the Creative Commons Attribution 4.0 License.
the Creative Commons Attribution 4.0 License.
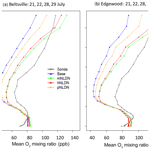
Simulating lightning NO production in CMAQv5.2: performance evaluations
Kristen M. Foley
Rohit Mathur
Shawn J. Roselle
Kenneth E. Pickering
Dale J. Allen
This study assesses the impact of the lightning nitric oxide (LNO) production schemes in the Community Multiscale Air Quality (CMAQ) model on ground-level air quality as well as aloft atmospheric chemistry through detailed evaluation of model predictions of nitrogen oxides (NOx) and ozone (O3) with corresponding observations for the US. For ground-level evaluations, hourly O3 and NOx values from the U.S. EPA Air Quality System (AQS) monitoring network are used to assess the impact of different LNO schemes on model prediction of these species in time and space. Vertical evaluations are performed using ozonesonde and P-3B aircraft measurements during the Deriving Information on Surface Conditions from Column and Vertically Resolved Observations Relevant to Air Quality (DISCOVER-AQ) campaign conducted in the Baltimore–Washington region during July 2011. The impact on wet deposition of nitrate is assessed using measurements from the National Atmospheric Deposition Program's National Trends Network (NADP NTN). Compared with the Base model (without LNO), the impact of LNO on surface O3 varies from region to region depending on the Base model conditions. Overall statistics suggest that for regions where surface O3 mixing ratios are already overestimated, the incorporation of additional NO from lightning generally increased model overestimation of mean daily maximum 8 h (DM8HR) O3 by 1–2 ppb. In regions where surface O3 is underestimated by the Base model, LNO can significantly reduce the underestimation and bring model predictions close to observations. Analysis of vertical profiles reveals that LNO can significantly improve the vertical structure of modeled O3 distributions by reducing underestimation aloft and to a lesser degree decreasing overestimation near the surface. Since the Base model underestimates the wet deposition of nitrate in most regions across the modeling domain with the exception of the Pacific Coast, the inclusion of LNO leads to reduction in biases and errors and an increase in correlation coefficients at almost all the NADP NTN sites. Among the three LNO schemes described in Kang et al. (2019), the hNLDN scheme, which is implemented using hourly observed lightning flash data from National Lightning Detection Network (NLDN), performs best for comparisons with ground-level values, vertical profiles, and wet deposition of nitrate; the mNLDN scheme (the monthly NLDN-based scheme) performed slightly better. However, when observed lightning flash data are not available, the linear regression-based parameterization scheme, pNLDN, provides an improved estimate for nitrate wet deposition compared to the base simulation that does not include LNO.
- Article
(8417 KB) - Full-text XML
- BibTeX
- EndNote
The potential importance of nitrogen oxides (NOx; NOx = NO + NO2) produced by lightning (LNOx) to regional air quality was recognized more than 2 decades ago (e.g., Novak and Pierce, 1993), but, in part due to the limited understanding of this NOx source (Schumann and Huntrieser, 2007; Murray, 2016; Pickering et al., 2016), LNOx emissions have only been added to regional chemistry and transport models during the last decade (e.g., Allen et al., 2012; Kaynak et al., 2008; Koshak et al., 2014; Smith and Mueller, 2010; Koo et al., 2010). Since NO and NO2 coexist in the atmosphere, it is often collectively referred to as LNOx; however, the immediate release of lightning flashes is just NO, and the schemes in Kang et al. (2019) also generate NO emissions only, so in this paper it is primarily referred to as LNO. As a result of efforts to reduce anthropogenic NOx emissions in recent decades (Simon et al., 2015; https://gispub.epa.gov/air/trendsreport/2018, last access: 2 October 2019), it is expected that the relative contribution of LNO to the tropospheric NOx burden and its subsequent impacts on atmospheric chemistry as one of the key precursors for ozone (O3), hydroxyl radical (OH), nitrate , and other species will increase in the United States and other developed countries (Kang and Pickering, 2018). The significant impact of LNO on process-based understanding of surface air quality was earlier reported by Napelenok et al. (2008), who found low biases in upper tropospheric NOx in the Community Multiscale Air Quality Model (CMAQ) (Byun and Schere, 2006) simulations without LNO emissions made it difficult to constrain ground-level NOx emissions using inverse methods and Scanning Imaging Absorption Spectrometer for Atmospheric Cartography (SCIAMACHY) NO2 retrievals (Bovensmann et al., 1999; Sioris et al., 2004; Richter et al., 2005). Appel et al. (2011) and Allen et al. (2012) reported that wet deposition at National Atmospheric Deposition Program (NADP) sites was underestimated by a factor of 2 when LNO was not included.
LNO production and distribution were parameterized initially in global models (e.g., Stockwell et al., 1999; Labrador et al., 2005), relying on the work of Price and Rind (1992) and Price et al. (1997), so that lightning flash frequency was parameterized as a function of the maximum cloud-top height. Other approaches for LNO parameterization include a combination of latent heat release and cloud-top height (Flatoy and Hov, 1997), convective precipitation rate (e.g., Allen and Pickering, 2002), convective available potential energy (Choi et al., 2005), or convectively induced updraft velocity (Allen et al., 2000; Allen and Pickering, 2002). More recently, Finney et al. (2014, 2016) adopted a lightning parameterization using upward cloud ice flux at 440 hPa (based upon definitions of deep convective clouds in the International Satellite Cloud Climatology Project (Rossow et al., 1996)) and implemented it in the United Kingdom Chemistry and Aerosol model (UKCA). With the availability of lightning flash data from the National Lightning Detection Network (NLDN) (Orville et al., 2002), recent LNO parameterization schemes have started to include the observed lightning flash information to constrain LNO in regional chemical transport models (CTMs) (Allen et al., 2012). In Kang et al. (2019), we described the existing LNO parameterization scheme that is based on the monthly NLDN (mNLDN) lightning flash data and an updated scheme using hourly NLDN (hNLDN) lightning flash data in the CMAQ lightning module. In addition, we also developed a scheme based on linear and log-linear regression parameters using multiyear NLDN-observed lightning flashes and model predicted convective precipitation rate (pNLDN). The preliminary assessment of these schemes based on total column LNO suggests that all the schemes provide reasonable LNO estimates in time and space, but during summer months the mNLDN scheme tends to produce the most LNO and the pNLDN scheme the least LNO.
The first study on the impact of LNO on surface air quality using CMAQ was conducted by Allen et al. (2012) and was followed by Wang et al. (2013) with different ways for parameterizing LNO production and different model configurations. In this study, we present performance evaluations using each of the LNO production schemes (mNLDN, hNLDN, and pNLDN) described by Kang et al. (2019) to provide estimates of LNO in CMAQ. In addition to the examination of differences in air quality estimates between these schemes, we compare the model predictions to Base model estimates without LNO and evaluate the estimates from all of the simulations against surface and airborne observations.
Section 2 describes the model configuration, simulation scenarios, analysis methodology, and observational data. Section 3 presents the analysis results, and Sect. 4 presents the conclusions.
2.1 The LNO schemes
In air quality models, three steps are involved in generating LNO emissions: (1) the identification of lightning flashes, (2) the production of the total column NO at model grid cells, and (3) the distribution of the column NO into model layers vertically. Three schemes to produce total column LNO emissions are examined in this study: mNLDN – based on monthly mean NLDN lightning flashes and convective precipitation predicted by the upstream meteorological model; hNLDN – directly uses the observed NLDN lightning flashes that are aggregated into hourly values and gridded onto model grid cells; and pNLDN – a linear and log-linear regression parameterization scheme derived using multiyear observed lightning flash rate and model predicted convective precipitation. After total column LNO is produced at model grid cells, it is distributed onto vertical model layers using the double-peak vertical distribution algorithm described in Kang et al. (2019), which also provides detailed description and formulation of all the LNO schemes.
2.2 The CMAQ model and simulation configurations
The CMAQ model (Appel et al., 2017) version 5.2 was configured with the Carbon Bond 6 (CB6) chemical mechanism (Yarwood et al., 2010) and the AERO6 aerosol module (Nolte et al., 2015). The meteorological inputs were provided by the Weather Research and Forecasting (WRF) model version 3.8, and the model-ready meteorological input files were created using version 4.2 of the meteorology–chemistry interface processor (MCIP; Otte and Pleim, 2010).
The modeling domain covers the entire contiguous United States (CONUS) and surrounding portions of northern Mexico and southern Canada, as well as the eastern Pacific and western Atlantic oceans. The model domain consists of 299 north–south grid cells by 459 east–west grid cells utilizing 12 km × 12 km horizontal grid spacing, 35 vertical layers with varying thickness extending from the surface to 50 hPa and an approximately 10 m midpoint for the lowest (surface) model layer. The simulation time period covers the months from April to September 2011 with a 10 d spin-up period in March.
Emission input data were based on the 2011 National Emissions Inventory (https://www.epa.gov/air-emissions-inventories, last access: 2 October 2019). The raw emission files were processed using version 3.6.5 of the Sparse Matrix Operator Kernel Emissions (SMOKE; https://www.cmascenter.org/smoke/, last access: 2 October 2019) processor to create gridded speciated hourly model-ready input emission fields for input to CMAQ. Electric generating unit (EGU) emissions were obtained using data from EGUs equipped with a continuous emission monitoring system (CEMS). Plume rise for point and fire sources were calculated in-line for all simulations (Foley et al., 2010). Biogenic emissions were generated in-line in CMAQ using BEIS versions 3.61 (Bash et al., 2016). All the simulations employed the bidirectional (bi-di) ammonia flux option for estimating the air-surface exchange of ammonia.
There are four CMAQ simulation scenarios for this study: (1) simulation without LNO (Base), (2) simulation with LNO generated by the scheme based on monthly information from the NLDN (mNLDN), (3) simulation with LNO generated by scheme based on hourly information from the NLDN (hNLDN), and (4) simulation with LNO generated by the scheme parameterizing lightning emissions based on modeled convective activity (pNLDN) as described in detail in Kang et al. (2019). All other model inputs, parameters and settings were the same across the four simulations. The vertical distribution algorithm is the same for all the LNO schemes as also described in Kang et al. (2019).
2.3 Observations and analysis techniques
To assess the impact of LNO on ground-level air quality, output from the various CMAQ simulations were paired in space and time with observed data from the U.S. EPA Air Quality System (AQS; https://www.epa.gov/aqs, last access: 2 October 2019) for hourly O3 and NOx. To evaluate the vertical distribution, measurements of trace species from the Deriving Information on Surface Conditions from Column and Vertically Resolved Observations Relevant to Air Quality (DISCOVER-AQ; http://www.nasa.gov/mission_pages/discover-aq, last access: 2 October 2019) campaign conducted in the Baltimore–Washington region (e.g., Crawford and Pickering, 2014; Anderson et al., 2014; Follette-Cook et al., 2015) were used. During this campaign, the NASA P-3B aircraft measured trace gases including O3, NO, and NO2. Vertical profiles were obtained over seven locations – Beltsville (Be), Padonia (Pa), Fair Hill (Fa), Aldino (Al), Edgewood (Ed), Essex (Es), and Chesapeake Bay (Cb) from approximately 0.3 to 5 km above ground level during P-3B flights over 14 d in July 2011. During this same period, ozonesonde measurements were taken that extended from ground level through the entire model column at two locations (Beltsville, MD, and Edgewood, MD, as shown in Fig. 1). Inclusion of LNO estimates in the CTM simulations also has an important impact on model estimated wet deposition of nitrate. Therefore, assessment was also performed using data from the National Atmospheric Deposition Program's National Trends Network (NADP NTN, http://nadp.slh.wisc.edu/ntn, last access: 2 October 2019).
Table 1Statistics of DM8HR O3 for all model cases over the domain and analysis regions in July 2011. The best performance metrics among the model cases are highlighted in bold.
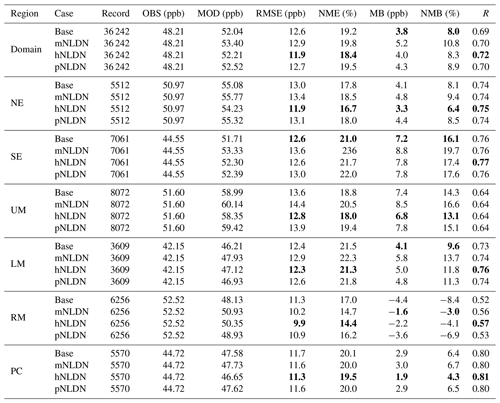
Table 2Statistics of daily mean NOx for all model cases over the domain and analysis regions in July 2011. The best performance metrics among the model cases are highlighted in bold.
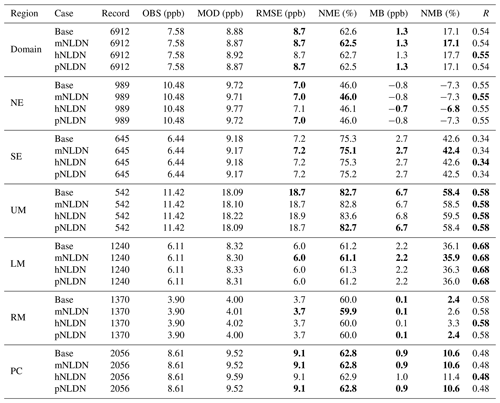
Since lightning activity and LNO exhibit distinct spatial variations (Kang and Pickering, 2018), analysis was conducted for the model domain over the contiguous United States and then for each region as shown in Fig. 1. Emphasis is placed on two regions, the southeast (SE) and the Rocky Mountains (RM), where lightning activity is more prevalent and LNO has the greatest impact on model predictions as shown in the Results section – increasing model bias in the SE and decreasing bias in the RM. The commonly used statistical metrics, root mean square error (RMSE), normalized mean error (NME), mean bias (MB), normalized mean bias (NMB), and correlation coefficient (R) in the model evaluation field, as defined in Kang et al. (2005) and Eder et al. (2006), were calculated to assess the basic performance differences among all the model cases for their ground-level air quality predictions.
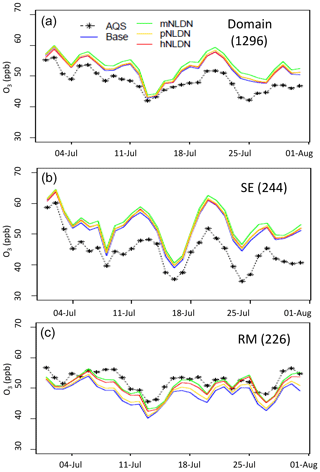
Figure 2Time series of regional-mean daily maximum 8 h O3 comparing observations (AQS) and CMAQ model predictions using the LNOx schemes to Base simulation for the domain (a), for SE (b), and for RM (c) in July 2011. The numbers in the parentheses following the region names are the number of AQS sites.
3.1 Ground-level evaluation for O3 and NOx
3.1.1 Statistical performance metrics
Tables 1 and 2 display the statistical model performance metrics for daily maximum 8 h (DM8HR) O3 and daily mean NOx mixing ratios over the domain and each analysis region for all four model cases in July 2011 (Base, mNLDN, hNLDN, and pNLDN). The best performance metrics among the model cases are highlighted in bold. As shown in Table 1, for DM8HR O3, the Base simulation has the lowest MB and NMB values over the domain, while hNLDN produced the smallest RMSE and NME values. The mNLDN generated the largest values for both error (RMSE and NME) and biases (MB and NMB), followed by pNLDN, and all model cases with LNO exhibit slightly higher correlation coefficients than the Base simulation. Additionally, the hNLDN simulation exhibited higher correlation and lower bias and error relative to the measurements indicating the value of higher-temporal-resolution lightning activity for representing the associated NOx emissions and their impacts on tropospheric chemistry.
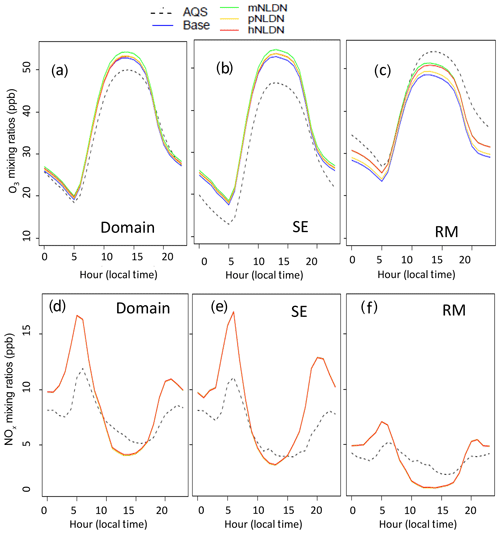
Figure 4Diurnal profiles for hourly O3 and NOx over the domain (a, d), SE (b, e), and RM (c, f) in July 2011.
Examining the regional results for DM8HR O3 in Table 1, the statistical measures indicate that in the northeast (NE), hNLDN outperformed all other model cases with the lowest errors and biases and highest correlation coefficient. In the southeast (SE), the Base simulation performed better with the lowest errors and mean biases, but the correlation coefficient (R) value for hNLDN is slightly higher. Among all the LNO cases, mNLDN produced the worst statistics in this region. Historically, CTMs tend to significantly overestimate surface O3 in the southeast US (Lin et al., 2008; Fiore et al., 2009; Brown-Steiner et al., 2015; Canty et al., 2015), and this is partially driven by a likely overestimation of anthropogenic NOx emissions (Anderson et al., 2014). Thus, even though lightning is known to impact ambient air quality, including this additional NOx source can worsen biases in model O3 in some locations and time periods due to other errors in the modeling system. As noted in Table 1, compared to the Base, the MB values in the SE increased by about 1.6 ppb with mNLDN and increased by less than 1 ppb with hNLDN and pNLDN. Nevertheless, the correlation coefficients for mNLDN and pNLDN were almost the same with the Base, and hNLDN was slightly higher (0.77 compared to 0.76). These correlations indicate that even though additional NOx increases the mean bias, when it is added correctly in time and space, as with the case of hNLDN, the spatial and temporal correlation are slightly improved. In the Upper Midwest (UM), the lowest errors and biases among the model cases are associated with hNLDN, while the worst performance is with mNLDN. In the Lower Midwest (LM), hNLDN performed comparable with the Base, with hNLDN having the highest correlation and lowest mean errors, while the Base has the lowest mean biases. The Rocky Mountain (RM) region is the only region that shows an underestimation of DM8HR O3. In this region all the model cases with LNO outperformed the Base case in all the metrics. Among the three model cases with LNO, mNLDN produced the lowest MB and NMB values, while hNLDN had the lowest RMSE and NME, and the highest correlation. In the Pacific Coast (PC) region, lightning activity is generally very low compared to other regions (Kang and Pickering, 2018). All model cases with LNO outperformed the Base case, especially hNLDN which had the lowest mean error and bias and highest correlation among all the cases.
Most of the NOx produced by lightning is distributed in the middle and upper troposphere with only a small portion being distributed close to the surface. As a result, the impact on ground-level NOx mixing ratios is small. Table 2 shows all the model cases produced similar statistics for the daily mean NOx mixing ratios at AQS sites across the domain and within all the subregions. Although the changes in model performance are small, the model cases with LNO exhibit similar or slightly better performance than the Base case.
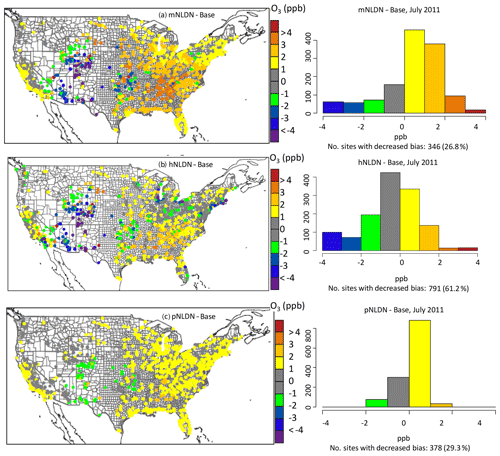
Figure 5Spatial maps of the mean bias of DM8HR O3 (model – observation) differences between model case with LNOx and the Base as well as the corresponding histograms indicating the number of sites with decreased mean bias for each pair of model cases in July 2011.
3.1.2 Time series
Figure 2 presents time series of regional-mean observed and modeled DM8HR O3 for the entire domain and the SE and RM regions during July 2011. Over the domain and in SE, all the model cases overestimate the mean DM8HR O3 mixing ratios on all days with the Base being the closest to the observations. The hNLDN is almost the same as the Base with slightly higher values on some days. Among all the cases, mNLDN produced the highest values on almost all days through the month, on the order of 1–2 ppb higher than the Base. In contrast, in the RM region, the Base significantly underestimates DM8HR O3 mixing ratios on all the days during the month, while all model cases with LNO improved model predictions relative to observations in the region. Among the three model cases with LNO, mNLDN produced the lowest bias for all the days, closely followed by hNLDN.
Figure 3 displays the average daily mean NOx mixing ratios at AQS sites over the same regions as in Fig. 2. On most of the days in July 2011, over the domain and in the SE, the model overestimate NOx values, and on almost half of the days the overestimation is significant (up to 100 %). As noted in Table 2, on average, the overestimation is ∼17 % over the domain and ∼43 % in SE. However in RM, the predicted NOx mixing ratios closely follow the daily observations and on average the modeled and observed magnitude is almost identical (∼3 % difference). All the model cases, with or without LNO, produced almost the same mean NOx mixing ratios at the surface. However, the different cases produce different levels of LNO in the middle and upper troposphere, resulting in differences in O3 production and transport which impact radiative forcing and also downwind ground-level O3 levels. We further explore these features in Sect. 3.2 which presents evaluation of modeled vertical pollutant distributions.
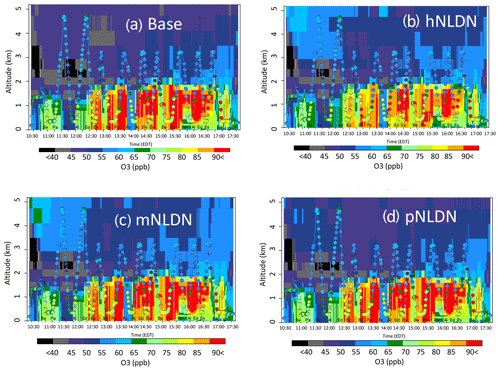
Figure 7Overlay of P-3B-observed O3 (1 min mean values) over the corresponding vertical cross sections of simulated values extracted at the flying locations on 28 July 2018, (a) Base, (b) hNLDN (c) mNLDN, and (d) pNLDN. The letters marked at the bottom of the plots are P-3B spiral sites, Be: Beltsville, Pa: Padonia, Fa: Fair Hill, Al: Aldino, Ed: Edgewood, and Es: Essex.
3.1.3 Diurnal variations
Diurnal plots are used to further examine differences in model evaluation for O3 and NOx. Figure 4 shows the mean diurnal profiles for hourly O3 and NOx over the entire domain, SE, and RM. On a domain mean basis, all model cases overestimate O3 during the daytime hours, while in the SE the overestimation spans all the hours. In RM, the model cases significantly underestimate O3 across all the hours except for a few early morning hours, when the model predicted values are very close to the observations. Among all the model cases, as expected, the most prominent differences occurred during the midday hours when the photochemistry is most active. However, the difference between hNLDN (and mNLDN) and the Base is also significant during the night in the RM region, even though the O3 levels are low. This may be attributed to NOx-related nighttime chemistry in part caused by freshly released NO by cloud-to-ground lightning flashes. The diurnal variations of NOx are similar over the domain and in the regions for all model cases. Appel et al. (2017) reported a significant overestimation of NOx mixing ratios at AQS sites during nighttime hours and underestimation during daytime hours. The bias pattern is identical for all of the LNO model cases evaluated here (Fig. 4).
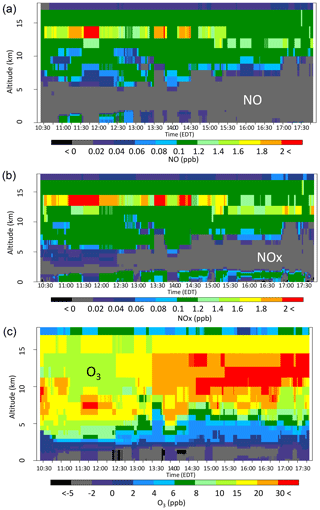
Figure 8The vertical-time difference between hNLDN and Base during the P-3B flight period on 28 July 2011 for (a) NO, (b) NOx, and (c) O3.
3.1.4 Spatial variations
Figure 5 shows the impact of the different LNO schemes on model performance for DM8HR O3 at AQS sites. The spatial maps show the difference in absolute MB between the cases with lightning NOx emissions and the Base and is calculated as follows. First, the absolute MB was calculated at each site for each case, e.g., , then the difference in absolute MB was calculated between model cases, e.g., –. The histograms of the differences in absolute MB between model cases in Fig. 5 are provided to show the distribution of the change in model performance across space, i.e., the frequency of an improvement in model performance versus a degradation in model performance between cases. As shown in Fig. 5, the mNLDN shows increased model bias in the east US and along the California coast, but reduced model bias in the RM. At a majority of the AQS sites, it increases the model bias (only decreases at 26.8 % (346) of the sites). The hNLDN also significantly reduces model bias in the RM with a moderate increase in the SE. Overall, in the hNLDN, the mean bias decreased at 61.2 % (791) of AQS sites. Similar to mNLDN, increases in mean bias are noted at 29.3 % (378) of the AQS sites in the pNLDN simulation. As noted in the histograms, the distribution of the model bias in the pNLDN is much narrower than both mNLDN and hNLDN, eliminating the large bias increases in mNLDN and the significant bias decreases in hNLDN.
3.2 Vertical evaluation for O3 and NOx
3.2.1 Ozone-sonde observations
A large source of uncertainty in the specification of LNO is its vertical allocation, which can impact the model's ability to accurately represent the variability in both chemistry and transport. To further assess the impact of the vertical LNO specification on model results, we compared vertical profiles of simulated model O3 with extensive ozonesonde measurements available during the study period. Figure 6 presents the vertical profiles for O3 sonde measurements and paired model estimates of all model cases at Beltsville, MD, and Edgewood, MD. At each location, observations from multiple days are available (one or two soundings per day) during the 2011 DISCOVER-AQ campaign in July 2011. The model evaluation was limited to days where the inclusion of LNO has an obvious impact (the mean vertical profiles of LNO cases are separable from that of the Base case) on the model estimates (21, 22, 28, and 29 July at Beltsville, and 21, 22, 28, 29, and 30 July at Edgewood). We paired the observed data with model estimates in time and space and averaged the model and observed values at each model layer. Only data below 12 km altitude are plotted in Fig. 6 to exclude possible influence of stratospheric air on O3. As can be seen in Fig. 6, at both locations the Base case underestimates O3 mixing ratios above about 1 km, but overestimates values closer to the surface. When LNO is included in the simulations, the predicted O3 mixing ratios increase relative to the Base case starting around 2 km, with greater divergence from the Base case at higher altitudes. The two model cases, hNLDN and mNLDN, produced similar O3 levels from the surface to about 6 km, but above that altitude the mNLDN ozone mixing ratios were higher. All the model cases with LNO performed much better aloft than the Base case. Near the surface, all the model cases overestimated O3, however hNLDN had smaller bias than the other simulations. This may be attributed to the fact that only hNLDN used the observed lightning flash data directly, and as a result, LNO was estimated more accurately in time and space. This improvement in model bias at the surface is further investigated in the next section using evaluation against P-3B measurements.
3.2.2 P-3B measurement
Extensive measurements of lower tropospheric chemical composition distributions over the northeastern US are available from instruments onboard the P-3B aircraft on 14 d of the DISCOVER-AQ campaign. We utilize measurements from one of the days (28 July 2011) with noticeable (the mean vertical profiles of LNO cases are separable from that of the Base case) lightning impacts, to evaluate the model simulations. Figure 7 shows measured O3 mixing ratios overlaid on the modeled vertical time section for 10:30–17:30 UTC. The color-filled circles represent measured O3 mixing ratios averaged over 60 s and the background is the model estimated vertical profiles from the grid cells containing the P-3B flight path for that hour and location. As indicated in the Base case (Fig. 7a), the model tends to overestimate O3 mixing ratios from the surface to about 2 km, but it tends to underestimate at altitudes above 2 km. The hNLDN reduced the overestimation below 2 km, e.g., fewer grid cells with mixing ratios above 90 ppb (shown in red). The other two cases (mNLDN and pNLDN) did not produce the same improvement near the surface. The hNLDN also decreases the underestimation aloft compared to the Base case with O3 mixing ratios in the 55–65 ppb range (light blue colors), better matching the measured values. This decrease in underestimation aloft is also seen in the mNLDN case, but to a lesser degree while the pNLDN case shows only slight improvement aloft over the Base simulation.
To further differentiate the three LNO model cases, Figs. 8–10 show the difference in the time sections between each of the model cases with LNO and the Base for NO, NOx, and O3 from all the model layers along the P-3B flight path on 28 July. As seen in Fig. 8, the hNLDN scheme injected most NO above 5 km with a peak between 13 and 14 km and only a small amount near the surface. After release into the atmosphere, NO is quickly converted into NO2 in the presence of O3, and these collectively result in the NOx vertical time section (local production plus transport) shown in the middle panel of Fig. 8. NOx is further mixed down through the time section and is more persistent along the flight path near the surface than NO is. As a result, significant O3 is produced above 3 km, and the maximum O3 difference appears between 9 and 14 km during the early afternoon hours (from 13:30 to 17:30 Eastern Daylight Time). However, from surface to about 2 km, O3 is reduced consistently across the entire period, and this is the result of O3 titration by NO from cloud-to-ground lightning flashes that must have been transported to this layer by storm downdrafts. Since O3 is significantly underestimated above 3 km and overestimated near the surface by the Base model, the inclusion of LNO greatly improved the model's performance under both conditions.
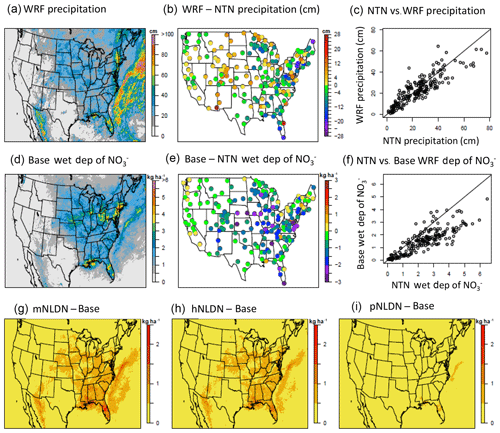
Figure 12(a)–(c) shows precipitation estimates from WRF (a), the bias in the WRF predicted precipitation at NTN locations (b), and the corresponding scatter plots (c). (d)–(f) shows wet deposition (dep) of nitrate estimates from the Base simulation (d), the bias in the Base model estimates of wet deposition of at NADP NTN locations (e), and the corresponding scatter plots (f). (g)–(i) shows the difference in the LNOx sensitivity simulations and the Base case estimates of wet deposition of for mNLDN – Base (g); hNLDN – Base (h), and pNLDN – Base (i). All maps are based on accumulated values (precipitation or wet deposition) during June–August 2011. Precipitation totals are in centimeters (cm) and wet deposition totals are in kilograms per hectare (kg ha−1).
Table 3Statistics of June–August 2011 accumulated precipitation (cm) and wet deposition of nitrate () for all model cases over the domain. The best performance metrics among the model cases are highlighted in bold.
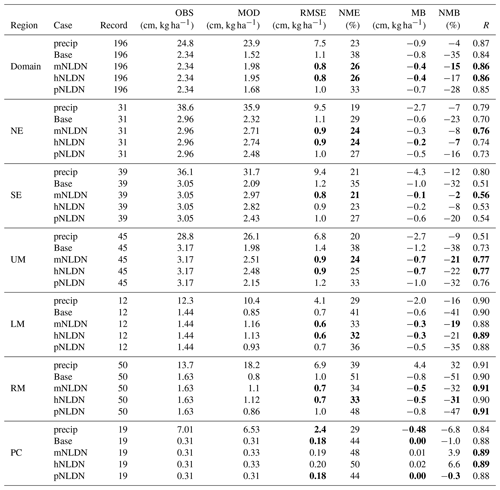
Comparison of Fig. 9 (mNLDN) with Fig. 8 (hNLDN) reveals that the time sections of NO and NOx are similar above 5 km but dramatically different near the surface. The near-surface increase in ambient NO noted in the hNLDN is absent in mNLDN, and in fact there are some small decreases in NO, although the reason for this is unclear. The increase in O3 aloft in the mNLDN case is similar to that seen in the hNLDN case. However, the near-surface reduction in O3 is almost absent. In the pNLDN case (Fig. 10), NO mixing ratios are much less than those in hNLDN and mNLDN in the upper layers as a result of less column NO being generated by the linear parameterization. The resulting NOx time section is also smoothed. The pNLDN time sections for NO, NOx, and O3 near the surface are similar to the mNLDN case with no change or small decreases compared to the Base case. O3 mixing ratios increase by more than 30 ppb during the afternoon hours between 10 and 13 km in the pNLDN case, however the increase is not as intense and widespread as the other cases. In summary, the hNLDN scheme produces estimates that are more consistent with measurements at the surface and aloft, compared to the other simulations, reflecting the advantage of using the spatially and temporally resolved observed lightning flash data. The model performance improvement for simulated O3 distributions also suggests robustness in the vertical distribution scheme when LNO is generated at the right time and location.
To corroborate the above time section distributions of NO, NOx, and O3 in the lightning cases, the lightning NO emissions are traced back to 28 July for each case. It is found that in all cases, the lightning NO was injected approximately 200 km upwind (northwest) of the flight path. The hNLDN case captured two injections: one occurred during the morning hours (05:00 to 07:00 EDT) and the other happened during the afternoon hours (after 02:30 EDT). Both mNLDN and pNLDN captured the afternoon lightning event at the later time (after 03:30 EDT for mNLDN and after 04:30 for pNLDN) with varying intensity, but neither captured the morning lightning event, which explains why the increase in NO and NOx in the hNLDN case (Fig. 8) did not occur in the mNLDN and pNLDN cases (Figs. 9 and 10). Also note that the significant increase of NO during the time period from 11:00 to 13:00 EDT occurred about 5 h after the lightning NO was injected at about 200 km upwind in the hNLDN case.
To expand on the evaluation in Figs. 7–10 which focused on measurements from 28 July 2011, we retrieved all the P-3B measurements on days with noticeable lightning impact (21, 22, 28, and 29 July). The 3-D paired observation–model data were grouped together by spiral site and the mean biases (model – observation) were plotted in Fig. 11 (a and b) for O3 and NO, respectively. The boxplots for O3 in Fig. 11a suggests that the Base exhibited larger bias with greater spread (i.e., larger interquartile range) than other model cases incorporating LNO at most of the locations where aircraft spirals were conducted. At all locations except Aldino, the lowest mean biases in simulated NO and O3 are noted in the hNLDN simulation.
3.3 Deposition evaluation for nitrate
In addition to contributing to tropospheric O3 formation, NOx oxidation also leads to gaseous nitric acid and particulate nitrate which are eventually removed from the atmosphere by dry and wet deposition of nitrate (). As a result, inclusion of NOx from lightning also plays an important role in nitrogen deposition modeling. To assess the impacts of incorporating LNO emissions on simulated oxidized nitrogen deposition, we compared model estimated amounts of precipitation from the NTN network (http://nadp.slh.wisc.edu/ntn/, last access: 2 October 2019) and wet deposition of with measurements from the NADP network (http://nadp.slh.wisc.edu/, last access: 2 October 2019). During summer months in 2011 (June–August) the WRF model generally reproduces the observed precipitation with a slight underestimate in the east, but the Base model simulation tends to underestimate wet deposition of across the domain, with the greatest underestimation in the SE and UM (See Table 3 and Fig. 12). All three LNO simulations increase wet deposition amounts of and decrease model bias in all regions. The bottom panel of Fig. 12 shows that the mNLDN simulation resulted in the largest increase over the Base model estimates. The NMB is reduced from −35 % in the Base to −15 % in mNLDN across the domain and from −32 % to −2 % in the SE. The hNLDN shows very similar model performance to the mNLDN case. In contrast, the wet deposition estimates from the pNLDN case are only slightly higher than the Base case, and as a result the evaluation statistics for pNLDN are very similar to the Base statistics. As discussed earlier, the mNLDN tends to produce the most LNO among the three LNO schemes, thus it results in the smallest errors in terms of wet deposition of when compared to the Base simulation that significantly underestimated wet deposition. It should be noted that in addition to the LNO contributions, errors in modeled precipitation amounts and patterns also likely influence the underestimation of wet deposition.
A detailed evaluation of lightning NOx emission estimation parameterizations available in the CMAQ modeling system was performed through comparisons of model simulation results with surface and aloft air quality measurements.
Our analysis indicates that incorporation of LNO emissions enhanced O3 production in the middle and upper troposphere, where O3 mixing ratios were often significantly underestimated without the representation of LNO. Though the impact on surface O3 varies from region to region and is also dependent on the accuracy of the NOx emissions from other sources, the inclusion of LNO, when it is injected at the appropriate time and location, can improve the model estimates. In regions where the Base model estimates of O3 were biased high, the inclusion of LNO further increased the model bias, and a systematic increase is noted in the correlation with measurements, suggesting that emissions from other sources likely drive the overestimation. Identifying how errors in emission inputs from different sources interact with errors in meteorological modeling of mixing and transport remains a challenging but critical task. Likewise, all the LNO schemes also enhanced the accumulated wet deposition of that was significantly underestimated by the Base model without LNO throughout the modeling domain except the Pacific Coast.
Uncertainty remains in modeling the magnitude and spatial, temporal, and vertical distribution of lightning produced NOx. LNO schemes are built on numerous assumptions and all current schemes also depend on the skill of the upstream meteorological models in describing convective activity. Nevertheless, these schemes reflect our best understanding and knowledge at the time when the schemes were implemented. The use of hourly information on lightning activity yielded LNO emissions that generally improved model performance for ambient O3 and NOx as well as oxidized nitrogen wet deposition amounts. As more high-quality data from both ground and satellite measurements become available, the performance of the LNO schemes will continue to improve.
Since the pNLDN scheme was developed using historical data correlating lightning activity with convective precipitation, the scheme could be employed for applications involving air quality forecasting and future projections when observed lightning information is not available.
CMAQ model documentation and released versions of the source code, including all model code used in his study, are available at https://doi.org/10.5281/zenodo.1167892 (US EPA Office of Research and Development, 2017).
The data processing and analysis scripts are available upon request. The WRF model is available for download through the WRF website (http://www2.mmm.ucar.edu/wrf/users/wrfv3.8/updates-3.8.html, last access: 2 October 2019; NCAR, 2018).
The raw lightning flash observation data used are not available to the public but can be purchased through Vaisala Inc. (https://www.vaisala.com/en/products/systems/lightning-detection, last access: 2 October 2019; Vaisala, 2019). The lightning data obtained from Vaisala Inc. is the cloud-to-ground lightning flashes over the contiguous United States. The immediate data behind the tables and figures are available from https://zenodo.org/record/3360744 (last access: 2 October 2019; Kang and Foley, 2019). Additional input and output data for CMAQ model utilized for this analysis are also available upon request.
DK collected the data, designed the algorithm, performed the model simulation, carried out the analysis, and wrote the paper. KF performed the data analysis and was involved in the writing of the paper. RM, SR, KP, and DA edited the paper.
The authors declare that they have no conflict of interest.
The views expressed in this paper are those of the authors and do not necessarily represent the views or policies of the U.S. EPA.
The authors thank Brian Eder, Golam Sarwar, and Janet Burke (U.S. EPA) for their constructive comments and suggestions during the internal review process.
This paper was edited by Slimane Bekki and reviewed by two anonymous referees.
Allen, D. J. and Pickering, K. E.: Evaluation of lightning flash rate parameterizations for use in a global chemical transport model, J. Geophys. Res., 107, 4711–4731, https://doi.org/10.1029/2002JD002066, 2002.
Allen, D., Pickering, K., Stenchikov, G., Thompson, A., and Kondo, Y.: A three-dimensional total odd nitrogen (NOy) simulation during SONEX using a stretched-grid chemical transport model, J. Geophys. Res., 105, 3851–3876, https://doi.org/10.1029/1999JD901029, 2000.
Allen, D. J., Pickering, K. E., Pinder, R. W., Henderson, B. H., Appel, K. W., and Prados, A.: Impact of lightning-NO on eastern United States photochemistry during the summer of 2006 as determined using the CMAQ model, Atmos. Chem. Phys., 12, 1737–1758, https://doi.org/10.5194/acp-12-1737-2012, 2012.
Anderson, D. C., Loughner, C. P., Diskin, G., Weinheimer, A., Canty, T. P., Salawitch, R. J, Worden, H. M., Fried, A., Mikoviny, T., Wisthaler, A., and Dickerson, R. R.: Measured and modeled CO and NOy in DISCOVER-AQ: An evaluation of emissions and chemistry over the eastern US, Atmos. Environ., 96, 78–87, https://doi.org/10.1016/j.atmosenv.2014.07.004, 2014.
Appel, K. W., Foley, K. M., Bash, J. O., Pinder, R. W., Dennis, R. L., Allen, D. J., and Pickering, K.: A multi-resolution assessment of the Community Multiscale Air Quality (CMAQ) model v4.7 wet deposition estimates for 2002–2006, Geosci. Model Dev., 4, 357–371, https://doi.org/10.5194/gmd-4-357-2011, 2011.
Appel, K. W., Napelenok, S. L., Foley, K. M., Pye, H. O., Hogrefe, C., Luecken, D. J., Bash, J. O., Roselle, S. J., Pleim, J. E., Foroutan, H., Hutzell1, W. D., Pouliot, G. O., Sarwar, G., Fahey, K. M., Gantt, G., Gilliam, R. C., Heath, N. K., Kang, D., Mathur, R., Schwede, D. B., Spero, T. L., Wong, D. C., and Young, J. O.: Description and evaluation of the Community Multiscale Air Quality (CMAQ) modeling system version 5.1, Geosci. Model Dev., 10, 1703–1732, https://doi.org/10.5194/gmd-10-1703-2017, 2017.
Bash, J. O., Baker, K. R., and Beaver, M. R.: Evaluation of improved land use and canopy representation in BEIS v3.61 with biogenic VOC measurements in California, Geosci. Model Dev., 9, 2191–2207, https://doi.org/10.5194/gmd-9-2191-2016, 2016.
Bovensmann, H., Burrows, J. P., Buchwitz, M., Frerick, J., Noël, S., Rozanov, V. V., Chance, K. V., and Goede, A. P. H.: SCIAMACHY: Mission Objectives and Measurement Modes, J. Atmos. Sci., 56, 127–150, 1999.
Brown-Steiner, B., Hess, P. G., and Lin, M. Y.: On the capabilities and limitations of GCCM simulations of summertime regional air quality: A diagnostic analysis of ozone and temperature simulations in the US using CESM CAM-Chem, Atmos. Environ., 101, 134–148, https://doi.org/10.1016/j.atmosenv.2014.11.001, 2015.
Byun, D. W. and Schere, K. L.: Rewiew of the governing equations, computational algorithms, and other components of the Models-3 Community Multiscale Air Quality (CMAQ) modeling system, Appl. Mech. Rev., 59, 51–77, 2006.
Canty, T. P., Hembeck, L., Vinciguerra, T. P., Anderson, D. C., Goldberg, D. L., Carpenter, S. F., Allen, D. J., Loughner, C. P., Salawitch, R. J., and Dickerson, R. R.: Ozone and NOx chemistry in the eastern US: evaluation of CMAQ/CB05 with satellite (OMI) data, Atmos. Chem. Phys., 15, 10965–10982, https://doi.org/10.5194/acp-15-10965-2015, 2015.
Choi, Y., Wang, Y., Zeng, T., Martin, R. V., Kurosu, T. P., and Chance, K.: Evidence of lightning NOx and convective transport of pollutants in satellite observations over North America, Geophys. Res. Lett., 32, L02805, https://doi.org/10.1029/2004GL021436, 2005.
Crawford, J. H. and Pickering, K. E.: DISCOVER-AQ: Advancing strategies for air quality observations for the next decade, EM, A&WMA, September, 2014.
Eder, B. K., Kang, D., Mathur, R., Yu, S., and Schere, K.: An operational evaluation of the Eta-CMAQ air quality forecast model, Atmos. Environ., 40, 4894–4905, 2006.
Finney, D. L., Doherty, R. M., Wild, O., Huntrieser, H., Pumphrey, H. C., and Blyth, A. M.: Using cloud ice flux to parametrize large-scale lightning, Atmos. Chem. Phys., 14, 12665–12682, https://doi.org/10.5194/acp-14-12665-2014, 2014.
Finney, D. L., Doherty, R. M., Wild, O., and Abraham, N. L.: The impact of lightning on tropospheric ozone chemistry using a new global lightning parameterization, Atmos. Chem. Phys., 16, 7507–7522, https://doi.org/10.5194/acp-16-7507-2016, 2016.
Fiore, A. M., Dentener, F. J., Wild, O., Cuvelier, C., Schultz, M. G., Hess, P., Textor, C., Schulz, M., Doherty, R. M., Horowitz, L. W., MacKenzie, I. A., Sanderson, M. G., Shindell, D. T., Stevenson, D. S., Szopa, S., Van Dingenen, R., Zeng, G., Atherton, C., Bergmann, D., Bey, I., Carmichael, G., Collins, W. J., Duncan, B. N., Faluvegi, G., Folberth, G., Gauss, M., Gong, S., Hauglustaine, D., Holloway, T., Isaksen, I. S. A., Jacob, D. J., Jonson, J. E., Kaminski, J. W., Keating, T. J., Lupu, A., Marmer, E., Montanaro, V., Park, R. J., Pitari, G., Pringle, K. J., Pyle, J. A., Schroeder, S., Vivanco, M. G., Wind, P., Wojcik, G., Wu, S., and Zuber, A.: Multimodel estimates of intercontinental sourcereceptor relationships for ozone pollution, J. Geophys. Res., 114, D04301, https://doi.org/10.1029/2008jd010816, 2009.
Flatoy, F. and Hov, O.: NOx from lightning and the calculated chemical composition of the free troposphere, J. Geophys. Res.-Atmos., 102, 21373–21381, https://doi.org/10.1029/97JD01308, 1997.
Foley, K. M., Roselle, S. J., Appel, K. W., Bhave, P. V., Pleim, J. E., Otte, T. L., Mathur, R., Sarwar, G., Young, J. O., Gilliam, R. C., Nolte, C. G., Kelly, J. T., Gilliland, A. B., and Bash, J. O.: Incremental testing of the Community Multiscale Air Quality (CMAQ) modeling system version 4.7, Geosci. Model Dev., 3, 205–226, https://doi.org/10.5194/gmd-3-205-2010, 2010.
Follette-Cook, M. B., Pickering, K. E., Crawford, J. H., Duncan, B. N., Loughner, C. P., Diskin, G. S., Fried, A., and Weinheimer, A. J.: Spatial and temporal variability of trance gas columns derived from WRF/Chem regional model output: Planning for geostationary observations of atmospheric composition, Atmos. Environ., 118, 28–44, https://doi.org/10.1016/j.atmosenv.2015.07.024, 2015.
Kang, D. and Foley, K.: Simulating Lightning NO Production in CMAQv5.2: Performance Evaluations, data set, https://doi.org/10.5281/zenodo.3360744, 2019.
Kang, D. and Pickering, K. E.: Lightning NOx emissions and the Implications for Surface Air Quality over the Contiguous United States, EM, A&WMA, November, 2018.
Kang, D., Eder, B. K., Stein, A. F., Grell, G. A., Peckham, S. E., and Mchenry, J.: The New England air quality forecasting pilot program: development of an evaluation protocol and performance benchmark, J. Air Waste Manage. Assoc., 55, 1782–1796, 2005.
Kang, D., Pickering, K. E., Allen, D. J., Foley, K. M., Wong, D., Mathur, R., and Roselle, S. J.: Simulating Lightning NO Production in CMAQv5.2: Evolution of Scientific Updates, Geosci. Model Dev., 12, 3071–3083, https://doi.org/10.5194/gmd-12-3071-2019, 2019.
Kaynak, B., Hu, Y., Martin, R. V., Russell, A. G., Choi, Y., and Wang, Y.: The effect of lightning NOx production on surface ozone in the continental United States, Atmos. Chem. Phys., 8, 5151–5159, https://doi.org/10.5194/acp-8-5151-2008, 2008.
Koo, B., Chien, C. J., Tonnesen, G., Morris, R., Johnson, J., Sakulyanontvittaya T., Piyachaturawat, P., and Yarwood, G.: Natural emissions for regional modeling of background ozone and particulate matter and impacts on emissions control strategies. Atmos Environ., 44, 2372–2382, https://doi.org/10.1016/j.atmosenv.2010.02.041, 2010.
Koshak, W., Peterson, H., Biazar, A., Khan, M., and Wang, L.: The NASA Lightning Nitrogen Oxides Model (LNOM): Application to air quality modeling, Atmos. Res., 135–136, 363–369, https://doi.org/10.1016/j.atmosres.2012.12.015, 2014.
Labrador, L. J., von Kuhlmann, R., and Lawrence, M. G.: The effects of lightning-produced NOx and its vertical distribution on atmospheric chemistry: sensitivity simulations with MATCH-MPIC, Atmos. Chem. Phys., 5, 1815–1834, https://doi.org/10.5194/acp-5-1815-2005, 2005.
Lin, J., Youn, D., Liang, X., and Wuebbles, D.: Global model simulation of summertime U.S. ozone diurnal cycle and its sensitivity to PBL mixing, spatial resolution, and emissions, Atmos. Environ., 42, 8470–8483, https://doi.org/10.1016/j.atmosenv.2008.08.012, 2008.
Murray, L. T.: Lightning NOx and Impacts on Air Quality, Curr Pollution Rep., 2, 115–133, https://doi.org/10.1007/s40726-016-0031-7, 2016.
Napelenok, S. L., Pinder, R. W., Gilliland, A. B., and Martin, R. V.: A method for evaluating spatially-resolved NOx emissions using Kalman filter inversion, direct sensitivities, and spacebased NO2 observations, Atmos. Chem. Phys., 8, 5603–5614, https://doi.org/10.5194/acp-8-5603-2008, 2008.
NCAR: WRF Model vrsion 3.8, updates, available at: http://www2.mmm.ucar.edu/wrf/users/wrfv3.8/updates-3.8.html (last access: 2 October 2019), 2018.
Nolte, C. G., Appel, K. W., Kelly, J. T., Bhave, P. V., Fahey, K. M., Collett Jr., J. L., Zhang, L., and Young, J. O.: Evaluation of the Community Multiscale Air Quality (CMAQ) model v5.0 against size-resolved measurements of inorganic particle composition across sites in North America, Geosci. Model Dev., 8, 2877–2892, https://doi.org/10.5194/gmd-8-2877-2015, 2015.
Novak, J. H. and Pierce, T. E.: Natural emissions of oxidant precursors, Water Air Soil Poll., 67, 57–77, 1993.
Orville, R. E., Huffines, G. R., Burrows, W. R., Holle, R. L., and Cummins, K. L.: The North American Lightning Detection Network (NALDN) – first results: 1998–2000, Mon. Weather Rev., 130, 2098–2109, 2002.
Otte, T. L. and Pleim, J. E.: The Meteorology-Chemistry Interface Processor (MCIP) for the CMAQ modeling system: updates through MCIPv3.4.1, Geosci. Model Dev., 3, 243–256, https://doi.org/10.5194/gmd-3-243-2010, 2010.
Pickering, K. E., Bucsela, E., Allen, D., Ring, A., Holzworth, R., and Krotkov, N.: Estimates of lightning NOx production based on OMI NO2 observations over the Gulf of Mexico, J. Geophys. Res.-Atmos., 121, 8668–8691, https://doi.org/10.1002/2015JD024179, 2016.
Price, C. and Rind, D.: A simple lightning parameterization for calculating global lightning distributions, J. Geophys. Res., 97, 9919–9933, https://doi.org/10.1029/92JD00719, 1992.
Price, C., Penner, J., and Prather, M.: NOx from lightning. 2. Constraints from the global atmospheric electric circuit, J. Geophys. Res., 102, 5943–5951, https://doi.org/10.1029/96JD02551, 1997.
Richter, A., Burrows, J. P., Nüß, H., Granier, C., and Niemeier, U.: Increase in tropospheric nitrogen dioxide over China observed from space, Nature, 437, 129–132, https://doi.org/10.1038/nature04092, 2005.
Rossow, W. B., Walker, A. W., Beuschel, D. E., and Roiter, M. D.: International Satellite Cloud Climatology Project (ISCCP) documentation of new cloud data sets, Tech. Rep. January, World Meteorological Organisation, WMO/TD 737, Geneva, 1996.
Schumann, U. and Huntrieser, H.: The global lightning-induced nitrogen oxides source, Atmos. Chem. Phys., 7, 3823–3907, https://doi.org/10.5194/acp-7-3823-2007, 2007.
Simon, H., Reff, A., Wells, B., Xing, J., and Frank, N.: Ozone trends across the United States over a period of decreasing NOx and VOC emissions. Environ. Sci. Technol., 49, 186–195, 2015.
Sioris, C. E., Kurosu, T. P., Martin, R. V., and Chance, K.: Stratospheric and tropospheric NO2 observed by SCIAMACHY: first results, Adv. Space Res., 34, 780–785, 2004.
Smith, S. N. and Mueller, S. F.: Modeling natural emissions in the Community Multiscale Air Quality (CMAQ) Model-I: building an emissions data base, Atmos. Chem. Phys., 10, 4931–4952, https://doi.org/10.5194/acp-10-4931-2010, 2010.
Stockwell, D. Z., Giannakopoulos, C., Plantevin, P. H., Carver, G. D., Chipperfield, M. P., Law, K. S., Pyle, J. A., Shallcross, D. E., and Wang, K. Y.: Modelling NOx from lightning and its impact on global chemical fields, Atmos. Environ., 33, 4477–4493, 1999.
US EPA Office of Research and Development: CMAQ (Version 5.2), Zenodo, https://doi.org/10.5281/zenodo.1167892, 2017.
Vaisala: Lightning Detection, available at: https://www.vaisala.com/en/products/systems/lightning-detection, last access: 2 October 2019.
Wang, L., Newchurch, M. J., Pour-Biazar, A., Kuang, S., Khan, M., Liu, X., Koshak, W., and Chance, K.: Estimating the influence of lightning on upper tropospheric ozone using NLDN lightning data and CMAQ model, Atmos. Environ., 67, 219–228, 2013.
Yarwood, G., Whitten, G. Z., Jung, J., Heo, G., and Allen, D. T.: Final Report: Development, Evaluation and Testing of Version 6 of the Carbon Bond Chemical Mechanism (CB6), available at: https://www.tceq.texas.gov/assets/public/implementation/air/am/contracts/reports/pm/5820784005FY1026-20100922-environ-cb6.pdf (last access: 2 October 2019), 2010.