the Creative Commons Attribution 4.0 License.
the Creative Commons Attribution 4.0 License.
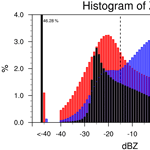
The importance of considering sub-grid cloud variability when using satellite observations to evaluate the cloud and precipitation simulations in climate models
Hua Song
Po-Lun Ma
Steven Ghan
Minghuai Wang
Satellite cloud observations have become an indispensable tool for evaluating general circulation models (GCMs). To facilitate the satellite and GCM comparisons, the CFMIP (Cloud Feedback Model Inter-comparison Project) Observation Simulator Package (COSP) has been developed and is now increasingly used in GCM evaluations. Real-world clouds and precipitation can have significant sub-grid variations, which, however, are often ignored or oversimplified in the COSP simulation. In this study, we use COSP cloud simulations from the Super-Parameterized Community Atmosphere Model (SPCAM5) and satellite observations from the Moderate Resolution Imaging Spectroradiometer (MODIS) and CloudSat to demonstrate the importance of considering the sub-grid variability of cloud and precipitation when using the COSP to evaluate GCM simulations. We carry out two sensitivity tests: SPCAM5 COSP and SPCAM5-Homogeneous COSP. In the SPCAM5 COSP run, the sub-grid cloud and precipitation properties from the embedded cloud-resolving model (CRM) of SPCAM5 are used to drive the COSP simulation, while in the SPCAM5-Homogeneous COSP run only grid-mean cloud and precipitation properties (i.e., no sub-grid variations) are given to the COSP. We find that the warm rain signatures in the SPCAM5 COSP run agree with the MODIS and CloudSat observations quite well. In contrast, the SPCAM5-Homogeneous COSP run which ignores the sub-grid cloud variations substantially overestimates the radar reflectivity and probability of precipitation compared to the satellite observations, as well as the results from the SPCAM5 COSP run. The significant differences between the two COSP runs demonstrate that it is important to take into account the sub-grid variations of cloud and precipitation when using COSP to evaluate the GCM to avoid confusing and misleading results.
Marine boundary layer (MBL) cloud, as a strong modulator of the radiative energy budget of the Earth–atmosphere system, is a major source of uncertainty in future climate change projections of the general circulation models (GCMs) (Cess et al., 1996; Bony and Dufresne, 2005). Improving MBL cloud simulations in the GCMs is one of the top priorities of the climate modeling community. As the cloud parameterization schemes in the GCMs become increasingly sophisticated, there is a strong need for comprehensive global satellite cloud observations for model evaluation and improvement. However, the fundamental definitions of clouds in GCMs differ dramatically from those used for satellite remote sensing, which hampers the use of satellite products for model evaluation. In order to overcome this obstacle, the Cloud Feedback Model Intercomparison Project (CFMIP) community has developed an integrated satellite simulator, the CFMIP Observation Simulator Package (COSP) (Zhang et al., 2010; Bodas-Salcedo et al., 2011). COSP has greatly facilitated and promoted the use of satellite data in the climate modeling community to expose and diagnose issues in GCM cloud simulations (e.g., Marchand et al., 2009; Zhang et al., 2010; Kay et al., 2012, 2016; Pincus et al., 2012; Song et al., 2018).
Warm rain is a unique and important feature of MBL clouds. It plays an important role in determining the macro- and micro-physical properties of MBL clouds, in particular, the cloud water budget (e.g., Stevens et al., 2005; Wood, 2005; Comstock et al., 2005). Many previous studies have investigated the warm rain simulation in GCMs using the COSP simulators. These studies reveal a common problem in the latest generation of GCMs; i.e., the drizzle in MBL clouds is too frequent in the GCM compared with satellite observations (e.g., Zhang et al., 2010; Franklin et al., 2013; Suzuki et al., 2015; Takahashi et al., 2017; Jing et al., 2017; Song et al., 2017; Bodas-Salcedo et al., 2008, 2011; Stephens et al., 2012; Nam and Quaas, 2012; Franklin et al., 2013; Jing et al., 2017). One possible reason for the excessive warm rain production in GCMs could be the model's inaccurate representation of physical processes, such as auto-conversion and accretion, that govern the precipitation efficiency in warm MBL clouds. Due to the lack of sub-grid variability of microphysical quantities in most large-scale models, the auto-conversion parameterization is overly aggressive, so that the models tend to produce precipitation too quickly (Lebsock et al., 2013; Song et al., 2017).
The radar observations of warm rain from CloudSat and collocated MODIS (Moderate Resolution Imaging Spectroradiometer) cloud observations are extremely useful data for assessing and improving the GCM simulations of MBL clouds and their precipitation process. However, the dramatic spatial resolution differences between the conventional GCM (∼100 km) and satellite observations (∼1 km) become a challenging obstacle for the satellite and GCM comparisons. To overcome this obstacle, the COSP first divides the grid-level cloud and precipitation properties (e.g., grid-mean cloud water and rain water) into the so-called “sub-columns” that are conceptually similar to “pixel” in satellite observation. Then, for each sub-column the COSP satellite simulators (e.g., COSP-CloudSat and COSP-MODIS) simulate the satellite measurements (e.g., radar reflectivity) and retrievals (e.g., MODIS cloud optical depth and effective radius) which become directly comparable with satellite data. Ideally, the sub-column generation in COSP should be consistent with the sub-grid cloud parameterization scheme in the host GCM. However, in practice sub-grid variations of cloud and precipitation are often ignored or treated crudely in the COSP simulation for a number of possible reasons. First of all, the COSP is an independent package, and it takes substantial efforts to implement in the COSP a sub-grid cloud generation scheme that is consistent with the host GCM. Secondly, a simple sub-column generation scheme helps alleviate the computational cost associated with the COSP simulation. Last but certainly not least, the users of the COSP might not be fully aware of the consequences of ignoring the sub-grid cloud and precipitation variability in the COSP simulations.
The current version (v1.4) of COSP provides a built-in highly simplified sub-column generator. It accounts only for the sub-grid variability of the types of hydrometeors and ignores the variability of mass and microphysics within each hydrometeor type. The water content and microphysical properties (i.e., droplet effective radius and optical thickness) of each hydrometeor are horizontally homogenous among all the sub-columns that are labeled as the same type (i.e., stratiform or convective). Here we refer to the current scheme as the “homogenous hydrometeor scheme”. The uncertainties and potential biases caused by the homogenous hydrometeor scheme can be significant and should not be overlooked. A simple hypothetical example is provided in Fig. 1 to illustrate the importance of accounting for the sub-grid variability of rainwater in simulating the CloudSat radar reflectivity. To be consistent with the two-moment cloud microphysics scheme (Morrison and Gettelman, 2008) that is widely used in the GCMs, we assume the sub-grid distribution of rainwater to follow the exponential distribution. In this example, the grid-mean rainwater mixing ratio () is set to be 0.03 g kg−1 (dashed blue line in Fig. 1a). Using the Quickbeam simulator (Haynes et al., 2007) in COSP, we simulated the corresponding 94 GHz CloudSat radar reflectivity, which is shown in Fig. 1b. The grid-mean radar reflectivity based on the exponentially distributed rainwater (i.e., with sub-grid variance) is about 4 dBZ (solid red line in Fig. 1b). In contrast, if the sub-grid variation of rainwater is ignored, the radar reflectivity corresponding to g kg−1 is 13 dBZ (dashed blue line in Fig. 1b). The substantial difference between the two indicates that ignoring the sub-grid variability of hydrometeors could cause significant overestimation of grid-mean radar reflectivity simulation, which in turn could complicate and even mislead the evaluation of GCMs.
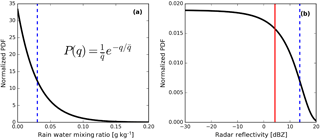
Figure 1(a) PDF of the rainwater mixing ratio for rainwater when the horizontal variability of rainwater is assumed to follow the exponential distribution. The vertical dashed blue line indicates the mean value of the rainwater mixing ratio as 0.03 g kg−1. (b) The corresponding PDF of the CloudSat radar reflectivity simulated by COSP assuming the Marshall and Palmer particle size distribution. The dashed blue line corresponds to the radar reflectivity based on the mean rainwater 0.03 g kg−1, and the solid red line corresponds to the grid-mean radar reflectivity based on the PDF of the rainwater mixing ratio.
The objective of this study is to investigate and demonstrate to the GCM modeling community the importance of considering the sub-grid variability of cloud and precipitation properties when evaluating the GCM simulations using COSP. Here we employ the Super-parameterized Community Atmosphere Model Version 5 (SPCAM5, Wang et al., 2015) to provide the sub-grid cloud and precipitation hydrometeor fields for a comparison study of the simulated radar reflectivity and warm rain frequencies by COSP. Fundamentally different from the convective cloud parameterization schemes in GCMs, SPCAM5 consists of a two-dimensional cloud-resolving model (CRM) embedded into each grid of a conventional CAM5 (Khairoutdinov and Randall, 2003; Wang et al., 2015). In SPCAM5, the sub-grid cloud dynamical and microphysical processes are explicitly resolved at a 4 km resolution using a two-dimensional version of the System for Atmospheric Modeling (Khairoutdinov and Randall, 2003) with the two-moment microphysics scheme (Morrison et al., 2005). We carry out two sensitivity tests: SPCAM5 COSP and SPCAM5-Homogeneous COSP. In the SPCAM5 COSP run, the sub-grid cloud and precipitation properties from the embedded CRMs of SPCAM5 are used to drive the COSP simulation. In the SPCAM5-Homogeneous COSP run, the default homogenous hydrometeor scheme of COSP mentioned above is used to generate the sub-grid cloud and precipitation fields for the COSP simulation. The outputs from the two runs are compared with the collocated CloudSat and MODIS observations to assess the potential problems in both runs, and also to understand the impacts of omitting sub-grid cloud variations in the COSP simulations.
The rest of the paper is organized as follows: Sect. 2 describes the model, COSP, and satellite data used in this study. Results are represented in Sect. 3. Finally, Sect. 4 provides general conclusions and remarks.
2.1 Model
The model used in this study is SPCAM5, an application of the Multiscale Modeling Framework (MMF) (Randall et al., 2003; Khairoutdinov et al., 2005, 2008; Tao et al., 2009) to CAM5 (Neale et al., 2010), which uses the finite volume dynamical core at 1.9∘ latitude × 2.5∘ longitude resolution with 30 vertical levels and a 600 s time step. The embedded 2-D CRM in each CAM5 grid cell includes 32 columns at 4 km horizontal grid spacing and 28 vertical layers coinciding with the lowest 28 CAM5 levels. The CRM runs with a 20 s time step. Details of the SPCAM5 can be found in Wang et al. (2011, 2015). The simulations are run in a “constrained meteorology” configuration (Ma et al., 2013, 2015) to facilitate model evaluation against observations, in which the model winds are nudged toward the Modern Era Reanalysis for Research Applications (MERRA) reanalysis with a relaxation timescale of 6 h (Zhang et al., 2014). The SPCAM5 simulations are performed from September 2008 to December 2010 (28 months). The last 24 months' (January 2009–December 2010) outputs of the simulations are used for analysis.
2.2 COSP
We used COSP Version 1.4, which has no scientific difference from the latest version, COSP2 (Swales et al., 2018). Currently, COSP provides simulations of ISCCP (International Satellite Cloud Climatology Project), CALIPSO (Cloud-Aerosol Lidar and Infrared Pathfinder Satellite Observation), CloudSat, MODIS, and MISR (Multi-angle Imaging SpectroRadiometer) cloud measurements and/or retrievals (Bodas-Salcedo et al., 2011). In this study, we will focus on the MODIS and CloudSat simulators (Pincus et al., 2012; Haynes et al., 2007). COSP has three major parts, each controlling a step of the pseudo-retrieval process: (1) the sub-column generator of COSP first distributes the grid-mean cloud and precipitation properties from GCMs into the so-called sub-columns that are conceptually similar to “pixels” in satellite remote sensing; (2) the satellite simulators simulate the direct measurements (e.g., CloudSat radar reflectivity and CALIOP backscatter) and retrieval products (e.g., MODIS cloud optical thickness and effective radius) for each sub-column using highly simplified radiative transfer and retrieval schemes; (3) the aggregation scheme averages the sub-column simulations back to grid level to obtain temporal–spatial averages that are comparable with aggregated satellite products (e.g., MODIS level-3 gridded monthly mean products).
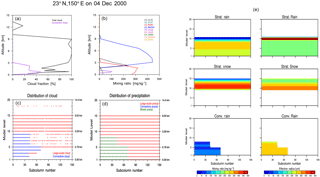
Figure 2At the single-grid 23∘ N and 150∘ E on 4 December 2010 in the CAM5-Base simulation (Song et al., 2017): (a) the grid-mean total (stratiform plus convective) and convective cloud fraction. (b) The grid-mean mixing ratios of cloud and precipitation hydrometeors (LS_CLIQ: large-scale (i.e., stratiform) cloud water; LS_CICE: large-scale cloud ice; LS_RAIN: large-scale rain; LS_SNOW: large-scale snow; LS_GRPL: large-scale graupel; CV_CLIQ: convective cloud water; CV_CICE: convective cloud ice; CV_RAIN: convective rain; CV_SNOW: convective snow). (c) The distribution of large-scale (red plus signs for frac_out=1) and convective (blue plus signs for frac_out=2) cloud among the sub-columns generated by the SCOPS scheme (i.e., frac_out from scops.f). (d) The distribution of large-scale (red plus signs for prec_frac=1), convective (blue plus signs for prec_frac=2), and mixed (green plus signs for prec_frac=3) precipitation among the sub-columns generated by the SCOPS-PREC scheme (i.e., prec_frac from prec_scops.f). (e) The mixing ratio (left panels) and effective radius (right panels) of three precipitation hydrometeor types among the sub-columns.
As mentioned in the Introduction, COSP-v1.4 has a highly simplified built-in sub-column generator based on the homogenous hydrometeor scheme. This scheme accounts only for the sub-grid variability of the types of hydrometeors and ignores the variability of mass and microphysics within each hydrometeor type. An example is provided in Fig. 2 to illustrate how this default sub-column generator of COSP-v1.4 distributes the grid-mean cloud and precipitation into the sub-columns. We arbitrarily selected a grid (23∘ N and 150∘ E) with both cloud and significant precipitation from our previous CAM5 simulation (CAM5-Base simulation in Song et al., 2017). Figure 2a shows the vertical profiles of the grid-mean total (stratiform plus convective) and convective cloud fractions at the selected grid box. Figure 2b shows the vertical profiles of the grid-mean mixing ratios of each type of hydrometeor. The sub-column generator of COSP takes the grid-mean cloud fractions, hydrometeor mixing ratios, and effective particle sizes (Fig. 2a and b) as inputs to generate the sub-columns for the later satellite measurement and retrieval simulation.
First, sub-columns (150 sub-columns are generated in our example) are assigned as either cloudy or clear at each model level by the Subgrid Cloud Overlap Profile Sampler (SCOPS), which was developed originally as part of the ISCCP simulator (Klein and Jakob, 1999; Webb et al., 2001). Figure 2c shows the distributions of cloudy sub-columns among the 150 sub-columns at each vertical level, indicated by variable frac_out produced in the scops.f routine. The sub-column at a certain vertical level is stratiform cloudy if frac_out=1, or connective cloudy if frac_out=2 at that vertical level. As illustrated in Fig. 2c, the SCOPS assigns cloud to the sub-columns in a manner consistent with the model's grid box average stratiform and convective cloud amounts (Fig. 2a) and its cloud overlap assumption, i.e., maximum-random overlap in this case. The next step is to determine which of the sub-columns generated by SCOPS contain precipitation hydrometeors, e.g., rain and snow. This step is necessary and critical for the COSP CloudSat radar simulator (Bodas-Salcedo et al., 2011) because radar reflectivity is highly sensitive to the precipitation hydrometeors due to their large particle size (L'Ecuyer and Stephens, 2002; Tanelli et al., 2008). The current sub-grid precipitation distribution scheme, “SCOPS-PREC”, is developed and described in Zhang et al. (2010). Figure 2d shows the masking of precipitation among the 150 sub-columns generated by SCOPS-PREC for the example grid. After the cloud and precipitation are masked, the last step is to specify the mass (i.e., mixing ratio) and effective radius of hydrometeors for all the sub-columns occupied by clouds and/or precipitation. The current scheme for this step is highly simplified. As shown in Fig. 2e, it assumes the mass and the microphysics of each type of hydrometeor to be horizontally homogeneous among all the sub-columns that are occupied by this type of hydrometeor at a given model level. In other words, at each model level the only difference among sub-columns is that they may be occupied by different types of hydrometeors (Zhang et al., 2010).
In this study, we have carried out two COSP simulations using the 2-year SPCAM5 CRM outputs to investigate the importance of considering the sub-grid variations of cloud and precipitation properties when evaluating the GCM simulations using COSP. The two COSP simulations are marked as SPCAM5 COSP and SPCAM5-Homogeneous COSP, respectively. For the SPCAM5 COSP simulation, we treat the sub-grid cloud and precipitation fields from the CRM of SPCAM5 outputs as sub-columns of COSP without using the COSP sub-column generator. For the SPCAM5-Homogeneous COSP simulation, we first average the sub-grid cloud and precipitation fields (including both clear and cloudy sub-grids) from the CRM of SPCAM5 to each CAM5 grid, and then input these grid-mean cloud and precipitation fields to the default COSP-v1.4 sub-column simulator described above to generate the sub-column fields. All the other processes of two COSP simulations are exactly the same. The COSP simulator outputs are produced from 6-hourly calculations and the number of sub-columns used here is 32. To derive the probability of precipitation, we made some simple in-house modifications in COSP v1.4 to write out the MODIS and CloudSat simulations for every sub-column. This allows us to derive the joint statistics of COSP-MODIS and COSP-CloudSat simulations and compare them with those derived from collocated MODIS and CloudSat level-2 products.
2.3 Satellite data
The cloud measurements from the A-Train satellite sensors, namely MODIS and CloudSat, are used for model-to-observation comparison. The newly released collection 6 (C6) Aqua-MODIS cloud products (Platnick et al., 2017) are used to evaluate cloud fraction, cloud optical thickness, and cloud droplet effective radius. For MBL cloud studies, CloudSat provides valuable information on the warm rain process that cannot be achieved by a passive sensor like MODIS. The direct measurement of CloudSat is the vertical profile of 94 GHz radar reflectivity by cloud and hydrometer particles (i.e., 2B-GEOPROF product), from which other information such as vertical distribution of clouds and precipitation can be derived. The CloudSat 2B-GEOPROF product (Marchand et al., 2008) is used for cloud vertical structure, radar reflectivity, and identification of precipitation in MBL clouds. To prepare for the comparison of joint statistics, we collocated 5 years (2006–2010) of pixel-level (i.e., level-2) MODIS and CloudSat observations using the collocation scheme developed in Cho et al. (2008). Due to the low sampling rate of CloudSat, we used 5 years (2006–2010) of observations, in comparison with the 2-year model simulation (2009–2010), to obtain enough statistics. A sensitivity study indicates that the inter-annual variability of MBL clouds is much smaller than the model-to-observation differences.
In this study, we focus on the tropical and subtropical regions between 45∘ S and 45∘ N (loosely referred to as “tropical and subtropical region”), where most stratocumulus and cumulus regimes are found. We avoid high latitudes because satellite observations, namely MODIS, may have large uncertainties at low solar zenith angles there (Kato and Marshak, 2009; Grosvenor and Wood, 2014; Cho et al., 2015).
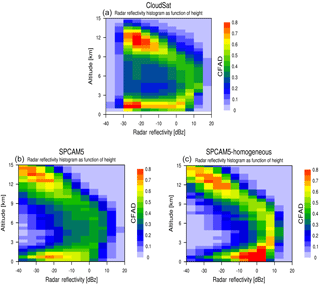
Figure 3Tropical-averaged radar reflectivity–height histogram in the CloudSat observation (a), the SPCAM5 CloudSat simulation (b), and the SPCAM5-Homogeneous CloudSat simulation (c).
First, we compare the Contoured Frequency by Altitude Diagram (CFAD) of tropical clouds derived based on SPCAM5 COSP and SPCAM5-Homogeneous COSP simulations with that derived from the CloudSat 2B-GEOPROF product in Fig. 3. The CFAD-based CloudSat observations display a typical boomerang-type shape that has been reported in many previous studies (Bodas-Salcedo et al., 2011; Zhang et al., 2010; Marchand et al., 2009). Focusing on the low clouds below 3 km, we observe a rather broad distribution of radar reflectivity with a maximum occurrence frequency around −30 to −20 dBZ followed by a long tail extending to about 10 dBZ. As pointed out in previous studies, the peak around −30 to −20 dBZ is due to non-precipitating MBL clouds and the precipitating clouds with increasing rain rate give rise to the long tail. The CFAD based on two COSP simulations exhibits some characteristics similar to the CloudSat observations, but also many noticeable differences. In particular, the two COSP simulations both produce a much narrower range of radar reflectivity for low clouds, with occurrence frequency clustered mostly around −25 dBZ in SPCAM5 COSP and around 0 dBZ in SPCAM5-Homogeneous COSP. These results show that using the oversimplified COSP sub-column generator (e.g., the homogeneous hydrometeor scheme) has non-negligible influences on the simulated radar reflectivity and produces artificially high occurrences of large radar reflectivity. Consistent with many previous studies (e.g., Bodas-Salcedo et al., 2008; Stephens et al., 2012; Nam and Quaas, 2012; Franklin et al., 2013; Jing et al., 2017), our results also reveal that GCMs tend to produce much larger radar reflectivity more frequently through the COSP simulator compared to the satellite observation.
The systematic biases in simulated radar reflectivity by the COSP homogeneous hydrometeor scheme might lead to the unjustified and biased evaluation of the warm rain production in GCMs, since cloud column maximum radar reflectivity (Zmax) is often used to distinguish precipitating from non-precipitating MBL clouds (Kubar and Hartmann, 2009; Lebsock and Su, 2014; Haynes et al., 2009).
Next we compare the simulated and observed probability density functions (PDFs) of Zmax for all the sub-columns that are marked as warm liquid clouds in the domain between 45∘ S and 45∘ N. The warm liquid clouds are defined by the cloud phase and cloud top pressure derived from the MODIS simulator by the criteria that cloud phase is liquid and cloud top pressure is between 900 and 500 hPa. Big differences in the PDFs of Zmax between the SPCAM5-Homogeneous COSP and the A-Train observations, and between SPCAM5-Homogeneous COSP and SPCAM5 COSP, are shown in Fig. 4. First, in the A-Train observations, about 46 % of warm liquid clouds detected by MODIS are not observed by CloudSat. These clouds are either too thin and therefore their radar reflectivity is too weak to be detected by CloudSat, or they are too low and therefore suffer from the surface clutter issue (Marchand et al., 2008). For those warm liquid clouds detected by both MODIS and CloudSat, the PDF of Zmax peaks around −25 dBZ. Second, in both COSP simulations, almost all warm liquid clouds derived by the MODIS simulator have a valid CloudSat radar reflectivity larger than −40 dBZ. The PDFs of Zmax in SPCAM5 reasonably resemble those in the A-Train observations. However, significantly different from the other two, the distribution of Zmax in SPCAM5-Homogeneous shifts to the large dBZ values and peaks around 0 dBZ. In previous studies (e.g., Takahashi et al., 2017), warm liquid clouds are categorized into three different modes by Zmax: non-precipitating mode ( dBZ), drizzle mode (−15 dBZ < dBZ), and rain mode ( dBZ). The simulated and observed PDFs of Zmax demonstrate that a large portion of warm liquid clouds is non-precipitating in the observations and SPCAM5 COSP, while most warm liquid clouds are precipitating (drizzle or rain) clouds in the SPCAM5-Homogeneous COSP. The use of the COSP homogeneous hydrometeor scheme gives us a dramatically different assessment of the warm rain production of MBL clouds in the SPCAM5 model; i.e., if we consider the sub-column variability of cloud and precipitation in the COSP simulation, we find that the SPCAM5 model can reproduce the observed warm rain production quite well. However, if we ignore the CRM sub-grid variability and use the homogeneous hydrometeor scheme, we may make the biased conclusion that the SPCAM5 model performs badly in the simulation of warm rain production.
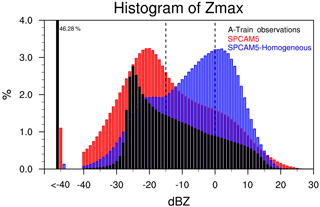
Figure 4The histograms of column maximum radar reflectivity for liquid clouds over oceanic regions from 45∘ S to 45∘ N in A-Train satellite observations, SPCAM5 COSP, and SPCAM5-Homogeneous COSP simulations.
More significant differences between the SPCAM5 COSP and SPCAM5-Homogeneous COSP simulations can be found from the spatial distributions of the probability of precipitation (POP) in MBL warm clouds (Fig. 5). Here, the POP for a given grid box is defined as the fraction of liquid-phase cloud identified by MODIS observations with Zmax larger than a certain threshold (i.e., −15 dBZ for drizzle or rain, 0 dBZ for rain, and 10 dBZ for heavy rain, respectively) according to the collocated CloudSat observations with respect to the total population liquid-phase clouds with the cloud top pressure between 500 and 900 hPa in the grid. Observations in Fig. 5 suggest that roughly a third of MBL clouds observed by MODIS in the tropical and subtropical region are likely precipitating (drizzle or rain), with a domain-averaged POP around 33 %. The POP of drizzle plus rain has a distinct pattern: smaller (∼15 %) in the coastal Sc regions and increasing to ∼50 % in the Cu cloud regions. The observed POPs of rain and heavy rain show similar spatial patterns to those of drizzle plus rain, with much smaller domain-averaged POP being about 12.5 % and 3.3 %, respectively.
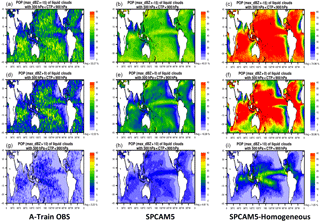
Figure 5Probability of precipitation (POP) of liquid clouds between 500 and 900 hPa levels in the satellite observations (a, d, g), the SPCAM5 COSP simulation (b, e, h), and the SPCAM5-Homogeneous COSP simulation (c, f, i). Three categories of precipitation: drizzle plus rain (column dBZ, a, b, c), rain (column dBZ, d, e, f), and strong rain only (column dBZ, g, h, i). Unit of POP is %.
In the same way as we define POP for observations, we define the POP for two COSP simulations as the ratio of sub-columns that have COSP-CloudSat simulated Zmax larger than a certain threshold with respect to the total number of liquid-phase clouds identified by COSP-MODIS. As shown in Fig. 5, two COSP simulations show dramatically different spatial distributions of POPs. The SPCAM5 COSP produces the similar POP patterns to those in the observations, with the domain-averaged POPs for drizzle or rain, rain, and heavy rain being about 43 %, 16 %, and 4.5 %, respectively. However, the POPs in the SPCAM5-Homogeneous COSP are substantially overestimated, with the domain-averaged POPs for drizzle or rain, rain, and heavy rain being about 75 %, 36 %, and 7 %, respectively. Using the COSP homogeneous hydrometeor scheme will lead to the conclusion that the drizzle or rain is triggered too frequently (more than double the observations) in the SPCAM5 model, which obviously is not a fair assessment.
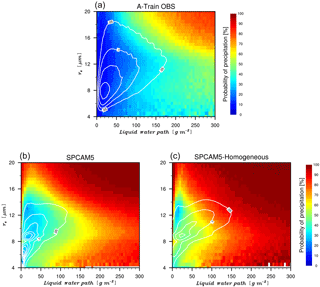
Figure 6POP (drizzle or rain) of liquid clouds at each LWP and liquid cloud effective radius in the satellite observations (a), the SPCAM5 COSP simulation (b), and the SPCAM5-Homogeneous COSP simulation (c). The white solid contours are joint PDF of LWP and liquid cloud effective radius. Units of POP and PDF are %.
Previous studies find that the warm rain production in MBL clouds is tightly related to the in-cloud microphysical properties of MBL clouds (e.g., Stevens et al., 2005; Wood, 2005; Comstock et al., 2005). Next, we check the dependence of POP on in-cloud properties' liquid water path (LWP) and on liquid cloud effective radius (re) in both observations and two COSP simulations. Figure 6 shows the POPs of drizzle or rain (i.e., dBZ) as a function of in-cloud LWP and re overlaid by the joint PDF of LWP and re (white contours) in the satellite observations and two COSP simulations. The observed POPs of warm liquid clouds increase monotonically with increasing in-cloud LWP and re, with high POPs concentrating on the domain with large values of LWP and re (i.e., LWP >200 g m−2 and re>15 µm). However, in the two COSP simulations, especially the SPCAM5-Homogeneous COSP, at each joint bin the POPs are much larger than those in the A-Train observations. When in-cloud LWP (re) is larger than 150 g m−2 (17 µm), the dependence of POPs on in-cloud re (LWP) is small. The joint PDFs of in-cloud LWP and re in the observations and two COSP simulations are also quite different. There are more occurrences with large LWP and re in the MODIS observations than the two COSP simulations. The SPCAM5 COSP simulations have two peaks of the joint PDFs, which are converted to one occurrence peak in the SPCAM5-Homogeneous COSP simulation by using the COSP homogeneous hydrometeor scheme.
Based on the above comparisons, we can see that the oversimplified COSP sub-column generator contributes to not only the narrow distribution of MBL cloud radar reflectivity, but also to unrealistically high POPs in the SPCAM5 model. Besides, it also changes the distribution of in-cloud microphysical properties, and the relationship between POPs and cloud microphysical properties as well.
This study presents a satellite-based evaluation of the warm rain production of MBL cloud in the SPCAM5 model using two COSP simulations (SPCAM5 COSP and SPCAM5-Homogeneous COSP), with the objective of demonstrating the importance of considering the sub-grid variability of cloud and precipitation when using COSP to evaluate GCM simulations. Through the SPCAM5 COSP simulations, in which the sub-column variability of cloud and precipitation is considered, we find that the SPCAM5 model can reproduce the observed warm rain production quite well. However, in the SPCAM5-Homogeneous COSP simulation, in which we ignore the CRM sub-grid variability and use the COSP homogeneous hydrometeor scheme, the simulated radar reflectivity and POPs in the SPCAM5 are significantly overestimated compared to the observations. Therefore, use of the COSP homogeneous hydrometeor scheme gives us a significantly different assessment of warm rain production of MBL clouds in the SPCAM5 model. Our results also indicate that the sub-grid variability of mass and microphysics of each hydrometeor type is key to the realistic simulation of radar reflectivity.
The systematic and significant biases due to the limitation of the current homogeneous hydrometeor scheme can mislead the evaluation of GCMs and should not be overlooked. In this regard, an improved sub-column generator needs to be developed for COSP to account for the sub-grid variances of cloud and/or hydrometer mass and microphysics. A recent study of Hillman et al. (2018) investigated the sensitivities of simulated satellite retrievals to subgrid-scale overlap and condensate heterogeneity, and demonstrated the systematic biases in the simulated MODIS cloud fraction and CloudSat radar reflectivity due to the oversimplified COSP sub-column generator. Their study also proposed a new scheme to replace the COSP current sub-column generator, and showed that the new scheme can produce much better satellite retrievals. Implementing their sub-column heterogeneous hydrometeor scheme in COSP may improve the GCM COSP simulations and give a better-justified assessment of the GCM performance in simulating warm rain processes and cloud microphysical properties.
On the other hand, since the assumptions of sub-grid variability of cloud and hydrometeors in different GCMs may be quite different, one universal sub-column hydrometeor scheme may be not applicable to all models. Based on this consideration, the latest version, COSP version 2, enhances flexibility by allowing for model-specific representation of sub-grid-scale cloudiness and hydrometeor condensates and encourages the users to implement the same sub-grid scheme as the host GCM for consistency (Swales et al., 2018). Nevertheless, our study also suggests that any evaluation study of warm rain production in GCMs by using COSP simulators should take this issue into account.
Details of SPCAM5 can be found in Wang et al. (2011, 2015). The host GCM in SPCAM5 is the Community Atmospheric Model, version 5 (see details on the CESM website at http://www.cesm.ucar.edu/models/cesm1.1/cam/, last access: 19 July 2018). SPCAM5 has recently been merged with CESM1.1.1 and released to the public (Randall et al., 2013; https://svn-ccsm-release.cgd.ucar.edu/model_development_releases/spcam2_0-cesm1_1_1, last access: 19 July 2018, registration required). Codes of COSP V1.4 can be found on the website at https://github.com/CFMIP/COSPv1 (last access: 19 July 2018). We used the collection 6 (C6) Aqua-MODIS cloud products (Platnick et al., 2017), which can be downloaded from the NASA website at https://lance3.modaps.eosdis.nasa.gov/data_products/ (last access: 19 July 2018). The CloudSat data are distributed by the CloudSat Data Processing Center. The CloudSat 2B-GEOPROF product we used is downloaded from the website at http://www.cloudsat.cira.colostate.edu/data-products/level-2b/2b-geoprof?term=42 (last access: 19 July 2018).
MW provided the source code of SPCAM5 and wrote the Fortran code to run COSP simulation with the sub-grid cloud and precipitation properties from the embedded CRMs of SPCAM5. PLM and SG provided the results of CAM5 simulations and helped us to find the excessive drizzle production problem in CAM5, which is partially due to the COSP's over-simplified sub-column generator. HS and ZZ carried out the SPCAM5 simulation, drafted the text and made the figures. All authors contributed to the editing of the manuscript.
The authors declare that they have no conflict of interest.
This research is supported by the U.S. Department of Energy (DOE), Office of
Science, Biological and Environmental Research, Regional and Global Climate
Mode Analysis Program (grant no. DE-SC0014641). The Pacific Northwest National Laboratory is operated for the DOE
by Battelle Memorial Institute under contract DE-AC05-76RLO 1830. Minghuai
Wang was supported by the Minister of Science and Technology of China
(2017YFA0604001). The computations in this study were performed at the UMBC
High Performance Computing Facility (HPCF). The facility is supported by the
U.S. National Science Foundation through the MRI program (grant nos.
CNS-0821258 and CNS-1228778) and the SCREMS program (grant no. DMS-0821311),
with substantial support from UMBC. The MODIS cloud products used in this
study are downloaded from the NASA Level-1 and Atmosphere Archive and
Distribution System from https://ladsweb.modaps.eosdis.nasa.gov/ (last
access: 19 July 2018). The CloudSat products are provided by the CloudSat
Data Processing Center from http://www.cloudsat.cira.colostate.edu/
(last access: 19 July 2018).
Edited by: Klaus Gierens
Reviewed by: two anonymous referees
Bodas-Salcedo, A., Webb, M. J., Brooks, M. E., Ringer, M. A., Williams, K. D., Milton, S. F., and Wilson, D. R.: Evaluating cloud systems in the Met Office global forecast model using simulated CloudSat radar reflectivities, J. Geophys. Res., 113, D00A13, https://doi.org/10.1029/2007JD009620, 2008.
Bodas-Salcedo, A., Webb, M. J., Bony, S., Chepfer, H., Dufresne, J.-L., Klein, S. A., Zhang, Y., Marchand, R., Haynes, J. M., Pincus, R., and John, V. O.: COSP: Satellite simulation software for model assessment, B. Am. Meteorol. Soc., 92, 1023–1043, https://doi.org/10.1175/2011BAMS2856.1, 2011.
Bony, S. and Dufresne, J.-L.: Marine boundary layer clouds at the heart of tropical cloud feedback uncertainties in climate models, Geophys. Res. Lett., 32, L20806, https://doi.org/10.1029/2005GL023851, 2005.
Cess, R., Zhang, M. H., Ingram, W. J., Potter, G. L., Alekseev, V., Barker, H. W., Cohen-Solal, E., Colman, R. A., Dazlich, D. A., Del Genio, A. D., Dix, M. R., Dymnikov, V., Esch, M., Fowler, L. D., Fraser, J. R., Galin, V., Gates, W. L., Hack, J. J., Kiehl, J. T., Le Treut, H., Lo, K. K.-W., McAvaney, B. J., Meleshko, V. P., Morcrette, J.-J., Randall, D. A., Roeckner, E., Royer, J.-F., Schlesinger, M. E., Sporyshev, P. V., Timbal, B., Volodin, E. M., Taylor, K. E., Wang, W., and Wetherald, R. T.: Cloud feedback in atmospheric general circulation models: An update, J. Geophys. Res.-Atmos., 101, 12791–12794, 1996.
Cho, H. M., Yang, P., Kattawar, G. W., Nasiri, S. L., Hu, Y., Minnis, P., Trepte, C., and Winker, D.: Depolarization ratio and attenuated backscatter for nine cloud types: Analyses based on collocated CALIPSO lidar and MODIS measurements, Opt. Express, 16, 3931–3948, 2008.
Cho, H. M., Zhang, Z., Meyer, K., Lebsock, M., Platnick, S., Ackerman, A. S., Di Girolano, L., C.-Labonnote, L., Cornet, C., Riedi, J., and Holz, R. E.: Frequency and causes of failed MODIS cloud property retrievals for liquid phase clouds over global oceans, J. Geophys. Res.-Atmos., 120, 2015JD023161, https://doi.org/10.1002/2015JD023161, 2015.
Comstock, K. K., Bretherton, C. S., and Yuter, S. E.: Mesoscale variability and drizzle in southeast Pacific stratocumulus, J. Atmos. Sci., 62, 3792–3807, https://doi.org/10.1175/JAS3567.1, 2005.
Franklin, C. N., Sun, Z., Bi, D., Dix, M., Yan, H., and Bodas-Salcedo, A.: Evaluation of clouds in access using the satellite simulator package COSP: regime-sorted tropical cloud properties, J. Geophys. Res.-Atmos., 118, 6663–6679, https://doi.org/10.1002/jgrd.50496, 2013.
Grosvenor, D. P. and Wood, R.: The effect of solar zenith angle on MODIS cloud optical and microphysical retrievals within marine liquid water clouds, Atmos. Chem. Phys., 14, 7291–7321, https://doi.org/10.5194/acp-14-7291-2014, 2014.
Haynes, J. M., Marchand, R. T., Luo, Z., Bodas-Salcedo, A., and Stephens, G. L.: A multi-purpose radar simulation package: QuickBeam, B. Am. Meteorol. Soc., 88, 1723–1727, 2007.
Haynes, J. M., L'Ecuyer, T. S., Stephens, G. L., Miller, S. D., Mitrescu, C., Wood, N. B., and Tanelli, S.: Rainfall retrieval over the ocean with spaceborne W-band radar, J. Geophys. Res.-Atmos., 114, D00A22, https://doi.org/10.1029/2008JD009973, 2009.
Hillman, B. R., Marchand, R. T., and Ackerman, T. P.: Sensitivities of simulated satellite views of clouds to subgrid-scale overlap and condensate heterogeneity, J. Geophys. Res.-Atmos.,https://doi.org/10.1029/2017jd027680, accepted, 2018.
Jing, X., Suzuki, K., Guo, H., Goto, D., Ogura, T., Koshiro, T., and Mülmenstädt, J.: A multimodel study on warm precipitation biases in global models compared to satellite observations, J. Geophys. Res.-Atmos., 122, 11806–11824, https://doi.org/10.1002/2017JD027310, 2017.
Kato, S. and Marshak, A.: Solar zenith and viewing geometry dependent errors in satellite retrieved cloud optical thickness: Marine Sccase, J. Geophys. Res.-Atmos., 114, D01202, https://doi.org/10.1029/2008JD010579, 2009.
Kay, J. E., Hillman, B. R., Klein, S. A., Zhang, Y., Medeiros, B., Pincus, R., Gettelman, A., Eaton, B., Boyle, J., Marchand, R., and Ackerman, T. P.: Exposing global cloud biases in the community atmosphere model (CAM) using satellite observations and their corresponding instrument simulators, J. Climate, 25, 5190–5207, https://doi.org/10.1175/JCLI-D-11-00469.1, 2012.
Kay, J. E., L'Ecuyer, T., Chepfer, H., Loeb, N., Morrison, A., and Cesana, G.: Recent Advances in Arctic Cloud and Climate Research, Current Climate Change Reports, 2, 159–169, 2016.
Khairoutdinov, M. F. and Randall, D. A.: Cloud resolving modeling of the ARM summer 1997 IOP: Model formulation, results, uncertainties, and sensitivities, J. Atmos. Sci., 60, 607–625, 2003.
Khairoutdinov, M., Randall, D., and DeMott, C.: Simulations of the atmospheric general circulation using a cloud-resolving model as a superparameterization of physical processes, J. Atmos. Sci., 62, 2136–2154, 2005.
Klein, S. A. and Jakob, C.: Validation and sensitivities of frontal clouds simulated by the ECWMF model, Mon. Weather Rev., 127, 2514–2531, https://doi.org/10.1175/1520-0493(1999)127<2514:VASOFC>2.0.CO;2, 1999.
Kubar, T. L. and Hartmann, D. L.: Understanding the importance of microphysics and macrophysics for warm rain in marine low clouds. Part I: Satellite observations, J. Atmos. Sci., 66, 2953–2972, https://doi.org/10.1175/2009JAS3071.1, 2009.
Lebsock, M. and Su, H.: Application of active spaceborne remote sensing for understanding biases between passive cloud water path retrievals, J. Geophys. Res.-Atmos., 119, 8962–8979, https://doi.org/10.1002/2014JD021568, 2014.
Lebsock, M., Morrison, H., and Gettelman, A.: Microphysical implications of cloud-precipitation covariance derived from satellite remote sensing, J. Geophys. Res.-Atmos., 118, 6521–6533, https://doi.org/10.1002/jgrd.50347, 2013.
L'Ecuyer, T. S. and Stephens, G. L.: An estimation-based precipitation retrieval algorithm for attenuating radars, J. Appl. Meteorol., 41, 272–285, 2002.
Ma, P.-L., Rasch, P. J., Wang, H., Zhang, K., and Easter, R. C.: The role of circulation features on black carbon transport into the Arctic in the Community Atmosphere Model version 5 (CAM5), J. Geophys. Res.-Atmos., 118, 4657–4669, https://doi.org/10.1002/jgrd.50411, 2013.
Ma, P.-L., Rasch, P. J., Wang, M., Wang, H., Ghan, S. J., Easter, R. C., Gustafson Jr., W. I., Liu, X., Zhang, Y., and Ma, H.-Y.: How does increasing horizontal resolution in a global climate model improve the simulation of aerosol-cloud interactions?, Geophys. Res. Lett., 42, 5058–5065, https://doi.org/10.1002/2015GL064183, 2015.
Marchand, R., Mace, G. G., Ackerman, T., and Stephens, G.: Hydrometeor detection using Cloudsat – An earth-orbiting 94-GHz cloud radar, J. Atmos. Ocean. Technol., 25, 519–533, https://doi.org/10.1175/2007JTECHA1006.1, 2008.
Marchand, R., Haynes, J., Mace, G. G., Ackerman, T., and Stephens, G.: A comparison of simulated cloud radar output from the multiscale modeling framework global climate model with CloudSat cloud radar observations, J. Geophys. Res.-Atmos., 114, D00A20, https://doi.org/10.1029/2008JD009790, 2009.
Morrison, H. and Gettelman, A.: A new two-moment bulk stratiform cloud microphysics scheme in the community atmosphere model, version 3 (CAM3). Part I: Description and numerical tests, J. Climate, 21, 3642–3659, 2008.
Morrison, H., Curry, J. A., and Khvorostyanov, V. I.: A new double-moment microphysics parameterization for application in cloud and climate models. Part I: Description, J. Atmos. Sci., 62, 1665–1677, 2005.
Nam, C. and Quaas, J.: Evaluation of clouds and precipitation in the ECHAM5 general circulation model using CALIPSO and CloudSat satellite data, J. Climate., 25, 4975–4992, https://doi.org/10.1175/JCLI-D-11-00347.1, 2012.
Neale, R. B., Collins, W. D., Rasch, P. J., Boville, B. A., Hack, J. J., McCaa, J. R., Williamson, D. L., Kiehl, J. T., and Briegleb, B.: Description of the NCAR community atmosphere model (CAM 5.0), Tech. Rep. TN–486+STR, 268 pp., Natl. Cent. for Atmos. Res., Boulder, Colo., 2010.
Pincus, R., Platnick, S., Ackerman, S. A., Hemler, R. S., and Hofmann, P.: Reconciling simulated and observed views of clouds: MODIS, ISCCP, and the limits of instrument simulators, J. Climate, 25, 120220120058001, https://doi.org/10.1175/JCLI-D-11-00267.1, 2012.
Platnick, S., Meyer, K. G., King, M. D., Wind, G., Amarasinghe, N., Marchant, B., Arnold, G. T., Zhang, Z., Hubanks, P. A., Holz, R. E., Yang, P., Ridgway, W. L., and Riedi, J.: The MODIS cloud optical and microphysical products: Collection 6 updates and examples from Terra and Aqua, IEEE T. Geosci. Remote, 55, 502–525, https://doi.org/10.1109/TGRS.2016.2610522, 2017.
Randall, D., Khairoutdinov, M., Arakawa, A., and Grabowski, W.: Breaking the cloud parameterization deadlock, B. Am. Meteorol. Soc., 84, 1547–1564, 2003.
Randall, D., Branson, M., Wang, M., Ghan, S., Craig, C., Gettelman, A., and Edwards, J.: A community atmosphere model with superparameterized clouds, Eos Trans. AGU, 94, 221–222, 2013.
Song, H., Zhang, Z., Ma, P.-L., Ghan, S., and Wang, M.: An Evaluation of Marine Boundary Layer Cloud Property Simulations in Community Atmosphere Model Using Satellite Observations: Conventional Sub-grid Parameterization vs. CLUBB, J. Climate, 31, 2299–2320, 2018.
Stephens, G. L., Vane, D. G., Boain, R. J., Mace, G. G., Sassen, K., Wang, Z., Illingworth, A. J., O'connor, E. J., Rossow, W. B., Durden, S. L., Miller, S. D., Austin, R. T., Bendetti, A., Mitrescu, C., and the CloudSat Science Team: The CloudSat Mission and the A-Train, B. Am. Meteorol. Soc., 83, 1771–1790, https://doi.org/10.1175/BAMS-83-12-1771, 2012.
Stevens, B., Vali, G., Comstock, K., Woods, R., Van Zanten, M. C., Austin, P. H., Bretherton, C. S., and Lenschow, D. H.: Pockets of open cells and drizzle in marine stratocumulus, B. Am. Meteorol. Soc., 86, 51–57, 2005.
Suzuki, K., Stephens, G., Bodas-Salcedo, A., Wang, M., Golaz, J.-C., Yokohata, T., and Koshiro, T.: Evaluation of the warm rain formation process in global models with satellite observations, J. Atmos. Sci., 72, 3996–4014, https://doi.org/10.1175/JAS-D-14-0265.1, 2015.
Swales, D. J., Pincus, R., and Bodas-Salcedo, A.: The Cloud Feedback Model Intercomparison Project Observational Simulator Package: Version 2, Geosci. Model Dev., 11, 77–81, https://doi.org/10.5194/gmd-11-77-2018, 2018.
Takahashi, H., Lebsock, M., Suzuki, K., Stephens, G., and Wang, M.: An investigation of microphysics and subgrid-scale variability in warm-rain clouds using the A-Train observations and a multiscale modeling framework, J. Geophys. Res.-Atmos., 122, 7493–7504, 2017.
Tanelli, S., Durden, S. L., Im, E., Pak, K. S., Reinke, D. G., Partain, P., Haynes, J. M., and Marchand, R. T.: CloudSat's Cloud Profiling Radar After Two Years in Orbit: Performance, Calibration, and Processing, IEEE T. Geosci. Remote, 46, 3560–3573, 2008.
Tao, W. K., Chern, J., Atlas, R., Randall, D., Lin, X., Khairoutdinov, M., Li, J.-L., Waliser, D. E., Hou, A., Peters-Lidard, C., Lau, W., and Simpson, J.: A multiscale modeling system developments, applications, and critical issues, B. Am. Meteorol. Soc., 90, 515–534, 2009.
Wang, M., Ghan, S., Easter, R., Ovchinnikov, M., Liu, X., Kassianov, E., Qian, Y., Gustafson Jr., W. I., Larson, V. E., Schanen, D. P., Khairoutdinov, M., and Morrison, H.: The multi-scale aerosol-climate model PNNL-MMF: model description and evaluation, Geosci. Model Dev., 4, 137–168, https://doi.org/10.5194/gmd-4-137-2011, 2011.
Wang, M., Larson, V., Ghan, S., Ovchinnikov, M., Schanen, D., Xiao, H., Liu, X., Guo, Z., and Rasch, P.: A multiscale modeling framework model (superparameterized CAM5) with a higher-order turbulence closure: Model description and low-cloud simulations, J. Adv. Model. Earth Syst., 7, 484–509, https://doi.org/10.1002/2014MS000375, 2015.
Webb, M., Senior, C., Bony, S., and Morcrette, J.: Combining ERBE and ISCCP data to assess clouds in the Hadley Centre, ECMWF and LMD atmospheric climate models, Clim. Dynam., 17, 905–922, 2001.
Wood, R.: Drizzle in stratiform boundary layer clouds. Part I: Vertical and horizontal structure, J. Atmos. Sci., 62, 3011–3033, 2005.
Zhang, K., Wan, H., Liu, X., Ghan, S. J., Kooperman, G. J., Ma, P.-L., Rasch, P. J., Neubauer, D., and Lohmann, U.: Technical Note: On the use of nudging for aerosol–climate model intercomparison studies, Atmos. Chem. Phys., 14, 8631–8645, https://doi.org/10.5194/acp-14-8631-2014, 2014.
Zhang, Y., Klein, S. A., Boyle, J., and Mace, G. G.: Evaluation of tropical cloud and precipitation statistics of Community Atmosphere Model version 3 using CloudSat and CALIPSO data, J. Geophys. Res.-Atmos., 115, D12205, https://doi.org/10.1029/2009JD012006, 2010.