, A., Lindsell, J. A., Lisingo, J., Lopez-Gonzalez, G., Lovett, J. C., Makana, J.-R., Malhi, Y., Marshall, A. R., Martin, J., Martin, E. H., Mbayu, F. M., Medjibe, V. P., Mihindou, V., Mitchard, E. T. A., Moore, S., Munishi, P. K. T., Ojo, L., Ondo, F. E., Peh, K. S.-H., Pickavance, G. C., Poulsen, A. D., Poulsen, J. R., Qie, L., Reitsma, J., Rovero, F., Swaine, M. D., Talbot, J., Taplin, J., Taylor, D. M., Thomas, D. W., Toirambe, B., Tshibamba Mukendi, J., Tuagben, D., Umunay, P. M., van der Heijden, G. M. F., Verbeeck, H., Vleminckx, J., Wilcock, S., Woll, H., Woods, J. T., and Zemagho, L.: Asynchronous carbon sink saturation in African and Amazonian tropical forests, Nature, 579, 80–87, https://doi.org/10.1038/s41586-020-2035-0, 2020.
Hyungjun, K.: Global Soil Wetness Project Phase 3 Atmospheric Boundary Conditions (Experiment 1), Data Integration and Analysis System (DIAS) [data set], https://doi.org/10.20783/DIAS.501, 2017.
Jain, P., Castellanos-Acuna, D., Coogan, S. C. P., Abatzoglou, J. T., and Flannigan, M. D.: Observed increases in extreme fire weather driven by atmospheric humidity and temperature, Nat. Clim. Change, 12, 63–70, https://doi.org/10.1038/s41558-021-01224-1, 2022.
Jolly, W. M., Cochrane, M. A., Freeborn, P. H., Holden, Z. A., Brown, T. J., Williamson, G. J., and Bowman, D. M. J. S.: Climate-induced variations in global wildfire danger from 1979 to 2013, Nat. Commun., 6, 7537, https://doi.org/10.1038/ncomms8537, 2015.
Jones, G. M. and Tingley, M. W.: Pyrodiversity and biodiversity: A history, synthesis, and outlook, Divers. Distrib., 28, 386–403, https://doi.org/10.1111/ddi.13280, 2022.
Jones, M. W., Abatzoglou, J. T., Veraverbeke, S., Andela, N., Lasslop, G., Forkel, M., Smith, A. J. P., Burton, C., Betts, R. A., van der Werf, G. R., Sitch, S., Canadell, J. G., Santín, C., Kolden, C., Doerr, S. H., and Le Quéré, C.: Global and Regional Trends and Drivers of Fire Under Climate Change, Rev. Geophys., 60, e2020RG000726, https://doi.org/10.1029/2020RG000726, 2022.
Jung, M., Reichstein, M., Ciais, P., Seneviratne, S. I., Sheffield, J., Goulden, M. L., Bonan, G., Cescatti, A., Chen, J., de Jeu, R., Dolman, A. J., Eugster, W., Gerten, D., Gianelle, D., Gobron, N., Heinke, J., Kimball, J., Law, B. E., Montagnani, L., Mu, Q., Mueller, B., Oleson, K., Papale, D., Richardson, A. D., Roupsard, O., Running, S., Tomelleri, E., Viovy, N., Weber, U., Williams, C., Wood, E., Zaehle, S., and Zhang, K.: Recent decline in the global land evapotranspiration trend due to limited moisture supply, Nature, 467, 951–954, https://doi.org/10.1038/nature09396, 2010.
Kattge, J., Knorr, W., Raddatz, T., and Wirth, C.: Quantifying photosynthetic capacity and its relationship to leaf nitrogen content for global-scale terrestrial biosphere models, Glob. Change Biol., 15, 976–991, https://doi.org/10.1111/j.1365-2486.2008.01744.x, 2009.
Kattge, J., Díaz, S., Lavorel, S., Prentice, I. C., Leadley, P., Bönisch, G., Garnier, E., Westoby, M., Reich, P. B., Wright, I. J., Cornelissen, J. H. C., Violle, C., Harrison, S. P., Van Bodegom, P. M., Reichstein, M., Enquist, B. J., Soudzilovskaia, N. A., Ackerly, D. D., Anand, M., Atkin, O., Bahn, M., Baker, T. R., Baldocchi, D., Bekker, R., Blanco, C. C., Blonder, B., Bond, W. J., Bradstock, R., Bunker, D. E., Casanoves, F., Cavender-Bares, J., Chambers, J. Q., Chapin III, F. S., Chave, J., Coomes, D., Cornwell, W. K., Craine, J. M., Dobrin, B. H., Duarte, L., Durka, W., Elser, J., Esser, G., Estiarte, M., Fagan, W. F., Fang, J., Fernández-Méndez, F., Fidelis, A., Finegan, B., Flores, O., Ford, H., Frank, D., Freschet, G. T., Fyllas, N. M., Gallagher, R. V., Green, W. A., Gutierrez, A. G., Hickler, T., Higgins, S. I., Hodgson, J. G., Jalili, A., Jansen, S., Joly, C. A., Kerkhoff, A. J., Kirkup, D., Kitajima, K., Kleyer, M., Klotz, S., Knops, J. M. H., Kramer, K., Kühn, I., Kurokawa, H., Laughlin, D., Lee, T. D., Leishman, M., Lens, F., Lenz, T., Lewis, S. L., Lloyd, J., Llusià, J., Louault, F., Ma, S., Mahecha, M. D., Manning, P., Massad, T., Medlyn, B. E., Messier, J., Moles, A. T., Müller, S. C., Nadrowski, K., Naeem, S., Niinemets, Ü., Nöllert, S., Nüske, A., Ogaya, R., Oleksyn, J., Onipchenko, V. G., Onoda, Y., Ordoñez, J., Overbeck, G., Ozinga, W. A., Patiño, S., Paula, S., Pausas, J. G., Peñuelas, J., Phillips, O. L., Pillar, V., Poorter, H., Poorter, L., Poschlod, P., Prinzing, A., Proulx, R., Rammig, A., Reinsch, S., Reu, B., Sack, L., Salgado‐Negret, B., Sardans, J., Shiodera, S., Shipley, B.,Siefert, A., Sosinski, E., Soussana, J. F., Swaine, E., Swenson, N., Thompson, K., Thornton, P., Waldram, M., Weiher, E., White, M., White, S., Wright, S. J., Yguel, B., Zaehle, S., Zanne, A. E., and Wirth, C: TRY – a global database of plant traits, Glob. Change Biol., 17, 2905–2935, https://doi.org/10.1111/j.1365-2486.2011.02451.x, 2011.
Kauffman, J. B., Cummings, D. L., and Ward, D. E.: Relationships of Fire, Biomass and Nutrient Dynamics along a Vegetation Gradient in the Brazilian Cerrado, J. Ecol., 82, 519, https://doi.org/10.2307/2261261, 1994.
Koven, C. D., Knox, R. G., Fisher, R. A., Chambers, J. Q., Christoffersen, B. O., Davies, S. J., Detto, M., Dietze, M. C., Faybishenko, B., Holm, J., Huang, M., Kovenock, M., Kueppers, L. M., Lemieux, G., Massoud, E., McDowell, N. G., Muller-Landau, H. C., Needham, J. F., Norby, R. J., Powell, T., Rogers, A., Serbin, S. P., Shuman, J. K., Swann, A. L. S., Varadharajan, C., Walker, A. P., Wright, S. J., and Xu, C.: Benchmarking and parameter sensitivity of physiological and vegetation dynamics using the Functionally Assembled Terrestrial Ecosystem Simulator (FATES) at Barro Colorado Island, Panama, Biogeosciences, 17, 3017–3044, https://doi.org/10.5194/bg-17-3017-2020, 2020.
Lasslop, G., Thonicke, K., and Kloster, S.: SPITFIRE within the MPI Earth system model: Model development and evaluation, J. Adv. Model. Earth Sy., 6, 740–755, https://doi.org/10.1002/2013MS000284, 2014.
Lasslop, G., Hantson, S., Harrison, S. P., Bachelet, D., Burton, C., Forkel, M., Forrest, M., Li, F., Melton, J. R., Yue, C., Archibald, S., Scheiter, S., Arneth, A., Hickler, T., and Sitch, S.: Global ecosystems and fire: Multi-model assessment of fire-induced tree-cover and carbon storage reduction, Glob. Change Biol., 26, 5027–5041, https://doi.org/10.1111/gcb.15160, 2020.
Latham, D. and Williams, E.: Lightning and Forest Fires, in: Forest Fires. Behavior and Ecological Effects, Academic Press, San Diego, 376–418, https://doi.org/10.1016/B978-012386660-8/50013-1, 2001.
Lawrence, D., Coe, M., Walker, W., Verchot, L., and Vandecar, K.: The Unseen Effects of Deforestation: Biophysical Effects on Climate, Front. For. Glob. Change, 5, 756115, https://doi.org/10.3389/ffgc.2022.756115, 2022.
Lawrence, D. M., Fisher, R. A., Koven, C. D., Oleson, K. W., Swenson, S. C., Bonan, G., Collier, N., Ghimire, B., van Kampenhout, L., Kennedy, D., Kluzek, E., Lawrence, P. J., Li, F., Li, H., Lombardozzi, D., Riley, W. J., Sacks, W. J., Shi, M., Vertenstein, M., Wieder, W. R., Xu, C., Ali, A. A., Badger, A. M., Bisht, G., van den Broeke, M., Brunke, M. A., Burns, S. P., Buzan, J., Clark, M., Craig, A., Dahlin, K., Drewniak, B., Fisher, J. B., Flanner, M., Fox, A. M., Gentine, P., Hoffman, F., Keppel-Aleks, G., Knox, R., Kumar, S., Lenaerts, J., Leung, L. R., Lipscomb, W. H., Lu, Y., Pandey, A., Pelletier, J. D., Perket, J., Randerson, J. T., Ricciuto, D. M., Sanderson, B. M., Slater, A., Subin, Z. M., Tang, J., Thomas, R. Q., Val Martin, M., and Zeng, X.: The Community Land Model Version 5: Description of New Features, Benchmarking, and Impact of Forcing Uncertainty, J. Adv. Model. Earth Sy., 11, 4245–4287, https://doi.org/10.1029/2018MS001583, 2019.
Lehmann, C. E. R., Archibald, S. A., Hoffmann, W. A., and Bond, W. J.: Deciphering the distribution of the savanna biome, New Phytol., 191, 197–209, https://doi.org/10.1111/j.1469-8137.2011.03689.x, 2011.
Li, F., Zeng, X. D., and Levis, S.: A process-based fire parameterization of intermediate complexity in a Dynamic Global Vegetation Model, Biogeosciences, 9, 2761–2780, https://doi.org/10.5194/bg-9-2761-2012, 2012.
Li, F., Levis, S., and Ward, D. S.: Quantifying the role of fire in the Earth system – Part 1: Improved global fire modeling in the Community Earth System Model (CESM1), Biogeosciences, 10, 2293–2314, https://doi.org/10.5194/bg-10-2293-2013, 2013.
Maréchaux, I. and Chave, J.: An individual-based forest model to jointly simulate carbon and tree diversity in Amazonia: description and applications, Ecol. Monogr., 87, 632–664, https://doi.org/10.1002/ecm.1271, 2017.
McLauchlan, K. K., Higuera, P. E., Miesel, J., Rogers, B. M., Schweitzer, J., Shuman, J. K., Tepley, A. J., Varner, J. M., Veblen, T. T., Adalsteinsson, S. A., Balch, J. K., Baker, P., Batllori, E., Bigio, E., Brando, P., Cattau, M., Chipman, M. L., Coen, J., Crandall, R., Daniels, L., Enright, N., Gross, W. S., Harvey, B. J., Hatten, J. A., Hermann, S., Hewitt, R. E., Kobziar, L. N., Landesmann, J. B., Loranty, M. M., Maezumi, S. Y., Mearns, L., Moritz, M., Myers, J. A., Pausas, J. G., Pellegrini, A. F. A., Platt, W. J., Roozeboom, J., Safford, H., Santos, F., Scheller, R. M., Sherriff, R. L., Smith, K. G., Smith, M. D., and Watts, A. C.: Fire as a fundamental ecological process: Research advances and frontiers, J. Ecol., 108, 2047–2069, https://doi.org/10.1111/1365-2745.13403, 2020.
Morton, D. C., Le Page, Y., DeFries, R., Collatz, G. J., and Hurtt, G. C.: Understorey fire frequency and the fate of burned forests in southern Amazonia, Philos. T. Roy. Soc. B, 368, 20120163, https://doi.org/10.1098/rstb.2012.0163, 2013.
Naudts, K., Ryder, J., McGrath, M. J., Otto, J., Chen, Y., Valade, A., Bellasen, V., Berhongaray, G., Bönisch, G., Campioli, M., Ghattas, J., De Groote, T., Haverd, V., Kattge, J., MacBean, N., Maignan, F., Merilä, P., Penuelas, J., Peylin, P., Pinty, B., Pretzsch, H., Schulze, E. D., Solyga, D., Vuichard, N., Yan, Y., and Luyssaert, S.: A vertically discretised canopy description for ORCHIDEE (SVN r2290) and the modifications to the energy, water and carbon fluxes, Geosci. Model Dev., 8, 2035–2065, https://doi.org/10.5194/gmd-8-2035-2015, 2015.
Needham, J. F., Arellano, G., Davies, S. J., Fisher, R. A., Hammer, V., Knox, R. G., Mitre, D., Muller-Landau, H. C., Zuleta, D., and Koven, C. D.: Tree crown damage and its effects on forest carbon cycling in a tropical forest, Glob. Change Biol., 28, 5560–5574, https://doi.org/10.1111/gcb.16318, 2022.
Nepstad, D. C., Stickler, C. M., Filho, B. S., and Merry, F.: Interactions among Amazon land use, forests and climate: prospects for a near-term forest tipping point, Philos. T. Roy. Soc. B, 363, 1737–1746, https://doi.org/10.1098/rstb.2007.0036, 2008.
Nobre, C. A., Sampaio, G., Borma, L. S., Castilla-Rubio, J. C., Silva, J. S., and Cardoso, M.: Land-use and climate change risks in the Amazon and the need of a novel sustainable development paradigm, P. Natl. Acad. Sci. USA, 113, 10759–10768, https://doi.org/10.1073/pnas.1605516113, 2016.
Pausas, J. G. and Keeley, J. E.: Abrupt Climate-Independent Fire Regime Changes, Ecosystems, 17, 1109–1120, https://doi.org/10.1007/s10021-014-9773-5, 2014.
Pellegrini, A. F. A., Anderegg, W. R. L., Paine, C. E. T., Hoffmann, W. A., Kartzinel, T., Rabin, S. S., Sheil, D., Franco, A. C., and Pacala, S. W.: Convergence of bark investment according to fire and climate structures ecosystem vulnerability to future change, Ecol. Lett., 20, 307–316, https://doi.org/10.1111/ele.12725, 2017.
Peterson, D. L. and Ryan, K.: Modeling postfire conifer mortality for long-range planning, Environ. Manage., 10, 797–808, 1986.
Pueyo, S., De Alencastro Graça, P. M. L., Barbosa, R. I., Cots, R., Cardona, E., and Fearnside, P. M.: Testing for criticality in ecosystem dynamics: the case of Amazonian rainforest and savanna fire: Criticality in Amazonia, Ecol. Lett., 13, 793–802, https://doi.org/10.1111/j.1461-0248.2010.01497.x, 2010.
Rabin, S. S., Melton, J. R., Lasslop, G., Bachelet, D., Forrest, M., Hantson, S., Kaplan, J. O., Li, F., Mangeon, S., Ward, D. S., Yue, C., Arora, V. K., Hickler, T., Kloster, S., Knorr, W., Nieradzik, L., Spessa, A., Folberth, G. A., Sheehan, T., Voulgarakis, A., Kelley, D. I., Prentice, I. C., Sitch, S., Harrison, S., and Arneth, A.: The Fire Modeling Intercomparison Project (FireMIP), phase 1: experimental and analytical protocols with detailed model descriptions, Geosci. Model Dev., 10, 1175–1197, https://doi.org/10.5194/gmd-10-1175-2017, 2017.
Radabaugh, K. R., Powell C. E., Bociu I., Clark B. C., and Moyer R. P.: Plant size metrics and organic carbon content of Florida salt marsh vegetation, Wetl. Ecol. Manag., 25, 443–455, 2017.
Ratnam, J., Bond, W. J., Fensham, R. J., Hoffmann, W. A., Archibald, S., Lehmann, C. E. R., Anderson, M. T., Higgins, S. I., and Sankaran, M.: When is a “forest” a savanna, and why does it matter?: When is a “forest” a savanna, Global Ecol. Biogeogr., 20, 653–660, https://doi.org/10.1111/j.1466-8238.2010.00634.x, 2011.
Reis, C. R., Jackson, T. D., Gorgens, E. B., Dalagnol, R., Jucker, T., Nunes, M. H., Ometto, J. P., Aragão, L. E. O. C., Rodriguez, L. C. E., and Coomes, D. A.: Forest disturbance and growth processes are reflected in the geographical distribution of large canopy gaps across the Brazilian Amazon, J. Ecol., 110, 2971–2983, https://doi.org/10.1111/1365-2745.14003, 2022.
Rothermel, R. C.: A mathematical model for predicting fire spread in wildland fuels, Res. Pap. INT-115, USDA Forest Service Intermountain Forest and Range Experiment Station, Ogden, UT, 40 pp., 1972.
Rothermel, R. C.: How to predict the spread and intensity of forest and range fires, U. S. Department of Agriculture, Forest Service, Intermountain Forest and Range Experiment Station, Ogden, UT, https://doi.org/10.2737/INT-GTR-143, 1983.
Ryan, C. M. and Williams, M.: How does fire intensity and frequency affect miombo woodland tree populations and biomass?, Ecol. Appl., 21, 48–60, https://doi.org/10.1890/09-1489.1, 2011.
Saatchi, S. S., Harris, N. L., Brown, S., Lefsky, M., Mitchard, E. T. A., Salas, W., Zutta, B. R., Buermann, W., Lewis, S. L., Hagen, S., Petrova, S., White, L., Silman, M., and Morel, A.: Benchmark map of forest carbon stocks in tropical regions across three continents, P. Natl. Acad. Sci. USA, 108, 9899–9904, https://doi.org/10.1073/pnas.1019576108, 2011.
Sankaran, M., Hanan, N. P., Scholes, R. J., Ratnam, J., Augustine, D. J., Cade, B. S., Gignoux, J., Higgins, S. I., Le Roux, X., Ludwig, F., Ardo, J., Banyikwa, F., Bronn, A., Bucini, G., Caylor, K. K., Coughenour, M. B., Diouf, A., Ekaya, W., Feral, C. J., February, E. C., Frost, P. G. H., Hiernaux, P., Hrabar, H., Metzger, K. L., Prins, H. H. T., Ringrose, S., Sea, W., Tews, J., Worden, J., and Zambatis, N.: Determinants of woody cover in African savannas, Nature, 438, 846–849, https://doi.org/10.1038/nature04070, 2005.
Sankaran, M., Ratnam, J., and Hanan, N.: Woody cover in African savannas: the role of resources, fire and herbivory, Global Ecol. Biogeogr., 17, 236–245, https://doi.org/10.1111/j.1466-8238.2007.00360.x, 2008.
Scheiter, S. and Higgins, S. I.: Impacts of climate change on the vegetation of Africa: an adaptive dynamic vegetation modelling approach, Glob. Change Biol., 15, 2224–2246, https://doi.org/10.1111/j.1365-2486.2008.01838.x, 2009.
Scheiter, S., Higgins, S. I., Osborne, C. P., Bradshaw, C., Lunt, D., Ripley, B. S., Taylor, L. L., and Beerling, D. J.: Fire and fire-adapted vegetation promoted C4 expansion in the late Miocene, New Phytol., 195, 653–666, https://doi.org/10.1111/j.1469-8137.2012.04202.x, 2012.
Scheiter, S., Langan, L., and Higgins, S. I.: Next-generation dynamic global vegetation models: learning from community ecology, New Phytol., 198, 957–969, https://doi.org/10.1111/nph.12210, 2013.
Schubauer, J. P. and Hopkinson, C. S.: Above- and belowground emergent macrophyte production and turnover in a coastal marsh ecosystem, Georgia1: Macrophyte production, Limnol. Oceanogr., 29, 1052–1065, https://doi.org/10.4319/lo.1984.29.5.1052, 1984.
Shuman, J.: Files and scripts to support manuscript Shuman et al 2023 FATES-SPITFIRE ecosystem assembly across tropics, 1.0, NGEE Tropics Data Collection [data set], https://doi.org/10.15486/ngt/1992487, 2023.
Shuman, J. K., Balch, J. K., Barnes, R. T., Higuera, P. E., Roos, C. I., Schwilk, D. W., Stavros, E. N., Banerjee, T., Bela, M. M., Bendix, J., Bertolino, S., Bililign, S., Bladon, K. D., Brando, P., Breidenthal, R. E., Buma, B., Calhoun, D., Carvalho, L. M. V., Cattau, M. E., Cawley, K. M., Chandra, S., Chipman, M. L., Cobian-Iñiguez, J., Conlisk, E., Coop, J. D., Cullen, A., Davis, K. T., Dayalu, A., De Sales, F., Dolman, M., Ellsworth, L. M., Franklin, S., Guiterman, C. H., Hamilton, M., Hanan, E. J., Hansen, W. D., Hantson, S., Harvey, B. J., Holz, A., Huang, T., Hurteau, M. D., Ilangakoon, N. T., Jennings, M., Jones, C., Klimaszewski-Patterson, A., Kobziar, L. N., Kominoski, J., Kosovic, B., Krawchuk, M. A., Laris, P., Leonard, J., Loria-Salazar, S. M., Lucash, M., Mahmoud, H., Margolis, E., Maxwell, T., McCarty, J. L., McWethy, D. B., Meyer, R. S., Miesel, J. R., Moser, W. K., Nagy, R. C., Niyogi, D., Palmer, H. M., Pellegrini, A., Poulter, B., Robertson, K., Rocha, A. V., Sadegh, M., Santos, F., Scordo, F., Sexton, J. O., Sharma, A. S., Smith, A. M. S., Soja, A. J., Still, C., Swetnam, T., Syphard, A. D., Tingley, M. W., Tohidi, A., Trugman, A. T., Turetsky, M., Varner, J. M., Wang, Y., Whitman, T., Yelenik, S., and Zhang, X.: Reimagine fire science for the anthropocene, PNAS Nexus, 1, pgac115, https://doi.org/10.1093/pnasnexus/pgac115, 2022.
Silva Junior, C. H. L., Aragão, L. E. O. C., Anderson, L. O., Fonseca, M. G., Shimabukuro, Y. E., Vancutsem, C., Achard, F., Beuchle, R., Numata, I., Silva, C. A., Maeda, E. E., Longo, M., and Saatchi, S. S.: Persistent collapse of biomass in Amazonian forest edges following deforestation leads to unaccounted carbon losses, Sci. Adv., 6, eaaz8360, https://doi.org/10.1126/sciadv.aaz8360, 2020.
Silvério, D. V., Brando, P. M., Balch, J. K., Putz, F. E., Nepstad, D. C., Oliveira-Santos, C., and Bustamante, M. M. C.: Testing the Amazon savannization hypothesis: fire effects on invasion of a neotropical forest by native cerrado and exotic pasture grasses, Philos. T. Roy. Soc. B, 368, 20120427, https://doi.org/10.1098/rstb.2012.0427, 2013.
Snell, J. A.: Direct estimation of surface fuel bulk density and loading in western Montana and northern Idaho, University of Montana, Missoula, MT, 1979.
Staver, A. C., Archibald, S., and Levin, S. A.: The Global Extent and Determinants of Savanna and Forest as Alternative Biome States, Science, 334, 230–232, https://doi.org/10.1126/science.1210465, 2011.
Staver, A. C., Brando, P. M., Barlow, J., Morton, D. C., Paine, C. E. T., Malhi, Y., Araujo Murakami, A., and Pasquel, J.: Thinner bark increases sensitivity of wetter Amazonian tropical forests to fire, Ecol. Lett., 23, 99–106, https://doi.org/10.1111/ele.13409, 2020.
Swann, A. L. S., Hoffman, F. M., Koven, C. D., and Randerson, J. T.: Plant responses to increasing CO2 reduce estimates of climate impacts on drought severity, P. Natl. Acad. Sci. USA, 113, 10019–10024, https://doi.org/10.1073/pnas.1604581113, 2016.
Teckentrup, L., Harrison, S. P., Hantson, S., Heil, A., Melton, J. R., Forrest, M., Li, F., Yue, C., Arneth, A., Hickler, T., Sitch, S., and Lasslop, G.: Response of simulated burned area to historical changes in environmental and anthropogenic factors: a comparison of seven fire models, Biogeosciences, 16, 3883–3910, https://doi.org/10.5194/bg-16-3883-2019, 2019.
Thonicke, K., Spessa, A., Harrison, S. P., Dong, L., and Carmona-Moreno, C.: The influence of vegetation, fire spread and fire behaviour on biomass burning and trace gas emissions: results from a process-based model, Biogeosciences, 7, 1991–2011, 2010.
Touma, D., Stevenson, S., Lehner, F., and Coats, S.: Human-driven greenhouse gas and aerosol emissions cause distinct regional impacts on extreme fire weather, Nat. Commun., 12, 212, https://doi.org/10.1038/s41467-020-20570-w, 2021.
Travis, S. E. and Grace, J. B.: Predicting performance for ecological restoration: a case study using Spartina alterniflora, Ecol. Appl., 20, 192–204, https://doi.org/10.1890/08-1443.1, 2010.
Uhl, C. and Kauffman, J. B.: Deforestation, Fire Susceptibility, and Potential Tree Responses to Fire in the Eastern Amazon, Ecology, 71, 437–449, https://doi.org/10.2307/1940299, 1990.
van der Werf, G. R., Randerson, J. T., Giglio, L., van Leeuwen, T. T., Chen, Y., Rogers, B. M., Mu, M., van Marle, M. J. E., Morton, D. C., Collatz, G. J., Yokelson, R. J., and Kasibhatla, P. S.: Global fire emissions estimates during 1997–2016, Earth Syst. Sci. Data, 9, 697–720, https://doi.org/10.5194/essd-9-697-2017, 2017.
Van Wilgen, B. W., Govender, N., Biggs, H. C., Ntsala, D., and Funda, X. N.: Response of Savanna Fire Regimes to Changing Fire-Management Policies in a Large African National Park: Fire Regimes in an African Park, Conserv. Biol., 18, 1533–1540, https://doi.org/10.1111/j.1523-1739.2004.00362.x, 2004.
Veldman, J. W. and Putz, F. E.: Grass-dominated vegetation, not species-diverse natural savanna, replaces degraded tropical forests on the southern edge of the Amazon Basin, Biol. Conserv., 144, 1419–1429, https://doi.org/10.1016/j.biocon.2011.01.011, 2011.
Veldman, J. W., Mostacedo, B., Peña-Claros, M., and Putz, F. E.: Selective logging and fire as drivers of alien grass invasion in a Bolivian tropical dry forest, Forest Ecol. Manag., 258, 1643–1649, https://doi.org/10.1016/j.foreco.2009.07.024, 2009.
Venevsky, S., Thonicke, K., Sitch, S., and Cramer, W.: Simulating fire regimes in human-dominated ecosystems: Iberian Peninsula case study, Glob. Change Biol., 8, 984–998, 2002.
Venevsky, S., Le Page, Y., Pereira, J. M. C., and Wu, C.: Analysis fire patterns and drivers with a global SEVER-FIRE v1.0 model incorporated into dynamic global vegetation model and satellite and on-ground observations, Geosci. Model Dev., 12, 89–110, https://doi.org/10.5194/gmd-12-89-2019, 2019.
Walker, A. P., Hanson, P. J., De Kawe, M. G., Medlyn, B. E., Zaehle, S., Asao, S., Dietze, M., Hickler, T., Huntingford, C., Iversen, C. M., Jain, A., Lomas, M., Luo, Y., McCarthy, H., Parton, W. J., Prentice, I. C., Thornton, P. E., Wang, S., Wang, Y. P., Warlind, D., Weng, E., Warren, J. M., Woodward, F. I., Oren, R., and Norby, R. J.: Comprehensive ecosystem model-data synthesis using multiple data sets at two temperate forest free-air CO2 enrichment experiments: Model performance at ambient CO2 concentration, J. Geophys. Res.-Biogeo., 119, 937–964, 2014.
Williams, R. J., Gill, A. M., and Moore, P. H. R.: Fire behaviour, in: Fire in Tropical Savannas: The Kapalga Experiment, edited by: Anderson, A. N., Cook, G. D., and Williams, R. J., Springer, New York, N.Y., 33–46, https://doi.org/10.1007/b97225, 2003.
Wotton, B. M., Alexander, M. E., and Taylor, S. W.: Updates and revisions to the 1992 Canadian Forest Fire Behavior Prediction System, Information Report GLC-X-10, Canadian Forest Service, Great Lakes Forestry Centre, Sault Ste. Marie, Ontario, Canada, Natural Resources Canada, 45 pp., 2009.
Yue, C., Ciais, P., Cadule, P., Thonicke, K., Archibald, S., Poulter, B., Hao, W. M., Hantson, S., Mouillot, F., Friedlingstein, P., Maignan, F., and Viovy, N.: Modelling the role of fires in the terrestrial carbon balance by incorporating SPITFIRE into the global vegetation model ORCHIDEE – Part 1: simulating historical global burned area and fire regimes, Geosci. Model Dev., 7, 2747–2767, https://doi.org/10.5194/gmd-7-2747-2014, 2014.
Zuleta, D., Arellano, G., Muller-Landau, H. C., McMahon, S. M., Aguilar, S., Bunyavejchewin, S., Cárdenas, D., Chang-Yang, C., Duque, A., Mitre, D., Nasardin, M., Pérez, R., Sun, I., Yao, T. L., and Davies, S. J.: Individual tree damage dominates mortality risk factors across six tropical forests, New Phytol., 233, 705–721, https://doi.org/10.1111/nph.17832, 2022.