the Creative Commons Attribution 4.0 License.
the Creative Commons Attribution 4.0 License.
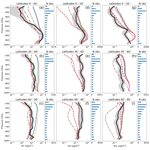
In-cloud scavenging scheme for sectional aerosol modules – implementation in the framework of the Sectional Aerosol module for Large Scale Applications version 2.0 (SALSA2.0) global aerosol module
Eemeli Holopainen
Harri Kokkola
Anton Laakso
Thomas Kühn
In this study we introduce an in-cloud wet deposition scheme for liquid and ice phase clouds for global aerosol–climate models which use a size-segregated aerosol description. For in-cloud nucleation scavenging, the scheme uses cloud droplet activation and ice nucleation rates obtained from the host model. For in-cloud impaction scavenging, we used a method where the removal rate depends on the wet aerosol size and cloud droplet radii. We used the latest release version of ECHAM-HAMMOZ (ECHAM6.3-HAM2.3-MOZ1.0) with the Sectional Aerosol module for Large Scale Applications version 2.0 (SALSA) microphysics package to test and compare our scheme. The scheme was compared to a scheme that uses fixed scavenging coefficients. The comparison included vertical profiles and mass and number distributions of wet deposition fluxes of different aerosol compounds and for different latitude bands. Using the scheme presented here, mass concentrations for black carbon, organic carbon, sulfate, and the number concentration of particles with diameters larger than 100 nm are higher than using fixed scavenging coefficients, with the largest differences in the vertical profiles in the Arctic. On the other hand, the number concentrations of particles smaller than 100 nm in diameter show a decrease, especially in the Arctic region. These results could indicate that, compared to fixed scavenging coefficients, nucleation scavenging is less efficient, resulting in an increase in the number concentration of particles larger than 100 nm. In addition, changes in rates of impaction scavenging and new particle formation (NPF) can be the main cause of reduction in the number concentrations of particles smaller than 100 nm. Without further adjustments in the host model, our wet deposition scheme produced unrealistically high aerosol concentrations, especially at high altitudes. This also leads to a spuriously long lifetime of black carbon aerosol. To find a better setup for simulating aerosol vertical profiles and transport, sensitivity simulations were conducted where aerosol emission distribution and hygroscopicity were altered. Vertical profiles of aerosol species simulated with the scheme which uses fixed scavenging rates and the abovementioned sensitivity simulations were evaluated against vertical profiles from aircraft observations. The lifetimes of different aerosol compounds were also evaluated against the ensemble mean of models involved in the Aerosol Comparisons between Observations and Models (AEROCOM) project. The best comparison between the observations and the model was achieved with our wet deposition scheme when black carbon was emitted internally mixed with soluble compounds instead of keeping it externally mixed. This also produced atmospheric lifetimes for the other species which were comparable to the AEROCOM model means.
The estimated radiation budget of the Earth has large uncertainties, and a majority of these uncertainties are related to the uncertainties in the direct and indirect effects of atmospheric aerosol (IPCC, 2014). Aerosol particles can affect the climate directly by scattering and absorbing radiation and indirectly through aerosol–cloud interactions (Haywood and Shine, 1997; Twomey, 1991; Albrecht, 1989). Thus, in order to estimate the radiation budget of the Earth correctly, aerosols and their physical properties affecting radiation and cloud formation have to be modeled realistically.
Black carbon (BC) is one of the aerosol compounds which has an effect on the Earth's radiation budget via absorbing solar radiation, accelerating the melting of snow and ice, and influencing cloud formation and life cycle (Bond et al., 2013). A large fraction of BC is emitted through incomplete combustion, which is due to anthropogenic activities (Bond et al., 2013). Due to its ability to darken snow and ice covers, BC has been found to be a major warming agent at high latitudes (AMAP, 2015). In addition, it has been proposed that the mitigation of BC is one of the possible means to slow Arctic warming (Stone et al., 2014).
Transport of aerosol particles to remote regions with only small amounts of emitted particles affects the local aerosol size distribution and composition (Rasch et al., 2000; Croft et al., 2010). In these areas, e.g., the Arctic, simulated aerosol and especially BC concentrations differ from those observed, as the transport to these regions is modeled poorly (Bourgeois and Bey, 2011; Sharma et al., 2013; Kristiansen et al., 2016). In addition, BC vertical profiles affect the uncertainty of its forcing emphasizing the need to improve BC vertical profiles in global aerosol–climate models (Samset et al., 2013). The vertical distribution of aerosol compounds is found to be affected by emissions, hygroscopicity, deposition, and microphysical processes, of which wet removal can be the cause of one of the major biases in the models (Kipling et al., 2016; Watson-Parris et al., 2019). Thus, one possible cause for problems in modeling long-range and vertical transport of BC is how wet removal of aerosol compounds is modeled (Bourgeois and Bey, 2011; Croft et al., 2016). Wet deposition processes are modeled very differently among global aerosol–climate models and, therefore, more research is needed to better parameterize and constrain wet deposition in models (Croft et al., 2009, 2010, 2016; Textor et al., 2006; Kipling et al., 2016).
Wet removal of aerosol particles from the atmosphere is a process where these particles are scavenged by hydrometeors and then carried to the surface by precipitation (Wang et al., 1978). There are two kinds of wet deposition processes: in-cloud and below-cloud scavenging (Slinn and Hales, 1971; Rasch et al., 2000; Zikova and Zdimal, 2016). In the process of in-cloud scavenging, aerosol species can enter the cloud droplets or ice crystals through a nucleation process, when they act as cloud condensation nuclei (CCN) or ice nuclei (IN). This process is called in-cloud nucleation scavenging (Pruppacher and Klett, 1997). In the process called in-cloud impaction scavenging, aerosol particles can be scavenged through collision with ice crystals or cloud droplets (Chate et al., 2003; Ladino et al., 2011). Aerosol compounds are then removed from the atmosphere when these cloud droplets or ice crystals grow to precipitation sizes (Pruppacher and Klett, 1997; Croft et al., 2010). Below-cloud scavenging is a process where rain droplets or snow crystals, which precipitate from the cloud, sweep aerosol particles below the cloud through collision (Chate et al., 2011). Observational studies have shown that below-cloud scavenging is strongly dependent on the rain droplet or snow crystal size distribution (Andronache, 2003; Andronache et al., 2006).
In recent years it has become evident that more detailed descriptions of wet deposition in global climate models are important (Korhonen et al., 2008; Garrett et al., 2010; Browse et al., 2012). In addition to transport, wet removal can affect the Arctic aerosol size distribution and its seasonal cycle (Korhonen et al., 2008; Croft et al., 2016). Even though the processes involved in wet removal are well known, it is still difficult to represent them well in global climate models (Eckhardt et al., 2015). In order to realistically describe the wet removal processes, a thorough knowledge of the microphysics of condensation and precipitation, as well as aerosol microphysics, is needed (Rasch et al., 2000).
Here, we describe our scheme for wet deposition using physical parameterizations for nucleation and impaction scavenging in liquid and ice clouds for sectional aerosol modules. The new aspects of this scheme, compared to the modal aerosol scheme already implemented in ECHAM-HAMMOZ, are that it calculates the in-cloud nucleation scavenging rates using the activated fraction in each size class in the liquid cloud case and the surface area of particles in the ice cloud case. Similar approaches for liquid cloud cases exist in other global models which use modal aerosol modules, e.g., MIRAGE and CAM5 (Easter et al., 2004; Wang et al., 2013). We further tested the sensitivity of our scheme to assumptions in aerosol emission distribution and hygroscopicity. The structure of the paper is as follows. In Sect. 2 we present details on in-cloud nucleation and impaction scavenging in general and introduce our in-cloud nucleation scavenging scheme for liquid and ice clouds. In addition, we present details on the aerosol module SALSA (Sectional Aerosol module for Large Scale Applications) and its components, which we used to test and evaluate our scheme and its sensitivity. In Sect. 2 we present the modifications performed for SALSA to include in-cloud impaction scavenging and the treatment of below-cloud scavenging. In the same section, we also present the ECHAM-HAMMOZ aerosol–chemistry–climate model and its setup, which is used for testing the scheme on a global scale. In Sect. 3 we present the evaluation of our scheme against a fixed scavenging coefficient scheme in terms of vertical profiles and wet deposition fluxes of different aerosol compounds. In addition, in the same section, we evaluate the vertical profiles of different aerosol compounds from the simulations against those from the Atmospheric Tomography (ATom) aircraft campaigns (Wofsy et al., 2018). We also compare the wet deposition fluxes, of different aerosol compounds, from different sensitivity simulations to each other. Finally, we compare the lifetimes from all of the simulations to the mean from several models in the Aerosol Comparisons between Observations and Models (AEROCOM) project.
In this section we will describe the in-cloud nucleation and impaction scavenging, for both liquid and ice phase clouds. For both of these cloud phases, the removal of aerosol particles is expressed in terms of a scavenging coefficient. The rate of change in the concentration of compound l in size class i, due to in-cloud nucleation and impaction scavenging, for both liquid and ice clouds, is of the form
where and are the fractions of activated particles due to nucleation scavenging in liquid and ice clouds, respectively, and and are the scavenging coefficients due to impaction scavenging in liquid and ice clouds, respectively (Croft et al., 2010). Furthermore, fcl is the cloud fraction, fliq is the liquid fraction of the total cloud water, Qliq is the sum of conversion rate of cloud liquid water to precipitation by autoconversion, accretion, and aggregation processes, Cliq is the cloud liquid water content, and fice, Qice, and Cice are the equivalent variables for ice (Croft et al., 2010). The values in Eq. (1) are in-cloud values (Croft et al., 2010).
2.1 In-cloud scavenging scheme for liquid clouds
The in-cloud process of nucleation scavenging refers to activation and growth of aerosol particles into cloud droplets (Köhler, 1936). When water vapor reaches supersaturation, a fraction of the aerosol population is activated to cloud droplets. After these cloud droplets have grown to precipitation size, the particles can be removed from the cloud through precipitation (Wang et al., 1978). The ability of an aerosol particle to activate to a cloud droplet depends on its size, chemical composition, and the ambient supersaturation (Köhler, 1936).
In aerosol modules of global climate models, the aerosol size distribution can be approximated by, for example, a modal or sectional discretization, which effectively separates the size distribution into different size classes (Stier et al., 2005; Kokkola et al., 2018a). In each size class the fraction of activated particles can be calculated as the portion of particles that exceed the critical diameter of activation in that size class (Köhler, 1936; Croft et al., 2010). However, many models describe the nucleation scavenging by assuming a constant scavenging coefficient for different aerosol size classes (Stier et al., 2005; Seland et al., 2008; de Bruine et al., 2018).
The current in-cloud nucleation scavenging scheme for liquid clouds introduced here calculates the scavenging coefficients of aerosol based on the fraction of activated particles in each size class, i.e., in Eq. (1). Thus, using the scheme requires that the atmospheric model incorporates a cloud activation parameterization that calculates size segregated cloud activation. Such parameterizations are, e.g., Abdul-Razzak and Ghan (2002) and Barahona and Nenes (2007).
In-cloud impaction scavenging, for liquid clouds, is a process where aerosol particles collide with existing cloud droplets and are thereby removed from the interstitial air of the cloud (Chate et al., 2003). This aerosol scavenging by cloud droplets is based on coagulation theory, which quantifies the rate of removal. This is further used to define the scavenging coefficients by impaction (Seinfeld and Pandis, 2006). Commonly, these scavenging coefficients, for the full aerosol particle distribution, can be calculated as
where dp is the wet diameter of the aerosol particle, Dliq is the cloud droplet diameter, K(dp,Dliq) is the collection efficiency between aerosol particles and cloud droplets, and n(Dliq,t) is the cloud droplet number distribution (Seinfeld and Pandis, 2006).
2.2 In-cloud scavenging scheme for ice clouds
In-cloud nucleation scavenging in ice clouds refers to the formation and growth of ice particles (Seinfeld and Pandis, 2006). When ice particles are formed, they can quickly grow into precipitation sizes and be removed from the cloud (Korolev et al., 2011). The formation of ice particles in the atmosphere usually requires an ice nucleus (IN), but they can also be formed without IN if the temperature is very low (Hobbs, 1993). Aerosol particles which can act as IN are usually insoluble (Marcolli et al., 2007). In addition, large particles are more efficient in acting as IN than small particles (Archuleta et al., 2005).
The nucleation rate, JT, which is the total number of ice crystals formed in a unit volume of air per unit time, can be expressed as the sum of the nucleation rate in a unit volume of liquid solution, JV, multiplied by the total collective volume of aerosol particles in a unit volume of air, Vt, and the nucleation rate on a unit surface area of liquid solution, JS, multiplied by the total collective surface area of aerosol particles in a unit volume of air, St (Tabazadeh et al., 2002). However, experimental studies and thermodynamic calculations for the ice–water–air system suggest that the total number of ice crystals formed is dominated by surface-based processes, so that JSSt≫JVVt (Tabazadeh et al., 2002). With this assumption the total nucleation rate can be simplified to
Global models usually give the total in-cloud ice nucleation rate, which is here segregated into size-resolved nucleation rates. Since we assume that the number of nucleated ice particles depends only on the aerosol surface area, the scavenging coefficient in ice-containing clouds in size class i is proportional to the ratio between nucleation rate in the size class and the total nucleation rate. Thus, for the scavenging coefficient, for the ice-containing clouds, we get the following in each size class:
where Si is the surface area concentration of size class i, ΔICNC is the ice crystal number concentration obtained from the ice cloud activation scheme, and ni is the number concentration in size class i. The total surface area in each size class is derived using the associated number or mass median wet aerosol radius.
2.3 SALSA
To test how the in-cloud wet deposition scheme affects simulated global aerosol concentrations, we used it with the SALSA2.0 in our ECHAM-HAMMOZ global model simulations. In addition, we tested how sensitive the simulated aerosol concentrations are to emission sizes, mixing, and aging when this scheme is used. SALSA is the sectional aerosol module of ECHAM-HAMMOZ global climate model. Details for calculations of aerosol emissions and chemistry in SALSA are presented in Kokkola et al. (2018a). SALSA is a very versatile aerosol microphysics module, which has been implemented in several models of very different spatial resolution (Kokkola et al., 2018a; Tonttila et al., 2017; Andersson et al., 2015; Kurppa et al., 2019). To describe the aerosol population, SALSA uses a hybrid bin sectional approach for calculating the evolution of the size distribution (Chen and Lamb, 1994; Kokkola et al., 2018a). In SALSA the aerosol population is divided into two subregions depending on their size. The first subregion is from 3 nm to 50 nm and the second is from 50 to 10 µm. These subregions are further divided into size sections defining the minimum and maximum diameter of the particles. In each size section the aerosol particles are assumed to be monodisperse, and chemistry and different microphysical processes are calculated for each size section separately. In addition, the second subregion is divided into externally mixed soluble and insoluble populations. A more detailed description of the newest SALSA version, SALSA2.0, is presented in Kokkola et al. (2018a).
Originally, SALSA used fixed scavenging coefficients, Fi, for different size classes i, in its wet deposition calculations. These coefficients include all the processes for in-cloud and below-cloud scavenging (Bergman et al., 2012). The fixed coefficients, for stratiform and convective clouds with different phases (liquid, mixed, and ice) and solubilities, are adapted for SALSA from the calculations presented by Stier et al. (2005), and they are presented in detail in Bergman et al. (2012). Here we refine the entire scavenging scheme by calculating the scavenging coefficients online.
We used the Abdul-Razzak and Ghan (2002) cloud activation scheme to derive the fraction of activated particles in each size class for our in-cloud nucleation scavenging calculations. However, the original activation scheme considers only the soluble material in particles and therefore neglects any possible insoluble material (Abdul-Razzak and Ghan, 2002). For computing the amount of cloud droplets formed, this is a good assumption, as usually most CCN-sized particles contain a large fraction of soluble material. However, when the insoluble fraction is large (> 0.99), the assumption may lead to an underestimation of scavenged particles. This is because for insoluble particles larger than 1 µm with thin soluble coating (for instance mineral dust), the insoluble fraction is ignored in the cloud activation calculation, and for those particles the activation is calculated as it would be calculated for particles with an equivalent dry size derived from of the soluble part of the particles, thus making them less prone to activation. Therefore, we modified the Abdul-Razzak and Ghan (2002) activation calculations to account for the insoluble core in particles. The calculations are otherwise the same, but the critical supersaturation for each size class is calculated using Eq. (17.38) in Seinfeld and Pandis (2006). The supersaturation calculations, used in the Abdul-Razzak and Ghan (2002) cloud activation, for particles containing an insoluble core are presented in Appendix A. As an input for the in-cloud nucleation scavenging coefficients in ice clouds, we used the ice crystal nucleation scheme described in Lohmann (2002). In our model, only particles which include mineral dust and black carbon are considered as ice nuclei (Lohmann et al., 2007).
As the in-cloud nucleation scavenging was changed into a more functional method, we also needed to alter the calculation of the in-cloud impaction scavenging. We calculate the in-cloud impaction scavenging in SALSA, for liquid clouds, using the same method as described in Croft et al. (2010). This method computes in-cloud impaction as a function of wet aerosol particle size (rp), wet median aerosol particle radius (rpg), and cloud droplet radii (Rliq). Using this same information from our monodisperse size classes for aerosol particles, we can assume that each size class is a log-normal mode, and the in-cloud impaction scavenging coefficients, for liquid clouds, are then obtained as
where Λm(rpg) is the mean mass scavenging coefficient, and it is defined as
and
which is called the scavenging coefficient in inverse time (Croft et al., 2010). In Eqs. (6) and (7) n(rp) is the aerosol number, Rliq is the cloud droplet radius, Ut(Rliq) is the terminal velocity of cloud droplets, E(Rliq,rpg) is the collision efficiency between the aerosol particles and cloud droplets, and n(Rliq) is the cloud droplet number (Croft et al., 2010).
The in-cloud impaction scavenging, for ice clouds, is calculated following Croft et al. (2010), but as our model assumes that the ice crystals are monodisperse, there is no need to integrate over ice crystal number distribution (Croft et al., 2010). Thus, the in-cloud impaction scavenging coefficients are
where Rice is the radius of the ice crystal in its maximum extent, Ut(Rice) is the terminal velocity of the ice crystals. and E(Rice,rpg) is the collection efficiency of the collisions between aerosol particles and ice crystals (Croft et al., 2010).
For below-cloud scavenging, we used the Croft et al. (2009) method, in which we approximated each size class as a log-normal mode. The size-dependent collection efficiency for rain and snow uses an aerosol and collector drop size parameterization described in detail in Croft et al. (2009). Several studies have found that below-cloud scavenging of aerosols does not contribute to the mass deposition budgets as much as in-cloud scavenging does (Croft et al., 2009, 2010; Flossmann and Wobrock, 2010). Thus, we did not analyze below-cloud scavenging separately in our simulations.
2.4 ECHAM-HAMMOZ
For testing the effect of the current wet-scavenging scheme on global aerosol properties, we used the latest stable version of ECHAM-HAMMOZ (ECHAM6.3-HAM2.3-MOZ1.0), a three-dimensional aerosol–chemistry–climate model. ECHAM6.3 is a general circulation model (GCM) and it solves the equations for divergence, temperature, surface pressure, and vorticity (Stier et al., 2005). These large-scale meteorological prognostic variables can be nudged towards data from operational weather forecast models (Stier et al., 2005; Kokkola et al., 2018a).
ECHAM6.3 is coupled with the Hamburg Aerosol Model (HAM), which calculates all of the aerosol properties within ECHAM-HAMMOZ. These properties include emissions, deposition, radiation, and microphysics (Stier et al., 2005; Tegen et al., 2019). HAM has a comprehensive parameterization for both modal and sectional microphysics representations of aerosol populations. In addition to BC, the aerosol compounds included in this study are organic carbon (OC), organic aerosol (OA) (here assumed to be 1.4 times the modeled OC mass), sulfate (SO4), mineral dust (DU), and sea salt (SS). ECHAM6.3 is further coupled to the chemistry model MOZ (not used here), which contains a detailed stratospheric and tropospheric reactive chemistry representation for 63 chemical species, including nitrogen oxides, tropospheric ozone, and hydrocarbons (Schultz et al., 2018; Horowitz et al., 2003). The model does not include secondary organic aerosols. In addition, the model assumes the same aerosol emission size distribution per compound and emission sector throughout the whole world. The SALSA global aerosol module is coupled in the ECHAM-HAMMOZ global climate model for all of the simulations presented in this study.
2.5 Simulations
We used a total of six different simulations to investigate the performance of the current wet deposition scheme. The first two simulations were done with the default wet deposition scheme of SALSA (hereafter referred to as “old”) and the wet deposition scheme introduced in this study (hereafter referred to as “current”). The treatment of aerosol aging is identical in baserun_old and baserun_new; i.e., there is no artificial transfer of insoluble particles to soluble size classes. However, aerosol mass can be transferred from the soluble to the insoluble population through coagulation. As will be shown later, in the default model configuration the current scheme resulted in spurious BC vertical profiles. To investigate the reasons for this, we carried out four additional sensitivity simulations where we changed the assumptions of emission size distribution, as well as internal mixing and aging of BC. A schematic of the aerosol emission number size distribution, (N), as a function of diameter Dp, for the different simulations is presented in Fig. 1. In addition, an overview over the different simulations and their illustrative colors and line styles in the upcoming figures are presented in Table 1.
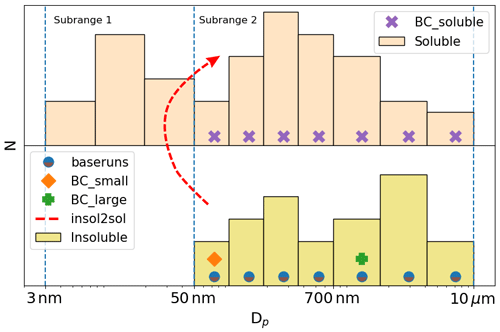
Figure 1Schematic representation of the number size distribution, (N), of aerosols in different simulations as a function of diameter Dp.
In the model simulations, the runs “baserun_new” and “baserun_old” are used to compare the current and old in-cloud scavenging schemes. The simulations “BC_small”, “BC_large”, “BC_soluble”, and “insol2sol” were conducted to evaluate the sensitivity of the current in-cloud scavenging scheme. These sensitivity studies were chosen based on the findings of Kipling et al. (2016), who studied how model processes affect the simulated aerosol vertical profiles. Their study indicated that the processes which have the strongest effect on aerosol vertical profiles in the HadGEM model are emission distribution, hygroscopicity, deposition, and microphysical processes (Kipling et al., 2016).
In the first two sensitivity runs, we altered the BC emission distribution for SALSA. This was done so that all of the BC emissions were directed to either size class of small or large insoluble particles, respectively. In the default configuration the BC emission size distributions are log-normal mass fraction distributions following AEROCOM emission recommendations (Stier et al., 2005; Dentener et al., 2006), which are remapped to the SALSA size classes. The mode radii (rm) and standard deviations σ for the original BC emission size distributions are rm=0.015 µm and σ=1.8, for fossil fuel emissions and rm=0.04 µm and σ=1.8, for wild-fire emissions (Dentener et al., 2006). In the BC_small simulation, we directed all BC emissions to an insoluble size class where particle diameter spans from 50 to 96.7 nm. In the BC_large simulation, we directed all BC emissions to an insoluble size class where particle diameter spans from 0.7 to 1.7 µm.
To study the sensitivity of the wet deposition scheme to BC hygroscopicity, we conducted a simulation where all BC emissions were directed to soluble size classes. The size distribution for the emissions was the same as for the baserun simulations when they are directed to the insoluble classes. This simulation is referred to as BC_soluble in the model simulations. In the fourth sensitivity study, called insol2sol, insoluble particles are transferred to parallel size classes of soluble particles. This allows for the separation of fresh and aged particles and is a method to simulate aerosol aging used also in other global aerosol models (e.g., Stier et al., 2005). The criterion for transfer is that particles activate at a supersaturation of 0.5 %.
2.6 Experimental setup
The simulations were performed with ECHAM-HAMMOZ for the year 2010, with the SALSA aerosol module, using 3-hourly data output, after a 6-month spin-up. The emissions were obtained from the ACCMIP (Emissions for Atmospheric Chemistry and Climate Model Intercomparison Project) emission inventories, which are interpolated, for the period 2000–2100 by using Representative Concentration Pathway 4.5 (RCP4.5) (Lamarque et al., 2010; van Vuuren et al., 2011). The model vorticity, divergence, and surface pressure were nudged towards ERA-Interim reanalysis data provided by ECMWF (European Centre for Medium-Range Weather Forecasts) (Simmons et al., 1989; Berrisford et al., 2011), and the sea surface temperature (SST) and sea ice cover (SIC) were also prescribed. SST and SIC were obtained from monthly mean climatologies from AMIP (Atmospheric Model Intercomparison Project). The analysis is made between the old and the current wet deposition scheme using the ECHAM-HAMMOZ global aerosol–climate model with the SALSA aerosol module. In addition, the sensitivity of the current scheme to emission sizes, aging, and hygroscopicity of BC-containing aerosol is tested using ECHAM-HAMMOZ with SALSA.
2.7 ATom aircraft measurements
To see how the current scheme and the sensitivity studies reproduce the vertical properties of different aerosol compounds, we compared the model simulations against aircraft measurements. The aircraft data were obtained from all NASA's ATom missions (1, 2, 3, and 4), and the dataset was merged data from all instruments which measure atmospheric chemistry, trace gases, and aerosols (Wofsy et al., 2018).
To get the best representative comparison between the ATom aircraft measurements and model data, the model data were sampled to the same time and locations of the aircraft measurements. For the collocation of model vertical profiles with observations, we used the Community Intercomparison Suite (CIS) tool (Watson-Parris et al., 2016).
BC concentrations were measured with a single-particle soot photometer (NOAA) (SP2) and OA and SO4 concentrations with a CU aircraft high-resolution time-of-flight aerosol mass spectrometer (HR-AMS) (Wofsy et al., 2018). The number concentration of particles with a diameter larger than 100 nm, N100, and total number concentration, Ntot were combined from the data measured with a nucleation-mode aerosol size spectrometer (NMASS), an ultra-high-sensitivity aerosol size spectrometer (UHSAS), and a laser aerosol spectrometer (LAS) (Brock et al., 2019; Wofsy et al., 2018).
3.1 Differences between simulated values of old and current wet deposition schemes
First, we compared how aerosol properties differ when using the old and the current wet deposition schemes. In order to assess how the two schemes affect aerosol transport and vertical profiles, we compared the modeled aerosol vertical profiles over the tropics (0–30∘ N), the midlatitudes (30–60∘ N), and the Arctic (60–90∘ N). Here we focused on SO4, OC (or OA), and BC as they are readily available from the ATom aircraft campaign measurements.
Figure 2 shows the vertical profile of BC, OC, and SO4 mass concentration simulated with the old and the current in-cloud wet deposition schemes. The different rows show different latitude bands, as horizontally averaged annual means. The figure illustrates that all three of the compounds show similar differences in the vertical profiles in all three latitude bands, between the two runs. The concentrations for each compound are higher for the current scheme compared to the old scheme for almost the entire vertical domain. The differences between the different wet deposition schemes are greatest at higher altitudes starting from approximately 900 hPa upwards. In the tropics, these differences in the profiles are smaller, compared to the other latitude bands, with a maximum relative difference of approximately 200 % for BC and OC and slightly exceeding 150 % for SO4. These maxima occur at approximately 200 hPa altitude. In the midlatitudes, the differences are slightly higher than in the tropics and the maximum differences in the values are at ∼ 300 hPa altitude. The current method shows ∼ 350 % higher concentrations at maximum for BC and SO4 and ∼ 400 % for OC. The Arctic shows the largest differences in the compound profiles in comparison to the other latitude bands. The difference is largest at ∼ 500 hPa altitude where the concentrations in the current scheme outweigh the concentrations in the old scheme by ∼ 600 % for BC, 650 % for OC, and 800 % for SO4. As emissions of these aerosol particles in the Arctic are low, most aerosol is transported into the Arctic from emission regions outside the Arctic. It is thus evident that the wet removal of these aerosol particles is reduced in the current scheme, which allows for the particles to be transported to higher altitudes and longer distances. In addition, we found that the model accumulates BC at the higher altitudes in simulations spanning several years (not shown), which can be considered spurious behavior.
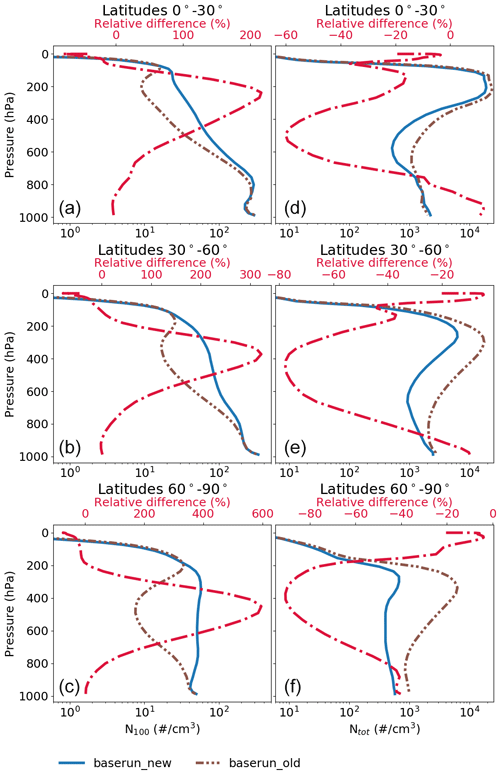
Figure 3Vertical profiles of the N100 (a–c) and Ntot (d–f) concentrations, simulated with old and current in-cloud wet deposition schemes in different latitude regions.
Figure 3 shows the vertical profile of the number concentration of particles with diameters larger than 100 nm, N100, and the total number concentration, Ntot. The N100 profiles show similar differences between the old and the current scheme as for the concentration profiles of different compounds in Fig. 2. In addition, the relative increase in the concentrations in the current wet deposition scheme is similar. This can be explained by changes in nucleation scavenging in the current scheme, which reduces the wet removal of large particles and thus increases the number concentration of large particles. Particles larger than 100 nm act as a condensation sink for H2SO4 and thus an increase in N100 leads to reduced new particle formation (NPF) and thus to decreased number concentrations of small particles. This can be seen in the Ntot profiles, which show a decrease in the current scheme. This difference is most pronounced in the Arctic, where the relative difference between the current and old schemes in the Ntot concentration reaches its maximum of ∼ 90 % at ∼ 400 hPa. In addition, the changes in rates of NPF and impaction scavenging in our current scheme result in an increased removal of small aerosol particles and thus reduce concentrations even more. These effects become evident when looking at size-resolved wet deposition fluxes.
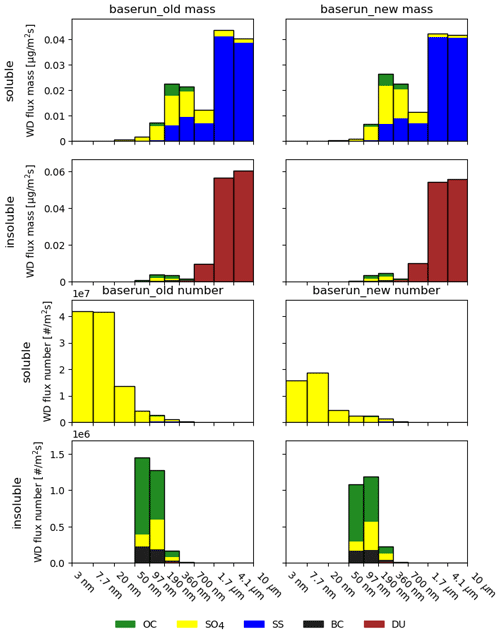
Figure 4Wet deposition flux size distributions of different aerosol compounds simulated with old (left column) and current (right column) in-cloud wet deposition schemes. The top four panels show the wet deposition flux for the mass distribution and the lower four for the number distribution. Different rows show values for the different solubility types.
The annual and global average size distributions of the wet deposition flux of the old and current in-cloud scavenging schemes are presented in Fig. 4. The wet deposition size distributions confirm what has been observed in the vertical aerosol profiles. There are only modest changes in the mass fluxes between the old and the current schemes. In the soluble population the largest difference is in the size class, which spans diameters between 190–360 nm, where the current scheme exceeds the value of the old scheme by 0.003 µg (m2 s)−1. On the other hand, in the size class 1.7–4.1 µm, the old scheme has a higher value by 0.002 µg (m2 s)−1. In the insoluble population the current scheme exceeds the value of the old scheme by approximately 0.002 µg (m2 s)−1 in the size class 190–360 nm, but in the largest size class the value of the old scheme is higher by 0.005 µg (m2 s)−1. As in steady state the total emissions of a compound must match its total removal, these differences mostly stem from changes in the interplay between dry- and wet deposition processes. However, the number flux in smaller than 50 nm size classes of the soluble population is halved, affecting mainly the removal of SO4 in the smallest size classes. In addition, there is a small increase of approximately 106 (m2 s)−1 in the current scheme in the size class between 190 and 360 nm. For larger than 360 nm size classes the changes are insignificant. These results can be explained by increased concentrations of medium-sized and large particles in the current scheme, which act as a condensation sink for SO4. This leads to fewer small particles as they are mainly formed through NPF from gaseous H2SO4. This effect can also be seen in Fig. 4 as a slight increase in removed sulfate mass in the accumulation-sized particles of both the soluble and insoluble aerosol populations. As a consequence of the atmospheric concentration of small particles, the wet deposition flux for the smallest size classes is reduced in the current scheme compared to the old.
The lifetime of different aerosol compounds was calculated by dividing the annual mean global mass burden of each compound by the annual mean emissions of the same compound (Lund et al., 2018). The lifetimes for different compounds can be found in Table 2. The global mean lifetime for BC was 9.23 d for the old scheme and 14.62 d for the current scheme. However, experimental studies from different aircraft campaigns indicate that the BC lifetime should be less than 5.5 d (Lund et al., 2018). This is a very interesting result: the more physical wet deposition scheme produces more spurious atmospheric lifetimes for BC. Consequently, the ability of the ECHAM-HAMMOZ global climate model, with the SALSA aerosol module, to reliably simulate aerosol vertical profiles and long-range transport of aerosol is also decreased when using the more physical scheme with the default model setup. This may be due to the fact that a more physical treatment of the wet deposition processes makes the model more sensitive to influences outside of the parameterization. We therefore performed further sensitivity simulations and compared their results to observational data.
3.2 Sensitivity simulations
As reported in the previous section, ECHAM-HAMMOZ, using the SALSA aerosol module, with the current, more physical scheme, in its default setup, produced spuriously long lifetimes of all aerosol compounds, especially BC. With the sensitivity simulations we aimed to explore different possibilities to improve the BC vertical profiles and long-range transport in the model. In order to increase nucleation scavenging of BC, we considered three different possibilities to make BC-containing particles more susceptible to cloud droplet activation. One way to achieve this is to emit BC into larger particles, which require less aging to be activated at a given supersaturation. This was tested in simulation BC_large. Another way is to mix BC with soluble compounds in order to enhance hygroscopicity of BC-containing particles and thus their cloud activation susceptibility. This can be done in two ways: either by emitting BC directly to soluble size classes (simulation BC_soluble) or by emitting BC to insoluble size classes and transferring particles to soluble classes after aging (simulation insol2sol). A third way is to emit BC into smaller size classes in order to facilitate the transfer of BC into larger, more easily activated particles by coagulation (simulation BC_small).
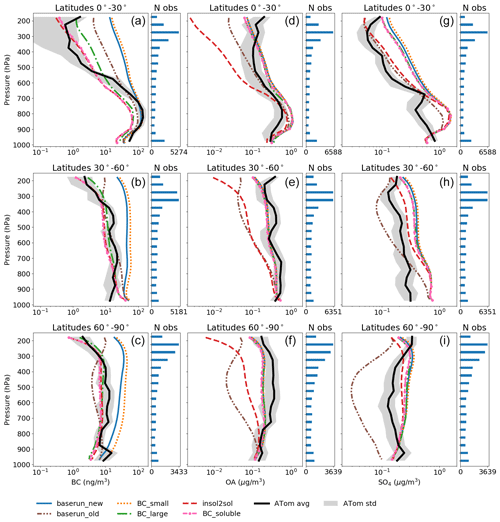
Figure 5Mean vertical profiles of BC (a–c), OA (d–f), and SO4 (g–i), modeled with different studies, compared to the mean of ATom aircraft measurements at different latitude bands. To the right of every panel is the number of observations measured by the device, at each vertical level, from the ATom aircraft measurement campaigns. Note the different units for the different compounds.
Figure 5 shows vertical profiles of BC, OA, and SO4 simulated with the current wet deposition scheme for the different sensitivity simulations and with the old scavenging scheme, together with the average values from ATom aircraft measurements. The gray shaded area shows the standard deviation for the aircraft measurements. For BC, the simulations baserun_old, BC_large, BC_soluble, and insol2sol show a better match with observed vertical profiles than the other simulations in every latitude band. These simulations fall between the standard deviation limits of the ATom aircraft simulations almost everywhere, with the exception of the tropics, where they underestimate the concentrations starting from ∼ 600 hPa downwards. In addition, in the tropics, BC_soluble and insol2sol represent the BC concentrations slightly better than BC_large and baserun_old between 500 and 300 hPa. BC_small and baserun_new overestimate the BC concentrations at all latitudes, except in the tropics at lower altitudes starting from ∼ 700 hPa downwards, where they represent the BC concentrations slightly better than the other sensitivity simulations. As we saw in the previous chapter, the reduced efficiency in the wet deposition increases BC concentrations at higher altitudes, which causes baserun_new to overestimate the BC concentrations. This is because the default emission sizes of BC particles are not very susceptible to cloud activation. In addition, although BC_small aimed at increasing BC wet removal by emitting BC to small particle sizes and thus enhancing their collection by coagulation to large particles, it is apparent that coagulation is not very efficient in doing so.
Compared to baserun_new, most of the sensitivity studies show better agreement of the modeled BC profiles with the measurements. However, it needs to also be checked how they affect OA and SO4 concentrations. At all latitude regions OA concentrations in all of the simulations show similar results as the measurements. Exceptions exist for insol2sol and baserun_old simulations, which underestimate OA concentrations in the midlatitudes as well as at higher altitudes in the tropics and the Arctic. In the tropics the insol2sol simulation underestimates OA concentrations starting from approximately 700 hPa upwards and baserun_old from approximately 400 hPa upwards. In addition, the old scheme underestimates the OA concentrations at higher altitudes in the midlatitudes and the Arctic. The shape of the curve of the old scheme is different compared to observations and the rest of the simulations, especially in the Arctic. The old scheme exhibits a concentration minimum between 400 and 500 hPa, while observations are near the maximum values at those altitudes. At most, insol2sol underestimates the measurements at the highest altitudes, in all of the latitude bands, where the concentrations are over 1 order of magnitude less than the measurements. As the aging of aerosol particles in insol2sol is simulated by moving all insoluble particles that can activate to cloud droplets at 0.5 % supersaturation, almost all OA that is originally emitted to insoluble size classes is moved to soluble size classes. Thus, this enhances the activation and consequently the wet deposition of OA. Faster wet removal reduces the amount of OA transported to higher altitudes and thus reduces the OA concentrations. OA concentrations from all other simulations fall between the standard deviation limits of the ATom aircraft measurements everywhere, with only a slight overestimation between approximately 900 and 800 hPa in the tropics.
For SO4, all of the sensitivity simulations show similar trends as the measurements but overestimate concentrations almost everywhere. In the tropics, the shape of the vertical profile in baserun_old is similar to the observations and the rest of the simulations. In the midlatitudes, the vertical profile in baserun_old shows stronger variation than observations and the rest of the simulations, overestimating the values below 800 hPa and overestimating them above 600 hPa. Over the Arctic, baserun_old underestimates concentrations throughout the whole column, the maximum difference to observed values being almost 1 order of magnitude. The effect that insol2sol has on OA concentrations is also visible in the SO4 profiles, but here the effect is much weaker. In the tropics, insol2sol and baserun_old show better agreement with the measurements from 700 hPa upwards than the other simulations, with only a slight overestimation. Between approximately 900 and 700 hPa, all of the simulations overestimate the measurements. This may be due to simplified sulfate chemistry in the model as SO4 is mainly formed through chemical transformation (Feichter et al., 1996). In the midlatitudes, all simulations overestimate the SO4 concentrations, with the exception of insol2sol and baserun_old. The insol2sol reproduces the SO4 profile slightly better than the other simulations from approximately 600 hPa upwards. However, near the surface, all simulations overestimate the SO4 concentrations by approximately half an order of magnitude. In the Arctic, all of the simulations have similar SO4 profiles with a slight overestimation between approximately 700 and 300 hPa altitude, with the exception of baserun_old. In addition, at the highest altitudes all of the simulations underestimate the SO4 concentrations. The different sensitivity tests do not alter the SO4 concentrations much compared to baserun_new because most of it condenses onto soluble particles. In addition, the new particles formed through nucleation are added to the soluble aerosol population. Thus, the SO4 vertical profiles are similar in all of the sensitivity simulations, with the exception of insol2sol where some of the SO4, which repartitions from the insoluble to the soluble population, is activated more efficiently.
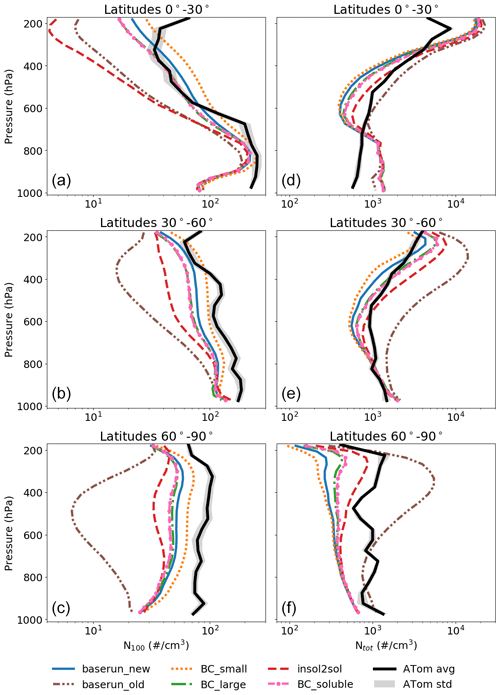
Figure 6Mean vertical profiles of the N100 (a–c) and Ntot (d–f) concentrations, modeled with different studies, compared to the mean of ATom aircraft measurements in different latitude regions.
Figure 6 shows the vertical profiles of N100 and Ntot, simulated with different studies, together with ATom aircraft measurements. From the figure we can see that N100 profiles between different sensitivity simulations are similar in the midlatitudes and the Arctic. In these latitude bands, the sensitivity simulations slightly underestimate the N100 concentrations when compared to the measurements, but the trend is similar throughout the entire vertical column. However, insol2sol underestimates the N100 profiles slightly more in the midlatitudes and the Arctic. In addition, baserun_old underestimates N100 profiles even more than the other simulations, especially in the Arctic, where the maximum difference occurs at approximately 500 hPa altitude and is more than 90 cm−3 less than observed values. In the tropics, the simulations show a good correlation with the measurements as almost all of the profiles follow the shape of the profile of the ATom aircraft measurements, except for the surface concentrations, which are underestimated by a factor of approximately 2.5 compared to the measurements. In addition, in the tropics, insol2sol and baserun_old underestimate N100 more than the other simulations from 800 hPa upwards. For insol2sol, this is also due to more efficient activation compared to baserun_new for medium-sized particles which reduces the transport to higher altitudes.
The Ntot profiles are similar in shape in all sensitivity simulations, with only a modest difference (600 cm−3 at maximum), mostly at higher altitudes. In the tropics the trend of the profiles varies between simulations and measurements. All of the simulations tend to overestimate the Ntot concentrations at the surface and at the highest altitudes by over 50 %. However, they underestimate the Ntot concentrations at approximately 400–700 hPa, with the exception of baserun_old, which overestimates these concentrations. In the midlatitudes, all of the simulations represent Ntot concentrations fairly well (approximately 500 cm−3 underestimation and 4000 cm−3 overestimation at most) when compared to the measurements, with the exception of baserun_old, which overestimates these concentrations at all altitudes with almost 1 order of magnitude at maximum. However, in the Arctic, all of the sensitivity simulations underestimate the Ntot profiles. At higher altitudes, starting from approximately 600 hPa upwards, insol2sol underestimates Ntot least, showing quite good agreement with the measurements with only around 300 cm−3 difference at most. The baserun_old simulation, on the other hand, shows good agreement with the measurements at highest altitudes and below 600 hPa but overestimates the Ntot profile between 600 and 200 hPa by over 5000 cm−3 at most.
One of the reasons for the differences in the Ntot and N100 surface concentrations may be due to a misrepresentation of the emitted particle size distribution. In ECHAM-HAMMOZ the same aerosol emission size distribution per compound and emission sector is assumed throughout the whole world, which is not very realistic for every aerosol particle source (Paasonen et al., 2016). At higher altitudes, the aerosol microphysical processes correct the aerosol size distribution towards more realistic profiles.
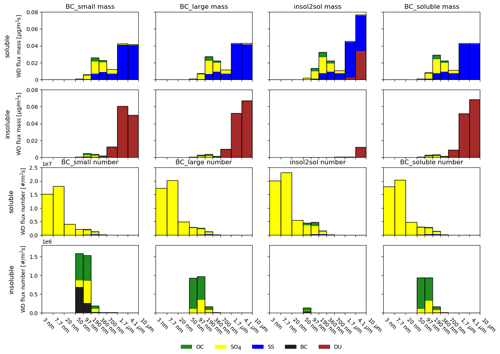
Figure 7Wet deposition flux size distributions of different aerosol compounds simulated with different sensitivity simulations. Each column represents a different sensitivity study and each row the solubility type. The top two rows show the mass size distribution of the wet deposition flux and the bottom two rows the number size distribution.
To investigate the effects of the different sensitivity studies further, we computed the size and mass distribution of the wet deposition flux (Fig. 7). The mass fluxes in the soluble population do not change much between baserun_new and the different sensitivity studies, except for the insol2sol simulation, which allows for sufficiently hygroscopic particles of the insoluble population to be repartitioned to the soluble population. This leads to an increase in DU mass in the soluble population and a decrease in the insoluble population. In addition to more efficient wet removal of DU due to this process, this also increases dry deposition and sedimentation (not shown) of DU in insol2sol. For the mass fluxes in the insoluble population, BC_large and BC_soluble show an increase in the largest size class for DU. This effect is due to more efficient removal of BC-containing particles, which allows for more SO4 to condense on larger, DU-containing particles, which enhances the activation of these particles.
The number fluxes in the soluble population for the different sensitivity simulations show most change in the two smallest size classes, which increase by a factor of approx 1.3 in the insol2sol simulation and approximately 1.1 for BC_large and BC_soluble when compared to baserun_new (shown in Fig. 4). These differences stem from changes in medium-sized and large particle concentrations, which act as a condensation sink for SO4 and thereby regulate the amount of SO4 available for new particle formation. In addition, there is a slight increase in OC in the insol2sol-simulated number distribution, which is being transferred from the insoluble population. Otherwise, there is no notable change in other compounds as the SO4 dominates the number distribution in the soluble population. The relative BC mass contribution to the wet deposition number flux of the insoluble aerosol population reflects the assumptions made in the different sensitivity studies very well. While for BC_large and BC_soluble the BC mass fraction in the medium-sized insoluble particles disappears, in BC_small the BC fraction in the 50 to 100 nm insoluble particles is about 3 times larger than in baserun_new (shown in Fig. 4). This shows that coagulation is not effective in moving BC from these small insoluble particles to large soluble particles. In insol2sol, most of the BC is transferred from the insoluble to the soluble aerosol population before removal, which can be seen in a strong decrease in removed insoluble aerosol number for that simulation.
In addition to the evaluation of the simulated vertical aerosol profiles, we used the modeled atmospheric lifetimes of all aerosol compounds as an indicator of the model skill in the different simulations. Here we estimated the atmospheric lifetime of a compound as the yearly and global mean mass burden of the compound divided by its total yearly mean emission. The compiled mean lifetimes for the different simulations and compounds as well as the mean and spread of lifetimes from several AEROCOM models (CAM5-ATRAS, EC-Earth, TM5, ECHAM-HAM, ECHAM-SALSA, ECMWF-IFS, EMEP, GEOS, GFDL-AM4, GISS-OMA, INCA, NorESM2, OsloCTM3, and SPRINTARS) are presented in Table 2 (Gliß et al., 2020). The spread is calculated as half the difference between the first and third quantiles (Gliß et al., 2020).
Table 2Lifetimes of compounds from different simulations as well as mean and spread from different AEROCOM models.

With the assumption that the AEROCOM mean atmospheric lifetimes are the current best guess, we can use Table 2 to select a simulation that best reproduces these mean lifetimes and therefore could be regarded as the best solution to address the overestimated BC lifetimes in baserun_new. However, we must keep in mind that AEROCOM means are global-climate-model-based results, and thus it is not completely certain that these lifetimes of different compounds reflect the actual lifetimes in the real atmosphere. While baserun_old, baserun_new, and BC_small overestimate the BC lifetime by factors of 1.6, 2.5, and 2.8, respectively, BC_large, insol2sol, and BC_soluble all produce BC lifetimes within 1 d of the AEROCOM mean. In addition, the BC lifetimes should be less than 5.5 d according to Lund et al. (2018). However, the different sensitivity studies also affect the atmospheric lifetimes of the other species, and some of them considerably. For instance, the lifetime of DU in insol2sol is almost 4.5 times shorter than the AEROCOM mean, while both BC_large and BC_soluble overestimate this mean only slightly by half a day. On the other hand, the atmospheric lifetime of OC in insol2sol is closest to the AEROCOM mean compared to all other simulations using the current wet deposition scheme. However, in this setup of ECHAM-HAMMOZ all OC is emitted as primary particles, while in reality a large fraction of the organic aerosol is formed as secondary organic aerosol (SOA) in the atmosphere. Modeling the processes leading to SOA formation more realistically would most likely affect the modeled OC lifetimes quite substantially. The atmospheric lifetime of SO4 in insol2sol is also closest to the AEROCOM mean, but BC_large and BC_soluble also model the SO4 lifetime fairly well. For SS, the atmospheric lifetime does not change when changing the wet removal algorithm or during any of the sensitivity tests as SS is only emitted to the soluble population. The lifetimes for all simulations are more than 0.7 d higher than the AEROCOM mean (about a factor of 2). This has already been discussed by Kokkola et al. (2018a) and Tegen et al. (2019).
We developed an in-cloud nucleation wet deposition scheme for liquid and ice clouds. For liquid clouds, the scavenging coefficients are calculated using the size-segregated fraction of activated particles from a cloud activation scheme. For ice clouds, the scavenging coefficients are calculated based on the surface area concentration of each size class (see Tabazadeh et al., 2002).
We used the SALSA microphysics scheme coupled with the ECHAM-HAMMOZ global aerosol–chemistry–climate model to evaluate the differences between the old and current wet deposition schemes. In addition, we used ECHAM-HAMMOZ with SALSA to test the sensitivity of the simulated aerosol concentrations to model assumptions of emission sizes, mixing, and aging when the current in-cloud wet deposition scheme was used. In its original setup, SALSA used fixed scavenging coefficients for modeling wet deposition. Here, we used the Abdul-Razzak and Ghan (2002) cloud activation scheme for the calculations of size-dependent nucleation scavenging coefficients in liquid clouds. For ice clouds, we used the scheme of Lohmann (2002) for providing the ice nucleation rates for the nucleation scavenging scheme (see Tabazadeh et al., 2002). The in-cloud impaction scavenging for SALSA was adapted from the method for the modal scheme by Croft et al. (2010).
Compared to using fixed scavenging coefficients, the current scheme showed an increase in BC, OA, and SO4 vertical profiles almost throughout the entire vertical domain for all latitude bands. In the Arctic region this increase was most pronounced, with a maximum increase of up to 800 %. The differences in vertical profiles had similar functional shapes in all latitude bands and for all three compounds. The increase was mainly due to a decrease in the nucleation scavenging of aerosol particles in the current scheme, which increased aerosol transport into the upper atmosphere and subsequently to the Arctic region. The current scheme also showed a significant increase of up to 600 % at maximum in the number concentration of particles larger than 100 nm, which was similar in shape to the change in aerosol compound mass. However, the number concentration of particles smaller than 100 nm decreased everywhere, with a maximum decrease of 90 % in the Arctic. This could imply that new particle formation was reduced in the current scheme due to the increased concentration of large particles, which increased the condensation sink for SO4. In addition, the changes in impaction scavenging rates in the current scheme compared to the original setup can reduce the number concentration of particles smaller than 100 nm (Croft et al., 2010).
An evaluation of the current wet deposition scheme against ATom aircraft measurements showed that, using the default setup of the host model, the current scheme overestimated BC mass concentrations, especially at higher altitudes. Additional sensitivity simulations showed that the model skill of reproducing measured vertical BC mass concentration profiles could be improved a lot by directing the BC emissions to larger or to more soluble size classes or by transferring BC-containing particles to soluble size classes after aging. These sensitivity studies also produced BC atmospheric lifetimes which were closest to the AEROCOM model mean (Gliß et al., 2020). Emitting BC to smaller size classes, on the other hand, overestimated the aerosol mass concentrations and BC atmospheric lifetime even more. However, changing the distribution of BC in the sensitivity simulations also affected the mass concentrations of other aerosol compounds. For instance, transferring insoluble particles to soluble size classes after aging led to an underestimation of the observed OA concentrations at higher altitudes, while in the other simulations OA concentrations fell between the standard deviation limits of ATom measurements almost everywhere. The modeled atmospheric lifetime of OA, on the other hand, compared best to the AEROCOM mean when transferring aged insoluble particles to soluble size classes. However, as in this study secondary processes of OA formation were neglected, we did not use OA as an indicator for the skill of our wet deposition scheme. For SO4, the insoluble-to-soluble transfer reproduced the observed concentrations slightly better at higher altitudes in the tropics. Nevertheless, all simulations showed similar results for SO4 concentrations, with only a slight overestimation when compared to the aircraft observations. In addition, SO4 atmospheric lifetimes did not vary much across the different sensitivity studies. All of the sensitivity studies reproduced aerosol number concentration profiles fairly well. However, the insoluble-to-soluble transfer considerably underestimated the concentrations of activation-sized particles at the highest altitudes in the tropics, which was strongly tied to the underestimation of OC at these altitudes. Furthermore, the atmospheric lifetime of atmospheric mineral dust (DU) was strongly underestimated in the simulation using insoluble-to-soluble transfer of aged particles. The atmospheric lifetimes of SS did not change between the different sensitivity studies. All in all, while reasonable BC vertical profiles and atmospheric lifetimes could be achieved with the current wet deposition scheme in three of the sensitivity studies, namely emitting BC to more hygroscopic or to larger particles or transferring insoluble, BC-containing particles, to soluble size classes, only the first option is really suitable. Emitting BC to large particles is quite unrealistic because the emission size of BC-containing particles is fairly well established (Tissari et al., 2008; Krecl et al., 2017; Corbin et al., 2018; Zhang et al., 2019) and insoluble-to-soluble transfer, on the other hand, leads to atmospheric lifetimes of DU that are too small.
To conclude, even though the current in-cloud wet deposition scheme is more physically sound than using fixed scavenging coefficients, it failed to reproduce global aerosol fields adequately in the default setup of the host model. This can be seen from the spuriously long lifetimes of all aerosol species. In particular, the BC atmospheric lifetime was almost 3 times as large as what observations indicate (Lund et al., 2018). Based on the results of our sensitivity simulations, the ECHAM-HAMMOZ global climate model with the SALSA aerosol module produces the best vertical profiles and aerosol lifetimes with the current scheme if BC is mixed with more soluble compounds at emission time. In the future, model development should include the study of the effects of the gas-to-particle partitioning of semivolatile compounds which could have a significant impact on the modeled aerosol vertical profiles. In addition, the issue of the level of mixing of BC with soluble compounds during emissions and in the subgrid-scale processing should be further investigated.
The calculations for the particles containing an insoluble core are based on the technical report by Kokkola et al. (2008), where the critical supersaturation is obtained as
where
and
where Dp,0 is the diameter of the insoluble core.
In Eq. (A3), A and B are obtained from Seinfeld and Pandis (2006). A describes the increase in water vapor pressure due to the curvature of the particle surface and is denoted as
where Mw is the molecular weight of water, ρw is the density of water, σw is the surface tension of the droplet, T is the temperature, and R is the universal gas constant. B is called the solute effect term and is denoted as
where ns is the moles of solute in a droplet.
Using this new expression for the critical supersaturation, the effective critical supersaturation, maximum supersaturation, and the number fraction of activated particles for each size class can be calculated using Eqs. (8), (9), and (12)–(15) from Abdul-Razzak and Ghan (2002).
The stand-alone zero-dimensional version of SALSA2.0 is distributed under the Apache-2.0 license and the code is available at https://github.com/UCLALES-SALSA/SALSA-standalone/releases/tag/2.0 (last access: 23 May 2018; Kokkola et al., 2018b) with https://doi.org/10.5281/zenodo.1251668.
The ECHAM6-HAMMOZ model is made available to the scientific community under the HAMMOZ Software License Agreement, which defines the conditions under which the model can be used. The license can be downloaded from https://redmine.hammoz.ethz.ch/attachments/291/License_ECHAM-HAMMOZ_June2012.pdf (last access: 29 June 2012; HAMMOZ consortium, 2012).
The model data can be reproduced using the model revision r5511 from the repository https://redmine.hammoz.ethz.ch/projects/hammoz/repository/changes/echam6-hammoz/branches/fmi/fmi_trunk (last access: 8 March 2019; HAMMOZ consortium, 2019a, b). The settings for the simulations are given in the same folder (“gmd-2020-220”).
The data for reproducing the figures and codes for the figures can be obtained directly from authors or from https://etsin.fairdata.fi/dataset/f3cb5807-66fe-4a0d-a20a-ac208d3aab5a (last access: 29 June 2020; Holopainen et al., 2020) with https://doi.org/10.23729/301df277-8147-4700-8652-ca491f2b58a6. All other input files are ECHAM-HAMMOZ standard and are available from the HAMMOZ repository (see https://redmine.hammoz.ethz.ch/projects/hammoz; HAMMOZ consortium, 2019a, b).
ATom aircraft data can be obtained through the Oak Ridge National Laboratory (ORNL) Distributed Active Archive Center (DAAC) https://daac.ornl.gov/cgi-bin/dsviewer.pl?ds_id=1581 (last access: 25 November 2019; Wofsy et al., 2018) with https://doi.org/10.3334/ORNLDAAC/1581.
EH, TK, and HK designed the outline of the paper. EH wrote the majority of the paper. EH performed all the climate simulations. EH, TK, and HK developed the current wet deposition scheme. TK and HK provided the calculations for particles containing an insoluble core. EH and AL modified the emission distributions for the sensitivity simulations. EH, TK, HK, and AL performed the data analysis for the climate simulations, and EH produced the figures. All the authors contributed to the writing of the paper.
The authors declare that they have no conflict of interest.
ECHAM-HAMMOZ is developed by a consortium composed of ETH Zürich, Max Planck Institut für Meteorologie, Forschungszentrum Jülich, University of Oxford, the Finnish Meteorological Institute, and the Leibniz Institute for Tropospheric Research and managed by the Center for Climate Systems Modeling (C2SM) at ETH Zürich. We thank NASA/ORNL DAAC for Atmospheric Tomography Mission (ATom) aircraft data.
This research has been supported by the Academy of Finland, Luonnontieteiden ja Tekniikan Tutkimuksen Toimikunta (grant nos. 317373, 308292), the European Research Council (CLIMASLOW, grant no. 678889), and the Tiina and Antti Herlin Foundation (grant no. 20190014).
This paper was edited by Jason Williams and reviewed by two anonymous referees.
Abdul-Razzak, H. and Ghan, S.: A parameterization of aerosol activation. Part 3: Sectional representation, J. Geophys. Res., 107, 1–6, https://doi.org/10.1029/2001JD000483, 2002. a, b, c, d, e, f, g, h
Albrecht, B. A.: Aerosols, Cloud Microphysics, and Fractional Cloudiness, Science, 245, 1227–1230, https://doi.org/10.1126/science.245.4923.1227, 1989. a
AMAP: AMAP assessment 2015: Black carbon and ozone as Arctic climate forcers, vol. 7, Arctic Monitoring and Assessment Programme (AMAP),, Oslo, Norway, 2015. a
Andersson, C., Bergström, R., Bennet, C., Robertson, L., Thomas, M., Korhonen, H., Lehtinen, K. E. J., and Kokkola, H.: MATCH-SALSA – Multi-scale Atmospheric Transport and CHemistry model coupled to the SALSA aerosol microphysics model – Part 1: Model description and evaluation, Geosci. Model Dev., 8, 171–189, https://doi.org/10.5194/gmd-8-171-2015, 2015. a
Andronache, C.: Estimated variability of below-cloud aerosol removal by rainfall for observed aerosol size distributions, Atmos. Chem. Phys., 3, 131–143, https://doi.org/10.5194/acp-3-131-2003, 2003. a
Andronache, C., Grönholm, T., Laakso, L., Phillips, V., and Venäläinen, A.: Scavenging of ultrafine particles by rainfall at a boreal site: observations and model estimations, Atmos. Chem. Phys., 6, 4739–4754, https://doi.org/10.5194/acp-6-4739-2006, 2006. a
Archuleta, C. M., DeMott, P. J., and Kreidenweis, S. M.: Ice nucleation by surrogates for atmospheric mineral dust and mineral dust/sulfate particles at cirrus temperatures, Atmos. Chem. Phys., 5, 2617–2634, https://doi.org/10.5194/acp-5-2617-2005, 2005. a
Barahona, D. and Nenes, A.: Parameterization of cloud droplet formation in large-scale models: Including effects of entrainment, J. Geophys. Res.-Atmos., 112, D16206, https://doi.org/10.1029/2007JD008473, 2007. a
Bergman, T., Kerminen, V.-M., Korhonen, H., Lehtinen, K. J., Makkonen, R., Arola, A., Mielonen, T., Romakkaniemi, S., Kulmala, M., and Kokkola, H.: Evaluation of the sectional aerosol microphysics module SALSA implementation in ECHAM5-HAM aerosol-climate model, Geosci. Model Dev., 5, 845–868, https://doi.org/10.5194/gmd-5-845-2012, 2012. a, b
Berrisford, P., Dee, D., Poli, P., Brugge, R., Fielding, K., Fuentes, M., Kållberg, P., Kobayashi, S., Uppala, S., and Simmons, A.: The ERA-Interim archive Version 2.0, Shinfield Park, Reading, 2011. a
Bond, T. C., Doherty, S. J., Fahey, D. W., Forster, P. M., Berntsen, T., DeAngelo, B. J., Flanner, M. G., Ghan, S., Kärcher, B., Koch, D., Kinne, S., Kondo, Y., Quinn, P. K., Sarofim, M. C., Schultz, M. G., Schulz, M., Venkataraman, C., Zhang, H., Zhang, S., Bellouin, N., Guttikunda, S. K., Hopke, P. K., Jacobson, M. Z., Kaiser, J. W., Klimont, Z., Lohmann, U., Schwarz, J. P., Shindell, D., Storelvmo, T., Warren, S. G., and Zender, C. S.: Bounding the role of black carbon in the climate system: A scientific assessment, J. Geophys. Res.-Atmos., 118, 5380–5552, https://doi.org/10.1002/jgrd.50171, 2013. a, b
Bourgeois, Q. and Bey, I.: Pollution transport efficiency toward the Arctic: Sensitivity to aerosol scavenging and source regions, J. Geophys. Res., 116, D08213, https://doi.org/10.1029/2010JD015096, 2011. a, b
Brock, C. A., Williamson, C., Kupc, A., Froyd, K. D., Erdesz, F., Wagner, N., Richardson, M., Schwarz, J. P., Gao, R.-S., Katich, J. M., Campuzano-Jost, P., Nault, B. A., Schroder, J. C., Jimenez, J. L., Weinzierl, B., Dollner, M., Bui, T., and Murphy, D. M.: Aerosol size distributions during the Atmospheric Tomography Mission (ATom): methods, uncertainties, and data products, Atmos. Meas. Tech., 12, 3081–3099, https://doi.org/10.5194/amt-12-3081-2019, 2019. a
Browse, J., Carslaw, K. S., Arnold, S. R., Pringle, K., and Boucher, O.: The scavenging processes controlling the seasonal cycle in Arctic sulphate and black carbon aerosol, Atmos. Chem. Phys., 12, 6775–6798, https://doi.org/10.5194/acp-12-6775-2012, 2012. a
Chate, D., Rao, P., Naik, M., Momin, G., Safai, P., and Ali, K.: Scavenging of aerosols and their chemical species by rain, Atmos. Environ., 37, 2477–2484, https://doi.org/10.1016/S1352-2310(03)00162-6, 2003. a, b
Chate, D., Murugavel, P., Ali, K., Tiwari, S., and Beig, G.: Below-cloud rain scavenging of atmospheric aerosols for aerosol deposition models, Atmospheric Research, 99, 528–536, https://doi.org/10.1016/j.atmosres.2010.12.010, 2011. a
Chen, J.-P. and Lamb, D.: Simulation of Cloud Microphysical and Chemical Processes Using a Multicomponent Framework. Part I: Description of the Microphysical Model, J. Atmosp. Sci., 51, 2613–2630, https://doi.org/10.1175/1520-0469(1994)051<2613:SOCMAC>2.0.CO;2, 1994. a
Corbin, J. C., Pieber, S. M., Czech, H., Zanatta, M., Jakobi, G., Massabò, D., Orasche, J., El Haddad, I., Mensah, A. A., Stengel, B., Drinovec, L., Mocnik, G., Zimmermann, R., Prévôt, A. S. H., and Gysel, M.: Brown and Black Carbon Emitted by a Marine Engine Operated on Heavy Fuel Oil and Distillate Fuels: Optical Properties, Size Distributions, and Emission Factors, J. Geophys. Res.-Atmos., 123, 6175–6195, https://doi.org/10.1029/2017JD027818, 2018. a
roft, B., Lohmann, U., Martin, R. V., Stier, P., Wurzler, S., Feichter, J., Posselt, R., and Ferrachat, S.: Aerosol size-dependent below-cloud scavenging by rain and snow in the ECHAM5-HAM, Atmos. Chem. Phys., 9, 4653–4675, https://doi.org/10.5194/acp-9-4653-2009, 2009. a, b, c, d
Croft, B., Lohmann, U., Martin, R. V., Stier, P., Wurzler, S., Feichter, J., Hoose, C., Heikkilä, U., van Donkelaar, A., and Ferrachat, S.: Influences of in-cloud aerosol scavenging parameterizations on aerosol concentrations and wet deposition in ECHAM5-HAM, Atmos. Chem. Phys., 10, 1511–1543, https://doi.org/10.5194/acp-10-1511-2010, 2010. a, b, c, d, e, f, g, h, i, j, k, l, m, n, o, p, q
Croft, B., Martin, R. V., Leaitch, W. R., Tunved, P., Breider, T. J., D'Andrea, S. D., and Pierce, J. R.: Processes controlling the annual cycle of Arctic aerosol number and size distributions, Atmos. Chem. Phys., 16, 3665–3682, https://doi.org/10.5194/acp-16-3665-2016, 2016. a, b, c
de Bruine, M., Krol, M., van Noije, T., Le Sager, P., and Röckmann, T.: The impact of precipitation evaporation on the atmospheric aerosol distribution in EC-Earth v3.2.0, Geosci. Model Dev., 11, 1443–1465, https://doi.org/10.5194/gmd-11-1443-2018, 2018. a
Dentener, F., Kinne, S., Bond, T., Boucher, O., Cofala, J., Generoso, S., Ginoux, P., Gong, S., Hoelzemann, J. J., Ito, A., Marelli, L., Penner, J. E., Putaud, J.-P., Textor, C., Schulz, M., van der Werf, G. R., and Wilson, J.: Emissions of primary aerosol and precursor gases in the years 2000 and 1750 prescribed data-sets for AeroCom, Atmos. Chem. Phys., 6, 4321–4344, https://doi.org/10.5194/acp-6-4321-2006, 2006. a, b
Easter, R. C., Ghan, S. J., Zhang, Y., Saylor, R. D., Chapman, E. G., Laulainen, N. S., Abdul-Razzak, H., Leung, L. R., Bian, X., and Zaveri, R. A.: MIRAGE: Model description and evaluation of aerosols and trace gases, J. Geophys. Res.-Atmos., 109, D20210, https://doi.org/10.1029/2004JD004571, 2004. a
Eckhardt, S., Quennehen, B., Olivié, D. J. L., Berntsen, T. K., Cherian, R., Christensen, J. H., Collins, W., Crepinsek, S., Daskalakis, N., Flanner, M., Herber, A., Heyes, C., Hodnebrog, Ø., Huang, L., Kanakidou, M., Klimont, Z., Langner, J., Law, K. S., Lund, M. T., Mahmood, R., Massling, A., Myriokefalitakis, S., Nielsen, I. E., Nøjgaard, J. K., Quaas, J., Quinn, P. K., Raut, J.-C., Rumbold, S. T., Schulz, M., Sharma, S., Skeie, R. B., Skov, H., Uttal, T., von Salzen, K., and Stohl, A.: Current model capabilities for simulating black carbon and sulfate concentrations in the Arctic atmosphere: a multi-model evaluation using a comprehensive measurement data set, Atmos. Chem. Phys., 15, 9413–9433, https://doi.org/10.5194/acp-15-9413-2015, 2015. a
Feichter, J., Kjellström, E., Rodhe, H., Dentener, F., Lelieveldi, J., and Roelofs, G.-J.: Simulation of the tropospheric sulfur cycle in a global climate model, Atmos. Environ., 30, 1693–1707, https://doi.org/10.1016/1352-2310(95)00394-0, 1996. a
Flossmann, A. I. and Wobrock, W.: A review of our understanding of the aerosol–cloud interaction from the perspective of a bin resolved cloud scale modelling, Atmos. Res., 97, 478–497, https://doi.org/10.1016/j.atmosres.2010.05.008, 2010. a
Garrett, T., Zhao, C., and Novelli, P.: Assessing the relative contributions of transport efficiency and scavenging to seasonal variability in Arctic aerosol, Tellus B, 62, 190–196, https://doi.org/10.1111/j.1600-0889.2010.00453.x, 2010. a
Gliß, J., Mortier, A., Schulz, M., Andrews, E., Balkanski, Y., Bauer, S. E., Benedictow, A. M. K., Bian, H., Checa-Garcia, R., Chin, M., Ginoux, P., Griesfeller, J. J., Heckel, A., Kipling, Z., Kirkevåg, A., Kokkola, H., Laj, P., Le Sager, P., Lund, M. T., Lund Myhre, C., Matsui, H., Myhre, G., Neubauer, D., van Noije, T., North, P., Olivié, D. J. L., Sogacheva, L., Takemura, T., Tsigaridis, K., and Tsyro, S. G.: Multi-model evaluation of aerosol optical properties in the AeroCom phase III Control experiment, using ground and space based columnar observations from AERONET, MODIS, AATSR and a merged satellite product as well as surface in-situ observations from GAW sites, Atmos. Chem. Phys. Discuss., https://doi.org/10.5194/acp-2019-1214, in review, 2020. a, b, c
HAMMOZ consortium: HAMMOZ Software Licence Agreement, available at: https://redmine.hammoz.ethz.ch/attachments/291/License_ECHAM-HAMMOZ_June2012.pdf, last access: 29 June 2012. a
HAMMOZ consortium: ECHAM-HAMMOZ model data, available at: https://redmine.hammoz.ethz.ch/projects/hammoz/repository/show/echam6-hammoz/branches/fmi/fmi_trunk, last access: 8 March 2019. a, b
HAMMOZ consortium: ECHAM-HAMMOZ input data, available at: https://redmine.hammoz.ethz.ch/projects/hammoz, last access: 8 March 2019. a, b
Haywood, J. M. and Shine, K. P.: Multi-spectral calculations of the direct radiative forcing of tropospheric sulphate and soot aerosols using a column model, Q. J. Roy. Meteor. Soc., 123, 1907–1930, https://doi.org/10.1002/qj.49712354307, 1997. a
Hobbs, P.: Aerosol-cloud-climate interactions, vol. 54, Academic Press, San Diego, 1993. a
Holopainen, E., Kokkola, H., Laakso, A., and Kühn, T.: In-cloud scavenging scheme for aerosol modules 2019–2020 data, Eemeli Holopainen https://doi.org/10.23729/301df277-8147-4700-8652-ca491f2b58a6, 2020. a
Horowitz, L. W., Walters, S., Mauzerall, D. L., Emmons, L. K., Rasch, P. J., Granier, C., Tie, X., Lamarque, J.-F., Schultz, M., and Brasseur, G. P.: A global simulation of tropospheric ozone and related tracers: Description and evaluation of MOZART, version 2, J. Geophys. Res.-Atmos., 108, 4784, https://doi.org/10.1029/2002JD002853, 2003. a
IPCC: Climate Change 2013 – The Physical Science Basis: Working Group I Contribution to the Fifth Assessment Report of the Intergovernmental Panel on Climate Change, Cambridge University Press, 659–740, https://doi.org/10.1017/CBO9781107415324.018, 2014. a
Kipling, Z., Stier, P., Johnson, C. E., Mann, G. W., Bellouin, N., Bauer, S. E., Bergman, T., Chin, M., Diehl, T., Ghan, S. J., Iversen, T., Kirkevåg, A., Kokkola, H., Liu, X., Luo, G., van Noije, T., Pringle, K. J., von Salzen, K., Schulz, M., Seland, Ø., Skeie, R. B., Takemura, T., Tsigaridis, K., and Zhang, K.: What controls the vertical distribution of aerosol? Relationships between process sensitivity in HadGEM3–UKCA and inter-model variation from AeroCom Phase II, Atmos. Chem. Phys., 16, 2221–2241, https://doi.org/10.5194/acp-16-2221-2016, 2016. a, b, c, d
Köhler, H.: The nucleus in and the growth of hygroscopic droplets, Trans. Faraday Soc., 32, 1152–1161, https://doi.org/10.1039/TF9363201152, 1936. a, b, c
Kokkola, H., Vesterinen, M., Anttila, T., Laaksonen, A., and Lehtinen, K. E. J.: Technical note: Analytical formulae for the critical supersaturations and droplet diameters of CCN containing insoluble material, Atmos. Chem. Phys., 8, 1985–1988, https://doi.org/10.5194/acp-8-1985-2008, 2008. a
Kokkola, H., Kühn, T., Laakso, A., Bergman, T., Lehtinen, K. E. J., Mielonen, T., Arola, A., Stadtler, S., Korhonen, H., Ferrachat, S., Lohmann, U., Neubauer, D., Tegen, I., Siegenthaler-Le Drian, C., Schultz, M. G., Bey, I., Stier, P., Daskalakis, N., Heald, C. L., and Romakkaniemi, S.: SALSA2.0: The sectional aerosol module of the aerosol–chemistry–climate model ECHAM6.3.0-HAM2.3-MOZ1.0, Geosci. Model Dev., 11, 3833–3863, https://doi.org/10.5194/gmd-11-3833-2018, 2018a. a, b, c, d, e, f, g
Kokkola, H., Tonttila, J., Romakkaniemi, S., Bergman, T., Laakso, A., Kühn, T., Mielonen, T., Kudzotsa, I., and Raatikainen, T.: SALSA-standalone 2.0, Zenodo, https://doi.org/10.5281/zenodo.1251669, 2018b. a
Korhonen, H., Carslaw, K. S., Spracklen, D. V., Ridley, D. A., and Ström, J.: A global model study of processes controlling aerosol size distributions in the Arctic spring and summer, J. Geophys. Res.-Atmos., 113, D08211, https://doi.org/10.1029/2007JD009114, 2008. a, b
Korolev, A., Emery, E., Strapp, J., Cober, S., Isaac, G., Wasey, M., and Marcotte, D.: Small Ice Particles in Tropospheric Clouds: Fact or Artifact? Airborne Icing Instrumentation Evaluation Experiment, B. Am. Meteorol. Soc., 92, 967–973, https://doi.org/10.1175/2010BAMS3141.1, 2011. a
Krecl, P., Johansson, C., Targino, A. C., Ström, J., and Burman, L.: Trends in black carbon and size-resolved particle number concentrations and vehicle emission factors under real-world conditions, Atmos. Environ., 165, 155–168, https://doi.org/10.1016/j.atmosenv.2017.06.036, 2017. a
Kristiansen, N. I., Stohl, A., Olivié, D. J. L., Croft, B., Søvde, O. A., Klein, H., Christoudias, T., Kunkel, D., Leadbetter, S. J., Lee, Y. H., Zhang, K., Tsigaridis, K., Bergman, T., Evangeliou, N., Wang, H., Ma, P.-L., Easter, R. C., Rasch, P. J., Liu, X., Pitari, G., Di Genova, G., Zhao, S. Y., Balkanski, Y., Bauer, S. E., Faluvegi, G. S., Kokkola, H., Martin, R. V., Pierce, J. R., Schulz, M., Shindell, D., Tost, H., and Zhang, H.: Evaluation of observed and modelled aerosol lifetimes using radioactive tracers of opportunity and an ensemble of 19 global models, Atmos. Chem. Phys., 16, 3525–3561, https://doi.org/10.5194/acp-16-3525-2016, 2016. a
Kurppa, M., Hellsten, A., Roldin, P., Kokkola, H., Tonttila, J., Auvinen, M., Kent, C., Kumar, P., Maronga, B., and Järvi, L.: Implementation of the sectional aerosol module SALSA2.0 into the PALM model system 6.0: model development and first evaluation, Geosci. Model Dev., 12, 1403–1422, https://doi.org/10.5194/gmd-12-1403-2019, 2019. a
Ladino, L., Stetzer, O., Hattendorf, B., Günther, D., Croft, B., and Lohmann, U.: Experimental Study of Collection Efficiencies between Submicron Aerosols and Cloud Droplets, J. Atmos. Sci., 68, 1853–1864, https://doi.org/10.1175/JAS-D-11-012.1, 2011. a
Lamarque, J.-F., Bond, T. C., Eyring, V., Granier, C., Heil, A., Klimont, Z., Lee, D., Liousse, C., Mieville, A., Owen, B., Schultz, M. G., Shindell, D., Smith, S. J., Stehfest, E., Van Aardenne, J., Cooper, O. R., Kainuma, M., Mahowald, N., McConnell, J. R., Naik, V., Riahi, K., and van Vuuren, D. P.: Historical (1850–2000) gridded anthropogenic and biomass burning emissions of reactive gases and aerosols: methodology and application, Atmos. Chem. Phys., 10, 7017–7039, https://doi.org/10.5194/acp-10-7017-2010, 2010. a
Lohmann, U.: Possible Aerosol Effects on Ice Clouds via Contact Nucleation, J. Atmos. Sci., 59, 647–656, https://doi.org/10.1175/1520-0469(2001)059<0647:PAEOIC>2.0.CO;2, 2002. a, b, c
Lohmann, U., Stier, P., Hoose, C., Ferrachat, S., Kloster, S., Roeckner, E., and Zhang, J.: Cloud microphysics and aerosol indirect effects in the global climate model ECHAM5-HAM, Atmos. Chem. Phys., 7, 3425–3446, https://doi.org/10.5194/acp-7-3425-2007, 2007. a
Lund, M. T., Samset, B. H., Skeie, R. B., Watson-Parris, D., Katich, J. M., Schwarz, J. P., and Weinzierl, B.: Short Black Carbon lifetime inferred from a global set of aircraft observations, Clim. Atmos. Sci., 1, 2397–3722, https://doi.org/10.1038/s41612-018-0040-x, 2018. a, b, c, d
Marcolli, C., Gedamke, S., Peter, T., and Zobrist, B.: Efficiency of immersion mode ice nucleation on surrogates of mineral dust, Atmos. Chem. Phys., 7, 5081–5091, https://doi.org/10.5194/acp-7-5081-2007, 2007. a
Paasonen, P., Kupiainen, K., Klimont, Z., Visschedijk, A., Denier van der Gon, H. A. C., and Amann, M.: Continental anthropogenic primary particle number emissions, Atmos. Chem. Phys., 16, 6823–6840, https://doi.org/10.5194/acp-16-6823-2016, 2016. a
Pruppacher, H. R. and Klett, J. D.: Microphysics of clouds and precipitation, Kluwer Academic Publishers, Dordrecht, Boston, London, 1997. a, b
Rasch, P. J., Feitcher, J., Law, J., Mahowald, N., Penner, J., Benkovitz, C., Genthon, C., Giannakopoulos, C., Kasibhatla, P., Koch, D., Levy, H., Maki, T., Prather, M., Roberts, D. L., Roelofs, G.-J., Stevenson, D., Stockwell, Z., Taguchi, S., Kritz, M., Chipperfield, M., Baldocchi, D., McMurry, P., Barrie, L., Balkanski, Y., Chatfield, R., Kjellstrom, E., Lawrence, M., Lee, H. N., Lelieveld, J., Noone, K. J., Seinfeld, J., Stenchikov, G., Schwartz, S., Walcek, C., and Williamson, D.: A comparison of scavenging and deposition processes in global models: results from the WCRP Cambridge Workshop of 1995, Tellus B, 52, 1025–1056, https://doi.org/10.1034/j.1600-0889.2000.00980.x, 2000. a, b, c
Samset, B. H., Myhre, G., Schulz, M., Balkanski, Y., Bauer, S., Berntsen, T. K., Bian, H., Bellouin, N., Diehl, T., Easter, R. C., Ghan, S. J., Iversen, T., Kinne, S., Kirkevåg, A., Lamarque, J.-F., Lin, G., Liu, X., Penner, J. E., Seland, Ø., Skeie, R. B., Stier, P., Takemura, T., Tsigaridis, K., and Zhang, K.: Black carbon vertical profiles strongly affect its radiative forcing uncertainty, Atmos. Chem. Phys., 13, 2423–2434, https://doi.org/10.5194/acp-13-2423-2013, 2013. a
Schultz, M. G., Stadtler, S., Schröder, S., Taraborrelli, D., Franco, B., Krefting, J., Henrot, A., Ferrachat, S., Lohmann, U., Neubauer, D., Siegenthaler-Le Drian, C., Wahl, S., Kokkola, H., Kühn, T., Rast, S., Schmidt, H., Stier, P., Kinnison, D., Tyndall, G. S., Orlando, J. J., and Wespes, C.: The chemistry–climate model ECHAM6.3-HAM2.3-MOZ1.0, Geosci. Model Dev., 11, 1695–1723, https://doi.org/10.5194/gmd-11-1695-2018, 2018. a
Seinfeld, J. H. and Pandis, S. N.: Atmospheric Chemistry and Physics: From air pollution to climate change, 2nd edition, vol. 2, John Wiley & Sons, Hoboken, New Jersey, 2006. a, b, c, d, e
Seland, O., Iversen, T., Kirkevåg, A., and Storelvmo, T.: Aerosol-climate interactions in the CAM-Oslo atmospheric GCM and investigation of associated basic shortcomings, Tellus A, 60, 459–491, https://doi.org/10.1111/j.1600-0870.2008.00318.x, 2008. a
Sharma, S., Ishizawa, M., Chan, D., Lavoué, D., Andrews, E., Eleftheriadis, K., and Maksyutov, S.: 16-year simulation of Arctic black carbon: Transport, source contribution, and sensitivity analysis on deposition, J. Geophys. Res.-Atmos., 118, 943–964, https://doi.org/10.1029/2012JD017774, 2013. a
Simmons, A. J., Burridge, D. M., Jarraud, M., Girard, C., and Wergen, W.: The ECMWF medium-range prediction models development of the numerical formulations and the impact of increased resolution, Meteorol. Atmos. Phys., 40, 28–60, https://doi.org/10.1007/BF01027467, 1989. a
Slinn, W. G. N. and Hales, J. M.: A Reevaluation of the Role of Thermophoresis as a Mechanism of In- and Below-Cloud Scavenging, J. Atmos. Sci., 28, 1465–1471, https://doi.org/10.1175/1520-0469(1971)028<1465:AROTRO>2.0.CO;2, 1971. a
Stier, P., Feichter, J., Kinne, S., Kloster, S., Vignati, E., Wilson, J., Ganzeveld, L., Tegen, I., Werner, M., Balkanski, Y., Schulz, M., Boucher, O., Minikin, A., and Petzold, A.: The aerosol-climate model ECHAM5-HAM, Atmos. Chem. Phys., 5, 1125–1156, https://doi.org/10.5194/acp-5-1125-2005, 2005. a, b, c, d, e, f, g, h
Stone, R., Sharma, S., Herber, A., Eleftheriadis, K., and Nelson, D.: A characterization of Arctic aerosols on the basis of aerosol optical depth and black carbon measurements, Elementa, 2, 000027, https://doi.org/10.12952/journal.elementa.000027, 2014. a
Tabazadeh, A., Djikaev, Y. S., and Reiss, H.: Surface crystallization of supercooled water in clouds, P. Natl. Acad. Sci. USA, 99, 15873–15878, https://doi.org/10.1073/pnas.252640699, 2002. a, b, c, d
Tegen, I., Neubauer, D., Ferrachat, S., Siegenthaler-Le Drian, C., Bey, I., Schutgens, N., Stier, P., Watson-Parris, D., Stanelle, T., Schmidt, H., Rast, S., Kokkola, H., Schultz, M., Schroeder, S., Daskalakis, N., Barthel, S., Heinold, B., and Lohmann, U.: The global aerosol–climate model ECHAM6.3–HAM2.3 – Part 1: Aerosol evaluation, Geosci. Model Dev., 12, 1643–1677, https://doi.org/10.5194/gmd-12-1643-2019, 2019. a, b
Textor, C., Schulz, M., Guibert, S., Kinne, S., Balkanski, Y., Bauer, S., Berntsen, T., Berglen, T., Boucher, O., Chin, M., Dentener, F., Diehl, T., Easter, R., Feichter, H., Fillmore, D., Ghan, S., Ginoux, P., Gong, S., Grini, A., Hendricks, J., Horowitz, L., Huang, P., Isaksen, I., Iversen, I., Kloster, S., Koch, D., Kirkevåg, A., Kristjansson, J. E., Krol, M., Lauer, A., Lamarque, J. F., Liu, X., Montanaro, V., Myhre, G., Penner, J., Pitari, G., Reddy, S., Seland, Ø., Stier, P., Takemura, T., and Tie, X.: Analysis and quantification of the diversities of aerosol life cycles within AeroCom, Atmos. Chem. Phys., 6, 1777–1813, https://doi.org/10.5194/acp-6-1777-2006, 2006. a
Tissari, J., Lyyränen, J., Hytönen, K., Sippula, O., Tapper, U., Frey, A., Saarnio, K., Pennanen, A., Hillamo, R., Salonen, R., Hirvonen, M.-R., and Jokiniemi, J.: Fine particle and gaseous emissions from normal and smouldering wood combustion in a conventional masonry heater, Atmos. Environ., 42, 7862–7873, https://doi.org/10.1016/j.atmosenv.2008.07.019, 2008. a
Tonttila, J., Maalick, Z., Raatikainen, T., Kokkola, H., Kühn, T., and Romakkaniemi, S.: UCLALES–SALSA v1.0: a large-eddy model with interactive sectional microphysics for aerosol, clouds and precipitation, Geosci. Model Dev., 10, 169–188, https://doi.org/10.5194/gmd-10-169-2017, 2017. a
Twomey, S.: Aerosol, clouds, and radiation., vol. 25A, Atmospheric Environment, The University of Arizona, Tuczon, AZ, USA, 1991. a
van Vuuren, D. P., Edmonds, J., Kainuma, M., Riahi, K., Thomson, A., Hibbard, K., Hurtt, G. C., Kram, T., Krey, V., Lamarque, J.-F., Masui, T., Meinshausen, M., Nakicenovic, N., Smith, S. J., and Rose, S. K.: The representative concentration pathways: an overview, Clim. Chang., 109, 5–31, https://doi.org/10.1007/s10584-011-0148-z, 2011. a
Wang, H., Easter, R. C., Rasch, P. J., Wang, M., Liu, X., Ghan, S. J., Qian, Y., Yoon, J.-H., Ma, P.-L., and Vinoj, V.: Sensitivity of remote aerosol distributions to representation of cloud–aerosol interactions in a global climate model, Geosci. Model Dev., 6, 765–782, https://doi.org/10.5194/gmd-6-765-2013, 2013. a
Wang, P., Grover, S., and Pruppacher, H.: On the Effect of Electric Charges on the Scavenging of Aerosol Particles by Clouds and Small Raindrops, J. Atmos. Sci., 35, 1735–1743, https://doi.org/10.1175/1520-0469(1978)035<1735:OTEOEC>2.0.CO;2, 1978. a, b
Watson-Parris, D., Schutgens, N., Cook, N., Kipling, Z., Kershaw, P., Gryspeerdt, E., Lawrence, B., and Stier, P.: Community Intercomparison Suite (CIS) v1.4.0: a tool for intercomparing models and observations, Geosci. Model Dev., 9, 3093–3110, https://doi.org/10.5194/gmd-9-3093-2016, 2016. a
Watson-Parris, D., Schutgens, N., Reddington, C., Pringle, K. J., Liu, D., Allan, J. D., Coe, H., Carslaw, K. S., and Stier, P.: In situ constraints on the vertical distribution of global aerosol, Atmos. Chem. Phys., 19, 11765–11790, https://doi.org/10.5194/acp-19-11765-2019, 2019. a
Wofsy, S., Afshar, S., Allen, H., Apel, E., Asher, E., Barletta, B., Bent, J., Bian, H., Biggs, B., Blake, D., Blake, N., Bourgeois, I., Brock, C., Brune, W., Budney, J., Bui, T., Butler, A., Campuzano-Jost, P., Chang, C., Chin, M., Commane, R., Correa, G., Crounse, J., Cullis, P., Daube, B., Day, D., Dean-Day, J., Dibb, J., Digangi, J., Diskin, G., Dollner, M., Elkins, J., Erdesz, F., Fiore, A., Flynn, C., Froyd, K., Gesler, D., Hall, S., Hanisco, T., Hannun, R., Hills, A., Hintsa, E., Hoffman, A., Hornbrook, R., Huey, L., Hughes, S., Jimenez, J., Johnson, B., Katich, J., Keeling, R., Kim, M., Kupc, A., Lait, L., Lamarque, J.-F., Liu, J., McKain, K., McLaughling, R., Meinardi, S., Miller, D., Montzka, S., Moore, F., Morgan, E., Murphy, D., Murray, L., Nault, B., Neuman, J., Newman, P., Nicely, J., Pan, X., Paplawsky, W., Peischl, J., Prather, M., Price, D., Ray, E., Reeves, J., Richardson, M., Rollins, A., Rosenlof, K., Ryerson, T., Scheuer, E., Schill, G., Schroder, J., Schwarz, J., St.Clair, J., Steenrod, S., Stephens, B., Strode, S., Sweeney, C., Tanner, D., Teng, A., Thames, A., Thompson, C., Ullmann, K., Veres, P., Vizenor, N., Wagner, N., Watt, A., Weber, R., Weinzierl, B., Wennberg, P., Williamson, C., Wilson, J., Wolfe, G., Woods, C., and Zeng, L.: ATom: Merged Atmospheric Chemistry, Trace Gases, and Aerosols, ORNL DAAC, Oak Ridge, Tennessee, USA, https://doi.org/10.3334/ORNLDAAC/1581, 2018. a, b, c, d, e
Zhang, X., Chen, X., and Wang, J.: A number-based inventory of size-resolved black carbon particle emissions by global civil aviation, Nat. Commun., 10, 534, https://doi.org/10.1038/s41467-019-08491-9, 2019. a
Zikova, N. and Zdimal, V.: Precipitation scavenging of aerosol particles at a rural site in the Czech Republic, Tellus B, 68, 27343, https://doi.org/10.3402/tellusb.v68.27343, 2016. a