the Creative Commons Attribution 4.0 License.
the Creative Commons Attribution 4.0 License.
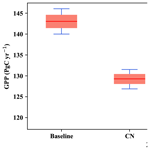
Modelling the terrestrial nitrogen and phosphorus cycle in the UVic ESCM
Makcim L. De Sisto
Andrew H. MacDougall
Nadine Mengis
Sophia Antoniello
Nitrogen (N) and phosphorus (P) biogeochemical dynamics are crucial for the regulation of the terrestrial carbon cycle. In Earth system models (ESMs) the implementation of nutrient limitations has been shown to improve the carbon cycle feedback representation and, hence, the fidelity of the response of land to simulated atmospheric CO2 rise. Here we aimed to implement a terrestrial N and P cycle in an Earth system model of intermediate complexity to improve projections of future CO2 fertilization feedbacks. The N cycle is an improved version of the Wania et al. (2012) N module, with enforcement of N mass conservation and the merger with a deep land-surface and wetland module that allows for the estimation of N2O and NO fluxes. The N cycle module estimates fluxes from three organic (litter, soil organic matter and vegetation) and two inorganic ( and ) pools and accounts for inputs from biological N fixation and N deposition. The P cycle module contains the same organic pools with one inorganic P pool; it estimates influx of P from rock weathering and losses from leaching and occlusion. Two historical simulations are carried out for the different nutrient limitation setups of the model: carbon and nitrogen (CN), as well as carbon, nitrogen and phosphorus (CNP), with a baseline carbon-only simulation. The improved N cycle module now conserves mass, and the added fluxes (NO and N2O), along with the N and P pools, are within the range of other studies and literature. For the years 2001–2015 the nutrient limitation resulted in a reduction of gross primary productivity (GPP) from the carbon-only value of 143 to 130 Pg C yr−1 in the CN version and 127 Pg C yr−1 in the CNP version. This implies that the model efficiently represents a nutrient limitation over the CO2 fertilization effect. CNP simulation resulted in a reduction of 11 % of the mean GPP and a reduction of 23 % of the vegetation biomass compared to the baseline C simulation. These results are in better agreement with observations, particularly in tropical regions where P limitation is known to be important. In summary, the implementation of the N and P cycle has successfully enforced a nutrient limitation in the terrestrial system, which has now reduced the primary productivity and the capacity of land to take up atmospheric carbon, better matching observations.
- Article
(8095 KB) - Full-text XML
- BibTeX
- EndNote
Terrestrial biogeochemical cycles are sensitive to changes in atmospheric CO2 concentrations and climate. Their global evolution will determine the capacity of vegetation and soils to store anthropogenic carbon (Goll et al., 2012). In terrestrial ecosystems carbon cycle feedbacks are constrained in part by the availability of nutrients (Fisher et al., 2012; Zaehle et al., 2014; Wieder et al., 2015; Du et al., 2020). Among nutrients, nitrogen (N) and phosphorus (P) are considered to be the most critical for limiting the primary productivity (Filipelli, 2002; Fowler et al., 2013). Both are fundamental functional needs for plant biochemistry, and their requirement is common in all vegetation taxa (Filipelli, 2002; Vitousek et al., 2010; Du et al., 2020). Regionally, the availability of nutrients can impair the photosynthetic efficiency of terrestrial vegetation and consequently their response to increasing atmospheric CO2. Hence, in Earth system models (ESMs) the representation of nutrient limitations is imperative to improve the accuracy of carbon feedback projections and estimation of carbon budgets.
The simulations from first-generation ESMs with carbon-only schemes have very likely overestimated the response of the terrestrial ecosystem to the increase in atmospheric CO2 concentrations (Hungate et al., 2003; Thorton et al., 2007), showing a high terrestrial carbon uptake response, which would require an unrealistically large nutrient supply. The addition of an N cycle to the land system in ESMs has shown an overall reduction in the effect of CO2 fertilization, especially in high latitudes, with a weaker response in low latitudes which are typically P limited in natural systems (Wang et al., 2007, 2010; Goll et al., 2017; Du et al., 2020; Wang et al., 2020).
The global distribution of N and P is dependent on the biogeochemical characteristics of each nutrient. N inputs are mainly from biological nitrogen fixation (BNF) and atmospheric deposition with little addition from rock weathering (Du et al., 2020). There are two types of N deposition from the atmosphere: wet (precipitation) and dry (particles). Among the two, wet deposition represents most of the atmospheric N input (Fowler et al., 2013; Dynarski et al., 2019). In contrast, the main input of P comes from rock weathering (mainly apatite) with less inputs from atmospheric deposition as dust particles. These characteristics are among the reasons for a global spatial pattern where young soils are usually N limited and old soils P limited (Filipelli, 2002; Fowler et al., 2013; Du et al., 2020). N accumulates rapidly from BNF where N fixers are abundant and slowly where atmospheric deposition is dominant. Thereby, old soils have a larger accumulation of N, especially in regions where N fixers are abundant. On the other hand, P input is limited by the parent material, and the bioavailability is further constrained by the retention of recalcitrant P in soils. Walker and Syers (1976) even suggested that P storage has a fixed total that cannot rapidly be replenished, as parent material is limited.
These notions led to the common conceptualization that high latitudes are N limited, while tropical regions are P limited. While this generalization is correct in most observational studies, the complex pattern of limitation is more intricate, and P limitation could be more common than is commonly inferred. Du et al. (2020) found that globally 43 % of the terrestrial system is relatively limited by P, while only 18 % is limited by N, with the rest being co-limited by both. Biochemically, the availability of N and P can directly limit one another. The addition of P has been shown to be positive for the N fixation, leading to the replenishment of N in ecosystems (Eisele et al., 1989). N supply on the other hand regulates the production of the enzyme phosphatase that cleaves ester P bonds in soil organic matter (McGill and Cole, 1981; Olander and Vitousek, 2000; Wang et al., 2007).
Biodiversity plays a crucial role in biogeochemical cycles. The fluxes and availability of N and P in soils depend on the interactions between the soil mineral matrix, plants and microbes (Cotrufo et al., 2013). For example, N input from atmospheric N2 fixation is mediated by a specialized group of microorganisms. Furthermore, the recycling of N from plants–soils–microbes determines the availability of N for plant uptake. Overall, the land biota dynamics impact the productivity, ecosystem resilience and stability (Yang et al., 2018). High diversity has been linked to enhanced vegetation productivity (Wagg et al., 2014). The diversity in terrestrial ecosystems is determined by biological, environmental and physicochemical processes. Anthropogenic activities can influence soil diversity, impacting the availability and cycling of N and P (Chen et al., 2019). For example, N and P fertilization has been shown to affect soil microbial biomass and composition (Ryan et al., 2009). Plant diversity is linked to soil health and functioning and is the core of the N and P cycles. Vegetation species' variable adaption to nutrient concentrations also plays a role in the availability of nutrients in soils and the biogeography of terrestrial vegetation. Overall, biodiversity constitutes an environmental resilience factor to abrupt changes (Van Oijen et al., 2020). However, implementing such dynamics remains far beyond the capabilities of the present-generation Earth system models. Several studies have found that in some ecosystems lack of N in soil usually leads to dominance of woody symbiotic N fixers (e.g. Menge et al., 2012). The availability of P is also impacted by the geochemical interactions in terrestrial soils, Vitousek et al. (2010) defined six mechanisms by which P is driven to limitation: loss by leaching; soil barriers that physically prevent access to roots; slow release of mineral P forms; P parent material; sequestration of P in soils and pools in the ecosystem; and, finally, anthropogenic input of nutrients.
Despite its importance P terrestrial limitation has been rare in Earth system modelling. The effect of P in tropical forests may be the key to better represent the vegetation biomass and the response to CO2 fertilization. The lack of P observational data is partly responsible for the difficulty of simulating P limitation in Earth system models (Spafford and MacDougall, 2021). However, several studies have attempted to provide reliable global P datasets (Yang et al., 2013; Hartmann et al., 2014; He et al., 2021) that could be used to develop more accurate models. Furthermore, many studies have shown that the inclusion of P into ESM structures is possible and that it improves the representation of vegetation biomass in tropical regions (Wang et al., 2007, 2010; Goll et al., 2012, 2017; Fleischer et al., 2019; Thum et al., 2019; Yang et al., 2019; Wang et al., 2020; Nakhavali et al., 2022). The addition of nutrient limitation has been observed to mainly affect the capacity of vegetation to absorb carbon (Wang et al., 2010; Goll et al., 2017; Wang et al., 2020). Therefore, the accumulation of carbon in the atmosphere is enhanced, leading to increases in temperature in simulations. These temperature changes are likely to have some impact on variables' sensitivity to atmospheric temperature changes. Furthermore, the decrease in vegetation biomass impacts variables sensible to the distribution and composition of plant functional types.
Intermediate-complexity Earth system models have a lower spatial representation and model structures that have been intentionally simplified in one or more ways. This simplification allows for long-term simulations that are typically not feasible in higher-complexity models. This class of model is not suitable for studying processes at small spatial scales. Hence, they are used in research questions that require large spatial and temporal scales (Weber, 2010). Current-generation Earth system models have already developed nutrient limitation to their model structure (e.g. Community Land Model, Lawrence et al., 2019; Joint UK Land Environment Simulator, Clark et al., 2011; Community Atmosphere–Biosphere Land Exchange model, Haverd et al., 2018; Australian Community Climate and Earth System Simulator, Ziehn et al., 2020). While CN models are more common, CNP models remain rarer. However, P cycles have been suggested to be included into Earth system models for its importance in tropical regions (Wang et al., 2010; Goll et al., 2012). The first attempt to include nutrient limitation in the University of Victoria Earth System Climate Model (UVic ESCM) was done by Wania et al. (2012) but was not included in the current publicly available version of the model due to the need for further improvement. We aim to describe a terrestrial N and P cycle adapted, developed and implemented for the UVic ESCM version 2.10. The main dynamics captured in this study are in the terrestrial system, especially vegetation. Furthermore, we intend to improve the current state of the previous N cycle implemented in the UVic ESCM, develop a new P cycle, and couple carbon N and P in order to improve the carbon cycle feedback projections.
2.1 Model description
The UVic ESCM is a climate model of intermediate complexity (ver. 2.10; Weaver et al., 2001; Mengis et al., 2020), and it contains a simplified moisture-energy balance atmosphere coupled with a three-dimensional ocean general circulation (Pacanowski, 1995) and a thermodynamic sea-ice model (Bitz et al., 2001). The model has a common horizontal resolution of 1.8∘ latitude and 3.6∘ longitude, and the oceanic module has a vertical resolution of 19 levels with a varying vertical thickness (50 m near the surface to 500 m in the deep ocean).
In version 2.10, the soil is represented by 14 subsurface layers with thickness exponentially increasing with depth with a surface layer of 0.1 m, a bottom layer of 104.4 m and a total layer of 250 m. Only the first eight layers have active hydrological processes (top 10 m); below that lies bedrock with thermal characteristics of granitic rocks. The soil carbon cycle is active in the top six layers up to a depth of 3.35 m (Avis, 2012; MacDougall et al., 2012). The soil respiration is a function of temperature and moisture (Meissner et al., 2003). The terrestrial vegetation is simulated by a top-down representation of interactive foliage and flora including dynamics (TRIFFID), representing vegetation interaction between five functional plant types: broadleaf trees, needleleaf trees, shrubs, C3 grasses and C4 grasses that compete for space in the grid following the Lotka–Volterra equations (Cox, 2001). Net carbon fluxes estimated in the model update the total areal coverage, leaf area index and canopy height for each plant functional type (PFT). For each PFT the carbon fluxes are derived from a photosynthesis–stomatal conductance model (Cox et al., 1998). The carbon uptake through photosynthesis is allocated to growth and respiration, and the vegetation carbon is transferred to the soil via litterfall and allocated to the soil as a decreasing function of depth (proportional to root distribution) and except for the top layer is only added to soil layers with temperatures above 1 ∘C.
Furthermore, permafrost carbon is prognostically generated within the model using a diffusion-based scheme meant to approximate the process of cryoturbation (MacDougall and Knutti, 2016). The sediment processes are modelled using an oxic-only calcium carbonate scheme (Archer, 1996). Terrestrial weathering is diagnosed from the spin-up net sediment flux and stays fixed at the preindustrial equilibrium value (Meissner et al., 2012). Mengis et al. (2020) merged the previous version of the UVic ESCM and evaluated its performance representing carbon and heat fluxes, as well as water cycles and ocean tracers. A full description of the model can be found in Mengis et al. (2020).
2.2 Nitrogen cycle
2.2.1 Nitrogen uptake
The new N cycle module was adapted from Wania et al. (2012). The module contains three organic (litter, soil organic matter and vegetation) and two inorganic (, ) N pools. The base structure is based on Gerber et al. (2010) with further modifications to fit the UVic ESCM scheme. is produced both from BNF and from the mineralization of organic N and can be taken up by plants (vegetation), leached or transformed into via nitrification. is produced through nitrification and can be taken up by plants, leached or denitrified into NO, N2O or N2. The inorganic N is distributed between leaf, root and wood, with wood having a fixed stoichiometry ratio and variable ratios for the leaf and root pools. Organic N leaves the living pools via litterfall into the litter pool which is either mineralized or transferred to the organic soil pool; a part of this N can be mineralized into the inorganic N pools. At the same time N can flow from the inorganic to the soil organic pool via immobilization. The CN ratios in leaves are determined by Eq. (1):
where Cleaf is the carbon content in leaves, and Nleaf is the N content in leaves. CNleaf is one of the most important nutrient limiters in the model. It controls the maximum carboxylation rate of RubisCO. Furthermore, it controls vegetation biomass. If the leaf C:N ratio is higher than CNleafmax (the maximum CN ratio parameter), terrestrial vegetation biomass is reduced.
The new version of the N cycle has been merged with a deep land-surface (MacDougall and Knutti, 2016) and a new wetland module (Nzotungicimpaye et al., 2021). Both inorganic N pools are transferred between soil layers following ground-water flow. Given this flow the distribution of N in layers was taken into account in the uptake calculations in Eqs. (2) and (3), and a root fraction was added (4), fixing the amount of root biomass per PFT per layer depth. The equations governing N uptake are
where and represent the N uptake. The left term is the active uptake, while the right term is the passive uptake (see Table 1); the latter is the transport of N via the transpiration water stream. Vmaxn is the maximum uptake rate for N; Croot is the root carbon biomass; [NH4(av)], [NO3(av)], and [Nmin(av)] are the NH4, NO3, and mineral N concentrations; is the half-saturation constant for N; and Qt is the transpiration rate. av represents the available portion of NH4 and NO3 in soil. This fraction is calculated as the total concentration of NH4 and NO3 divided by sorption factors (10 and 1, respectively) following Wania et al. (2012). The equation for root fraction is
where Ztop and Zbot represent the top layer and bottom layer depth, respectively, while D and dr are the depth of the soil layer and the root depth. The depth of the soil layer represents the depth of each specific soil layer. Root depth is a PFT-based parameter that represents the depth of the roots. Given the multiple-soil-layer setup, the root fraction modifies the value of root carbon, creating a more realistic representation of the uptake root depth reach for each PFT given the multiple-soil-layer setup.
2.2.2 Denitrification
The N cycle was merged with a wetland module that allowed for the estimation of anoxic fractions for each soil layer, based on Gedney and Cox (2003). The anoxic fraction is taken to be the saturated fraction of the soil layer that is shielded from O2 by the saturated soil layer above. The anoxia representation led to denitrification to be added to the N model, accounting for the largest exit pathway for N in the terrestrial biosphere. The anaerobic respiration is estimated from Eq. (5):
where Ran is the anaerobic respiration, is the ideal respiration rate via NO3 reduction, ft and fm are temperature and moisture functions, Cs is the concentration of organic carbon, Af is the anaerobic fraction, and Kn is the half-saturation of N oxides (Li et al., 2000). The temperature and moisture soil functions are taken directly from Cox (2001) and are represented by the following equations:
where in ft, q10=2, and ts is the soil temperature in ∘C. In fm, S is the soil moisture, SW is the wilting point of soil moisture, and S0 is the optimum soil moisture. Fluxes of N2O and NO to the atmosphere are computed based on the “leaky pipe” conceptualization of soil–nitrogen processes (Firestone and Davidson, 1989). In the leaky pipe conceptual model N2O and NO leak out of reactions of one species of nitrogen into another, namely nitrification (NH4 to NO3) and denitrification (NO3 to N2). The size of the holes is determined by the soil processes. For implementation in the UVic ESCM the size of the holes is fixed, but the partitioning ratio between NO and N2O changes based on the water-filled pore space of the soil layer. The ratio is parameterized based on an empirical relationship derived by Davidson et al. (2000):
where SU is the water-filled pore space. Thus, the model produces a total flux of both NO and N2O for nitrification and denitrification, which is partitioned between the two species based on the above relationship. The NO flux is added to the atmosphere and redeposited as part of the N deposition flux. The N2O flux is added to the N2O pool in the atmosphere, which has a characteristic half-life of 90.78 years (Myhre et al., 2013). Decayed N2O is assumed to become part of the atmospheric N2 pool.
2.2.3 Mass balance N cycle
In the Wania et al. (2012) N cycle module, under N limitation (CNleaf>CNleafmax), the N available was increased artificially by reducing the leaching by up to 100 % and if necessary the immobilization by 50 %. These mechanics created an unrealistic increase in N in soils and thereby defied the mass balance conservation of the module.
Here, the vegetation can no longer take up extra N from leaching or immobilization under nutrient limitation. Instead, under nutrient limitation wood and root carbon mass is transferred as litter (emulating a dying vegetation) until the correct ratio is met. Section 2.4 presents a detailed explanation of nutrient limitation for N and P.
Gerber et al. (2010)Wania et al. (2012)MacDougall and Knutti (2016)Wania et al. (2012)Avis (2012)Avis (2012)Avis (2012)Li et al. (2000)Wania et al. (2012)2.3 Phosphorus cycle
The P cycle is based on Wang et al. (2007, 2010) and Goll et al. (2017) with some equations where modified from Wania et al. (2012) to have a better consistency with N estimations in the new soil layer model. The module contains four inorganic (labile, sorbed, strongly sorbed and occluded) and three organic P pools: vegetation (leaf, root and wood), litter and soil organic P.
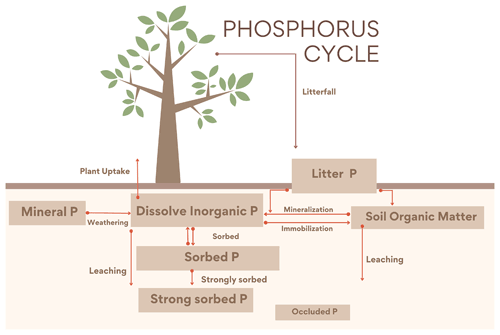
Figure 2Diagram representing the UVic ESCM CNP P cycle. Weathering from mineral P is the only input into the soils. There are four inorganic pools (dissolved inorganic, adsorbed, strongly sorbed and occluded P) and three organic pools (vegetation (root, wood and leaf), litter and soil organic matter). As in Wang et al. (2010), the flux from strongly sorbed P to the occluded pool is not represented here; instead it is assumed to be a fraction of the total soil P.
2.3.1 Input
The P module estimates weathering input following Wang et al. (2010) and is driven by a fixed estimate (Table 3) of P release assigned by soil order divided into 12 classes from the US Department of Agriculture (USDA) soil order map.
Table 3Constants for P input from Wang et al. (2010). The values change depending on the weathering state of the soil type. Highly weathered soils have lower values.
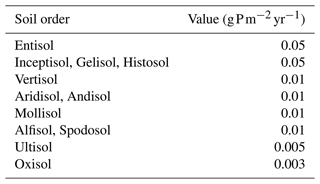
Additionally, an extra input structure was tested in the model but was not used for the P results in this study. It was implemented to compare the benefits of a static and a dynamic weathering scheme into the P pool. In this method weathering depends on runoff following Hartmann et al. (2014) using the lithological world map with 16 different classes generated by Hartmann and Moosdorf (2012). Equation (9) shows the estimation of the chemical weathering rate:
where FCW () is the chemical weathering rate, q is the runoff (mm yr−1) and bi is the factor for each lithological class i; shielding correction functions were not applied. The chemical weathering is defined as the total fluvial export of Ca + Mg + K + SiO2 and carbonate-derived CO3; bcarbonate and bsilicate are chemical weathering parameters associated with carbonate and silicate rocks, respectively, found in Hartmann et al. (2014). Here we only apply the Wang et al. (2010) approach, as we found it to be more controllable and advantageous for the planned coupling of the P flux from land into the ocean. Hartmann et al. (2014) requires the estimation of runoff by the model structure. Hence, while representing a dynamical P release, it needs to be carefully assessed so that no extreme overestimation or underestimation is represented regionally. The Wang et al. (2010) approach provides constant input without variability, which in this particular case is favourable.
2.3.2 Inorganic soil phosphorus
Inorganic P (Psoil) in soil follows the dynamics described in Goll et al. (2017) in Eq. (11), where each time step of a fixed fraction (ks) of P is adsorbed, and the rest is dissolved (1−ks). This fraction is based on the Hedley fractionation method (Hedley and Stewart, 1982), which is dependent on soil orders. The dataset has commonly been used to assess the different P forms in soil. The adsorbed P is regulated by ks in Eq. (12) as determined by the soil order in the Hedley dataset:
where Pwea is the P released by rock weathering, Plitmin is the P mineralized from the P litter pool, Porgmin is the P mineralized from the soil organic P, Pleach is the leached inorganic P, Pup is the P uptake by plants, Psorb is the amount of P sorbed, τsorb is the rate of strong sorption and Pimm is the P immobilized from the inorganic P pool. The estimation of Psoil based on Goll et al. (2017) is originally taken from Goll et al. (2012). Here, the sum of Psorb and Psoil constitutes the inorganic P pool in soil. Hence, the loss given by the rate of strong sorption is applied to the total inorganic P pool. The estimation of occluded P followed the Wang et al. (2010) approach, and based on Cross and Schlesinger (1995) the pool was assumed to be 35 % of the total soil P. Pleach and Pup were determined as in Eqs. (13) and (14) based on an adaptation of Wania et al.'s (2012) representation of leaching and uptake of N in the new soil layer model version:
where QD is the runoff. Vmaxp is the P maximum uptake rate, is the half-saturation constant for P, Croot is the root carbon and Froot is the root fraction.
2.3.3 Organic soil phosphorus
After uptake, P is distributed in three vegetation compartments: leaf, root and wood. Leaf and root have a dynamic value that varies between a minimum and a maximum, while wood has a fixed CP ratio. The vegetation P biomass dynamics are determined from the difference between the amount of uptake and the loss from litterfall as in Eq. (15), and the litterfall is estimated as the CP ratio of the original model litterfall as in Eq. (16):
where Vegp is the vegetation P change over time; PLF is the P litterfall; and Litleaf, Litroot, and Litwood are the carbon litterfall rates for vegetation carbon. The leaf CP ratio is determined as
where Cleaf is the carbon content in leaves, and Pleaf is the P content in leaves. CPleaf is one of the most important nutrient limiters in the model. The limiting effect of CPleaf is when its value is higher than the maximum CPleaf ratio parameter CPleafmax. This leads to biomass reduction. In contrast to CNleaf, CPleaf does not control the maximum carboxylation rate of RubisCO. A more detailed description of nutrient limitation can be found in Sect. 2.4. The litter biomass is added to the P litter pool (Plit), and its dynamic is based on Wang et al. (2007) as in Eq. (18):
where τlit is the rate constant for litter carbon decomposition (0.42 yr−1), Plitmin is the biochemical P litter mineralization, Ptase is the biochemical P mineralization rate, Utase is the maximum rate of P biochemical mineralization, λup is the N plant root cost to uptake P, λPtase is the critical value of the N cost of root P uptake above which phosphate production starts and Kptase is the Michaelis–Menten constant for biochemical P mineralization. Here, the N cost refers to the N required for protein structures involved in the metabolization of P in plants. Ptase is a constant value.
The decomposed soil litter is transferred to the soil organic P pool (Psom); the dynamics of Psom are adapted from Wang et al. (2007) as in Eq. (21):
where the first term represents the litter P input, while the other two are the Psom decomposition and mineralization. ε is the microbial growth efficiency (0.6), τs is the rate constant for soil carbon decomposition and Porgmin is the biochemical P mineralization. The immobilization is determined from the NP ratio of the N immobilization estimated by Wania et al. (2012).
2.4 Nitrogen and phosphorus limitation
The N cycle limits the terrestrial vegetation productivity in two distinct ways: it first limits the photosynthesis efficiency by controlling the maximum carboxylation rate of RubisCO (Vcmax). The RubisCO enzyme plays a crucial role in the photosynthesis biochemistry by catalysing the carboxylation reactions in the Calvin cycle and has been found to be linearly related to the N leaf content (Walker et al., 2014). The original equation for Vcmax takes into account a fixed N leaf (Cox et al., 1999); this is replaced by Wania et al. (2012) in the first N cycle using the calculated inverse average canopy leaf ratio (CNinvleaf). In this representation the plant productivity is reduced when CNleaf increases. Vcmax is calculated as
where λ is the constant of proportionality and 0.004 of C3 and 0.008 of C4 PFTs (Cox et al., 1999). Both N and P share the second form of limitation, where stoichiometric N and P limitation reduces the vegetation biomass. If the C:N ratio is too high, wood and root carbon biomass is transferred to the litter pool until the normal C:N ratio is reached (see Table 4).
The model assumes nutrient limitation when the estimated CN and CP leaf ratio is higher than the maximum CN (CNleafmax) and CP (CPleafmax) ratio in leaves. For grids with nutrient limitation the carbon in leaves is reduced to match the maximum CN or CP ratios in leaves. The carbon that is reduced is transferred to the litter pool. This reduction can happen for one or both nutrients until the ratio is met. The following equations regulate the reduction of biomass based on nutrient limitation:
where Cleaflimitedn and Cleaflimitedp are the carbon concentrations in leaves if the system is considered to be limited. Cleafdiffn and Cleafdiffp are the carbons lost due to nutrient limitation, and their values are summed in the litterfall equation when the system is nutrient limited.
2.5 Model runs and validation
The three different terrestrial biogeochemical versions, C, CN and CNP, were run for a historical simulation from 1850 to 2020. The C version serves as a baseline run representing the original version of the UVic ESCM ver. 2.10 (Mengis et al., 2020), the CN version is the modified version of the Wania et al. (2012) N model and CNP is the newest coupled model that includes P. Historical simulations are forced with fossil CO2 emissions, dynamically determined land use change emissions, non-CO2 greenhouse gas (GHG), sulfate aerosol, volcanic anomaly and solar. Furthermore, 24 historical simulations were run to assess the model sensitivity of six key parameters (CPleafmax, CNleafmax, Rleafp, Rleafn, Vmaxp, Vmaxn) in N and P limitation over terrestrial vegetation. The parameters were perturbed by increasing and reducing their value by 10 % and 20 % individually. CPleafmax and CNleafmax are the maximum leaf CP or CN ratios, respectively. If the values of CPleaf and CNleaf are above these thresholds, the model will take the system to be nutrient limited by either P or N. Rleafn and Rleafp are parameters that represent the resorption of N and P in leaves. This partly controls the loss of N and P from vegetation to the litter pool. Vmaxp and Vmaxn are the P and N maximum uptake rates.
Goll et al. (2017)Wang et al. (2010)Wang et al. (2010)Machado and Furlani (2004)Wang et al. (2007)Wang et al. (2007)Wang et al. (2007)Wang et al. (2007)Wang et al. (2007)Wang et al. (2007)Wang et al. (2007)Cox et al. (1999)It should to be noted that the porting of the N cycle from version 2.9 to 2.10 of the UVic ESCM and later model spin-up could slightly alter the results presented in Mengis et al. (2020). Hence, our baseline model is slightly different from the standard UVic ESCM ver. 2.10. The N cycle is compared to Zaehle et al. (2010), Li et al. (2000), Yang et al. (2009) and Wania et al. (2012). The N2O flux was compared with the Emissions Database for Global Atmospheric Research (EDGAR ver. 6.0; Crippa et al., 2021) dataset, which provided emission time series from 1970 until 2015 for non-CO2 GHGs for all countries.
For the P cycle, we used as benchmark for the carbon cycle the UVic ESCM version 2.10 model calibration values and references, which included the Le Quere et al. (2018) datasets. The total soil P was calibrated with the He et al. (2021) dataset. The labile and sorbed pools were calibrated using the Yang et al. (2013) P distribution map dataset. For the use of the He et al. (2021) dataset we transformed the units with Eq. (28):
where Psoil is the total P soil concentration (kg P m−2), Bkdensity (kg m−3) is the bulk density taken from the International Geosphere–Biosphere Programme Data and Information System (IGBP-DIS) (Global Soil Data Task Group, 2014), SLD (m) is the soil layer depth, and Pdataset (kg P (kg soil)−1) is the He et al. (2021) dataset. The foliar stoichiometry was compared to the latitudinal trend from Reich and Oleksyn's (2004) N:P observations.
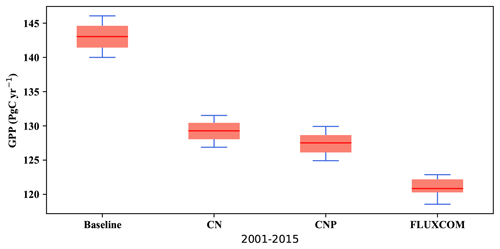
Figure 3Modelled yearly gross primary productivity (GPP) from 2001 to 2015 versus the FLUXCOM GPP dataset (Jung et al., 2019).
One of the challenges of modelling nutrients in terrestrial systems is the lack of observations and validation datasets. Furthermore, the existing range of values for N and P variables is highly uncertain. This large range in values makes it difficult to accurately tune models. Although, improvements are in sight, with new artificial-intelligence-derived global datasets beginning to become available (He et al., 2021). Model validation has been advancing quickly in the last decade (Spafford and MacDougall, 2021) with tools such as the International Land Model Benchmarking (Collier et al., 2018) that significantly improve terrestrial model validation. However, there are limited variables available to compare to nutrient model development. The increase in the addition of nutrient structures in ESMs (Arora et al., 2020) suggests the need for terrestrial nutrient validation tools to improve model accuracy in the developmental phase. Moreover, a terrestrial nutrient model intercomparison project would unify global efforts to improve the representation of N and P in ESMs.
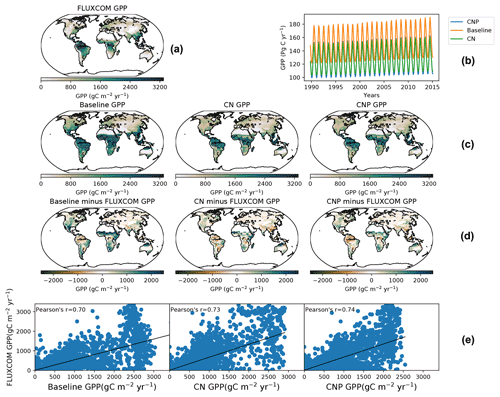
Figure 4(a) The FLUXCOM GPP dataset from 2000–2010 and (b) seasonal GPP from 1990–2015 for the baseline, CN and CNP. (c) The second line shows the global GPP from 2000–2010 for the baseline, CN and CNP. (d) The third line shows the difference between the baseline, CN and CNP as well as the FLUXCOM GPP dataset. Panel (e) shows the correlation of the baseline, CN and CNP with the FLUXCOM GPP dataset.
3.1 Carbon cycle
3.1.1 Land global primary productivity
The global gross productivity in CN and CNP resulted in a better agreement with the FLUXCOM GPP dataset (Jung et al., 2019) as shown in Fig. 3, with both CN and CNP overestimating the terrestrial global GPP average less than the baseline simulation. Compared to the baseline simulation (143 Pg yr−1) both nutrient-limited model versions showed a reduced mean GPP from the years 2001–2015 with CN at 130 Pg yr−1 and CNP at 127 Pg yr−1. Furthermore, the modifications for the N cycle in regard to the mass balance changes resulted in the reduction in mean GPP from 129 Pg yr−1 (Wania et al., 2012) to 122 Pg yr−1 in the 1990s. The GPP distributions from the baseline, CN and CNP reproduce FLUXCOM dataset values reasonably well (Fig. 4). The seasonal pattern of GPP is also well represented within out simulations as shown in Fig. 4. The addition of nutrients improves the representation of GPP, where CNP had the highest correlation with the FLUXCOM GPP dataset. The high GPP in the baseline simulation can be explained by the overestimation of the vegetation biomass, especially broadleaf trees in tropical regions, as stated in Mengis et al. (2020). The representation of vegetation biomass is linked to the PFT fractions in the model. In the CN and CNP simulations the reduction in biomass is critical for the reduction in terrestrial productivity, especially in tropical regions where P availability has been shown to be a limiting factor for GPP (Du et al., 2020). Similarly to the findings of Wania et al. (2012), Bonan and Levis (2010), and Zaehle et al. (2010), the addition of nutrient limitation in ESMs seems to reduce GPP. Furthermore, locally in Amazonian soils, Nakhavali et al. (2022) found that the inclusion of P reduces the model GPP and NPP outputs by 5.1 % and 4.5 %, respectively, for a site simulation. Similarly to Nakhavali et al. (2022), we found an overall reduction in GPP in the Amazonian region.
The nutrient limitation reduced the amount of land–atmosphere carbon flux in the simulations. The cumulative land uptake from 1850–2005 was 150 Pg C yr−1 in CNP, lower than the version 2.10 calibration in Mengis et al. (2020) (177 Pg C yr−1). This change in response is crucial for understanding the future dynamics in the Shared Socioeconomic Pathway (SSP) projections, as terrestrial vegetation is expected to decrease its capacity to store carbon in the future (Goll et al., 2012). Overall, the carbon feedback values are in concordance with the ranges of the Global Carbon Project used in Mengis et al. (2020) (Le Quere et al., 2018) where the cumulative carbon flux was estimated to be 141 Pg C yr−1 from 1850–2005. The atmosphere to land carbon flux follows the Global Carbon Project (GCP) dataset's (Le Quere et al., 2018) magnitude closely.
Similarly to Wania et al. (2012), we found higher values of NPP for CN (77.4 Pg C yr−1) compared to the baseline simulation (74.2 Pg C yr−1), while CNP (72 Pg C yr−1) resulted in lower values due to the reduction in tropical vegetation biomass. CN and CNP results are close to the upper range (21.5 to 69.3 Pg C yr−1) of simulated NPP shown in Li et al. (2015). The reduction in tropical biomass mainly in broadleaf tree carbon is reflected in the fraction of the PFT shown in the model output. Wania et al. (2012) argued that the reason behind the high NPP was the dependence of autotrophic respiration on N content in the leaf, root and stem, which are based on the original MOSES/TRIFFID version (Cox et al., 1999). In CN and CNP, the reduction in wood CN ratios and increase in N leaf content compared to baseline simulations, in which N leaf fluctuates from a minimum to a maximum value, gives place to the reduction in the maintenance respiration, which reduces the autotrophic respiration and consequently NPP. Furthermore, in the new CNP version, while wood CN remains fixed, the stoichiometrical reduction in wood carbon by the lack of P availability decreases wood carbon even more, especially in tropical forests and other tropical ecosystems.
3.1.2 Atmospheric CO2 concentration
The simulated CNP atmospheric CO2 concentration matches observations very closely, and the addition of N and P has shown an improvement in the representation of the model accumulation of carbon in the atmosphere. The CO2 concentration has improved compared to the evaluated 2.10 version of the UVic ESCM where from 1960 to 2010 the simulation deviated above the observed curve (Δ78 ppm in the simulation compared to Δ73 ppm observations; Mengis et al., 2020). Compared with the CN and baseline simulations (Fig. 3), CNP provides a more accurate representation of the atmospheric CO2 concentration. Thus the nutrient limitation has effectively reduced the CO2 fertilization effect on the terrestrial vegetation. Consequently, CN and CNP show a larger pool of atmospheric CO2.
3.1.3 Terrestrial vegetation
Given that tropical forests and savannas are commonly limited by the availability of P, the simulated vegetation biomass representation is affected by the absence of nutrient limitation in ESMs. Nakhavali et al. (2022) found that the addition of P improved the vegetation estimations and the carbon cycle response to rising CO2 for the Amazonian region, basing their study on a site representative of 60 % of the Amazonian soils.
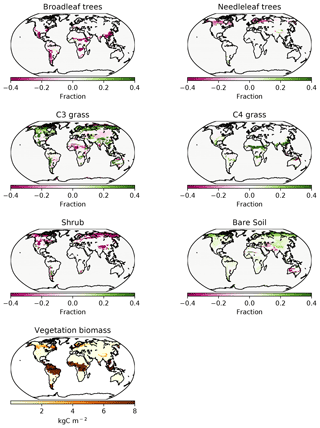
Figure 6PFT fractions in the UVic ESCM for 1980–2010, CNP minus baseline. The last plot at the bottom shows CNP global biomass distribution.
In the CNP version of the model, broadleaf tree coverage declined in tropical and subtropical latitudes (Fig. 6) with the largest changes located in south eastern Asia, Africa and South America. The reduction in vegetation biomass ranged from 6 %–20 % in South America and Africa, while a higher reduction of 20 %–30 % was present in south eastern Asia. The magnitude of the continental difference can be attributed to the base internal vegetation biomass model version bias (Mengis et al., 2020). Additionally, CN and CNP show a shift in coverage where broadleaf trees are taken over by C3 grass.
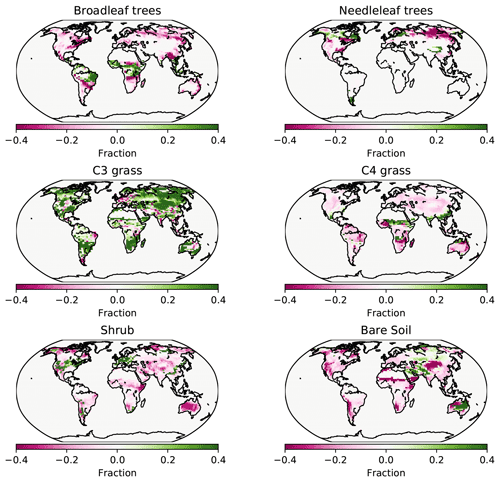
Figure 7PFT fractions in the UVic ESCM for 2008–2012, CNP minus the Poulter et al. (2015) PFT dataset.
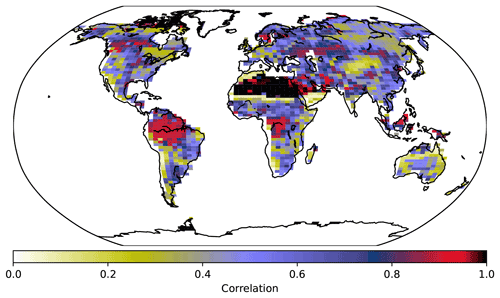
Figure 8PFT fractions across grid cells in the UVic ESCM for 2008–2012, CNP correlation with the Poulter et al. (2015) PFT dataset.
Needleleaf trees were reduced in North America and Europe. Both CN and CNP simulations' vegetation carbon resulted in a decrease in vegetation biomass with 456 and 525 Pg C, respectively, compared to the baseline simulation (594 Pg C), similar to Zaehle et al. (2010). Overall CNP shows a high correlation with all PFT coverage when compared with the Poulter et al. (2015) PFT dataset. In tropical regions our model seems to represent vegetation closely to the data (Figs. 7 and 8).
The total vegetation carbon is similar to Wania et al. (2012), with tropical forests having a range from 8–16 and 4–12 kg C m−2 in temperate and boreal forests with means of 10.50 and 6.7 kg C m−2, respectively, compared to 12–16 and 4–12 kg C m−2 and means of 13.4 and 7.3 kg C m−2. The latitudinal mean shows a decrease in the range of vegetation carbon in tropical latitudes of 1–1.5 and 0.4–0.8 kg C m−2 in northern template latitudes. These results indicate that the main reduction in vegetation carbon is in the tropics, which agrees with the general N and P global pattern (Du et al., 2020). Consistent with Wania et al. (2012), the vegetation carbon outputs are similar to those of Malhi et al. (1999), with 12.1 kg C m−2 for tropical and 5.7–6.4 for temperate and boreal forests.
3.2 Nitrogen cycle
3.2.1 Nitrogen distribution
The soil N ranges from 0 to 1.5 kg N m−2 with lower N in the tropics and increasing N towards the temperate regions. Globally, the CNP-simulated soil N is reduced compared to the original N structure in the UVic ESCM version 2.9 presented by Wania et al. (2012). The primary differences between the Wania et al. (2012) N cycle and the current version are the soil layer structure and the stoichiometry response to N limitation. In the former, N could be transferred from other pools when N was outside of the ratio threshold and thereby could be considered to be limiting vegetation.
This result is also lower than the 0 to 4.8 kg N m−2 from the IGBP-DIS database (Global Soil Data Task Group, 2000). Wania et al. (2012) stated that the N content in the model is dependent on soil carbon fixed via a fixed CN ratio. Given this, lower carbon values can lower soil N values in CN simulations. Thereby, lower carbon in soil could be a strong reason why our results have less N than the IGBP-DIS database (Global Soil Data Task Group, 2000) and Wania et al. (2012). However, our values fall within the range of uncertainty. Our model estimates a mean BNF for 2010–2020 of 119 Tg N yr−1. This value is above 35 Tg N yr−1 from Braghiere et al. (2022) and within the range of 52–130 Tg N yr−1 presented by Davies-Barnard and Friedlingstein (2020).
3.2.2 Vegetation nitrogen
The total amount of vegetation N (2.20 Pg N) was lower than the previous N cycle (2.94 Pg N, Wania et al., 2012). These values are similar to Zaehle et al. (2010) (3.8 Pg N) and Wang et al. (2018) (3.9 PgN) but lower than Li et al. (2000) (16 Pg N) and Yang et al. (2009) (18 Pg N). Our tropical (30 to 45 g N m−2) and boreal forest vegetation N (20 to 35 g N m−2) results are lower than the results from Wania et al. (2012) (30 to 40 g N m−2) and those of Xu-ri and Prentice (2008) and Yang et al. (2009) (both studies ranged between 150 and 400 g N m−2).
The global pattern of the CN ratio is similar to the Wania et al. (2012) structure with the highest located in tropical regions, especially South America and south eastern Asia. Tropical forests show a value that ranges from 230–280 C:N (Fig. 9) compared to 250–300 C:N shown by Wania et al. (2012). The reduction in wood carbon in the tropics by P limitation in CNP lowered the C:N ratios. Our values are within the observational range of uncertainty (95–730) stated in Martius (1992).
The distribution of vegetation N resembles the results of Du et al. (2020) where N's primary effect is in higher latitudes. The PFT fraction changes show that N mainly limits North and central America (BR and NL), Chile (BR), the Argentinian Patagonia (BR), northern Europe (NL), and eastern Asia (BR) (Fig. 6). However, there seems to be N limitation in tropical Africa and Asia in our model simulations. Even though our model does not represent co-limitation, the stoichiometric limitation does seem to indirectly capture this effect.
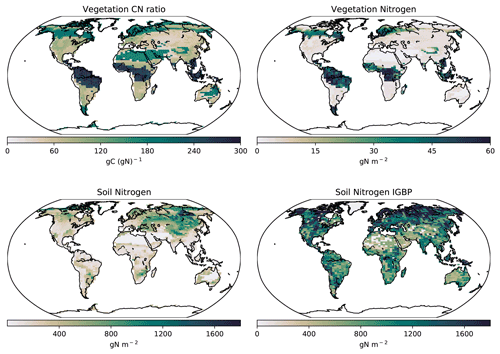
Figure 9Modelled global soil and vegetation N in the CNP version of the UVic ESCM from 1980–1999. The lower-right map corresponds to the soil N from the IGBP-DIS dataset (Global Soil Data Task Group, 2000).
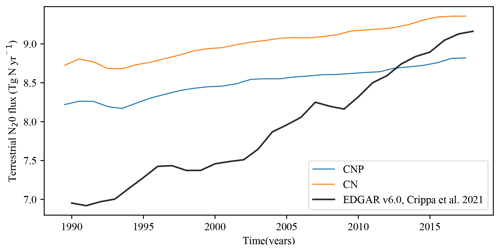
Figure 10The CNP and CN global soil N2O emissions vs. the EDGAR version 6.0 N2O dataset (Crippa et al., 2021).
3.2.3 N2O fluxes
The multilayer model has allowed for the estimation of anoxic regions, and, hence, a major improvement in the model is the quantification of terrestrial N2O flux. Figure 10 shows CN and CNP N2O fluxes from 1990 to 2018. Compared to the EDGAR version 6 dataset (Crippa et al., 2021), our model simulates N2O fluxes relatively well, agreeing mostly in the last 10 years of the values. However, we observed an overestimation from 1990 to 2010. The CN version of the model fits within the lower natural (natural soil, atmospheric N deposition on land) and anthropogenic (agriculture, fossil fuel and industry) emission range (8.9–14.3 Tg N yr−1) given by the Global Carbon Project (Tian, 2020), while CNP falls just below the lower-range value. The reduction in N in the model system by the P effect is shown by these results; the reduction in vegetation biomass and then litterfall reduces the amount of N transfer to the N soil pool, limiting the natural denitrification. The lack of oceanic production of N2O in the model makes the comparison with the global total N2O flux impossible at the moment. The total estimates for N2O emissions are 4.2 to 11.4 Tg N yr−1 for anthropogenic and 8.0 to 12.0 Tg N yr−1 for natural emissions, as given by the Global Carbon Project (Tian, 2020). Assuming an ocean output of a mid-range emission (3.4 Tg N yr−1), the model simulations are close to the lower range of the emission reported with CN (13.3 Tg N yr−1) and CNP (12.1 Tg N yr−1). Lighting and atmospheric production, biomass burning (addition of N2O to atmospheric pool), and post-deforestation pulse effects are not taken into account in the model structure, and that could improve the fit of the simulation to a mid-range-level value.
3.3 Phosphorus cycle
3.3.1 Inputs and losses
The P global weathering rate estimated is 3 Tg P yr−1, similar to 2 Tg P yr−1 in Wang et al. (2010). Fertilization inputs of 1 Tg P yr−1 (Filipelli, 2002) were added as an option to the model but were not used for the current simulations, and dust deposition is not accounted for. Hence, the only P input into the system in this experimental setup comes from rock weathering. Regarding the P weathering representation, the Hartmann et al. (2014) approach was tested at first, but the Wang et al. (2010) weathering scheme resulted in a better, simplified and controllable input. Although, the Hartmann et al. (2014) approach was found to be superior since P input is dynamic, incorporating model runoff and lithological map distribution. A dynamic P input will also require a better representation of P losses in order to maintain a steady state.
The P weathering was set so that the loss by leaching (3 Tg P yr−1) was comparable to the riverine input stated in Filipelli (2002) of 4–6 Tg P yr−1. The gap corresponds to anthropogenic inputs not included here. The preindustrial P input to the ocean from riverine input is 2–3 Tg P yr−1, and human activities, especially agriculture (fertilizers) and water waste, roughly correspond to a doubling of the P input.
3.3.2 Land P pools and storages
The total inorganic and organic P values are similar to those shown in the results of Smil (2000), Mackenzie (2002) and Wang et al. (2010) (Table 7), although organic P is slightly underestimated in the model (3.5 Pg P). This underestimation is likely the result of the lack of P fertilization on land. The labile P, sorbed P, strongly sorbed P and occluded pools are values comparable to Wang et al. (2010).
Smil (2000)Mackenzie (2002)Wang et al. (2010)Wang et al. (2018)Smil (2000)Mackenzie (2002)Wang et al. (2010)Yang et al. (2013)Wang et al. (2010)Yang et al. (2013)Wang et al. (2010)Wang et al. (2010)Wang et al. (2010)Wang et al. (2010)Smil (2000)Wang et al. (2018)Wang et al. (2018)Mackenzie (2002)Wang et al. (2010)Wang et al. (2018)Globally the total soil P distribution (Fig. 11) is comparable to the He et al. (2021) dataset, which is one of the few terrestrial P concentration maps available. Overall, the model simulates less global P, especially in northern latitudes, most likely due to the oversimplified weathering scheme that underestimated the inputs in higher latitudes.
Latitudinally, the tropical soils showed the lowest P with the exception of highlands and mountains, while P increased sequentially towards the northern latitudes as shown in He et al. (2021). The labile P shows a similar distribution as Yang et al. (2013) with tropical regions being relatively depleted compared to other regions due to the high adsorption and occlusion by the soils.
In contrast with N, P inputs are limited by the mineral (apatite) concentration and weathering rate rather than biologically fixed. Most of the P is retained by soils, leaving a small labile fraction for biological uptake. Because P mineral weathering and chemical recycling in the soils are so constraining, our linear model approach for adsorption based on Goll et al. (2017) might overestimate the impact of adsorption and occlusion in tropical soils. It is also worth noting that the biological impact on the adsorption–desorption dynamics is missing in most P modules in ESMs. The release of P from mineral grains can be enhanced by either the reduction in pH due to respiration or the direct addition of organic acids by plant roots (Schlesinger, 1997).
3.3.3 Phosphorus in vegetation
The terrestrial vegetation shows a slight underestimation in comparison with other models. The new stoichiometry limitation scheme of the model plays an important role in the vegetation biomass and could be the reason for the underestimated values, especially for tropical regions. However, the range of P in terrestrial vegetation is still uncertain, with several studies showing a range from 1.8–3.0 Pg P (Smil, 2000). Although Wang et al. (2010) have dismissed those values as overestimations given an overall N:P ratio of 10–20 g N g P−1, 3 Pg P is simply too high to be met.
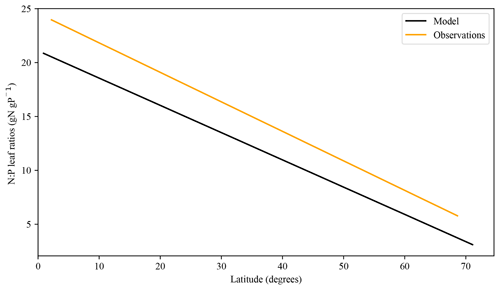
Figure 12Modelled N:P leaf ratio trend vs. an empirical relationship derived from Reich and Oleksyn (2004).
The foliar stoichiometry seems to approximately follow the N:P ratio field measurements of Reich and Oleksyn (2004) (Fig. 12). The tropical regions show some underestimated values in our model; the low amount of labile P and the later decrease in broadleaf tree biomass could be responsible for the low numbers. Similarly, Nakhavali et al. (2022) show model values of 4–15 g P m−2 for an Amazonian site, which surpasses our results.
A more complex adsorption–desorption scheme might be beneficial for solving the underestimation for tropical latitudes, as those regions are heavily sorbed and lose most of the input P, even though the need for a proper global P vegetation dataset is imperative to have proper ranges in global distributions. The mechanical reduction in vegetation stoichiometrically by the model structure might also be too simplistic to represent P limitation in the tropics.
3.4 Parameter sensitivity
We perturbed six parameters (CPleafmax, CNleafmax, Rleafp, Rleafn, Vmaxp, Vmaxn) over historical simulations to assess the model sensitivity in terms of limitation of N and P. All of the above parameters play an important role in the nutrient limitation structure of the model. Pleafmax and Nleafmax control terrestrial vegetation when the stoichiometrical limitation is set to be enforced on terrestrial vegetation, and Rleafp, Rleafp, Vmaxp and Vmaxn control the uptake, litterfall and allocation of nutrients in leaves. In each case, default values were increased and decreased by 10 % and 20 % while holding other parameters constant. The results were compared to model simulations with all parameters held constant and set to default values. The cumulative atmosphere–land carbon flux was used to measure the effect of the perturbation, since the limitation directly affects this flux.
The results of the sensitivity study show that the model's sensitivity varies with different parameters (Table 8). The UVic ESCM is the most sensitive to perturbations of CPleafmax and CNleafmax because both directly determine the threshold by which vegetation carbon is reduced and nutrient limitation is defined. The model seems to be the most sensitive to changes in CPleafmax. The regulation of this parameter is very useful for calibrating woody vegetation in tropical regions to improve the cover representation. The other parameters have a lower impact on the atmosphere–land carbon flux, ranging from −3.23 % to +1.60 %.
The UVic ESCM has been a critical tool in developing the cumulative emissions framework for climate mitigation (Zickfeld et al., 2009; Matthews et al., 2009; Matthews and Weaver, 2009; MacDougall and Knutti, 2016; Mengis et al., 2018; Tokarska et al., 2019). Due to its low computational cost and strict enforcement of matter and energy conservation, the model is capable of conducting a host of simulations beyond the limits of most other models but with higher resolution than other intermediate-complexity models (e.g. Montenegro et al., 2007; Matthews and Caldeira, 2008; Keller et al., 2014; MacDougall and Knutti, 2016; MacDougall, 2017; Pahlow et al., 2020; Kvale et al., 2021) . As terrestrial nutrient limitation constrains the carbon cycle in nature, the new N and P modules allow for addressing research questions relating to carbon budgets, carbon cycle and CH4 feedbacks, carbon dioxide removal, and permafrost carbon cycles, among other questions. Furthermore, the N and P cycles can represent critical environmental and climate processes such as the release of N2O, agricultural impacts on terrestrial soils and coastal lines, eutrophication, anoxic events, and nutrient fluxes from land to the ocean.
A number of limitations have been identified with the developed N and P modules that relate to the degree of complexity or the lack of large-scale datasets available. Due to the lack of global estimates of nutrient pools and fluxes based on field measurements, many of the parameters or parameterizations in this model are poorly constrained. In general, these are the following model limitations that are planned to be improved in future model development projects:
-
The model does not include a dynamic nutrient leaf resorption rate. Under nutrient limitations, this rate can increase as a strategy to conserve nutrients (Reed et al., 2012). Thus, the effect of limitation in our model might be overestimated.
-
There is a static input of P from weathering. To control the P input we chose to estimate weathering flux by adding a fixed amount. This oversimplification could add more uncertainty to the P pools and can be overcome using a runoff-based weathering scheme. Moreover, we do not account for P atmospheric dust deposition.
-
The sorption–desorption dynamics of P in soil are oversimplified. We chose the Goll et al. (2017) approach because it was a simpler way to represent this process. However, a more complex solution might improve the distribution of P globally.
-
An ocean N2O output is absent. Consequently, we are unable to estimate the total amount of a dynamically evolving N2O concentration at this time. As N2O is the third most important greenhouse gas (IPCC, 2022), its incorporation into the model is a priority.
-
The model does not account for root uptake constrains of N and P on terrestrial vegetation. This includes spatial representations of mycorrhizal associations and the carbon cost of nitrogen and phosphorus uptake from soil (Shi et al., 2016; Braghiere et al., 2021, 2022).
The CNP model is primarily designed to improve carbon cycle feedbacks under current and future climate conditions. The use of nutrient limitation improves the land–atmosphere dynamics. In simulations, this improvement has a significant impact on atmospheric CO2 concentrations. In future studies, we intend to assess the impact of nutrient limitation on different SSP scenarios and key carbon cycle benchmark metrics. Furthermore, the model can be used to improve the vegetation representation in ESMs. Finally, the CNP model may be used to generate coastal nutrient input and to integrate terrestrial nutrient biogeochemical processes with oceanic processes.
The N and P cycles simulated here fit into the range of uncertainty shown in datasets and other modelling efforts. Generally, our values fall into the lower range of the spectrum. N is limited in mainly high latitudes, especially in northern regions, but does show some limitation in tropical Africa and Asia. P limitations are greater in tropical regions and reduced the vegetation biomass compared to the carbon-only version of the model, bringing the model closer to observations (Mengis et al., 2020).
The two nutrient limitation models have improved the representation of the atmospheric carbon concentration in simulations forced with CO2 emissions using the Keeling curve as benchmark data. The land–atmospheric flux fits other simulation datasets and has been reduced from Mengis et al.'s (2020) values. Overall N and P addition has improved the carbon cycle feedbacks simulated in historical simulations. The GPP is lowered, especially in the tropics, mainly due to the reduction in woody vegetation biomass.
Many improvements remain to be made in our model structure. In regard to the N cycle, denitrification processes need to be improved, and N2O fluxes, while of the same magnitude as observations, lack the trend shown in other benchmark datasets. The complexity of the P cycle could be improved, especially the input and sorption processes. Finally both N and P cycles could gain accuracy from adding dynamic leaf reabsorption rates that have been shown to change when nutrient limitation is present in the ecosystem and that can be used as in Du et al. (2020) to clearly map the limitation pattern. Despite these limitations the improved model has shown higher fidelity to observations and is expected to improve projections of future key carbon cycle feedbacks.
The model code and data used for this paper are available at https://doi.org/10.5683/SP3/GXYZKU (De Sisto, 2022).
AHMD initiated the redevelopment of the N module. MDS ported the N module to the UVic ESCM version 2.10 and further improved it. MDS developed the P cycle. MDS wrote the paper, and AHMD provided supervisory support. NM contributed to the interpretation and validation of the model results. SA contributed to the visual representation of the P module structure and model results.
The contact author has declared that none of the authors has any competing interests.
Publisher's note: Copernicus Publications remains neutral with regard to jurisdictional claims in published maps and institutional affiliations.
Andrew H. MacDougall and Makcim L. De Sisto are grateful for the support from the Natural Sciences and Engineering Research Council of Canada's Discovery Grants programme and support from Compute Canada (now the Digital Research Alliance of Canada). We are indebted to Michael Eby for early advice on implementing the nitrogen version of the model and for providing the model code for the original N cycle version of UVic ESCM.
This research has been supported by the Natural Sciences and Engineering Research Council of Canada’s Discovery Grants programme.
This paper was edited by Hans Verbeeck and reviewed by three anonymous referees.
Archer, D.: A data-driven model of the global calcite lysocline, Global Biogeochem. Cy., 10, 511–526, https://doi.org/10.1029/96GB01521, 1996. a
Arora, V. K., Katavouta, A., Williams, R. G., Jones, C. D., Brovkin, V., Friedlingstein, P., Schwinger, J., Bopp, L., Boucher, O., Cadule, P., Chamberlain, M. A., Christian, J. R., Delire, C., Fisher, R. A., Hajima, T., Ilyina, T., Joetzjer, E., Kawamiya, M., Koven, C. D., Krasting, J. P., Law, R. M., Lawrence, D. M., Lenton, A., Lindsay, K., Pongratz, J., Raddatz, T., Séférian, R., Tachiiri, K., Tjiputra, J. F., Wiltshire, A., Wu, T., and Ziehn, T.: Carbon–concentration and carbon–climate feedbacks in CMIP6 models and their comparison to CMIP5 models, Biogeosciences, 17, 4173–4222, https://doi.org/10.5194/bg-17-4173-2020, 2020. a
Avis, C. A.: Simulating the Present-Day and Future Distribution of Permafrost in the UVic Earth System Climate Model, PhD thesis, University of Victoria, http://hdl.handle.net/1828/4030, 2012. a, b, c, d
Bitz, C. M., Holland, M. M., Weaver, A. J., and Eby, M.: Simulating the ice-thickness distribution in a coupled, J. Geophys. Res., 106, 2441–2463, https://doi.org/10.1029/1999JC000113, 2001. a
Bonan, G. B. and Levis, S.: Quantifying carbon-nitrogen feedbacks in the Community Land Model (CLM4), Geophys. Res. Lett., 37, 2261–2282, 2010. a
Braghiere, R. K., Fisher, J. B., Fisher, R. A., Shi, M., Steidinger, B. S., Sulman, B. N., Soudzilovskaia, N. A., Yang, X., Liang, J., Peay, K. G., Crowther, T. W., and Phillips, R. P.: Mycorrhizal distributions impact global patterns of carbon and nutrient cycling. Geophysical Research Letters, 48, e2021GL094514, https://doi.org/10.1029/2021GL094514, 2021. a
Braghiere, R. K., Fisher, J. B., Allen, K., Brzostek, E., Shi, M., Yang, X., Ricciuto, D. M., Fisher, R. A., Zhu, Q., and Phillips, R. P.: Modeling global carbon costs of plant nitrogen and phosphorus acquisition, J. Adv. Model. Earth Sy., 14, e2022MS003204, https://doi.org/10.1029/2022MS003204, 2022. a, b
Chen, X., Dunfield, K., Fraser, T. D., Wakelin, S., Richardson, A., and Condron, L. M.: Soil biodiversity and biogeochemical function in managed ecosystems, Soil Res., 58, 1–20, https://doi.org/10.1071/SR19067, 2019. a
Clark, D. B., Mercado, L. M., Sitch, S., Jones, C. D., Gedney, N., Best, M. J., Pryor, M., Rooney, G. G., Essery, R. L. H., Blyth, E., Boucher, O., Harding, R. J., Huntingford, C., and Cox, P. M.: The Joint UK Land Environment Simulator (JULES), model description – Part 2: Carbon fluxes and vegetation dynamics, Geosci. Model Dev., 4, 701–722, https://doi.org/10.5194/gmd-4-701-2011, 2011. a
Collier, N., Hoffman, F. M., Lawrence, D. M., Keppel-Aleks, G., Koven, C. D., Riley, W. J., Mu, M., and Randerson, J. T.: The International Land Model Benchmarking (ILAMB) System: Design, Theory, and Implementation, J. Adv. Model. Earth Sy., 10, 2731–2754, https://doi.org/10.1029/2018MS001354, 2018. a
Cotrufo, M. F., Wallenstein, M. D., Boot, C. M., Denef, K., and Paul, E.: The Microbial Efficiency-Matrix Stabilization (MEMS) framework integrates plant litter decomposition with soil organic matter stabilization: do labile plant inputs form stable soil organic matter?, Glob. Change Biol., 19, 988–995, https://doi.org/10.1111/gcb.12113, 2013. a
Cox, P. M.: Description of the TRIFFID dynamic global vegetation model, Tech. Rep. 24, Hadley Centre, Met Office, London Road, Bracknell, Berks, RG122SY, UK, 2001. a, b
Cox, P., Huntingford, C., and Harding, J.: A canopy conductance and photosynthesis model for use in a GCM land surface scheme, J. Hydrol., 212–213, 79–94, https://doi.org/10.1016/S0022-1694(98)00203-0, 1998. a
Cox, P. M., Betts, R. A., Bunton, C. B., Essery, R. L. H., Rowntree, P. R., and Smith, J.: The impact of new land surface physics on the GCM simulation of climate and climate sensitivity, Clim. Dynam., 15, 183–203, 1999. a, b, c, d
Crippa, M., Guizzardi, D., Muntean, M., Schaaf, E., Lo Vullo, E., Solazzo, E., Monforti-Ferrario, F., Olivier, J., and Vignati, E.: EDGAR v6.0 Greenhouse Gas Emissions, European Commission, Joint Research Centre (JRC) [data set], http://data.europa.eu/89h/97a67d67-c62e-4826-b873-9d972c4f670b (last access: 15 January 2023), 2021. a, b, c
Cross, A. F and Schlesinger, W. H.: A literature review and evaluation of the Hedley fractionation: applications to the biogeochemical cycle of soil phosphorus in natural ecosystems, Geoderma, 64, 197–214, 1995. a
Davidson, E.,Keller, M, Erickson, H., Verchot, L., and Veldkamp, E.: Testing a conceptual model of soil emissions of nitrous and nitric oxides: using two functions based on soil nitrogen availability and soil water content, the hole-in-the-pipe model characterizes a large fraction of the observed variation of nitric oxide and nitrous oxide emissions from soils, AIBS Bulletin, 50, 667–680, 2000. a
Davies-Barnard, T. and Friedlingstein, P.: The global distribution of biological nitrogen fixation in terrestrial natural ecosystems, Global Biogeochem. Cy., 34, e2019GB006387, https://doi.org/10.1029/2019GB006387, 2020. a
De Sisto, M.: Modelling the terrestrial nitrogen and phosphorus cycle in the UVic ESCM, V1, Borealis [code, data set], https://doi.org/10.5683/SP3/GXYZKU, 2022. a
Du, E., Terrer, C., Pellegrini, A., Ahlstrom, A., Van Lissa, C., Zhao, X., Xia, N., Wu, X., and Jackson, R.: Global patterns of terrestrial nitrogen and phosphorus limitation, Nat. Geosci., 13, 221–226, https://doi.org/10.1038/s41561-019-0530-4, 2020. a, b, c, d, e, f, g, h, i, j
Dynarski, K. A., Morford, S. L., Mitchell, S. A., and Houlton, B. Z.: Bedrock nitrogen weathering stimulates biological nitrogen fixation, Ecology, 100, 1–10, 2019. a
Eisele, K., Schimel, D., Kapustka, L., Parton, W.: Effects of available P and N:P ratios on non-symbioticdinitrogen fixation in tall grass prairie soils, Oecologia, 79, 471–474, 1989. a
Filippelli, G.: The global phosphorus cycle, in phosphates: Geochemical, geobiological, and materials importance, Rev. Mineral. Geochem., 48, 391–425. 2002. a, b, c, d, e
Firestone, M. and Davidson, E.: Microbiological basis of NO and N2O production and consumption in soil. Exchange of trace gases between terrestrial ecosystems and the atmosphere, Wiley, 47, 7–21, 1989. a
Fisher, J., Badgley, G., and Blyth, E.: Global nutrient limitation in terrestrial vegetation, Global Biogeochem. Cy., 6, GB3007, https://doi.org/10.1029/2011GB004252, 2012. a
Fleischer, K., Dolman, A. J., van der Molen, M. K., Rebel, K. T., Erisman, J. W., Wassen, M. J., Pak, B., Lu, X., Rammig, A., and Wang, Y.: Nitrogen deposition maintains a positive effect on terrestrial carbon sequestration in the 21st century despite growing phosphorus limitation at regional scales, Global Biogeochem. Cy., 33, 810–824, https://doi.org/10.1029/2018GB005952, 2019. a
Fowler, D., Coyle, M., Skiba, U., Sutton, M. A., Cape, J. N., Reis, S., Sheppard, L. J., Jenkins, A., Grizzetti, B., Galloway, J. N., Vitousek, P., Leach, A., Bouwman, A. F., Butterbach-Bahl, K., Dentener, F., Stevenson, D., Amann, M., and Voss, M.: The global nitrogen cycle in the twenty-first century, Philos. T. Roy. Soc. B, 368, 20130164, https://doi.org/10.1098/rstb.2013.0164, 2013. a, b, c
Gedney, N. and Cox, P.: The sensitivity of global climate model simulations to the representation of soil moisture heterogeneity, J Hydrometeorol., 4, 1265–1275, 2003. a
Gerber, S., Hedin, L. O., Oppenheimer, M., Pacala, S. W., and Shevliakova, E.: Nitrogen cycling and feedbacks in a global dynamic land model, Global Biogeochem. Cy., 24, 121–149, 2010. a, b
Global Soil Data Task Group: Global gridded surfaces of selected soil characteristics (IGBP-DIS), Oak Ridge National Laboratory Distributed Active Archive Center, Oak Ridge, Tennessee, USA, http://www.daac.ornl.gov (last access: 15 October 2022), 2000. a, b, c
Global Soil Data Task Group: Global Soil Data Products CD-ROM Contents (IGBP-DIS), Oak Ridge National Laboratory Distributed Active Archive Center [data set], Oak Ridge, Tennessee, USA, https://doi.org/10.3334/ORNLDAAC/565, 2014. a
Goll, D. S., Brovkin, V., Parida, B. R., Reick, C. H., Kattge, J., Reich, P. B., van Bodegom, P. M., and Niinemets, Ü.: Nutrient limitation reduces land carbon uptake in simulations with a model of combined carbon, nitrogen and phosphorus cycling, Biogeosciences, 9, 3547–3569, https://doi.org/10.5194/bg-9-3547-2012, 2012. a, b, c, d, e
Goll, D. S., Vuichard, N., Maignan, F., Jornet-Puig, A., Sardans, J., Violette, A., Peng, S., Sun, Y., Kvakic, M., Guimberteau, M., Guenet, B., Zaehle, S., Penuelas, J., Janssens, I., and Ciais, P.: A representation of the phosphorus cycle for ORCHIDEE (revision 4520), Geosci. Model Dev., 10, 3745–3770, https://doi.org/10.5194/gmd-10-3745-2017, 2017. a, b, c, d, e, f, g, h, i
Hartmann, J. and Moosdorf, N.: The new global lithological map database GLiM: a representation of rock properties at the Earth surface, Geochem. Geophy. Geosy., 13, 1–37, https://doi.org/10.1029/2012GC004370, 2012. a
Hartmann, J., Moosdorf, N., Lauerwald, R., Hinderer, M., and West, A.: Global chemical weathering and associated P-release – The role of lithology, temperature and soil properties, Chem. Geol. 363, 145–163, https://doi.org/10.1016/j.chemgeo.2013.10.025, 2014. a, b, c, d, e, f
Haverd, V., Smith, B., Nieradzik, L., Briggs, P. R., Woodgate, W., Trudinger, C. M., Canadell, J. G., and Cuntz, M.: A new version of the CABLE land surface model (Subversion revision r4601) incorporating land use and land cover change, woody vegetation demography, and a novel optimisation-based approach to plant coordination of photosynthesis, Geosci. Model Dev., 11, 2995–3026, https://doi.org/10.5194/gmd-11-2995-2018, 2018. a
He, X., Augusto, L., Goll, D. S., Ringeval, B., Wang, Y., Helfenstein, J., Huang, Y., Yu, K., Wang, Z., Yang, Y., and Hou, E.: Global patterns and drivers of soil total phosphorus concentration, Earth Syst. Sci. Data, 13, 5831–5846, https://doi.org/10.5194/essd-13-5831-2021, 2021. a, b, c, d, e, f, g, h
Hedley, M. and Stewart, J.: Method to measure microbial phosphate in soils, Soil Biol. Biochem., 14, 377–385, 1982. a
Hungate, B., Dukes, J., Shaw, M, Luo, Y., and Field, C.: Nitrogen and Climate Change, Science (NY), 302, 1512–3, https://doi.org/10.1126/science.1091390, 2003. a
IPCC: Climate Change 2022: Impacts, Adaptation, and Vulnerability. Contribution of Working Group II to the Sixth Assessment Report of the Intergovernmental Panel on Climate Change, edited by: Pörtner, H.-O., Roberts, D. C., Tignor, M., Poloczanska, E. S., Mintenbeck, K., Alegría, A., Craig, M., Langsdorf, S., Löschke, S., Möller, V., Okem, A., Rama, B., Cambridge University Press, https://doi.org/10.1017/9781009325844.001, 2022. a
Jung, M., Koirala, S., Weber, U., Ichii, K., Gans, F., CampsValls, G., Papale, D., Schwalm, C., Tramontana, G., and Reichstein, M.: The FLUXCOM ensemble of global landatmosphere energy fluxes, Scientific Data, 6, 74, https://doi.org/10.1038/s41597-019-0076-8, in press, 2019. a, b
Keller, D. P., Feng, E. Y., and Oschlies, A.: Potential climate engineering effectiveness and side effects during a high carbon dioxide-emission scenario, Nat. Commun., 5, 1–11, https://doi.org/10.1038/ncomms4304, 2014. a
Kvale, K., Prowe, A. E. F., Chien, C. T., Landolfi, A., and Oschlies, A.: Zooplankton grazing of microplastic can accelerate global loss of ocean oxygen, Nat. Commun., 12, 2358, https://doi.org/10.1038/s41467-021-22554-w, 2021. a
Lampitt, R. and Achterberg, E., Anderson, R., Hughes, J., Iglesias, M., Kelly, B., Lucas, M., Popova, E., Sanders, R., Shepherd, J., Smythe, D., and Yool, A.: Ocean fertilization: a potential meansof geoengineering?, Philos. T. R. Soc. A, 366, 3919–3945, https://doi.org/10.1098/rsta.2008.0139, 2008.
Lawrence, D. M., Fisher, R. A., Koven, C. D., Oleson, K. W., Swenson, S. C., Bonan, G., Collier, N., Ghimire, B., Kampenhout, L., Kennedy, D., Kluzek, E., Lawrence, P., Li, F., Li, H., Lombardozzi, D, Riley, W., Sacks, W., Shi, M., Vertenstein, M., and Zeng, X.: The Community Land Model version 5: Description of new features, benchmarking, and impact of forcing uncertainty, J. Adv. Model. Earth Sy., 11, 4245–4287, https://doi.org/10.1029/2018MS001583, 2019. a
Le Quéré, C., Andrew, R. M., Friedlingstein, P., Sitch, S., Hauck, J., Pongratz, J., Pickers, P. A., Korsbakken, J. I., Peters, G. P., Canadell, J. G., Arneth, A., Arora, V. K., Barbero, L., Bastos, A., Bopp, L., Chevallier, F., Chini, L. P., Ciais, P., Doney, S. C., Gkritzalis, T., Goll, D. S., Harris, I., Haverd, V., Hoffman, F. M., Hoppema, M., Houghton, R. A., Hurtt, G., Ilyina, T., Jain, A. K., Johannessen, T., Jones, C. D., Kato, E., Keeling, R. F., Goldewijk, K. K., Landschützer, P., Lefèvre, N., Lienert, S., Liu, Z., Lombardozzi, D., Metzl, N., Munro, D. R., Nabel, J. E. M. S., Nakaoka, S., Neill, C., Olsen, A., Ono, T., Patra, P., Peregon, A., Peters, W., Peylin, P., Pfeil, B., Pierrot, D., Poulter, B., Rehder, G., Resplandy, L., Robertson, E., Rocher, M., Rödenbeck, C., Schuster, U., Schwinger, J., Séférian, R., Skjelvan, I., Steinhoff, T., Sutton, A., Tans, P. P., Tian, H., Tilbrook, B., Tubiello, F. N., van der Laan-Luijkx, I. T., van der Werf, G. R., Viovy, N., Walker, A. P., Wiltshire, A. J., Wright, R., Zaehle, S., and Zheng, B.: Global Carbon Budget 2018, Earth Syst. Sci. Data, 10, 2141–2194, https://doi.org/10.5194/essd-10-2141-2018, 2018. a, b, c
Li, C., Aber, J., Stange, F., Butterbach-Bahl, K., and Papen, H.: A process-oriented model of N2O and NO emissions from forest soils: 1. Model development, J. Geophys. Res.-Atmos., 105, 4369–4384, 2000. a, b, c, d
Li, S., Lü, S., Liu, Y., Gao, Y., and Ao, Y.: Variations and trends of terrestrial NPP and its relation to climate change in the 10 CMIP5 models, J. Earth Syst. Sci., 124, 395–403, https://doi.org/10.1007/s12040-015-0545-1, 2015. a
MacDougall, A. H.: The oceanic origin of path-independent carbon budgets, Sci. Rep.-UK, 7, 10373, https://doi.org/10.1038/s41598-017-10557-x, 2017. a
MacDougall, A. H. and Knutti, R.: Projecting the release of carbon from permafrost soils using a perturbed parameter ensemble modelling approach, Biogeosciences, 13, 2123–2136, https://doi.org/10.5194/bg-13-2123-2016, 2016. a, b, c, d, e
MacDougall, A. H., Avis, C. A., and Weaver, A. J.: Significant contribution to climate warming from the permafrost carbon feedback, Nat. Geosci., 5, 719–721, 2012. a
Machado, C. and Furlani, A.: KINETICS OF PHOSPHORUS UPTAKE AND ROOT MORPHOLOGY OF LOCAL AND IMPROVED VARIETIES OF MAIZE, Sci. Agric. (Piracicaba, Braz.), 61, 69–76, 2004. a
Mackenzie, F., Ver, L. M., and Lerman, A.: Century-scale nitrogen and phosphorus controls of the carbon cycle, Chem. Geol., 190, 13–32, 2002. a, b, c, d
Malhi, Y., Baldocchi, D. D., and Jarvis, P. G.: The carbon balance of tropical, temperate and boreal forests, Plant Cell Environ., 22, 715–740, 1999. a
Martius, C.: Density, humidity, and nitrogen content of dominant wood species of floodplain forests (varzea) in Amazonia, Holz Roh. Werkst., 50, 300–303, 1992. a
Matthews, H. and Caldeira, K.: Stabilizing climate requires near-zero emissions, Geophys. Res. Lett., 35, L04705, https://doi.org/10.1029/2007GL032388, 2008. a
Matthews, H. and Weaver, A.: Committed climate warming, Nat. Geosci., 3, 142–143, https://doi.org/10.1038/ngeo813, 2010. a
Matthews, H., Gillett, N., Stott, P., and Zickfeld, K.: The proportionality of global warming to cumulative carbon emissions, Nature, 459, 829–832, https://doi.org/10.1038/nature08047, 2009. a
McGill, W. and Cole, C.: Comparative aspects of cycling of organic C, N, S, and P through soil organic matter, Geoderma, 26, 267–286, 1981. a
Meissner, K. J., Weaver, A. J., Matthews, H. D., and Cox, P. M.: The role of land surface dynamics in glacial inception: a study with the UVic Earth System Model, Clim. Dynam., 21, 515–537, https://doi.org/10.1007/s00382-003-0352-2, 2003. a
Meissner, K. J., McNeil, B. I., Eby, M., and Wiebe, E. C.: The importance of the terrestrial weathering feedback for multi-millennial coral reef habitat recovery, Global Biogeochem. Cy., 26, 1–20, https://doi.org/10.1029/2011GB004098, 2012. a
Menge D., Hedin, L., and Pacala S.: Nitrogen and Phosphorus Limitation over Long-Term Ecosystem Development in Terrestrial Ecosystems, PLOS ONE, 7, e42045, https://doi.org/10.1371/journal.pone.0042045, 2012. a
Mengis, N., Partanen, A. I., Jalbert, J., and Matthews, H. D.: 1.5 ∘C carbon budget dependent on carbon cycle uncertainty and future non-CO2 forcing, Sci. Rep.-UK, 8, 5831, https://doi.org/10.1038/s41598-018-24241-1, 2018. a
Mengis, N., Keller, D. P., MacDougall, A. H., Eby, M., Wright, N., Meissner, K. J., Oschlies, A., Schmittner, A., MacIsaac, A. J., Matthews, H. D., and Zickfeld, K.: Evaluation of the University of Victoria Earth System Climate Model version 2.10 (UVic ESCM 2.10), Geosci. Model Dev., 13, 4183–4204, https://doi.org/10.5194/gmd-13-4183-2020, 2020. a, b, c, d, e, f, g, h, i, j, k
Montenegro, A., Brovkin, V., Eby, M., Archer, D., and Weaver, A. J.: Long term fate of anthropogenic carbon, Geophys. Res. Lett., 34, L19707, https://doi.org/10.1029/2007GL030905, 2007. a
Myhre, G., Stocker, F., Qin, D., Plattner, G, Tignor,M., Allen, S., Boschung, J., Nauels, A., Xia, Y., Bex, V., and Midgley, P. (Eds.): Anthropogenic and natural radiative forcing. Working Group I Contribution to the Intergovernmental Panel on Climate Change Fifth Assessment Report Climate Change 2013: The Physical Science Basis, Cambridge University Press, ISBN 9781107057991, 2013. a
Nakhavali, M. A., Mercado, L. M., Hartley, I. P., Sitch, S., Cunha, F. V., di Ponzio, R., Lugli, L. F., Quesada, C. A., Andersen, K. M., Chadburn, S. E., Wiltshire, A. J., Clark, D. B., Ribeiro, G., Siebert, L., Moraes, A. C. M., Schmeisk Rosa, J., Assis, R., and Camargo, J. L.: Representation of the phosphorus cycle in the Joint UK Land Environment Simulator (vn5.5_JULES-CNP) , Geosci. Model Dev., 15, 5241–5269, https://doi.org/10.5194/gmd-15-5241-2022, 2022. a, b, c, d, e
Nzotungicimpaye, C.-M., Zickfeld, K., MacDougall, A. H., Melton, J. R., Treat, C. C., Eby, M., and Lesack, L. F. W.: WETMETH 1.0: a new wetland methane model for implementation in Earth system models, Geosci. Model Dev., 14, 6215–6240, https://doi.org/10.5194/gmd-14-6215-2021, 2021. a
Olander, L. and Vitousek, P.: Regulation of soil phosphatase and chitinase activity by N and P availability, Biogeochemistry, 49, 175–190, 2000. a
Pacanowski, R. C.: MOM 2 Documentation, users guide and reference manual, GFDL Ocean Group Technical Report 3, Geophys, Fluid Dyn. Lab., Princet. Univ., Princeton, NJ, 1995. a
Pahlow, M., Chien, C.-T., Arteaga, L. A., and Oschlies, A.: Optimality-based non-Redfield plankton–ecosystem model (OPEM v1.1) in UVic-ESCM 2.9 – Part 1: Implementation and model behaviour, Geosci. Model Dev., 13, 4663–4690, https://doi.org/10.5194/gmd-13-4663-2020, 2020. a
Poulter, B., MacBean, N., Hartley, A., Khlystova, I., Arino, O., Betts, R., Bontemps, S., Boettcher, M., Brockmann, C., Defourny, P., Hagemann, S., Herold, M., Kirches, G., Lamarche, C., Lederer, D., Ottlé, C., Peters, M., and Peylin, P.: Plant functional type classification for earth system models: results from the European Space Agency's Land Cover Climate Change Initiative, Geosci. Model Dev., 8, 2315–2328, https://doi.org/10.5194/gmd-8-2315-2015, 2015. a, b, c
Reed, S. C., Townsend, A. R., Davidson, E. A., and Cleveland, C.: Stoichiometric patterns in foliar nutrient resorption across multiple scales, New Phytol., 196, 173–180, https://doi.org/10.1111/j.1469-8137.2012.04249.x, 2012. a
Reich, P. B. and Oleksyn, J.: Global patterns of plant leaf N and P in relation to temperature and latitude, P. Natl Acad. Sci. USA, 101, 11001–11006, https://doi.org/10.1073/pnas.0403588101, 2004. a, b
Ryan, P. R., Dessaux, Y., Thomashow, L. S., and Weller, D. M.: Rhizosphere engineering and management for sustainable agriculture, Plant Soil, 321, 363–383, https://doi.org/10.1007/s11104-009-0001-6, 2009. a
Schlesinger, W. H.: Biogeochemistry: An Analysis of Global Change, Academic Press, San Diego, USA, 588 pp., ISBN-10 0-12-625155-X, 1997. a
Shi, M., Fisher, J. B., Brzostek, E. R., and Phillips, R. P.: Carbon cost of plant nitrogen acquisition: global carbon cycle impact from an improved plant nitrogen cycle in the Community Land Model, Glob. Change Biol., 22, 1299–1314, 2016. a
Smil, V.: Phosphorus in the environment: natural flows and human interferences, Annu. Rev. Energ. Env., 25, 53–88, 2000. a, b, c, d, e
Spafford, L. and MacDougall, A. H.: Validation of terrestrial biogeochemistry in CMIP6 Earth system models: a review, Geosci. Model Dev., 14, 5863–5889, https://doi.org/10.5194/gmd-14-5863-2021, 2021. a, b
Thornton, P., Lamarque, J., Rosenbloom, N., and Mahowald, N.: Influence of carbon-nitrogen cycle coupling on land model response to CO2 fertilization and climate variability, Global Biogeochem. Cy., 21, GB4018, https://doi.org/10.1029/2006GB002868, 2007. a
Thum, T., Caldararu, S., Engel, J., Kern, M., Pallandt, M., Schnur, R., Yu, L., and Zaehle, S.: A new model of the coupled carbon, nitrogen, and phosphorus cycles in the terrestrial biosphere (QUINCY v1.0; revision 1996), Geosci. Model Dev., 12, 4781–4802, https://doi.org/10.5194/gmd-12-4781-2019, 2019. a
Tian, H., Xu, R., Canadell, J. G., Thompson, R. L., Winiwarter, W., Suntharalingam, P., Davidson, E. A., Ciais, P., Jackson, R. B., Janssens-Maenhout, G., Prather, M. J., Regnier, P., Pan, N., Pan, S., Peters, G. P., Shi, H., Tubiello, F. N., Zaehle, S., Zhou, F., Arneth, A., Battaglia, G., Berthet, S., Bopp, L., Bouwman, A. F., Buitenhuis, E. T., Chang, J., Chipperfield, M. P., Dangal, S. R. S., Dlugokencky, E., Elkins, J. W., Eyre, B. D., Fu, B., Hall, B., Ito, A., Joos, F., Krummel, P. B., Landolfi, A., Laruelle, G. G., Lauerwald, R., Li, W., Lienert, S., Maavara, T., MacLeod, M., Millet, D. B., Olin, S., Patra, P. K., Prinn, R. G., Raymond, P. A., Ruiz, D. J., van der Werf, G. R., Vuichard, N., Wang, J., Weiss, R. F., Wells, K. C., Wilson, C., Yang, J., and Yao, Y.: A comprehensive quantification of global nitrous oxide sources and sinks, Nature, 586, 248–256, https://doi.org/10.1038/s41586-020-2780-0, 2020. a, b
Tokarska, K. B., Zickfeld, K., and Rogelj, J.: Path independence of carbon budgets when meeting a stringent global mean temperature target after an overshoot, Earths Future, 7, 1283–1295, https://doi.org/10.1029/2019EF001312, 2019. a
Van Oijen, M., Barcza, Z., Confalonieri, R., Korhonen, P., Kroel-Dulay, G., Lellei-Kovács, E., Louarn, G., Louault, F., Martin, R., Moulin, T., Movedi, E., Picon-Cochard, C., Rolinski, S., Viovy, N., Wirth, S., and Bellocchi, G.: Incorporating Biodiversity into Biogeochemistry Models to Improve Prediction of Ecosystem Services in Temperate Grasslands: Review and Roadmap, Agronomy, 10, 259, https://doi.org/10.3390/agronomy10020259, 2020. a
Vitousek, P. M., Porder, S., Houlton, B. Z., and Chadwick, O. A.: Terrestrial phosphorus limitation: mechanisms, implications, and nitrogen–phosphorus interactions, Ecol. Appl., 20, 5–15, https://doi.org/10.1890/08-0127.1, 2010. a, b
Wagg, C., Bender, S., Widmer, F., and Van der Heijden, M.: Soil biodiversity and soil community composition determine ecosystem multifunctionality, P. Natl. Acad. Sci. USA, 111, 5266–5270, https://doi.org/10.1073/pnas.1320054111, 2014. a
Walker, A., Beckerman, A., Gu, L., Kattge, J., Cernusak, L., Domingues, T., Scales, J., Wohlfahrt, G., Wullschleger, S., and Woodward, I.: The relationship of leaf photosynthetic traits – Vcmax and Jmax – to leaf nitrogen, leaf phosphorus, and specific leaf area: A meta-analysis and modeling study, Ecol. Evol., 4, 3218–3235, 2014. a
Walker, T. and Syers, J.: The fate of phosphorus during pedogenesis, Geoderma, 15, 1–19, https://doi.org/10.1016/0016-7061(76)90066-5, 1976. a
Wang, Y., Houlton, B., and Field, C.: A model of biogeochemical cycles of carbon, nitrogen, and phosphorus including symbiotic nitrogen fixation and phosphatase production, Global Biogeochem. Cy., 21, 3218–3235, 2007. a, b, c, d, e, f, g, h, i, j, k, l, m
Wang, Y. P., Law, R. M., and Pak, B.: A global model of carbon, nitrogen and phosphorus cycles for the terrestrial biosphere, Biogeosciences, 7, 2261–2282, https://doi.org/10.5194/bg-7-2261-2010, 2010. a, b, c, d, e, f, g, h, i, j, k, l, m, n, o, p, q, r, s, t, u, v, w, x, y
Wang, Y., Ciais, P., Goll, D., Huang, Y., Luo, Y., Wang, Y.-P., Bloom, A. A., Broquet, G., Hartmann, J., Peng, S., Penuelas, J., Piao, S., Sardans, J., Stocker, B. D., Wang, R., Zaehle, S., and Zechmeister-Boltenstern, S.: GOLUM-CNP v1.0: a data-driven modeling of carbon, nitrogen and phosphorus cycles in major terrestrial biomes, Geosci. Model Dev., 11, 3903–3928, https://doi.org/10.5194/gmd-11-3903-2018, 2018. a, b, c, d, e
Wang, Z., Tian, H., Yang, J., Shi, H., Pan, S., Yao, Y., Banger, K., and Yang, Q.: Coupling of phosphorus processes with carbon and nitrogen cycles in the dynamic land ecosystem model: Model structure, parameterization, and evaluation in tropical forests, J. Adv. Model. Earth Sy., 12, e2020MS002123, https://doi.org/10.1029/2020MS002123, 2020. a, b, c
Wania, R., Meissner, K. J., Eby, M., Arora, V. K., Ross, I., and Weaver, A. J.: Carbon-nitrogen feedbacks in the UVic ESCM, Geosci. Model Dev., 5, 1137–1160, https://doi.org/10.5194/gmd-5-1137-2012, 2012. a, b, c, d, e, f, g, h, i, j, k, l, m, n, o, p, q, r, s, t, u, v, w, x, y, z, aa
Weaver, A. J., Eby, M., Wiebe, E. C., Bitz, C. M., Duffy, P. B., Ewen, T. L., Fanning, A. F., Holland, M. M., MacFadyen, A., Matthews, H. D., Meissner, K. J., Saenko, O., Schmittner, A., Wang, H. X., and Yoshimori, M.: The UVic Earth System Climate Model: Model description, climatology, and applications to past, present and future climates, Atmos. Ocean, 39, 361–428, 2001. a
Weber, S.: The utility of Earth system Models of Intermediate Complexity (EMICs), WIREs Clim. Change, 1, 243–252, https://doi.org/10.1002/wcc.24, 2010. a
Wieder, W., Cleveland, C., Smith, W., and Todd, K.: Future productivity and carbon storage limited by terrestrial nutrient availability, Nat. Geosci., 8, 441–444, https://doi.org/10.1038/ngeo2413, 2015. a
Xu-Ri and Prentice, I. C.: Terrestrial nitrogen cycle simulation with a dynamic global vegetation model, Glob. Change Biol., 14, 1745–1764, 2008. a
Yang, G., Wagg, C., Veresoglou, S. D., Hempel, S., and Rillig, M. C.: How soil biota drive ecosystem stability, Trends Plant Sci., 23, 1057–1067, https://doi.org/10.1016/j.tplants.2018.09.007, 2018. a
Yang, X., Post, W. M., Thornton, P. E., and Jain, A.: The distribution of soil phosphorus for global biogeochemical modeling, Biogeosciences, 10, 2525–2537, https://doi.org/10.5194/bg-10-2525-2013, 2013. a, b, c, d, e
Yang, X., Ricciuto, D. M., Thornton, P. E., Shi, X., Xu, M., Hoffman, F., and Norby, R. J.: The effects of phosphorus cycle dynamics on carbon sources and sinks in the Amazon region: a modeling study using ELM v1, J. Geophys. Res.-Biogeo., 124, 3686–3698, https://doi.org/10.1029/2019JG005082, 2019. a
Yang, X. J., Wittig, V., Jain, A. K., and Post, W.: Integration of nitrogen cycle dynamics into the Integrated Science Assessment Model for the study of terrestrial ecosystem responses to global change, Global Biogeochem. Cy., 23, 121–149, 2009. a, b, c
Zaehle, S., Friend, A. D., Friedlingstein, P., Dentener, F., Peylin, P., and Schulz, M.: Carbon and nitrogen cycle dynamics in the OCN land surface model: 2. Role of the nitrogen cycle in the historical terrestrial carbon balance, Global Biogeochem. Cy., 24, GB1006, https://doi.org/10.1029/2009GB003522, 2010. a, b, c, d
Zaehle, S., Medlyn, B. E., de Kauwe, M. G., Walker, A. P., Dietze, M. C., Hickler, T., Luo, Y., Wang, Y. P., El-Masri, B., Thornton, P., Jain, A., Wang, S., Warlind, D., Weng, E., Parton, W., Iversen, C. M., Gallet-Budynek, A., Mccarthy, H., Finzi,A.,Hanson, P., Colin I., Oren R., and Norby, R.: Evaluation of 11 terrestrial carbon-nitrogen cycle models against observations from two temperate Free-Air CO2 Enrichment studies, New Phytol., 202, 803–822, https://doi.org/10.1111/nph.12697, 2014. a
Zickfeld, K., Eby, M., Matthews, H. D., and Weaver, A. J.: Setting cumulative emissions targets to reduce the risk of dangerous climate change, P. Natl. Acad. Sci. USA, 106, 16129–16134, https://doi.org/10.1073/pnas.0805800106, 2009. a
Ziehn, T., Chamberlain, M., Law, R., Lenton, A., Bodman, R., Dix, M., Stevens L., Wang, Y., and Srbinovsky, J.: The Australian Earth System Model: ACCESS-ESM1.5, Journal of Southern Hemisphere Earth Systems Science, 70, 193–214, 2020. a