the Creative Commons Attribution 4.0 License.
the Creative Commons Attribution 4.0 License.
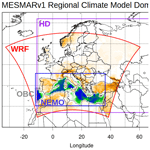
MESMAR v1: a new regional coupled climate model for downscaling, predictability, and data assimilation studies in the Mediterranean region
Yassmin Hesham Essa
Vincenzo de Toma
Alessandro Anav
Gianmaria Sannino
Rosalia Santoleri
Chunxue Yang
Regional coupled and Earth system models are fundamental numerical tools for climate investigations, downscaling of predictions and projections, process-oriented understanding of regional extreme events, and many more applications. Here we introduce a newly developed coupled regional modeling framework for the Mediterranean region, called MESMAR (Mediterranean Earth System model at ISMAR) version 1, which is composed of the Weather Research and Forecasting (WRF) atmospheric model, the NEMO oceanic model, and the hydrological discharge (HD) model, coupled via the OASIS coupler. The model is implemented at about ∘ of horizontal resolution for the ocean and river routing, while roughly twice coarser for the atmosphere, and it is targeted to long-term investigations. We focus on the evaluation of skill score metrics from several sensitivity experiments devoted to (i) understanding the best vertical physics configuration for NEMO, (ii) identifying the impact of the interactive river runoff, and (iii) choosing the best-performing physics–microphysics suite for WRF in the regional coupled system. The modeling system has been developed for downscaling reanalyses and long-range predictions, as well as coupled data assimilation experiments. We then formulate and show the performance of the system when weakly coupled data assimilation is embedded in the system (variational assimilation in the ocean and spectral nudging in the atmosphere), in particular for the representation of extreme events like intense Mediterranean cyclones (i.e., medicanes). Finally, we outline plans for future extension of the modeling framework.
- Article
(6086 KB) - Full-text XML
- BibTeX
- EndNote
Climate changes are known to pose severe threats to the safety and livelihood of the human population as well as to marine and terrestrial ecosystems. This, in turn, requires increasingly accurate and spatially detailed climate services, many of which require the use of regional climate models (RCMs) capable of achieving horizontal resolutions that global climate models (GCMs) cannot achieve due to computational limits (Giorgi, 2020). RCMs are usually atmospheric models with physics suites targeted to long-term simulations; in recent years, several research centers have added an interactive ocean component to form atmosphere–ocean coupled RCMs, typically implemented at resolutions of 1–20 km. Thus, these coupled RCMs are generally able to resolve the mesoscale eddies in the ocean and provide a superior representation of the atmosphere–ocean exchanges and local energetics, thus adding value to global climate simulations (e.g., Rockel et al., 2008; Feser et al., 2011; Rummukainen, 2016). RCMs are used to downscale global reanalyses and thus for monitoring purposes (e.g., Rockel, 2015) or to downscale short- and long-term forecasts and climate projections over areas of interest – for example, through the Coordinated Regional Climate Downscaling Exercises (CORDEX) initiatives; see, e.g., Ruti et al. (2016) and Reale et al. (2022a, b) for the MED-CORDEX exercise over the Mediterranean region. Indeed, the prediction of high-impact weather and climate events can benefit significantly from the use of RCMs, when, for instance, the enhanced representation of net heat fluxes is important (see, e.g., Akhtar et al., 2018). The reader is referred, for example, to the review by Giorgi et al. (2019) for a historical perspective on the development of RCMs and open challenges, such as uncertainties in high-resolution configurations, misspecification of lateral boundaries and radiative forcing (Foley, 2010), and the use of multimodel simulations (Rummukainen et al., 2015).
In addition, RCMs provide a numerical tool for process-oriented investigations, data assimilation and observing network assessments, and predictability experiments. Coupled data assimilation in regional climate models is still largely unexplored, besides some pioneering applications (see, e.g., Li et al., 2020), but represents a high potential for regional predictability gain, linked, among several factors, to the correction of imbalances at initial time and/or at the lateral boundaries, as well as the maximization of the benefits of the regional observing networks (e.g., Penny et al., 2019).
In the Mediterranean Sea, heat content anomalies are an important precursor of society-impacting and strongly (air–sea) coupled phenomena, such as for instance heavy precipitation events (HPEs). This makes the use of RCMs appealing for both short-range predictability problems and long-term climate applications such as regional reanalyses and the dynamical downscaling of long-range predictions. The retrospective analyses of the most devastating HPEs have outlined the importance of the anomalously warm sea surface temperatures in the Mediterranean cyclogenesis, which are responsible for enhancing moisture fluxes and convection, associated also with complex interactions with orography (e.g., Lebeaupin Brossier et al., 2013; Cassola et al., 2016). The importance of anomalous sea surface temperature (SST) has been proven crucial, especially in the western Mediterranean region, but its impact encompasses all Mediterranean HPEs (e.g., Duffourg and Ducrocq, 2011). Consequently, recent studies have shown that predictive skills of weather forecasts made by numerical weather prediction (NWP) systems significantly increase when the atmospheric models are coupled to ocean models, allowing interactive feedback with the ocean (Lebeaupin Brossier et al., 2015; Hirons et al., 2018).
Within the Mediterranean region, there is also an important occurrence of severe mesoscale cyclones with tropical-like features, referred to as medicanes (Mediterranean hurricanes) (Flaounas et al., 2022). In a changing climate, such phenomena are expected to increase in intensity (Cavicchia et al., 2014). Coupled models can enhance the correct simulation and prediction of medicanes through, e.g., high-resolution downscaling modeling approaches (Cavicchia and von Storch, 2012), correct initialization of coupled simulations (Ricchi et al., 2017), and air–sea feedbacks (Akhtar et al., 2014), including feedbacks between anomalously warm sea surface temperature and features like atmospheric rivers (Flaounas et al., 2022). Additionally, strongly coupled data assimilation (e.g., Storto et al., 2018b) is expected to improve the representation of these strongly coupled events, because of the optimization of the observing network (Li and Toumi, 2018; Zhang and Emanuel, 2018) and the importance of the upper-ocean heat content in modulating the hurricane intensity (Scoccimarro et al., 2018). Therefore, one focus of the present work is to evaluate the sensitivity of the prediction of intensity and track of past events of medicanes to different configurations of the data assimilation system.
In this article, we present the first consolidated version of a regional climate model developed at the Institute of Marine Sciences (ISMAR) of the National Research Council of Italy (CNR) in collaboration with the Italian National Agency for New Technologies, Energy and Sustainable Economic Development (ENEA). The system is called MESMAR (Mediterranean Earth System model at ISMAR) and, in the configuration presented here, includes atmosphere, ocean, and hydrology components at a spatial resolution of 7–14 km. It covers the Mediterranean basin and is intended for downscaling and predictability exercises and as a test bed for coupled data assimilation experiments. A unique feature of our system is that it embeds the latest versions of state-of-the-art numerical models for representing oceanic, atmospheric, and hydrological processes, with a full coupling setup, and, more importantly, that the system includes a state-of-the-science data assimilation capability. It is the first time, to our knowledge, that a regional climate model over the Mediterranean region can ingest oceanic and atmospheric observational information.
In the following sections, we detail the configuration of the system (Sect. 2) and the results from a few notable sensitivity experiments that led to the reference configuration (Sect. 3); we then assess the ocean heat budget in the reference, assimilation-blind, experiment (Sect. 4). Next, we focus on the configuration and assessment metrics in a series of weakly coupled assimilation experiments (Sect. 5). Finally, Sect. 6 concludes and discusses the main achievements and plans.
We detail in this section the configuration of the coupled model components, including the coupler settings.
2.1 Atmospheric model
The atmospheric model component is the Weather Research and Forecasting (WRF) community model, version 4.3.3 (Skamarock et al., 2021), implemented over the Mediterranean and European regions at 15 km of horizontal resolution and 41 vertical hybrid levels. The WRF domain (Fig. 1) extends from northern Africa (south) to the middle of the Scandinavian Peninsula (north), as well as from the North Atlantic (west) to western Asia (east). The domain is adopted after Anav et al. (2021), using the geographical static data (e.g., topography, land use, leaf area index, albedo, among others) provided by WRF through the WRF Preprocessing System (WPS) package and repository.
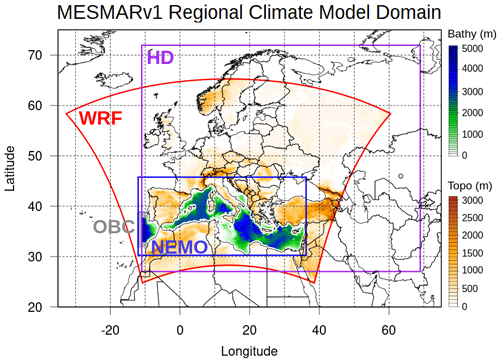
Figure 1Computational domain of the MESMAR (v1) regional climate model, showing the extension of the three modeling components (WRF in the atmosphere, NEMO in the ocean, and HD as hydrology model). Filled contours represent the bathymetry and topography over the NEMO and the WRF domains, respectively. The open boundary condition (OBC) shaded area shows the region of application of the NEMO lateral boundary conditions.
WRF uses a non-hydrostatic core, and the time step is set equal to 60 s. The suite of physical, microphysical, and subgrid parametrization options comes in most cases from the MED-CORDEX sensitivity experiments (see, e.g., Fita et al., 2019, for an older setup) or has been specifically tested (see also Sect. 3). In particular, the Thompson et al. (2008) microphysics is used, while the radiation is modeled with the rapid radiative transfer model for general circulation models (RRTMG; Iacono et al., 2008). The land model component is the Noah-MP (multi-physics) land surface model (Niu et al., 2011) with four soil layers. The Mellor–Yamada turbulent closure of Nakanishi and Niino (2006) is adopted, while the Grell and Freitas (2014) cumulus parametrization is used.
Lateral boundary conditions are imposed over a 10-grid-point sponge layer closer to the four boundaries, through a classical relaxation formulation with linear ramping functions across the 10 grid points (Davies and Turner, 1977). In hindcast mode (e.g., for simulations or reanalysis downscaling), the lateral boundary conditions are taken from 3-hourly fields of the European Centre for Medium-Range Weather Forecasts (ECMWF) ERA5 reanalysis (Hersbach et al., 2020).
2.2 Ocean model
The primitive-equation NEMO ocean model (Madec et al., 2017), version 4.0.7, is the ocean model component of MESMAR v1, developed and maintained by the homonymous NEMO consortium. The model covers the Mediterranean Sea region from an Atlantic box to the Dardanelles (see Fig. 1) over a regular domain at a horizontal resolution of about 7 km and with 72 vertical depth levels with partial steps at the bottom. The (baroclinic) model time step is set to 450 s, while the barotropic time step is equal to 6 s, using the split-explicit free-surface scheme (Shchepetkin and McWilliams, 2005).
In our setup of NEMO, the shortwave radiation extinction coefficients are specified using the three-band spectral discretization of Morel and Maritorena (2001), with three-dimensional attenuation coefficients deduced from the chlorophyll concentration, taken in turn from the level-4 (L4) monthly fields of Brewin et al. (2015) and distributed by the Copernicus Marine Service. Horizontal diffusivity (modeled with a Laplacian operator) and viscosity (modeled with a bi-Laplacian operator) coefficients are set equal to 80 m s−2 and 4.5×109 m2 s−4, respectively. These values are increased by 20 % in the proximity of the Strait of Gibraltar and the Aegean Sea. The generic length scale (GLS) scheme (Umlauf and Burchard, 2003) is used for vertical mixing; GLS is a general framework for vertical mixing, and we adopt the Mellor and Yamada (1982) turbulence closure, with the stability function of Canuto et al. (2001). An alternative configuration of the system (shown for comparison in Sect. 3.2) uses the turbulent kinetic energy (TKE) vertical mixing scheme, implemented with the same parameters as in Storkey et al. (2018).
In hindcast mode, the lateral boundary conditions are provided by the ECMWF ocean reanalysis ORAS5 (Zuo et al., 2019). Lateral boundary conditions are imposed as follows: barotropic velocities and sea surface height through the Flather scheme (Flather, 1994), baroclinic velocities specified at the boundary grid points from the external sources, and temperature and salinity through a flux relaxation scheme that gradually relax the tracer fields towards the external fields over the 10 inner grid points closer to the boundaries. ORAS5 was chosen among the Copernicus Marine Service reanalyses (Global Reanalysis Ensemble Product, Storto et al., 2019; and GLORYS12, Lellouche et al., 2021) as it provides the best sea surface height validation skill score statistics against altimetry data compared to the other reanalyses. In preliminary experiments, the average root-mean-square error of 3.5 cm was found when using ORAS5, compared to 4.0 cm when other reanalyses were used (not shown), while temperature and salinity have comparable skill scores across the experiments using different GREP reanalyses as lateral forcing.
The river runoff is imposed through the hydrological discharge model (see next section), except at the Dardanelles, where it is set equal to the monthly climatology of the Black Sea outflow into the Mediterranean Sea, as given by Kourafalou and Barbopoulos (2003).
2.3 Hydrological discharge model
MESMAR v1 includes interactive river runoff, estimated by the hydrological discharge (HD) model, version 5.1 (Hagemann et al., 2020), developed and maintained by Helmholtz-Zentrum Hereon. The HD model implements a horizontal resolution of ∘ degree over the European continent, and it contains specific developments for coupled simulations, including the support for the OASIS coupler (Ho-Hagemann et al., 2020). Its hydrological core stems from the MPI model (Hagemann and Dümenil Gates, 2001).
The HD time step is set to 30 min, which is generally a higher frequency than most implementations, and it is chosen to ease the coupler exchanges (see below). A discharge-dependent river flow velocity is used. Additionally, we have modified the routine responsible to map the river discharge onto oceanic NEMO points to include a smoothing function, which conservatively spread the discharge from one ocean point to the 5×5 neighboring grid points. This is required to avoid instability problems where large discharge occurs.
2.4 Coupler
The coupler used by MESMAR v1 is OASIS (OASIS3-MCT_5.0, Craig et al., 2017), which is a flexible parallel coupler developed by the Centre Européen de Recherche et de Formation Avancée en Calcul Scientifique (CERFACS). We use first-order conservative remapping to interpolate all the fields exchanged from one model to the other; the coupling frequency is set to 30 min for all exchanged fields.
Table 1 summarizes the fields exchanged through the coupler from and to the different model components. Note that the air–sea fluxes over the oceans are computed within WRF, following the surface scheme of Janjić (1994). Additionally, the skin sea surface temperature scheme of Zeng and Beljaars (2005) is adopted to diagnose the diurnally varying skin SST within the WRF bulk formulas. WRF passes also the atmospheric pressure fields to NEMO, for the latter to account for the inverse barometer effect in the sea level computations (see, e.g., Wunsch and Stammer, 1997). Note also that WRF communicates to HD to provide fields of surface and subsurface runoff, which are then routed to the river mouths and remapped onto the NEMO coastal points directly by HD.
2.5 Experimental setup and verification datasets
Depending on the specific application and test, several setups have been used for MESMAR v1. In general and unless otherwise specified, atmospheric initial conditions are provided by the ERA5 atmospheric reanalysis; oceanic initial conditions are provided by the GLORYS12 ocean reanalysis (Lellouche et al., 2021), which has a horizontal resolution close to our model setup; river routing initial conditions are provided by a previous standalone run of the HD model (that is, run in uncoupled mode with input surface and subsurface runoff from a standalone WRF simulation); and coupler initial conditions (i.e., the exchanged fields) are set to zero. No model spinup is considered for the experiments presented hereafter. Lateral boundary conditions are given by ERA5 and ORAS5, for the atmospheric and oceanic lateral forcing, respectively, as specified in Sect. 2.2 and 2.3, unless otherwise specified. The system has run on the ECMWF Atos machine, using 171 cores (160 dedicated to WRF, 10 to NEMO, and 1 to HD). One month of simulation has taken approximately 5 wall-clock hours, i.e., about 10 000 core hours per simulated year.
For verifying the experiments, we use several independent datasets. EN4 profile and objective analyses (Good et al., 2013) are used for the verification of ocean temperature and salinity. Daily SST analyses from the Copernicus Marine Service (Pisano et al., 2020) are used for the verification of sea surface temperature from the experiments; E-OBS terrestrial data (Cornes et al., 2018) are used for the verification of near-surface atmospheric variables; ocean heat content is retrieved from the ocean monitoring indicator (OMI) of the Copernicus Marine Service (https://doi.org/10.48670/moi-00261); and the NOAA/ESRL Radiosonde Database (https://ruc.noaa.gov/raobs/, last access: 14 August 2023) is used for the verification of upper-air model variables. In the verification of the assimilation experiments (Sect. 5), skill score metrics are computed on the background fields, i.e., before the observations are ingested in the system.
Selected sensitivity experiments are presented in this section to provide a rationale for the choice of individual schemes or parametrizations.
3.1 Impact of the interactive river discharge
The effect of the interactive river runoff is summarized in this section. In particular, we have tested for 2 years (2015–2016) the use of the climatological runoff, taken from the ORCA12 standard configuration of NEMO and adapted by Bourdalle-Badie and Treguier (2006) from the Dai and Trenberth (2002) compilation of river runoff data. This experiment corresponds to the uncoupled runoff, namely what is customarily done in most oceanic applications (e.g., ocean reanalyses; see, e.g., Storto et al., 2019), and it is compared with the standard MESMAR configuration where the river discharge is provided interactively by the HD model. This exercise aims at assessing the qualitative impact of the WRF–HD-derived runoff; however, the experimental period for this test is relatively short, and assessing the detailed impact of the interannual variations requires dedicated multi-decadal experiments, which are expensive and beyond the scope of the present paper. Thus, we mostly verify that the land–ocean coupling configuration leads to satisfactory results in terms of the Mediterranean freshwater budget over the short 2-year experimental period, although we cannot be conclusive on longer timescales.
Differences between the climatological river runoff and the one derived from WRF–HD are visible in Fig. 2, in terms of total discharge and area-averaged sea surface salinity for the whole Mediterranean Sea (excluding the Atlantic box from the model domain). In general, the interactive land–ocean coupling leads to a total discharge smaller than that with climatological runoff and a shift in the minima/maxima of the yearly cycle (minima from September–October to November–December, maxima from February–May to April–August). Compared to the bias-corrected river discharge from JRA55-do (Tsujino et al., 2018), the difference in discharge is lower than the ORCA12 climatology, at least for the year 2016, but the seasonal offset is more pronounced. Accordingly, the sea surface salinity (SSS) increases year-round and results in a lower bias compared to the UK Met Office (UKMO) EN4 SSS objective analyses (Good et al., 2013), as shown in Fig. 2 (bottom panel). In particular, the time-averaged map of sea surface salinity anomalies (Fig. 3, top panel) caused by the interactive land–ocean coupling highlights the salinity increase in several coastal areas of the Mediterranean Sea, particularly the Gulf of Lion, the Adriatic Sea, and the Levantine basin, with values exceeding 1 psu along the major river mouths of the Mediterranean basin. Slight freshening of the surface waters is visible in the Aegean Sea, off Sicily, and in front of major lagoons (Akyatan and Lake of Tunis), but the overall effect of the interactive river discharge is a salinification of the surface waters by 0.06 psu on average, during the 2-year study period. By comparing the SSS of the simulation without interactive discharge with the EN4 SSS analyses (bottom panel of Fig. 3), it is possible to see that in many regions, such as the Ionian, Adriatic, and Aegean seas, among many coastal areas, the corrections borne by the interactive discharge go in the direction of mitigating the salinity bias.
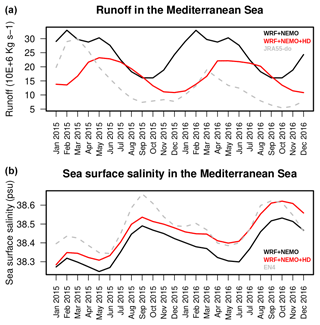
Figure 2Monthly means of river discharge into the ocean (a) and sea surface salinity (b) averaged over the Mediterranean Sea from the experiments with and without the interactive river discharge. Also shown for reference are the bias-corrected discharge from the JRA55-do reanalysis and the sea surface salinity from the UKMO EN4 objective analyses.
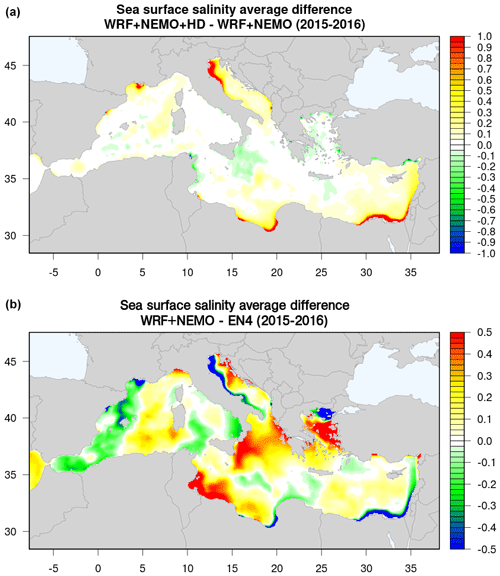
Figure 3Average sea surface salinity difference (2015–2016) between the experiments with and without the interactive river discharge (a) and between the experiment without the interactive discharge and the EN4 sea surface salinity analyses (b) over the Mediterranean Sea. Values are in practical salinity units (psu).
Skill score metrics computed against all available in situ profiles extracted from the UKMO EN4 profile dataset are shown in Fig. 4. Profiles of bias and RMSE of salinity confirm the positive impact of the land–ocean coupling that penetrates up to about 200 m of depth. Fresh biases are significantly mitigated in the top 100 m, while RMSE shows improvements from the surface to the halocline. The results indicate that the WRF–HD–NEMO system has great potential for improving the representation of the water cycle in the Mediterranean region. Indeed, the use of the interactive river runoff allows us to close the water cycle in the regional basin, besides the improvement of the performances of the regional coupled model in representing the salinity variations.
3.2 NEMO vertical physics
Several sensitivity tests were performed to identify the best vertical mixing configuration for the NEMO model. Here, we show the results from the two best-performing implementations of the GLS and TKE schemes (as described in Sect. 2.2). Two coupled simulations were performed for the period 1993–2021. Figure 5 shows the winter and summer bias of sea surface temperature computed against the daily SST analyses. Compared to the GLS scheme, the TKE induces enhanced mixing in summer (that is, weakened stratification), with colder biases in most areas and notably in the Adriatic Sea. GLS has overall positive and smaller biases than TKE in the southern part of the domain, which leads to stronger stability of the model (that is, stronger stratification). In winter, TKE has a relevant warm bias, especially in the western basin. These biases are propagated onto the near-surface air temperature (not shown), indicating that the GLS vertical mixing implementation has a better impact over the sea, while the differences led by the use of the two schemes are in general negligible over land, where biases are dominated by other factors, such as land surface processes (e.g., Davin et al., 2016).
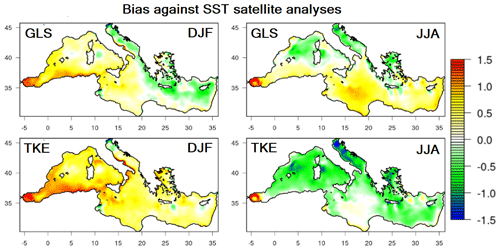
Figure 5Winter (DJF) and summer (JJA) biases of sea surface temperature against satellite-based analyses from the Copernicus Marine Service for the GLS and TKE experiments with different oceanic vertical mixing schemes.
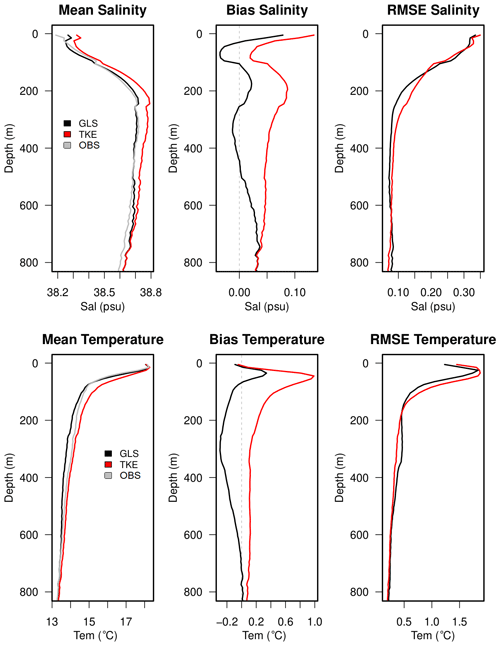
Figure 6As for Fig. 4 but for the mean, bias, and RMSE profiles against Argo floats for the GLS and TKE experiments with different oceanic vertical mixing schemes. Mean profiles also report data from the observations (in gray).
The surface signature is confirmed by the skill score profiles against in situ data (Fig. 6), which highlights the better performances obtained with GLS than TKE, visible up to about 800 m of depth. Looking at salinity, the TKE shows too many salty waters year-round. Furthermore, the RMSE is smaller with GLS in the top 50 m of depth and between 150 and 600 m of depth. At the sea surface, both simulations are too salty compared to the mean observed profile in the upper 50 m (top middle panel of Fig. 6), yet the GLS scheme significantly mitigates the salinity overestimation; elsewhere, the impact is neutral. Year-round, the improvements in temperature are visible to about 200 m of depth. The TKE-enhanced mixing leads to a less sharp thermocline compared to GLS and the mean observed profile. Moreover, GLS shows a significant bias reduction (0.4 ∘C with GLS against almost 1 ∘C with TKE) on the temperature peak in the upper 50 m. This reduction occurs mostly near the surface (in summertime) and around the thermocline (in wintertime) (not shown).
3.3 WRF configuration
In the initial phase of the MESMAR implementation, we performed many sensitivity experiments, both coupled and uncoupled, to identify the best-performing suite of physics and microphysics schemes. Here, we report results from the configurations for which we performed long experiments (1993–2021) in coupled configuration (i.e., with NEMO and HD). In addition to the configuration described in detail in Sect. 2 (and called REF), we performed two other experiments: the first (W01) has different microphysics (Morrison two moments), surface layer (revised MM5 scheme), boundary layer (YSU), and cumulus (Betts–Miller–Janjic) schemes, compiled together similarly to a previous configuration of WRF as in Anav et al. (2021). The second experiment (W02) is as W01 but with the less advanced Noah land surface model, replacing Noah-MP in W01 and REF. Table 2 and Figs. 7–9 report validation statistics and climatology maps for wind speed at 10 m, air temperature at 2 m, and total precipitation, compared to the E-OBS data.
Table 2List of sensitivity experiments performed, with the list of physics and microphysics parametrizations used in WRF and mean absolute error results against the E-OBS dataset for wind speed (m s−1), 2 m temperature (K), and precipitation (mm d−1). MAE values report the statistics year-round and, in brackets, for winter (DJF) and summer (JJA) separately.
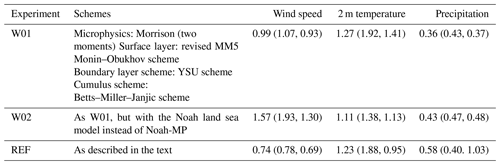
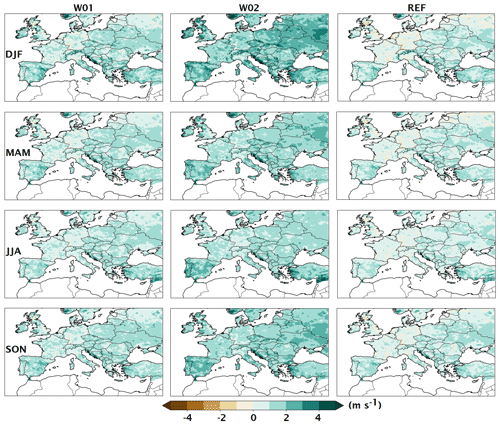
Figure 7Differences between the three experiments presented in the text and the E-OBS wind speed (m s−1) for the four seasons: DJF, MAM, JJA, and SON.
The comparison with E-OBS wind speed (Fig. 7) indicates, year-round, that all experiments have a positive bias and that the best performances are achieved by REF, which shows a rather low bias between 0 and 2 m s−1 (0.74 m s−1 on average; see Table 2). The other two experiments (especially W02 in autumn and wintertime) exhibit more pronounced positive biases. Differences in 2 m temperature performances are smaller (Fig. 8), with W02 on average outperforming the two others. All the experiments reproduce the winter cold bias on northeastern Europe, already found in several configurations of WRF (see, e.g., Anav et al., 2021), with W01 exhibiting the coldest bias therein. Unlike W01 and W02, REF does not exhibit a large warm bias in summertime over Europe. Finally, in the comparison with precipitation from E-OBS (Fig. 9), REF is found to be the wettest model during summer. While it is difficult to identify precisely the causes of the wet bias, previous studies identify the combination of the microphysics and planetary boundary layer (PBL) scheme as the most responsible for the summer wet biases (e.g., Mooney et al., 2013). Although there is no configuration better than the others concerning all atmospheric parameters, the reference configuration is chosen, as it provides the best near-surface atmospheric circulation and keeps reasonably low biases in air temperature. We have also verified the experiments against oceanic observations (sea surface temperature and height), but differences are not statistically significant and have not been shown here.
In this section, we evaluate the Mediterranean Sea warming and heat budget for the period 1993–2021 from a long MESMAR simulation, which implements the optimal configuration of WRF and NEMO as detailed in the previous section. The Mediterranean Sea is a climate change hot spot, which warms at a higher rate than the global ocean (Lionello and Scarascia, 2018) and whose warming is expected to accelerate in the future (Soto-Navarro et al., 2020; Reale et al., 2022a, b; Cos et al., 2022). Thus, assessing the potential of the coupled regional model in capturing the ocean heat content (OHC) variability is a fundamental exercise to validate its applicability for climate monitoring.
The reference simulation does not contain any observational constraint, besides the lateral boundaries forced in the ECMWF ERA5 and ORAS5 atmospheric and oceanic reanalyses. Therefore, we do not expect the warming rate to be close to that observed, as both the atmosphere and ocean models are free to evolve following their internal physics. However, the coupled model simulation may still be able to capture to some extent the interannual variations of the ocean heat content. As a preliminary exercise, we have verified that the accuracy of this simulation in the ocean is rather steady over time when the temperature is compared to verifying in situ observations over the basin (not shown).
The top left panel of Fig. 10 shows the OHC from MESMAR and, for comparison, the OHC compiled as the ocean monitoring indicator (OMI) of the Copernicus Marine Service. The OMI is the ensemble mean of several global and regional sources that include both objective analyses and reanalyses. Results show that the increase in OHC is underestimated in MESMAR: the warming rate for the full period, calculated as the linear trend of OHC, is 1.39 W m−2 in the OMI and 0.24 W m−2 in MESMAR. However, the interannual variations of OHC match very well between the two time series. This is shown by the dashed red curve in the top left panel of Fig. 10, which is the MESMAR interannual variations with the linear trend rectified to match that of the Copernicus Marine Environment Monitoring Service (CMEMS) OMI. In this case, the interannual variations almost overlap with those from CMEMS OMI. Events like the 2002–2005 cooling and the successive sharp warming during 2006–2011 (mostly due to the North Atlantic forcing variability; see, e.g., Iona et al., 2018) are, indeed, well captured.
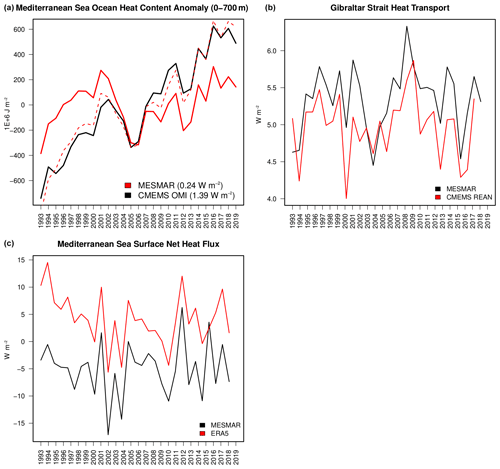
Figure 10Mediterranean Sea upper-ocean (0–700 m) ocean heat content (OHC, a), incoming heat transport at the Strait of Gibraltar (b), and net air–sea heat flux (downward, c), during the 1993–2020 period for the MESMAR reference simulation. Also shown for comparison are values of OHC from the Copernicus Marine Service ocean monitoring indicator (OMI), Gibraltar heat transport from the Copernicus Marine Service regional reanalysis, and net air–sea flux from the ECMWF ERA5 reanalysis. Panel (a) also reports the OHC time series from MESMAR, rectified with the observed long-term OHC trend (dashed red line), while its legend indicates the OHC linear trend (in brackets).
To understand the representation of the causes of the warming in MESMAR, we have analyzed separately the two warming sources in the Mediterranean basin, using a box approach where OHC tendencies equal the sum of lateral heat transports and net downward air–sea heat flux and assuming that heat contributions from rivers and the Dardanelles Strait are negligible (Harzallah et al., 2018). The bottom panel of Fig. 10 shows the net downward air–sea heat flux in MESMAR and ERA5; the interannual variations in the two datasets are very well correlated; however, long-term values indicate an important underestimation of the MESMAR net fluxes, equal to W m−2 against 4.15±4.78 W m−2 (ERA5); namely, the average difference is large and exceeds 9 W m−2. It also should be noted that during the first 5 years, the net heat flux in ERA5 appears unrealistically large, even exceeding 10 W m−2. It is well known that the ensemble dispersion of models and reanalyses in simulating the net heat flux is very large (e.g., Harzallah et al., 2018); however, long-term closed heat budget in the Mediterranean Sea requires the net heat flux to be slightly negative in order to compensate for the positive heat inflow from the Strait of Gibraltar (see Jordà et al., 2017, and later in this section), implying an overestimation of ERA5 and an underestimation of MESMAR.
In terms of heat transport, the top right panel of Fig. 10 shows the incoming heat transports at the Strait of Gibraltar from MESMAR and the Copernicus Marine Service regional reanalysis (Escudier et al., 2021). The two time series show close variations and equal long-term means (between the error bars), equal to 5.34±0.44 W m−2 (MESMAR) and 4.97±0.43 W m−2 (CMEMS). The values are also well aligned with other in situ and model-based estimates, for instance, 5.2, 5.0, and 4.9 (respectively MacDonald et al., 1994; Astraldi et al., 1999; Harzallah et al., 2018).
The very close values of lateral incoming heat transports mean that differences are due only to the atmospheric radiative forcing into the ocean. In particular, MESMAR leads to too small an air–sea flux, while the use of ERA5 leads to much too warm a flux. The CMEMS reanalysis, which assimilates data, can instead rectify the fluxes.
Concerning regional warming (Fig. 11, left panels), MESMAR provides similar patterns compared to the CMEMS reanalysis, with maximum warming on the eastern side of the Mediterranean. This confirms the ability of the coupled regional model to capture interannual variations and spatial patterns. The two right panels show the net air–sea heat flux, which reveals that the MESMAR underestimation of heat uptake from the atmosphere is rather homogenous, as patterns are close to those of the ERA5 reanalysis; the largest differences are located in the Ionian basin, and there exists some correspondence between the underestimation of air–sea fluxes therein and the less pronounced warming (Fig. 11, bottom panels). In particular, we found that both turbulent fluxes (sensible and latent heat) are, together, overestimated by about 5 W m−2 in MESMAR, and the incoming solar radiation is underestimated by another 5 W m−2, compared to ERA5.
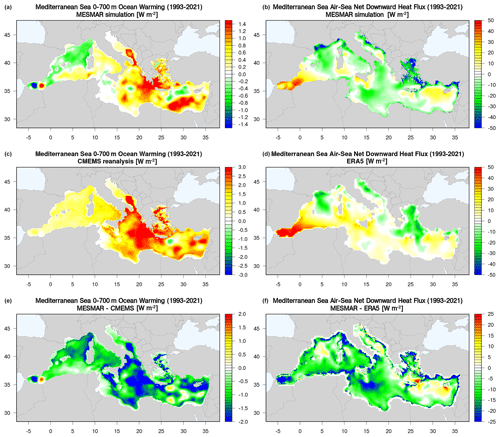
Figure 11Upper-ocean (0–700 m) ocean warming (OHC linear trend) from the MESMAR reference simulation (a) and the Copernicus Marine Service regional reanalysis (c). Long-term mean net air–sea flux from the MESMAR reference simulation (b) and the ECMWF ERA5 reanalysis (d). Panels (e) and (f) report the differences between MESMAR and the reanalysis dataset.
5.1 Weakly coupled assimilation configuration
One important application of regional coupled models is the possibility to downscale multi-decadal climate reconstructions from both atmospheric and oceanic reanalyses (e.g., Vannucchi et al., 2021) and short-range predictability studies. To this end, MESMAR implements a weakly coupled data assimilation system, where the oceanic state is constrained by a three-dimensional variational (3DVAR) data assimilation system (Storto et al., 2018) and the atmospheric state by a spectral nudging scheme (Choi and Lee, 2016), which is already part of the WRF modeling system.
The 3DVAR scheme implements stationary background-error covariances estimated from the dataset of differences between two long-term simulations with different physics options in both the WRF and NEMO configurations. In particular, this anomaly dataset is obtained for the period 1994–2020 from the differences between two experiments with different ocean and atmospheric physics, shown in the previous sections (one configuration embedding the TKE vertical mixing scheme and other atmospheric schemes as in W02; see Sect. 3.3). Preliminary tests (not shown) indicated that using pairs of experiments with different physics for estimating background-error covariances led to better skill scores than the use of anomalies from climatology from a long-term simulation (see Storto and Randriamampianina, 2010; Storto et al., 2014, for a discussion on the approach to estimate background-error covariances). Background-error covariances are modeled through the application of multi-variate spatially varying empirical orthogonal functions (EOFs) – for vertical covariances – and a first-order recursive filter with spatially varying correlation length scales for the horizontal correlations, as in Storto et al. (2014).
The assimilated observations include all in situ profiles (XBT and CTD casts, moorings, floats, and gliders), extracted from the UKMO EN4 dataset (Good et al., 2013). Observational errors and variational quality control are adopted as in Storto (2016), which allows for non-linear weighting of the observations.
At the sea surface, a relaxation scheme is applied to correct air–sea heat and freshwater fluxes by nudging the sea surface temperature (SST) and sea surface salinity (SSS) to SST and SSS analyses, taken from the CNR ISMAR SST analyses (Pisano et al., 2020) and the UKMO EN4 objective analyses (Good et al., 2013), respectively. The relaxation timescales are set equal to 15 and 300 d for SST and SSS, respectively, after several preliminary sensitivity experiments aimed to identify the best-scoring configuration (not shown). The use of surface relaxation allows time-consistent ingestion of surface data for multi-decadal simulations and results in improved near-surface skill scores.
In the atmosphere, a spectral nudging scheme is applied in WRF, which nudges the large-scale component of wind, temperature, and humidity toward the ECMWF ERA-5 reanalysis (Hersbach et al., 2020). Large scales are defined based on fast Fourier transform (FFT) decomposition, with the six and five wavenumber cutoffs, which are equivalent to about 850 km in MESMAR (see, e.g., Omrani et al., 2015, for more information on the WRF spectral nudging capability). The nudging timescale is equal to 1 h for wind and temperature and 1 d for humidity. For comparison, full-field nudging, namely with the same nudging timescales as spectral nudging but applied to all spatial scales, is also shown in the next section, to evaluate different ways to constrain the atmospheric fields.
The assimilation time window is set to 3 d, namely every 3 d the ocean state is corrected by employing the 3DVAR analysis increments; in reanalysis mode, the atmospheric spectral nudging is continuous and uses 3-hourly fields from ERA5.
5.2 Experiments and results
Several experiments have been performed to identify the best-scoring configuration for oceanic and atmospheric data assimilation. Here, we show only the impact of activating different components of data assimilation, combining, in particular, the cases of no-assimilation (OC0) and assimilation (OC1), in the ocean, and no-assimilation (AT0), full-field (AT1), and spectral nudging (AT2), in the atmosphere. The ocean assimilation experiments OC1 include both the surface relaxation and the variational assimilation of profiles; in the non-assimilative experiments OC0, both are switched off. The summary of experiments, along with selected validation skill scores, is reported in Table 3. All these experiments have been run for 3 years (2018–2020) and initialized from the reference simulation shown in Sect. 4.
Table 3List of experiments performed and shown in Sect. 5 of the text, with different assimilation setups (AT0, AT1, and AT2 refer to no atmospheric data assimilation, full-filed nudging, and spectral nudging, respectively; OC0 and OC1 refer to no oceanic data assimilation and variational ocean data assimilation, respectively). Right-side columns report total skill scores as RMSE for some selected parameters: air temperature at 850 hPa (K), wind speed in the layer 1000–850 hPa (m s−1), 500 hPa geopotential (m), SST (∘C), seawater temperature (∘C), and salinity (psu) in the top 50 m of depth.
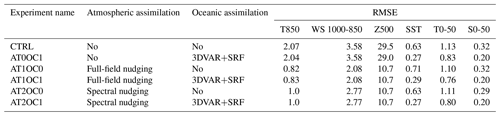
Skill scores in Table 3 indicate slight improvements (1 % to 2 %) on the atmospheric skill scores when the ocean data assimilation is switched on (AT0OC1 versus CTRL) and similarly for the impact on oceanic skill scores when atmospheric data assimilation is active (e.g., AT2OC0 versus CTRL). The largest impact on the skill scores is achieved when the assimilation of each model component is active. It is worth noting that spectral nudging leads to slightly worse accuracy for temperature and wind, while RMSE remains unchanged for the geopotential. This is implicit in the scale-selective constraint of the spectral nudging; however, SST skill scores are most benefited by the spectral nudging, suggesting that full-field nudging may, to some extent, interfere negatively with the air–sea flux computation.
Figure 12 details the bias and RMSE profiles in the atmosphere for the six experiments, verified against radiosondes. Spectral nudging provides less biased near-surface air temperature values, although RMSE is the smallest with full-field nudging, indicating that the temporal variability is better captured in the latter case. Qualitatively similar results hold for wind speed, while humidity skill scores are not significantly impacted by the data assimilation settings, partly due to the smaller nudging coefficient than for other parameters, and the dominating effect of microphysics parametrizations.
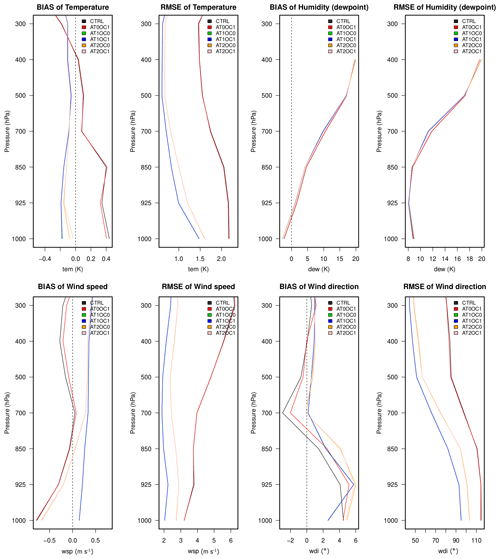
Figure 12Skill score metrics (bias and RMSE) profiles for the data assimilation experiments calculated for selected atmospheric parameters (air temperature and humidity, wind speed and direction) against radiosonde observations extracted from the RUC NOAA/ESRL archive.
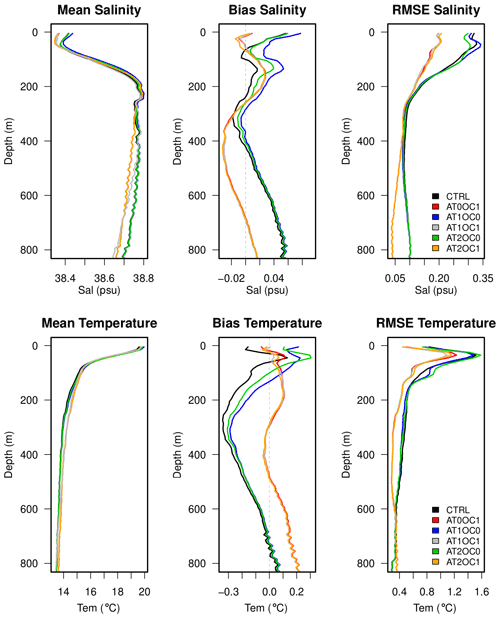
Figure 13As for Fig. 12 but for the oceanic skill score metrics profiles calculated against Argo float data extracted from the UKMO EN4 profile dataset.
Seawater temperature and salinity skill scores are presented in Fig. 13, as profiles of mean state, bias, and RMSE. Salinity is characterized by salty biases in all assimilation-blind experiments in the top 100 m of depth. The assimilation scheme successfully corrects the bias and approximately halves the RMSE in the upper ocean. For temperature, the CTRL experiment is characterized by sea surface cold bias, while experiments with only atmospheric data assimilation are characterized by warm bias. The adoption of variational ocean data assimilation rectifies both types of bias and leads to consistently small RMSE throughout the water column. The benefits of the different assimilation schemes on the SST skill scores are shown in Fig. 14 for two selected pairs of experiments as RMSE differences. The RMSE is calculated against SST analyses from satellite data (Pisano et al., 2020). The top panel shows the impact of spectral versus full-field nudging (positive values indicate the superiority of spectral nudging). In most areas of the Mediterranean Sea, and dominantly in the western part of it, spectral nudging outperforms full-field nudging, likely due to the effective spatial resolution which is not degraded in the full-field nudging. The impact of ocean data assimilation (bottom panel) is large and rather homogenous throughout the model domain, peaking east of the Strait of Gibraltar.
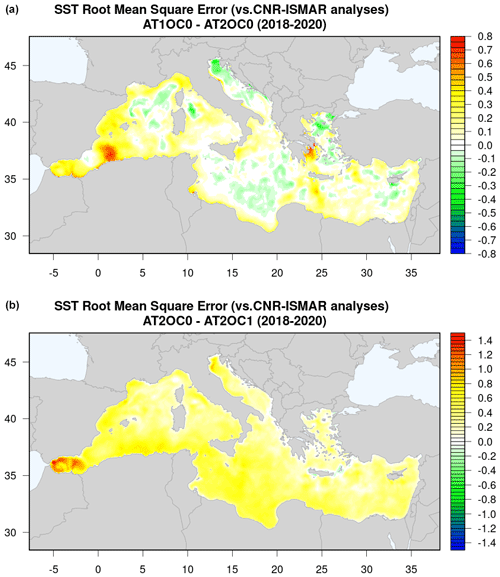
Figure 14SST RMSE differences between AT1OC0 and AT2OC0 (a) and between AT2OC0 and AT2OC1 (b) to show, respectively, the impact of spectral nudging and oceanic data assimilation on the SST RMSE, calculated against the Copernicus Marine Service satellite-based analyses.
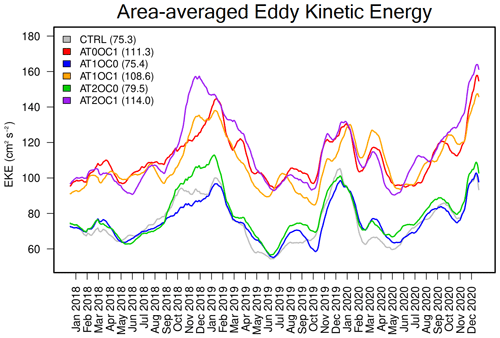
Figure 15Eddy kinetic energy (EKE) over the Mediterranean Sea for the period 2018–2020 and the different data assimilation experiments presented in the text. The EKE is calculated from the sea surface height using geostrophic velocities.
To better understand how spectral nudging is not disruptive to the upper-ocean circulation, Fig. 15 shows the eddy kinetic energy (EKE) from the different experiments, calculated from the sea surface height using the geostrophic velocities (e.g., Wang et al., 2019). The time series show that ocean data assimilation significantly impacts the EKE, although altimetry is not assimilated, as in previous global ocean studies (Storto et al., 2016). Such an increase is in the range of 44 %–48 % depending on the atmospheric data assimilation configuration. However, while full-field nudging leads to a decrease in EKE of about 2.5 % (AT1OC1 versus AT0OC1), spectral nudging provides an additional 3 % increase (AT2OC1 versus AT0C1), indicating its slight benefits in reproducing the mesoscale ocean circulation. This is also confirmed by the validation against surface current speed from drifters (not shown), which highlights a slight improvement (of the order of 1 %–1.5 %) when spectral nudging and ocean data assimilation are adopted, compared to the CTRL or full-field nudging experiments.
5.3 Impact on the representation of Mediterranean hurricanes
We conclude our assessment with the skill scores relative to the representation of Mediterranean hurricane (medicane) events. In particular, during the 2018–2020 period, two events of strong intensity occurred in the eastern part of the Mediterranean. These two events are Medicane Zorbas (27 September–2 October 2018) and Medicane Ianos (14–21 September 2020). We looked at the reanalyzed and forecasted events, also in comparison with the ECMWF ERA5 reanalysis, for the different assimilation configurations presented earlier. Tracks are calculated from the grid points corresponding to the minimum surface pressure.
The top panels of Fig. 16 show the two medicanes' tracks – calculated as the location of the minimum sea level pressure – from the observed best track and the experiments with atmospheric data assimilation, in reanalysis mode (i.e., continuous data assimilation). CTRL and AT0OC1 are not shown as their errors in reproducing the medicane tracks are very large; namely, with no atmospheric data assimilation, the representation of the medicane tracks is very poor, no matter whether the ocean data assimilation is switched on or off. Table 4 summarizes medicane verification skill scores. All experiments can capture the tracks of the medicanes, with positioning errors of the order of 36–38 and 23–31 km for the two events, respectively. The smallest distance errors are for AT2OC0 and AT1OC1, respectively, although the differences are small. However, spectral nudging provides the best skill scores for the minimum pressure and the maximum wind speed, visible in Table 4 and the bottom panels of Fig. 16. Additionally, ocean data assimilation further improves the representation of the baric minima for both events, leading to another 10 % improvement in terms of pressure minima RMSE. To a lesser extent, the improvement occurs also for wind speed maxima (about 3 % improvement). These results indicate that while the adoption of atmospheric spectral nudging is crucial in capturing the medicane evolution, namely its track, ocean data assimilation can provide a significant additional improvement in capturing the intensity of the events. This proves the added value of the coupled modeling and the potential of coupled data assimilation to increase medicane predictability.
Table 4RMSE values calculated for the different data assimilation experiments presented in the text in reanalysis mode (i.e., with continuous data assimilation) and the ECMWF ERA5 reanalysis for the two medicanes, Medicane Zorbas (September–October 2018) and Medicane Ianos (September 2020). Parameters assessed are the position (distance with the best-observed track, in km), along-track sea level pressure (hPa), and near-track maximum wind speed (m s−1).
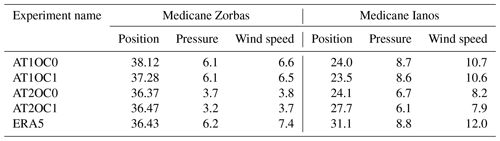
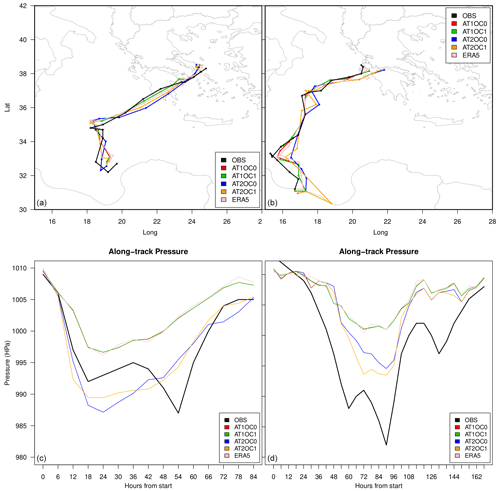
Figure 16Medicane tracks (a, b) and along-track sea level pressure (c, d) during the two medicane events presented in the text (Zorbas, a, c; and Ianos, b, d). The experiments are run in reanalysis mode (continuous data assimilation), and the ECMWF ERA5 reanalysis is shown for comparison.
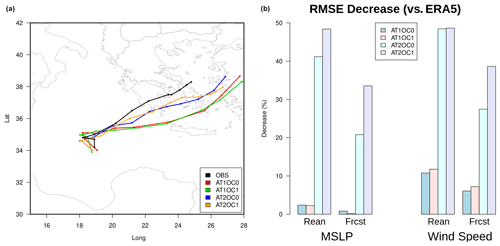
Figure 17(a) As for Fig. 16a but for the forecasts initialized on 28 September with the different data assimilation configurations and run in forecast mode. (b) RMSE percent decrease (positive percentage means improvement) compared to the corresponding ECMWF ERA5 reanalysis and forecast for mean sea level pressure (MSLP) and wind speed.
Similar diagnostics have been assessed and calculated in forecasting mode for Medicane Zorbas. In particular, several forecasts were initialized on 28 September from the initial conditions provided by their respective assimilation experiments, and the unconstrained coupled model without any data constraint was then run in forecasting mode for the following 5 d. Results are summarized in Fig. 17 in terms of forecasted track and RMSE decreases compared to the corresponding ERA5 forecasts. The spectral nudging can better capture the medicane landing, while full-field nudging significantly deviates the track southwards. The use of ocean data assimilation provides a small impact on the forecasted track; however, in terms of mean sea level pressure and wind speed forecasts, there occurs significant improvement when the initialization includes oceanic observations: about a 10 % RMSE decrease (compared to ERA5) for both parameters. This confirms the non-negligible potential of oceanic data assimilation on hurricane predictability (Zhang and Emanuel, 2018).
In this work, we have introduced the configuration of a new high-resolution regional climate model for the Mediterranean region (MESMAR) and presented several assessment results. While there exist already several regional coupled and climate models over this region (e.g., Lionello et al., 2003; Lebeaupin Brossier and Drobinski, 2009; Artale et al., 2010; Akhtar et al., 2018; Nabat et al., 2020; Reale et al., 2020; Anav et al., 2021), our goal is to set up an affordable numerical framework, to be possibly upgraded in the future, to study the predictability of specific events through downscaling exercises and state-of-the-art coupled data assimilation algorithms. The main objective of the present work is to present the configuration and the basic performances of the system and evaluate weakly coupled data assimilation experiments. Our system embeds the latest versions of numerical models and notably includes a data assimilation system capable of ingesting observational information from the atmosphere and the ocean.
The model is composed of WRF, NEMO, and HD as atmospheric, oceanic, and hydrology components, respectively, implemented at 15 km and ∘ of horizontal resolution. Several sensitivity experiments have been performed to identify the optimal coupled model configuration. Our non-exhaustive selection focused on the benefits of a re-tuned oceanic vertical mixing scheme, the positive impact of interactive river discharge on the upper-ocean salinity skill scores, and the physics–microphysics parametrizations' suite of WRF on near-surface biases. We have shown that with the optimal configuration, the spatial and temporal variability of the ocean heat uptake is well captured for the period 1993–2021, although some offset in the air–sea net heat fluxes exists, providing an ocean warming weaker than observed in the regional climate model.
Next, we have implemented and assessed a weakly coupled data assimilation system, where atmospheric data assimilation is formulated in terms of scale-selective (spectral) nudging to relax WRF towards the ECMWF ERA5 reanalyses at the scales of about 850 km and larger. The oceanic data assimilation component includes a variational scheme capable of assimilating all observations available in the Mediterranean Sea, with a temporal frequency and assimilation window of 3 d. In a series of 3-year experiments combining different setups of the atmospheric and oceanic data assimilation, we have demonstrated the benefits of the spectral nudging on sea surface skill scores, oceanic eddy kinetic energy, and medicane event representation, while the ocean data assimilation is found to be crucial not only in the oceanic skill score metrics but also for medicane intensity predictions and, to some extent, in the low-troposphere skill scores. The final configuration including spectral nudging and ocean variational data assimilation will serve as the basis for regionally downscaling global atmospheric and oceanic reanalyses from ECMWF and as the basis for downscaling monthly to seasonal predictions.
Future extensions of MESMAR will go mostly in three directions. First, the horizontal resolution of the models can be enhanced: while our long-term applications make it difficult to reach a convection-resolving spatial resolution, the atmospheric model resolution could be increased to reduce the spatial resolution factor compared to NEMO. To this end, we plan in the future to have a high-resolution version of the system, at about 5 km, for use in short experiments. Second, the regional climate model can be extended to include other model components and to turn into an Earth system model (ESM); for instance, wave modeling components and biogeochemical modeling can be embedded in the system to provide an ESM correspondence of MESMAR. Finally, the system is being upgraded to include a strongly coupled data assimilation system, where the data assimilation state vector and the observation operators seamlessly include both atmospheric and oceanic parameters (as in Storto et al., 2018). This will pave the way for a systematic assessment of the impact of coupled observation operators and initial conditions in both short- and long-range prediction systems and will require preliminary studies on the optimal characterization of the coupled background-error covariances.
The NEMO ocean model code (v4.0.7) is available at https://forge.ipsl.jussieu.fr/nemo/wiki (NEMO, 2023), the WRF atmospheric model code (v4.3.3) is available at https://github.com/wrf-model/WRF (wrf-model, 2023), and the HD hydrological discharge model (v5.1) is available at https://doi.org/10.5281/zenodo.5707587 (Hagemann and Ho-Hagemann, 2021).
The frozen version of the MESMAR v1 code used in this paper is available at https://doi.org/10.5281/zenodo.7898938 (Storto et al., 2023).
Data and scripts used within the paper are available at https://doi.org/10.5281/zenodo.7899115 (Storto, 2023).
AS, YH, VdT, CY, and AA have contributed to the model developments and model validation; GS and RS have provided guidance on the model developments. AS drafted the initial version of the manuscript; all coauthors have revised the paper.
The contact author has declared that none of the authors has any competing interests.
Publisher’s note: Copernicus Publications remains neutral with regard to jurisdictional claims in published maps and institutional affiliations.
We acknowledge the use of ECMWF's computing and archive facilities in this research. We specifically acknowledge Sebastien Masson (LOCEAN/IPSL) and Sophie Valcke (CERFACS) for their valuable support in the use of the NEMO and WRF interfaces to the OASIS coupler, Stefan Hagemann and Ha Thi Minh Hagemann (Hereon) for their kind support in using the HD model, and Hao Zuo and Magdalena Balmaseda (ECMWF) for providing near-real-time ECMWF ocean data for the ocean boundary conditions of MESMAR. We thank three anonymous reviewers and the editor for their valuable suggestions that improved the quality of this paper.
This research has been supported by the Regione Lazio (POR Lazio grant A0375-2020-36508, Gruppi di ricerca 2020) and the Ministero dell'Istruzione, dell'Università e della Ricerca (ICSC – National Research Center for High Performance Computing, Big Data and Quantum Computing).
This paper was edited by Riccardo Farneti and reviewed by three anonymous referees.
Akhtar, N., Brauch, J., Dobler, A., Béranger, K., and Ahrens, B.: Medicanes in an ocean–atmosphere coupled regional climate model, Nat. Hazards Earth Syst. Sci., 14, 2189–2201, https://doi.org/10.5194/nhess-14-2189-2014, 2014.
Akhtar, N., Brauch, J., and Ahrens, B.: Climate modeling over the Mediterranean Sea: impact of resolution and ocean coupling, Clim. Dynam., 51, 933–948, 2018.
Anav, A., Carillo, A., Palma, M., Struglia, M. V., Turuncoglu, U. U., and Sannino, G.: The ENEA-REG system (v1.0), a multi-component regional Earth system model: sensitivity to different atmospheric components over the Med-CORDEX (Coordinated Regional Climate Downscaling Experiment) region, Geosci. Model Dev., 14, 4159–4185, https://doi.org/10.5194/gmd-14-4159-2021, 2021.
Artale, V., Calmanti, S., Carillo, A., Dell'Aquila, A., Herrmann, M., Pisacane, G., Ruti, P. M., Sannino, G., Struglia, M. V., Giorgi, F., Bi, X., Pal, J. S., Rauscher, S., and The PROTHEUS Group: An atmosphere–ocean regional climate model for the Mediterranean area: assessment of a present climate simulation, Clim. Dynam., 35, 721–740, https://doi.org/10.1007/s00382-009-0691-8, 2010.
Astraldi, M., Balopoulos, S., Candela, J., Font, J., Gacic, M., Gasparini, G. P., Manca, B., Theocharis, A., and Tintore, J.: The role of straits and channels in understanding the characteristics of Mediterranean circulation, Prog. Oceanogr., 44, 65–108, 1999.
Bourdalle-Badie, R. and Treguier, A. M.: A climatology of run-off for the global ocean-ice model ORCA025, report MOO-RP-425-365-MER, Mercator-Ocean: Toulouse, France, 8 pp., https://www.drakkar-ocean.eu/publications/reports/runoff-mercator-06.pdf (last access: 14 August 2023), 2006.
Brewin, R. J. W., Sathyendranath, S., Müller, D., Brockmann, C., Deschamps, P.-Y., Devred, E., Doerffer, R., Fomferra, N., Franz, B., Grant, M., Groom, S., Horseman, A., Hu, C., Krasemann, H, Lee, Z. P., Maritorena, S., Mélin, F., Peters, M., Platt, T., Regner, P., Smyth, T., Steinmetz, F., Swinton, J., Werdell, J., and White, G. N.: The Ocean Colour Climate Change Initiative: III. A round-robin comparison on in-water bio-optical algorithms, Remote Sens. Environ., 162, 271–294, https://doi.org/10.1016/j.rse.2013.09.016, 2015.
Canuto, V. M., Howard, A., Cheng, Y., and Dubovikov, M. S.: Ocean turbulence. part I: One-point closure model-momentum and heat vertical diffusivities, J. Phys. Oceanogr., 31, 1413–1426, 2001.
Cassola, F., Ferrari, F., Mazzino, A., and Miglietta, M. M.: The role of the sea on the flash floods events over Liguria, Geoph. Res. Lett., 43, 3534–3542, 2016.
Cavicchia, L. and von Storch, H.: The simulation of medicanes in a high-resolution regional climate model, Clim. Dynam., 39, 2273–2290, https://doi.org/10.1007/s00382-011-1220-0, 2012.
Cavicchia, L., von Storch, H., and Gualdi, S.: Mediterranean Tropical-Like Cyclones in Present and Future Climate, J. Climate, 27, 7493–7501, https://doi.org/10.1175/JCLI-D-14-00339.1, 2014.
Choi, S.-J. and Lee, D.-K.: Impact of spectral nudging on the downscaling of tropical cyclones in regional climate simulations, Adv. Atmos. Sci., 33, 730–742, https://doi.org/10.1007/s00376-016-5061-y, 2016.
Cornes, R., van der Schrier, G., van den Besselaar, E. J. M., and Jones, P. D.: An Ensemble Version of the E-OBS Temperature and Precipitation Datasets, J. Geophys. Res.-Atmos., 123, 9391–9409, https://doi.org/10.1029/2017JD028200, 2018.
Cos, J., Doblas-Reyes, F., Jury, M., Marcos, R., Bretonnière, P.-A., and Samsó, M.: The Mediterranean climate change hotspot in the CMIP5 and CMIP6 projections, Earth Syst. Dynam., 13, 321–340, https://doi.org/10.5194/esd-13-321-2022, 2022.
Craig, A., Valcke, S., and Coquart, L.: Development and performance of a new version of the OASIS coupler, OASIS3-MCT_3.0, Geosci. Model Dev., 10, 3297–3308, https://doi.org/10.5194/gmd-10-3297-2017, 2017.
Dai, A. and Trenberth, K. E.: Estimates of freshwater discharge from continents: Latitudinal and seasonal variations, J. Hydrometeorol., 3, 660–687, 2002.
Davies, H. C. and Turner, R. E.: Updating prediction models by dynamical relaxation: An examination of the technique, Q. J. Roy. Meteor. Soc., 103, 225–245, 1977.
Davin, E. L., Maisonnave, E., and Seneviratne, S. I.: Is land surface processes representation a possible weak link in current Regional Climate Models?, Environ. Res. Lett., 11, 1–8, https://doi.org/10.1088/1748-9326/11/7/074027, 2016.
Duffourg, F. and Ducrocq, V.: Origin of the moisture feeding the Heavy Precipitating Systems over Southeastern France, Nat. Hazards Earth Syst. Sci., 11, 1163–1178, https://doi.org/10.5194/nhess-11-1163-2011, 2011.
Escudier, R., Clementi, E., Cipollone, A., Pistoia, J., Drudi, M., Grandi, A., Lyubartsev, V., Lecci, R., Aydogdu, A., Delrosso, D., Omar, M., Masina, S., Coppini, G., and Pinardi, N.: A High Resolution Reanalysis for the Mediterranean Sea, Front. Earth Sci., 9, 702285, https://doi.org/10.3389/feart.2021.702285, 2021.
Feser, F., Rockel, B., von Storch, H., Winterfeldt, J., and Zahn, M.: Regional Climate Models Add Value to Global Model Data: A Review and Selected Examples, B. Am. Meteorol. Soc., 92, 1181–1192, https://doi.org/10.1175/2011BAMS3061.1, 2011.
Fita, L., Polcher, J., Giannaros, T. M., Lorenz, T., Milovac, J., Sofiadis, G., Katragkou, E., and Bastin, S.: CORDEX-WRF v1.3: development of a module for the Weather Research and Forecasting (WRF) model to support the CORDEX community, Geosci. Model Dev., 12, 1029–1066, https://doi.org/10.5194/gmd-12-1029-2019, 2019.
Flaounas, E., Davolio, S., Raveh-Rubin, S., Pantillon, F., Miglietta, M. M., Gaertner, M. A., Hatzaki, M., Homar, V., Khodayar, S., Korres, G., Kotroni, V., Kushta, J., Reale, M., and Ricard, D.: Mediterranean cyclones: current knowledge and open questions on dynamics, prediction, climatology and impacts, Weather Clim. Dynam., 3, 173–208, https://doi.org/10.5194/wcd-3-173-2022, 2022.
Flather, R. A.: A Storm Surge Prediction Model for the Northern Bay of Bengal with Application to the Cyclone Disaster in April 1991, J. Phys. Oceanogr., 24, 172–190, https://doi.org/10.1175/1520-0485(1994)024<0172:ASSPMF>2.0.CO;2, 1994.
Foley, A. M.: Uncertainty in regional climate modelling: A review, Prog. Phys. Geogr.-Earth and Environment, 34, 647–670, https://doi.org/10.1177/0309133310375654, 2010.
Giorgi, F.: Regional Dynamical Downscaling. Oxford Research Encyclopedia of Climate Science, https://oxfordre.com/climatescience/view/10.1093/acrefore/9780190228620.001.0001/acrefore-9780190228620-e-784 (last access: 22 June 2023), 2020.
Giorgi, F.: Thirty years of regional climate modeling: Where are we and where are we going next?, J. Geophys. Res.-Atmos., 124, 5696–5723, https://doi.org/10.1029/2018JD030094, 2019.
Good, S. A., Martin, M. J., and Rayner, N. A.: EN4: quality controlled ocean temperature and salinity profiles and monthly objective analyses with uncertainty estimates, J. Geophys. Res.-Oceans, 118, 6704–6716, https://doi.org/10.1002/2013JC009067, 2013.
Grell, G. A. and Freitas, S. R.: A scale and aerosol aware stochastic convective parameterization for weather and air quality modeling, Atmos. Chem. Phys., 14, 5233–5250, https://doi.org/10.5194/acp-14-5233-2014, 2014.
Hagemann, S. and Ho-Hagemann, H. T. M.: The Hydrological Discharge Model – a river runoff component for offline and coupled model applications (5.1.0), Zenodo [code], https://doi.org/10.5281/zenodo.5707587, 2021.
Hagemann, S. and Dümenil Gates, L.: Validation of the hydrological cycle of ECMWF and NCEP reanalyses using the MPI hydrological discharge model, J. Geophys. Res., 106, 1503–1510, 2001.
Hagemann, S., Stacke, T., and Ho-Hagemann, H.: High resolution discharge simulations over Europe and the Baltic Sea catchment, Front. Earth Sci., 8, 12, https://doi.org/10.3389/feart.2020.00012, 2020.
Harzallah, A., Jordà, G., Dubois, C., Sannino, G., Carillo, A., Li, L., Arsouze, T., Cavicchia, L., Beuvier, J., and Akhtar, N.: Long term evolution of heat budget in the Mediterranean Sea from Med-CORDEX forced and coupled simulations, Clim. Dynam., 51, 1145–1165, https://doi.org/10.1007/s00382-016-3363-5, 2018.
Hersbach, H., Bell, B., Berrisford, P., Hirahara, S., Horányi, A., Muñoz-Sabater, J., Nicolas, J., Peubey, C., Radu, R., Schepers, D., Simmons, A., Soci, C., Abdalla, S., Abellan, X., Balsamo, G., Bechtold, P., Biavati, G., Bidlot, J., Bonavita, M., De Chiara, G., Dahlgren, P., Dee, D., Diamantakis, M., Dragani, R., Flemming, J., Forbes, R., Fuentes, M., Geer, A., Haimberger, L., Healy, S., Hogan, R., Hólm, E., Janisková, M., Keeley, S., Laloyaux, P., Lopez, P., Lupu, C., Radnoti, G., de Rosnay, P., Rozum, I., Vamborg, F., Villaume, S., and Thépaut, J.-N.: The ERA5 global reanalysis, Q. J. R. Meteor. Soc., 146, 1999–2049, https://doi.org/10.1002/qj.3803, 2020.
Hirons, L. C., Klingaman, N. P., and Woolnough, S. J.: The impact of air sea interactions on the representation of tropical precipitation extremes. J. Adv. Model. Earth Sy., 10, 550559, https://doi.org/10.1002/2017MS001252, 2018.
Ho-Hagemann, H. T. M., Hagemann, S., Grayek, S., Petrik, R., Rockel, B., Staneva, J., Feser, F., and Schrum, C.: Internal variability in the regional coupled system model GCOAST-AHOI, Atmos., 11, 227, https://doi.org/10.3390/atmos11030227, 2020.
Iacono, M. J., Delamere, J. S., Mlawer, E. J., Shephard, M. W., Clough, S. A., and Collins, W. D.: Radiative forcing by long-lived greenhouse gases: Calculations with the AER radiative transfer models, J. Geophys. Res., 113, D13103, https://doi.org/10.1029/2008JD009944, 2008.
Iona, A., Theodorou, A., Sofianos, S., Watelet, S., Troupin, C., and Beckers, J.-M.: Mediterranean Sea climatic indices: monitoring long-term variability and climate changes, Earth Syst. Sci. Data, 10, 1829–1842, https://doi.org/10.5194/essd-10-1829-2018, 2018.
Janjić, Z. I.: The Step-Mountain Eta Coordinate Model: Further Developments of the Convection, Viscous Sublayer, and Turbulence Closure Schemes, Mon. Weather Rev., 122, 927–945, https://doi.org/10.1175/1520-0493(1994)122<0927:TSMECM>2.0.CO;2, 1994.
Jordà, G., Von Schuckmann, K., Josey, S. A., Caniaux, G., García-Lafuente, J., Sammartino, S., Özsoy, E., Polcher, J., Notarstefano, G., Poulain, P.-M., Adloff, F., Salat, J., Naranjo, C., Schroeder, K., Chiggiato, J., Sannino, G., and Macías, D.: The Mediterranean Sea Heat and Mass Budgets: Estimates, Uncertainties and Perspectives, Prog. Oceanogr., 156, 174–208, https://doi.org/10.1016/j.pocean.2017.07.001, 2017.
Kourafalou, V. H. and Barbopoulos, K.: High resolution simulations on the North Aegean Sea seasonal circulation, Ann. Geophys., 21, 251–265, https://doi.org/10.5194/angeo-21-251-2003, 2003.
Lebeaupin Brossier, C. and Drobinski, P.: Numerical high-resolution air-sea coupling over the Gulf of Lions during two Tramontane/Mistral events, J. Geophys. Res., 114, D10110, https://doi.org/10.1029/2008JD011601, 2009.
Lebeaupin Brossier, C., Drobinski, P., Béranger, K., Bastin, S., and Orain, F.: Ocean memory effect on the dynamics of coastal heavy precipitation preceded by a mistral event in the northwestern Mediterranean, Q. J. Roy. Meteor. Soc., 139, 1583–1597, https://doi.org/10.1002/qj.2049, 2013.
Lebeaupin Brossier, C., Bastin, S., Béranger, K., and Dobrinski, P.: Regional mesoscale air sea coupling impacts and extreme meteorological events role on the Mediterranean Sea water budget, Clim. Dynam., 44, 1029, https://doi.org/10.1007/s00382-014-2252-z, 2015.
Lellouche, J.-M., Greiner, E., Bourdallé-Badie, R., Garric, G., Melet, A., Drévillon, M., Bricaud, C., Hamon, M., Le Galloudec, O., Regnier, C., Candela, T., Testut, C.-E., Gasparin, F., Ruggiero, G., Benkiran, M., Drillet, Y., and Le Traon, P.-Y.: The Copernicus Global 1/12∘ Oceanic and Sea Ice GLORYS12 Reanalysis, Front. Earth Sci., 9, 698876, https://doi.org/10.3389/feart.2021.698876, 2021.
Li, M., Zhang, S., Wu, L., Lin, X., Chang, P., Danabasoglu, G., Wei, Z., Yu, X., Hu, H., Ma, X., Ma, W., Jia, D., Liu, X., Zhao, H., Mao, K., Ma, Y., Jiang, Y., Wang, X., Liu, G., and Chen, Y.: A high-resolution Asia-Pacific regional coupled prediction system with dynamically downscaling coupled data assimilation, Sci. Bull., 65, 1849–1858, https://doi.org/10.1016/j.scib.2020.07.022, 2020.
Li, Y. and Toumi, R.: Improved tropical cyclone intensity forecasts by assimilating coastal surface currents in an idealized study, Geophys. Res. Lett., 45, 10019–10026, https://doi.org/10.1029/2018GL079677, 2018.
Lionello, P. and Scarascia, L.: The relation between climate change in the Mediterranean region and global warming, Reg. Environ. Change, 18, 1481–1493, https://doi.org/10.1007/s10113-018-1290-1, 2018.
Lionello, P., Martucci, G., and Zampieri, M.: Implementation of a Coupled Atmosphere-Wave-Ocean Model in the Mediterranean Sea: Sensitivity of the Short Time Scale Evolution to the Air-Sea Coupling Mechanisms, J. Atmos. Ocean Sci., 9, 65–95, https://doi.org/10.1080/1023673031000151421, 2003.
MacDonald, A. M., Candela, J., and Bryden, H. L.: An estimate of the net heat transport through the Strait of Gibraltar, in: Seasonal and interannual variability of the Western Mediterranean Sea, edited by: LaViolette, P. E., Coastal Estuarine Stud. AGU, Washington DC, 13–32, https://doi.org/10.1029/CE046p0013, 1994.
Madec, G. and The NEMO System Team: NEMO Ocean Engine. Note Du Pole De Modélisation. Paris, France: Institut Pierre-Simon Laplace, Zenodo, https://doi.org/10.5281/zenodo.3248739, 2017.
Mellor, G. L. and Yamada, T.: Development of a turbulence closure model for geophysical fluid problems, Rev. Geophys., 20, 851–875, 1982.
Mooney, P. A., Mulligan, F. J., and Fealy, R.: Evaluation of the Sensitivity of the Weather Research and Forecasting Model to Parameterization Schemes for Regional Climates of Europe over the Period 1990–95, J. Climate, 26, 1002–1017, https://doi.org/10.1175/JCLI-D-11-00676.1, 2013.
Morel, A. and Maritorena, S.: Bio-optical properties of oceanic waters: A reappraisal, J. Geophys. Res.-Oceans, 106, 7163–7180, https://doi.org/10.1029/2000JC000319, 2001.
Nabat, P., Somot, S., Cassou, C., Mallet, M., Michou, M., Bouniol, D., Decharme, B., Drugé, T., Roehrig, R., and Saint-Martin, D.: Modulation of radiative aerosols effects by atmospheric circulation over the Euro-Mediterranean region, Atmos. Chem. Phys., 20, 8315–8349, https://doi.org/10.5194/acp-20-8315-2020, 2020.
Nakanishi, M. and Niino, H.: An Improved Mellor–Yamada Level-3 Model: Its Numerical Stability and Application to a Regional Prediction of Advection Fog, Bound.-Lay. Meteorol., 119, 397–407, https://doi.org/10.1007/s10546-005-9030-8, 2006.
NEMO: https://forge.ipsl.jussieu.fr/nemo/wiki, last access: 14 August 2023.
Niu, G.-Y., Yang, Z.-L., Mitchell, K. E., Chen, F., Ek, M. B., Barlage, M., Kumar, A., Manning, K., Niyogi, D., Rosero, E., Tewari, M., and Xia, Y.: The community Noah land surface model with multiparameterization options (Noah-MP): 1. Model description and evaluation with local-scale measurements, J. Geophys. Res., 116, D12109, https://doi.org/10.1029/2010JD015139, 2011.
Omrani, H., Drobinski, P., and Dubos, T.: Using nudging to improve global-regional dynamic consistency in limited-area climate modeling: What should we nudge?, Clim. Dynam., 44, 1627–1644, 2015.
Penny, S. G., Akella, S., Balmaseda, M. A., Browne, P., Carton, J. A., Chevallier, M., Counillon, F., Domingues, C., Frolov, S., Heimbach, P., Hogan, P., Hoteit, I., Iovino, D., Laloyaux, P., Martin, M. J., Masina, S., Moore, A. M., de Rosnay, P., Schepers, D., Sloyan, B. M., Storto, A., Subramanian, A., Nam, S., Vitart, F., Yang, C., Fujii, Y., Zuo, H., O'Kane, T., Sandery, P., Moore, T., and Chapman, C. C.: Observational Needs for Improving Ocean and Coupled Reanalysis, S2S Prediction, and Decadal Prediction, Front. Mar. Sci., 6, 391, https://doi.org/10.3389/fmars.2019.00391, 2019.
Pisano, A., Marullo, S., Artale, V., Falcini, F., Yang, C., Leonelli, F. E., Santoleri, R., and Buongiorno Nardelli, B.: New evidence of mediterranean climate change and variability from sea surface temperature observations, Remote Sens., 12, 132, https://doi.org/10.3390/rs12010132, 2020.
Reale, M., Giorgi, F., Solidoro, C., Di Biagio, V., Di Sante, F., Mariotti, L., Farneti, R., and Sannino, G.: The regional Earth system Model RegCM-ES: Evaluation of the Mediterranean climate and marine biogeochemistry, J. Adv. Model. Earth Sy., 12, e2019MS001812, https://doi.org/10.1029/2019MS001812, 2020.
Reale, M., Cabos Narvaez, W. D., Cavicchia, L., Conte, D., Coppola, E., Flaounas, E., Giorgi, F., Gualdi, S., Hochman, A., Li, L., Lionello, P., Podrascanin, Z., Salon, S., Sanchez-Gomez, E., Scoccimarro, E., Sein, D. V., an Somot, S.: Future projections of Mediterranean cyclone characteristics using the Med-CORDEX ensemble of coupled regional climate system models, Clim. Dynam., 58, 2501–2524, https://doi.org/10.1007/s00382-021-06018-x, 2022a.
Reale, M., Cossarini, G., Lazzari, P., Lovato, T., Bolzon, G., Masina, S., Solidoro, C., and Salon, S.: Acidification, deoxygenation, and nutrient and biomass declines in a warming Mediterranean Sea, Biogeosciences, 19, 4035–4065, https://doi.org/10.5194/bg-19-4035-2022, 2022b.
Ricchi, A., Miglietta, M. M., Barbariol, F., Benetazzo, A., Bergamasco, A., Bonaldo, D., Cassardo, C., Falcieri, F. M., Modugno, G., Russo, A., Sclavo, M., and Carniel, S.: Sensitivity of a Mediterranean Tropical-Like Cyclone to Different Model Configurations and Coupling Strategies, Atmosphere, 8, 92, https://doi.org/10.3390/atmos8050092, 2017.
Rockel, B.: The Regional Downscaling Approach: a Brief History and Recent Advances, Curr. Clim. Change Rep., 1, 22–29, https://doi.org/10.1007/s40641-014-0001-3, 2015.
Rockel, B., Castro, C. L., Pielke Sr., R. A., von Storch, H., and Lencini, G.: Dynamical downscaling: Assessment of model system dependent retained and added variability for two different regional climate models, J. Geophys. Res., 113, D21107, https://doi.org/10.1029/2007JD009461, 2008.
Rummukainen, M.: Added value in regional climate modeling, Wires Clim. Change, 7, 145–159, https://doi.org/10.1002/wcc.378, 2016.
Rummukainen, M., Rockel, B., Bärring, L., Christensen, J. H., and Reckermann, M.: Twenty-First-Century Challenges in Regional Climate Modeling, B. Am. Meteorol. Soc., 96, ES135–ES138, https://doi.org/10.1175/BAMS-D-14-00214.1, 2015.
Ruti, P. M., Somot, S., Giorgi, F., Dubois, C., Flaounas, E., Obermann, A., Dell’Aquila, A., Pisacane, G., Harzallah, A., Lombardi, E., Ahrens, B., Akhtar, N., Alias, A., Arsouze, T., Aznar, R., Bastin, S., Bartholy, J., Béranger, K., Beuvier, J., Bouffies-Cloché, S., Brauch, J., Cabos, W., Calmanti, S., Calvet, J.-C., Carillo, A., Conte, D., Coppola, E., Djurdjevic, V., Drobinski, P., Elizalde-Arellano, A., Gaertner, M., Galàn, P., Gallardo, C., Gualdi, S., Goncalves, M., Jorba, O., Jordà, G., L’Heveder, B., Lebeaupin-Brossier, C., Li, L., Liguori, G., Lionello, P., Maciàs, D., Nabat, P., Önol, B., Raikovic, B., Ramage, K., Sevault, F., Sannino, G., Struglia, M. V., Sanna, A., Torma, C., and Vervatis, V.: Med-CORDEX Initiative for Mediterranean Climate Studies, B. Am. Meteorol. Soc., 97, 1187–1208, https://doi.org/10.1175/BAMS-D-14-00176.1, 2016.
Scoccimarro, E., Bellucci, A., Storto, A., Gualdi, S., Masina, S., and Navarra, A.: Remote sub-surface ocean temperature as a predictor of Atlantic hurricane activity, P. Natl. Acad. Sci. USA, 115, 11460–11464, https://doi.org/10.1073/pnas.1810755115, 2018.
Skamarock, W. C., Klemp, J., Dudhia, J., Gill, D. O., Liu, Z., Berner, J., Wang, W., Powers, J. G., Duda, M., Barker, D., and Huang, X.-Y.: A Description of the Advanced Research WRF Model Version 4.3 (No. NCAR/TN-556+STR), https://doi.org/10.5065/1dfh-6p97, 2021.
Shchepetkin, A. F. and McWilliams, J. C.: The regional oceanic modeling system (ROMS): a splitexplicit, free-surface, topography-following-coordinate oceanic model, Ocean Modell., 9, 347–404, 2005.
Soto-Navarro, J., Jordá, G., Amores, A., Cabos, W., Somot, S., Sevault, F., Macías, D., Djurdjevic, V., Sannino, G., Li, L., and Sein, D.: Evolution of Mediterranean Sea water properties under climate change scenarios in the Med-CORDEX ensemble, Clim. Dynam., 54, 2135–2165, https://doi.org/10.1007/s00382-019-05105-4, 2020.
Storkey, D., Blaker, A. T., Mathiot, P., Megann, A., Aksenov, Y., Blockley, E. W., Calvert, D., Graham, T., Hewitt, H. T., Hyder, P., Kuhlbrodt, T., Rae, J. G. L., and Sinha, B.: UK Global Ocean GO6 and GO7: a traceable hierarchy of model resolutions, Geosci. Model Dev., 11, 3187–3213, https://doi.org/10.5194/gmd-11-3187-2018, 2018.
Storto, A.: Variational quality control of hydrographic profile data with non-Gaussian errors for global ocean variational data assimilation systems, Ocean Model., 104, 226–241, https://doi.org/10.1016/j.ocemod.2016.06.011, 2016.
Storto, A.: MESMAR v1: A new regional coupled climate model for downscaling, predictability, and data assimilation studies in the Mediterranean region, Article data (1.0), Zenodo [data set], https://doi.org/10.5281/zenodo.7899115, 2023.
Storto, A. and Randriamampianina, R.: Ensemble variational assimilation for the representation of background error covariances in a high-latitude regional model, J. Geophys. Res., 115, D17204, https://doi.org/10.1029/2009JD013111, 2010.
Storto, A., Masina, S., and Dobricic, S.: Estimation and Impact of Nonuniform Horizontal Correlation Length Scales for Global Ocean Physical Analyses, J. Atmos. Ocean. Tech., 31, 2330–2349, https://doi.org/10.1175/JTECH-D-14-00042.1, 2014.
Storto, A., Masina, S., and Navarra, A.: Evaluation of the CMCC eddy-permitting global ocean physical reanalysis system (C-GLORS, 1982–2012) and its assimilation components, Q. J. Roy. Meteor. Soc., 142, 738–758, https://doi.org/10.1002/qj.2673, 2016.
Storto, A., Oddo, P., Cipollone, A., Mirouze, I., and Lemieux-Dudon, B.: Extending an oceanographic variational scheme to allow for affordable hybrid and four-dimensional data assimilation, Ocean Model., 128, 67–86, https://doi.org/10.1016/j.ocemod.2018.06.005, 2018a.
Storto, A., Martin, M. J., Deremble, B., and Masina, S.: Strongly Coupled Data Assimilation Experiments with Linearized Ocean–Atmosphere Balance Relationships, Mon. Weather Rev., 146, 1233–1257, https://doi.org/10.1175/MWR-D-17-0222.1, 2018b.
Storto, A., Masina, S., Simoncelli, S., Iovino, D., Cipollone, A., Drevillon, M., Drillet, Y., von Schuckman, K., Parent, L., Garric, G., Greiner, E., Desportes, C., Zuo, H., Balmaseda, M. A., and Peterson, K. A.: The added value of the multi-system spread information for ocean heat content and steric sea level investigations in the CMEMS GREP ensemble reanalysis product, Clim. Dynam., 53, 287–312, https://doi.org/10.1007/s00382-018-4585-5, 2019.
Storto, A., Hesham Essa, Y., de Toma, V., Anav, A., Sannino, G., Santoleri, R., and Yang, C.: MESMAR v1: A new regional coupled climate model for downscaling, predictability, and data assimilation studies in the Mediterranean region, Coupled model code (1.0), Zenodo [code], https://doi.org/10.5281/zenodo.7898938, 2023.
Thompson, G., Field, P. R., Rasmussen, R. M., and Hall, W. D.: Explicit Forecasts of Winter Precipitation Using an Improved Bulk Microphysics Scheme. Part II: Implementation of a New Snow Parameterization, Mon. Weather Rev., 136, 5095–5115, https://doi.org/10.1175/2008MWR2387.1, 2008.
Tsujino, H., Urakawa, S., Nakano, H., Small, R. J., Kim, W. M., Yeager, S. G., Danabasoglu, G., Suzuki, T., Bamber, J. L., Bentsen, M., Böning, C. W., Bozec, A., Chassignet, E. P., Curchitser, E., Boeira Dias, F., Durack, P. J., Griffies, S. M., Harada, Y., Ilicak, M., Josey, S. A., Kobayashi, C., Kobayashi, S., Komuro, Y., Large, W. G., Le Sommer, J., Marsland, S. J., Masina, S., Scheinert, M., Tomita, H., Valdivieso, M., and Yamazaki, D.: JRA-55 based surface dataset for driving ocean-sea-ice models (JRA55-do)., Ocean Model., 130, 79–139, https://doi.org/10.1016/j.ocemod.2018.07.002, 2018.
Umlauf, L. and Burchard, H.: A generic length-scale equation for geophysical turbulence models, J. Mar. Res., 61, 235–265, 2003.
Vannucchi, V., Taddei, S., Capecchi, V., Bendoni, M., and Brandini, C.: Dynamical Downscaling of ERA5 Data on the North-Western Mediterranean Sea: From Atmosphere to High-Resolution Coastal Wave Climate, J. Mar. Sci. Eng., 9, 208, https://doi.org/10.3390/jmse9020208, 2021.
Wang, M., Du, Y., Qiu, B., Xie, S., and Feng, M.: Dynamics on Seasonal Variability of EKE Associated with TIWs in the Eastern Equatorial Pacific Ocean, J. Phys. Oceanogr., 49, 1503–1519, https://doi.org/10.1175/JPO-D-18-0163.1, 2019.
wrf-model: WRF, GitHub [code], https://github.com/wrf-model/WRF (last access: 14 August 2023.
Wunsch, C. and Stammer, D.: Atmospheric loading and the oceanic “inverted barometer” effect, Rev. Geophys., 35, 79–107, https://doi.org/10.1029/96RG03037, 1997.
Zeng, X. and Beljaars, A.: A prognostic scheme of sea surface skin temperature for modeling and data assimilation, Geophys. Res. Lett., 32, L14605, https://doi.org/10.1029/2005gl023030, 2005.
Zhang, F. and Emanuel, K.: Promises in air-sea fully coupled data assimilation for future hurricane prediction, Geophys. Res. Lett., 45, 13173–13177, https://doi.org/10.1029/2018GL080970, 2018.
Zuo, H., Balmaseda, M. A., Tietsche, S., Mogensen, K., and Mayer, M.: The ECMWF operational ensemble reanalysis–analysis system for ocean and sea ice: a description of the system and assessment, Ocean Sci., 15, 779–808, https://doi.org/10.5194/os-15-779-2019, 2019.
- Abstract
- Introduction
- Earth system model configuration
- Sensitivity experiments
- Reference simulation
- Data assimilation
- Summary, discussion, and future extensions
- Code availability
- Data availability
- Author contributions
- Competing interests
- Disclaimer
- Acknowledgements
- Financial support
- Review statement
- References
- Abstract
- Introduction
- Earth system model configuration
- Sensitivity experiments
- Reference simulation
- Data assimilation
- Summary, discussion, and future extensions
- Code availability
- Data availability
- Author contributions
- Competing interests
- Disclaimer
- Acknowledgements
- Financial support
- Review statement
- References