the Creative Commons Attribution 4.0 License.
the Creative Commons Attribution 4.0 License.
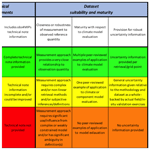
Observations for Model Intercomparison Project (Obs4MIPs): status for CMIP6
Duane Waliser
Peter J. Gleckler
Robert Ferraro
Karl E. Taylor
Sasha Ames
James Biard
Michael G. Bosilovich
Otis Brown
Helene Chepfer
Luca Cinquini
Paul J. Durack
Veronika Eyring
Pierre-Philippe Mathieu
Tsengdar Lee
Simon Pinnock
Gerald L. Potter
Michel Rixen
Roger Saunders
Jörg Schulz
Jean-Noël Thépaut
Matthias Tuma
The Observations for Model Intercomparison Project (Obs4MIPs) was initiated in 2010 to facilitate the use of observations in climate model evaluation and research, with a particular target being the Coupled Model Intercomparison Project (CMIP), a major initiative of the World Climate Research Programme (WCRP). To this end, Obs4MIPs (1) targets observed variables that can be compared to CMIP model variables; (2) utilizes dataset formatting specifications and metadata requirements closely aligned with CMIP model output; (3) provides brief technical documentation for each dataset, designed for nonexperts and tailored towards relevance for model evaluation, including information on uncertainty, dataset merits, and limitations; and (4) disseminates the data through the Earth System Grid Federation (ESGF) platforms, making the observations searchable and accessible via the same portals as the model output. Taken together, these characteristics of the organization and structure of obs4MIPs should entice a more diverse community of researchers to engage in the comparison of model output with observations and to contribute to a more comprehensive evaluation of the climate models.
At present, the number of obs4MIPs datasets has grown to about 80; many are undergoing updates, with another 20 or so in preparation, and more than 100 are proposed and under consideration. A partial list of current global satellite-based datasets includes humidity and temperature profiles; a wide range of cloud and aerosol observations; ocean surface wind, temperature, height, and sea ice fraction; surface and top-of-atmosphere longwave and shortwave radiation; and ozone (O3), methane (CH4), and carbon dioxide (CO2) products. A partial list of proposed products expected to be useful in analyzing CMIP6 results includes the following: alternative products for the above quantities, additional products for ocean surface flux and chlorophyll products, a number of vegetation products (e.g., FAPAR, LAI, burned area fraction), ice sheet mass and height, carbon monoxide (CO), and nitrogen dioxide (NO2). While most existing obs4MIPs datasets consist of monthly-mean gridded data over the global domain, products with higher time resolution (e.g., daily) and/or regional products are now receiving more attention.
Along with an increasing number of datasets, obs4MIPs has implemented a number of capability upgrades including (1) an updated obs4MIPs data specifications document that provides additional search facets and generally improves congruence with CMIP6 specifications for model datasets, (2) a set of six easily understood indicators that help guide users as to a dataset's maturity and suitability for application, and (3) an option to supply supplemental information about a dataset beyond what can be found in the standard metadata. With the maturation of the obs4MIPs framework, the dataset inclusion process, and the dataset formatting guidelines and resources, the scope of the observations being considered is expected to grow to include gridded in situ datasets as well as datasets with a regional focus, and the ultimate intent is to judiciously expand this scope to any observation dataset that has applicability for evaluation of the types of Earth system models used in CMIP.
- Article
(1389 KB) - Full-text XML
- BibTeX
- EndNote
State, national, and international climate assessment reports are growing in their importance as a scientific resource for climate change understanding and assessment of impacts crucial for economic and political decision-making (WorldBank, 2011; IPCC, 2014; NCA, 2014; EEA, 2015). A core element of these assessment reports is climate model simulations that not only provide a projection of the future climate but also information relied on in addressing adaptation and mitigation questions. These quantitative projections are the product of extremely complex multicomponent global and regional climate models (GCMs and RCMs, respectively). Because of the critical input such models provide to these assessments, and in light of significant systematic biases that potentially impact their reliability (e.g., Meehl et al., 2007; Waliser et al., 2007, 2009; Gleckler et al., 2008; Reichler and Kim, 2008; Eyring and Lamarque, 2012; Whitehall et al., 2012; IPCC, 2013; Stouffer et al., 2017), it is important to expand the scrutiny of them through the systematic application of observations from gridded satellite and reanalysis products as well as in situ station networks. Enabling such observation-based multivariate evaluations is needed for assessing model fidelity, performing quantitative model comparison, gauging uncertainty, and constructing defensible multimodel ensemble projections. These capabilities are all necessary to provide a reliable characterization of future climate that can lead to an informed decision-making process.
Optimizing the use of the plethora of observations for model evaluation is a challenge, albeit facilitated to a considerable degree by the vast strides the Coupled Model Intercomparison Project (CMIP) community has made in implementing systematic and coordinated experimentation in support of climate modeling research (Meehl et al., 2007; Taylor et al., 2012; Eyring et al., 2016a). CMIP is a flagship project of the World Climate Research Programme and is overseen by its working group on Coupled Modelling (WGCM). This architecture includes an increasingly complex set of simulation experiments designed to address specific science questions and to facilitate model evaluation (Meehl et al., 2007; Taylor et al., 2012; Eyring et al., 2016), highly detailed specifications for model output1 (e.g., Taylor et al., 2009; Juckes et al., 2020), and adoption of a distributed approach to manage and disseminate the rapidly increasing data volumes of climate model output (Williams et al., 2016). The highly collaborative infrastructure framework for CMIP has been advancing since the first World Climate Research Programme (WCRP) Model Intercomparison Project (MIP; Gates, 1992), with a payoff that became especially evident during CMIP3 (Meehl et al., 2007) when the highly organized and readily available model results facilitated an enormous expansion in the breadth of analysis that could be undertaken (Taylor et al., 2012; Eyring et al., 2016). The systematic organization of model results and their archiving and dissemination were catalytic in developing a similar vision for observations as described in this article.
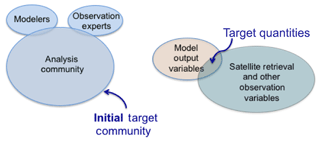
Figure 1Two schematics that illustrate key motivations and guiding considerations for obs4MIPs. Left: depiction of the large and growing community of scientists undertaking the climate model analysis who are not necessarily experts in modeling or in the details of the observations. Right: depiction of the large number of quantities available from model output (e.g., CMIP) and obtained from satellite retrievals, highlighting that a much smaller subset fall in the intersection but are of greatest relevance to model evaluation.
As the significance of the climate projections has grown in regards to considerations of adaptation and mitigation measures so has the need to quantify model uncertainties and identify and improve model shortcomings. For these purposes, it is essential to maximize the use of available observations. For instance, observations enable evaluation of a model's climatological mean state, annual cycle, and variability across timescales, as a partial gauge of model fidelity in representing different Earth system processes. The genesis of the obs4MIPs effort stemmed from the impression that there were many observations that were not being fully exploited for model evaluation. A notable driver of the early thinking and developments in obs4MIPs was the recognition – partly from the success of the CMIP experimental architecture in providing greater model output accessibility – that much of the observation-based model evaluation research was being conducted by scientists without an expert's understanding of either the observations being employed or the climate models themselves. Nevertheless, there was a clear imperative, given the discussion above, to encourage and assist the growing class of climate research scientists who were beginning to devote considerable effort to the evaluation and analysis of climate model simulations and projections (left part of Fig. 1). A sister effort, “CREATE-IP” (initially conceived as ana4MIPs), has been advanced to make reanalysis data available with a similar objective (Potter et al., 2018).
While the infrastructure advances made by CMIP had established an obvious precedent, the daunting prospect of dealing in a similar way with the plethora of observation quantities was challenging, even when only considering satellite data. Within the NASA holdings, for example, there have been over 50 Earth observation missions flown, each producing between 1 to nearly 100 quantities, and thus there are likely on the order of 1000 NASA satellite geophysical quantities that might be candidates for migration to obs4MIPs, with many more when accounting for other (EUMETSAT, NOAA, ESA, JAXA, etc.) satellite datasets. Key to making initial progress was the recognition, illustrated in the right part of Fig. 1, that only a fraction (perhaps about 10 %) of the available observation variables could be directly compared with the available CMIP output variables of which there are over a thousand. The aspirations and framework for obs4MIPs were developed with these considerations in mind. Since the initial implementation of obs4MIPs, there has been an intention to expand the breadth of datasets, including a better match of derived quantities and model output variables, e.g., through using simulators (e.g., Bodas-Salcedo et al., 2011) and an increased capacity to host the datasets, as well as to describe and disseminate them. In addition, for the first time in CMIP, evaluation tools are available that make full use of the obs4MIPs data for routine evaluation of the models (Eyring et al., 2016) as soon as the output is published to the Earth System Grid Federation (ESGF) (e.g., the Earth System Model Evaluation Tool, ESMValTool; Eyring et al., 2020; the PCMDI Metrics Package (PMP); Gleckler et al., 2016; the NCAR Climate Variability Diagnostics Package; Phillips et al., 2014; and the International Land Model Benchmarking (ILAMB) package; Collier et al., 2018).
In the next section, the history and initial objectives of the obs4MIPs project are briefly summarized. Section 3 describes the needs and efforts to expand the scope of obs4MIPs beyond its initial objectives, particularly for including a wider range of observational resources in preparation for CMIP6. Section 4 provides an updated accounting of the obs4MIPs dataset holdings; descriptions of a number of new features, including updated dataset specifications, dataset indicators, and accommodation for supplementary material; and a brief description of the alignment and intersection of obs4MIPs and CMIP model evaluation activities. Section 5 discusses challenges and opportunities for further expansion and improvements to obs4MIPs and potential pathways for addressing them.
In late 2009, the Jet Propulsion Laboratory (JPL, NASA) and the Program for Climate Model Diagnostics and Intercomparison (PCMDI, United States Department of Energy, DOE) began discussions on ways to better utilize global satellite observations for the systematic evaluation of climate models, with the fifth phase of the WCRP's CMIP5 in mind. A 2 d workshop was held at PCMDI in October 2010 that brought together experts on satellite observation, modeling, and climate model evaluation (Gleckler et al., 2011). The objectives of the meeting were to (1) identify satellite datasets that were well suited to provide observation reference information for CMIP model evaluation; (2) define a common template for documentation of observations, particularly with regard to model evaluation; and (3) begin considerations of how to make the observations and technical documentation readily available to the CMIP model evaluation community.
From the presentations and discussions at the PCMDI workshop and during the months following, the initial tenets, as well as the name of the activity, were developed (Teixeira et al., 2011). Consensus was reached on (1) the use of the CMIP5 model output list of variables (Taylor et al., 2009) as a means to define which satellite variables would be considered for inclusion; (2) the need for a “technical note” for each variable that would describe the origins, algorithms, validation and/or uncertainty, guidance on methodologies for applying the data to model evaluation, contact information, relevant references, etc. and that would be limited to a few pages targeting users who might be unfamiliar with satellites and models; (3) having the observation data technically aligned with the CMIP model output (i.e., CMIP's specific application of the NetCDF Climate and Forecast (CF) Metadata Conventions); and (4) hosting the observations on the Earth System Grid Federation (ESFG) of archive nodes so that they would appear side by side with the model output. The name “obs4MIPs” was suggested to uniquely identify the data in the ESGF archive and distinguish it from the diversity of other information hosted there.
Along with outlining the initial objectives and tenets of the pilot effort, a first set of about a dozen NASA satellite observation datasets was identified and deemed particularly appropriate for climate model evaluations relevant to CMIP and associated IPCC assessment reports, based on their maturity and long-standing community use. The initial set included temperature and humidity profiles from the Atmospheric InfraRed Sounder (AIRS) and the Microwave Limb Sounder (MLS), ozone profiles from the Tropospheric Emission Spectrometer (TES), sea surface height (SSH) from TOPEX/Jason (jointly with CNES – Centre National d'Etudes Spatiales), sea surface temperature (SST) from the Advanced Microwave Sounder Radiometer-E (AMSR-E, jointly with JAXA – Japanese Aerospace Exploration Agency), shortwave and longwave all-sky and clear-sky radiation fluxes at the top of the atmosphere from the Clouds and the Earth's Radiant Energy System (CERES), cloud fraction from MODIS, and column water vapor from the Special Sensor Microwave Imager (SSMI). All these initial datasets were global, or nearly so, and had monthly time resolution spanning record lengths between 8 and 19 years. By late 2011 these datasets were archived, with their associated technical notes, on the JPL ESGF node. Further information on the development and scope of the obs4MIPs effort during this period was captured in Teixeira et al. (2014).
With the success of this pilot effort, NASA and DOE sought to broaden the activity and engage more satellite teams and agencies by establishing an obs4MIPs Working Group early in 2012 that included members from DOE, three NASA centers, and NOAA. In the subsequent year, this working group helped identify and shepherd a number of additional datasets into the obs4MIPs project. These included ocean surface wind vectors and speed from QuikSCAT, precipitation from the Tropical Rainfall Mapping Mission (TRMM) and the Global Precipitation Climatology Project (GPCP), aerosol optical depth from the Moderate Resolution Imaging Spectroradiometer (MODIS) and the Multi-angle Imaging SpectroRadiometer (MISR), aerosol extinction profiles from Cloud-Aerosol Lidar and Infrared Pathfinder Satellite Observation (CALIPSO), surface radiation fluxes from CERES, and sea ice from the National Snow and Ice Data Center (NSIDC). Two of the datasets included higher-frequency sampling, with TRMM providing both monthly and 3-hourly values and GPCP providing both monthly and daily values.
All of the datasets contributed to obs4MIPs thus far are gridded products, and many cover a substantial fraction of the Earth. Most of the data discussed above were provided on a 1∘ × 1∘ (longitude × latitude) grid which was an appropriate target for the CMIP5 generation of models. More recently, data are being included at the highest gridded resolution available rather than mapping them to another grid. Calculation of monthly averages, which may be nontrivial especially for data derived from polar-orbiting instruments, is determined on a case-by-case basis and is described in the “Tech Note” of each product. Most of the products that have been introduced into obs4MIPs to date are based on satellite measurements, but other gridded products based on in situ measurements are envisioned to become a part of an expanding set of gridded products available via obs4MIPs. Ongoing discussions include the possibility of also including some in situ data.
Since its inception, obs4MIPs has continually engaged the climate modeling and model evaluation communities and endeavored to make them aware of its progress. Awareness and community support were fostered in part through the publications and workshops mentioned above (Gleckler et al., 2011; Teixeira et al., 2014; Ferraro et al., 2015), as well as through the WCRP, the Committee on Earth Observing Satellites (CEOS), and the Coordination Group of Meteorological Satellites (CGMS) through their Joint Working Group on Climate (JWGC).2 The JWGC published in 2017 an inventory3 integrating information on available and planned satellite datasets from all CGMS and CEOS agencies. The inventory is updated annually and serves as one resource of candidate datasets that might be suitable for obs4MIPs. Based on overlapping interests, the first international contributions to obs4MIPs were cultivated from the Climate Feedback Model Intercomparison Project (CFMIP) and the European Space Agency (ESA) through its Climate Change Initiative (Hollmann et al., 2013) and its Climate Model User Group (CMUG).
CFMIP4 was established through leadership from the UK Met Office, the Bureau of Meteorology Research Centre (BMRC), and Le Laboratoire de Météorologie Dynamique (LMD), in 2003, as a means to bring comprehensive sets of observations on clouds and related parameters to bear on the understanding of cloud–climate feedback and its representation in climate models. In addition to the modeling experiments, a deliberate and systematic strategy for archiving the satellite data relevant to the CFMIP effort was developed and implemented (see Tsushima et al., 2017; Webb et al., 2017, for recent summary information), and it was aligned with the obs4MIPs strategy and goals. Crucially, this alignment included the use of CF-compliant format, hosting the data on the ESGF, and having a focus on observed quantities and diagnostics that are fully consistent with outputs from the CFMIP Observations Simulator Package (COSP; Bodas-Salcedo et al., 2011) for the evaluation of clouds and radiation in numerical models. Based on this relatively close alignment, CFMIP provided over 20 satellite-based observed quantities as contributions to obs4MIPs. These include a number of cloud and aerosol variables from CALIPSO, CloudSat, and the Polarization & Anisotropy of Reflectances for Atmospheric Sciences coupled with Observations from a Lidar (PARASOL) satellite missions as well as the International Satellite Cloud Climatology Project (ISCCP).
ESA established the Climate Modeling User Group (CMUG)5 to provide a climate system perspective at the center of its Climate Change Initiative (CCI)6 and to host a dedicated forum bringing the Earth observation and climate modeling communities together. Having started at approximately the same time as obs4MIPs with overlapping goals, communication between the two activities was established at the outset. Through the CCI, a number of global datasets were being produced that overlapped with the model evaluation goals of obs4MIPs, and CMUG/CCI succeeded in making early contributions to obs4MIPs. These included an SST product developed from the Along Track Scanning Radiometers (ATSR) aboard ESA's ERS-1, ERS-2, and Envisat satellites, specifically the ATSR Reprocessing for Climate (ARC) product, as well as the ESA GlobVapour project merged MERIS and EUMETSAT's SSM/I water vapor column product.
The growing international and multiagency interest in obs4MIPs and its initial success meant there was potential to broaden the support structure of obs4MIPs and further expand international involvement. The establishment of the WCRP Data Advisory Council (WDAC)7 in late 2011 provided a timely opportunity to foster further development. During 2012, as the WDAC developed its priorities and identified initial projects to focus on, obs4MIPs was proposed as an activity that could contribute to the objectives of the WDAC and could be served by WDAC oversight and promotion. Based on this proposal and ensuing discussions, a WDAC Task Team on Observations for Model Evaluation (subsequently here, simply the “Task Team”) was formed in early 2013. The terms of reference for the Task Team included (1) establishing data and metadata standards for observational and reanalysis datasets consistent with those used in major climate model intercomparison efforts, (2) encouraging the application of these standards to observational datasets with demonstrated utility for model evaluation, (3) eliciting community input and providing guidance and oversight to establish criteria and a process by which candidate obs4MIPs datasets might be accepted for inclusion, (4) assisting in the coordination of obs4MIPs and related observation-focused projects (e.g., CFMIP, CREATE-IP – formerly ana4MIPs), (5) overseeing an obs4MIPs website,8 (6) recommending enhancements that might be made to ESGF software to facilitate management of and access to such projects, and (7) coordinating the above activities with major climate model intercomparison efforts (e.g., CMIP) and liaising with other related WCRP bodies, such as WCRP Model Advisory Council (WMAC), including recommend additions and improvements to CMIP standard model output to facilitate observation-based model evaluation. Membership of the Task Team9 draws on international expertise in observations, reanalyses, and climate modeling and evaluation, as well as program leadership and connections to major observation-relevant agencies (e.g., ESA, EUMETSAT, NASA, NOAA, and DOE).
One of the first activities undertaken by the Task Team was to organize a meeting of experts in satellite data products and global climate modeling for the purpose of planning the evolution of obs4MIPs in support of CMIP6 (Ferraro et al., 2015). The meeting, held in late spring of 2014 at NASA Headquarters, was sponsored by DOE, NASA, and WCRP. It brought together over 50 experts in both climate modeling and satellite data from the United States, Europe, Japan, and Australia. The objectives for the meeting included the following: (1) review and assess the framework, working guidelines, holdings, and ESGF implementation of obs4MIPs in the context of CMIP model evaluation; (2) identify underutilized and potentially valuable satellite observations and reanalysis products for climate model evaluation, in conjunction with a review of CMIP model output specifications, and recommend changes and additions to datasets and model output to achieve better alignment; and (3) provide recommendations for new observation datasets that target critical voids in model evaluation capabilities, including important phenomena, subgrid-scale features, higher temporal sampling, in situ and regional datasets, and holistic Earth system considerations (e.g., carbon cycle and composition).
Apart from recommendations of specific datasets to include in obs4MIPs in preparation for CMIP6, there were several consensus recommendations that have driven subsequent and recent obs4MIPs developments and expansion activities:
-
expand the inventory of datasets hosted by obs4MIPs,
-
include higher-frequency datasets and higher-frequency model output,
-
develop a capability to accommodate reliable and defendable uncertainty measures,
-
include datasets and data specification support for datasets involving offline simulators,
-
consider hosting reanalysis datasets in some fashion but with appropriate caveats,
-
include gridded in situ datasets and consider other in situ possibilities, and
-
provide more information on the degree of correspondence between model and observations.
For more details on the discussion and associated recommendations, see Ferraro et al. (2015). In the following section, we highlight the considerations and progress that have been made towards these and other recommendations for expanding and improving obs4MIPs.
With the recommendations of the planning meeting in hand and with CMIP6 imminent, a number of actions were taken by the obs4MIPs Task Team and the CMIP Panel (a WCRP group that oversees CMIP). For the most part, these have provided the means to widen the inventory, to make the process of contributing datasets to obs4MIPs more straightforward, and to develop additional features that benefit the users.
4.1 Additional obs4MIPs datasets
CMIP6-endorsed MIPs were required to specify the model output they needed to perform useful analyses (Eyring et al., 2016), and these formed what is now the CMIP6 data request (Juckes et al., 2020). The obs4MIPs Task Team responded by encouraging and promoting a wider range of observation-based datasets and released a solicitation for new datasets in the fall of 2015 that added emphasis on higher frequency, as well as basin- to global-scale gridded in situ data. The solicitation also placed a high priority on data products that might be of direct relevance to the CMIP6-endorsed model intercomparison projects.10 The outcomes of the solicitation and status of the obs4MIPs holdings are described below.
As of August 2019, the holdings for obs4MIPs11 include over 80 observational datasets.12 The datasets include contributions from NASA, ESA, CNES, JAXA, and NOAA, with the data being hosted at a number of ESGF data nodes, including PCMDI (LLNL), IPSL, GSFC (NASA), GFDL (NOAA), British Atmospheric Data Centre (BADC), and the German Climate Computing Center (DKRZ). Along with the previously discussed datasets, there are additional SST and water vapor products, and outgoing longwave radiation (OLR) and sea ice datasets. Some of these include both daily and monthly sampled data.
There are a number of datasets that have been provided through the ESA CCI effort, including aerosol optical thickness contribution from the ATSR-2 and AATSR missions, ocean wind speed from SSM/I, total column methane and CO2 from ESA, and a near-surface ship-based CO2 product from the Surface Ocean CO2 Atlas (SOCAT); the latter three are particularly important for the carbon cycle component of Earth system models. A new and somewhat novel dataset is expected to be contributed which will provide regional OLR data based on the Geostationary Earth Radiation Budget (GERB) instrument aboard EUMETSAT's geostationary operational weather satellites. In this case, the data coverage is for Europe and Africa only but with sampling that resolves the diurnal cycle.
In the fall of 2015, the Task Team raised awareness of obs4MIPs by explicitly inviting the observational community to contribute to obs4MIPs. The call, which was communicated by WCRP and through other channels, set the end of March 2016 as the deadline for submission. The call made explicit the desire to include observational datasets that had a regional focus, provided higher-frequency sampling, and in particular were aligned with CMIP6 experimentation and model output (Eyring et al., 2016). The response to this call resulted in proposals for nearly 100 new datasets, with several notable new contribution types. This includes proposals for a number of in situ gridded products, merged in situ and satellite products, and regional datasets. Examples include global surface temperature, multivariate ocean and land surface fluxes, sea ice and snow, ice sheet mass changes, ozone, complete regional aggregate water and energy budget products, soil moisture, cloud, aerosol, temperature and humidity profiles, surface radiative flux, and chlorophyll concentrations.
Not long after polling the observational community about possible additions to obs4MIPs, efforts began in earnest within the CMIP community to dramatically expand the CMIP5 model output lists for CMIP6. This expansion was primarily driven by the more comprehensive experimental design for CMIP6 and desire for more in-depth model diagnosis and secondarily by the greater availability of observations. It soon became clear that despite risks of slowing the momentum of obs4MIPs, it was better to postpone the inclusion of new datasets until the data standards for CMIP6 were solidified. This took more than 2 years (given CMIP6's scope and complexity), and only when that effort was largely completed in late 2017 was it possible to begin working to ensure that obs4MIPs data standards would remain technically close to those of CMIP.
4.2 Obs4MIPs data specifications (ODS)
The primary purpose of obs4MIPs is to facilitate comparison of observational data to model output from WCRP intercomparison projects, notably CMIP. To accomplish this, the organization of CMIP and obs4MIPs data must be closely aligned, including the data structure and metadata requirements and how they are ingested into the Earth System Grid Federation (ESGF) infrastructure, which is relied on for searching and accessing the data. The original set of obs4MIPs dataset contributions adhered to guidelines (ODS V1.0, circa 2012) that were based on the CMIP5 data specifications. Now, the obs4MIPs data specifications have been refined to be largely consistent with the CMIP6 data specifications, which will not change until the community begins to configure a next generation (CMIP7).
Updates to the Obs4MIPs Data Specifications (ODS2.1) include accommodation via global attributes that allow for unique identification of datasets and associated institutions, source types, and dataset versions (i.e., types of observations).13 In addition, the global attributes are constructed to facilitate organization of the obs4MIPs datasets and in particular for providing a useful set of options (or facets) for data exploration via the ESGF search engine.
Meeting the obs4MIPs (or CMIP6) data requirements is facilitated by using the Climate Model Output Rewriter (CMOR3; Doutriaux et al., 2017).14 Use of CMOR3 is not required for producing obs4MIPs data, but it is strongly recommended because CMOR3 ensures that the necessary metadata for distributed data searching are included. The version of CMOR used in the initial phase of obs4MIPs was designed for model output, and some special adaptations were required when applying it to various gridded observations. Fortunately, during the period when the CMIP6/obs4MIPs data standards were being developed, important improvements were made to CMOR3 which included streamlining how it could be used for processing gridded observations.
With the updates to ODS2.1 and CMOR3 completed,15 new and revised datasets are once again being added to obs4MIPs, and with additional enhancements in place (Sect. 4.1–4.4), that effort is expected to be the main priority for obs4MIPs throughout the research phase of CMIP6. For data providers interested in contributing to obs4MIPs, please see “How to Contribute” on the obs4MIPs website.16 Efforts to further improve the process, as well as additional considerations for future directions, are discussed in Sect. 5.
4.3 Obs4MIPs dataset indicators
Obs4MIPs has implemented a set of dataset indicators that provide information on a dataset's technical compliance with obs4MIPs standards and its suitability for climate model evaluation. The motivation for including this information is twofold. First, the indicators provide users with an overview of key features of a given dataset's suitability for model evaluation. For example, does the dataset adhere to the key requirements of obs4MIPs (e.g., having a technical note and adhering to the obs4MIPs data specifications that is required to enable ESGF searching)? Similarly, are model and observation comparisons expected to be straightforward (e.g., is direct comparison with model output possible or will it require the use of special coding applied to the model output to make it comparable)? Another relevant consideration is the degree to which the dataset has previously been used for model evaluation and whether publications exist that document such use. Second, the indicators allow for a wider spectrum of observations to be included in obs4MIPs. In the initial stages of obs4MIPs, only relatively mature datasets – those already widely adopted by the climate model evaluation community – were considered acceptable. While this helped ensure the contributions were relevant for model evaluation, it also limited the opportunity for other or newer datasets to be exposed for potential use in model evaluation.
The establishment of the indicators will facilitate the monitoring and characterization of the increasingly broad set of obs4MIPs products hosted on the ESGF and will guide users in determining which observational datasets might be best suited for their purposes. There are six indicators grouped into three categories: two indicators are associated with obs4MIPs technical requirements, three indicators are related to measures of dataset maturity and suitability for climate model evaluation, and one indicator is a measure of the comparison complexity associated with using the observation for model evaluation. These indicators, grouped by these categories, along with their potential values are given in Fig. 2a. Each of the values is color coded so that the indicators can be readily shown in a dataset search as illustrated by Fig. 2b. In the present framework, still to be fully exercised, the values of the indicators for a given dataset are intended to be assigned, in consultation with the dataset provider, by the obs4MIPs Task Team. Note that the values of the indicators can change over time as a dataset and/or its use for model evaluation matures or as the degree to which the dataset aligns with obs4MIPs technical requirements improves. To accommodate this, the values of the indicators will be version-controlled via the obs4MIPs GitHub repository. Additional information on the indicators and how they are assigned can be found on the obs4MPs website. In brief, these indicators are meant to serve as an overall summary, using qualitative distinctions, of a dataset's suitability for climate model evaluation. They do not represent an authoritative or in-depth scientific evaluation of particular products as attempted by more ambitious and comprehensive efforts such as the GEWEX Data and Analysis Panel (GDAP) (e.g., Schröder et al., 2019).
4.4 Obs4MIPs dataset supplemental information
As a result of the obs4MIPs-CMIP6 meeting in 2015 (Ferraro et al., 2015), many data providers and users made the case that obs4MIPs should accommodate optional inclusion of ancillary information with a dataset. Ancillary information might include quantitative uncertainty information, codes that provide transfer functions or forward models to enable a closer comparison between models and observations, the ability to include data flags, verification data, additional technical information, etc. Note that with the new obs4MIPs data specifications, “observational ensembles” (which provide a range of observationally based estimates of a variable that might result from reasonable processing choices of actually measured quantities) are accommodated as a special dataset type and are not relegated to “Supplemental Information”. The inclusion of Supplemental Information for an obs4MIPs dataset is optional, and the provision for accommodating such information is considered a “feature” of the current framework of obs4MIPs (see example in Sect. 4.5). In the future, there may be better ways to accommodate such information, as one particular limitation is that the Supplemental Information is not searchable from the ESGF search engine, although its existence is readily apparent and accessible once a particular dataset is located via a search. Additional information for data providers on how to include supplementary information is available on the obs4MPs website.
4.5 Example datasets and model and observation comparison
Here we illustrate how the obs4MIPs conventions and infrastructure are applied using CERES outgoing longwave radiation and TES ozone. First, following the obs4MIPs data specifications (ODS2.1; Sect. 4.2), data contributors provide some basic “registered content” (RC; see footnote 14) which includes a “source_id”, identifying the common name of the dataset (e.g., CERES) and version number (e.g., v4.0). The source_id (CERES-4-0) identifies at a high level the dataset version, which in some cases (as with CERES) applies for more than one variable. Another attribute is “region” which for CERES is identified as “global”. Controlled vocabulary (CV) provides many options for the region attribute as defined by the CF conventions. Yet another example is the “Nominal Resolution”, providing an approximate spatial resolution which in the case of the CERES-4-0 data is “1×1∘”. These and other attributes defined by ODS2.1 are included as search facets on the obs4MIPs website. Details of how these and other metadata definitions are described in detail on the obs4MIPs website.
Once the data (uniquely identified via source_id) are
registered on the obs4MIPs GitHub repository (footnote 15), the obs4MIPs
Task Team works with the data provider to agree on a set of dataset
indicators. In the case of the CERES data, the current status of the
obs4MIPs data indicators is given as
“[]”. The color coding is described in Sect. 4.3 and
refinements will be posted on the obs4MIPs website.17 As
discussed above, these qualitative indicators provide an overall summary of
a dataset's suitability for climate model evaluation.
As described in Sect. 4.4, a new feature of obs4MIPs permits data providers to include Supplemental Information (SI). These data or metadata are “free form” in that they might not adhere to any obs4MIPs or other conventions. When a user finds data via an ESGF/CoG search, SI information, if available, will be accessible adjacent to the data indicators and technical note. Figure 2 provides an example of this for the TES ozone data. And finally, Figs. 3 and 4 show sample results from two model evaluation packages used in CMIP analyses (Eyring et al., 2016c; Gleckler et al., 2016), with other examples of obs4MIPs data being used in the literature (e.g., Covey et al., 2016; Tian and Dong, 2020).
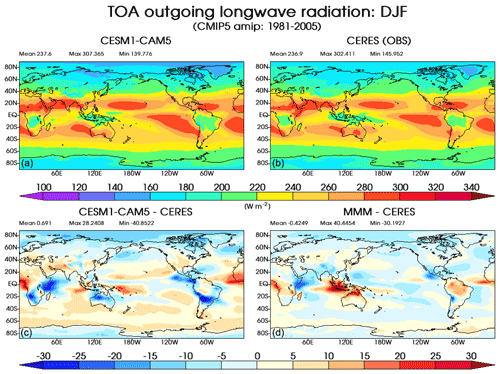
Figure 3An illustration of a model–observation comparison using obs4MIPs datasets. This four panel figure shows December–January–February (DJF) climatological mean (1981–2005) results for an individual model (a), the CERES-4-0 EBAF dataset (b), a difference map of the two upper panels (c) and a difference between the CMIP5 multi-model-mean (MMM) and CERES observations (d). The averaging period of the CERES-4-0 DJF mean is 2005–2018 (units are W m−2).
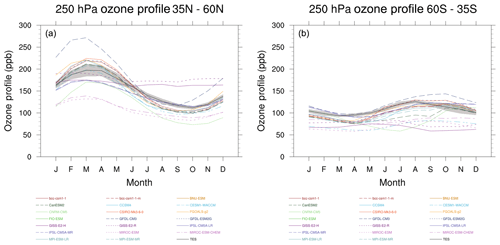
Figure 4An illustration of a model–observation comparison using obs4MIPs datasets. Tropospheric ozone annual cycle calculated from CMIP5 rcp4.5 simulations and AURA-TES observations, averaged over the years 2006–2009, for the NH (a) and SH (b) mid-latitudes (35–60∘) at 250 hPa. The individual model simulations are represented by the different colored lines while AURA-TES is shown as the black line (with ±1σ shown in gray).
4.6 Intersection with CMIP6 model evaluation activities
Initially, the primary objective of obs4MIPs was to enable the large and diverse CMIP model evaluation community to obtain better access to and supporting information on useful observational datasets. Obs4MIPs as an enabling mechanism continues to be the primary objective; however, it is now evident that there is added value beyond its original intent. In addition to providing data for researchers, obs4MIPs will be a critical link in support of current community efforts to develop routine and systematic evaluation (e.g., Gleckler et al., 2016; Eyring et al., 2016b, c, 2020; Righi et al., 2020; Phillips et al., 2014; Lee et al., 2018; Collier et al., 2018). With the rapid growth in the number of experiments, models, and output volumes, these developing evaluation tools promise to produce a first-look high-level set of evaluation and characterization summaries, well ahead of the more in-depth analyses expected to come from the climate research community. As CMIP6 data volumes are expected to grow to tens of petabytes, increasingly some model evaluation will likely take place where the data resides. These server-side evaluation tools will rely on observational data provided via obs4MIPs.
This article summarizes the current status of obs4MIPs in support of CMIP6, including the number and types of new datasets and the new extensions and capabilities that will facilitate providing and using obs4MIPs datasets. Notable highlights include (1) the recent contribution of over 20 additional datasets making the total number of datasets about 100, with about 100 or more resulting from the 2016 obs4MIPs data call that are ready for preparation and inclusion; (2) updated obs4MIPs data specifications that parallel, for the observations, the changes and extensions made for CMIP6 model data; (3) an updated CMOR3 package to give observation data providers a ready and consistent means for dataset formatting required for publication on the ESG (Earth System Grid); (4) a set of dataset indicators providing a quick accounting and assessment of a dataset's suitability and maturity for model evaluation; and (5) a provision for including supplementary information for a dataset, information that is not accommodated by the standard obs4MIPs file conventions (e.g., code, uncertainty information, ancillary data). A number of these capabilities and directions were fostered by the discussions and recommendations in the 2014 obs4MIPs meeting (Ferraro et al., 2015).
It is worth highlighting that a number of the features mentioned above, particularly the dataset indicators, have been implemented to allow for a broader variety of observations – in terms of dataset maturity, alternatives for the same geophysical quantity, and immediate relevance for climate model evaluation – to be included. Specifically, in the initial stages of obs4MIPs, the philosophy was to try to identify the “best” dataset for the given variable and/or focus only on observations that had been widely used by the community. More recently, guided by input from the 2015 obs4MIPs meeting and consistent with community model evaluation practices, it was decided that having multiple observation datasets of the same quantity (e.g., datasets derived from different satellites or based on different algorithm approaches) was a virtue. Moreover, as models add complexity and new output variables are produced, and as new observation datasets become available, it may take time to determine how to best use a new observation dataset for model evaluation. In this case, rather than waiting to include a dataset in obs4MIPs while ideas were being explored, it was decided that obs4MIPs could facilitate the maturation process and benefit the model evaluation enterprise better by including any dataset that holds some promise for model evaluation as soon as a data provider is willing and able to accommodate the dataset preparation and publication steps.
Additional considerations being discussed by the obs4MIPs Task Team are the requirements for assignments of DOIs to the datasets and how to facilitate this process. An important step has been made as it may be possible to provide DOIs via the same mechanism adopted by CMIP6 (Stockhause and Lautenschlager, 2017) and input4MIPs (Durack et al., 2018). In addition, there is discussion about how often to update and/or extend datasets and whether or not to keep old datasets once new versions have been published. Here, a dataset “extension” is considered to be adding new data to the end of the time series of data with no change in the algorithms, whereas a dataset “update” involves a revision to the algorithm. At present, the guidance from the Task Team is to extend the datasets, if feasible, with every new year of data, and if an update is provided, this would formally represent a new version of the dataset with the previous one(s) remaining a part of the obs4MIPs archive. The Task Team also has undertaken considerable deliberations on how to handle reanalysis datasets, given that they often serve as an observational reference for model evaluation applications. Initially, the archive contained a selected set of variables from the major reanalysis efforts reformatted to adhere to the same standards as obs4MIPS. These data remain available in the ESGF archive and are designated Analysis for Model Intercomparison Project (ana4MIPs). The dataset is static and not updated as new data become available. A new initiative called the Collaborative REAnalysis Technical Environment (CREATE) (Potter et al., 2018) is curating recent and updated reanalysis data for intercomparison and model evaluation purposes. The CREATE project offers an expanded variable list relative to ana4MIPs and is updated with the newest available data as they are produced by the reanalysis centers. The key variables are offered for most variables at 6 h, monthly, and for precipitation daily time resolution. The service also contains a reanalysis ensemble and spread designated as the Multiple Reanalysis Ensemble version 3 (MRE3).
Finally, obs4MIPs' growing capabilities for accommodating a greater number and broader range of datasets are pointing towards adoption of the obs4MIPs framework for hosting in situ datasets that have value for climate model evaluation. In fact, a likely emphasis of future obs4MIPs Task Team efforts will be to develop an approach to accommodate in situ data. This potential widening of scope in turn suggests the possibility for using the obs4MIPs framework to serve the function of curating and providing observation datasets for the monitoring and study of a more extensive range of environmental processes and phenomena, not specifically focusing on climate model evaluation.
See https://esgf-node.llnl.gov/projects/obs4mips/ (last access: 26 June 2020) (obs4MIPs, 2020).
DW and PJG led the initial drafting of the article. All authors contributed to the development of the obs4MIPs architecture and implementation progress, as well as the final form of the article.
The authors declare that they have no conflict of interest.
The authors would like to acknowledge the contributions from the observation providers and the associated space and research agencies that have made these contributions possible (see Sects. 2–4), as well as the Earth System Grid Federation (ESGF) for providing the platform for archive and dissemination. The authors would also like to acknowledge the support of the World Climate Research Programme (WCRP), specifically the WCRP Data Advisory Council (WDAC) for facilitating the maintenance and expansion of obs4MIPs and providing a task team framework to carry out these objectives, as well as the WGCM Infrastructure Panel (WIP) for its fundamental role in developing data protocols, standards, and documentation in support of CMIP. Some work was supported by the U.S. National Aeronautics and Space Administration (NASA) and the U.S. Department of Energy by Lawrence Livermore National Laboratory under contract DE-AC52-07NA27344 under the auspices of the Office of Science, Climate and Environmental Sciences Division, Regional and Global Model Analysis Program (LLNL release number: LLNL-JRNL-795464). The contributions of Duane Waliser and Robert Ferraro to this study were carried out on behalf of the Jet Propulsion Laboratory, California Institute of Technology, under a contract with the National Aeronautics and Space Administration. The contributions of Otis Brown and James Biard to this study were supported by the National Oceanic and Atmospheric Administration through the Cooperative Institute for Climate and Satellites – North Carolina, under cooperative agreement NA14NES432003. We would like to acknowledge the help of Birgit Hassler (DLR) in preparing the ozone model–observation comparison figures with the ESMValTool and Jiwoo Lee (PCMDI) for the comparison figures with the PMP.
This research has been supported by NASA (obs4MIPs Task), the U.S. Department of Energy by Lawrence Livermore National Laboratory (grant no. DE-AC52-07NA27344), and the Cooperative Institute for Climate and Satellites – North Carolina (grant no. NA14NES432003).
This paper was edited by Richard Neale and reviewed by two anonymous referees.
Bodas-Salcedo, A., Webb, M. J., Bony, S., Chepfer, H., Dufresne, J. L., Klein, S. A., Zhang, Y., Marchand, R., Haynes, J. M., Pincus, R., and John, V. O.: COSP: Satellite simulation software for model assessment, B. Am. Meteorol. Soc., 92, 1023–1043, https://doi.org/10.1175/2011BAMS2856.1, 2011.
Collier, N., Hoffman, F. M., Lawrence, D. M., Keppel-Aleks, G., Koven, C. D., Riley, W. J., Mu, M., and Randerson, J. T.: The International Land Model Benchmarking System (ILAMB): Design and Theory, J. Adv. Model. Earth Syst., 10, 2731–2754, https://doi.org/10.1029/2018MS001354, 2018.
Covey, C., Gleckler, P. J., Doutriaux, C., Williams, D. N., Dai, A., Fasullo, J., Trenberth, K., and Berg, A.: Metrics for the diurnal cycle of precipitation: Toward routine benchmarks for climate models, J. Climate, 29, 4461–4471, https://doi.org/10.1175/JCLI-D-15-0664.1, 2016.
Doutriaux, C., Taylor, K. E., and Nadeau, D.: CMOR3 and PrePARE Documentation, 128 pp., Lawrence Livermore National Laboratory, Livermore, CA, 2017.
Durack, P. J., Taylor, K. E., Eyring, V., Ames, S. K., Hoang, T., Nadeau, D., Doutriaux, C., Stockhause, M., and Gleckler, P. J.: Toward standardized data sets for climate model experimentation, Eos, 99, https://doi.org/10.1029/2018EO101751, 2018.
EEA: Overview of reported national policies and measures on climate change mitigation in Europe in 2015, Information reported by Member States under the European Union Monitoring Mechanism Regulation Rep., Publications Office of the European Union, Luxembourg, 2015.
Eyring, V. and Lamarque, J.-F.: Global chemistry-climate modeling and evaluation, EOS, Transactions of the American Geophysical Union, 93, 539–539, 2012.
Eyring, V., Bony, S., Meehl, G. A., Senior, C. A., Stevens, B., Stouffer, R. J., and Taylor, K. E.: Overview of the Coupled Model Intercomparison Project Phase 6 (CMIP6) experimental design and organization, Geosci. Model Dev., 9, 1937–1958, https://doi.org/10.5194/gmd-9-1937-2016, 2016a.
Eyring, V., Gleckler, P. J., Heinze, C., Stouffer, R. J., Taylor, K. E., Balaji, V., Guilyardi, E., Joussaume, S., Kindermann, S., Lawrence, B. N., Meehl, G. A., Righi, M., and Williams, D. N.: Towards improved and more routine Earth system model evaluation in CMIP, Earth Syst. Dynam., 7, 813–830, https://doi.org/10.5194/esd-7-813-2016, 2016b.
Eyring, V., Righi, M., Lauer, A., Evaldsson, M., Wenzel, S., Jones, C., Anav, A., Andrews, O., Cionni, I., Davin, E. L., Deser, C., Ehbrecht, C., Friedlingstein, P., Gleckler, P., Gottschaldt, K.-D., Hagemann, S., Juckes, M., Kindermann, S., Krasting, J., Kunert, D., Levine, R., Loew, A., Mäkelä, J., Martin, G., Mason, E., Phillips, A. S., Read, S., Rio, C., Roehrig, R., Senftleben, D., Sterl, A., van Ulft, L. H., Walton, J., Wang, S., and Williams, K. D.: ESMValTool (v1.0) – a community diagnostic and performance metrics tool for routine evaluation of Earth system models in CMIP, Geosci. Model Dev., 9, 1747–1802, https://doi.org/10.5194/gmd-9-1747-2016, 2016c.
Eyring, V., Bock, L., Lauer, A., Righi, M., Schlund, M., Andela, B., Arnone, E., Bellprat, O., Brötz, B., Caron, L.-P., Carvalhais, N., Cionni, I., Cortesi, N., Crezee, B., Davin, E., Davini, P., Debeire, K., de Mora, L., Deser, C., Docquier, D., Earnshaw, P., Ehbrecht, C., Gier, B. K., Gonzalez-Reviriego, N., Goodman, P., Hagemann, S., Hardiman, S., Hassler, B., Hunter, A., Kadow, C., Kindermann, S., Koirala, S., Koldunov, N. V., Lejeune, Q., Lembo, V., Lovato, T., Lucarini, V., Massonnet, F., Müller, B., Pandde, A., Pérez-Zanón, N., Phillips, A., Predoi, V., Russell, J., Sellar, A., Serva, F., Stacke, T., Swaminathan, R., Torralba, V., Vegas-Regidor, J., von Hardenberg, J., Weigel, K., and Zimmermann, K.: ESMValTool v2.0 – Extended set of large-scale diagnostics for quasi-operational and comprehensive evaluation of Earth system models in CMIP, Geosci. Model Dev., https://doi.org/10.5194/gmd-2019-291, accepted, 2020.
Ferraro, R., Waliser, D. E., Gleckler, P., Taylor, K. E., and Eyring, V.: Evolving obs4MIPs To Support Phase 6 Of The Coupled Model Intercomparison Project (CMIP6), B. Am. Meteorol. Soc., 96, 131–133, https://doi.org/10.1175/BAMS-D-14-00216.1, 2015.
Gates, W. L.: AMIP: the Atmospheric Model Intercomparison Project, B. Am. Meteorol. Soc., 73, 1962–1970, 1992.
Gleckler, P., Taylor, K. E., and Doutriaux, C.: Performance metrics for climate models, J. Geophys. Res.-Atmos., 113, D06104, https://doi.org/10.1029/2007jd008972, 2008.
Gleckler, P., Ferraro, R., and Waliser, D. E.: Better use of satellite data in evaluating climate models contributing to CMIP and assessed by IPCC: Joint DOE-NASA workshop; LLNL, 12–13 October 2010, EOS, 92, 172, 2011.
Gleckler, P. J., Doutriaux, C., Durack, P. J., Taylor, K. E., Zhang, Y., Williams, D. N., Mason, E., and Servonnat, J.: A more powerful reality test for climate models, Eos, 97, https://doi.org/10.1029/2016EO051663, 2016.
Gleckler, P. J., Taylor, K. E., Durack, P. J., Ferraro, R., Baird, J., Finkensieper, S., Stevens, S., Tuma, M., and Nadeau, D.: The obs4MIPs data specifications 2.1, in preparation, 2020.
Hollmann, R., Merchant, C. J., Saunders, R., Downy, C., Buchwitz, M., Cazenave, A., Chuvieco, E., Defourny, P., de Leeuw, G., Forsberg, R., Holzer-Popp, T., Paul, F., Sandven, S., Sathyendranath, S., van Roozendael, M., and Wagner, W.: The ESA Climate Change Initiative: Satellite Data Records for Essential Climate Variables, B. Am. Meteorol. Soc., 94, 1541–1552, https://doi.org/10.1175/BAMS-D-11-00254.1, 2013.
IPCC: Climate Change 2013: The Physical Science BasisRep., 1535 pp., IPCC, Geneva, Switzerland, 2013.
IPCC: Climate Change 2014: Synthesis Report. Contribution of Working Groups I, II and III to the Fifth Assessment Report of the Intergovernmental Panel on Climate Change Rep., 151 pp., IPCC, Geneva, Switzerland, 2014.
Juckes, M., Taylor, K. E., Durack, P. J., Lawrence, B., Mizielinski, M. S., Pamment, A., Peterschmitt, J.-Y., Rixen, M., and Sénési, S.: The CMIP6 Data Request (DREQ, version 01.00.31), Geosci. Model Dev., 13, 201–224, https://doi.org/10.5194/gmd-13-201-2020, 2020.
Lee, H., Goodman, A., McGibbney, L., Waliser, D. E., Kim, J., Loikith, P. C., Gibson, P. B., and Massoud, E. C.: Regional Climate Model Evaluation System powered by Apache Open Climate Workbench v1.3.0: an enabling tool for facilitating regional climate studies, Geosci. Model Dev., 11, 4435–4449, https://doi.org/10.5194/gmd-11-4435-2018, 2018.
Meehl, G. A., Covey, C., Delworth, T., Latif, M., McAvaney, B., Mitchell, J. F. B., Stouffer, R. J., and Taylor, K. E.: The WCRP CMIP3 multi-model dataset: A new era in climate change research, B. Am. Meteorol. Soc., 88, 1383–1394, 2007.
NCA: U. S. National Climate Assessment Rep., 1717 Pennsylvania Avenue, NW, Suite 250, Washington, D.C., 2014.
obs4MIPs: Observations for Model Intercomparisons Project, available at: https://esgf-node.llnl.gov/projects/obs4mips/, last access: 26 June 2020.
Phillips, A. S., Deser, C., and Fasullo, J.: Evaluating modes of variability in climate models, Eos, Transactions American Geophysical Union, 95, 453–455, 2014.
Potter, G. L., Carriere, L., Hertz, J. D., Bosilovich, M., Duffy, D., Lee, T., and Williams, D. N.: Enabling reanalysis research using the collaborative reanalysis technical environment (CREATE), B. Am. Meteorol. Soc., 99, 677–687, https://doi.org/10.1175/BAMS-D-17-0174.1, 2018.
Reichler, T. and Kim, J.: How well do coupled models simulate today's climate?, B. Am. Meteorol. Soc., 89, 303, https://doi.org/10.1175/bams-89-3-303, 2008.
Righi, M., Andela, B., Eyring, V., Lauer, A., Predoi, V., Schlund, M., Vegas-Regidor, J., Bock, L., Brötz, B., de Mora, L., Diblen, F., Dreyer, L., Drost, N., Earnshaw, P., Hassler, B., Koldunov, N., Little, B., Loosveldt Tomas, S., and Zimmermann, K.: Earth System Model Evaluation Tool (ESMValTool) v2.0 – technical overview, Geosci. Model Dev., 13, 1179–1199, https://doi.org/10.5194/gmd-13-1179-2020, 2020.
Schröder, M., Lockhoff, M., Shi, L., August, T., Bennartz, R., Brogniez, H., Calbet, X., Fell, F., Forsythe, J., Gambacorta, A., Ho, S.-P., Kursinski, E. R., Reale, A., Trent, T., and Yang, Q.: The GEWEX Water Vapor Assessment: Overview and Introduction to Results and Recommendations, Remote Sens., 11, 251, https://doi.org/10.3390/rs11030251, 2019.
Stockhause, M. and Lautenschlager, M.: CMIP6 Data Cita-tion of Evolving Data, Data Science Journal, 16, 1–13, https://doi.org/10.5334/dsj-2017-030, 2017.
Stouffer, R. J., Eyring, V., Meehl, G. A., Bony, S., Senior, C., Stevens, B., and Taylor, K. E.: CMIP5 Scientific Gaps and Recommendations for CMIP6, B. Am. Meteorol. Soc., 98, 95–105, 2017.
Taylor, K. E., Stouffer, R. J., and Meehl, G. A.: A Summary of the CMIP5 Experiment Design, White paper, available at: https://pcmdi.llnl.gov/mips/cmip5/docs/Taylor_CMIP5_design.pdf (last access: 27 June 2016), 2009.
Taylor, K. E., Stouffer, R. J., and Meehl, G. A.: An Overview of CMIP5 and the Experiment Design, B. Am. Meteorol. Soc., 93, 485–498, 2012.
Teixeira, J., Waliser, D., Ferraro, R., Gleckler, P., and Potter, G., Satellite observations for CMIP5 simulations, CLIVAR Exchanges, Special Issue on the WCRP Coupled Model Intercomparison Project Phase 5 – CMIP5, CLIVAR Exchanges, 16, 46–47, 2011.
Teixeira, J., Waliser, D. E., Ferraro, R., Gleckler, P., Lee, T., and Potter, G.: Satellite Observations for CMIP5: The Genesis of Obs4MIPs, B. Am. Meteorol. Soc., 95, 1329–1334, https://doi.org/10.1175/BAMS-D-12-00204.1, 2014.
Tian, B. and Dong, X.: The double-ITCZ bias in CMIP3, CMIP5, and CMIP6 models based on annual mean precipitation, Geophys. Res. Lett., 47, e2020GL087232, https://doi.org/10.1029/2020GL087232, 2020.
Tsushima, Y., Brient, F., Klein, S. A., Konsta, D., Nam, C. C., Qu, X., Williams, K. D., Sherwood, S. C., Suzuki, K., and Zelinka, M. D.: The Cloud Feedback Model Intercomparison Project (CFMIP) Diagnostic Codes Catalogue – metrics, diagnostics and methodologies to evaluate, understand and improve the representation of clouds and cloud feedbacks in climate models, Geosci. Model Dev., 10, 4285–4305, https://doi.org/10.5194/gmd-10-4285-2017, 2017.
Waliser, D., Seo, K. W., Schubert, S. and Njoku, E.: Global water cycle agreement in the climate models assessed in the IPCC AR4, Geophys. Res. Lett., 34, L16705, https://doi.org/10.1029/2007GL030675, 2007.
Waliser, D. E., Li, J. F., Woods, C., Austin, R., Bacmeister, J., Chern, J., Genio, A. D., Jiang, J., Kuang, Z., Meng, H., Minnis, P., Platnick, S., Rossow, W. B., Stephens, G., Sun-Mack, S., Tao, W. K., Tompkins, A., Vane, D., Walker, C., and Wu, D.: Cloud Ice: A Climate Model Challenge With Signs and Expectations of Progress, J. Geophys. Res., 114, D00A21, https://doi.org/10.1029/2008JD010015, 2009.
Webb, M. J., Andrews, T., Bodas-Salcedo, A., Bony, S., Bretherton, C. S., Chadwick, R., Chepfer, H., Douville, H., Good, P., Kay, J. E., Klein, S. A., Marchand, R., Medeiros, B., Siebesma, A. P., Skinner, C. B., Stevens, B., Tselioudis, G., Tsushima, Y., and Watanabe, M.: The Cloud Feedback Model Intercomparison Project (CFMIP) contribution to CMIP6, Geosci. Model Dev., 10, 359–384, https://doi.org/10.5194/gmd-10-359-2017, 2017.
Whitehall, K., Mattmann, C., Waliser, D., Kim, J., Goodale, C., Hart, A., Ramirez, P., Zimdars, P., Crichton, D., Jenkins, G., Jones, C., Asrar, G., and Hewitson, B.: Building model evaluation and decision support capacity for CORDEX, WMO Bulletin, 61, 29–34, 2012.
Williams, D., Balaji, V., Cinquini, L., Denvil, S., Duffy, D., Evans, B., Ferraro, R., Hansen, R., Lautenschlager, M., and Trenham, C.: A Global Repository for Planet-Sized Experiments and Observations, B. Am. Meteorol. Soc., 97, 803–816, https://doi.org/10.1175/BAMS-D-15-00132.1, 2016.
WorldBank: Climate change and fiscal policy: A report for APECRep., Washington, D.C., 2011.
https://goo.gl/v1drZl (last access: 26 June 2020)
http://ceos.org/ourwork/workinggroups/climate/ (last access: 26 June 2020)
https://climatemonitoring.info/ecvinventory/ (last access: 26 June 2020)
http://cfmip.metoffice.com (last access: 26 June 2020) and http://climserv.ipsl.polytechnique.fr/cfmip-obs/ (last access: 26 June 2020)
http://www.esa-cmug-cci.org (last access: 26 June 2020)
http://cci.esa.int (last access: 26 June 2020)
http://www.wcrp-climate.org/wdac-overview (last access: 26 June 2020)
https://www.earthsystemcog.org/projects/obs4mips/ (last access: 26 June 2020)
https://esgf-node.llnl.gov/projects/obs4mips/governance/ (last access: 26 June 2020)
http://www.wcrp-climate.org/modelling-wgcm-mip-catalogue/modelling-wgcm-cmip6-endorsed-mips (last access: 26 June 2020)
http://www.earthsystemcog.org/projects/obs4mips/ (last access: 26 June 2020)
Not all datasets may be visible on the ESGF unless all nodes are on line.
https://esgf-node.llnl.gov/projects/obs4mips/DataSpecifications (last access: 26 June 2020); Gleckler et al. (2020)
https://cmor.llnl.gov (last access: 26 June 2020)
https://github.com/pcmdi/obs4mips-cmor-tables (last access: 26 June 2020)
https://esgf-node.llnl.gov/projects/obs4mips/HowToContribute (last access: 26 June 2020)
https://esgf-node.llnl.gov/projects/obs4mips/DatasetIndicators (last access: 26 June 2020)