the Creative Commons Attribution 4.0 License.
the Creative Commons Attribution 4.0 License.
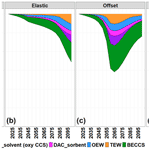
GCAM-CDR v1.0: enhancing the representation of carbon dioxide removal technologies and policies in an integrated assessment model
Raphael Apeaning
Garrett Guard
This paper introduces GCAM-CDR 1.0, an integrated assessment model for climate policy based on the open-source Global Change Analysis Model (GCAM). GCAM-CDR extends GCAM v5.4 by enabling users to model additional carbon dioxide removal (CDR) technologies and additional policies and controls related to CDR. New CDR technologies include terrestrial enhanced weathering with basalt, ocean liming, and additional versions of direct air capture. New CDR policies and controls include integration of bioenergy with carbon capture and storage (BECCS) into the CDR market, interregional trade in CDR, exogenous control over the rate of growth of CDR, the ability to set independent targets for emissions abatement and CDR, and a variety of mechanisms for setting demand for CDR at the regional and/or global level. These extensions enhance users' ability to study the potential roles of CDR in climate policy.
- Article
(1691 KB) - Full-text XML
-
Supplement
(1251 KB) - BibTeX
- EndNote
Carbon dioxide removal (CDR) involves capturing carbon dioxide (CO2) from the atmosphere or ocean and storing or sequestering it for decades, centuries, or millennia. There are many different approaches to CDR, ranging from forest restoration and regenerative agriculture to direct air capture (DAC), bioenergy with carbon capture and storage (BECCS), and enhanced weathering (Fuss et al., 2018; National Academies of Science, Engineering, and Medicine, 2018). CDR has long played an important role in modeled climate mitigation pathways (Fuss et al., 2014), but until recently, most integrated assessment models (IAMs) have only included a few approaches to CDR and offered limited flexibility with respect to policies related to CDR.
This paper describes GCAM-CDR 1.0, a variant of the Global Change Analysis Model (GCAM), whose purpose is to offer a wider variety of CDR technologies, policies, and controls. GCAM is an IAM developed by the Joint Global Change Resource Institute (JGCRI), which is a partnership between the University of Maryland and Pacific Northwest National Laboratory (PNNL) in the United States. GCAM-CDR was developed by the Institute for Carbon Removal Law and Policy (ICRLP) at American University.
Section 2 describes GCAM-CDR. Section 3 presents some results from scenarios designed to compare the behavior of GCAM-CDR with GCAM 5.4 and demonstrate some of the new capabilities of GCAM-CDR. Section 4 offers some discussion of those results and the use of GCAM-CDR for studying the roles that CDR might play in climate policy.
2.1 Overview of GCAM 5
GCAM-CDR 1.0 is based on GCAM 5.4 (Bond-Lamberty et al., 2021). Calvin et al. (2019) describe GCAM 5.1 in detail. Here we provide a brief overview of GCAM that covers essential context for understanding GCAM-CDR and important updates since version 5.1.
GCAM is a recursive–dynamic, partial-equilibrium IAM. It is used to study long-term climate change scenarios and policies. The model contains five interconnected modules: climate, energy, water, land (including agriculture), and socioeconomics. The energy and socioeconomics modules represent 32 distinct geopolitical regions, while the water and land modules represent 384 land–water basins.
GCAM operates by seeking a set of market-clearing prices in each period for goods and services in the energy, land, and water modules, given exogenously specified socioeconomic inputs and policy constraints. This process is myopic rather than intertemporally optimizing: in each time step, the model uses only information available at that time step; it does not use information about future supply, demand, consumption, or policy. The end result is a vector of supply, demand, and price projections for each good and service for each period in each region of the model, as well as projections of emissions and global climate variables.
Users can simulate climate policies in GCAM by imposing exogenously specified prices on CO2 emissions and/or non-CO2 greenhouse gas (GHG) emissions, quantity-based constraints on those emissions, subsidies, taxes, renewable energy portfolio standards, and similar policies. Users can also use GCAM to identify a series of emissions taxes that achieves an exogenously specified climate target, such as limiting radiative forcing in 2100 to 1.9 W m−2.
GCAM has represented CDR technologies for over a decade. It has included bioenergy with carbon capture and storage (BECCS) technologies since at least version 3 (Luckow et al., 2010). BECCS has played an important role in deep decarbonization scenarios in GCAM, as in other IAMs (Fuss et al., 2014; Calvin et al., 2019; Köberle, 2019; Riahi et al., 2021). More recently, GCAM 5.4 has added direct air capture (DAC) technologies (Fuhrman et al., 2020, 2021; Bond-Lamberty et al., 2021). As discussed in Sect. 2.4, the options for modeling CDR policy in GCAM 5.4 remain somewhat limited. GCAM's default behavior is to drive the deployment of both BECCS and, when included, DAC by paying BECCS and DAC technologies at the price of carbon for each ton of carbon they remove.
2.2 Overview of GCAM-CDR
GCAM-CDR differs from GCAM in two main ways. First, it includes several new CDR technologies, bundled together in a CDR sector, all of which is achieved by adding XML input files. Second, it offers users a wider variety of policy options for shaping CDR markets and driving demand for CDR, which requires both new XML input files and changes to the source code. These changes are described in more detail in Sect. 2.3 and 2.4, respectively.
In addition to these new features, the other main change is that GCAM-CDR slightly reorganizes parts of the energy system to more cleanly separate biofuels from fossil fuels. The purpose of this “bioseparation” is to enable better control over BECCS. GCAM 5.4 represents liquid and gaseous biofuels as becoming intermingled with fossil-based liquids and gas upstream in the energy sector. This allows for an elegant system of accounting for carbon flows and associated pricing (Kyle et al., 2011), but it creates challenges for tracking and managing BECCS. For example, the system allows gas-fired power plants with carbon capture and storage (CCS) to transition gradually from low-emission technologies to negative-emission technologies by switching from fossil gas to biogas, but the amount of CDR achieved by such power plants is opaque to other parts of the model at runtime and therefore hard to control via policies that do not treat CDR and CO2 emissions symmetrically. The bioseparation input files included with GCAM-CDR keep bioliquids and biogas separate from their fossil-based counterparts upstream from potential BECCS applications but allows them to intermingle as usual in downstream sectors, such as transportation, where CCS is not available. This creates some deviations from GCAM 5.4's behavior, partly because of differences in the way GCAM parameterizes competition between technologies at different points in the energy system and partly because the bioseparation entails that biofuel-fired power plants and fossil-fuel-fired power plants are distinct technologies rather than technologies that can transition from one fuel to the other over time. The reorganization of the energy system is described in more detail in the model documentation, and the deviations introduced by the bioseparation files are quantified in Sect. 3.
Some minor changes to the source code, such as enabling regional tracking of CO2 emissions independently of a carbon price, were also necessary to implement new policies and technologies. All changes to the source code are entirely backward compatible with GCAM 5.4: any input files that work with GCAM 5.4 will work with GCAM-CDR and will produce virtually indistinguishable results in the two models, as documented in Sect. 3. Changes to the source code are described in the model documentation and visible in the commit history of the code repository on GitHub, which was initially populated with the source code for GCAM 5.4.
2.3 New CDR sector and technologies
GCAM-CDR 1.0 introduces several technologies whose primary purpose is to permanently remove CO2 from the atmosphere. These technologies, which are grouped into a CDR supply sector, include two types of DAC, a terrestrial enhanced weathering (TEW) technology that involves spreading basalt on cropland, and ocean liming as an approach to ocean alkalinization or ocean enhanced weathering (OEW). The DAC technologies in GCAM-CDR resemble but are not identical to those in GCAM 5.4. Figure 1 depicts schematic representations of the default primary CDR technologies included in GCAM-CDR 1.0. Each technology produces an abstract good called CDR as an output, which can be interpreted as a certificate verifying the permanent removal of a unit of CO2 from the atmosphere. The following subsections describe each technology in more detail, with key parameters given in the Supplement.
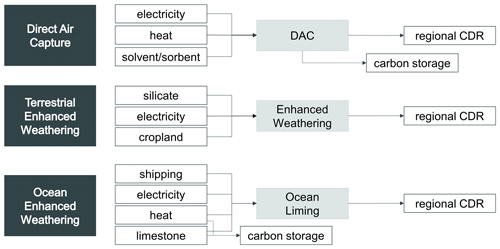
Figure 1Schematic diagrams of new CDR technologies in GCAM-CDR 1.0. Direct air capture (DAC) technologies take electricity, heat, and either a solvent or sorbent as inputs, send CO2 to geological storage, and produce an abstract good called CDR as an output. Terrestrial enhanced weathering requires silicate (basalt), electricity, and “cropland” as inputs and produces CDR as an output. Ocean liming requires limestone, electricity, heat, and international shipping as inputs, sends process emissions to carbon storage, and produces CDR as an output. For more detailed diagrams related to each technology, see the Supplement.
These represent a limited selection of the rapidly growing variety of technologies for accomplishing DAC, OEW, or TEW. For example, liquid-solvent DAC could be fired by hydrogen rather than natural gas, solid-sorbent DAC could be heated with industrial heat pumps rather than waste heat, and still other DAC technologies operate at room temperature with electricity as the primary energy input. Other proposals exist for adding alkalinity to the ocean and weathering rocks on land. The goal here is not to capture the full variety of CDR technologies but rather to enable users to explore the way that a portfolio of CDR options and CDR policies affect long-term climate scenarios. Users who are interested in modeling a greater variety of CDR technologies can adapt the existing XML inputs to add other technologies, and investigating such technologies promises to be a fruitful avenue of research.
To ensure that these new CDR technologies grow gradually, GCAM-CDR offers two main ways for users to constrain growth. The first is for users to specify demand for CDR in ways that grow gradually. The second is a flexible adaptation of the approach used to constrain growth in DAC in GCAM 5.4. In the latter approach, CDR technologies compete with a dummy technology that neither consumes energy nor removes CO2. The total output from “real” CDR technologies is limited to a certain quantity in each period, set by the user, and the model adjusts the prices in the CDR sector to ensure that the dummy technology captures any excess CDR demand (see the Supplement for more details). Users can easily customize or eliminate this constraint on the growth of CDR technologies. Users can also choose whether to subject BECCS to the growth constraint or allow it to grow and operate independently of the new CDR technologies.
2.3.1 High-heat, liquid-solvent-based DAC
GCAM-CDR represents a high-heat, liquid-solvent-based approach to DAC, similar to the one developed by the Canadian company Carbon Engineering (Keith et al., 2018; National Academies of Science, Engineering, and Medicine, 2018). In our implementation, this technology takes natural gas and electricity as its main inputs and sends captured CO2 to regional carbon storage markets, which allocate it to onshore or offshore geological storage. We assume an oxy-fired calciner that simultaneously captures atmospheric CO2 and the emissions from the natural gas, sequestering both together.
This technology very closely resembles one of the DAC technologies introduced in GCAM 5.4, which is described by Fuhrman et al. (2020). Some parameters are based on work by Realmonte et al. (2019). Note that GCAM 5.4 also includes an all-electric, high-heat DAC technology, which GCAM-CDR does not.
2.3.2 Low-heat, solid-sorbent-based DAC
GCAM-CDR represents a low-heat, solid-sorbent-based approach to DAC, similar to the one developed by the Swiss company Climeworks (Wurzbacher et al., 2016; National Academies of Science, Engineering, and Medicine, 2018; McQueen et al., 2020). In our implementation, this technology takes waste heat and electricity as its main inputs and sends captured CO2 to the carbon storage market, which allocates it to onshore or offshore geological storage. The waste heat is modeled endogenously as a byproduct of thermal power plants and industrial energy use.
This technology loosely resembles one of the other DAC technologies introduced in GCAM 5.4 but differs in that the GCAM-CDR version uses waste heat from other processes, whereas the GCAM 5.4 version uses electric heat pumps. Some parameters are based on work by Realmonte et al. (2019).
2.3.3 Terrestrial enhanced weathering with basalt
GCAM-CDR represents an approach to terrestrial enhanced weathering that involves crushing basalt and spreading it on cropland, similar to the approaches described in several recent studies (Strefler et al., 2018; Beerling et al., 2020; Kelland et al., 2020). The default implementation of this technology takes basalt and electricity as inputs as well as an abstract input called “cropland.” The cropland input represents the limited supply of agricultural land on which to spread basalt, and it exhibits an upward-sloping supply curve to represent the increasing marginal cost of applying basalt to increasingly distant or hard-to-access areas. The CO2 captured through TEW is assumed to be stored mainly in bicarbonate and carbonate minerals, but these are not explicitly modeled; removals are modeled simply as negative CO2 emissions.
GCAM-CDR also includes an alternative, more experimental implementation that models basalt not as a standalone CDR technology, but as a type of fertilizer, based on studies suggesting that crushed basalt can increase agricultural yields (Nunes et al., 2014; Edwards et al., 2017; Beerling et al., 2020; Kelland et al., 2020). In this implementation, terrestrial enhanced weathering is excluded from the CDR sector and moved instead to the fertilizer sector. It operates as a partial substitute for nitrogen fertilizer, resulting in a mix of nitrogen fertilizer and basalt being applied to croplands. The modeled rate of application follows the experiment described by Kelland et al. (2020), in which yield increases from basalt application approximately match the boost provided by nitrogen fertilizer in GCAM. In this implementation, limits on terrestrial enhanced weathering arise from the endogenous limits on fertilizer demand, which are based on the area of land under cultivation, demand for food, etc. Further constraints arise from exogenously specified limits on the share of fertilizer demand that can be satisfied by basalt application.
The default implementation of enhanced weathering as a standalone CDR technology ignores the fertilization effects of basalt because the magnitude of that effect is still relatively unknown, having been documented only in a few case studies. We include the alternative implementation anyway to help users explore the agricultural and carbon implications of using basalt as a substitute for synthetic fertilizer, while acknowledging the large uncertainties around the fertilization effect.
There are various other approaches to terrestrial enhanced weathering that are not included in GCAM-CDR 1.0. For instance, some researchers have proposed using alkaline industrial waste (Renforth, 2019) or more reactive minerals, such as dunite or olivine (Strefler et al., 2018). Others have proposed spreading crushed minerals in forests or unmanaged lands rather than or in addition to croplands (Goll et al., 2021).
2.3.4 Ocean alkalinization with lime
GCAM-CDR includes ocean liming as an approach to ocean alkalinization or ocean enhanced weathering, similar to approaches described by Renforth et al. (2013) and Caserini et al. (2021). Ocean liming involves depositing lime into the open ocean, where it begins a short cascade of inorganic chemical reactions that lead to the ocean storing additional carbon as bicarbonates, allowing the ocean to absorb more CO2 from the atmosphere while counteracting ocean acidification. Because of the modularity of the alkalinity calculations in GCAM's climate module, Hector, the beneficial effects of ocean liming on ocean alkalinity are not explicitly modeled in GCAM-CDR 1.0.
In GCAM-CDR, ocean liming uses natural gas, limestone, electricity, and an abstract good produced as a byproduct of international shipping. We assume an oxy-flash calcination process, as described by Renforth et al. (2013), that captures and sequesters the process emissions from both natural gas and limestone during calcination.
The use of the international shipping byproduct serves both to endogenize the cost of depositing the lime in the ocean while allowing GCAM-CDR to model low-cost opportunities to deposit lime from ships during ballast legs. It also provides an endogenous upper limit to the amount of ocean liming that is feasible. Following Caserini et al. (2021), we assume that lime would be spread from cargo ships, and that ocean liming cannot use more than 13 % of global shipping capacity, although total capacity does increase endogenously in response to demand from the ocean liming industry. Users could easily modify GCAM-CDR to allow for purpose-built OEW fleets to overcome this limitation. See the Supplement for details.
There are various other approaches to ocean alkalinization that are not included in GCAM-CDR 1.0. For example, some researchers have proposed dumping silicate minerals into the ocean (Köhler et al., 2013); spreading crushed silicates on beaches or coastal seabeds (Meysman and Montserrat, 2017); exposing carbonate minerals to seawater in an artificial, high-CO2 environment (Xing et al., 2022); releasing carbonate and bicarbonate produced as a by-product of hydrogen production (Rau et al., 2018); or electrochemically separating seawater and pumping acidity into deep water (Tyka et al., 2022).
2.4 CDR policy options
Although it is not normally described this way, GCAM 5.4 implicitly implements a very specific CDR policy: any technology that removes a ton of carbon from the atmosphere receives a subsidy equal to the carbon price, which has to be repaid if and only if the carbon is re-emitted downstream (earlier versions of GCAM work similarly). GCAM models raw biofuels (e.g., corn for ethanol or wood pellets) as removing carbon from the atmosphere, thus earning a subsidy (the carbon implications of growing and harvesting the biomass are handled separately by GCAM's land-use module). If those biofuels are consumed without CCS, the carbon is re-emitted and the subsidy is repaid, but technologies that capture and sequester the carbon in those biofuels effectively “keep” the original subsidy for themselves. Thus, BECCS technologies end up receiving a subsidy, which is equal to the carbon price, for each ton of (biologically derived) carbon they sequester. Furthermore, in the default set-up for GCAM 5.4, it is implicitly assumed that this subsidy comes out of revenues from a carbon price and that the total available subsidy for CDR can only exceed revenues from the carbon price by a fixed amount, referred to as the “negative emissions budget”. CDR services can be traded between regions if and only if the regions are subject to a shared greenhouse gas constraint policy, such as a quantitative limit on global CO2 emissions, in which case negative emissions in one region can offset emissions in another region.
In the real world, each part of this system would have to result from specific choices in the design of carbon pricing and climate policy. Yet in GCAM, the model behavior can seem like a natural consequence of pricing GHG emissions rather than a specific policy that connects demand for CDR to a price on emissions in a particular way. This is especially easy to overlook because much of this behavior is either set in the source code or emerges from more general carbon accounting mechanisms in GCAM rather than any policy explicitly represented in the model inputs.
GCAM 5.4 adds the ability to exogenously specify maximum demand for DAC, with actual demand responding endogenously to the carbon price. When users model CO2 or GHG constraints or certain kinds of climate policy targets, however, GCAM 5.4 implicitly models any demand for DAC as an offset for other emissions: setting DAC to remove n metric tons of CO2 from the atmosphere permits an additional (n−k) tons of GHG emissions, measured in CO2 eq., where k equals gross emissions from the energy inputs to DAC. This builds in the kind of “moral hazard” or “mitigation deterrence” that is frequently raised as a concern in the modeling or deployment of CDR (Anderson and Peters, 2016; McLaren, 2020). A further problem is that, in GCAM 5.4, demand for DAC and that for BECCS are quasi-independent of each other; for technical reasons inside the model, DAC and BECCS technologies cannot compete directly with one another to satisfy overall demand for CDR (but see Sect. 2.4.2 on indirect competition).
GCAM-CDR offers a much wider range of CDR policy options, including the ability for users to mimic the approaches used in GCAM 5.4. We describe those options below.
2.4.1 Paying for CDR by different mechanisms
In GCAM 5.4, negative emissions receive a subsidy equivalent to the carbon tax, as explained above. For example, if technologies face a tax of USD 100 t−1 on carbon emissions, a technology that removes a ton of carbon from the atmosphere will receive a subsidy of USD 100. Since carbon prices in GCAM can easily reach hundreds or even thousands of dollars – well above the projected long-run costs of CDR – this implies that the CDR industry is modeled as enjoying large economic rents (meaning, roughly, profits in excess of what is required to make the industry economically viable in a market system).
While GCAM-CDR can mimic that behavior, it also offers an alternative at which CDR technologies are paid at market rates, mirroring the way that prices of other goods and services in GCAM are modeled. This mechanism pays each technology based on the price of the CDR sector, which is determined by the weighted average cost of its constituent technologies, thereby eliminating the large economic rents implied by the carbon-price-based approach (optionally, this alternative mechanism can apply to BECCS technologies as well as the new CDR technologies, in which case they receive a market-based payment for CDR services that is just enough to induce them to supply the amount of CDR demanded of them at that price. See Sect. 2.4.2). Including this option allows users to model situations in which market competition for CDR services or successful cost discovery by policymakers drives prices down to eliminate economic rents. This is particularly important because the high rents are partially responsible for the scale and rapidity of BECCS adoption in deep mitigation scenarios in GCAM.
Users can also apply the full suite of other price manipulation mechanisms in GCAM (e.g., fixed taxes, subsidies, portfolio standards) to CDR technologies in GCAM-CDR.
2.4.2 Integrating BECCS into the CDR market
In GCAM 5.4, BECCS and DAC do not directly compete to satisfy demand for CDR. GCAM contains several bioenergy and BECCS technologies in different parts of the energy system, mainly in the electricity sector and the refining sector. In GCAM 5.4, the amount of carbon removed via BECCS is determined by the amount of energy demanded from each of the various BECCS technologies. That, in turn, depends on the cost of those technologies relative to their competitors, after accounting for the value of any taxes and subsidies to the various technologies, including the carbon-price-based subsidies that BECCS technologies enjoy because they do not re-emit captured CO2 to the atmosphere. This means that in GCAM 5.4, the amount of CDR from BECCS and that from DAC are determined somewhat independently from each other. DAC deployment affects the amount of BECCS only insofar as it changes the carbon price. This interaction occurs because changes to the carbon price change the after-tax prices of BECCS technologies and their competitors. Conversely, the amount of BECCS has a similarly indirect effect on the amount of DAC deployed: deploying BECCS can reduce the carbon price, and in general, deployment of DAC is an increasing function of the carbon price, bounded at the top by the exogenously specified maximum demand. In situations where neither BECCS nor DAC influence the carbon price (e.g., in fixed-tax scenarios), they do not compete at all.
GCAM-CDR overcomes this limitation by offering users a simple way to allow BECCS technologies to compete more directly with other forms of CDR. If a user enables BECCS integration into the CDR market, as is done by default in the configuration files provided with GCAM-CDR 1.0, BECCS technologies compete directly with other CDR technologies for a share of the CDR market. This involves a number of changes. First, the carbon-price-based subsidy to BECCS is neutralized. Second, the demand for CDR from BECCS is determined in the CDR sector using GCAM's standard algorithm for distributing market share among competing technologies, based on an endogenously calculated price. GCAM's solution algorithm adjusts the price paid to BECCS technologies for their CDR services until the amount of CDR demanded of them, collectively, equals the amount that they are willing to provide. This price is generally lower than the cost of CDR via other technologies, as BECCS technologies treat it as a subsidy to the revenue from producing electricity or other energy carriers. Given the way that GCAM allocates market share between competing technologies, this means that BECCS usually captures a significant share of the CDR market, but not all of it (a similar approach is used in the case of the fertilizer-substitute implementation of TEW).
Note that integrating BECCS into the CDR market subjects it to the overall constraint on CDR growth described in Sect. 2.3. This leads to much slower adoption of BECCS than typically occurs in rapid decarbonization scenarios in GCAM 5.4.
2.4.3 Interregional trade in CDR
GCAM-CDR allows users to enable trade in CDR services between geopolitical regions. The trade is implemented using the same Armington-style approach that GCAM 5.4 uses for many other commodities, such as corn. A supply sector is created that can draw from CDR output in any region in a multi-region or global market, and any region in that market can purchase CDR services either directly from its own market or through the multi-region market. This could be interpreted as an international clearinghouse for CDR services, in which demand for CDR in one region (e.g., Canada) can be satisfied by CDR in a different region (e.g., China).
When trade in CDR is enabled, GCAM-CDR assumes zero “home bias” (Zhao et al., 2021), meaning that each region regards “imported” CDR as a perfect substitute for domestic CDR. Users may customize trade preferences by adding non-zero home bias at the regional level or by adding global, non-price-based preferences for CDR services from some regions over others.
By default, each region's share of the global CDR market is initially weighted according to that region's share of global GHG emissions in a reference scenario. Taking these weights into account, GCAM-CDR then allocates market share across regions based on economic efficiency, so that regions with more cost-effective CDR options will supply proportionally more CDR. Users can easily modify these initial weightings, but some unequal weighting is necessary to avoid the implausible outcome that each region would supply very similar amounts of CDR, regardless of their geographical or economic size, industrial capacity, and other relevant factors. Weighting by GHG emissions in the baseline case captures a variety of key drivers of CDR capacity, including economic output, total industrial capacity, and agricultural land available for enhanced weathering.
Enabling trade also makes it possible for users to set demand for CDR at the global level and have GCAM-CDR distribute it across geopolitical regions based on regions' capacity for CDR and the cost of CDR in each region.
2.4.4 Mechanisms for setting demand for CDR
In GCAM 5.4, overall demand for CDR equals the quantity of CDR via BECCS induced by a given carbon price plus the demand for DAC, if any, which is based on the carbon price and the exogenously specified maximum demand for DAC. Users cannot easily specify total demand for CDR.
GCAM-CDR 1.0 provides a number of approaches to specify total demand for CDR, as shown in Table 1. The three basic ways of setting CDR demand are as follows: exogenously specifying a quantity of CO2 to remove, allowing demand for CDR to vary endogenously with the carbon price, and setting CDR equal to some fraction or multiple of the emissions in particular regions and/or sectors. These can be combined with one another (e.g., by setting a base demand at a certain level and then allowing additional demand to be driven by the carbon price).
Users can also configure the model so that “unsatisfied” demand accumulates over time. If the CDR sector is unable to satisfy demand in a particular period (including historical periods) because of a lack of available technologies or user-imposed constraints on the growth of CDR, that demand can be automatically added to future periods at a user-specified rate.
To compare the model behavior of GCAM-CDR and GCAM 5.4 and to demonstrate some of GCAM-CDR's new capabilities, we report results from four sets of scenarios. Like GCAM 5.4, GCAM-CDR produces roughly 2 GB of output from each scenario. So, rather than present results in their entirety, we focus on key variables and summary metrics.
3.1 Description of the scenarios
Figure 2 illustrates the four sets of scenarios and the relationships between the various scenarios.
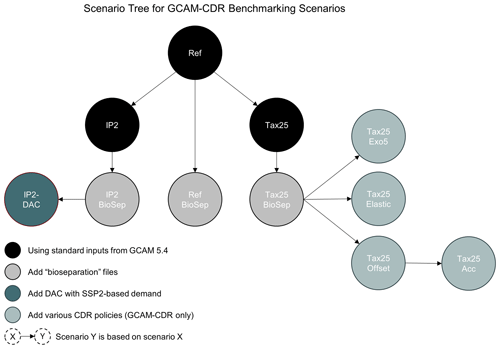
Figure 2Scenarios used to compare outputs between GCAM-CDR and GCAM 5.4 and to demonstrate some of GCAM-CDR's capabilities.
The first set (shown in black in Fig. 2) serves to demonstrate that GCAM-CDR is fully backward compatible with GCAM 5.4 and produces identical outputs when given identical inputs. Each scenario in this set uses default GCAM 5.4 input files and one of three climate policies. The reference (“Ref”) scenario includes no climate policy files and so features no new climate policy beyond what is already reflected in historical calibration data. A fixed-tax scenario (“Tax25”) includes a fixed carbon tax starting at USD 25 t−1 in 2025 and rising at 5 % per year. A scenario (“IP2”) based on Illustrative Pathway 2 from An IPCC Special Report on the impacts of global warming of 1.5 ∘C (IPCC, 2018; Rogelj et al., 2018) includes a net greenhouse gas-emissions constraint designed to limit warming to 1.5 ∘C at the end of the century. All three scenarios in this set are run in both GCAM 5.4 and GCAM-CDR using identical input files.
The second set (shown in gray in Fig. 2, directly below the first set) serves to quantify the deviations in model behavior caused by the bioseparation files described in Sect. 2.1. The scenarios in this set are identical to the first set, except that each scenario (“Ref Biosep”, “Tax25 Biosep”, and “IP2 Biosep”) also includes the bioenergy separation files discussed in Sect. 2. None of these bioseparation scenarios involve any technological CDR besides BECCS. All three scenarios in this set are run in both GCAM 5.4 and GCAM-CDR using identical input files.
The third set (shown in dark blue on the left edge of Fig. 2) serves to demonstrate GCAM-CDR's ability to emulate the behavior of GCAM 5.4 with respect to technological CDR, using GCAM-CDR's technology definition and demand-setting mechanisms. This set consists of a single scenario (“IP2-DAC”) run with slightly different input files in GCAM 5.4 and GCAM-CDR. We first run the IP2 scenario in GCAM 5.4 using GCAM 5.4's implementation of DAC and its SSP2-based assumptions about DAC demand, as developed by Fuhrman et al. (2021). This yields a scenario similar to the high-overshoot scenario described by Fuhrman et al. (2020). We then run the same scenario in GCAM-CDR using GCAM-CDR's implementation of DAC, exogenously setting regional demand for CDR to match demand for DAC in the GCAM 5.4 version of the scenario. For this scenario, we do not integrate BECCS into the CDR sector, allow trade in CDR between regions, or impose exogenous constraints on the growth of CDR.
The fourth set (shown in lighter blue on the right side of Fig. 2) serves to demonstrate some of GCAM-CDR's new capabilities. This set is run only in GCAM-CDR because they depend on functionality not available in GCAM 5.4. Specifically, they use GCAM-CDR's full suite of CDR technologies, integrating BECCS into the CDR market, allowing trade in CDR between regions, and limiting the growth of CDR output to 15 % per year. Each scenario uses the same carbon price trajectory as in the Tax25 scenario, but with a different CDR policy from Table 1: an exogenous global demand of 5 Gt C (18.33 Gt CO2) per year (“Exo5”); an elastic demand that responds endogenously to the carbon price (“Elastic”); an “offset” demand that attempts to offset 100 % of CO2 emissions in each period, starting in 2025 (“Offset”); and a scenario that seeks to offset 100 % of CO2 emissions in each period from 2005 onward and allows unsatisfied demand from earlier periods to be satisfied later in the century as CDR capacity grows (“Acc”).
3.2 Deviations from GCAM 5.4 are small
The first two sets of scenarios (shown in black and gray, respectively, in the center of Fig. 2) quantify the deviations introduced by GCAM-CDR's base configuration without CDR. The overall deviations in primary energy by source are quantified in Fig. 3, using the taxicab distance metric developed by Krey and Riahi (2013). This distance metric, which normalizes deviations between models or scenarios to the overall scale of primary energy consumption, has been used to measure intermode consistency in various IAM studies (Clarke et al., 2012; Iyer et al., 2019; Cohen et al., 2021).
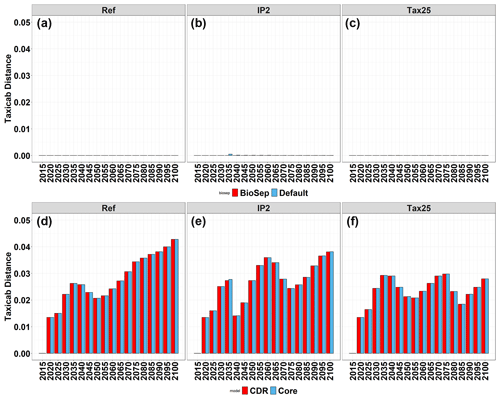
Figure 3The between-model taxicab distance for primary energy consumption by source in GCAM-CDR 1.0 and GCAM 5.4 using standard GCAM 5.4 inputs in scenarios that include no new climate policy (a), an ambitious carbon constraint (b), and a moderate carbon price (c). Panels (d-f) show the within-model taxicab distance between outputs with and without the ”bioseparation” files, using the same climate policies as in (a-c).
Without the bioseparation files, the models behave virtually identically. We hypothesize that the minuscule differences that do arise result from differences in the rounding of floating point numbers, caused by differences in compiler output between the two executable files, which cause the solution algorithm to follow very slightly different paths. When the bioseparation files are included, both models deviate noticeably from the “unseparated” scenarios. The deviations differ qualitatively depending on the climate policy, but they remain small overall. Using the taxicab metric, the overall “distance” between equivalent scenarios is <0.05 in every period and usually <0.03. The taxicab distance between the unseparated and bioseparated scenario in each period is virtually identical across models, implying very similar model behavior in response to the bioseparation files.
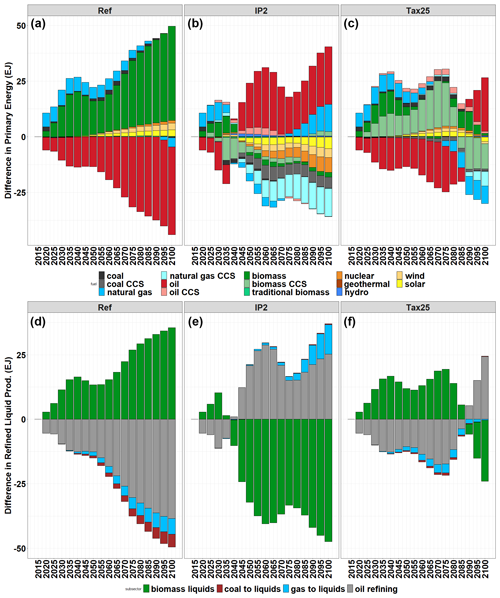
Figure 4Absolute difference in primary energy sources (a–c) and refined-liquids inputs (d–f) when adding “bioseparation” files to scenarios with no new climate policy (a, d), an ambitious carbon constraint (b, e), or a moderate carbon price (c, f).
The bioseparation files do cause shifts in energy sources that are non-negligible in absolute terms, though, as shown in Fig. 4. Specifically, the bioseparation files induce a noticeable shift in consumption of some energy sources, especially oil and biomass, but the shifts are qualitatively different depending on the scenario's climate policy. This is most prominent in the refined-liquids sector. When carbon prices are low or nonexistent, as in Tax25 and Ref scenarios, refined-petroleum products are partially displaced by additional bioliquids (for a sense of scale, the increase in bioliquids in 2100 in the Ref_BioSep scenario amounts to a change in feedstock for 10.9 % of global refined-liquids production). When carbon prices are high, as in most of the IP2 scenario or the end of the Tax25 scenario, the reverse happens: refined-petroleum products increase and bioliquids decrease, relative to the equivalent unseparated scenarios. This is partly driven by the bioseparation file's exclusion of bioliquids from the industrial feedstock sector, which can consume large quantities of bioliquids when the carbon price is high enough. (GCAM counts the creation of industrial feedstocks from carbonaceous liquids as a form of CO2 sequestration. GCAM 5.4 normally constrains that sector to use mainly petroleum-based fuels, but at high enough carbon prices, large amounts of bioliquids are also used for feedstocks because they receive a subsidy for doing so. Due to the highly variable duration of sequestration in industrial feedstocks, GCAM-CDR excludes bioliquids from the industrial feedstock sector altogether to prevent dubious claims of CDR in that sector, resulting in noticeably different behavior in that sector. While it is possible in principle to use bioliquids as a feedstock for industrial liquids, GCAM currently lacks sufficient detail in the industrial feedstock sector to distinguish between long-term and short-term sequestration of carbon, and so the inclusion of bioliquids in feedstocks implausibly distorts the model results).
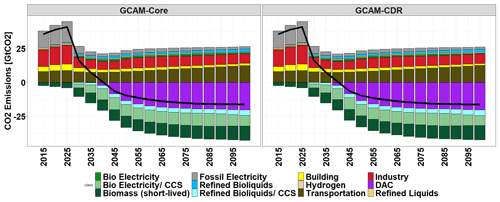
Figure 5A comparison of GCAM-CDR and GCAM 5.4 with DAC and BECCS using identical demand for DAC. The dark green negative emissions, labeled “Biomass (short-lived),” refer to CO2 that is captured from the atmosphere during the growth of biomass but re-emitted in another sector (e.g., transportation) shortly after harvesting. By contrast, negative emissions from “Bio Electricity/CCS” and “Refined Bioliquids/CCS” refer to CO2 that is captured from the atmosphere during the growth of biomass and then permanently sequestered in geological formations.
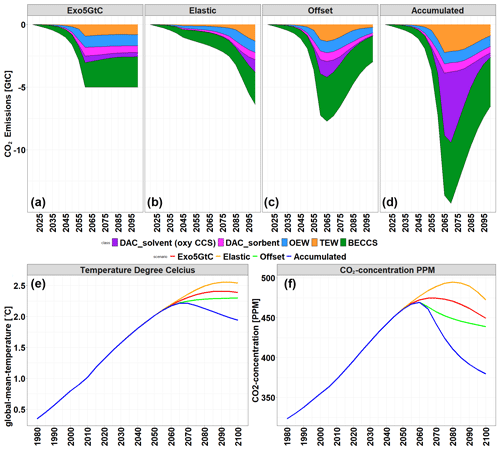
Figure 6Results from GCAM-CDR with different CDR policies. Different policies produce significantly different amounts of CDR once the exogenous constraint on CDR growth has ceased to be binding on CDR output. (a) “Exo5GtC” imposes a global CDR demand of 5 Gt C (18.33 Gt CO2) per year. (b) “Elastic” sets CDR demand to rise with the carbon price, reaching 6.39 Gt C (23.43 Gt CO2) globally in 2100. (c) “Offset” sets CDR demand to attempt to offset regional CO2 emissions in each period after 2020, subject to CDR capacity constraints. (d) “Accumulated” attempts to offset all CO2 emissions from 2005 onward, with unsatisfied demand from earlier in the century being satisfied later in the century as CDR capacity increases. Different CDR policies lead to very different temperature (e) and CO2 concentration (f) trajectories later in the century, with end-of-century values differing by more than 0.5 ∘C and roughly 100 ppm between the highest and lowest scenarios.
3.3 Similar CDR policies produce similar results in both models
Scenario IP2-DAC demonstrates GCAM-CDR's ability to emulate the behavior of DAC and BECCS in GCAM 5.4, despite the minor differences in the implementation of DAC between the two models and the major differences in the way users specify CDR demand. Figure 5 shows virtually identical sectoral emissions trajectories in both models' implementation of the scenario.
3.4 Different CDR policies produce very different outcomes
The last set of scenarios (shown in light blue in Fig. 2) demonstrates that, keeping all else constant, configuring CDR policies differently leads to very different outcomes. Each scenario is derived from the CTax25_BioSep scenario. Each scenario therefore includes the same trajectory of carbon taxes, beginning at USD 25 t−1 in 2025 and rising at 5 % per year. The scenarios differ from CTax25_BioSep in two ways: all scenarios include GCAM-CDR's full suite of CDR technologies, along with interregional trade in CDR services, and each scenario includes a different CDR policy from Table 1 (see Sect. 2.4.4). Note that all four scenarios contain an identical, exogenous constraint on the growth of CDR, which limits global growth in CDR output to 15 % per year.
Figure 6 depicts the CDR output by technology, as well as the impact of these different policies on the CO2 concentrations and global mean temperature anomaly.
GCAM-CDR builds on GCAM 5.4 in two main ways: by introducing some additional CDR technologies and by introducing a wider range of more flexible CDR-related policies. The former represents an incremental improvement, especially given the overlap between the CDR technologies represented in GCAM-CDR and those introduced in GCAM 5.4. The latter represents a significant improvement because it enables users to study a much broader range of possible climate policies.
Future research directions using GCAM-CDR are likely to include analyses of both possible CDR policies and various CDR technologies. With respect to the former, questions abound about the impact of different kinds of CDR policies on outcomes related to climate, socioeconomics, health, energy and materials usage, sustainability, and the equitable division of responsibility for CDR. With respect to the latter, one likely avenue for further research includes the introduction of additional CDR technologies, especially those that use different energy or material inputs, such as DAC powered entirely by electricity, and those with significant potential co-benefits, such as soil carbon sequestration, biochar, carbon utilization, or carbon-negative hydrogen.
At the same time, IAMs should not be mistaken for crystal balls, and GCAM-CDR is no exception. Given the large uncertainties around key parameters with respect to CDR, as well as harder-to-model uncertainties surrounding sociotechnical systems for CDR, model results must be interpreted thoughtfully when trying to explore these issues. As the saying goes, “models are for insights, not numbers.” Although IAMs report projections in exquisitely quantitative detail, these numbers should not be taken too literally. To illustrate this point, consider the relative output of high-heat DAC and low-heat DAC depicted in Fig. 6. Competition between CDR technologies in GCAM-CDR is based mainly on cost. While cost parameters for these technologies reflect the best current estimates, the cost of DAC technologies several decades from now remains highly uncertain. It is also worth noting that GCAM 5.4 (and therefore GCAM-CDR) projects higher per-unit energy costs than are used in many of the long-term cost estimates in the scientific literature, which changes the competitiveness of more energy-intensive forms of CDR relative to more capital-intensive forms. GCAM-CDR's projections should not, therefore, be taken as definitive statements about the relative cost or promise of any technology. Instead, they should be examined for important dynamics. For instance, low-heat DAC in GCAM-CDR uses waste heat from thermal power plants and industrial sources rather than producing heat from natural gas or other sources. This gives it an advantage in scenarios with lower overall CDR demand but a distinct disadvantage in scenarios with very high overall CDR demand, such as the accumulated scenario, in which there simply is not enough waste heat available in GCAM-CDR to power vast quantities of low-heat DAC. This lesson applies broadly to CDR approaches that tap into waste streams, such as DAC using curtailed renewable electricity or enhanced weathering using alkaline wastes. It also implies a complicated relationship between scalability, inputs, and the projected long-term demand for CDR. As always, the question is what can be legitimately inferred from the model's behavior and what new questions are raised by that behavior.
GCAM-CDR is an open source model. The version of GCAM-CDR described in this paper is archived on GitHub and Zenodo (https://doi.org/10.5281/zenodo.7261646, Apeaning et al., 2022). The complete model and a user guide are available at https://github.com/icrlp/gcam-cdr (last access: 1 February 2023). Configuration files for the scenarios reported in this paper are available in the Supplement.
Data sets referred to in this paper, such as the historical calibration data for GCAM, are embedded in the GCAM-CDR XML inputs. Those inputs can be downloaded with the model code, as described in the “Code availability” statement.
The supplement related to this article is available online at: https://doi.org/10.5194/gmd-16-1105-2023-supplement.
RA, GG, and DRM all participated in the development of GCAM-CDR. DRM led the writing of the paper with contributions from RA and GG. Simulations in the paper were designed by DRM, RA, and GG; they were conducted and analyzed by RA. Figures were produced by DRM and RA.
The contact author has declared that none of the authors has any competing interests.
Publisher's note: Copernicus Publications remains neutral with regard to jurisdictional claims in published maps and institutional affiliations.
This research, including the development of GCAM-CDR, has been supported by the Alfred P. Sloan Foundation (grant no. G-2019-12475).
This paper was edited by Daniel Huppmann and reviewed by Jay Fuhrman and one anonymous referee.
Anderson, K. and Peters, G.: The trouble with negative emissions, 354, 182–183, https://doi.org/10.1126/science.aah4567, 2016.
Apeaning, R., Guard, G., and Morrow, D. R.: GCAM-CDR 1.0.2, Zenodo [code], https://doi.org/10.5281/zenodo.7261646, 2022.
Beerling, D. J., Kantzas, E. P., Lomas, M. R., Wade, P., Eufrasio, R. M., Renforth, P., Sarkar, B., Andrews, M. G., James, R. H., Pearce, C. R., Mercure, J.-F., Pollitt, H., Holden, P. B., Edwards, N. R., Khanna, M., Koh, L., Quegan, S., Pidgeon, N. F., Janssens, I. A., Hansen, J., and Banwart, S. A.: Potential for large-scale CO2 removal via enhanced rock weathering with croplands, 583, 242–248, https://doi.org/10.1038/s41586-020-2448-9, 2020.
Bond-Lamberty, B., Patel, P., Lurz, J., Smith, S., abigailsnyder, pkyle, kvcalvin, Dorheim, K. R., Link, R., mbins, skim301, S, A., Feng, L., Turner, S. W. D., cwroney, Lynch, C., jhoring, Khan, Z., Haewon, mwisepnnl, mollycharles, Iyer, G., Shiklomanov, A., swaldhoff, Plevin, R., matteomuratori, amundra, Hartin, C., and Narayan, K.: JGCRI/gcam-core: GCAM 5.4, Zenodo [code], https://doi.org/10.5281/zenodo.5093192, 2021.
Calvin, K., Patel, P., Clarke, L., Asrar, G., Bond-Lamberty, B., Cui, R. Y., Di Vittorio, A., Dorheim, K., Edmonds, J., Hartin, C., Hejazi, M., Horowitz, R., Iyer, G., Kyle, P., Kim, S., Link, R., McJeon, H., Smith, S. J., Snyder, A., Waldhoff, S., and Wise, M.: GCAM v5.1: representing the linkages between energy, water, land, climate, and economic systems, Geosci. Model Dev., 12, 677–698, https://doi.org/10.5194/gmd-12-677-2019, 2019.
Caserini, S., Pagano, D., Campo, F., Abbà, A., De Marco, S., Righi, D., Renforth, P., and Grosso, M.: Potential of Maritime Transport for Ocean Liming and Atmospheric CO2 Removal, Front. Clim., 3, https://doi.org/10.3389/fclim.2021.575900, 2021.
Clarke, L., Krey, V., Weyant, J., and Chaturvedi, V.: Regional energy system variation in global models: Results from the Asian Modeling Exercise scenarios, Energ. Econom., 34, S293–S305, https://doi.org/10.1016/j.eneco.2012.07.018, 2012.
Cohen, S. M., Iyer, G. C., Brown, M., Macknick, J., Wise, M., Binsted, M., Voisin, N., Rice, J., and Hejazi, M.: How structural differences influence cross-model consistency: An electric sector case study, Renew. Sust. Energ. Rev., 144, 111009, https://doi.org/10.1016/j.rser.2021.111009, 2021.
Edwards, D. P., Lim, F., James, R. H., Pearce, C. R., Scholes, J., Freckleton, R. P., and Beerling, D. J.: Climate change mitigation: potential benefits and pitfalls of enhanced rock weathering in tropical agriculture, 13, 20160715, https://doi.org/10.1098/rsbl.2016.0715, 2017.
Fuhrman, J., McJeon, H., Patel, P., Doney, S. C., Shobe, W. M., and Clarens, A. F.: Food–energy–water implications of negative emissions technologies in a +1.5 ∘C future, Nat. Clim. Change, 10, 920–927, https://doi.org/10.1038/s41558-020-0876-z, 2020.
Fuhrman, J., Clarens, A., Calvin, K., Doney, S. C., Edmonds, J. A., O'Rourke, P., Patel, P., Pradhan, S., Shobe, W., and McJeon, H.: The role of direct air capture and negative emissions technologies in the shared socioeconomic pathways towards +1.5 ∘C and +2 ∘C futures, Environ. Res. Lett., 16, 114012, https://doi.org/10.1088/1748-9326/ac2db0, 2021.
Fuss, S., Canadell, J. G., Peters, G. P., Tavoni, M., Andrew, R. M., Ciais, P., Jackson, R. B., Jones, C. D., Kraxner, F., Nakicenovic, N., Le Quéré, C., Raupach, M. R., Sharifi, A., Smith, P., and Yamagata, Y.: Betting on negative emissions, Nat. Clim. Change, 4, 850–853, https://doi.org/10.1038/nclimate2392, 2014.
Fuss, S., Lamb, W. F., Callaghan, M. W., Hilaire, J., Creutzig, F., Amann, T., Beringer, T., Garcia, W. de O., Hartmann, J., Khanna, T., Luderer, G., Nemet, G. F., Rogelj, J., Smith, P., Vicente, J. L. V., Wilcox, J., Dominguez, M. del M. Z., and Minx, J. C.: Negative emissions – Part 2: Costs, potentials and side effects, Environ. Res. Lett., 13, 063002, https://doi.org/10.1088/1748-9326/aabf9f, 2018.
Goll, D. S., Ciais, P., Amann, T., Buermann, W., Chang, J., Eker, S., Hartmann, J., Janssens, I., Li, W., Obersteiner, M., Penuelas, J., Tanaka, K., and Vicca, S.: Potential CO2 removal from enhanced weathering by ecosystem responses to powdered rock, Nat. Geosci., 14, 545–549, https://doi.org/10.1038/s41561-021-00798-x, 2021.
IPCC: Summary for Policymakers, in: Global Warming of 1.5 ∘C. An IPCC Special Report on the impacts of global warming of 1.5 ∘C above pre-industrial levels and related global greenhouse gas emissions pathways, in the context of strengthening the global response to the threat of climate change, sustainable development, and efforts to eradicate poverty, edited by: Masson-Delmotte, V., Zhai, P., Pörtner, H. O., Roberts, D., Skea, J., Shukla, P. R., Pirani, A., Moufouma-Okia, W., Péan, C., Pidcock, R., Connors, S., Matthews, J. B. R., Chen, Y., Zhou, X., Gomis, M. I., Lonnoy, E., Maycock, T., Tignor, M., and Waterfield, T., Cambridge University Press, Cambridge, UK and New York, NY, USA, https://doi.org/10.1017/9781009157940.001, 2018.
Iyer, G. C., Brown, M., Cohen, S. M., Macknick, J., Patel, P., Wise, M., Binsted, M., and Voisin, N.: Improving consistency among models of overlapping scope in multi-sector studies: The case of electricity capacity expansion scenarios, Renew. Sustain. Energ. Rev., 116, 109416, https://doi.org/10.1016/j.rser.2019.109416, 2019.
Keith, D. W., Holmes, G., St. Angelo, D., and Heidel, K.: A Process for Capturing CO2 from the Atmosphere, Joule, 2, 1573–1594, https://doi.org/10.1016/j.joule.2018.05.006, 2018.
Kelland, M. E., Wade, P. W., Lewis, A. L., Taylor, L. L., Sarkar, B., Andrews, M. G., Lomas, M. R., Cotton, T. E. A., Kemp, S. J., James, R. H., Pearce, C. R., Hartley, S. E., Hodson, M. E., Leake, J. R., Banwart, S. A., and Beerling, D. J.: Increased yield and CO2 sequestration potential with the C4 cereal Sorghum bicolor cultivated in basaltic rock dust-amended agricultural soil, Global Change Biol., 26, 3658–3676, https://doi.org/10.1111/gcb.15089, 2020.
Köberle, A. C.: The Value of BECCS in IAMs: a Review, Curr. Sustain. Renew. Energ. Rep., 6, 107–115, https://doi.org/10.1007/s40518-019-00142-3, 2019.
Köhler, P., Abrams, J. F., Völker, C., Hauck, J., and Wolf-Gladrow, D. A.: Geoengineering impact of open ocean dissolution of olivine on atmospheric CO2, surface ocean pH and marine biology, Environ. Res. Lett., 8, 014009, https://doi.org/10.1088/1748-9326/8/1/014009, 2013.
Krey, V. and Riahi, K.: Risk Hedging Strategies Under Energy System and Climate Policy Uncertainties, in: Handbook of Risk Management in Energy Production and Trading, vol. 199, edited by: Kovacevic, R. M., Pflug, G. Ch., and Vespucci, M. T., Springer US, Boston, MA, 435–474, https://doi.org/10.1007/978-1-4614-9035-7_17, 2013.
Kyle, G. P., Luckow, P., Calvin, K. V., Emanuel, W. R., Nathan, M., and Zhou, Y.: GCAM 3.0 Agriculture and Land Use: Data Sources and Methods, Report PNNL-21025, Pacific Northwest National Lab, Richmond, WA, https://doi.org/10.2172/1036082, 2011.
Luckow, P., Wise, M. A., Dooley, J. J., and Kim, S. H.: Large-scale utilization of biomass energy and carbon dioxide capture and storage in the transport and electricity sectors under stringent CO2 concentration limit scenarios, Int. J. Greenh. Gas Control, 4, 865–877, https://doi.org/10.1016/j.ijggc.2010.06.002, 2010.
McLaren, D.: Quantifying the potential scale of mitigation deterrence from greenhouse gas removal techniques, Clim. Change, 162, 2411–2428, https://doi.org/10.1007/s10584-020-02732-3, 2020.
McLaren, D. P., Tyfield, D. P., Willis, R., Szerszynski, B., and Markusson, N. O.: Beyond “Net-Zero”: A Case for Separate Targets for Emissions Reduction and Negative Emissions, Front. Clim., 1, https://doi.org/10.3389/fclim.2019.00004, 2019.
McQueen, N., Psarras, P., Pilorgé, H., Liguori, S., He, J., Yuan, M., Woodall, C. M., Kian, K., Pierpoint, L., Jurewicz, J., Lucas, J. M., Jacobson, R., Deich, N., and Wilcox, J.: Cost Analysis of Direct Air Capture and Sequestration Coupled to Low-Carbon Thermal Energy in the United States, Environ. Sci. Technol., 54, 7542–7551, https://doi.org/10.1021/acs.est.0c00476, 2020.
Meysman, F. J. R. and Montserrat, F.: Negative CO2 emissions via enhanced silicate weathering in coastal environments, Biol. Lett., 13, 20160905, https://doi.org/10.1098/rsbl.2016.0905, 2017.
National Academies of Science, Engineering, and Medicine: Negative Emissions Technologies and Reliable Sequestration: A Research Agenda, National Academies Press, Washington, D.C., https://doi.org/10.17226/25259, 2018.
Nunes, J. M. G., Kautzmann, R. M., and Oliveira, C.: Evaluation of the natural fertilizing potential of basalt dust wastes from the mining district of Nova Prata (Brazil), J. Cleaner Prod., 84, 649–656, https://doi.org/10.1016/j.jclepro.2014.04.032, 2014.
Rau, G. H., Willauer, H. D., and Ren, Z. J.: The global potential for converting renewable electricity to negative-CO2-emissions hydrogen, Nat. Clim. Change, 8, 621–625, https://doi.org/10.1038/s41558-018-0203-0, 2018.
Realmonte, G., Drouet, L., Gambhir, A., Glynn, J., Hawkes, A., Köberle, A. C., and Tavoni, M.: An inter-model assessment of the role of direct air capture in deep mitigation pathways, Nat. Commun., 10, 1–12, https://doi.org/10.1038/s41467-019-10842-5, 2019.
Renforth, P.: The negative emission potential of alkaline materials, Nat. Commun., 10, 1–8, https://doi.org/10.1038/s41467-019-09475-5, 2019.
Renforth, P., Jenkins, B. G., and Kruger, T.: Engineering challenges of ocean liming, Energy, 60, 442–452, https://doi.org/10.1016/j.energy.2013.08.006, 2013.
Riahi, K., Bertram, C., Huppmann, D., Rogelj, J., Bosetti, V., Cabardos, A.-M., Deppermann, A., Drouet, L., Frank, S., Fricko, O., Fujimori, S., Harmsen, M., Hasegawa, T., Krey, V., Luderer, G., Paroussos, L., Schaeffer, R., Weitzel, M., van der Zwaan, B., Vrontisi, Z., Longa, F. D., Després, J., Fosse, F., Fragkiadakis, K., Gusti, M., Humpenöder, F., Keramidas, K., Kishimoto, P., Kriegler, E., Meinshausen, M., Nogueira, L. P., Oshiro, K., Popp, A., Rochedo, P. R. R., Ünlü, G., van Ruijven, B., Takakura, J., Tavoni, M., van Vuuren, D., and Zakeri, B.: Cost and attainability of meeting stringent climate targets without overshoot, Nat. Clim. Change, 1–7, https://doi.org/10.1038/s41558-021-01215-2, 2021.
Rogelj, J., Shindell, D., Jiang, K., Fifita, S., Forster, P., Ginzburg, V., Handa, C., Kheshgi, H., Kobayashi, S., Kriegler, E., Mundaca, L., Séférian, R., and Vilariño, M. V.: 2018: Mitigation Pathways Compatible with 1.5 ∘C in the Context of Sustainable Development, in: Global Warming of 1.5 ∘C. An IPCC Special Report on the impacts of global warming of 1.5 ∘C above pre-industrial levels and related global greenhouse gas emission pathways, in the context of strengthening the global response to the threat of climate change, sustainable development, and efforts to eradicate poverty, edited by: Masson-Delmotte, V., Zhai, P., Pörtner, H.-O., Roberts, D., Skea, J., Shukla, P. R., Pirani, A., Moufouma-Okia, W., Péan, C., Pidcock, R., Connors, S., Matthews, J. B. R., Chen, Y., Zhou, X., Gomis, M. I., Lonnoy, E., Maycock, T., Tignor, M., and Waterfield, T., Intergovernmental Panel on Climate Change, 93–174, https://doi.org/10.1017/9781009157940.004, 2018.
Strefler, J., Amann, T., Bauer, N., Kriegler, E., and Hartmann, J.: Potential and costs of carbon dioxide removal by enhanced weathering of rocks, Environ. Res. Lett., 13, 034010, https://doi.org/10.1088/1748-9326/aaa9c4, 2018.
Tyka, M. D., Arsdale, C. V., and Platt, J. C.: CO2 capture by pumping surface acidity to the deep ocean, Energy Environ. Sci., 15, 786–798, https://doi.org/10.1039/D1EE01532J, 2022.
Wurzbacher, J. A., Gebald, C., Brunner, S., and Steinfeld, A.: Heat and mass transfer of temperature–vacuum swing desorption for CO2 capture from air, Chem. Eng. J., 283, 1329–1338, https://doi.org/10.1016/j.cej.2015.08.035, 2016.
Xing, L., Pullin, H., Bullock, L., Renforth, P., Darton, R. C., and Yang, A.: Potential of enhanced weathering of calcite in packed bubble columns with seawater for carbon dioxide removal, Chem. Eng. J., 431, 134096, https://doi.org/10.1016/j.cej.2021.134096, 2022.
Zhao, X., Calvin, K. V., Wise, M. A., and Iyer, G.: The role of global agricultural market integration in multiregional economic modeling: Using hindcast experiments to validate an Armington model, Econ. Anal. Policy, 72, 1–17, https://doi.org/10.1016/j.eap.2021.07.007, 2021.