the Creative Commons Attribution 4.0 License.
the Creative Commons Attribution 4.0 License.
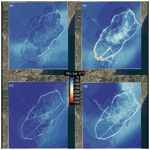
CALIOPE-Urban v1.0: coupling R-LINE with a mesoscale air quality modelling system for urban air quality forecasts over Barcelona city (Spain)
Jaime Benavides
Michelle Snyder
Marc Guevara
Albert Soret
Carlos Pérez García-Pando
Fulvio Amato
Xavier Querol
The NO2 annual air quality limit value is systematically exceeded in many European cities. In this context, understanding human exposure, improving policy and planning, and providing forecasts requires the development of accurate air quality models at the urban (street level) scale. We describe CALIOPE-Urban, a system coupling CALIOPE – an operational mesoscale air quality forecast system based on the HERMES (emissions), WRF (meteorology) and CMAQ (chemistry) models – with the urban roadway dispersion model R-LINE. Our developments have focused on Barcelona city (Spain), but the methodology may be replicated for other cities in the future. WRF drives pollutant dispersion and CMAQ provides background concentrations to R-LINE. Key features of our system include the adaptation of R-LINE to street canyons, the use of a new methodology that considers upwind grid cells in CMAQ to avoid double counting traffic emissions, a new method to estimate local surface roughness within street canyons, and a vertical mixing parameterisation that considers urban geometry and atmospheric stability to calculate surface level background concentrations. We show that the latter is critical to correct the night-time overestimations in our system. Both CALIOPE and CALIOPE-Urban are evaluated using two sets of observations. The temporal variability is evaluated against measurements from five traffic sites and one urban background site for April–May 2013. While both systems show a fairly good agreement at the urban background site, CALIOPE-Urban shows a better agreement at traffic sites. The spatial variability is evaluated using 182 passive dosimeters that were distributed across Barcelona during 2 weeks for February–March 2017. In this case, the coupled system also shows a more realistic distribution than the mesoscale system, which systematically underpredicts NO2 close to traffic emission sources. Overall CALIOPE-Urban improves mesoscale model results, demonstrating that the combination of both scales provides a more realistic representation of NO2 spatio-temporal variability in Barcelona.
- Article
(13827 KB) - Full-text XML
- BibTeX
- EndNote
Persistent exposure to high NO2 atmospheric concentrations in cities causes detrimental health effects (e.g. Sunyer et al., 2015; Barone-Adesi et al., 2015). In 2016, 19 out of the 28 European Union (EU) countries reported NO2 exceedances of the annual air quality limit value (40 µg m−3) mostly at urban traffic monitoring stations (EEA, 2018). In this context there is a need for NO2 data at the street level in urban areas that enable individuals and communities to mitigate the problem by, for example, walking in less polluted streets or reducing traffic in school areas. However, both the poor density of air quality monitoring stations and the resolution of mesoscale air quality modelling systems (on the order of 1 km grid resolution) do not adequately represent the NO2 concentration gradients that typically occur near heavily trafficked streets (Duyzer et al., 2015; Borge et al., 2014). Urban dispersion models can estimate these gradients but their use has been typically limited to historic periods, partly because the needed background concentrations and meteorological input have been approximated using observations (Vardoulakis et al., 2003).
In order to overcome these limitations, coupling the regional and urban scales offline by downscaling the regional model using a dispersion kernel has been successfully applied in some cities (Beevers et al., 2012; Moussafir et al., 2014; Isakov et al., 2014; Jensen et al., 2017; Maiheu et al., 2017; Kim et al., 2018; Hood et al., 2018; Fagerli et al., 2019). For instance, Hood et al. (2018) coupled a regional climate–chemistry model with 5 km horizontal resolution (EMEP4UK) with the fine-scale model ADMS-URBAN to simulate air quality over London in 2012. They compared the coupled system results with the regional model and the fine-scale model run separately. Authors found that both the fine-scale model and the coupled system performed better than the regional for NO2 at both annual mean and hourly concentration levels due to the explicit treatment of traffic emissions within the city. In addition, Jensen et al. (2017) estimated annual NO2 concentrations at 2.4 million addresses in Denmark using the Operational Street Pollution Model (OSPM) coupled with the Danish Eulerian Hemispheric Model (DEHM) for regional background concentrations and the Urban Background Model (UBM) for urban background obtaining a good correlation in Copenhagen (r2=0.70) against 98 measurement sites for NO2 in the year 2012. Maiheu et al. (2017) covered a broader spatial context, estimating EU-wide NO2 annual average levels at 100 m resolution with a regional model coupled with a dispersion kernel-based method. The approach does not produce hourly concentration levels and approximates road-link level traffic emissions by distributing the regional grid cell traffic emissions to each road-link based on road capacity. Hence, it provides more spatial detail than previous EU-scale NO2 assessment studies, but more specific methods are required to resolve air quality in cities. In this sense, there is a lack of air quality urban forecasting methodologies that can be applied to a diverse range of cities and that consistently resolve at least some of the major challenges already identified by the community, i.e. (1) downscaling regional meteorology to the street level as required to drive pollutant dispersion and (2) obtaining background concentrations from the mesoscale system avoiding the double counting of traffic emissions. Additionally, we consider vertical mixing with background air a key process to be resolved when coupling the regional and urban scales.
Different approaches to downscale mesoscale meteorology are found in the research literature. Brousse et al. (2016) applied the Weather Research and Forecasting meteorological model (WRF) using the building effect parameterisation (Martilli et al., 2002) over Madrid considering WUDAPT Local Climate Zone data (Bechtel et al., 2015). This approach increases the mesoscale model's ability to resolve urban processes but does not reproduce the specific meteorological conditions in each street as required by dispersion models. Kochanski et al. (2015) used a simplified computational fluid dynamics model in combination with WRF to estimate wind conditions at the street level. Hood et al. (2018) estimate an urban canopy flow field at the same resolution of the regional model. This calculation is based on the variation in surface roughness within the city. This approach includes the variation in some atmospheric stability parameters (e.g. friction velocity), but it neglects the variation in vertical mixing with background air depending on atmospheric stability and urban geometry. Conversely, Jensen et al. (2017) do not consider atmospheric stability within the street canyon model OSPM and within the vertical mixing with background air. The approach presented here to downscale mesoscale meteorology to the street scale describing wind conditions and atmospheric stability in each street can be a promising solution to drive dispersion models and vertical mixing.
Background concentrations can be obtained from observations or mesoscale models, which are commonly used in forecasting applications. However, coupling mesoscale and urban dispersion models can lead to a double counting of traffic emissions. To avoid double counting, Arunachalam et al. (2014) multiply urban background site observations by an estimated ratio between two mesoscale air quality simulations. The first run contains all the emission sources and the second neglects traffic emissions. Lefebvre et al. (2011) and Stocker et al. (2014) run first the urban dispersion model at mesoscale grid resolution with only traffic emissions and subtract its result from the mesoscale model simulation, which includes all the emission sources. Then, street-scale model outputs are added to the result from the prior computation at finer resolution. Although these methods avoid double counting emissions they do not explicitly account for vertical mixing, a process that occurs at the intersection of regional and street scales. Urban air quality models such as SIRANE (Soulhac et al., 2011) have already implemented vertical mixing depending on local meteorology. In this study, we will show that this process may be relevant and explain some systematic errors found in the literature: night-time NO2 concentration values tend to be overestimated and afternoon values tend to be underestimated in traffic areas (e.g. Hood et al., 2018). Further efforts are necessary to explicitly resolve processes happening among scales and to correct these biases in the mentioned periods of the day.
This work describes a methodology to couple the mesoscale air quality forecasting system CALIOPE (Baldasano et al., 2011) with the Research LINE source dispersion model (RLINE; Snyder et al., 2013) and its evaluation over the city of Barcelona, Spain. In Barcelona, chronic NO2 exceedances have been recorded since the year 2000, and according to the local public health agency about 68 % of citizens were exposed to NO2 levels above the annual air quality limit value in 2016 (ASPB, 2017). Barcelona has a very high vehicle density (approx. 5500 vehicles km−2) and the majority of passenger cars are diesel (65 %) (Barcelona City Council, 2017). Located in the northeast of the Iberian Peninsula, Barcelona is surrounded by the Mediterranean Sea, two rivers and a mountain range. Due to its coastal emplacement, during the warm season, transport and dispersion of air pollutants within the city are dominated by the breeze blowing in from the sea during daytime and from the land during night-time. This pattern persists under the presence of high-pressure systems accompanied by clear skies and warm temperatures in the summer season. In contrast, the winter season is dominated by northwestern advection, typically cleaning the atmosphere of the city (Jorba et al., 2011). Our aim is to produce more accurate NO2 concentrations with CALIOPE-Urban, the coupled system, than with the mesoscale system alone and give a more realistic representation of NO2 spatial distribution and temporal variability across the city. To achieve these objectives a set of system enhancements has been implemented: an adaptation of R-LINE to dense urban areas (e.g. street canyons), a background model to estimate background concentrations at roof level, a parameterisation of the vertical mixing to estimate background concentrations within the street that considers atmospheric stability and urban geometry, and a local surface roughness parameterisation to estimate turbulent parameters within a street canyon. The mesoscale system has been executed using the operational forecast configuration. We compare the estimated temporal variability of NO2 concentrations from the coupled modelling system with those derived from CALIOPE and with ambient street level measurements (i.e. five traffic sites and one urban background site) in April and May 2013. Its spatial variability is evaluated using a 2-week measurement campaign that deployed 182 NO2 passive dosimeters across Barcelona in February and March 2017.
CALIOPE-Urban estimates hourly NO2 concentrations by coupling the CALIOPE mesoscale air quality forecasting system, providing background concentrations, meteorological data and road-link traffic emissions, with the R-LINE dispersion model adapted to street canyons. Here we introduce and describe the components of the coupled model as depicted in Fig. 1.
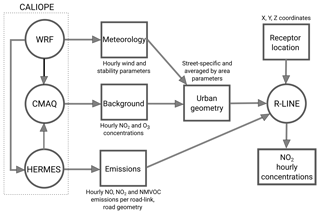
Figure 1CALIOPE-Urban workflow. Models are represented by circles and data by rectangular shapes. CALIOPE is left untouched. Meteorology and background from WRF and CMAQ are combined with urban geometry to create inputs for R-LINE. R-LINE dispersion is left untouched, after adjusting meteorology and surface roughness for local urban geometry.
2.1 Mesoscale air quality forecasting system CALIOPE
CALIOPE (Baldasano et al., 2011) integrates the Weather Research and Forecasting model version 3 (WRF; Skamarock and Klemp, 2008), the High-Elective-Resolution Modelling Emission System (HERMES v2.0; Guevara et al., 2013), the Community Multiscale Air Quality Modeling System version 5.0.2 (CMAQ; Byun and Schere, 2006) and the mineral Dust REgional Atmospheric Model (BSC-DREAM8b; Basart et al., 2012). The mesoscale system is run over Europe at a 12 km×12 km horizontal resolution, Iberian Peninsula at 4 km×4 km and the Catalonian domain, including Barcelona, at 1 km×1 km. CALIOPE results have been evaluated in detail elsewhere (e.g. Pay et al., 2014).
In our system, WRF uses the Global Forecasting System (GFS) model initial/boundary conditions from the National Centers for Environmental Prediction (NCEP) to forecast the mesoscale meteorological conditions. Three nested domains are designed to provide a final high-resolution run over the Catalonian domain. In the vertical, WRF is configured with 38 sigma layers up to 50 hPa, where 11 cover the planetary boundary layer (PBL). Our WRF setup utilises the rapid radiation transfer model for long-wave radiation and Dudhia for short-wave radiation, the Kain–Fritsch cumulus parameterisation, the single-moment three-class microphysics scheme, the Yonsei University PBL scheme, and the Noah land-surface model based on the CORINE land-use data from the year 2006.
For the mesoscale model, pollutant emissions are obtained from the high-resolution emission model HERMES v2.0 gridded up to 1 km×1 km and temporal (1 h) resolution. HERMES v2.0 estimates atmospheric emissions for Europe and Spain according to the Selected Nomenclature for Air Pollution (SNAP) and taking the year 2009 as the reference period. Emissions are estimated for nitrogen oxides (NOx), non-methane volatile organic compounds (NMVOCs), sulfur dioxide, carbon monoxide, ammonia, total suspended particles, PM10 and PM2.5 fractions. The final model output consists of hourly, gridded and speciated emissions according to the CB05 chemical mechanism used by the chemical transport model CMAQ. For Europe, HERMES v2.0 implements a SNAP sector-dependent spatial, temporal and speciation treatment of the original annual EMEP gridded emissions (Ferreira et al., 2013). For Spain, the model uses a bottom-up approach for pollutant sources including point (e.g. power plants, industries), maritime (e.g. ports), air traffic (e.g. airports), agricultural machinery (e.g. tractors and harvesters) and road transport. For the rest of pollutant sources a combination of top-down approaches (i.e. residential/commercial combustion, energy consumption statistics combined with a population map) and downscaling methodologies (i.e. use of solvents, extraction and distribution of fossil fuels; specific spatial proxies and temporal profiles assigned to the Spanish National Emission Inventory by categories at third level of SNAP) is adopted. The results of the HERMES v2.0 model have been used to support several air quality evaluation and planning studies (e.g. Baldasano et al., 2014; Soret et al., 2014) as well as emission inventory intercomparison exercises (Guevara et al., 2017).
The chemical transport model used in the CALIOPE system is CMAQ v5.0.2. It uses the CB05 gas-phase chemical mechanism, the AERO5 aerosol scheme and an in-line photolysis calculation. CMAQ vertical levels are collapsed from the 38 WRF levels to 15 layers up to 50 hPa with six layers falling within the PBL. We use MOZART-4 as boundary conditions for the European domain.
2.2 Street-scale dispersion model: R-LINE
R-LINE is a near-road Gaussian dispersion model (Snyder et al., 2013) that incorporates state-of-the-art Gaussian dispersion curves (Venkatram et al., 2013) to simulate dispersion of road source emissions. The model resolves either numerically or analytically the integration of the contributions of point sources along a street segment (Snyder and Heist, 2013). The first option is more accurate and the latter spends less time computing dispersion. The analytical version is best suited for near-ground-level sources and receptors. In order to estimate NO2 concentrations, R-LINE incorporates a chemistry module to resolve simple NO to NO2 chemistry with the generic reaction set (GRS; Valencia et al., 2018) considering the chemical reactions in Table 1. The GRS chemistry mechanism solves the photochemistry of NO2 assuming clear-sky conditions. Thus, it does not consider cloud effects on the NO2 photolysis rate, one of its major limitations. R-LINE has been applied to estimate exposure to traffic-related air pollutants in a large-scale study in Detroit, United States (Isakov et al., 2014). However, to our knowledge it has not been applied to European cities, where street canyon morphology dominates. Hence, in order to apply R-LINE over Barcelona its meteorology has been adapted to street canyons as described in Sect. 2.3.1 and the background concentrations are obtained from the CMAQ model considering local meteorology and urban geometry as described in Sect. 2.3.3.
2.3 Coupling CALIOPE with R-LINE
CALIOPE and R-LINE are coupled offline: first CALIOPE is run over Europe, the Iberian Peninsula and Catalonia and then R-LINE is executed for Barcelona city. This approach addresses two main challenges that have already been highlighted in the research literature: (1) downscaling regional meteorology to the street scale to drive pollutant dispersion and (2) obtaining background concentrations from the mesoscale model without double counting traffic emissions in regional- and street-scale models. In addition to these challenges, we consider it relevant to couple meteorology and background concentrations in a consistent way, taking into account atmospheric stability and urban geometry when estimating background contribution within urban streets. Here we describe our methodology when coupling the models to mitigate these challenges.
2.3.1 Meteorology
Most buildings in Barcelona have lower heights than the WRF bottom layer (40.6 m depth). WRF results are assumed to represent over-roof wind and stability conditions because its midpoint height (20.3 m) is similar to the average building height () in a typical neighbourhood of Barcelona (e.g. Eixample district, 20.7 m). WRF is executed consistently with the forecasting air quality system CALIOPE, giving a constant surface roughness (z0) equal to 1 m over the urban area. In order to apply R-LINE over Barcelona, its meteorology has been adapted to street canyons. We have developed a methodology to estimate specific z0 based on urban geometry (e.g. building height, street width). Once z0 is adjusted, the displacement height (dispht), friction velocity (u*), convective velocity scale (w*), PBL height and Monin–Obukhov length (L) are recalculated (Cimorelli et al., 2005). The increase in z0 generally leads to a larger dispht, u*, w* and PBL height. Therefore, L is less stable and atmospheric conditions are more convective. Ultimately, these adjustments have an effect on the way the winds are profiled and on the rate of dispersion of the roadway emissions within the urban area.
The geometrical parameters used for z0 calculation are divided into two categories: (1) averaged over an area of 250 m×250 m (planar building density, bd; average building height, ; and building height standard deviation, bhdev) and (2) specific aspect ratio (ar) for each street segment consisting of street-averaged building height divided by street width. The geometrical parameters are calculated from a Barcelona City Council dataset containing 2-D geometries and number of floors for each building (Barcelona City Council, 2016), assuming 3 m height for each floor.
To estimate specific z0 for each street segment we propose a new morphometric method inspired by previous studies in the literature. z0 is composed by the WRF's background roughness (z0bg) and the one estimated locally (Eq. 1), which incorporates building height influence through the range parameter scaled by two parabolic ratios based on aspect ratio (arr) and building density (bdr). The range parameter (Eq. 2) and z0 increase with following most morphometric methods (e.g. Macdonald et al., 1998). In addition, range and z0 increase with an increasing bhdev. This assumption is based on Kent et al. (2017), who compared nine methods to estimate z0, concluding that methods considering height variability through bhdev (i.e. a higher bhdev brings an increase in z0) provide better results (e.g. Kanda et al., 2013). The parameter C multiplying the equation for range calculation is an empirical constant set to 1∕20 after calibrating the system with the NO2 measurements used in this work for CALIOPE-Urban evaluation. The displacement height is calculated following R-LINE methodology given a factor of displacement height (facdispht) equal to 5 (Eq. 3) as suggested by Snyder and Heist (2013).
To model the influence of building density and aspect ratio, we use the findings of Oke (1988) based on wind tunnel and experimental studies. Oke concluded that over-roof air roughness and satisfactory dispersion within the street canyon are maximum under similar geometrical conditions. Specifically, showing that ar equals 0.65 and bd equals 0.25 gives maximum roughness for overlying air and optimal dispersion conditions in the street canyon.
In practice, z0 increases with an increasing ar to a maximum of ar=0.65 and decreases for ar>0.65 (Eq. 4). Additionally, an increasing bd produces higher z0 until a maximum at bd =0.25 and decreases for higher bd (Eq. 5). We model these ratios using parabolic shapes ranging from 0 to 1. Both urban characteristics are modelled using one parabola to the left of the maximum and another to the right due to the unsymmetrical distribution of the parameter values within Barcelona city (see Fig. A1 in Appendix A). The parabolic ratios will be maximum (i.e. equal to 1) if the roughness effect is maximum. The ratios are prevented from having negative values by setting a minimum of 0.
In addition to the z0 adjustment, we adjust the wind speed and direction to more closely represent the winds blowing down the street as constrained by the buildings, which is called “channelling” (similarly to Fisher et al., 2006). We have adapted R-LINE to incorporate the orientation of roadways (and thus the buildings) where the wind direction follows the street direction. This leads to a recalculation of the wind direction and speed for each roadway before emissions are dispersed within a city. Wind speed channelling is parameterised following Soulhac et al. (2008), who showed that mean velocity along a canyon for any wind direction is directly proportional to the cosine of the angle between street direction and over-roof wind direction (i.e. angle of incidence).
where wsch means channelled wind speed at roof level; the wind speed at roof level (wsbh) is taken from the WRF bottom layer in metres per second and θ is the angle of incidence. The minimum value of the right component is set to avoid an unrealistic zero value for wind speed. Its value of 0.1 is defined in line with Kastner-Klein et al. (2001), who showed that the minimum longitudinal mean flow velocity component at canyon top is equivalent to 0.12 times the above-canyon wind speed for perpendicular over-roof winds according to their wind tunnel experiments. Then, to estimate wind speed at street level a logarithmic profile incorporated within R-LINE that is based on similarity theory (Monin and Obukhov, 1954) is used. In this work, we assume that recirculation flows within street canyons are negligible because R-LINE computes concentrations averaged over an hour, when recirculation and vehicle-induced turbulence are assumed to contribute to a well mixed, more homogeneous air mass driven by variable wind conditions. Additionally, evaluation of the potential impact of including recirculating flows across the canyon is not possible without multiple simultaneous meteorological and pollutant measurements at a fine temporal scale, which are currently not available for Barcelona city.
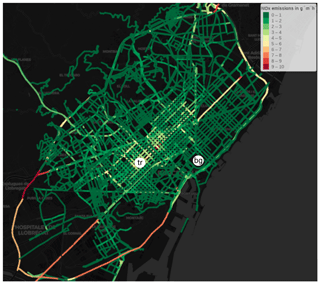
Figure 2NOx emissions in grammes per metre per hour in Barcelona city at 07:00 UTC on 11 April 2013 and location of the two fixed monitoring stations used to estimate the NO2∕NOx ratio. White circles with letters inside represent the stations: tr is Eixample traffic station and bg is Ciutadella Park urban background station.
2.3.2 Emissions
HERMES v2.0 provides hourly NOx and NMVOC road transport emissions at the road link level, which are used by the R-LINE model algorithms to account for NO2 near-road chemistry (Valencia et al., 2018). Road transport emissions (i.e. exhaust, evaporative, wear and resuspension) are estimated combining the tier 3 method described in the EMEP/EEA air pollutant emission inventory guidebook (fully incorporated in version 5.1 of the COPERT IV software) with a digitised traffic network that contains specific information by road stretch for daily average traffic, mean speed circulation, temporal profiles and vehicular park profiles. We note that HERMES v2.0 uses COPERT IV, which does not incorporate revised emission factors of NOx related to the “dieselgate” scandal. Hence, NOx emissions from traffic may be underestimated. Input activity data are obtained by combining different datasets, including traffic data from the Barcelona Automatic Traffic Counting Equipment and vehicle composition profiles derived from a remote-sensing campaign performed in different areas of Barcelona during 2010 (Barcelona City Council, 2010). In Barcelona, higher levels of traffic emissions are found in the city centre and in the highways surrounding the city (Fig. 2). In order to produce emissions in grammes per metre per second for straight street segments as required by R-LINE, we converted the digitised road network curved segments in HERMES to straight segments with no intermediate vertices using the Douglas–Peucker algorithm in the QGIS simplify geometries tool (QGIS Development Team, 2017).
We have estimated the NO2∕NOx ratio following Carslaw and Beevers (2004), which produces an approximation to the NO2 primary contribution. This method relates total Ox (NO2 + O3) to total NOx (NO2 + NO) at a traffic monitoring station subtracting Ox and NOx from a background site in order to remove the effect of background and to only calculate the contribution at the traffic site. As the traffic station we used the Eixample site and as the urban background station we used Ciutadella Park (see Fig. 2), which is located upwind of the dominant wind direction. Figure 3 compares Ox to NOx in Eixample after subtracting the background represented by Ciutadella from the beginning of October to end of February for the years 2012 to 2016. The photochemical season (April–September) is not used to avoid greater scatter than it is found in the winter months as shown by Clapp and Jenkin (2001). The Ox slope value of 18.9 % is considered an estimate of the potential primary NO2 contribution from vehicles at the Eixample traffic station. This value is consistent with studies conducted in other cities with a large diesel vehicle fleet (e.g. Carslaw et al., 2016; Wild et al., 2017) and is assumed to represent the NO2∕NOx ratio in Barcelona in the present work.
2.3.3 Background concentrations
We use the upwind urban background scheme (UBS) to avoid the double counting of traffic emissions when coupling the mesoscale with the street-scale model. The UBS makes a selective choice of CMAQ cells as sketched in Fig. 4 to estimate over roof background concentrations. For each hour, a polygon covering upwind air masses (white) is created. In the figure, the average distance traversed by air masses during an hour (10.8 km) is estimated for WRF's bottom layer wind speed (3 m s−1 in the image). Squares falling within the scheme polygon represent CMAQ cells and their colour refers to cell pollutant values (e.g. NO2 at peak traffic hours may be higher within the city than over the Mediterranean Sea). Grid cell values falling over the scheme polygon are inverse-distance averaged to produce the background estimate of the scheme. Under calm conditions, only the upwind cell is chosen. This method is inspired by Berkowicz (2000), who apply a similar concept based on air mass trajectory to develop a background model.
Background concentrations are required at each receptor in CALIOPE-Urban. Urban dispersion models are typically run at a very high spatial resolution (e.g. 20 m×20 m). Running the UBS every 20 m would have a high computational cost due to its spatial computations, and background concentration values are not expected to vary substantially over tens of metres because CMAQ produces results with 1 km×1 km spatial resolution. Hence, we first run the UBS to produce background concentration values at CMAQ grid cell centroids; then we apply a bilinear interpolation method to provide background at very high spatial resolution.
In addition to the UBS we implement a background decay method to calculate the surface level background concentrations assuming that the UBS provides the concentration at rooftop level. To calculate street level NO2 concentrations, the vertical distribution of pollutants is solved first using the background decay method, applied uniformly to all pollutants, and then the GRS chemical mechanism is solved. The relationship between rooftop and surface level concentrations is assumed to depend on atmospheric stability, localised surface roughness and urban geometry (see Fig. 5 as an illustration of the background decay concept). In the research literature, the influence of atmospheric stability on vertical mixing within a street canyon has been demonstrated using experimental measurements (Rotach, 1995) and wind tunnel experiments (Salizzoni et al., 2009), and it has been implemented in some dispersion models (e.g. Soulhac et al., 2011; Kim et al., 2018). The ratio of wind speeds at surface and rooftop levels (wssfc∕wsbh) estimated by R-LINE using similarity theory (Monin and Obukhov, 1954) is used as a proxy for the vertical mixing. Using this ratio, we calculate facbg that represents the adimensional vertical mixing variable that is multiplied to rooftop background concentration to obtain surface level background concentration at a given height. Wind channelling does not affect the ratio wssfc∕wsbh because we assume that channelling equally affects winds at surface and rooftop levels.
In order to diminish the effect of afternoon underestimations from the regional system near traffic, background levels under convective situations are enhanced. We consider the upward heat flux at the surface (hflux) as representing convective conditions for values higher than 0.30. This value is set to exclude slightly stable night hours with low positive hflux values mainly caused by the urban heat island (i.e. Barcelona city has been found to be 2.9 ∘C warmer than its periphery by Moreno-Garcia, 1994). The following parameterisation is used for cases with bd higher than 0.1,
where , with m being an empirical parameter set to 0.35 after system calibration with NO2 measurements; hflux is upward heat flux at the surface (W m−2). Surface background concentrations for convective situations are maximum for bd equal to 0.25 consistent with the z0 estimation in Sect. 2.3.1. Conversely, we assume that for bd close to zero, surface background concentrations tend linearly to rooftop level background concentrations. The threshold bd=0.1 is based on Grimmond and Oke (1999), who set it as an inferior limit for real cities and show that below this value an isolated flow regime governs. Within this regime, street level and over-roof air is well mixed due to the low building density. Hence, for cases with bd equal to or lower than 0.1, facbg tends linearly to 1 following
Equation (8) shows linear variations between the point at bd=0 and facbg=1 and the point at bd=0.1 with the corresponding facbg value from Eq. (7).
2.4 Execution setup
We have run CALIOPE-Urban for receptors as far as 250 m from roads with sufficient annual average daily traffic (AADT) (i.e. 2000 vehicles per day following Jensen et al., 2017) and receptors further away directly receive CMAQ values interpolated. The 250 m limit is chosen as similar but less restrictive (i.e. to allow longer distances under stable hours) than the one used in Beevers et al. (2012), who used 225 m for London. To smooth out the variation between system outputs, we define a transition area (i.e. 140 to 250 m) where receptors are given concentration values weighted by distance. For temporal and spatial evaluation runs, we locate receptors at the specific coordinates of the measurement sites.
To obtain high-resolution concentration maps for the entire city, we set the spatial context as the minimum rectangle where Barcelona municipality is contained and extended it by 250 m buffers that include the highways surrounding the city. The context is covered by a regular receptor grid of 10 m resolution. R-LINE execution loops over each hour, road and receptor to estimate the contribution from each source to each receptor.
Aiming to understand the impact on accuracy of the local parameterisation for background and meteorology and the impact of using the analytical approach for dispersion, we have run CALIOPE-Urban with different configurations. In Table 2, we describe the different scenarios that have been run. As seen in the table, the CALIOPE-Urban and the CALIOPE-Urban analytical configurations make use of the developed local parameterisations for background and meteorology. In contrast, the CALIOPE-Urban-nl (non-local) configuration does not apply the local parameterisations for background and meteorology. Instead, it uses as background the UBS output without vertical mixing and it omits the use of wind channelling and specific stability parameters for each street segment based on local z0. We show this configuration's results in order to understand if the new implementations in this work contribute substantially to improve the system's ability to simulate NO2 concentrations in Barcelona. R-LINE dispersion algorithm options (i.e. analytical and numerical) are described in Sect. 2.2. For meteorological options, we refer to Sect. 2.3.1. The background method is described in Sect. 2.3.3.
We use three datasets of observations to evaluate the performance of CALIOPE-Urban to reproduce the temporal and spatial variation in NO2 concentrations within Barcelona city. Figure 6 shows the locations of measurements used in this study, which are described below.
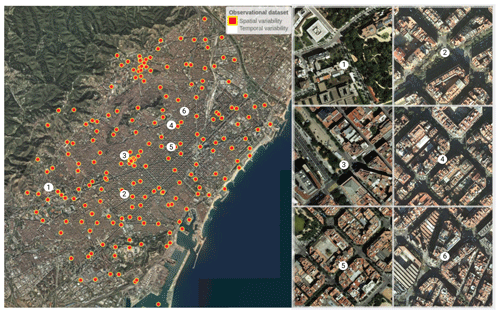
Figure 6Passive dosimeter and monitoring site locations used in the evaluation of CALIOPE-Urban in this work. Red dots with a yellow border represent passive dosimeters (spatial performance) and white numbered dots depict monitoring site emplacements (temporal variability). White dots numbered 1 (Palau Reial), 2 (Eixample) and 3 (Gràcia-Sant Gervasi) are air quality monitoring sites and 4 (213 Industria Street), 5 (445 Valencia Street) and 6 (309 Industria Street) correspond to mobile units. Basemap source: Esri (2019).
3.1 NO2 temporal variability: street canyon campaign and permanent XVPCA network
To evaluate the NO2 temporal variability we use hourly NO2 concentrations reported by the official monitoring network in Catalonia (XVPCA) and from an experimental campaign conducted using mobile units in April and May 2013 in Barcelona (Amato et al., 2014). The official monitoring network has 10 stations in Barcelona and only two of them (i.e. Gràcia-Sant Gervasi and Eixample) are considered representative of near-traffic conditions and provide NO2 hourly levels. Measured data from three sites of the official network are used in this study: Eixample and Gràcia-Sant Gervasi (traffic) and Palau Reial (background). Both traffic sites are located in complex wide areas where several streets intersect (see sites 2 and 3 in Fig. 6 and in the description of Table 3). Palau Reial station (i.e. site 1 in Fig. 6) is located in a medium bd area of the city, 300 m away from a heavily trafficked street. This dataset is complemented with observations from an experimental campaign where mobile units placed at the parking lane of several street segments measured air quality parameters at 3 m height. For this study, we used data gathered every 30 min and aggregated to hourly levels for homogeneity at 213 Industria Street, 309 Industria Street and 445 Valencia Street. These streets present a marked canyon pattern (see sites 4, 5 and 6 in Fig. 6 and description Table 3) where the aspect ratio is approximately 1. In Barcelona, different street geometrical patterns cohabit. For example, the Eixample district, which has the highest number of inhabitants and the greatest population density (33 000 inhabitants km−2), is characterised by a marked street canyon pattern. Most of its canyons are about 20 to 25 m high and 20 m wide (i.e. ar=1 and higher than 1). Experimental campaign sites are considered traffic sites in this work because they are exposed to similar AADT and traffic density (td) compared to official traffic sites as shown in Table 3. We apply Eq. (9) to obtain td over an area of 1 km2.
where td is expressed in vehicles per square kilometre per second, s represents the street segment, n is the number of street segments over the circular area of 1 km2 centred on the measurement site, ls represents the length of the street segment s in metres and tf represents the traffic flow passing a transverse section of the street segment s expressed in vehicles per second. To obtain tf, AADT is divided by 3600⋅24 and multiplied by a temporal factor (i.e. 1.47) representing a typical factor for the morning traffic peak in Barcelona.
Table 3Morphometric and traffic description of measurement sites used in CALIOPE-Urban evaluation. The measurement height of the official network sites and the mobile sites is 3 m. AADT from the nearest street is considered. The traffic density (td) is estimated following Eq. (9). The Palau Reial td is not included because it is an urban background site not directly exposed to high traffic.
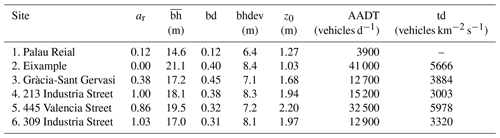
3.2 NO2 spatial variability: passive dosimeter campaign across the city
With the objective of representing the NO2 spatial variability, 212 passive dosimeters were located in Barcelona from 28 February to 15 March 2017 as depicted by red dots with a yellow border in Fig. 6. In every square kilometre of surface there were at least two dosimeters, representing the background and traffic conditions at 2.2–2.5 m height. The 100 background dosimeters were placed more than 10 m away from the road and the 112 traffic dosimeters were located less than 3 m away from the road and at least 25 m away from intersections. To ensure the equivalence of measurements to standard conditions, these were corrected through comparison with reference equipment from several sites of the XVPQA network. After a preliminary inspection of the location of the dosimeters, we discarded data from 30 dosimeters to avoid results that could not be interpreted for several reasons (e.g. dosimeter and simulated road at different heights, highway covered by a tunnel near dosimeter location that is not considered in the emission inventory, lack of emission sources near dosimeter).
Section 4.1 presents the temporal variability of NO2 concentrations estimated by CALIOPE and CALIOPE-Urban compared to observations at the six sites described in Sect. 3.1. Section 4.2 describes the results in terms of the spatial variation during the 2-week passive dosimeter campaign described in Sect. 3.2. Model performance is quantified using performance measures as described by Chang and Hanna (2004) and using assessment target plots (defined in the FAIRMODE initiative; Janssen et al., 2017). The performance statistics used here are the geometric mean bias (GeoMean), the fraction of model results within a factor of 2 of observations (FAC2), the geometric standard deviation (GeoSD), the correlation coefficient (R), the mean bias (MB) and the root-mean-square error (RMSE). The mathematical expressions of these statistics can be found in the Appendix C.
4.1 Temporal variation in NO2 concentrations within urban streets
The scatter plots of Fig. 7 compare CALIOPE and CALIOPE-Urban outputs with observations based on hourly, daily mean and maximum modelled concentrations at the six sites described in Sect. 3.1 for April and May 2013. In general, CALIOPE-Urban shows a greater agreement for hourly, daily means and maximum concentrations but tends to underpredict daily peak concentrations at sites not exposed to very high traffic intensity (i.e. sites where urban background contribution predominates like Gràcia-Sant Gervasi). During the study period most daily maxima (i.e. 56 %) occur at morning or evening traffic peak times (i.e. 06:00–07:00 or 18:00–20:00 UTC) when atmospheric conditions are typically stable and traffic intensity is high.
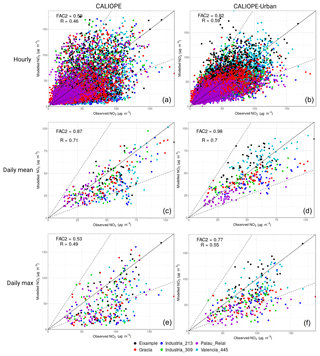
Figure 7Scatter plot of hourly (a, b), daily mean (c, d) and daily maximum (e, f) modelled concentrations against observed concentrations with colours representing monitoring sites for CALIOPE (a, c, e) and CALIOPE-Urban (b, d, f). Purple represents Palau Reial, the urban background site. The other colours represent traffic sites as described in Sect. 3.1.
Table 4 shows the model performance statistics computed with hourly data, including the CALIOPE-Urban-nl run. We compare CALIOPE-Urban and CALIOPE-Urban-nl to assess the difference in performance derived by the use of the local developments described in Sect. 2.3. All systems perform well at urban background sites and only CALIOPE-Urban gives good agreement with observations in traffic sites. The greatest difference between CALIOPE and CALIOPE-Urban system performance is produced at the 445 Valencia Street site due to its street canyon morphology (ar=0.86). At this site, the mean transport is well resolved by the channelled winds, and its high AADT produces a high increase in traffic emissions within R-LINE. CALIOPE-Urban-nl largely overestimates NO2 concentrations at this site for several reasons: it directly uses the output of UBS for background, instead of applying the vertical mixing that reduces background at street level especially under stable conditions; z0 is given the WRF value (z0=1.0), which is much lower than its locally estimated value (i.e. z0=2.2; see Table 3), that enhances dispersion decreasing concentration levels; lastly pollutant dispersion is not channelled within the street, so higher contributions of nearby streets may be expected.
Table 4NO2 model evaluation statistics calculated at six sites (described in Sect. 3) for hourly concentrations during April and May 2013 for CALIOPE, CALIOPE-Urban and CALIOPE-Urban without local developments (CALIOPE-Urban-nl). Bold numbers represent model results with better performance for each statistic and site.
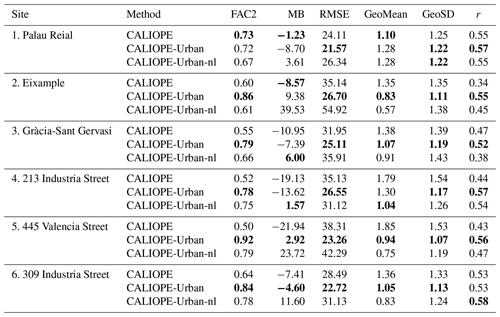
Conversely, CALIOPE-Urban underestimations at 213 and 309 Industria Street and Gràcia-Sant Gervasi may be due to an unrealistically low AADT level on the street segment close to the site. We work with AADT data that are based on the outputs of the traffic model used by Barcelona City Council that may be underestimating traffic. Another explanation may be an underestimation of local background levels within the area mostly during the afternoon. The afternoon underestimations in the mesoscale system could be caused by an overestimation of the mixing that produces a too low background NO2 concentration level. This issue is difficult to correct because background concentrations used in the system are dependent on mesoscale concentrations, which are underestimated during daytime. In Table B1 in Appendix B, same statistics are computed for daily mean results, finding results similar to in the hourly analysis. In addition, the analytical version of CALIOPE-Urban is shown to produce results for hourly concentrations similar to the numerical version in Table B2 in Appendix B. This result may be interesting for forecasting applications at the urban scale that require high resolution because the analytical dispersion algorithm spends approximately half the time computing in comparison to the numerical dispersion algorithm as shown in Table 2.
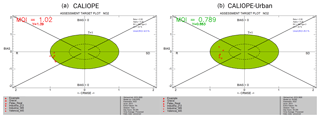
Figure 8NO2 model assessment target plots for CALIOPE (left) and CALIOPE-Urban (right). Symbols correspond to the six measurement sites described in Sect. 3.1 and the MQI for each site is represented by the distance between the circle origin and the site symbol.
Figure 8 shows NO2 assessment target plots for CALIOPE and CALIOPE-Urban. In the plots the centred root-mean-square error (CRMSE) for each measurement station is plotted against the normalised bias. Distance from circle origin gives an estimate for the model quality indicator (MQI; Thunis and Cuvelier, 2016) that measures general model accuracy depending on measurement uncertainty. MQI values below 1 (i.e. green shading area) are considered to comply with the model quality objective. All sites in the CALIOPE-Urban simulation fall within the green shaded area (i.e. complying with FAIRMODE's model quality objective). In contrast, four out of six in CALIOPE lie within the green shaded area, clearly showing the positive effect of the street-scale model in the coupled system.
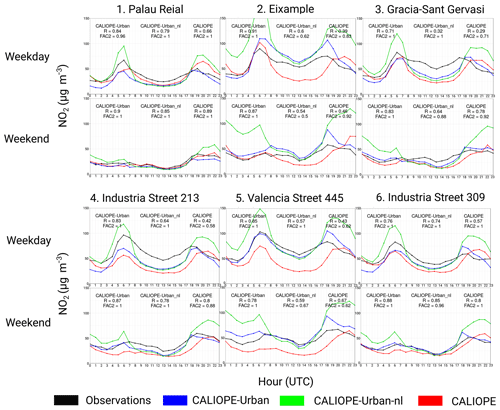
Figure 9NO2 average daily cycle at all sites described in Sect. 3.1 during April and May 2013 for weekday and weekend. Observations are represented as black coloured lines, red lines are CALIOPE, blue lines are CALIOPE-Urban and green lines represent CALIOPE-Urban without local developments (CALIOPE-Urban-nl).
Figure 9 shows averaged daily cycles for weekday and weekend periods for the six sites described in Sect. 3.1 for CALIOPE, CALIOPE-Urban and CALIOPE-Urban-nl. In general, all systems show a significant change between weekday and weekend in accordance with observations. The overall dynamic is well reproduced by all systems but CALIOPE tends to underestimate the afternoon levels and overestimate night-time values. CALIOPE-Urban-nl overestimates night-time values and morning peaks. CALIOPE-Urban partly corrects CALIOPE afternoon underestimations close to high traffic (i.e. Valencia Street and Eixample stations) but still underestimates at low traffic sites. CALIOPE's tendency to overestimate the evening peak and night values may bring CALIOPE-Urban to generally overestimate for those hours as found in the literature near road sites (Hood et al., 2018). However, the vertical mixing implemented in CALIOPE-Urban decreases background concentration mixing from aloft during night hours because under stable atmospheric conditions vertical mixing is reduced compared to daylight hours, which are more convective. This effect can be noticed in the difference between CALIOPE-Urban and CALIOPE-Urban-nl from 00:00 to 06:00 and from 18:00 to 23:00 (UTC) at traffic sites (i.e. sites 2, 3, 4, 5 and 6 in Fig. 9), where CALIOPE-Urban concentration levels correct the night overestimations seen in CALIOPE-Urban-nl. Such a result shows the benefit of considering the vertical stability in the coupling procedure of the mesoscale and the street-scale dispersion model.
There is a noticeable difference between CALIOPE-Urban's accuracy at 213 Industria Street and 445 Valencia Street given similar observations and CALIOPE levels at both sites. Although both sites are located in areas with considerable traffic activity, Valencia Street site has higher modelled traffic emissions, resulting in higher local pollutant concentrations, and a higher density of vehicles per square kilometre as described in Table 3. Consequently, to improve CALIOPE-Urban accuracy, an increase in local simulated traffic at 213 Industria Street site could bring a model accuracy improvement. However, the lack of observational traffic count data at the monitoring sites does not permit us to explore the precision of the input AADT information considered in HERMES v2.0 at those locations.
Table 5NO2 model evaluation statistics calculated at 182 passive dosimeter valid sites (described in Sect. 3.2) during 2 weeks from 28 February to 15 March 2017 for CALIOPE, CALIOPE-Urban and CALIOPE-Urban-nl mean concentrations. Bold numbers represent model results with better performance for each statistic and site. Results are shown for all (including traffic and urban background), only urban background and only traffic sites.
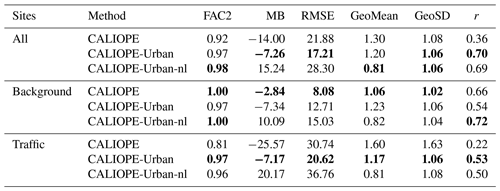
4.2 Spatial variation in NO2 concentrations across the city
We evaluate CALIOPE and CALIOPE-Urban NO2 in terms of spatial variations across Barcelona city using measurements from 182 valid passive dosimeters as described in Sect. 3.2. Table 5 gives statistics at the 182 sites where passive dosimeters measured NO2 concentrations for a 2-week period (28 February–15 March 2017) for CALIOPE, CALIOPE-Urban and CALIOPE-Urban-nl (without local developments).
Considering all sites, CALIOPE-Urban shows a much better correlation coefficient (0.70 vs. 0.36) than CALIOPE due to its good performance at traffic sites. Compared to CALIOPE-Urban-nl their correlation is similar. If we consider only urban background sites, CALIOPE shows a greater correlation coefficient than CALIOPE-Urban (0.66 vs. 0.54) and a MB closer to 0. In addition, CALIOPE-Urban-nl gives a better correlation than both systems. A potential explanation for this result is related to the error compensation shown in the temporal evaluation (Sect. 4.1). CALIOPE and CALIOPE-Urban-nl may compensate for the underestimation during daytime with the overestimation during night-time. In contrast, CALIOPE-Urban may not compensate for the daytime underestimations with overestimated night values because the background is reduced due to low vertical mixing effect during night-time (stable) hours. An enhanced daytime NO2 background contribution would improve CALIOPE-Urban accuracy at urban background sites.
For traffic sites, CALIOPE shows a strong underestimation (MB µg m−3) and CALIOPE-Urban gives MB levels closer to 0. CALIOPE-Urban underestimations may be influenced by afternoon underestimations and a misrepresentation of traffic emissions in some areas of the city. In contrast, CALIOPE-Urban-nl gives a high MB and the highest RMSE among the three systems. This tendency to overestimate near traffic of CALIOPE-Urban-nl may be due to the reasons stated in Sect. 4.1. In general, closer to intense traffic CALIOPE-Urban is very sensitive to emissions and its dispersion characterises the spatial variability for the study period well. Reproducing spatial gradients near intense traffic is crucial in a city like Barcelona given its high vehicle density and NO2 concentration levels.
Figure 10 shows the difference between CALIOPE and CALIOPE-Urban results and measurements (panels a, b) and scatter plots at all sites (panels c, d) distinguished with colours by site type (e.g. traffic site, urban background site).
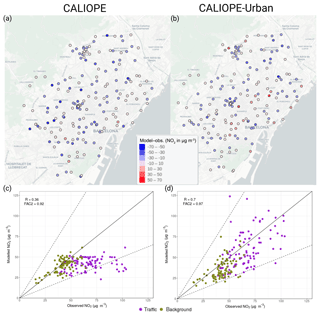
Figure 10(a, b) NO2 concentration difference (model – observations) for 2-week averaged values during the dosimeter campaign in 2017; CALIOPE is left (a) and CALIOPE-Urban is right (b). (c, d) Scatter plot of modelled vs. observed concentrations with colours representing site type (olive green for background and purple for traffic) for CALIOPE (c) and CALIOPE-Urban (d). Correlation (R) and agreement factor of 2 (FAC2) are computed for all sites.
In Fig. 10a the concentration difference map of CALIOPE shows an overall underestimation, represented by blue dots. This underestimation is found to be systematic at traffic sites in the scatter of Fig. 10c (purple dots), where modelled values barely exceed 50 µg m−3 while most of the observed values at traffic sites are above that value. In contrast, the CALIOPE-Urban difference map (Fig. 10b) shows a more mixed picture with a broader representation of white dots (bias close to 0) but also more red ones in the city centre and close to the highways. For CALIOPE-Urban's scatter, most of the model results at traffic sites are within the 1:2 and 1:0.5 dashed lines, showing a better agreement at traffic sites than CALIOPE (Fig. 10d). In CALIOPE-Urban's difference map, we see a spatial pattern with average bias close to 0 in the city centre, where traffic is denser and close to the highways surrounding the city. The appearance of red dots may indicate that CALIOPE-Urban overestimates close to high trafficked areas while CALIOPE underestimates in these areas. This may be due to an overestimation of traffic emissions or background concentrations in these areas. In contrast, in locations where traffic is not very intense (see Fig. 2 for NOx emissions) CALIOPE-Urban shows systematic underestimations. This result may be derived from the systematic underestimation of midday NO2 concentrations in low-traffic areas as shown in Sect. 4.1.
4.3 Major uncertainty sources
Here we discuss potential sources of error in our model by analysing episodes when the model was skilful compared with episodes when the model was not. Our analysis solely considers the meteorological and background concentration inputs as potential sources of error. While road traffic emission estimates may introduce large errors, we lack observations of traffic counts at the measurement site locations to properly assess them.
We calculated daily the RMSE of the hourly modelled NO2 concentrations versus the observed values at the six sites described in Sect. 3.1 during the period April and May 2013. For each site we picked the 10 d with the highest RMSE and 10 d with the lowest RMSE as potential candidates. Then, we put together the candidates of all sites and we chose the most frequent 5 d (i.e. from good and bad performance candidate days) for both CALIOPE and CALIOPE-Urban, finding that both systems share to a large extent the days with skill (four out of five days) and without (three out of five). This result shows that the coupled system performance is highly dependent on the mesoscale model performance. To explore errors potentially caused by R-LINE inputs, in Fig. 11 we compare the five days with less skill (i.e. 11, 16, 17 April and 7, 8 May) and the five days with more skill (i.e. 7, 20 April and 18, 19, 25 May) with observations for wind speed (ws), street level NO2 and background NO2.
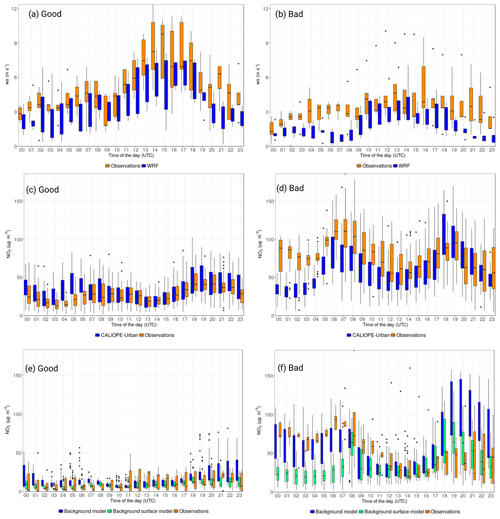
Figure 11Box plots by time of the day of good (a, c, e) and bad performance days (b, d, f) for CALIOPE-Urban inputs and observations with dots representing outliers. Panels (a, b) represent WRF and observed wind speeds at Barcelona airport (10 m height); panels (c, d) show observed and modelled NO2 concentrations for the six sites in Sect. 3.1; panels (e, f) depict NO2 observed concentrations at the Ciutadella urban background station and background model averaged results at the six sites. Observed values are orange and modelled results are blue. Light green represents background model results at the surface level.
On skilful days, winds are relatively strong and well represented in WRF (Fig. 11a). Poor skills appear when the observed wind speed is low. Because WRF largely underestimates wind speeds (Fig. 11b) and NO2 concentrations are underestimated under calm conditions (Fig. 11d), other processes (e.g. atmospheric stability) may have a greater importance in this case. In our coupling under very stable atmospheric situations, dispersion is reduced and background injection from the overlying atmosphere is limited. This control mechanism adapts the system to specific street conditions, regulating dispersion and background injection. For these days, an extended observational dataset would be needed to better understand the model behaviour.
To analyse the background concentrations from the mesoscale simulation as a potential error source, we compared NO2 observations from the Ciutadella urban background station with hourly modelled concentrations averaged over the six sites. We aimed to compare the modelled background concentrations (i.e. excluding local vehicular traffic contribution) with the most representative urban background observation, which in our case is the Ciutadella site. The results shown in Fig. 11e, f represent concentrations provided by upwind CMAQ grid cells depending on wind speed and direction (blue) as described in Sect. 2.3.3 downscaled to surface level using the vertical decay method (green). As expected, observed NO2 concentrations on days with calm conditions and therefore poor skill are higher than on those with enhanced ventilation and better skills. The background model reproduces the variation during both types of days well but overestimates concentrations during night-time (19:00–22:00 UTC), particularly during days with calm conditions. This problem is partially corrected by using the background vertical decay method as seen in Fig. 11f and in Fig. 9. In addition, NO2 concentrations are underestimated at the beginning of the day (01:00–04:00 UTC). The fact that the averaged diurnal cycle in Fig. 9 shows similar error patterns suggests that NO2 background concentrations greatly influence NO2 street level concentrations in agreement with Degraeuwe et al. (2017).
4.4 Hourly variation in street NO2 concentrations
Hourly street NO2 concentrations are expected to vary spatially and temporally with higher values close to intense traffic sites during rush hour. Figure 12 shows high-resolution (10 m×10 m) NO2 concentration maps at four different hours on Thursday 11 April 2013 (i.e. 00:00, 07:00, 12:00 and 18:00 UTC). This day is chosen because it shows a marked diurnal cycle with maxima consistent with the morning and evening traffic peaks (i.e. 06:00–07:00 or 18:00–20:00 UTC). Higher concentrations are found at 07:00 and 18:00 UTC where high traffic emissions are concentrated (i.e. highways surrounding the city and city centre) because traffic intensity is higher at these hours of the day and the atmosphere tends to be stable, making pollutant dispersion more difficult. Conversely, lower concentrations are found at 00:00 UTC due to the lower traffic intensity and at 12:00 UTC. At 12:00 UTC traffic intensity is considerably higher than at 00:00 UTC but the atmosphere is more convective and pollutant dispersion is enhanced.
In agreement with Duyzer et al. (2015) our modelling results show that the Eixample and Gràcia-Sant Gervasi traffic stations do not represent the highest NO2 concentrations in Barcelona. The highest levels are found in street canyons exposed to very high traffic intensity and not as well ventilated as the above-mentioned locations, and in open areas near highways surrounding the city. For example, measurements at the 445 Valencia Street site show 20 % higher concentrations than at Eixample and Gràcia-Sant Gervasi traffic sites on average during the morning peak on weekdays (see Fig. 9). Hence, additional monitoring sites located within highly trafficked streets are clearly needed to better represent the highest NO2 concentration levels in Barcelona.
This study describes the development of a coupled regional- to street-scale modelling system, CALIOPE-Urban, which provides high-spatial- and high-temporal-resolution (up to 10 m × 10 m, hourly) NO2 concentrations for Barcelona. It couples the mesoscale air quality forecasting system CALIOPE (WRF-HERMES-CMAQ-BSC-DREAM8b) with the urban roadway dispersion model, R-LINE. For each regional 1 km × 1 km grid cell, meteorological data from WRF and background concentrations from CMAQ are used as input combined with traffic emissions from the HERMES emission model at road link level. R-LINE has been adapted to Barcelona’s geometrical conditions by considering specific meteorology and background concentrations for each street. CALIOPE-Urban NO2 simulations are compared with CALIOPE and with observations for temporal evaluation, using data from five traffic sites and one urban background site during April and May 2013, and for spatial evaluation, with NO2 concentrations measured by 182 passive dosimeters distributed across the entire city during 2 weeks in February-March 2017.
CALIOPE-Urban methodology adapts dynamically to street conditions by coupling the meteorology and background using street-specific surface roughness based on urban geometry. It adapts the R-LINE dispersion model to compact cities using channelled winds to drive dispersion and using recalculated meteorological parameters for each street. Regarding background concentrations, it estimates over-roof levels using an upwind background scheme and gives surface concentrations by applying a vertical mixing parameterisation based on urban geometry and atmospheric stability. The upwind background scheme avoids double counting traffic emissions in regional and dispersion models by using upwind grid cell concentrations to estimate over-roof background concentrations. Doing so we omit the use of the grid cell over the estimated area, where traffic emissions are considered in the dispersion model. To estimate background concentrations at surface level, the vertical mixing parameterisation enhances background mixing from the overlying atmosphere under daytime convective atmospheric conditions and limits background air mixing during night-time (stable) hours. For the transition from urban to suburban areas, CALIOPE-Urban implements a smooth variation for wind conditions, background and total concentrations.
Temporally, CALIOPE-Urban agrees better with observations than CALIOPE at the five traffic sites evaluated, where the contribution of local emissions predominates. For the urban background site of Palau Reial, both systems give similar (good) results. For traffic sites, the coupled system shows better agreement in highly trafficked areas where local dispersion plays a crucial role. Regarding the diurnal average cycle at the observation sites, both systems follow the overall daily cycle in the observations but CALIOPE-Urban predicts better morning peaks, and corrects the afternoon levels at traffic sites as well as the systematic night-time overestimation produced by the regional system. The vertical mixing of rooftop background concentrations to surface levels based on atmospheric stability and urban geometry appears to be a good method to correct the strong positive bias of the mesoscale model under stable atmospheric conditions during the evening.
Spatially, CALIOPE-Urban performs better than CALIOPE at the dosimeters located close to traffic. This result is because R-LINE explicitly resolves road traffic emission dispersion simulating the high gradients of NO2 observed levels that occur within a mesoscale system grid cell. CALIOPE-Urban gives more overestimation close to highly trafficked areas. This behaviour may be produced by an overestimation of traffic emissions in these roads or by underestimating dispersion. For dosimeters located more than 10 m away from traffic both systems perform reasonably well. The higher the traffic in the surrounding area, the better CALIOPE-Urban performance is compared to the regional system.
When exploring the main error sources, overall both systems produce results that are either accurate or inaccurate on the same days. This fact suggests that coupled system results are highly influenced by the regional system results. Furthermore, we find that CALIOPE-Urban gives higher errors (i.e. stronger underestimations) under stable conditions with light winds and low PBL height than under more convective conditions, with stronger winds and higher PBL heights. Another potential source of uncertainty is the integration within HERMES v2.0 of COPERT IV instead of COPERT V, which considers diesel NOx exceedances derived from the dieselgate scandal for EURO 5 and EURO 6 diesel cars (Brown et al., 2018). In future work, we plan to update HERMES v2.0 with the new emission factors released by COPERT V and examine the influence of traffic emissions in CALIOPE-Urban results. Finally, we consider an additional source of uncertainty, the assumption of clear-sky conditions in the photolysis rate calculation of the GRS chemistry mechanism.
For high-resolution air quality forecasts, we show that CALIOPE-Urban using either the numerical or the analytical dispersion algorithm gives good results. However, an entire city system execution using the analytical configuration takes approximately half the time compared to the numerical one. Hence, the analytical dispersion algorithm may be a suitable option for forecasting applications when sources, such as roadways, and receptors are located near the ground.
We show that traffic monitoring stations in Barcelona do not represent the highest NO2 concentrations in the city. We find the highest levels in heavily trafficked street canyons that are not well ventilated and near highways in the city surroundings. As a consequence, we consider that additional monitoring sites located in these areas may better characterise the range of NO2 concentration levels in Barcelona and give a better representation of human exposures.
This study has demonstrated that CALIOPE-Urban improves the accuracy of model outputs estimating NO2 concentrations in Barcelona compared to CALIOPE. The methodology is replicable in cities where a mesoscale chemistry transport model provides NO2 simulations if urban geometrical data are available. The next step is to implement CALIOPE-Urban in the operational forecasting system for Barcelona to provide NO2 concentrations at street level, and explore emission impacts due to improved NOx emission estimates.
Copies of the code are readily available upon request from the corresponding authors. Observational data in this work have been provided by co-authors from the Institute of Environmental Assessment and Water Research, IDAEA-CSIC, Spain. Contact them if interested in these datasets.
Table B1NO2 model evaluation statistics calculated at six sites (described in Sect. 3) during April and May 2013 for CALIOPE and CALIOPE-Urban daily mean concentrations. Bold numbers represent model results with better performance for each statistic and site.
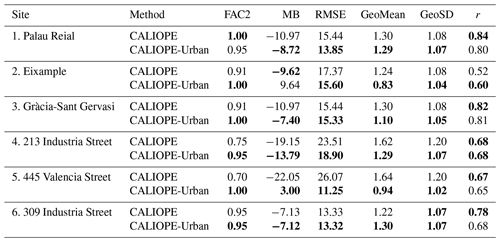
Table B2NO2 model evaluation statistics calculated at six sites (described in Sect. 3) during April and May 2013 for hourly concentrations of CALIOPE-Urban, CALIOPE-Urban analytical or CALIOPE-Urban-nl (non-local) configurations. Bold numbers represent model results with better performance for each statistic and site.
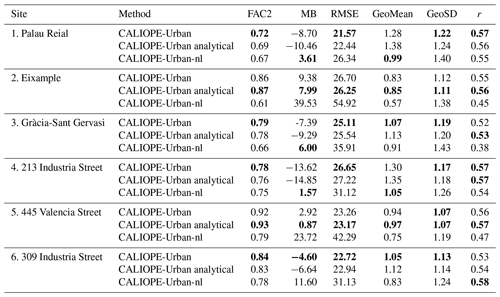
Here we define the model evaluation statistics used to compare observed measurements (obs) with modelled concentrations (mod): the geometric mean bias (GeoMean), the fraction of model results within a factor of 2 of observations (FAC2), the geometric standard deviation (GeoSD), the correlation coefficient (R), the mean bias (MB) and the root-mean-square error (RMSE).
Here mod is the modelled concentrations, obs is the observed concentrations, the overbar () represents the average over a dataset d, F is considered to be 2, eri is the inverse of error function, AF is the proportion of the ratio, σd is the standard deviation of d, n is the number of paired modelled and observed concentrations and subscripts represent a value between 1 and n. For further details on the evaluation statistics we refer to Chang and Hanna (2004).
JB developed the code. JB, MS and OJ designed the research. FA and XQ provided the observational data. CPGP, MG, FA, XQ and AS contributed to the discussion of the results. JB wrote the original paper, and all authors contributed to the review and editing of the paper.
The authors declare that they have no conflict of interest.
This work was started while Michelle Snyder was a researcher at the University of North Carolina Institute for the Environment. Michelle Snyder's efforts throughout the project were completely voluntary.
Jaime Benavides' PhD work is funded by grant BES-2014-070637 from the FPI programme by the Spanish Ministry of the Economy and Competitiveness. Jaime Benavides developed part of this work as a research visitor at the Institute for the Environment at UNC funded by mobility grant EEBB-I-17-12296 from the same ministry. IDAEA-CSIC acknowledges the Barcelona City Council for their support to the experimental campaign. Carlos Pérez García-Pando acknowledges the long-term support from the AXA Chair in Sand and Dust Storms (AXA Research Fund), as well as the support received through the Ramón y Cajal programme (grant RYC-2015-18690) of the Spanish Ministry of Economy and Competitiveness.
This research has been supported by the Spanish Ministry of the Economy and Competitiveness (grant nos. CGL2013-46736-R, CGL2016-75725-R and RTI2018-099894-BI00), as well as the Catalan Government (grant no. RIS3CAT-COM15-1-0011-04).
This paper was edited by Jason Williams and reviewed by two anonymous referees.
Amato, F., Karanasiou, A., Cordoba, P., Alastuey, A., Moreno, T., Lucarelli, F., Nava, S., Calzolai, G., and Querol, X.: Effects of Road Dust Suppressants on PM Levels in a Mediterrazean Urban Area, Environ. Sci. Technol., 48, 8069–8077, https://doi.org/10.1021/es502496s, 2014. a
Arunachalam, S., Valencia, A., Akita, Y., Serre, M., Omary, M., Garcia, V., and Isakov, V.: A Method for Estimating Urban Background Concentrations in Support of Hybrid Air Pollution Modeling for Environmental Health Studies, Int. J. Environ. Res. Pu., 11, 10518–10536, https://doi.org/10.3390/ijerph111010518, 2014. a
ASPB: Avaluació de la qualitat de l'aire a la ciutat de Barcelona 2016, Agència de Salut Pública de Barcelona, 1–54, 2017. a
Baldasano, J., Pay, M., Jorba, O., Gassó, S., and Jiménez-Guerrero, P.: An annual assessment of air quality with the CALIOPE modeling system over Spain, Sci. Total Environ., 409, 2163–2178, https://doi.org/10.1016/j.scitotenv.2011.01.041, 2011. a, b
Baldasano, J., Soret, A., Guevara, M., Martínez, F., and Gassó, S.: Integrated assessment of air pollution using observations and modelling in Santa Cruz de Tenerife (Canary Islands), Sci. Total Environ., 473-474, 576–588, https://doi.org/10.1016/j.scitotenv.2013.12.062, 2014. a
Barcelona City Council: Evaluation of the NOx and PM10 emission reductions from traffic in Barcelona city based on the characterization of the vehicle pool, PECQ 2011–2020, 1–109, available at: https://w110.bcn.cat/MediAmbient/Continguts/Documents/Documentacio/4-AvaluacioEmissionsParcMobilBCN.pdf (last access: 21 June 2019), 2010. a
Barcelona City Council: CartoBCN v.1.1.0, available at: http://w20.bcn.cat/cartobcn/ (last access: 21 June 2019), 2016. a
Barcelona City Council: Caracterització dels vehicles i les seves emissions a l’àrea metropolitana de Barcelona, Tech. rep., 1–670, 2017. a
Barone-Adesi, F., Dent, J. E., Dajnak, D., Beevers, S., Anderson, H., Kelly, F. J., Cook, D. G., and Whincup, P. H.: Long-Term Exposure to Primary Traffic Pollutants and Lung Function in Children: Cross-Sectional Study and Meta-Analysis, PloS one, 10, e0142565, https://doi.org/10.1371/journal.pone.0142565, 2015. a
Basart, S., Pérez, C., Nickovic, S., Cuevas, E., and Baldasano, J.: Development and evaluation of the BSC-DREAM8b dust regional model over northern Africa, the mediterranean and the middle east, Tellus B, 64, 1–23, https://doi.org/10.3402/tellusb.v64i0.18539, 2012. a
Bechtel, B., Alexander, P., Böhner, J., Ching, J., Conrad, O., Feddema, J., Mills, G., See, L., and Stewart, I.: Mapping Local Climate Zones for a Worldwide Database of the Form and Function of Cities, ISPRS Int. J. Geo-Inf., 4, 199–219, https://doi.org/10.3390/ijgi4010199, 2015. a
Beevers, S., Kitwiroon, N., Williams, M., and Carslaw, D.: One way coupling of CMAQ and a road source dispersion model for fine scale air pollution predictions, Atmos. Environ., 59, 47–58, https://doi.org/10.1016/j.atmosenv.2012.05.034, 2012. a, b
Berkowicz, R.: A simple model for urban background pollution, Enviro. Monit. Assess., 65, 259–267, https://doi.org/10.1023/A:1006466025186, 2000. a
Borge, R., Lumbreras, J., Pérez, J., De la Paz, D., Vedrenne, M., de Andrés, J. M., and Rodríguez, M. E.: Emission inventories and modeling requirements for the development of air quality plans. Application to Madrid (Spain), Sci. Total Environment, 466-467, 809–819, https://doi.org/10.1016/j.scitotenv.2013.07.093, 2014. a
Brousse, O., Martilli, A., Foley, M., Mills, G., and Bechtel, B.: WUDAPT, an efficient land use producing data tool for mesoscale models? Integration of urban LCZ in WRF over Madrid, Urban Climate, 17, 116–134, https://doi.org/10.1016/j.uclim.2016.04.001, 2016. a
Brown, P., Wakeling, D., Pang, Y., and Murrells, T.: Methodology for the UK's Road Transport Emissions Inventory, United Kingdom Government, Department for Business, Energy & Industrial Strategy, Tech. Rep. ED59803130, 1–50, 2018. a
Byun, D. and Schere, K.: Review of the governing equations, computational algorithms, and other components of the Models-3 Community Multiscale Air Quality (CMAQ) modeling system, Appl. Mech. Rev., 59, 51–77, https://doi.org/10.1115/1.2128636, 2006. a
Carslaw, D. and Beevers, S.: Investigating the potential importance of primary NO2 emissions in a street canyon, Atmos. Environ., 38, 3585–3594, https://doi.org/10.1016/j.atmosenv.2004.03.041, 2004. a
Carslaw, D., Murrells, T. P., and Keenan, M.: Have vehicle emissions of primary NO2 peaked ?, Faraday Discuss., 189, 439–454, https://doi.org/10.1039/c5fd00162e, 2016. a
Chang, J. and Hanna, S.: Air quality model performance evaluation, Meteorol. Atmos. Phys., 87, 167–196, https://doi.org/10.1007/s00703-003-0070-7, 2004. a, b
Cimorelli, A., Perry, S., Venkatram, A., Weil, J., Paine, R., Wilson, R., Lee, R., Peters, W., and Brode, R.: AERMOD : A Dispersion Model for Industrial Source Applications. Part I: General Model Formulation and Boundary Layer Characterization, J. Appl. Meteorol., 44, 682–693, https://doi.org/10.1175/JAM2227.1, 2005. a
Clapp, L. and Jenkin, M.: Analysis of the relationship between ambient levels of O3, NO2 and NO as a function of NOx in the UK, Atmos. Environ., 35, 6391–6405, https://doi.org/10.1016/S1352-2310(01)00378-8, 2001. a
Degraeuwe, B., Thunis, P., Clappier, A., Weiss, M., Lefebvre, W., Janssen, S., and Vranckx, S.: Impact of passenger car NOx emissions on urban NO2 pollution – Scenario analysis for 8 European cities, Atmos. Environ., 171, 21330–21337, https://doi.org/10.1016/j.atmosenv.2017.10.040, 2017. a
Duyzer, J., van den Hout, D., Zandveld, P., and van Ratingen, S.: Representativeness of air quality monitoring networks, Atmos. Environ., 104, 88–101, https://doi.org/10.1016/j.atmosenv.2014.12.067, 2015. a, b
EEA: Air quality in Europe – 2018 report, EEA report No. 12/2018, 1–88, https://doi.org/10.2800/777411, 2018. a
Esri: World Imagery [basemap], World Imagery Map 2019, available at: http://www.arcgis.com/home/item.html?id=10df2279f9684e4a9f6a7f08febac2a9, last access: 12 February 2019.
Fagerli, H., Denby, B., and Wind, P.: Assessment of LRT contribution to cities in Europe using uEMEP?, available at: https://www.unece.org/fileadmin/DAM/env/documents/2017/AIR/EMEP/uEMEP_EMEPSB2017_HF.pptx, last access: 21 June 2019. a
Ferreira, J., Guevara, M., Baldasano, J. M., Tchepel, O., Schaap, M., Miranda, A. I., and Borrego, C.: A comparative analysis of two highly spatially resolved European atmospheric emission inventories, Atmos. Environ., 75, 43–57, https://doi.org/10.1016/j.atmosenv.2013.03.052, 2013. a
Fisher, B., Kukkonen, J., Piringer, M., Rotach, M. W., and Schatzmann, M.: Meteorology applied to urban air pollution problems: concepts from COST 715, Atmos. Chem. Phys., 6, 555–564, https://doi.org/10.5194/acp-6-555-2006, 2006. a
Grimmond, C. and Oke, T.: Aerodynamic Properties of Urban Areas Derived from Analysis of Surface Form, J. Appl. Meteorol., 38, 1262–1292, https://doi.org/10.1175/1520-0450(1999)038<1262:APOUAD>2.0.CO;2, 1999. a
Guevara, M., Martínez, F., Arévalo, G., Gassó, S., and Baldasano, J.: An improved system for modelling Spanish emissions: HERMESv2.0, Atmos. Environ., 81, 209–221, https://doi.org/10.1016/j.atmosenv.2013.08.053, 2013. a
Guevara, M., Lopez-Aparicio, S., Cuvelier, C., Tarrason, L., Clappier, A., and Thunis, P.: A benchmarking tool to screen and compare bottom-up and top-down atmospheric emission inventories, Air Qual. Atmos. Hlth., 10, 627–642, https://doi.org/10.1007/s11869-016-0456-6, 2017. a
Hood, C., MacKenzie, I., Stocker, J., Johnson, K., Carruthers, D., Vieno, M., and Doherty, R.: Air quality simulations for London using a coupled regional-to-local modelling system, Atmos. Chem. Phys., 18, 11221–11245, https://doi.org/10.5194/acp-18-11221-2018, 2018. a, b, c, d, e
Isakov, V., Arunachalam, S., Batterman, S., Bereznicki, S., Burke, J., Dionisio, K., Garcia, V., Heist, D., Perry, S., Snyder, M., and Vette, A.: Air Quality Modeling in Support of the Near-Road Exposures and Effects of Urban Air Pollutants Study (NEXUS), Int. J. Env. Res. Pub. He., 11, 8777–8793, https://doi.org/10.3390/ijerph110908777, 2014. a, b
Janssen, S., Guerreiro, C., Viaene, P., Georgieva, E., and Thunis, P.: Guidance Document on Modelling Quality Objectives Benchmarking, Version 2.1, Forum for air quality modelling in Europe, Tech. Rep. February, available at: http://fairmode.jrc.ec.europa.eu/document/fairmode/WG1/Guidance_MQO_Bench_vs2.2.pdf (last access: 21 June 2019), 2017. a
Jensen, S. S., Ketzel, M., Becker, T., Christensen, J., Brandt, J., Plejdrup, M., Winther, M., Nielsen, O., Hertel, O., and Ellermann, T.: High resolution multi-scale air quality modelling for all streets in Denmark, Transportation Research Part D: Transport and Environment, 52, 322–339, https://doi.org/10.1016/j.trd.2017.02.019, 2017. a, b, c, d
Jorba, O., Pandolfi, M., Spada, M., Baldasano, J. M., Pey, J., Alastuey, A., Arnold, D., Sicard, M., Artiñano, B., Revuelta, M. A., and Querol, X.: The DAURE field campaign: meteorological overview, Atmos. Chem. Phys. Discuss., 11, 4953–5001, https://doi.org/10.5194/acpd-11-4953-2011, 2011. a
Kanda, M., Inagaki, A., Miyamoto, T., Gryschka, M., and Raasch, S.: A New Aerodynamic Parametrization for Real Urban Surfaces, Bound.-Lay. Meteorol., 148, 357–377, https://doi.org/10.1007/s10546-013-9818-x, 2013. a
Kastner-Klein, P., Fedorovich, E., and Rotach, M. W.: A wind tunnel study of organised and turbulent air motions in urban street canyons, J. Wind Eng. Ind. Aerod., 89, 849–861, https://doi.org/10.1016/S0167-6105(01)00074-5, 2001. a
Kent, C., Grimmond, S., Barlow, J., Gatey, D., Kotthaus, S., Lindberg, F., and Halios, C.: Evaluation of Urban Local-Scale Aerodynamic Parameters: Implications for the Vertical Profile of Wind Speed and for Source Areas, Bound.-Lay. Meteorol., 164, 183–213, https://doi.org/10.1007/s10546-017-0248-z, 2017. a
Kim, Y., Wu, Y., Seigneur, C., and Roustan, Y.: Multi-scale modeling of urban air pollution: development and application of a Street-in-Grid model (v1.0) by coupling MUNICH (v1.0) and Polair3D (v1.8.1), Geosci. Model Dev., 11, 611–629, https://doi.org/10.5194/gmd-11-611-2018, 2018. a, b
Kochanski, A., Pardyjak, E., Stoll, R., Gowardhan, A., Brown, M., and Steenburgh, W.: One-way coupling of the WRF-QUIC Urban dispersion modeling system, J. Appl. Meteorol. Clim., 54, 2119–2139, https://doi.org/10.1175/JAMC-D-15-0020.1, 2015. a
Lefebvre, W., Vercauteren, J., Schrooten, L., Janssen, S., Degraeuwe, B., Maenhaut, W., de Vlieger, I., Vankerkom, J., Cosemans, G., Mensink, C., Veldeman, N., Deutsch, F., Van Looy, S., Peelaerts, W., and Lefebre, F.: Validation of the MIMOSA-AURORA-IFDM model chain for policy support: Modeling concentrations of elemental carbon in Flanders, Atmos. Environ., 45, 6705–6713, https://doi.org/10.1016/j.atmosenv.2011.08.033, 2011. a
Macdonald, R., Griffiths, R., and Hall, D.: An improved method for the estimation of surface roughness of obstacle arrays, Atmos. Environ., 32, 1857–1864, https://doi.org/10.1016/S1352-2310(97)00403-2, 1998. a
Maiheu, B., Lefebvre, W., Walton, H., Dajnak, D., Janssen, S., Williams, M., Blyth, L., and Beevers, S.: Improved Methodologies for NO2 Exposure Assessment in the EU, Tech. Rep. 2, VITO, available at:http://ec.europa.eu/environment/air/publications/models.htm (last access: 20 June 2019), 2017. a, b
Martilli, A., Clappier, A., and Rotach, M.: An urban surface exchange parameterisation for mesoscale models, Bound.-Lay. Meteorol., 104, 261–304, https://doi.org/10.1023/A:1016099921195, 2002. a
Monin, A. and Obukhov, A.: Osnovnye zakonomernosti turbulentnogo peremeshivanija v prizemnom sloe atmosfery (Basic Laws of Turbulent Mixing in the Atmosphere Near the Ground), Trudy geofiz. inst. AN SSSR, 24, 163–187, 1954. a, b
Moreno-Garcia, M.: Intensity and form of the urban heat island in barcelona, Int. J. Climatol., 14, 705–710, https://doi.org/10.1002/joc.3370140609, 1994. a
Moussafir, J., Olry, C., Nibart, M., Albergel, A., Armand, P., Duchenne, C., and Thobois, L.: AIRCITY: a very high resolution atmospheric dispersion modeling system for Paris, American Society of Mechanical Engineers, Fluids Engineering Division (Publication) FEDSM, 1–5, FEDSM2014-21820, https://doi.org/10.1115/FEDSM2014-21820, 2014. a
Oke, T.: Street design and urban canopy layer climate, Energ. Buildings, 11, 103–113, https://doi.org/10.1016/0378-7788(88)90026-6, 1988. a
Pay, M. T., Martínez, F., Guevara, M., and Baldasano, J. M.: Air quality forecasts on a kilometer-scale grid over complex Spanish terrains, Geosci. Model Dev., 7, 1979–1999, https://doi.org/10.5194/gmd-7-1979-2014, 2014. a
QGIS Development Team: QGIS Geographic Information System, Tech. rep., Open Source Geospatial Foundation, available at: http://qgis.osgeo.org (last access: 20 June 2019), 2017. a
Rotach, M. W.: Profiles of turbulence statistics in and above an urban street canyon, Atmos. Environ., 29, 1473–1486, https://doi.org/10.1016/1352-2310(95)00084-C, 1995. a
Salizzoni, P., Soulhac, L., and Mejean, P.: Street canyon ventilation and atmospheric turbulence, Atmos. Environ., 43, 5056–5067, https://doi.org/10.1016/j.atmosenv.2009.06.045, 2009. a
Skamarock, W. and Klemp, J.: A time-split nonhydrostatic atmospheric model for weather research and forecasting applications, J. Comput. Phys., 227, 3465–3485, https://doi.org/10.1016/j.jcp.2007.01.037, 2008. a
Snyder, M. and Heist, D.: User’s Guide for R-LINE Model Version 1.2 A Research LINE source model for near-surface releases, Tech. rep., USEPA, available at: https://www.cmascenter.org/r-line/documentation/1.2/RLINE_UserGuide_11-13-2013.pdf (last access: 20 June 2019), 2013. a, b
Snyder, M., Venkatram, A., Heist, D., Perry, S., Petersen, W., and Isakov, V.: RLINE: a line source dispersion model for near-surface releases, Atmos. Environ., 77, 748–756, https://doi.org/10.1016/j.atmosenv.2013.05.074, 2013. a, b
Soret, A., Guevara, M., and Baldasano, J.: The potential impacts of electric vehicles on air quality in the urban areas of Barcelona and Madrid (Spain), Atmos. Environ., 99, 51–63, https://doi.org/10.1016/j.atmosenv.2014.09.048, 2014. a
Soulhac, L., Perkins, R., and Salizzoni, P.: Flow in a street canyon for any external wind direction, Bound.-Lay. Meteorol., 126, 365–388, https://doi.org/10.1007/s10546-007-9238-x, 2008. a
Soulhac, L., Salizzoni, P., Cierco, F. X., and Perkins, R.: The model SIRANE for atmospheric urban pollutant dispersion; part I, presentation of the model, Atmos. Environ., 45, 7379–7395, https://doi.org/10.1016/j.atmosenv.2011.07.008, 2011. a, b
Stocker, J., Hood, C., Carruthers, D., Seaton, M., and Johnson, K.: The development and evaluation of an automated system for nesting ADMS-URBAN in regional photochemical models, 13th Annual CMAS Conference, Chapel Hill, NC, 27–29 October 2014, 1–6, 2014. a
Sunyer, J., Esnaola, M., Alvarez-Pedrerol, M., Forns, J., Rivas, I., López-Vicente, M., Suades-González, E., Foraster, M., Garcia-Esteban, R., Basagaña, X., Viana, M., Cirach, M., Moreno, T., Alastuey, A., Sebastian-Galles, N., Nieuwenhuijsen, M., and Querol, X.: Association between Traffic-Related Air Pollution in Schools and Cognitive Development in Primary School Children: A Prospective Cohort Study, PLoS Med., 12, e1001792, https://doi.org/10.1371/journal.pmed.1001792, 2015. a
Thunis, P. and Cuvelier, C.: Concepts/User’s, DELTA Version 5.4 Guide/Diagrams, Tech. rep., available at: http://fairmode.jrc.ec.europa.eu/Document/fairmode/WG1/DELTA_UserGuide_V5_4.pdf (last access: 20 June 2019), 2016. a
Valencia, A., Venkatram, A., Heist, D., Carruthers, D., and Arunachalam, S.: Development and evaluation of the R-LINE model algorithms to account for chemical transformation in the near-road environment, Transport. Res. D-Tr. E., 59, 464–477, https://doi.org/10.1016/j.trd.2018.01.028, 2018. a, b
Vardoulakis, S., Fisher, B., Pericleous, K., and Gonzalez-Flesca, N.: Modelling air quality in street canyons: A review, Atmos. Environ, 37, 155–182, https://doi.org/10.1016/S1352-2310(02)00857-9, 2003. a
Venkatram, A., Snyder, M., Heist, D., Perry, S., Petersen, W., and Isakov, V.: Re-formulation of plume spread for near-surface dispersion, Atmos. Environ., 77, 846–855, https://doi.org/10.1016/j.atmosenv.2013.05.073, 2013. a
Wild, R., Dubé, W., Aikin, K., Eilerman, S., Neuman, J., Peischl, J., Ryerson, T. B., and Brown, S. S.: On-road measurements of vehicle NO2∕NOx emission ratios in Denver, Colorado, USA, Atmos. Environ., 148, 182–189, https://doi.org/10.1016/j.atmosenv.2016.10.039, 2017. a
- Abstract
- Introduction
- Methods
- Observational datasets
- Results and discussion
- Conclusions
- Code availability
- Appendix A: Extended urban geometry characterisation
- Appendix B: Extended performance evaluation
- Appendix C: Description of model evaluation statistics
- Author contributions
- Competing interests
- Disclaimer
- Acknowledgements
- Financial support
- Review statement
- References
- Abstract
- Introduction
- Methods
- Observational datasets
- Results and discussion
- Conclusions
- Code availability
- Appendix A: Extended urban geometry characterisation
- Appendix B: Extended performance evaluation
- Appendix C: Description of model evaluation statistics
- Author contributions
- Competing interests
- Disclaimer
- Acknowledgements
- Financial support
- Review statement
- References