the Creative Commons Attribution 4.0 License.
the Creative Commons Attribution 4.0 License.
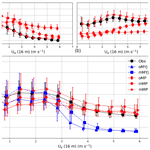
Evaluation and development of surface layer scheme representation of temperature inversions over boreal forests in Arctic wintertime conditions
Julia Maillard
Jean-Christophe Raut
François Ravetta
In this study, the Noah land surface model used in conjunction with the Mellor–Yamada–Janjić surface layer scheme (hereafter, Noah-MYJ) and the Noah multiphysics scheme (Noah-MP) from the Weather Research and Forecasting (WRF) 4.5.1 mesoscale model are evaluated with regard to their performance in reproducing positive temperature gradients over forested areas in the Arctic winter. First, simplified versions of the WRF schemes, recoded in Python, are compared with conceptual models of the surface layer in order to gain insight into the dependence of the temperature gradient on the wind speed at the top of the surface layer. It is shown that the WRF schemes place strong limits on the turbulent collapse, leading to lower surface temperature gradient at low wind speeds than in the conceptual models. We implemented modifications to the WRF schemes to correct this effect. The original and modified versions of Noah-MYJ and Noah-MP are then evaluated compared to long-term measurements at the Ameriflux Poker Flat Research Range, a forest site in interior Alaska. Noah-MP is found to perform better than Noah-MYJ because the former is a two-layer model which explicitly takes into account the effect of the forest canopy. Indeed, a non-negligible temperature gradient is maintained below the canopy at high wind speeds, leading to overall larger gradients than in the absence of vegetation. Furthermore, the modified versions are found to perform better than the original versions of each scheme because they better reproduce strong temperature gradients at low wind speeds.
- Article
(1562 KB) - Full-text XML
- BibTeX
- EndNote
Surface-based temperature inversions (SBIs) are extremely frequent in the cold, dark conditions of the Arctic winter (Serreze et al., 1992; Bradley et al., 1992). The usual pattern is that cloudy conditions are associated with a near-neutral surface layer, while clear skies are associated with strong SBIs (Malingowski et al., 2014). However, modelling temperature inversions remains a challenge and an area of ongoing study (Steeneveld et al., 2006; Sterk et al., 2013; Holtslag et al., 2013; Baas et al., 2017).
One of the main difficulties is with modelling the turbulent heat fluxes. Typical Monin–Obukhov stability theory (MOST) assumes constant fluxes in the surface layer and so-called z-less scaling (Monin and Obukhov, 1954; Wyngaard and Coté, 1972), and its limits of applicability have been discussed (Grachev et al., 2008). This has led to the recognition of different turbulent regimes. The first, called the weakly stable regime, is fully consistent with MOST. In this regime, the turbulent heat fluxes increase with increasing temperature gradient because more heat is available to be transported. The inertial range in the turbulence spectra is well defined and exhibits a Kolmogorov slope of (Kaimal and Finnigan, 1994). The other is the strongly stable regime, where turbulent sensible heat fluxes instead decrease with increasing temperature gradient because the effect of strong stability leads to a turbulence decay. In this regime, Kolmogorov turbulence becomes intermittent and driven by processes at larger timescales, such as the Coriolis force (Grachev et al., 2008) or gravity waves (Sorbjan and Czerwinska, 2013). However, it does not disappear entirely so that the flow never becomes laminar (Grachev et al., 2013).
There is general agreement on the nature of these two turbulence regimes (although sometimes a third “transitional” regime is considered). However, the separation between the two is debated: traditionally, the Richardson number (Ri) or Monin–Obukhov parameter (ζ) is used. Grachev et al. (2013), for example, suggested that a gradient or flux Richardson number of Ri=0.2 was a lower threshold for the strongly stable state, while Mahrt et al. (2014) found that ζ=0.06 separated the two states. More recent works have focused on the impact of wind speeds, or wind shear, on determining the regime (Sun et al., 2012; van de Wiel et al., 2007; van de Wiel et al., 2012) in a framework called minimum wind speed for sustainable turbulence (MWST). For example, van Hooijdonk et al. (2015), building on the work of van de Wiel et al. (2012), used external forcings to the surface layer (such as a constant wind speed, replacing the synoptic pressure gradient and downwards radiative fluxes) to determine a new parameter called the shear capacity. This parameter has been found to better predict the stability regime than the traditional local parameters such as ζ or Ri. In this new framework, the stability regime is not solely a feature of the turbulence but of the surface layer as a whole.
Determining the stability regime and the turbulent heat fluxes is, however, only one part of determining the SBI strength. This depends on the surface temperature, which is in turn determined by the surface energy budget (SEB). Analysis of measurements in the Antarctic has shown that plotting ΔT (the temperature difference between the surface and 10 m) versus the wind speed at 10 m under clear-sky winter conditions reveals two distinct regimes, separated by a transition: one at low wind speeds and high ΔT and the other at high wind speeds and low ΔT (Vignon et al., 2017). This characteristic shape was termed the “S” shape (although the S is technically backwards) because the transition exhibited some non-monotonous behaviour. The transition between the two regimes was found to agree well with predictions from MWST. Drawing on these studies, a small analytical model was developed by van de Wiel et al. (2017) and was shown to reproduce the “S” shape.
MWST therefore offers a promising framework for the analysis and modelling of SBIs. For the moment, however, these analyses have been restricted to the extreme conditions of Antarctica, where the surface is vegetation-free snow and ice. The Arctic and sub-Arctic also experience regular inversions with strong implications for pollution dispersion. However, a large part of this region is covered by forest, which is known to impact the turbulent heat fluxes (Batchvarova et al., 2001). For example, unstable stratification may remain within the canopy layer even when the overlying air layer is very stable (Jacobs et al., 1992), and gradients directly above the canopy may be modified by the roughness sublayer (Mölder et al., 1999; Babić et al., 2016). Forest canopies also act as grey bodies, both emitting and absorbing long-wave fluxes. In seeking to extend the use of MWST, it is therefore important to consider the impact of trees. Another important question concerns the coherence of mesoscale models with MWST. Vignon et al. (2018), for example, showed that the Laboratoire de Météorologie Dynamique – Zoom (LMDZ) model reproduced an S-shaped transition of surface temperature gradient with wind speed, with the shape of the transition depending on the stability function used. This represents a promising new framework for improving the representation of surface layer temperature inversions.
This paper therefore has two coupled aims. The first is to investigate the impact of wind speed on the temperature gradient in clear-sky, winter conditions over a forest surface using a multiyear observational dataset from a forest of interior Alaska. The second is to evaluate and improve the performance of Weather Research and Forecasting (WRF) model schemes in representing the temperature gradient at this forest site. Here, the parts of the WRF code responsible for calculating the surface temperature gradient are referred to as the Surface Energy Budget and Surface Layer (SEB-SL) model because they calculate the turbulent energy exchanges in the surface layer and solve the surface energy budget. In WRF, the SEB-SL model is often split into two parts: the surface layer scheme and the land surface model. In Sect. 2, conceptual SEB-SL models are introduced and used to gain insight into the development of temperature inversions and shed light on two different WRF SEB-SL schemes. In Sect. 3, the measurements from the Ameriflux Poker Flat Research Range near Fairbanks, Alaska, are presented. In Sect. 4, two WRF SEB-SL schemes are presented, and modifications are proposed. Lastly, the behaviour of the two original WRF schemes and their modified versions is compared based on the Ameriflux measurements (Sect. 5).
In this section, conceptual models of the surface layer are presented (Sect. 2.1). These models are used to gain insight into the impact of different variables (and, especially, of the wind speed) on the resulting surface–air temperature gradient in order to help with the analysis of the WRF surface layer schemes.
2.1 Model presentation
A single-layer conceptual SEB-SL model was developed by van de Wiel et al. (2017) to study the impact of the wind speed on the near-surface temperature gradient. In the presence of trees or other tall vegetation, however, the introduction of a second layer becomes necessary. Here, such a model is developed. It is composed of the surface, a “canopy” layer where the air is in thermodynamic equilibrium with the vegetation, and an overlying air layer (Fig. 1). The effects of the canopy on the long-wave radiative and turbulent fluxes are then taken into account. In the following, the equations and notations draw on the one-layer model of van de Wiel et al. (2017). The surface emissivity is assumed to be equal to 1, which is a good approximation for snow-covered surfaces. The short-wave radiation and the latent heat fluxes are neglected in Arctic wintertime conditions.
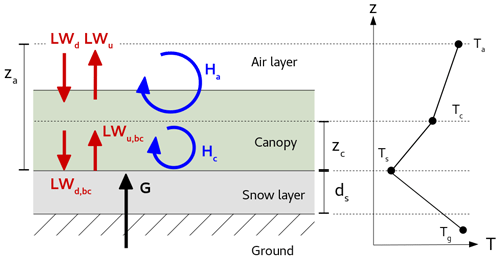
Figure 1Schematic of the two-layer model described in this section. LWd and LWu are the downwards and upwards long-wave fluxes above the canopy, and LWd,bc and LWu,bc are the downwards and upwards long-wave fluxes below the canopy. Ha is the turbulent sensible heat flux between the canopy and overlying air. Hc is the turbulent sensible heat flux between the canopy and surface. G is the conduction flux through the snow.
The surface energy balance equation in this system can be written as (Appendix A)
where is the difference between the surface temperature (Ts) and the air temperature at canopy height (Tc). is the difference between the canopy temperature and the air temperature at height za, corresponding to the top of the surface layer (Ta). Tg is the ground temperature (Fig. 1). (where LWd is the downwards long-wave flux) is termed the isothermal net radiation: indeed, it is equal to the net long-wave flux if Ts=Ta (Holtslag and Bruin, 1988). , where λs is the snow conductivity and ds the snow depth. ρ is the air density, Cp the heat capacity of the air and Uc the wind speed at height zc. For the time being, Uc is very roughly estimated to be proportional to Ua: for example, . ϵc is the canopy emissivity, and CD,c is the turbulent diffusion coefficient for the canopy-to-surface heat exchange.
A second equation can be obtained by considering the canopy energy balance (Appendix A):
where Ua is the wind speed at height za, and CD,a is the turbulent diffusion coefficient for the air–canopy heat exchange. This is very similar to the one-layer model of van de Wiel et al. (2012), except that the energy source term (here, the first line of Eq. 2) has an additional term, which is proportional to ΔTcs. The difficulty in solving Eqs. (1) and (2) to obtain ΔTcs and ΔTac is that the turbulent diffusion coefficients depend on the stability:
where κ=0.4 is the van Kármán constant, and z0s and z0c are the roughness lengths of snow or of the canopy, respectively. Here, d is the displacement height due to the presence of the canopy. The snow and canopy momentum roughness length is assumed to be equal to the heat roughness length , both referred to as . is the Obukhov parameter, with L being the Monin–Obukhov length. ψ is the integral stability function, which tends to 0 when ζ≈0 and tends to infinity with increasing ζ. The turbulent diffusion coefficients therefore tend to at weak stability and 0 at strong stability. Many different expressions of ψ are found in the literature (Businger et al., 1971; Holtslag and Bruin, 1988). Usually, these are classified as “short tail” (i.e. with a very sharp decrease so that CD quickly drops to 0 at increasing stability) or “long tail” (i.e. the transition is smoother so that some turbulent sensible heat flux is maintained for longer). There are also other ways to estimate the below-canopy turbulent diffusion coefficient CD,c, for example by assuming an exponential wind profile in the canopy as in Mahat et al. (2013). However, Eq. (3) is the simplest expression and is utilised for illustrative purposes.
2.2 Weakly and strongly stable limits
The first insights into the behaviour of ΔTcs and ΔTac can be gained by studying the asymptotic cases: the weakly and strongly stable limits, defined by Ua→∞ and Ua→0, respectively, while keeping ΔTas>0. Here only the case where ϵc=1 (corresponding to an opaque canopy) is considered. In this situation, and if turbulence is completely collapsed (i.e. ), Eqs. (1) and (2) lead to the following values for the temperature gradients:
and therefore,
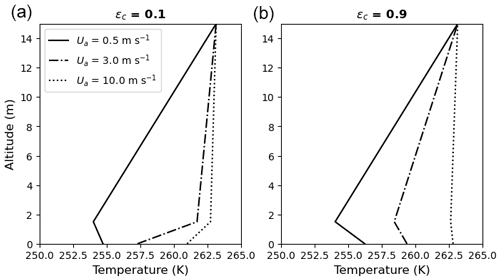
Figure 2Profile calculated by the theoretical model for Qi=50 W m−2, °C, °C and Λs=1 W m−2 K−1, for three values of the wind speed Ua (0.5, 3 and 10 m s−1). The turbulence was solved iteratively using the Ameriflux stability function. The only difference between the two graphs is the canopy emissivity: (a) ϵc=0.1 and (b) ϵc=0.9.
The total temperature gradient ΔTas (between the air and the surface) in Eq. (5) is very similar to the one-layer case but with an “equivalent snow conductivity” that is twice the real value.
It can also be noted that ΔTac will usually be positive for typical Arctic winter values of the different parameters (Table 1) because radiation is the dominant process, unless the snow cover is very thin. For example, in a very cold, high-synoptic-pressure situation with Qi=70 W m−2, °C and Tg=0 °C, ΔTac will be positive unless the snow depth is less than 7 cm. Similarly, in a warmer, cloudier situation with Qi=30 W m−2, °C and Tg=0 °C, ΔTac will be positive unless the snow depth is less than 8 cm. For the same reason, ΔTcs will usually be negative. In short, the very stable case is characterised by a temperature decrease from the surface to the canopy and an increase from the canopy to the overlying air. This is illustrated in Fig. 2 (continuous line, corresponding to Ua=0.5 m s−1).
This is contradictory to the idea that CD,c collapses to 0 because in the presence of a negative temperature gradient, buoyancy effects may generate turbulence without significant mechanical shear. Indeed, solving Eqs. (1) and (2) numerically for m s−1 (using appropriate schemes for calculating the turbulent diffusion coefficients such as those described in Sect. 4.1.1) shows that CD,cUc maintains a value of around 0.0017 m s−1. Therefore, while the surface layer as a whole may be considered strongly stable (because Ta−Ts is very large), this may not be the case for the canopy layer. This is in agreement with Batchvarova et al. (2001), who found that the canopy layer may remain unstable even when the air aloft is very stably stratified.
2.3 Transition between the weakly and strongly stable limits
Next, the turbulence is solved iteratively using the Ameriflux stability function (Sect. 3.2) over a complete range of Ua values. This makes it possible to study the behaviour of the inversion outside of the weakly and strongly stable regimes and for different values of the canopy emissivity. The result of this estimation is shown in Fig. 3a, b for three values of ϵc. ΔTac exhibits the same S shape as in the one-layer conceptual model of van de Wiel et al. (2017). On the other hand, ΔTcs is larger in the weakly stable regime than in the strongly stable regime (where it is negative, in coherence with the above discussion), and its shape is more dependent on values of the canopy emissivity. For ϵc=1, ΔTcs appears to tend to 0 at large values of Ua while keeping negative values. On the other hand, for ϵc=0.5 and 0.1, it turns positive before decreasing with increasing wind speeds, therefore reaching a maximum somewhere between 2 and 6 m s−1. In sum, the total temperature difference (ΔTas) decreases more slowly than in the one-layer model, even when accounting for the long-tailed function chosen. The transition between the two modes (weak winds associated with strong inversions and high winds associated with weak inversions) is much more gradual in this two-layer conceptual model than the characteristic marked inverted S shape reported in the one-layer conceptual model of van de Wiel et al. (2017). This phenomenon corresponds to observations over a surface covered by trees that attenuate the transition.
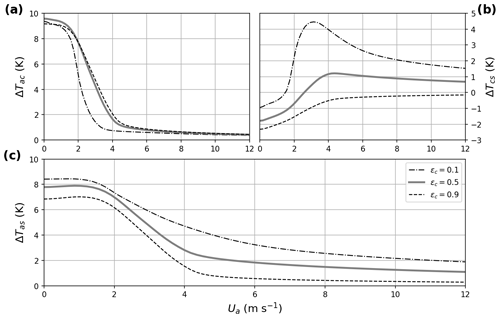
Figure 3(a) ΔTac as a function Ua, as calculated by the conceptual model. The different line styles correspond to different values of the canopy emissivity. The transition between the two regimes has been calculated using the MYJ algorithm (Sect. 4.1.1) with the Ameriflux stability function (Sect. 3.2). All curves have been calculated using Qi=50 W m−2, °C, °C and Λs=1 W m−2 K−1. (b), (c) The same as (a) but for ΔTcs and ΔTas, respectively.
This behaviour can be understood in the following way. At low wind speeds, the dominant process influencing the canopy layer is radiation: it emits more than it receives and therefore loses its heat to both the surface and the air above. As a result, it is colder than both. Although some turbulence remains due to buoyancy, this is not enough to compensate for the radiative heat loss. At high wind speeds, on the other hand, the whole SL is well mixed. Turbulence is the dominant process, linking the canopy layer to both the surface and the air above and maintaining their temperatures closely.
Starting from the strongly stable state, a small increase in wind speed will lead to increased turbulence mixing and a positive heat flow from the air above to the canopy. The canopy temperature will therefore increase. If the canopy has a strong emissivity, this increase in temperature will lead to increased radiation downwards to the surface and a corresponding increase in surface temperature. The canopy and surface will therefore warm at relatively the same pace (Fig. 2, right). On the other hand, if the emissivity of the canopy is low, it will not as easily convert its increased temperature into radiation. Its temperature will therefore increase rapidly without contributing to warming the surface, leading to a high ΔTcs (Fig. 2, left). As the wind speed continues to increase, the canopy temperature will eventually be more or less equal to the temperature of the air above, and the surface continues to warm, thus leading to a decrease in ΔTcs.
The measurements at the Ameriflux Poker Flat Research Range were used to evaluate the behaviour of the WRF surface layer schemes and the suggested modifications. In this section, they are presented, and a first analysis of the link between wind speed and temperature gradient is put forward.
3.1 Site description
The Ameriflux PRR site is located in the Poker Flat Research Range (65°07′24.4′′ N, 147°29′15.2′′ W), around 30 km away from Fairbanks (interior Alaska). It has been operating since 2010, when it was established as part of the JAMSTEC-IARC Collaboration Study (JICS) (Sugiura et al., 2011), and its data are made available online on the Ameriflux website (Kobayashi et al., 2019) (https://ameriflux.lbl.gov/sites/siteinfo/US-Prr, last access: 22 April 2024). Its 17 m measurement tower is implanted in a black spruce forest with sparsely distributed and short trees (Fig. 4). The tree density, as measured in 2010, was 3967 trees ha−1, and the average tree height was 2.44 m: the tallest tree was 6.4 m, but 75 % of trees were shorter than 3 m (Nakai et al., 2013). The leaf area index (LAI) was 0.73 (Nakai et al., 2013). Both of these values are much smaller than those found in Noah-MP for evergreen forests (Table 3).
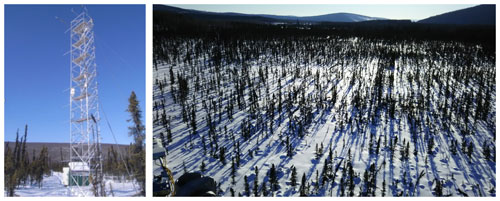
Figure 4Left: photo of the 17 m high measurement tower at the Poker Flat Research Range at the Ameriflux site. Right: photo of the PRR site as seen from the measurement tower. Credit: Lisa Johnson.
Table 2Meteorological variables measured at the Ameriflux PRR site, including instruments and measurement heights (Nakai et al., 2013). Note that wind speed is also measured at 11 m, with the sonic anemometer. Nakai et al. (2013) indicate that temperature was also measured at 7.5 m, but these measurements do not appear to be available on the Ameriflux website.
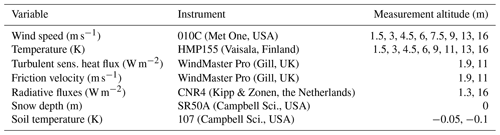
The Ameriflux PRR measurements that are used here are summarised in Table 2. These include wind speeds and temperatures at eight different heights (from 1.5 to 16 m), as well as turbulent and radiative fluxes. The surface temperature was calculated from the radiative flux measurements at 1.3 m, assuming a snow surface emissivity of 0.99. As this study focuses on the clear-sky surface layer in wintertime conditions, the data were curated accordingly. Only time points in the months of November–March with snow depth greater than 10 cm were kept. As no measurements of the cloud cover are available at the PRR site, clear-sky instants were defined as those with net long-wave radiation less than −30 W m−2. Indeed, as is typical in high-latitude sites, the net long-wave flux (Rn) distribution at the PRR site was bimodal; the low-Rn mode was considered to correspond to the absence of clouds and the high-Rn mode to their presence. As the PRR site is located slightly below the Arctic circle, there is still some solar radiation at the surface in the wintertime. In order to simplify the analysis, only time points with downwelling short-wave radiation of less than 30 W m−2 were kept; as the snow albedo is very high, this corresponds to a net short-wave flux of less than 5 W m−2 and therefore to negligible short-wave impact. Lastly, measurements with a latent heat flux greater than 5 W m−2 in absolute value were discarded.
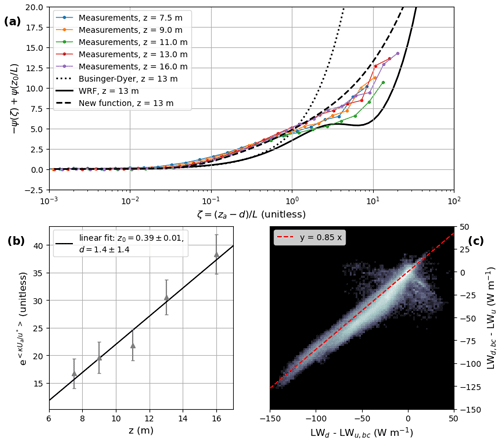
Figure 5(a) Momentum integral stability function as a function of ζ determined from the PRR site measurement (coloured lines) and calculated using the Businger–Dyer and WRF formulations (black lines). The dashed line corresponds to the newly determined function for ψ (Eq. 7). (b) Determination of the PRR site above-canopy momentum roughness length and displacement height. (c) 2D histogram of vs. . The red line corresponds to y=0.85x, yielding a canopy emissivity of 0.15.
3.2 PRR stability function
The average emissivity of the canopy layer ϵc=fvegϵv can be calculated from above- and below-canopy radiation measurements:
As shown in Fig. 5c, this gives a best estimation of ϵc≈0.15. This is in accordance with the Noah-MP calculation of fveg and ϵv as a function of LAI. Indeed, measured LAI at the PRR site is 0.73, yielding fveg≈0.3 (Eq. 12) and ϵv≈0.5.
The value of z0m,c can also be calculated from the sonic anemometer data. Indeed, at weak stability (ζ≪1), the wind profile is approximately logarithmic:
Therefore, d and z0m,c can be determined through a linear regression of against z when the data are restricted to values of . Here, z0m,c was found to be 0.39 m with m (Fig. 5b), which makes sense for a forest environment with short trees. The integral stability function ψ can also be determined from the data (assuming, as is often done, that it is the same for momentum and heat). The aim of this determination is to reproduce the measurements over the zone of transition, which is approximately between ζ=0.1 and ζ=1. For higher values (i.e. ), the specific values of ψ are less problematic because in this range the turbulent heat flux will tend to collapse anyway. In order to determine ψ,
is plotted as a function of ζ (Fig. 5a). Because is negligible compared to ζ, Ψ is approximately equal to −ψ(ζ) at the first order. Here, we found that measured ψ is more long tailed than the Businger–Dyer (Businger et al., 1971) or WRF (Eq. 9) functions. The intermediate zone (ζ between 0.1 and 1) exhibits a marked difference between the Businger-Dyer and WRF functions and the measurements. In fact, when plotted on a log–log scale, it became apparent that Ψ was proportional to at low values of ζ and proportional to ζ2 at high values of ζ. This differs from the often-used z-less scaling, which implies that ψ must be proportional to ζ at least up to ζ≈0.1 (Monin and Obukhov, 1954; Grachev et al., 2013) as is the case for the WRF and Businger–Dyer functions. Measurement error may explain some of the difference at ζ<0.1 as Ψ is very small in these conditions.
For our purposes, we determined an analytical expression of ψ to best fit to the Ψ measurements and left aside the question of the z-less scaling. The following expression for ψ was therefore considered:
This was chosen because arctan (x) tends to when x tends to ±∞, with a smooth transition around x=0. r(ζ), which therefore tends to 0.5 at low values of ζ and 2 at high values of ζ, similar to observations. The b and c coefficients must be chosen so that the timing and speed of the transition between the and ζ2 asymptotes match the observations. The function Ψ was therefore fitted for the different heights (z=7.5, 9, 11, 13 and 16 m), yielding coefficients which varied between the ranges [4.5,5.5], and [10,40] for a, b and c, respectively. Plotting the function with these different parameters revealed little difference in behaviour over this range. Values of a=5, b=20 and c=0.1 were found to give a good fit to the observations (Fig. 5a). This expression of ψ was termed the PRR stability function.
The Ameriflux PRR site characteristics are summarised in Table 3. Although the location of the PRR site is given as evergreen needleleaf forest by the MODerate resolution Imaging Spectroradiometer (MODIS) land use categories as well as by the Ameriflux website, its canopy height and turbulent and radiative characteristics are actually the most similar to a wooded or mixed tundra.
3.3 Link between temperature gradients and wind speed at the Ameriflux PRR site
The average temperature profile (and its difference from the temperature at 16 m) is shown in Fig. 6a. The impact of wind speed on the surface layer temperature profile is clear. To highlight the importance of the wind speed on the regime stability, data are gathered in two groups according to their wind speed values: Ua< 2 m s−1 or Ua> 4 m s−1. This distinction is only used in Fig. 6 for illustrative purpose. This separation is based on the distribution of the bulk Richardson number Rb at 16 m (Fig. 6b): 65 % of the data with Ua>4 m s−1 indeed have an Rb≤0.25, and 99.2 % of the data with Ua<2 m s−1 have an Rb>0.25. The two modes are therefore clearly separated with a negligible overlap.
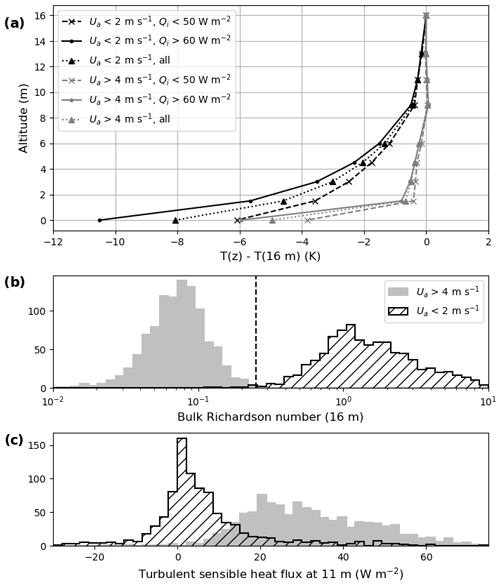
Figure 6(a) Average temperature difference from 16 m for wind speeds at 16 m smaller than 2 m s−1 (black lines) and higher than 4 m s−1 (grey lines). The continuous lines correspond to Qi>60 W m−2 and dashed lines to Qi<50 W m−2. (b) Histogram of Rb values calculated at 16 m, for wind speeds greater than 4 m s−1 (filled grey) or smaller than 2 m s−1 (hashed black). (c) Histogram of turbulent sensible heat flux measured at 11 m (identical colours).
For Ua<2 m s−1, the temperature decreases rapidly all the way down to the surface, and the Richardson number is overwhelmingly greater than 0.25 (Fig. 6b), which is the traditionally cited limit value beyond which turbulence collapses. However, while the turbulent sensible heat flux has a low mean value of 4 W m−2, its distribution remains quite spread out, with 5th and 95th percentiles of −12 and 30 W m−2, respectively (Fig. 6c). This indicates that there is some remaining turbulence.
For Ua>4 m s−1, the temperature gradient is very weak (approximately 0.5 °C) down to 1.5 m, with a strong temperature gradient remaining in the last metres. The top to bottom temperature difference is nevertheless smaller than for Ua<2 m s−1, leading to Rb values that are smaller than 0.25. Accordingly, the turbulent sensible heat flux is much larger than for the lower wind speeds: its mean is 32 W m−2, with 90 % of values between 11 and 60 W m−2. The fact that both Rb and the turbulent sensible heat flux have clearly distinct distributions for wind speeds greater than 4 and lower than 2 m s−1 suggests that a threshold wind speed for sustainable turbulence probably occurs in this range. It should further be noted that while only the bulk Richardson number at 16 m is calculated here, the distributions are similar at other altitudes higher than 6 m. The impact of the radiative input (Qi) is also clear in Fig. 6a. All the observed values have been gathered in two groups that do not overlap and are delimited by their values of the isothermal net radiation Qi. Thresholds of 50 and 60 W m−2 hence provide a clear view of the impact of Qi on the temperature profiles as a function of the wind speed. The average profiles corresponding to values of Qi>60 W m−2 exhibit a larger temperature gradient than those corresponding to values lower than 50 W m−2, especially at low wind speeds. This is in accordance with Sect. 2: greater radiative cooling leads to a larger SBI.
The relationship between the average air-to-surface temperature difference and wind speed is shown in Fig. 7c. decreases with Ua, reaching a minimum for Ua>5 m s−1, and there is a clear distinction between the averages corresponding to Qi lower than 50 and greater than 60 W m−2, respectively. ΔTas can further be broken down into (Fig. 7b) and (Fig. 7a). ΔTac exhibits a very clear S shape, collapsing to less than 1 K at wind speeds higher than 4 m s−1. ΔTcs, on the other hand, is maximum around 3 m s−1 for both ranges of Qi. These behaviours are reminiscent of the two-layer model (Sect. 2.1): the main difference here is that ΔTcs remains positive instead of decreasing to negative values at low wind speeds.
Examination of the temperature profiles and gradients in relation to wind speed at the PRR site therefore suggests that a two-layer model may be able to reproduce the temperature gradients, with the temperature at 1.5 m being a proxy for the canopy temperature. The observations are compared to the models in more detail in Sect. 5.
In this section, two WRF SEB-SL models are presented (Sect. 4.1). Then modifications to these schemes are suggested, and their evaluation method compared to the Ameriflux measurements is explained (Sect. 4.2).
4.1 WRF SEB-SL modules
Within the WRF code, the SEB-SL model is split into two parts. First, the surface layer module calculates the turbulent diffusion coefficient. Then, the land surface model uses the turbulent diffusion coefficient to solve the SEB and determine the surface temperature. Many different schemes are available for each module. In this work, we have chosen to focus on the Noah land surface model (Noah-LSM) (Chen and Dudhia, 2001; Ek et al., 2003), used in conjunction with the Mellor–Yamada–Janjić (MYJ) surface layer scheme (Janjić, 1994), and the Noah multiphysics scheme (Niu et al., 2011; He et al., 2023), which is nominally a land surface model but actually functions as an entire SEB-SL model, as it calculates its own turbulent diffusion coefficients. In the rest of this article, the Noah-LSM and MYJ combination is termed Noah-MYJ.
4.1.1 Noah-MYJ
When there is snow cover, Noah-LSM functions very similarly to the one-layer model of van de Wiel et al. (2017). The snowpack is considered a single layer, while the soil is subdivided into four layers for which the heat diffusion equation is solved, yielding the topmost soil layer temperature Tg. In the MYJ scheme, CD is calculated as
where m2 s−1 is the air kinematic viscosity; Rb the bulk Richardson number; and Rc a critical Richardson number, here equal to 0.505. Here, u* is the friction velocity. The momentum roughness length z0m is fixed according to the land use type and vegetation, while heat roughness length z0h depends on the stability (through the Richardson number). Note that because this is a one-layer model, there are no separate snow and canopy roughness lengths.
ψ has the following expression in stable conditions (i.e. ζ ≥ 0):
This is similar but not equal to the Holtslag integral stability function (Holtslag and Bruin, 1988) up to values of ζ≈ 1. Indeed, ζ has a set maximum value of 1. This means that CD never goes to 0, some turbulent sensible heat flux is always maintained and the very stable regime is not independent of Ua. For low values of the momentum roughness length, the distinction between very and weakly stable regimes even completely disappears, with ΔTas instead decreasing almost linearly as a function of Ua. Solving this form of the equation for CD requires L to be known, which in turn requires knowledge of both u* and the turbulent sensible heat flux and thus CD. The solving procedure is therefore iterative.
4.1.2 Noah-MP
Recently, Noah-MP has been introduced as an updated version of Noah-LSM, introducing, among others, a vegetation energy balance, a layered snowpack and soil moisture–groundwater interaction (Niu et al., 2011; He et al., 2023). Each grid node is divided into a vegetated and a non-vegetated fraction. The non-vegetated fraction surface temperature is calculated similarly to Noah-LSM, except that the snowpack is divided into up to three different layers, and the ground heat flux is calculated through the topmost snow layer only. The vegetated fraction calculation is a more complex version of a two-layer model, where the vegetation temperature is considered to be different from the air temperature in the canopy. The vegetation acts as a grey body with emissivity ϵv and exchanges sensible heat with the canopy air. The canopy air is transparent to long-wave radiation, simply exchanging sensible heat with the surface, the overlying air and the vegetation. In short, the radiative and sensible heat budgets of the canopy layer in Sect. 2.1 are separated. In practice, however, the temperature difference between the vegetation and the canopy air did not exceed 0.5 K during our runs so that a simple two-layer model provides a good approximation for the behaviour of Noah-MP. Therefore, we do not detail the calculation of the tree–canopy–air sensible heat exchange.
Table 3Noah-MP surface characteristics for four different land use types: evergreen needleleaf forest, mixed forest, wooded tundra and mixed tundra. The characteristics include the leaf area index (LAI), canopy height (hcan), canopy momentum roughness length (z0m,c), displacement height d, vegetation fraction (fveg) and vegetation emissivity (ϵv). The last column shows the surface characteristics at the Ameriflux Poker Flat Research Range in interior Alaska, which are presented in Sect. 3.1.
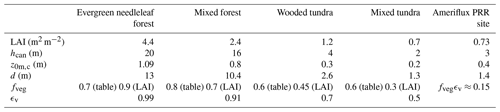
The turbulent diffusion coefficient for the canopy-to-overlying-air sensible heat exchange, CD,a, is calculated using the “original Noah” scheme with a roughness length and displacement height which depend on the land use category; the displacement height is calculated as , where hcan is the canopy top height. This original Noah scheme is identical to the MYJ scheme described above, except that it uses the Businger–Dyer stability function (Businger et al., 1971). CD,c is calculated by assuming an exponential wind profile, similar to what is described in Mahat et al. (2013):
where n is the exponential decay coefficient, which depends on the leaf area index (LAI), canopy top height and stability, and z0m,s is the below-canopy ground roughness length. In this article, the below-canopy ground cover is always assumed to be snow.
The total grid box surface temperature is then calculated from the two values obtained for the vegetated (Ts,v) and non-vegetated parts (Ts,nv):
where fveg is the vegetation fraction in each model grid box. There are multiple calculation options for this parameter in Noah-MP. It can either be taken from the vegetation parameter table, which is also used by Noah-LSM, or be determined from the LAI using the following formula:
LAI itself can either be taken from the Noah-MP parameter table or determined “dynamically” by a carbon budget subroutine. Typical values of LAI and fveg for different land use categories are shown in Table 3.
It should be noted that the vegetation emissivity used in Noah-MP is not equivalent to the canopy emissivity in the simple two-layer model described in Sect. 2.1. In effect, Noah-MP supposes that the vegetated fraction has an emissivity of ϵv, and the non-vegetated fraction has an emissivity of 0: the average canopy emissivity (such as that used by the model in Sect. 2.1) is therefore ϵc=fvegϵv.
4.2 Modifications to the WRF SEB-SL schemes
In this section, two one-layer and two two-layer models are compared (Table 4). The one-layer models include the original Noah-MYJ (oMYJ), presented in Sect. 4.1, and a modified version of this scheme (mMYJ). The two-layer models include the original Noah-MP (oMP) and a modified version of Noah-MP (mMP).
Table 4Summary of the four surface models evaluated in this study. For all four models, z0m,c was set to 0.4 m and λs to 0.3 W m−1 K−1.
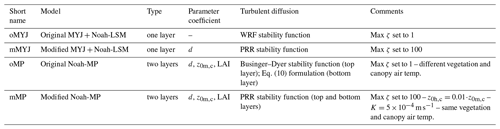
The guiding principle for the modifications to both original models was to improve the modelled dependency of the temperature inversion on the wind speed, in particular the transition between the two regimes. This included removing the imposed maximum on ζ so that a truly stable regime is allowed to develop. The stability function was also modified to a more long-tail formulation (Sect. 3.2): this makes the transition more gradual and avoids the non-monotonicity associated with Eq. (9) at ζ>1. Furthermore, a displacement height is added in the mMYJ model.
Modifications implemented in mMP included forcing the vegetation and canopy air temperature to be equal so that the energy balance for the vegetated part is as described in Sect. 2.1. The canopy-to-ground turbulent diffusion coefficient was also calculated as in the MYJ surface layer instead of using Eq. (10). Lastly, a constant coefficient m s−1 was added to CD,aUa. This effectively imposes a lower limit on the turbulent diffusion coefficient in a gradual way, without having to force a minimum which would create a discontinuity. At wind speeds greater than 3 m s−1, this constant coefficient is negligible compared to the calculated value of CD,aUa. It should be noted that in effect, the original Noah-MP also imposes such a limit through indirect methods (for example, by imposing a minimum value of 1 m s−1 for Ua or a maximum value of 1 for ζ). The reasons for imposing a lower limit on the turbulence are explored further in Sect. 5.1.
4.3 Model evaluation
In order to evaluate the models “offline”, the oMYJ and oMP models were extracted from the WRF framework and recoded in Python in a minimal form; i.e. only the parts relating to the surface temperature calculation were kept. In particular, all latent heat flux calculations were ignored; the snow conductivity was assumed to be constant, equal to 0.3 W m−1 K−1, and snow depth and ground temperature were used as input variables rather than being calculated. mMYJ and mMP were similarly coded in Python.
Input parameters to all four models are set to correspond to the characteristics of the PRR site as determined in Sect. 3.2: i.e. d=1.4 m, m and m. For oMP and mMP, fveg was determined from the LAI using Eq. (12). For the two modified versions, the stability function used is the one determined from the Poker Flat Research Range measurements (Sect. 3.2).
Here, the top of the surface layer was considered to be the top of the measurement tower; i.e. za=16 m. The five input variables to the Python models are the measured air temperature at 16 m, wind speed at 16 m, downwards long-wave flux above the canopy, snow depth and ground temperature. The output is the surface temperature (and canopy temperature for the two-layer models). Running the models over the entirety of the curated PRR dataset yielded 5412 modelled values of surface temperature, which were then compared to the corresponding 5412 measured values of Ts. The results are analysed in Sect. 5.2.
5.1 Modelled impact of the wind speed on the development of temperature inversions
The outputs of the one-layer models (oMYJ and mMYJ) are shown in blue in Fig. 7c. Both tend to have similar values to the observations for low wind speeds, although oMYJ does not reach a constant regime because ζ is limited to values of 1. Because this limit is removed in mMYJ, it better reproduces two regimes separated by a transition; this transition is however more gradual because the PRR stability function was used. Both models, however, predict too small values of ΔTas at high wind speeds compared to the observations.
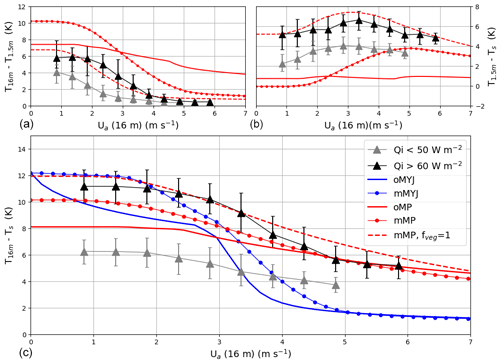
Figure 7(a) Average temperature difference between za=16 and 1.5 m (ΔTac) as a function of wind speed at 16 m. The black and grey lines indicate averaged measurements for Qi>60 W m−2 and Qi<50 W m−2, respectively. (b), (c) The same as (a) but for ΔTcs and ΔTas, respectively. The continuous and dotted blue lines correspond to the output of the oMYJ and mMYJ models, respectively, for input values Qi=65 W m−2, Ta=263.15 K, Tg=271.15 K and Λs=1 W m−2 K−1 (as already used in the asymptotic model in Fig. 2). The continuous and dotted red lines correspond to the output of the oMP and mMP models, respectively, with the same input values of Qi, Ta, Tg and Λs. The dashed red line corresponds to the same simulation as the dotted red line, except that fveg=1.
The two-layer models, on the other hand, both show a much more gradual decrease in ΔTas with Ua. Indeed, the decrease is so gradual in the output of oMP that it is not possible to discern two distinct regimes – even though the stability function used is Businger–Dyer, which is very short tailed (see Fig. 3a, black lines compared to the grey line which corresponds to the PRR stability function). One reason for this is that many limits are placed to maintain turbulence: u* cannot become larger than 0.07 m s−1; ζ must remain smaller than 1; and when the wind speed is used for calculating the turbulent diffusion coefficient, it takes a minimum value of 1 m s−1 (this is only the case within the surface layer modules so that WRF still outputs wind speed values less than 1 m s−1). Although it is true that some turbulence is always maintained, as shown by the measurements at the PRR site (Fig. 6), the result is that the Noah-MP model outputs too low ΔTas values at very low wind speeds. oMP also does not reproduce the individual behaviour of ΔTcs and ΔTac: its calculated ΔTac does not have an S shape as a function of wind speed, and ΔTcs exhibits no maximum.
The behaviour of mMP is more satisfactory. ΔTac shows a clear transition between a low-wind-speed, high-gradient state and high-wind-speed state where the gradient is close to 0. ΔTcs has a maximum between 3 and 5 m s−1 (depending on the value of fveg). ΔTas, finally, is close to the observed value in both the high- and the low-wind-speed limits. Two things must be noted here: first, that values of ΔTcs remain positive at low wind speeds because, as noted in Sect. 4.2, a constant K equal to m s−1 has been added to CD,aUa. Similar to the limits imposed in oMP, this serves to maintain a certain level of turbulence and avoid the collapse of the turbulent sensible heat flux. Without this, ΔTcs would decrease much more strongly, as described in Sect. 2.1. Adding a constant, as opposed to imposing a maximum value, is a more gradual method which does not distort the shape of the transition. The constant value is chosen to best represent the observations and should be discussed in regard to other datasets.
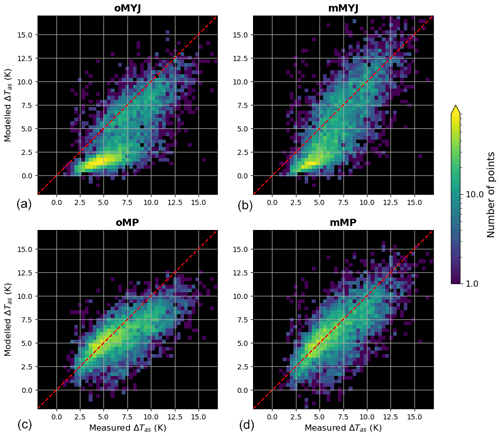
Figure 82D histograms of the modelled vs. measured for the four models. (a, b) One-layer models (a – oMYJ; b – mMYJ). (c, d) Two-layer models (c – oMP; d – mMP). The colour represents the number of points, in a lognormal scale, and the dashed red line corresponds to the 1:1 line.
Secondly, two versions of mMP are shown in Fig. 7. The first corresponds to fveg=0.3 and ϵv=0.5, which are the values which would be calculated by WRF from a LAI of 0.73 according to Eq. (12). The second corresponds to fveg=1 and ϵv=0.15. The results are substantially different, especially concerning the canopy temperature (and therefore ΔTcs and ΔTac). Indeed, as outlined in Sect. 2.1, the canopy tends to become colder than the surface for higher values of ϵv, and this is the case for the simulation with fveg=0.3. Furthermore, the transition wind speed (for ΔTac) and wind speed at maximum ΔTac are shifted to lower values for the simulation with fveg=1. These two sets of values both correspond to ϵc=0.15 and therefore to the same radiative flux balance. However, the difference in outcome suggests that due to the turbulent fluxes, separating a bare fraction from a vegetated fraction is not equivalent to considering only one layer but with lower emissivity. The simulation with fveg=1 seems to perform better. One possible explanation is linked to the size of the eddies transporting heat. If they are of similar size to the typical distance between the trees, the turbulent transport of heat would not necessarily behave differently over a bare fraction than over a vegetated fraction. Instead, all turbulent transport would occur in an averaged manner. Indeed, the turbulent characteristics calculated in Sect. 3.2 are likely representative of this average, depending on the instrument footprint. At the PRR site, average tree distance is approximately 2.2 m, assuming that the trees are homogeneously distributed (which seems reasonable from site photos, Fig. 4). It is, however, not clear whether this is a robust feature of the two-layer models. Indeed, oMP does not appear to perform better when fveg is set to 1 and ϵc to 0.15: its calculated values of ΔTas decrease more rapidly with wind speed and therefore remain smaller than the measured temperature difference over the whole wind speed range (not shown). In the following, the values of fveg=0.3 and ϵv=0.5 are used for both two-layer models.
5.2 Evaluation of WRF SEB-SL model compared to the PRR site measurements
The models are then run over all the PRR measurement points. Compared to the above analysis, this makes it possible to evaluate their behaviour for a wide variety of input values. Overall, all models capture some of the variability in ΔTas, probably due to the influence of the downwards radiative fluxes. It is clear however that the one-layer models always underestimate ΔTas when the measured ΔTas is the lowest, which corresponds to conditions of high wind speeds (Fig. 8). oMYJ also underestimates ΔTas when the measured ΔTas is very high, but this effect has been corrected in mMYJ by allowing for a stronger decrease in turbulence. The root mean square error (RMSE) of mMYJ is therefore approximately 2.8 K as opposed to 3.4 K for the original MYJ scheme.
The two-layer models both perform better than the one-layer models, supporting the idea that they are more adapted for use in a forest environment. The original Noah-MP model cannot reproduce strong values of ΔTas because of excessive forced turbulence; mMP fares better in that regard. Its RMSE is slightly better (2.2 instead of 2.3 K). Note that running mMP with fveg=1 and ϵv=0.15 leads to an RMSE of 2.1 K. To gain insight into the reliability of the model, all available observations are selected and binned according to their wind speed Ua values in intervals of width of 0.5 m s−1. This eliminates assumptions regarding input parameters such as the net radiation at the surface. Results are shown in Fig. 9.
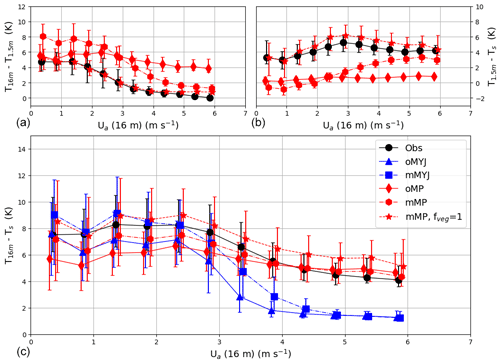
Figure 9(a) Median temperature difference between za=16 m and 1.5 m (ΔTac) as a function of wind speed at 16 m. The black line indicates measurements binned according to their wind speed Ua values in intervals of width of 0.5 m s−1. (b), (c) The same as (a) but for ΔTcs and ΔTas, respectively. The continuous and dotted blue lines correspond to the output of the oMYJ and mMYJ models, respectively. The continuous and dotted red lines correspond to the output of the oMP and mMP models, respectively. The dashed red line corresponds to the same simulation as the dotted red line, except that fveg=1. The error bars on the measured and modelled values represent the interquartile range (25th and 75th percentiles).
It clearly shows that MYJ, whether in its original (oMYJ) or its modified (mMYJ) versions, reproduces a too sharp transition due to the fact that it only considers a single layer and strongly differs from the observations when Ua values become larger than 2.5 m s−1. mMYJ is in better agreement with the observations when the wind speed is weaker because the modelled ΔTas values are obtained in a constant regime and enhanced by 2 K. This is the consequence of removing the limitation of ζ values to 1.
Regarding the two-layer models, oMP slightly underestimates the strength of the inversion for small values of the wind speed Ua, even though the results are not too far from the error bars: the interquartile intervals barely overlap with those of the observed values. On the other hand, it appears obvious that it is actually due to compensation errors in the two layers taken individually: ΔTac is overestimated, while ΔTcs is underpredicted.
The two versions of mMP by far provide the best results compared to the observations, especially when fveg=1 (Fig. 9). It captures the dependency of the two individual layers (atmosphere–canopy and canopy–surface) on the wind speed well. A key result is that the transition between the two modes is much more gradual in two-layer models. Over a forest area or in multi-layer models, the S shape, reported in one-layer models, becomes blurred: this smoother transition between the two modes can be ascribed to the presence of trees, which attenuates the S shape. This is the reason why two-layer models perform better.
A simple two-layer analytical model of the stable surface layer was developed and contrasted with the existing one-layer models of van de Wiel et al. (2017). The two-layer model predicted a more gradual dependency of ΔTas on the wind speed than the one-layer models with equivalent roughness lengths and stability function. The top layer exhibited the S-shape dependence of the temperature gradient on the wind speed, which is typical of one-layer models. The bottom layer, on the other hand, had a maximum temperature gradient at the transition wind speed. However, results depended strongly on the value of the first layer emissivity. Insights gained from the theoretical models were applied to study two surface layer/land surface model modules in WRF: Noah-MP and the Noah-MYJ combination. It was found that these models tend to set very restrictive boundaries on the turbulent diffusion coefficients and stability parameters so that strong temperature gradients cannot be reached.
A combined approach was then used to study the performance of different surface layer models in more detail. First, an extensive set of measurements from the Poker Flat Research Range was analysed. It was found that under clear-sky, snow-covered nighttime conditions, the temperature gradient depended strongly on both the downwards long-wave flux and the wind speed. When the wind speed at 16 m was smaller than 2 m s−1, the temperature profile showed a very strong inversion down to the surface, and the Richardson number was larger than 0.25, the traditional “cutoff” value for turbulence. Nevertheless, some turbulent sensible heat flux remained. On the other hand, when the wind speed was larger than 4 m s−1, the temperature profile was roughly constant down to 1.5 m, below which a strong temperature gradient remained. The Richardson number was then below 0.25, corresponding to the traditional weakly stable regime. Furthermore, the dependence of the individual-layer temperature inversion on wind speed was qualitatively similar to the theoretical two-layer model.
Four different SEB-SL models were then coded into Python: the Noah-MYJ combination, Noah-MP and modified versions of the two. These were first compared to the observations qualitatively and then by inputting measured values of temperature and wind speed at different altitudes into the surface layer and comparing the outputted value of ΔTas to the measurements. It was found that the two-layer models both gave better results than the one-layer models, which tended to predict too low temperature gradients at high wind speeds. Over a forest area, the presence of trees indeed tends to attenuate the transition between the two modes. On the other hand, the original Noah-MP predicted too low temperature gradients at low wind speeds. All in all, the modified Noah-MP gave the best results, especially for the individual-layer temperature gradients.
Open questions remain concerning the impact of local parameters on the simulations. Although the PRR site is classified as evergreen needleleaf forest by the MODIS land use categories, its characteristics are actually rather similar to a wooded or mixed tundra: its trees are indeed very short and spaced out, and its emissivity and roughness length are quite low for a forest site. These parameters were shown to impact the behaviour of the lowest-layer temperature gradient. Indeed, at high emissivities, the canopy layer is theoretically predicted to become colder than the surface. Furthermore, the value of the turbulent diffusion parameter for the surface-to-canopy air heat exchanges is taken from Monin–Obukhov similarity theory, which assumes a log-wind profile. Other parametrisations, such as the logarithmic and exponential profiles of Mahat et al. (2013), which are implemented in Noah-MP, could conceivably yield better results in a denser forest. It would therefore be necessary to test the behaviour of the model compared to a denser forest site with higher trees.
This study focuses on the clear-sky surface layer in an Arctic winter context. Clear-sky periods have been identified as those when the net long-wave radiation was less than −30 W m−2 (Graham et al., 2017; Maillard et al., 2021). Wintertime conditions have been selected in periods between November and March when the downwelling short-wave radiation was lower than 30 W m−2, the latent heat flux less than 5 W m−2 and the snow depth greater than 10 cm. Outside these conditions, the original conceptual models have not been modified. The implementation of conceptual model improvements in WRF should be followed by a testing phase to find out how the mesoscale model performs outside these restrictive conditions.
The major perspective arising from the present paper is the implementation of the modified SEB-SL models into the main WRF framework. Once this is done, the impact of the modifications on model output can be tested over real cases. Because wind speed can vary locally due to topography in the continental Arctic, the modifications suggested here are therefore expected to impact the spatial distribution of near-surface SBIs during winter anticyclonic episodes, with resulting consequences for the modelling of pollutant dispersion and pollution episodes. Another major question concerns the impact of clouds on the surface layer stability and SBI and how transitions between cloudy- and clear-surface layers are represented by WRF.
The surface energy balance corresponding to the system in Fig. 1 is
where LWd,bc and LWu,bc are the downwards and upwards fluxes below the canopy level, G is the ground heat flux, and Hc is the turbulent sensible heat exchange between the canopy and the surface. Each flux can then be parametrised as follows:
where ϵc is the canopy emissivity, Tc the canopy temperature, Ts the surface temperature and LWd the downwards long-wave flux above the canopy level. If Ta is the air temperature above the canopy, Tc can be written as , where . Hence , assuming that . A first-order Taylor expansion leads to .
Similarly, .
where ρ is the air density, Cp the heat capacity of air, Uc the canopy wind speed and CD,c the turbulent diffusion coefficient for the surface-to-canopy sensible heat exchange.
where λs is the snow conductivity, ds the snow depth and Tg the ground temperature. The surface energy balance equation can then be reorganised to Eq. (1):
Similarly, the energy balance applied to the canopy layer yields
where LWu is the upwards long-wave flux measured above the canopy level.
where Ua is the air wind speed and CD,a the turbulent diffusion coefficient for the canopy-to-air sensible heat exchange. The downwards fluxes can be written as
Similarly, the algebraic sum of the upwards fluxes is
This transforms to
Summing Eqs. (1) and (A10) then yields Eq. (2):
The modified simplified versions, recoded in Python, of the Noah-MYJ and Noah-MP schemes from the WRF 4.5.1 mesoscale model and data from the Ameriflux Poker Flat Research Range (PRR) site used in this paper are permanently archived at https://doi.org/10.5281/zenodo.8347090 (Maillard et al., 2023).
JM developed and coded the models, performed the data treatment and analysis, and prepared the paper. FR and JCR provided supervision and guidance and edited the paper.
The contact author has declared that none of the authors has any competing interests.
Publisher’s note: Copernicus Publications remains neutral with regard to jurisdictional claims made in the text, published maps, institutional affiliations, or any other geographical representation in this paper. While Copernicus Publications makes every effort to include appropriate place names, the final responsibility lies with the authors.
Funding for the Ameriflux data portal was provided by the US Department of Energy's Office of Science. The US Poker Flat Research Range (PRR) site is supported by the JAMSTEC and IARC/UAF collaboration study (JICS), the Arctic Challenge for Sustainability project (ArCS; September 2015–March 2020), and ArCSII (since July 2020). Computer modelling benefited from access to IDRIS HPC resources (GENCI allocations A011017141 and A013017141) and the IPSL mesoscale computing centre.
This project has received funding from the European Union's Horizon 2020 research and innovation programme under grant agreement no. 101003826 via project CRiceS (Climate Relevant interactions and feedbacks: the key role of sea ice and Snow in the polar and global climate system).
This paper was edited by Yongze Song and reviewed by two anonymous referees.
Baas, P., van de Wiel, B. J. H., van der Linden, S. J. A., and Bosveld, F. C.: From Near-Neutral to Strongly Stratified: Adequately Modelling the Clear-Sky Nocturnal Boundary Layer at Cabauw, Bound.-Lay. Meteorol., 166, 217–238, https://doi.org/10.1007/s10546-017-0304-8, 2017. a
Babić, K., Rotach, M. W., and Klaić, Z. B.: Evaluation of local similarity theory in the wintertime nocturnal boundary layer over heterogeneous surface, Agr. Forest Meteorol., 228-229, 164–179, https://doi.org/10.1016/j.agrformet.2016.07.002, 2016. a
Batchvarova, E., Gryning, S.-E., and Hasager, C. B.: Regional Fluxes Of Momentum And Sensible Heat Over A Sub-Arctic Landscape During Late Winter, Bound.-Lay. Meteorol., 99, 489–507, https://doi.org/10.1023/A:1018982711470, 2001. a, b
Bradley, R. S., Keimig, F. T., and Diaz, H. F.: Climatology of surface based inversions in the North American Arctic, J. Geophys. Res., 97, 15699–15712, https://doi.org/10.1029/92jd01451, 1992. a
Businger, J. A., Wyngaard, J. C., Izumi, Y., and Bradley, E. F.: Flux-Profile Relationships in the Atmospheric Surface Layer, Journal of Atmospheric Science, 28, 181–189, https://doi.org/10.1175/1520-0469(1971)028<0181:fprita>2.0.co;2, 1971. a, b, c
Chen, F. and Dudhia, J.: Coupling an Advanced Land Surface–Hydrology Model with the Penn State–NCAR MM5 Modeling System. Part I: Model Implementation and Sensitivity, Mon. Weather Rev., 129, 569–585, https://doi.org/10.1175/1520-0493(2001)129<0569:CAALSH>2.0.CO;2, 2001. a
Ek, M. B., Mitchell, K. E., Lin, Y., Rogers, E., Grunmann, P., Koren, V., Gayno, G., and Tarpley, J. D.: Implementation of Noah land surface model advances in the National Centers for Environmental Prediction operational mesoscale Eta model, J. Geophys. Res.-Atmos., 108, 8851, https://doi.org/10.1029/2002jd003296, 2003. a
Grachev, A. A., Andreas, E. L., Fairall, C. W., Guest, P. S., and Persson, P. O. G.: Turbulent measurements in the stable atmospheric boundary layer during SHEBA: ten years after, Acta Geophys., 56, 142–166, https://doi.org/10.2478/s11600-007-0048-9, 2008. a, b
Grachev, A. A., Andreas, E. L., Fairall, C. W., Guest, P. S., and Persson, P. O. G.: The Critical Richardson Number and Limits of Applicability of Local Similarity Theory in the Stable Boundary Layer, Bound.-Lay. Meteorol., 147, 51–82, https://doi.org/10.1007/s10546-012-9771-0, 2013. a, b, c
Graham, R. M., Rinke, A., Cohen, L., Hudson, S. R., Walden, V. P., Granskog, M. A., Dorn, W., Kayser, M., and Maturilli, M.: A comparison of the two Arctic atmospheric winter states observed during N-ICE2015 and SHEBA, J. Geophys. Res.-Atmos., 122, 5716–5737, https://doi.org/10.1002/2016jd025475, 2017. a
He, C., Valayamkunnath, P., Barlage, M., Chen, F., Gochis, D., Cabell, R., Schneider, T., Rasmussen, R., Niu, G.-Y., Yang, Z.-L., Niyogi, D., and Ek, M.: Modernizing the open-source community Noah with multi-parameterization options (Noah-MP) land surface model (version 5.0) with enhanced modularity, interoperability, and applicability, Geosci. Model Dev., 16, 5131–5151, https://doi.org/10.5194/gmd-16-5131-2023, 2023. a, b
Holtslag, A. A. M. and Bruin, H. A. R. D.: Applied Modeling of the Nighttime Surface Energy Balance over Land, J. Appl. Meteorol. Clim., 27, 689–704, https://doi.org/10.1175/1520-0450(1988)027<0689:AMOTNS>2.0.CO;2, 1988. a, b, c
Holtslag, A. A. M., Svensson, G., Baas, P., Basu, S., Beare, B., Beljaars, A. C. M., Bosveld, F. C., Cuxart, J., Lindvall, J., Steeneveld, G. J., Tjernström, M., and Van de Wiel, B. J. H.: Stable Atmospheric Boundary Layers and Diurnal Cycles: Challenges for Weather and Climate Models, B. Am. Meteorol. Soc., 94, 1691–1706, https://doi.org/10.1175/BAMS-D-11-00187.1, 2013. a
Jacobs, A. F. G., van Boxel, J. H., and Shaw, R. H.: The dependence of canopy layer turbulence on within-canopy thermal stratification, Agr. Forest Meteorol., 58, 247–256, https://doi.org/10.1016/0168-1923(92)90064-B, 1992. a
Janjić, Z. I.: The Step-Mountain Eta Coordinate Model: Further Developments of the Convection, Viscous Sublayer, and Turbulence Closure Schemes, Mon. Weather Rev., 122, 927–945, https://doi.org/10.1175/1520-0493(1994)122<0927:TSMECM>2.0.CO;2, 1994. a
Kaimal, J. and Finnigan, J. J.: Atmospheric Boundary Layer Flows: Their Structure and Measurement, Oxford University Press, ISBN 0195062396, https://www.ebook.de/de/product/3257373/j_c_kaimal_j_j_finnigan_atmospheric_boundary_layer_flows_their_structure_and_measurement.html (last access: 22 April 2024), 1994. a
Kobayashi, H., Ikawa, H., and Suzuki, R.: AmeriFlux BASE US-Prr Poker Flat Research Range Black Spruce Forest (Ver. 3-5), AmeriFlux [data set], https://doi.org/10.17190/AMF/1246153, 2019. a
Mahat, V., Tarboton, D. G., and Molotch, N. P.: Testing above- and below-canopy representations of turbulent fluxes in an energy balance snowmelt model, Water Resour. Res., 49, 1107–1122, https://doi.org/10.1002/wrcr.20073, 2013. a, b, c
Mahrt, L., Sun, J., and Stauffer, D.: Dependence of Turbulent Velocities on Wind Speed and Stratification, Bound.-Lay. Meteorol., 155, 55–71, https://doi.org/10.1007/s10546-014-9992-5, 2014. a
Maillard, J., Ravetta, F., Raut, J.-C., Mariage, V., and Pelon, J.: Characterisation and surface radiative impact of Arctic low clouds from the IAOOS field experiment, Atmos. Chem. Phys., 21, 4079–4101, https://doi.org/10.5194/acp-21-4079-2021, 2021. a
Maillard, J., Raut, J.-C., and Ravetta, F.: SLM_Polar (1.0.0), Zenodo [code and data set], https://doi.org/10.5281/zenodo.8347090, 2023. a
Malingowski, J., Atkinson, D., Fochesatto, J., Cherry, J., and Stevens, E.: An observational study of radiation temperature inversions in Fairbanks, Alaska, Polar Sci., 8, 24–39, https://doi.org/10.1016/j.polar.2014.01.002, 2014. a
Mölder, M., Grelle, A., Lindroth, A., and Halldin, S.: Flux-profile relationships over a boreal forest – roughness sublayer corrections, Agr. Forest Meteorol., 98–99, 645–658, https://doi.org/10.1016/S0168-1923(99)00131-8, 1999. a
Monin, A. and Obukhov, A.: Basic laws of turbulent mixing in the surface layer of the atmosphere, Tr. Geofiz. Inst., Akad. Nauk SSSR, 24, 163–187, 1954. a, b
Nakai, T., Kim, Y., Busey, R. C., Suzuki, R., Nagai, S., Kobayashi, H., Park, H., Sugiura, K., and Ito, A.: Characteristics of evapotranspiration from a permafrost black spruce forest in interior Alaska, Polar Sci., 7, 136–148, https://doi.org/10.1016/j.polar.2013.03.003, 2013. a, b, c, d
Niu, G.-Y., Yang, Z.-L., Mitchell, K. E., Chen, F., Ek, M. B., Barlage, M., Kumar, A., Manning, K., Niyogi, D., Rosero, E., Tewari, M., and Xia, Y.: The community Noah land surface model with multiparameterization options (Noah-MP): 1. Model description and evaluation with local-scale measurements, J. Geophys. Res.-Atmos., 116, D12109, https://doi.org/10.1029/2010JD015139, 2011. a, b
Serreze, M. C., Kahl, J. D., and Schnell, R. C.: Low-level temperature inversions of the Eurasian Arctic and comparisons with Soviet drifting station data, J. Climate, 5, 615–629, https://doi.org/10.1175/1520-0442(1992)005<0615:lltiot>2.0.co;2, 1992. a
Sorbjan, Z. and Czerwinska, A.: Statistics of Turbulence in the Stable Boundary Layer Affected by Gravity Waves, Bound.-Lay. Meteorol., 148, 73–91, https://doi.org/10.1007/s10546-013-9809-y, 2013. a
Steeneveld, G. J., Van de Wiel, B. J. H., and Holtslag, A. A. M.: Modelling the Arctic Stable Boundary Layer and its Coupling to the Surface, Bound.-Lay. Meteorol., 118, 357–378, https://doi.org/10.1007/s10546-005-7771-z, 2006. a
Sterk, H. A. M., Steeneveld, G. J., and Holtslag, A. A. M.: The role of snow-surface coupling, radiation, and turbulent mixing in modeling a stable boundary layer over Arctic sea ice, J. Geophys. Res.-Atmos., 118, 1199–1217, https://doi.org/10.1002/jgrd.50158, 2013. a
Sugiura, K., Suzuki, R., Nakai, T., Busey, B., Hinzman, L., Park, H., Kim, Y., Nagai, S., Saito, K., Cherry, J., Ito, A., Ohata, T., and Walsh, J.: Supersite as a common platform for multi-observations in Alaska for a collaborative framework between JAMSTEC and IARC, JAMSTEC Report of Research and Development, 12, 61–69, https://doi.org/10.5918/jamstecr.12.61, 2011. a
Sun, J., Mahrt, L., Banta, R. M., and Pichugina, Y. L.: Turbulence Regimes and Turbulence Intermittency in the Stable Boundary Layer during CASES-99, Journal of Atmospheric Science, 69, 338–351, https://doi.org/10.1175/jas-d-11-082.1, 2012. a
van de Wiel, B. J. H., Moene, A. F., Steeneveld, G. J., Hartogensis, O. K., and Holtslag, A. A. M.: Predicting the Collapse of Turbulence in Stably Stratified Boundary Layers, Flow, Turbulence and Combustion, 79, 251–274, https://doi.org/10.1007/s10494-007-9094-2, 2007. a
Van de Wiel, B. J. H., Moene, A. F., Jonker, H. J. J., Baas, P., Basu, S., Donda, J. M. M., Sun, J., and Holtslag, A. A. M.: The Minimum Wind Speed for Sustainable Turbulence in the Nocturnal Boundary Layer, Journal of Atmospheric Science, 69, 3116–3127, https://doi.org/10.1175/jas-d-12-0107.1, 2012. a, b, c
Van de Wiel, B. J. H., Vignon, E., Baas, P., van Hooijdonk, I. G. S., van der Linden, S. J. A., van Hooft, J. A., Bosveld, F. C., de Roode, S. R., Moene, A. F., and Genthon, C.: Regime Transitions in Near-Surface Temperature Inversions: A Conceptual Model, Journal of the Atmospheric Sciences, 74, 1057–1073, https://doi.org/10.1175/jas-d-16-0180.1, 2017. a, b, c, d, e, f, g
van Hooijdonk, I. G. S., Donda, J. M. M., Clercx, H. J. H., Bosveld, F. C., and van de Wiel, B. J. H.: Shear Capacity as Prognostic for Nocturnal Boundary Layer Regimes, Journal of Atmospheric Science, 72, 1518–1532, https://doi.org/10.1175/JAS-D-14-0140.1, 2015. a
Vignon, E., van de Wiel, B. J. H., van Hooijdonk, I. G. S., Genthon, C., van der Linden, S. J. A., van Hooft, J. A., Baas, P., Maurel, W., Traullé, O., and Casasanta, G.: Stable boundary-layer regimes at Dome C, Antarctica: observation and analysis, Q. J. Roy. Meteor. Soc., 143, 1241–1253, https://doi.org/10.1002/qj.2998, 2017. a
Vignon, E., Hourdin, F., Genthon, C., Van de Wiel, B. J., Gallée, H., Madeleine, J.-B., and Beaumet, J.: Modeling the Dynamics of the Atmospheric Boundary Layer Over the Antarctic Plateau With a General Circulation Model, J. Adv. Model. Earth Sy., 10, 98–125, https://doi.org/10.1002/2017ms001184, 2018. a
Wyngaard, J. C. and Coté, O. R.: Cospectral similarity in the atmospheric surface layer, Q. J. Roy. Meteor. Soc., 98, 590–603, https://doi.org/10.1002/qj.49709841708, 1972. a
- Abstract
- Introduction
- Conceptual models of the surface layer
- Measurements at the Ameriflux Poker Flat Research Range
- Description of and suggested modifications to the WRF surface layer models
- Results
- Conclusions and perspectives
- Appendix A: Conceptual two-layer model development
- Code and data availability
- Author contributions
- Competing interests
- Disclaimer
- Acknowledgements
- Financial support
- Review statement
- References
- Abstract
- Introduction
- Conceptual models of the surface layer
- Measurements at the Ameriflux Poker Flat Research Range
- Description of and suggested modifications to the WRF surface layer models
- Results
- Conclusions and perspectives
- Appendix A: Conceptual two-layer model development
- Code and data availability
- Author contributions
- Competing interests
- Disclaimer
- Acknowledgements
- Financial support
- Review statement
- References