the Creative Commons Attribution 4.0 License.
the Creative Commons Attribution 4.0 License.
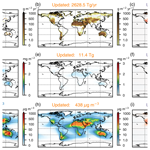
An updated aerosol simulation in the Community Earth System Model (v2.1.3): dust and marine aerosol emissions and secondary organic aerosol formation
Yujuan Wang
Peng Zhang
Jie Li
Yaman Liu
Jiawei Li
Zhiwei Han
Aerosols constitute important substance components of the Earth's atmosphere and have a profound influence on climate dynamics, radiative properties, and biogeochemical processes. Here we introduce updated emission schemes for dust, sea-salt, and marine primary organic aerosols (MPOA), as well as augmented secondary organic aerosol (SOA) formation pathways within the Community Earth System Model (CESM; version 2.1.3). The modified dust emission scheme shifts the original hotspot-like dust emission to a more continuous distribution, improving the dust aerosol optical depth (DAOD) simulations at stations in north Africa and central Asia. This update also reduces dust residence time from 4.1 to 1.6 d, enhancing concentration simulations downwind of dust source regions. For sea-salt emissions, we incorporate an updated sea surface temperature (SST) modulation and introduce a relative-humidity-dependent correction factor for sea-salt particle size, with SST having a significantly larger impact on sea-salt emissions (16.1 %) compared to the minor effect of humidity (−0.3 %). We then extend our modelling to incorporate emissions of marine primary organic aerosols (MPOA) as mixed externally with sea-salt aerosols, coupled offline with the ocean component Parallel Ocean Program (POP2). The results underscore the substantial influence of phytoplankton diversity on MPOA emissions, with 148 % variability simulated among different phytoplankton types, highlighting the role of biological variability in aerosol modelling. Furthermore, we refine the model's chemical mechanisms by including the irreversible aqueous uptake of dicarbonyl compounds as a new pathway for SOA formation, contributing an additional 37 % to surface SOA concentrations. These improvements enrich the ability of the CESM to use intricate linkages between different components of the Earth system, thereby enabling a more comprehensive description of natural aerosol emissions, chemical processes, and their impacts.
- Article
(14969 KB) - Full-text XML
-
Supplement
(4346 KB) - BibTeX
- EndNote
Aerosols play a critical role in shaping Earth's energy budget and atmospheric properties (Dickerson et al., 1997). Intercomparison among AeroCom (Aerosol Comparisons between Observations and Models intercomparison project) models indicates that the highest emissions among aerosol species are from sea-salt aerosols, with dust aerosols following as the second-largest contributor (Textor et al., 2006). In terms of aerosol mass burden, dust aerosols are the most dominant, constituting approximately 75 % of the total atmospheric aerosol burden (Kok et al., 2021a). These aerosols from natural sources stand out as key components due to their abundance and distribution throughout the Earth's atmosphere. While secondary organic aerosols (SOA) form through atmospheric chemical reactions rather than direct emissions, they constitute a major component of fine particulate matter, profoundly impacting the climate and human health (Heal et al., 2012; Jimenez et al., 2009).
Dust aerosols, primarily originating from arid and semi-arid regions, are aerosols with size distributions that are highly variable over time and space (Mahowald et al., 2014; Tegen and Lacis, 1996). Emitted into the atmosphere through wind erosion processes, dust aerosol emissions display significant spatial and temporal variability spanning multiple orders of magnitude and are sensitive to climate and land-cover and land-use change (Kok et al., 2021b; Mahowald et al., 2006). Transported across continents and oceans, dust aerosols play a critical role in various Earth system processes (Kok et al., 2023), including air quality and population health (Mallone et al., 2011), cloud condensation nuclei (CCN) and ice nuclei (IN) formation (Koehler et al., 2009; Murray et al., 2012), radiation absorption and scattering (Kok et al., 2017), and nutrient deposition in oceans (Schroth et al., 2009). However, accurate observations to quantify dust emissions and their three-dimensional distribution remain challenging. Robust modelling approaches are therefore needed to simulate the global dust cycle, particularly regarding the initial emission processes, to enhance our understanding of their impacts on the Earth system.
Sea-salt aerosols, generated through the breaking of sea waves, constitute a substantial fraction of atmospheric aerosols in and around oceans. Locally, sea-salt aerosols can affect the microphysical characteristics of ocean clouds (Platnick and Twomey, 1994), the intensity of tropical cyclones (Jiang et al., 2019), and even El Niño variability (Yang et al., 2016). Expanded to industrialized regions, investigations suggest the potential for sea-salt aerosols to moderate the direct radiative forcing of anthropogenic aerosols (Chen et al., 2020; Liao and Seinfeld, 2005). Yet, due to the scarcity of comprehensive measurement of the spatial and temporal evolution of sea-salt emissions, emission estimation mainly relies on model simulations. As sea-salt aerosol emissions vary with ocean surface conditions (e.g. wind speed, seawater temperature, and ambient humidity), it is necessary to incorporate these influencing factors into parameterization schemes (Lewis and Schwartz, 2004). Here, we seek to improve representation of dust and sea-salt aerosols through updates to the foundational portrayal and modification terms in emission schemes.
While it is generally sea-salt aerosols that dominate the overall mass loading of sea spray aerosols, the biogenic organic fraction, known as marine primary organic aerosols (MPOA), has also been found to make up a significant portion of the submicron aerosol mass concentrations during plankton bloom periods (O'Dowd et al., 2004). Given the complexity of refining the parameterization of the organic content of the sea surface microlayer at the sea–air interface (Burrows et al., 2014), many recent MPOA emission schemes are built on sea surface chlorophyll a concentration (Chl a) as an indicator of the organic mass fraction of sea spray aerosols (Gantt et al., 2009; O'Dowd et al., 2008; Rinaldi et al., 2013). This correlates with the use of Chl a as a proxy for marine phytoplankton biomass (Cullen et al., 1982). Previous studies have utilized the global satellite-retrieved observation record of Chl a (Gantt et al., 2012; Meskhidze et al., 2011; Zhao et al., 2021). Here we exploit the strength of multi-sphere modelling in Earth system models to employ an ocean biogeochemistry model in the simulation of MPOA emissions. The influence of different phytoplankton functional types can also be explored (Langmann et al., 2008; Roelofs, 2008; Spracklen et al., 2008).
Secondary organic aerosol, as a major component of global submicron atmospheric organic aerosol, is formed by the oxidation of anthropogenic and biogenic volatile organic compounds (VOCs; Kanakidou et al., 2005; Tsigaridis et al., 2014; Shrivastava et al., 2017). Its formation and subsequent dispersion affect air quality, climate forcing, and human health on a global scale (Hallquist et al., 2009). In addition, previous studies have shown that SOA plays a significant role in the regional occurrence of severe haze pollution events (Huang et al., 2014; Li et al., 2021). Constrained by the complexity of the chemical composition and formation processes of SOA, its representation in atmospheric chemistry models varies, from the simplified approach using prescribed SOA emissions based on proportional values of precursor emissions (Chin et al., 2002; Colarco et al., 2010) to the advanced volatility basis set (VBS) approach (Donahue et al., 2006; Hodzic et al., 2016). Due to the uncertainties and limited knowledge, current model simulations and SOA observations are still highly uncertain (Tsigaridis et al., 2014). Li et al. (2021) found that the aqueous uptake of dicarbonyls is an important pathway for the formation of SOA, especially during haze events. Here, we include this new pathway in the chemical mechanism to investigate its impact on SOA formation.
This study focuses on improving the representation of atmospheric aerosols based on the conceptualization of CoAerM (the Common Aerosol Module), which is derived from previous work (Han et al., 2004; Li et al., 2021, 2024; Li and Han, 2016). The modifications encompass natural aerosol emissions such as dust, sea-salt, and MPOA, as well as the formation of SOA, within the framework of the Community Earth System Model (CESM). The CESM is a comprehensive framework comprising sophisticated atmosphere, ocean, land, sea-ice, land-ice, runoff, and wave model components (Danabasoglu et al., 2020). This framework provides an expansive suite of options for configuring model components and physical parameterizations, enabling simulations of atmospheric composition changes and aerosol behaviours interacting with intricate elements in the Earth system. The updated aerosol schemes we develop are embedded into the atmospheric component of the CESM version 2.1.3, the Community Atmosphere Model (version 6 with chemistry; CAM6-chem). More specifically, we employ an integration of the Community Land Model (version 5; CLM5) and Parallel Ocean Program (POP2). The effects of the modified schemes and the sensitivity to specific changes are then described and compared. We organize the paper as follows: Sect. 2 presents the overall methodology, including detailed descriptions of the modifications to schemes and sources of measurements and satellite retrievals. Section 3 evaluates simulated emissions and concentrations through comparison with observations and examines individual effects of scheme modifications. Summarized conclusions are provided in Sect. 4.
2.1 Modifications to the schemes
2.1.1 Dust emission scheme
Vertical dust flux
Online calculated dust emissions from wind erosion generally depend on the wind shear stress near the land surface and combine with characterizations of land surface properties, including vegetation cover, soil properties, and surface roughness. These parameterizations are either based on experiments (Gillette and Passi, 1988; Marticorena and Bergametti, 1995) or derived from microphysical processes (Shao, 2004). The standard dust emission scheme in CAM6-chem follows the Dust Entrainment and Deposition (DEAD) model (Zender et al., 2003a), which is a semi-empirical expression. The total vertical dust flux (Fdust,j) emitted into size bin j is modelled from the initial vertical-emission flux calculated in CLM5 (φCLM,j) when the friction velocity (u∗s) exceeds the threshold friction velocity (u∗t):
Here T is a global tuning factor; S is an empirical geomorphic dust source function, also referred to as the soil erodibility factor; fb represents the bare-soil fraction of the grid cell suitable for dust entrainment; α is the sandblasting mass efficiency as a function of soil clay content; the saltation constant cs is set to 2.61; ρa is the air density; g is the gravitational acceleration; and χi,j is the mass proportion from each source mode i transported to the bin j. Note that friction velocity (u∗s) already accounts for the increase in friction velocity caused by the saltation process, known as Owen's effect (Owen, 1964). We modify the power law relationship calculated in CLM5 based on Gillette and Passi (1988):
where CGP is the calibration factor from the Gillette–Passi scheme (set to . It should be noted that in all equations, the prime symbol ′ denotes the variables in the modified schemes.
Surface roughness correction factor
Threshold friction velocity (u∗t) represents the minimum velocity at which dust particles begin to move and is commonly expressed as
where u∗t0 is the ideal threshold friction velocity, which depends on optimal-saltation soil particles (Dosp), and CFw and CFd are correction factors (CF) introduced to account for the soil moisture inhibition effect and the drag partition effect on u∗t, respectively. In CAM6-chem-DEAD, the soil moisture CF adopts the parameterization of Fécan et al. (1999), but the drag partition CF is inactive. We activate the drag partition parameterization (Darmenova et al., 2009; Marticorena and Bergametti, 1995; Tian et al., 2021) in this updated scheme to include the effect of non-erodible roughness elements. is expressed as
where z0 and z0s are aerodynamic roughness length and smooth roughness length.
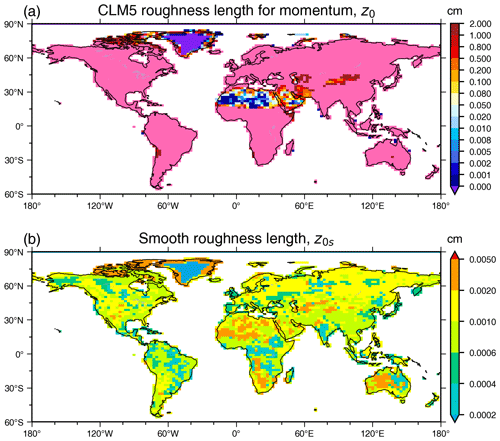
Figure 1Aerodynamic roughness length z0 (a) and smooth roughness length z0s (b) used in the updated dust emission schemes.
The aerodynamic roughness length (z0) refers to the roughness length of the exposed ground including the nonerodible elements, which dissipate part of the wind momentum for soil particle saltation. Zender et al. (2003a) used a globally constant value of 0.01 cm for z0. In this work, we choose to use the “patch roughness length over vegetation, momentum” computed natively in CLM5. As shown in Fig. 1a, the updated z0 range is visibly larger in comparison to the constant 0.01 cm.
The smooth roughness length (z0s) is defined as the roughness length of the uncovered ground, that is, the roughness length of the potentially erodible portion of the ground with any nonerodible elements removed. Zender et al. (2003a) used a globally constant value of 0.0033 cm. This was estimated from a relation to the soil texture (Marticorena and Bergametti, 1995) when assuming the area mean diameter of particles to be 0.1 cm:
Here Dp,dust is the underlying particle size. In this work, we introduce the geographic variability in Dp,dust. We first categorize the soil texture in the model according to the United States Department of Agriculture (USDA) soil textural triangle (USDA, 2023) based on the CLM-provided clay and sand content of the underlying soil. The resulting distribution is shown in Fig. S1. Corresponding to the soil aggregate particle size distribution of different texture classifications, Dp,dust can be represented as the median diameter of the coarse mode (Mokhtari et al., 2012; Tian et al., 2021). z0s can then be calculated from Eq. (6) (Fig. 1b). Following the application of the surface roughness correction factor, the simulated threshold friction velocity exhibits comparable changes, with variations that differ across different regions (Fig. S2).
Vegetation effects
The original scheme employs fb to define the possible regions for wind erosion, which is expressed as
where flake and fsnow are the lake and snow cover fraction of a grid cell; fliq is the soil liquid water fraction in the top layer; and LAI is short for total leaf area index, which is used to indicate the inhibiting effect of vegetation cover on dust emissions. The threshold LAI, LAIt, is set to 0.3, above which dust emissions are assumed to be none (Mahowald et al., 2006). Yet experiments show that dust emissions could occur with high vegetation cover (Okin, 2008). In this work, we employ the reduction factors (RFs) of different vegetation cover types to characterize the vegetation effects (Han et al., 2004; Park and In, 2003). Thus, the modified expression of is
where PFT is short for plant functional type. fpft and RFpft are the fraction of the grid cell and the reduction factor of the ith type of PFT prescribed from CLM5. To include all the effects of PFTs within a land unit, we do the sum at this subgrid scale. We show the seasonal variability in the updated in Fig. 2. It is clear that the primary dust source regions around the globe exhibit relatively high values, indicating areas susceptible to wind erosion. Furthermore, regions with distinct seasonal variations are concentrated in central and East Asia, particularly during boreal winter (DJF) when reaches its lowest values in these regions.
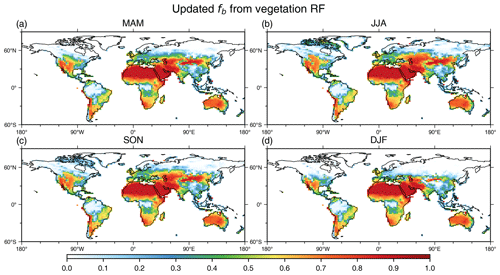
Figure 2Seasonal mean of updated calculated based on vegetation reduction effects: (a) March–April–May (MAM), (b) June–July–August (JJA), (c) September–October–November (SON), and (d) December–January–February (DJF). The units are dimensionless.
The original scheme also uses an empirical source function generated from geomorphic information of dust provenance to shape the distribution of dust emissions toward the so-called preferential regions (Albani et al., 2014; Mahowald et al., 2006; Zender et al., 2003b). However, the application of the source function has been found to underpredict the dust emission in East Asia, as dust source regions are not well characterized (Wu et al., 2021, 2019). Besides, the constraint of the source function on the modelling of dust emission suffers from the characteristics of the hotspot-like discrete distribution (Fig. S3). Here we integrate land use data to modify the spreads in dust source regions. Dust emissions are allowed on barren ground and in areas with some shrubs or low grass.
Mode mapping
Subsequent to the computation of the total dust flux in CLM5, CAM6-chem allocates the emitted dust aerosols in the aerosol module. In this study, the four-mode version of the Modal Aerosol Module (MAM4; Liu et al., 2016) is used. Dust aerosols are distributed into three modes: an Aitken mode, an accumulation mode, and a coarse mode, with emission diameter ranges of 0.01–0.1, 0.1–1.0, and 1.0–10.0 µm, respectively. The original prescribed emission mass proportion is derived from the “brittle fragmentation” theory (Kok, 2011), allocating fractions of 0.00165 %, 1.1 %, and 98.9 % to the three modes, respectively. Here we modify the apportioning fractions of the accumulation mode and coarse mode according to the observed mass-size distribution of vertical dust flux in major Chinese source regions (Han et al., 2004) to 14 % and 86 %, respectively. The mass fractions for the two modes are determined using the weighted mean distributions and mode diameter ranges (Li and Han, 2016).
2.1.2 Sea-salt emission scheme
Emissions of sea-salt aerosols are mostly modelled according to the mechanism of the bursting of whitecap bubbles entrained in breaking waves caused by surface wind. In the default emission scheme in CAM6-chem, quantification of emitted sea-salt particles with a dry-particle radius (rdry) range of 1.4–5 µm is thus expressed as a source density function dependent on wind speed drawn from laboratory observations (Monahan et al., 1986):
where r80 is the sea-salt particle radius at 80 % ambient relative humidity (RH; ∼1.814rdry), U10 is the 10 m wind speed, and . For sea-salt particles with rdry ranging from 0.01 to 1.4 µm, a scheme that considers the impact of sea surface temperature (SST) on whitecap cover is used (Mårtensson et al., 2003). The source density flux is expressed as
where the whitecap cover (fraction) is , Ddry is the dry-particle diameter, and T is the SST (°C). [ak(Ddry)T+bk(Ddry)] is a polynomial term representing the SST effects with coefficients that vary with particle size. It was determined by laboratory experiments on synthetic seawater.
In this work, the sea-salt emission scheme is refined by incorporating two key modifications. First, the dependence of the source function on SST is updated using an empirical parameterization derived from cruise measurements (Jaeglé et al., 2011). We also employ an extended source function, which has been optimized for particles with a radius under 0.1 µm (Gong, 2003). The modified source density function is as follows:
where and .
Second, an RH-dependent sea-salt particle size correction factor is introduced, representing the influence of ambient humidity on sea-salt emission (Zhang et al., 2005, 2006). As previously described, sea-salt source functions are mostly presented in the form of r80-based, and r80 is usually set as a multiple of rdry. By incorporating the information on ambient RH, the source function can be expressed as
where C80 is the correction term.
2.1.3 Implementing MPOA emission into the CESM
In the MAM4 module, the fourth aerosol mode is a primary carbon mode used to investigate the ageing of primary carbonaceous aerosols (Liu et al., 2016). Aerosol species of black carbon and primary organic matter (POM) are emitted in primary carbon mode and then aged in the accumulation mode. The original emission setting, however, referred to primary organic aerosols emitted in the primary carbon mode (denoted “pom_a4”) as terrestrial sources. These sources typically encompass terrestrial biomass burning and fossil fuel and biofuel combustion. In this study, we implement MPOA emissions internally mixed with sea salt in the accumulation modes. This configuration has been validated to be in best agreement with observations among different assumptions of mixing state and changes in amount (Burrows et al., 2022). We calculate MPOA emissions from the organic mass fraction of sea spray aerosols (OMSSA) and updated sea-salt mass emissions (:
Parameterization of OMSSA is related to Chl a and 10 m wind speed following Gantt et al. (2011). The formula is expressed as
The majority of previous modelling research on MPOA used satellite-based observational Chl a concentrations (Gantt et al., 2012; Wang et al., 2020; Zhao et al., 2021; Li et al., 2024). In this study, model-simulated Chl a will be used instead.
2.1.4 Offline coupling with the ocean biogeochemical component
To integrate modelled Chl a results in the MPOA emission scheme, our modification involves coupling with the Marine Biogeochemistry Library (MARBL) embedded in the ocean component POP2. MARBL functions as a prognostic ocean biogeochemistry model, facilitating the simulation of marine ecosystem dynamics and cycling of essential elements, including carbon and nitrogen (Long et al., 2021). It offers the capacity for adaptable ecosystem configurations of varying complexity by allowing modifications to phytoplankton and zooplankton functional types. In this study, we preserve the ecosystem configuration in the MARBL-CESM2, which explicitly includes three phytoplankton functional groups (diatoms, diazotrophs, and “small” pico and nano phytoplankton) and one zooplankton group.
Considering computational efficiency, we use the offline approach to drive the MPOA emission calculation with the surface Chl a of the three phytoplankton functional groups derived from the pre-processed POP2 run. We set up an ocean biogeochemistry run with the standard ocean component set of the CESM2 (G-component set). This setup includes active POP2 and the sea-ice component, together with a data atmosphere and stub land component. The simulation is conducted at approximately 1° resolution from the year 2000 to the year 2012, with the first 10 years as spin-up time and the last 3 years for regridding. Chl a data are read directly during run time in CAM6-chem from the netCDF-format files with a monthly interval.
2.1.5 SOA formation via aqueous reaction
The default SOA parameterization in CAM6-chem uses the VBS scheme, which groups the precursors of SOA into five bins by volatility, with saturation concentrations ranging from 0.01 to 100 µg m−3 at 300 K (Tilmes et al., 2019). CAM6-chem explicitly simulates nine types of SOA precursors that oxidize mainly by OH, O3, and NO3 to form gas-phase semivolatile condensable sources of SOA (see Table 1 in Tilmes et al., 2019). However, the irreversible aqueous uptake of dicarbonyls (mainly glyoxal and methylglyoxal) has not been integrated into the chemical mechanism, and this process contributes significantly to the formation and the total burden of SOA (Fu et al., 2008, 2009; McNeill et al., 2012; Li et al., 2021). We add the irreversible uptake of dicarbonyl gases (glyoxal and methylglyoxal) by aqueous particles in the model. The uptake rate k is given by
where r is the particle radius, Dg is the gas-phase molecular diffusion coefficient, ν is the mean gas molecular speed, γ is the reactive uptake coefficient, and A is the aerosol surface area. The reactive uptake coefficient for glyoxal and methylglyoxal is adopted as following Li et al. (2021).
2.2 Model configurations and experiments
We run the CESM2 with the following configurations. To reproduce more-realistic meteorological conditions, we use the FCSD (https://docs.cesm.ucar.edu/models/cesm2/config/2.1.3/compsets.html) component set, which couples CAM6-chem with active land and sea-ice components, as well as the data ocean and slab land ice components in all simulations. CAM6-chem is configured to run with the finite volume dynamical core and troposphere/stratosphere chemistry. Anthropogenic emissions of other aerosols and precursors are from the Climate Model Intercomparison Program (CMIP6) historical inventory (Eyring et al., 2016). The meteorological nudging applies to air temperature, relative humidity, and horizontal wind components using the Modern-Era Retrospective Analysis for Research and Applications version 2 atmospheric forcing dataset (MERRA-2; Atmospheric Chemistry Observations & Modeling/National Center for Atmospheric Research/University Corporation for Atmospheric Research, and Climate and Global Dynamics Division/National Center for Atmospheric Research/University Corporation for Atmospheric Research, 2018) with a 6 h relaxation. The accuracy of the modelled wind speeds is decisive for all aerosol emissions involved in this study. Sea surface temperature (SST) and sea-ice cover fields are prescribed from historical data.
We consider a “CYCLE” simulation set, simulating from the year 2009 to the year 2012, with the 1st year as the spin-up period and the last 3 years used for analysis. CAM6-chem and CLM5 are executed at a horizontal resolution of 1.9° × 2.5° in latitude and longitude and a vertical resolution of 32 levels. The simulation set consists of a control run that implements the default emission schemes and another run incorporating all the aforementioned modifications. The results are analysed with a focus on the global scale, aiming to capture the stable state rather than abrupt perturbations.
A case study of dust events in East Asia during March 2021 is conducted to evaluate the updated dust emission schemes. This simulation set employs a finer grid with a resolution of 0.9° latitude × 1.25° longitude since the analysis is focused on the regional scale. In this case, the simulation period spans from 1 January 2021 to 1 April 2021 with a spin-up time of more than 2 months.
A set of sensitivity experiments was done to evaluate the dependence of the sea-salt aerosol emission schemes on two major modifications. First, we test the impact of SST correction by comparing the original scheme with simulations using the source function proposed by Gong (2003, referred to as the Gong function hereafter) without SST correction and with the SST correction from Jaeglé et al. (2011). Second, we assess the influence of an RH correction by running simulations with and without the RH-dependent correction factor using the Gong function.
Also, experiments on MPOA emission schemes involve a comparison of MPOA emissions simulated from input Chl a from several different species sources. The SOA experiment includes a control group (default chemical mechanism) and an experimental group (modified chemical mechanism) to investigate the effect of dicarbonyl uptake on SOA formation.
2.3 Observational measurements
2.3.1 Dust aerosol optical depth (DAOD) based on satellite retrievals
We use dust aerosol optical depth (DAOD) data on two timescales in this study for analysis: one is directly calculated from MODIS/Aqua gridded products and the other is a climatological dataset of global DAOD derived from MODIS aerosol retrievals (Song et al., 2021a).
To facilitate comparison with the model results in the dust event case, we apply the formula proposed by Pu and Ginoux (2018), combining the Ångström exponent (α) and single-scattering albedo (ω) to calculate the daily DAOD from the MODIS/Aqua level 3 atmosphere daily joint product (MYD08_D3, collection 6.1). The MYD08_D3 product provides 1° × 1° grid values for atmospheric aerosol particle parameters (e.g. aerosol optical depth, abbreviated as AOD; α,;and ω) retrieved using the Deep Blue aerosol algorithm.
Regarding the mean state of the dust cycle, we compare the model results with the globally aggregated monthly mean DAOD dataset. This climatological dataset is also derived from MODIS/Aqua satellite retrievals, with a spatial resolution of 1° and temporal coverage from 2003 to 2019 (Song et al., 2021a).
2.3.2 Coarse-mode AOD from AERONET stations
We also use the coarse-mode AOD (CAOD) from the ground-based Aerosol Robotic Network (AERONET) measurements (Holben et al., 2001) at selected stations to evaluate the model performance against observations. Monitoring stations located in major dust source regions with data availability of at least 1 full year during the CYCLE simulation periods are considered valid (see Fig. 3, orange dots). To align the AERONET CAOD record with model results of DAOD, we perform interpolation to obtain CAOD at 550 nm using the Ångström exponent from the AERONET level 2 data, ().
2.3.3 Aerosol concentration from EANET stations
To validate the model performance at simulating sea-salt aerosol, in situ ground observations of aerosol concentration in remote coastal areas are collected from EANET (Acid Deposition Monitoring Network in East Asia; EANET, 2023). Locations are shown in Fig. 3. We use the data for the CYCLE simulation period. In addition to the recorded PM10 concentration, the sea-salt aerosol mass concentration is calculated from the ion concentration as (Quinn and Bates, 2005)
2.3.4 MPOA concentration from publications
To validate the MPOA concentration, we use measurements reported in previous publications, which commonly refer to the water-insoluble organic mass (WIOM) fraction of submicron marine aerosols as MPOA. This differentiation is made as the soluble organic fraction represents the more-oxidized portion originating from a secondary source (Rinaldi et al., 2010). Two measurement sites are considered in this paper (Fig. 3). One is Mace Head (53.33° N, 9.90° W), with temporal coverage from January 2002 to June 2009 (Rinaldi et al., 2013). The other is Amsterdam Island (37.80° S, 77.57° E) with temporal coverage from January 2005–November 2007 (Sciare et al., 2009). We apply an organic-mass-to-organic-carbon ratio (OM OC) of 1.4 to align the WIOM measurements with simulated MPOA concentrations.
2.3.5 PM10 concentration from stations
To evaluate the model simulation of dust events in East Asia, ground measurements of PM10 concentrations are collected at stations in China and South Korea. PM10 measurements for Chinese stations are obtained from the China National Environmental Monitoring Centre and data for South Korean stations are obtained from the Air Korea website (Air Korea stations: https://www.airkorea.or.kr/web/sidoQualityCompare?itemCode=10007, last access: 17 March 2024). Daily PM10 concentrations are used. Locations are shown in Fig. 3.
In the following discussion, the evaluation metrics used are Kendall's correlation coefficient (R) and the root-mean-square error (RMSE). Kendall's correlation, which does not assume a specific data distribution, is used to assess the statistical dependence between observed and simulated values. RMSE measures the average error between observations and simulated results.
We discuss the revised dust aerosol emission scheme in Sect. 3.1, where we evaluate the modifications by comparing simulations with measurements, followed by a detailed case study of a significant dust event that occurred in March 2021 in East Asia. In Sect. 3.2, the revised sea-salt emission scheme is evaluated and the impacts of each of the two key modifications for sea-salt aerosols are then investigated. The simulation of the MPOA is evaluated in Sect. 3.3, followed by a discussion of the impact of phytoplankton species on MPOA emissions. The update of the SOA formation scheme is discussed in Sect. 3.4.
3.1 Dust emission scheme
3.1.1 Model evaluation
To align model results more closely with observations, a global tuning factor is often used in global dust simulations. Earlier studies (e.g. Klose et al., 2021; Li et al., 2022) opted to tune global dust emissions to achieve a simulated global annual mean DAOD of approximately 0.03. This is a global-scale constraint proposed based on multiple satellite retrievals combined with modelling analysis (Ridley et al., 2016). The CAM6-chem name list variable (dust_emis_fact) is implemented for our dust tuning. This tuning parameter is influenced by factors such as the dynamical scheme, chemistry scheme, and resolution settings (Li et al., 2022). In our study, we set this parameter to 0.57 for the original simulations and 0.29 for the updated simulations to meet the DAOD constraint mentioned above.
Table 2Global dust emissions and wet depositions in CYCLE simulation sets.

∗ The ratio of wet deposition to total deposition (dry and wet deposition) is listed in parentheses next to the wet deposition.
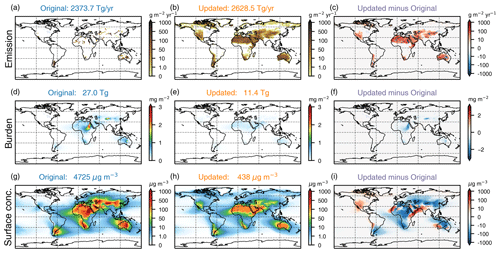
Figure 4Annual mean dust emissions (a–c), burdens (d–f), and surface dust aerosol concentrations (g–i) for the period from 2010 to 2012. Simulation results from the original (a, d, g) and updated (b, e, h) emission schemes are shown, along with the differences between the updated and original schemes (c, f, i). The global total dust emission, global total dust burden, or global maximum surface dust concentration is also given at the top of each panel.
With the modifications applied, we simulate a global annual total dust emission of 2628 Tg, compared to 2374 Tg before implementing these changes (Table 2). Both emission estimates align well within the estimated ranges reported by the AeroCom models (514 to 4313 Tg – Huneeus et al., 2011; 1840±902 Tg – Textor et al., 2006) and those from the CMIP5 models that consider similar size ranges (735 to 8186 Tg), as summarized in Wu et al. (2020). The global annual dust deposition, including both dry and wet depositions, is modelled as 2658 Tg in the updated scheme, compared to 2390 Tg in the original scheme. Wet deposition accounts for 62 % of total deposition in the original simulation and is reduced to 24 % in the updated simulation (Table 2), consistent with the 12 %–39 % range of wet-deposition fractions in CMIP5 models (Wu et al., 2020). The adjustment of apportioning fractions for the accumulation and coarse modes based on observed mass size distribution (Han et al., 2004) results in an increase in annual dust emissions in the accumulation mode that reaches 368 Tg. The global distribution of annual dust emissions, dust burdens, and surface dust aerosol concentrations simulated with the default and modified schemes is presented in Fig. 4. Both the modified and the original schemes capture intense dust emissions from the major dust emission regions globally, with the primary distinction lying in the spatial distribution. The original scheme targets dust emissions to scattered hotspots based on the geomorphic erodibility factor (Fig. S3), while the updated scheme exhibits a more-continuous regional distribution of dust emissions. This is due to the adoption of land use distribution combined with to determine the areas where wind erosion processes are likely to occur (Fig. 2). In major dust-emitting regions, such as north Africa, East Asia, the Middle East, central Asia, Australia, and South America, the updated scheme simulates broader dust emission areas but with lower emissions than the original scheme. In the northwestern part of North America, the updated scheme models larger dust emissions compared to the original scheme (7.6 g m−2 yr−1), with some areas experiencing particularly strong dust emissions (∼100 g m−2 yr−1). It is worth noting that the updated scheme simulates emissions in high-latitude dust (north of 50° N), which is absent in the original scheme. The simulated emissions, mostly below 10 g m−2 yr−1, concentrate in the paraglacial area of the sub-Arctic, consistent with known dust observations and recorded dust storm occurrences in this region (Bullard et al., 2016; Prospero et al., 2012).
Despite the updated scheme leading to more-extensive dust emission coverage, with emissions rising from 2374 to 2628 Tg (an 11 % increase), the global dust burden reduces notably from 27.0 to 11.4 Tg (a 58 % decrease). This reduction is likely attributed to a more-uniform distribution of dust burdens, as the updated emissions are evenly distributed without significant spikes in specific locations. For the distribution of near-surface dust aerosol mass concentrations, the updated scheme simulates lower concentrations in the main dust source regions compared to the original scheme. Additionally, in the areas downwind of certain major deserts, such as the Patagonian Desert in South America, the Nubian Desert in north Africa, the Gobi Desert in East Asia, and in the northern Middle East and Australia, dust aerosols appear to have not spread the same distances after the modification, possibly influenced by the size distribution and deposition processes (Table 2). The overall dust aerosol residence time (the ratio of burden to deposition) is shortened from 4.1 to 1.6 d in the updated scheme, suggesting that dust aerosols are not transported as far as previously thought. Nevertheless, in regions where emissions were augmented in the updated scheme, such as the western Sahara, the Altiplano in South America (Lindau et al., 2021), and the southern part of the Middle East (including the Horn of Africa), dust concentrations increase after the modification. Additionally, the increase in dust emissions simulated by the updated scheme in southwestern North America leads to a substantial simulated dust aerosol concentration, reaching a regional mean of 18 µg m−3. This is close to the seasonal peak measured by a monitoring network in the United States (Hand et al., 2017).
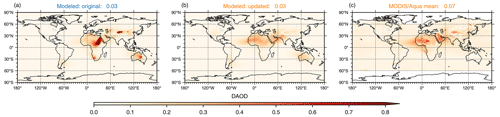
Figure 5Annual mean DAOD from the original (a) and the updated (b) simulation results and MODIS/Aqua observations (c) (Song et al., 2021a) for the period from 2010 to 2012.
In line with the distribution of dust burdens, the updated scheme simulates a smaller DAOD over major dust source regions compared to the original scheme (Fig. 5a and b). Observations from the climatological dataset derived from MODIS/Aqua show the maximum DAOD over the central and western parts of north Africa, the Middle East, and the Taklamakan Desert in East Asia (Fig. 5c). In comparison with the observations, the updated scheme captures the regional maxima of DAOD over central and western north Africa and the Middle East, albeit underestimating them, in particular over central north Africa. The updated scheme also reproduces the DAOD distribution in central and East Asia as the observations, but it tends to overestimate DAOD near the Thar Desert and underestimates DAOD near the Taklamakan Desert. The original scheme, on the other hand, simulates the regional extremes of DAOD in central Australia and southern Africa, which are not as evident in the observations. Overall, the updated scheme provides simulation results for DAOD that are closer to observations than the original scheme.
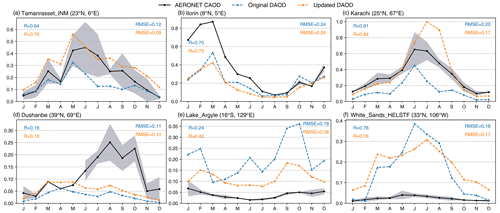
Figure 6The seasonal cycle (a–f) of monthly mean simulated DAOD (coloured lines) and AERONET CAOD (black lines) at selected stations (see Fig. 3). The blue and yellow lines represent the simulations from the original and updated schemes, respectively. The RMSE and R are noted in the corresponding coloured font for each simulation. The shading on the observations illustrates the standard deviation of the monthly mean CAOD over the months with sufficient data. The panels show north Africa (a, b), central Asia (c, d), Australia (e), and North America (f).
We evaluate the month-to-month variability in the modelled DAOD using the CAOD obtained from AERONET measurements. The AERONET CAOD in dusty regions can be used for comparison with DAOD (Pu and Ginoux, 2018). The results presented are from the stations located in the main dust source regions (north Africa, the Middle East and central Asia, Australia, and North America; Fig. 3).
At two stations located close to dust sources, Tamanrasset_INM (north Africa; Fig. 3a), and Karachi (central Asia; Fig. 3c), the original scheme underestimates DAOD compared to the observations but captures the seasonal variability within the year. The metrics of the DAOD time series indicate that the updated simulations exhibit better correlation and smaller RMSEs at these two stations. Especially for the Karachi station, despite the overestimation of the DAOD peak during the summer months of JJA, the updated scheme simulates a DAOD that aligns more notably closely with observations. For sites in downwind regions with some distance from the dust source, Ilorin (north Africa), Dushanbe (central Asia), and White_Sands_HELSTF (North America), the original and updated scheme results are both in better agreement with AERONET for months of the year with less-intense dust but deviate considerably for peak dust months. Finally, at stations where the results of the original schemes differ significantly from observations, such as Lake_Argyle (Australia), the updated scheme shows improved correlation results but still simulates poorly. This discrepancy may be attributed to the coarse resolution of the model, which fails to simulate dust transportation.
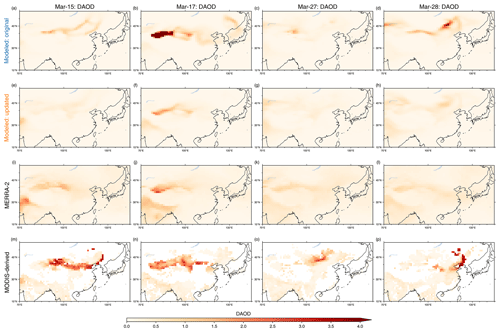
Figure 7Daily mean DAOD in East Asia during the March 2021 dust event. Simulation results from the original (first row) and updated (second row) emission schemes are shown, along with the MERRA-2 reanalysis (third row) and MODIS-derived results (last row). Note that the MODIS DAOD values are calculated according to Sect. 2.3.
3.1.2 Case: East Asia dust events in March 2021
On 15–20 March and 27–29 March 2021, East Asia was hit by two intense dust events (World Meteorological Organization (WMO) news report: https://wmo.int/media/news/severe-sand-and-dust-storm-hits-asia/, last access: 25 February 2024). During both dust events, dust originated in the central Gobi Desert and severely degraded the air quality across most parts of Mongolia and China. In the context of regional analysis of the updated dust emission scheme, a case study is conducted. Figure 7 displays the daily mean DAOD from model results, MERRA-2 reanalysis products, and MODIS-derived results during the dust events. Both the original and updated schemes simulate the presence of large DAOD values in the Gobi Desert and the Taklamakan Desert on 15, 17, and 28 March, consistent with the reanalysis and satellite data. According to the synoptic analysis, the dust impact area in the Taklamakan Desert was confined to the Tarim Basin due to the local easterly wind transport (Gui et al., 2022). The DAOD simulated by the original scheme notably exceeds that of the updated scheme, although the latter aligns more closely with the DAOD values of MERRA-2. The DAOD obtained from MODIS is consistently larger and suffers from discontinuity in this comparison.
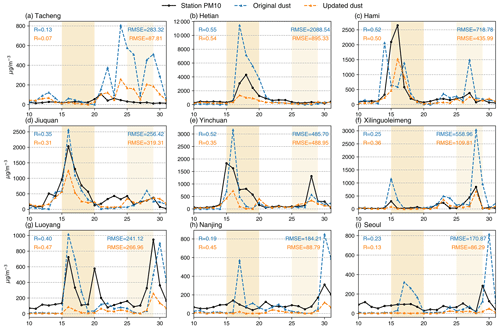
Figure 8Time series (a–i) of daily mean simulated dust aerosol concentrations (coloured lines) and station PM10 concentrations (black lines) at selected stations (i). The blue and yellow lines represent the simulations from the original and updated schemes, respectively. The RMSE and R are noted in the corresponding coloured font for each simulation. The shading denotes the duration of the two dust events.
The day-to-day evolutions of the PM10 concentrations measured at the stations and the dust aerosol concentrations simulated by the model are displayed in Fig. 8. Stations located near the dust source regions (Fig. 8a–f) experienced poor air quality during dust events, with PM10 concentrations ranging from 1000 to 4000 µg m−3. During these peaks in daily mean PM10 concentrations, the updated scheme generally simulates smaller values compared to the original scheme, resulting in a closer match to observed values at the Tacheng, Hetian, Hami, and Xilinguoleimeng stations. Notably, at the Hami station, while the original scheme incorrectly simulates two dust aerosol concentration peaks around 15 March, the updated scheme aligns with observations by simulating one peak on 16 March. Additionally, at Jiuquan and Yinchuan, stations situated at the southwestern edge of the Gobi Desert, both the original and updated schemes display false peaks in simulations. Dust was transported downwind to central and eastern China, such as Luoyang and Nanjing, which exhibit better simulation results with the updated scheme at both sites. This improvement comes from the updated scheme showing a weaker dust concentration at peak times. Observations from Seoul indicate that PM10 concentrations reached 285 µg m−3 on 29 March, indicating a long-range spread of influence from the dust event. While the original scheme simulates peak dust aerosol concentrations during each of the two dust events at this site, the updated scheme only shows a minor increase on 30 March. This discrepancy is attributed to the shorter dust aerosol residence time in the updated scheme, which limits the dust's transport over longer distances.
3.2 Sea-salt emission scheme
3.2.1 Model evaluation
With the modifications applied, we simulate a global annual total sea-salt emission of 3185 Tg, compared to 3000 Tg from the original scheme simulation (Table 3). Results of the sea-salt emissions are comparatively smaller than previous estimates from global models (Jaeglé et al., 2011; Liu et al., 2012; Spada et al., 2013; Weng et al., 2020), which suffer from significant discrepancies due to different settings of the sea-salt cutoff radius in aerosol schemes. Nevertheless, our results fall into the midrange of the values estimated from the historical CMIP5 simulations compiled in IPCC AR5 (1400–6800 Tg yr−1; Intergovernmental Panel on Climate Change, IPCC, 2014) and are also consistent with the broader range observed in CMIP6 simulations (2624–64939 Tg yr−1; Thornhill et al., 2021). The simulated sea-salt burden is 9.1 Tg, slightly higher than the 8.3 Tg from the original scheme. Both values are in agreement with the estimates by the AeroCom models (3.4–11.6 Tg; Textor et al., 2006).
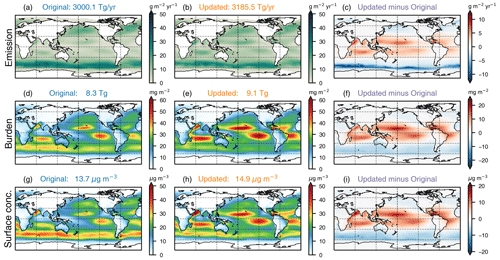
Figure 9Annual mean sea-salt emissions (a–c), burdens (d–f), and surface sea-salt aerosol concentrations (g–i) for the period from 2010 to 2012. Simulation results from the original (a, d, g) and updated (b, e, h) emission schemes are shown, along with the differences between the updated and original schemes (c, f, i). The global total sea-salt emission, global total sea-salt burden, or global mean surface sea-salt concentration is also given at the top of each panel.
Figure 9 displays the global spatial distribution of annual sea-salt emissions, burdens, and surface sea-salt aerosol concentrations simulated with the default and modified schemes. The modifications to the sea-salt emission scheme exhibit a clear impact on the emission distributions. In the Southern Ocean, where the original scheme simulates the most intense sea-salt emissions, a notable decrease of 34 Tg yr−1 (40–65° S) is modelled by the updated scheme. Conversely, emissions increase in tropical and subtropical oceans, particularly in the Arabian Sea within the Indian Ocean. These shifts in sea-salt emissions correspond to changes in the spatial distribution of the sea-salt burden and near-surface sea-salt aerosol concentrations, characterized by an increase in the region between 30° S and 35° N and a decrease outside this latitude range.
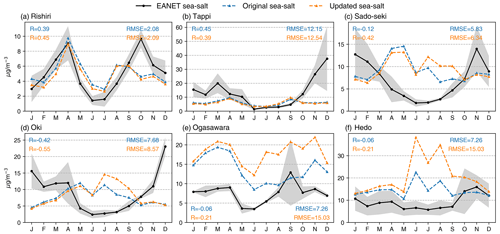
Figure 10The seasonal cycle of monthly mean simulated sea-salt aerosol concentrations (coloured lines) and EANET sea-salt concentrations (black lines) at selected stations. The locations of the stations are indicated in Fig. 3. The blue and yellow lines represent the simulations from the original and updated schemes, respectively. The RMSE and R are noted in the corresponding coloured font for each simulation. The shading on the observations illustrates the standard deviation of the monthly mean concentration over the months with sufficient data. Note that EANET sea-salt concentrations are calculated from Na+ and Cl− ion concentrations using Eq. (17).
We compare the simulation results of both schemes with the EANET aerosol concentration measurements (Fig. 10). Among the listed stations (a–f, ordered by descending latitude), the original scheme captures the seasonal variations in sea-salt aerosol concentrations at Rishiri, with an improvement in correlation observed in the updated scheme. Conversely, for the Tappi station, there is little difference in results between the updated and original schemes, and neither reproduces the December peaks in the observations. The updated scheme shows higher negative correlation coefficients in areas such as Sado-seki and Oki where the results of the original scheme deviate significantly (with negative R values) from the observations. We attribute the bias at these two island stations to the low model resolution. Firstly, the 2° resolution used in the model is insufficient to resolve these islands, making it difficult to accurately represent the specific conditions at the stations. Secondly, the coarse resolution results in grid point values that do not accurately reflect the actual conditions, particularly affecting the model's ability to capture fine-scale variability in wind speed. This limitation is critical for simulating sea-salt aerosol generation, as fine-scale wind variations are essential in regions with complex coastal topography or variable oceanic conditions. Another island station, Ogasawara, situated furthest from the continent and least affected by continental anthropogenic emissions, exhibits overestimation in both the original and updated schemes when compared with observed sea-salt aerosol concentrations, although the June minimum is captured. However, the observed PM10 concentrations at this station are well in agreement with the modelled sea-salt aerosol concentrations from the original scheme (see Fig. S4e). In contrast, the updated simulation scheme exhibits higher biases during months with higher SSTs (July to October, >25 °C) by a factor of approximately 1.8. For the Hedo station on Okinawa Island, simulated sea-salt aerosol concentrations from the updated scheme differ from those of the original scheme by a factor of 1.8 on average during the months of high SSTs (June to September, >25 °C). This is associated with the adoption of a different SST dependence function.
3.2.2 Dependence of the sea-salt emission scheme on SST and RH
We evaluate the impact of two major modifications on sea-salt aerosol emission schemes through a set of sensitivity experiments. Figure 11 presents relative differences in annual mean emissions of submicron, coarse-mode, and total sea-salt aerosols between sensitivity simulations and the original scheme. The adoption of the extended Gong source function for sea-salt aerosol emissions, optimized for sea-salt particles smaller than 0.2 µm (Gong, 2003), leads to substantial changes in emissions of different particle sizes. Specifically, the simulated emission of submicron sea-salt aerosol decreases, while that of coarse-mode sea-salt aerosol increases, as depicted in Fig. 11b, d, g, and the emissions listed in Table 4.
Firstly, we discuss the effect of the sea surface temperature (SST) correction factor on the modelled sea-salt emission. The simulation using the Gong function without the SST correction factor (Fig. 11a) predicts a reduction in submicron sea-salt aerosol emissions in low-latitude and midlatitude oceans alongside an increase in high-latitude oceans, compared to the original scheme. This contrasts with the simulation results of coarse-mode emissions using the Gong function combined with SST correction proposed by Jaeglé et al. (2011; Fig. 11e). This contrast is in fact attributed to the fact that the original scheme employs a polynomial SST correction for sea-salt particles below a particle diameter of 2.8 µm and no SST constraints for particles with diameters larger than 2.8 µm. Thus, Fig. 11e demonstrates the effect of SST correction more straightforwardly. That is, in tropical oceans, sea-salt aerosol emissions are modelled as increasing due to warm SST, while emissions are relatively suppressed in polar regions. The impact of SST constraint is negligible in midlatitude oceans. Besides, upon applying the SST correction factor (Fig. 11b), the updated scheme simulates lower submicron sea-salt aerosol emissions globally, with major differences in the North Atlantic and regions affected by cold currents on the east coast of the Pacific Ocean. Yet, in the open ocean of the North Pacific, the tropical central Pacific, and the tropical Indian Ocean, the Gong function combined with SST correction yields relatively minor differences from the original scheme.
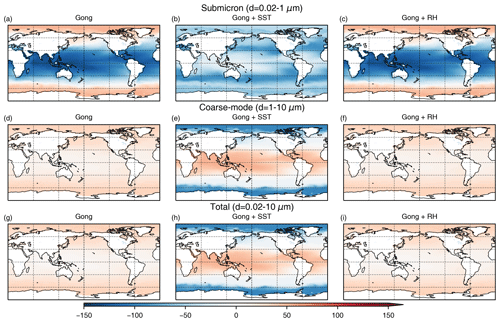
Figure 11Relative differences (%) in simulated annual sea-salt emissions between sensitivity simulations and the original scheme. The first row is for submicron sea salt, the second for the coarse-mode sea-salt, and the third for the total sea-salt simulated. The aerosol size ranges are also given at the top of each panel.
Turning to the effect of the relative humidity (RH) correction factor, a comparison between the first and third columns in Fig. 11 reveals minimal impact on sea-salt emissions. Globally, there is a slight overall increase, averaging 0.3 %. Still, the interplay between oceanic conditions and aerosol generation is intricate, with SST modulation showing a more pronounced impact than RH correction, according to simulation results.
3.3 MPOA emission scheme
3.3.1 Model evaluation
The total mass of MPOA emitted globally is 8.5 Tg yr−1 during 2010-2012 in our simulation. This result is within the range estimated for submicron MPOA in previous modelling studies, i.e. from 2.3 to 14.6 Tg yr−1 (Burrows et al., 2022; Gantt et al., 2011, 2012; Langmann et al., 2008; Meskhidze et al., 2011; Spracklen et al., 2008; Vignati et al., 2010). The MPOA burden is modelled as 0.048 Tg. Figure 12 shows the distribution of simulated annual MPOA emissions, burdens, and surface concentrations. Given the dependence on biological activity, the spatial pattern of MPOA emissions largely follows that of sea surface chlorophyll concentrations (Fig. S5a). Maximum emissions are modelled to be in the eastern equatorial Pacific Ocean, the subtropical Pacific and Atlantic oceans, and the Southern Ocean. The model result for the global mean sea surface Chl a is 0.16 mg m−3, while the MODIS/Aqua satellite products suggest a global mean value of 0.45 mg m−3. Note that the model output for Chl a can influence the model performance at simulating MPOA emissions.
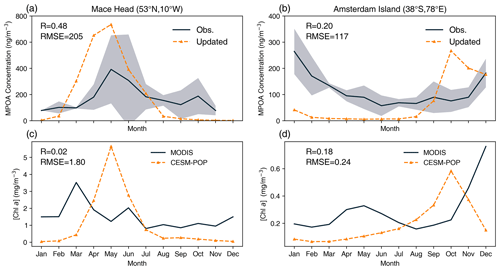
Figure 13The seasonal cycle of monthly mean simulated MPOA concentrations (coloured lines) and measured MPOA concentrations (black lines) at Mace Head (a) and Amsterdam Island (b). The locations of the stations are indicated in Fig. 3. Kendall's correlation coefficient (R) and RMSE are noted on each panel. The shading on the observations illustrates the standard deviation of the monthly mean concentration over the months with sufficient data. Additional comparison of modelled and MODIS-derived Chl a at these locations is provided (c, d).
We also evaluate the model simulation of MPOA concentrations using measurements from two representative sites. The first site, Mace Head (53.33° N, 9.90° W), located near biologically productive waters in the North Atlantic Ocean, indicates high MPOA concentrations from April to July in observations. However, the model simulates high concentrations from March to July, capturing the peak in May but overestimating it by 1.9. Additionally, the model fails to reproduce other high concentrations observed in October, possibly due to limitations at simulating Chl a (Fig. 13c). Another observation site is Amsterdam Island (37.80° S, 77.57° E), which is subject to windy and biologically active currents in the Southern Ocean. Observations show a peak in January, whereas the model predicts that the peak occurs 3 months earlier, in October. Notably, at both sites, the model underestimates MPOA concentrations compared to measurements during months of low phytoplankton activity. By comparing the monthly mean Chl a from our POP2 simulation with MODIS-derived values at these locations (Fig. 13c, d), it can be seen that the modelled biases in MPOA correspond to those in Chl a during months with lower Chl a levels. This suggests that the biases in MPOA simulations are closely tied to the biases in the modelled Chl a, underlining the importance of efficient plankton representation in predicting MPOA concentrations. Another possible explanation could be the use of a uniform OM OC value of 1.4. However, OM OC values vary spatially and seasonally, typically ranging from 1.3 to 2.1 according to observations (Philip et al., 2014).
3.3.2 Effects of the phytoplankton species on MPOA emissions
We illustrate the possible effects of phytoplankton species on MPOA emissions through a set of comparison experiments. Figure 14 shows the distribution of seasonal MPOA emissions modelled by Chl a from different phytoplankton species. Following the MARBL module that integrates marine biogeochemistry into the CESM2-POP2 component, phytoplankton is configured to represent three functional groups: diatoms, diazotrophs, and small phytoplankton. These groups are distributed across global oceans based on factors such as nutrient limitation, light availability, and temperature limitation, as well as phytoplankton grazing or mortality. The resulting chlorophyll distribution is distinctive (refer to Fig. S7), shaping the modelled MPOA emissions in the atmospheric component model substantially.
In the boreal spring (MAM) and summer (JJA), MPOA emissions are simulated to peak in the North Pacific and North Atlantic regions, primarily due to the prevalence of diatoms and small phytoplankton, which are limited by nutrients such as iron and nitrogen in these areas. Conversely, during the boreal autumn (SON) and winter (DJF), elevated MPOA emissions are modelled in the oceans south of 30° S, attributed to the growth of small phytoplankton in this region. Throughout the year, consistently high MPOA emissions are modelled in the eastern equatorial Pacific, driven by the high chlorophyll concentration in this region as simulated by POP2 models. However, satellite observations suggest that the chlorophyll concentration in this area is not as high as the simulated one (Fig. S5). Regarding diazotrophs, distribution of Chl a from this group is primarily concentrated in warmer sea areas as a result of temperature constraints. Due to the lower abundance compared to diatoms and small phytoplankton, diazotrophs have a less significant impact on MPOA emissions. The substantial role of biodiversity in shaping the composition of the Earth's atmosphere is reflected in our modelling results. However, the biological processes that produce these particles are poorly characterized, leading to large uncertainties in the estimation of global MPOA emissions.
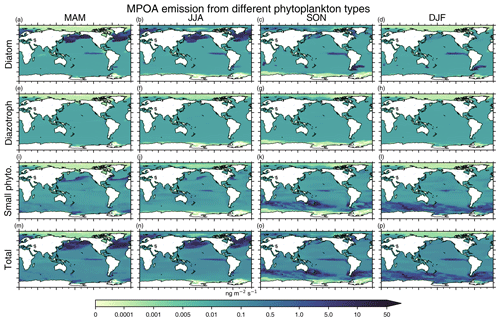
Figure 14Seasonal MPOA emissions resulting from Chl a of different types of phytoplankton. The first row is from diatoms, the second from diazotrophs, and the third from small phytoplankton (denoted Small phyto.). The fourth row is the simulated emissions resulting from all the phytoplankton types above.
3.4 Impact of dicarbonyls on SOA formation
Chemical pathways including gas-phase and heterogeneous reactions play a significant role in SOA formation. The impact of dicarbonyls on SOA formation is illustrated by comparing the simulations with and without irreversible aqueous uptake of dicarbonyls in the chemical mechanism. Figure 15 shows the global distribution of surface SOA concentrations and the atmospheric burden simulated by the default (without the uptake) and modified (with the uptake) schemes. The Amazon, central Africa, East Asia, and Southeast Asia are the main regions with high surface concentrations of SOA in both schemes. Inclusion of the aqueous reaction pathway for SOA formation resulted in a global increase in surface SOA concentrations, with an average increase of about 37 %. Growth is concentrated in central Africa, East Asia, and Southeast Asia (Fig. 15c), which is related to the spatial distribution of glyoxal and methylglyoxal (Fig. S8). In addition, we find that the effect of dicarbonyls on SOA formation shows significant seasonal variation, with higher contributions in boreal summer (JJA) and winter (DJF) and relatively lower contributions in spring (MAM) and autumn (SON). Regionally, high values in summer are mainly observed in Southeast Asia, North America, and the Amazon, while in winter, they are concentrated in central Africa and South Asia (Fig. S9a). Biogenic emissions of isoprene, the primary precursor of dicarbonyl compounds (Fu et al., 2008; Kelly et al., 2018), are the main drivers of these spatiotemporal variations (Fig. S9b).
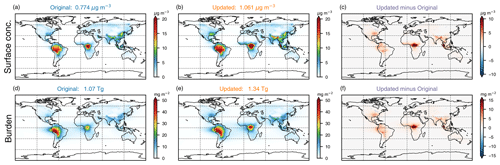
Figure 15Annual mean surface SOA concentrations (a–c) and burdens (d–f) for the period from 2010 to 2012. Simulation results from the original (a, d) and updated (b, e) chemical schemes are shown, along with the differences between the updated and original schemes (c, f). The global mean surface SOA concentration or global total SOA burden is also given at the top of each panel.
Table 5Global atmospheric burden and depositions of SOA with (updated scheme) and without (original scheme) the irreversible aqueous uptake of dicarbonyls in CAM6-chem.
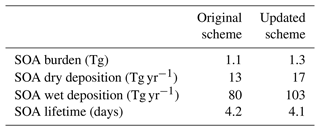
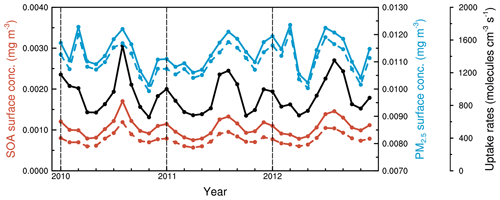
Figure 16The global monthly mean of SOA surface concentrations (red lines), PM2.5 surface concentrations (blue lines), and the uptake rates of dicarbonyls (solid black line) simulated in CAM6-chem for the period from 2010 to 2012. The solid and dashed lines represent the updated and original schemes, respectively.
Differences in atmospheric burden, depositions, and lifetime of SOA between the two schemes are shown in Table 4. In the new-scheme simulation, the total atmospheric burden and the depositions of SOA increased by about 25 % and 30 %, respectively. The lifetime of SOA against depositions is slightly reduced from 4.2 to 4.1 d, which is consistent with Hodzic et al. (2016). The contributions of glyoxal and methylglyoxal to SOA formation are 14.3 and 24.1 Tg yr−1, respectively, and occur mainly in the equatorial lower troposphere (Fig. S10). These values are higher than those estimated by Fu et al. (2008) in GEOS-Chem (6.4 and 16 Tg yr−1, respectively), which may be related to the differences in the simulation of dicarbonyl and aerosol surface area and other configurations (e.g. meteorology, emissions) between different models (Tsigaridis et al., 2014; Hodzic et al., 2020). Previous studies have shown that during heavy-haze episodes, organic aerosols can account for up to half of the PM mass, with a significant contribution from SOA (Huang et al., 2014; Zhao et al., 2019). Figure 16 shows the simulated global monthly surface mean concentrations of SOA and PM2.5 during 2010–2012. The model suggests that the irreversible aqueous uptake rate of dicarbonyls increases notably (solid black line) when heavy-haze events occur, resulting in a strong increase in SOA concentrations. The results indicate that the aqueous pathway through dicarbonyls can improve the underestimation of observed SOA concentrations during severe-haze episodes (Xing et al., 2019; Li et al., 2021).
This study sets out to develop updated emission schemes for natural aerosol species based on the CoAerM, including dust, sea salt, and MPOA, and SOA formation, which include the irreversible aqueous uptake of dicarbonyls, in the CESM2. For dust emissions, the modified scheme confines dust deflation to erodible areas, based on land use distribution instead of the original geomorphology-based hotspot-like source function, and integrates reduction factors for vegetation effects. Roughness length and soil texture from the land component, CLM5, are also incorporated to update friction velocity correction threshold factors. The updated scheme yields a more-continuous distribution of dust emission areas and complements the emissions in North America and the sub-Arctic. Notably, DAOD simulations at stations in central Asia (Karachi) and north Africa (Tamanrasset_INM) show more consistent alignment with observations in the updated scheme. Also, the updated scheme acts to shorten the residence time of dust aerosols from 4.1 to 1.6 d, resulting in notable changes in simulated dust burden and associated DAOD simulations, particularly in areas downwind of the dust source region. The simulation of dust aerosol concentrations during dust events is improved by the updated scheme in the region downwind of dust propagation. The sea-salt emission scheme is modified through updating the dependence of the source function on SST and introducing a relative-humidity-dependent correction factor for sea-salt particle size. These modifications align emissions more intuitively with oceanic conditions and sea-salt production mechanisms. The modulation of sea-salt emission by SST is more pronounced in the simulations of the updated scheme, resulting in an increase in sea-salt emission in the tropical and subtropical oceans and a decrease in the Southern Ocean. The RH correction factor exerts an enhancing effect across the globe, but the effect is very mild, resulting about a 0.3 % decrease in sea-salt emissions.
Moreover, we extend the CESM's abilities to capture the link between marine biology and atmospheric chemistry by including the MPOA emission scheme. Coupled offline with ocean component POP2, the representation of phytoplankton chlorophyll distribution by the ocean biogeochemistry module, MARBL, plays a crucial part in modelling MPOA emissions. In our simulations, the total global mass of MPOA emitted during 2010–2012 is 8.5 Tg yr−1. Our simulations reproduce the seasonal cycle observed at the North Atlantic station (Mace Head). However, the bias in the simulation of the peak month at the Southern Ocean station (Amsterdam Island) may be related to the model's simulation of the dominance of small phytoplankton in this region. We further compare the effect of spatial variability in different phytoplankton species on MPOA emission simulations, highlighting the significance of biological diversity in shaping aerosol emissions, with 148 % variability simulated among different phytoplankton types. For the formation of SOA, we consider the irreversible aqueous uptake of dicarbonyl compounds (glyoxal and methylglyoxal) in the chemical mechanism. The results show that this pathway makes an important contribution to the surface SOA concentrations (an additional 37 % of surface SOA concentrations), especially during severe-haze events. Accurate simulation of SOA requires further research into incorporating additional processes and optimizing model parameters. Collectively, these modifications make the CESM a comprehensive tool for elucidating the complexities of aerosol emissions and transformation from different spheres in the Earth system, such as the land and ocean, thus facilitating the potential for improved evaluation of their impacts on climate processes and feedback.
The CESM2 is an open-source community project, and the model code for version 2.1.3 is available at https://github.com/ESCOMP/CESM/tree/release-cesm2.1.3 (last access: 5 June 2024; UCAR/NCAR, 2020). The code used for the updated aerosol schemes in this study is permanently archived on Zenodo at https://doi.org/10.5281/zenodo.11488849 (Wang et al., 2024).
Output data from the model are available from the authors upon request. The data used in this article are available as follows:
-
the daily MODIS/Aqua Level 3 product (https://doi.org/10.5067/MODIS/MYD08_D3.061, Platnick et al., 2015),
-
DAOD climatology derived from MODIS retrievals (https://drive.google.com/drive/folders/1aQVupe7govPwR6qmsqUbR4fJQsp1DBCX?usp=sharing (Song et al., 2021b),
-
AERONET measurements of AOD (https://aeronet.gsfc.nasa.gov/cgi-bin/webtool_aod_v3, last access: 5 June 2024; Holben et al., 2001), and
-
MERRA-2 reanalysis of DAOD (https://doi.org/10.5067/KLICLTZ8EM9D (Global Modeling and Assimilation Office, 2015).
The supplement related to this article is available online at: https://doi.org/10.5194/gmd-17-7995-2024-supplement.
YW implemented the updates of the emission schemes for dust, sea salt, and MPOA; ran the simulation; and performed the model evaluation, investigation, and formal analysis. PZ implemented the updated SOA formation scheme and performed the analysis. YZ, JiaL, and ZH conceived of the project and acquired funding. JieL and YL provided the observational data for SOA and commented on the related discussion. JiaL contributed EANET data. YW performed the visualization and prepared the original draft with input from PZ. Review and editing were performed by all co-authors.
The contact author has declared that none of the authors has any competing interests.
Publisher's note: Copernicus Publications remains neutral with regard to jurisdictional claims made in the text, published maps, institutional affiliations, or any other geographical representation in this paper. While Copernicus Publications makes every effort to include appropriate place names, the final responsibility lies with the authors.
This study has been supported by the National Key Research and Development Program of China (grant no. 2019YFA0606803). The authors thank all the scientists, software engineers, and administrators who contributed to the development of the CESM project. We also appreciate the science teams of EANET, AERONET, MODIS, and VIIRS for their work related to data maintenance.
This research has been supported by the National Key Research and Development Program of China (grant no. 2019YFA0606803).
This paper was edited by Samuel Remy and reviewed by two anonymous referees.
Acid Deposition Monitoring Network in East Asia EANET: EANET, https://www.eanet.asia/, last access: 19 September 2023.
Albani, S., Mahowald, N. M., Perry, A. T., Scanza, R. A., Zender, C. S., Heavens, N. G., Maggi, V., Kok, J. F., and Otto-Bliesner, B. L.: Improved dust representation in the Community Atmosphere Model, J. Adv. Model. Earth Sy., 6, 541–570, https://doi.org/10.1002/2013MS000279, 2014.
Atmospheric Chemistry Observations & Modeling/National Center for Atmospheric Research/University Corporation for Atmospheric Research, and Climate and Global Dynamics Division/National Center for Atmospheric Research/University Corporation for Atmospheric Research: MERRA2 Global Atmosphere Forcing Data, Research Data Archive at the National Center for Atmospheric Research, Computational and Information Systems Laboratory [data set], https://doi.org/10.5065/XVAQ-2X07, 2018.
Bullard, J. E., Baddock, M., Bradwell, T., Crusius, J., Darlington, E., Gaiero, D., Gassó, S., Gisladottir, G., Hodgkins, R., McCulloch, R., McKenna-Neuman, C., Mockford, T., Stewart, H., and Thorsteinsson, T.: High-latitude dust in the Earth system, Rev. Geophys., 54, 447–485, https://doi.org/10.1002/2016RG000518, 2016.
Burrows, S. M., Ogunro, O., Frossard, A. A., Russell, L. M., Rasch, P. J., and Elliott, S. M.: A physically based framework for modeling the organic fractionation of sea spray aerosol from bubble film Langmuir equilibria, Atmos. Chem. Phys., 14, 13601–13629, https://doi.org/10.5194/acp-14-13601-2014, 2014.
Burrows, S. M., Easter, R. C., Liu, X., Ma, P.-L., Wang, H., Elliott, S. M., Singh, B., Zhang, K., and Rasch, P. J.: OCEANFILMS (Organic Compounds from Ecosystems to Aerosols: Natural Films and Interfaces via Langmuir Molecular Surfactants) sea spray organic aerosol emissions – implementation in a global climate model and impacts on clouds, Atmos. Chem. Phys., 22, 5223–5251, https://doi.org/10.5194/acp-22-5223-2022, 2022.
Chen, Y., Cheng, Y., Ma, N., Wei, C., Ran, L., Wolke, R., Größ, J., Wang, Q., Pozzer, A., Denier van der Gon, H. A. C., Spindler, G., Lelieveld, J., Tegen, I., Su, H., and Wiedensohler, A.: Natural sea-salt emissions moderate the climate forcing of anthropogenic nitrate, Atmos. Chem. Phys., 20, 771–786, https://doi.org/10.5194/acp-20-771-2020, 2020.
Chin, M., Ginoux, P., Kinne, S., Torres, O., Holben, B. N., Duncan, B. N., Martin, R. V., Logan, J. A., Higurashi, A., and Nakajima, T.: Tropospheric Aerosol Optical Thickness from the GOCART Model and Comparisons with Satellite and Sun Photometer Measurements, J. Atmos. Sci., 59, 461–483, https://doi.org/10.1175/1520-0469(2002)059<0461:TAOTFT>2.0.CO;2, 2002.
Colarco, P., Da Silva, A., Chin, M., and Diehl, T.: Online simulations of global aerosol distributions in the NASA GEOS-4 model and comparisons to satellite and ground-based aerosol optical depth, J. Geophys. Res., 115, 2009JD012820, https://doi.org/10.1029/2009JD012820, 2010.
Cullen, J. J., Reid, F. M. H., and Stewart, E.: Phytoplankton in the surface and chlorophyll maximum off southern California in August, 1978, J. Plankton Res., 4, 665–694, https://doi.org/10.1093/plankt/4.3.665, 1982.
Danabasoglu, G., Lamarque, J.-F., Bacmeister, J., Bailey, D. A., DuVivier, A. K., Edwards, J., Emmons, L. K., Fasullo, J., Garcia, R., Gettelman, A., Hannay, C., Holland, M. M., Large, W. G., Lauritzen, P. H., Lawrence, D. M., Lenaerts, J. T. M., Lindsay, K., Lipscomb, W. H., Mills, M. J., Neale, R., Oleson, K. W., Otto-Bliesner, B., Phillips, A. S., Sacks, W., Tilmes, S., van Kampenhout, L., Vertenstein, M., Bertini, A., Dennis, J., Deser, C., Fischer, C., Fox-Kemper, B., Kay, J. E., Kinnison, D., Kushner, P. J., Larson, V. E., Long, M. C., Mickelson, S., Moore, J. K., Nienhouse, E., Polvani, L., Rasch, P. J., and Strand, W. G.: The Community Earth System Model Version 2 (CESM2), J. Adv. Model. Earth Sy., 12, e2019MS001916, https://doi.org/10.1029/2019MS001916, 2020.
Darmenova, K., Sokolik, I. N., Shao, Y., Marticorena, B., and Bergametti, G.: Development of a physically based dust emission module within the Weather Research and Forecasting (WRF) model: Assessment of dust emission parameterizations and input parameters for source regions in Central and East Asia, J. Geophys. Res.-Atmos., 114, D14201, https://doi.org/10.1029/2008JD011236, 2009.
Dickerson, R. R., Kondragunta, S., Stenchikov, G., Civerolo, K. L., Doddridge, B. G., and Holben, B. N.: The Impact of Aerosols on Solar Ultraviolet Radiation and Photochemical Smog, Science, 278, 827–830, https://doi.org/10.1126/science.278.5339.827, 1997.
Donahue, N. M., Robinson, A. L., Stanier, C. O., and Pandis, S. N.: Coupled Partitioning, Dilution, and Chemical Aging of Semivolatile Organics, Environ. Sci. Technol., 40, 2635–2643, https://doi.org/10.1021/es052297c, 2006.
Eyring, V., Bony, S., Meehl, G. A., Senior, C. A., Stevens, B., Stouffer, R. J., and Taylor, K. E.: Overview of the Coupled Model Intercomparison Project Phase 6 (CMIP6) experimental design and organization, Geosci. Model Dev., 9, 1937–1958, https://doi.org/10.5194/gmd-9-1937-2016, 2016.
Fécan, F., Marticorena, B., and Bergametti, G.: Parametrization of the increase of the aeolian erosion threshold wind friction velocity due to soil moisture for arid and semi-arid areas, Ann. Geophys., 17, 149–157, https://doi.org/10.1007/s00585-999-0149-7, 1999.
Fu, T.-M., Jacob, D. J., Wittrock, F., Burrows, J. P., Vrekoussis, M., and Henze, D. K.: Global budgets of atmospheric glyoxal and methylglyoxal, and implications for formation of secondary organic aerosols, J. Geophys. Res.-Atmos., 113, D15303, https://doi.org/10.1029/2007JD009505, 2008.
Fu, T.-M., Jacob, D. J., and Heald, C. L.: Aqueous-phase reactive uptake of dicarbonyls as a source of organic aerosol over eastern North America, Atmos. Environ., 43, 1814–1822, https://doi.org/10.1016/j.atmosenv.2008.12.029, 2009.
Gantt, B., Meskhidze, N., and Kamykowski, D.: A new physically-based quantification of marine isoprene and primary organic aerosol emissions, Atmos. Chem. Phys., 9, 4915–4927, https://doi.org/10.5194/acp-9-4915-2009, 2009.
Gantt, B., Meskhidze, N., Facchini, M. C., Rinaldi, M., Ceburnis, D., and O'Dowd, C. D.: Wind speed dependent size-resolved parameterization for the organic mass fraction of sea spray aerosol, Atmos. Chem. Phys., 11, 8777–8790, https://doi.org/10.5194/acp-11-8777-2011, 2011.
Gantt, B., Johnson, M. S., Meskhidze, N., Sciare, J., Ovadnevaite, J., Ceburnis, D., and O'Dowd, C. D.: Model evaluation of marine primary organic aerosol emission schemes, Atmos. Chem. Phys., 12, 8553–8566, https://doi.org/10.5194/acp-12-8553-2012, 2012.
Gillette, D. A. and Passi, R.: Modeling dust emission caused by wind erosion, J. Geophys. Res.-Atmos., 93, 14233–14242, https://doi.org/10.1029/JD093iD11p14233, 1988.
Global Modeling and Assimilation Office (GMAO): MERRA-2 tavg1_2d_aer_Nx: 2d,1-Hourly,Time-averaged,Single-Level,Assimilation,Aerosol Diagnostics V5.12.4 (M2T1NXAER), Goddard Space Flight Center Distributed Active Archive Center (GSFC DAAC) [data set], https://doi.org/10.5067/KLICLTZ8EM9D, 2015.
Gong, S. L.: A parameterization of sea-salt aerosol source function for sub- and super-micron particles, Global Biogeochem. Cycles, 17, 1097, https://doi.org/10.1029/2003GB002079, 2003.
Gui, K., Yao, W., Che, H., An, L., Zheng, Y., Li, L., Zhao, H., Zhang, L., Zhong, J., Wang, Y., and Zhang, X.: Record-breaking dust loading during two mega dust storm events over northern China in March 2021: aerosol optical and radiative properties and meteorological drivers, Atmos. Chem. Phys., 22, 7905–7932, https://doi.org/10.5194/acp-22-7905-2022, 2022.
Hallquist, M., Wenger, J. C., Baltensperger, U., Rudich, Y., Simpson, D., Claeys, M., Dommen, J., Donahue, N. M., George, C., Goldstein, A. H., Hamilton, J. F., Herrmann, H., Hoffmann, T., Iinuma, Y., Jang, M., Jenkin, M. E., Jimenez, J. L., Kiendler-Scharr, A., Maenhaut, W., McFiggans, G., Mentel, Th. F., Monod, A., Prévôt, A. S. H., Seinfeld, J. H., Surratt, J. D., Szmigielski, R., and Wildt, J.: The formation, properties and impact of secondary organic aerosol: current and emerging issues, Atmos. Chem. Phys., 9, 5155–5236, https://doi.org/10.5194/acp-9-5155-2009, 2009.
Han, Z., Ueda, H., Matsuda, K., Zhang, R., Arao, K., Kanai, Y., and Hasome, H.: Model study on particle size segregation and deposition during Asian dust events in March 2002, J. Geophys. Res., 109, D19205, https://doi.org/10.1029/2004JD004920, 2004.
Hand, J. L., Gill, T. E., and Schichtel, B. A.: Spatial and seasonal variability in fine mineral dust and coarse aerosol mass at remote sites across the United States, J. Geophys. Res.-Atmos., 122, 3080–3097, https://doi.org/10.1002/2016JD026290, 2017.
Heal, M. R., Kumar, P., and Harrison, R. M.: Particles, air quality, policy and health, Chem. Soc. Rev., 41, 6606–6630, https://doi.org/10.1039/C2CS35076A, 2012.
Hodzic, A., Kasibhatla, P. S., Jo, D. S., Cappa, C. D., Jimenez, J. L., Madronich, S., and Park, R. J.: Rethinking the global secondary organic aerosol (SOA) budget: stronger production, faster removal, shorter lifetime, Atmos. Chem. Phys., 16, 7917–7941, https://doi.org/10.5194/acp-16-7917-2016, 2016.
Hodzic, A., Campuzano-Jost, P., Bian, H., Chin, M., Colarco, P. R., Day, D. A., Froyd, K. D., Heinold, B., Jo, D. S., Katich, J. M., Kodros, J. K., Nault, B. A., Pierce, J. R., Ray, E., Schacht, J., Schill, G. P., Schroder, J. C., Schwarz, J. P., Sueper, D. T., Tegen, I., Tilmes, S., Tsigaridis, K., Yu, P., and Jimenez, J. L.: Characterization of organic aerosol across the global remote troposphere: a comparison of ATom measurements and global chemistry models, Atmos. Chem. Phys., 20, 4607–4635, https://doi.org/10.5194/acp-20-4607-2020, 2020.
Holben, B. N., Tanré, D., Smirnov, A., Eck, T. F., Slutsker, I., Abuhassan, N., Newcomb, W. W., Schafer, J. S., Chatenet, B., Lavenu, F., Kaufman, Y. J., Castle, J. V., Setzer, A., Markham, B., Clark, D., Frouin, R., Halthore, R., Karneli, A., O'Neill, N. T., Pietras, C., Pinker, R. T., Voss, K., and Zibordi, G.: An emerging ground-based aerosol climatology: Aerosol optical depth from AERONET, J. Geophys. Res.-Atmos., 106, 12067–12097, https://doi.org/10.1029/2001JD900014, 2001 (data available at: https://aeronet.gsfc.nasa.gov/cgi-bin/webtool_aod_v3, last access: 5 June 2024).
Huang, R.-J., Zhang, Y., Bozzetti, C., Ho, K.-F., Cao, J.-J., Han, Y., Daellenbach, K. R., Slowik, J. G., Platt, S. M., Canonaco, F., Zotter, P., Wolf, R., Pieber, S. M., Bruns, E. A., Crippa, M., Ciarelli, G., Piazzalunga, A., Schwikowski, M., Abbaszade, G., Schnelle-Kreis, J., Zimmermann, R., An, Z., Szidat, S., Baltensperger, U., Haddad, I. E., and Prévôt, A. S. H.: High secondary aerosol contribution to particulate pollution during haze events in China, Nature, 514, 218–222, https://doi.org/10.1038/nature13774, 2014.
Huneeus, N., Schulz, M., Balkanski, Y., Griesfeller, J., Prospero, J., Kinne, S., Bauer, S., Boucher, O., Chin, M., Dentener, F., Diehl, T., Easter, R., Fillmore, D., Ghan, S., Ginoux, P., Grini, A., Horowitz, L., Koch, D., Krol, M. C., Landing, W., Liu, X., Mahowald, N., Miller, R., Morcrette, J.-J., Myhre, G., Penner, J., Perlwitz, J., Stier, P., Takemura, T., and Zender, C. S.: Global dust model intercomparison in AeroCom phase I, Atmos. Chem. Phys., 11, 7781–7816, https://doi.org/10.5194/acp-11-7781-2011, 2011.
Intergovernmental Panel on Climate Change (IPCC): Clouds and Aerosols, in: Climate Change 2013: The Physical Science Basis. Contribution of Working Group I to the Fifth Assessment Report of the Intergovernmental Panel on Climate Change, Cambridge University Press, Cambridge, New York, 571–657, https://doi.org/10.1017/CBO9781107415324.004, 2014.
Jaeglé, L., Quinn, P. K., Bates, T. S., Alexander, B., and Lin, J.-T.: Global distribution of sea salt aerosols: new constraints from in situ and remote sensing observations, Atmos. Chem. Phys., 11, 3137–3157, https://doi.org/10.5194/acp-11-3137-2011, 2011.
Jiang, B., Wang, D., Shen, X., Chen, J., and Lin, W.: Effects of sea salt aerosols on precipitation and upper troposphere/lower stratosphere water vapour in tropical cyclone systems, Sci. Rep., 9, 15105, https://doi.org/10.1038/s41598-019-51757-x, 2019.
Jimenez, J. L., Canagaratna, M. R., Donahue, N. M., Prevot, A. S. H., Zhang, Q., Kroll, J. H., DeCarlo, P. F., Allan, J. D., Coe, H., Ng, N. L., Aiken, A. C., Docherty, K. S., Ulbrich, I. M., Grieshop, A. P., Robinson, A. L., Duplissy, J., Smith, J. D., Wilson, K. R., Lanz, V. A., Hueglin, C., Sun, Y. L., Tian, J., Laaksonen, A., Raatikainen, T., Rautiainen, J., Vaattovaara, P., Ehn, M., Kulmala, M., Tomlinson, J. M., Collins, D. R., Cubison, M. J., E., Dunlea, J., Huffman, J. A., Onasch, T. B., Alfarra, M. R., Williams, P. I., Bower, K., Kondo, Y., Schneider, J., Drewnick, F., Borrmann, S., Weimer, S., Demerjian, K., Salcedo, D., Cottrell, L., Griffin, R., Takami, A., Miyoshi, T., Hatakeyama, S., Shimono, A., Sun, J. Y., Zhang, Y. M., Dzepina, K., Kimmel, J. R., Sueper, D., Jayne, J. T., Herndon, S. C., Trimborn, A. M., Williams, L. R., Wood, E. C., Middlebrook, A. M., Kolb, C. E., Baltensperger, U., and Worsnop, D. R.: Evolution of Organic Aerosols in the Atmosphere, Science, 326, 1525–1529, https://doi.org/10.1126/science.1180353, 2009.
Kanakidou, M., Seinfeld, J. H., Pandis, S. N., Barnes, I., Dentener, F. J., Facchini, M. C., Van Dingenen, R., Ervens, B., Nenes, A., Nielsen, C. J., Swietlicki, E., Putaud, J. P., Balkanski, Y., Fuzzi, S., Horth, J., Moortgat, G. K., Winterhalter, R., Myhre, C. E. L., Tsigaridis, K., Vignati, E., Stephanou, E. G., and Wilson, J.: Organic aerosol and global climate modelling: a review, Atmos. Chem. Phys., 5, 1053–1123, https://doi.org/10.5194/acp-5-1053-2005, 2005.
Kelly, J. M., Doherty, R. M., O'Connor, F. M., and Mann, G. W.: The impact of biogenic, anthropogenic, and biomass burning volatile organic compound emissions on regional and seasonal variations in secondary organic aerosol, Atmos. Chem. Phys., 18, 7393–7422, https://doi.org/10.5194/acp-18-7393-2018, 2018.
Klose, M., Jorba, O., Gonçalves Ageitos, M., Escribano, J., Dawson, M. L., Obiso, V., Di Tomaso, E., Basart, S., Montané Pinto, G., Macchia, F., Ginoux, P., Guerschman, J., Prigent, C., Huang, Y., Kok, J. F., Miller, R. L., and Pérez García-Pando, C.: Mineral dust cycle in the Multiscale Online Nonhydrostatic AtmospheRe CHemistry model (MONARCH) Version 2.0, Geosci. Model Dev., 14, 6403–6444, https://doi.org/10.5194/gmd-14-6403-2021, 2021.
Koehler, K. A., Kreidenweis, S. M., DeMott, P. J., Petters, M. D., Prenni, A. J., and Carrico, C. M.: Hygroscopicity and cloud droplet activation of mineral dust aerosol, Geophys. Res. Lett., 36, L08805, https://doi.org/10.1029/2009GL037348, 2009.
Kok, J. F.: A scaling theory for the size distribution of emitted dust aerosols suggests climate models underestimate the size of the global dust cycle, P. Natl. Acad. Sci. USA, 108, 1016–1021, https://doi.org/10.1073/pnas.1014798108, 2011.
Kok, J. F., Ridley, D. A., Zhou, Q., Miller, R. L., Zhao, C., Heald, C. L., Ward, D. S., Albani, S., and Haustein, K.: Smaller desert dust cooling effect estimated from analysis of dust size and abundance, Nat. Geosci., 10, 274–278, https://doi.org/10.1038/ngeo2912, 2017.
Kok, J. F., Adebiyi, A. A., Albani, S., Balkanski, Y., Checa-Garcia, R., Chin, M., Colarco, P. R., Hamilton, D. S., Huang, Y., Ito, A., Klose, M., Leung, D. M., Li, L., Mahowald, N. M., Miller, R. L., Obiso, V., Pérez García-Pando, C., Rocha-Lima, A., Wan, J. S., and Whicker, C. A.: Improved representation of the global dust cycle using observational constraints on dust properties and abundance, Atmos. Chem. Phys., 21, 8127–8167, https://doi.org/10.5194/acp-21-8127-2021, 2021a.
Kok, J. F., Adebiyi, A. A., Albani, S., Balkanski, Y., Checa-Garcia, R., Chin, M., Colarco, P. R., Hamilton, D. S., Huang, Y., Ito, A., Klose, M., Li, L., Mahowald, N. M., Miller, R. L., Obiso, V., Pérez García-Pando, C., Rocha-Lima, A., and Wan, J. S.: Contribution of the world's main dust source regions to the global cycle of desert dust, Atmos. Chem. Phys., 21, 8169–8193, https://doi.org/10.5194/acp-21-8169-2021, 2021b.
Kok, J. F., Storelvmo, T., Karydis, V. A., Adebiyi, A. A., Mahowald, N. M., Evan, A. T., He, C., and Leung, D. M.: Mineral dust aerosol impacts on global climate and climate change, Nat. Rev. Earth Environ., 4, 71–86, https://doi.org/10.1038/s43017-022-00379-5, 2023.
Langmann, B., Scannell, C., and O'Dowd, C.: New Directions: Organic matter contribution to marine aerosols and cloud condensation nuclei, Atmos. Environ., 42, 7821–7822, https://doi.org/10.1016/j.atmosenv.2008.09.002, 2008.
Lewis, E. R. and Schwartz, S. E.: Sea Salt Aerosol Production Fluxes: Estimates and Critical Analysis, in: Sea Salt Aerosol Production: Mechanisms, Methods, Measurements and Models, American Geophysical Union (AGU), 299–344, https://doi.org/10.1002/9781118666050.ch5, 2004.
Li, J. and Han, Z.: Aerosol vertical distribution over east China from RIEMS-Chem simulation in comparison with CALIPSO measurements, Atmos. Environ., 143, 177–189, https://doi.org/10.1016/j.atmosenv.2016.08.045, 2016.
Li, J., Han, Z., Sun, Y., Li, J., and Liang, L.: Chemical formation pathways of secondary organic aerosols in the Beijing-Tianjin-Hebei region in wintertime, Atmos. Environ., 244, 117996, https://doi.org/10.1016/j.atmosenv.2020.117996, 2021.
Li, J., Han, Z., Fu, P., Yao, X., and Liang, M.: Seasonal characteristics of emission, distribution, and radiative effect of marine organic aerosols over the western Pacific Ocean: an investigation with a coupled regional climate aerosol model, Atmos. Chem. Phys., 24, 3129–3161, https://doi.org/10.5194/acp-24-3129-2024, 2024.
Li, L., Mahowald, N. M., Kok, J. F., Liu, X., Wu, M., Leung, D. M., Hamilton, D. S., Emmons, L. K., Huang, Y., Sexton, N., Meng, J., and Wan, J.: Importance of different parameterization changes for the updated dust cycle modeling in the Community Atmosphere Model (version 6.1), Geosci. Model Dev., 15, 8181–8219, https://doi.org/10.5194/gmd-15-8181-2022, 2022.
Liao, H. and Seinfeld, J. H.: Global impacts of gas-phase chemistry-aerosol interactions on direct radiative forcing by anthropogenic aerosols and ozone, J. Geophys. Res.-Atmos., 110, D18208, https://doi.org/10.1029/2005JD005907, 2005.
Lindau, F. G. L., Simões, J. C., Delmonte, B., Ginot, P., Baccolo, G., Paleari, C. I., Di Stefano, E., Korotkikh, E., Introne, D. S., Maggi, V., Garzanti, E., and Andò, S.: Giant dust particles at Nevado Illimani: a proxy of summertime deep convection over the Bolivian Altiplano, The Cryosphere, 15, 1383–1397, https://doi.org/10.5194/tc-15-1383-2021, 2021.
Liu, X., Easter, R. C., Ghan, S. J., Zaveri, R., Rasch, P., Shi, X., Lamarque, J.-F., Gettelman, A., Morrison, H., Vitt, F., Conley, A., Park, S., Neale, R., Hannay, C., Ekman, A. M. L., Hess, P., Mahowald, N., Collins, W., Iacono, M. J., Bretherton, C. S., Flanner, M. G., and Mitchell, D.: Toward a minimal representation of aerosols in climate models: description and evaluation in the Community Atmosphere Model CAM5, Geosci. Model Dev., 5, 709–739, https://doi.org/10.5194/gmd-5-709-2012, 2012.
Liu, X., Ma, P.-L., Wang, H., Tilmes, S., Singh, B., Easter, R. C., Ghan, S. J., and Rasch, P. J.: Description and evaluation of a new four-mode version of the Modal Aerosol Module (MAM4) within version 5.3 of the Community Atmosphere Model, Geosci. Model Dev., 9, 505–522, https://doi.org/10.5194/gmd-9-505-2016, 2016.
Long, M. C., Moore, J. K., Lindsay, K., Levy, M., Doney, S. C., Luo, J. Y., Krumhardt, K. M., Letscher, R. T., Grover, M., and Sylvester, Z. T.: Simulations With the Marine Biogeochemistry Library (MARBL), J. Adv. Model. Earth Sy., 13, e2021MS002647, https://doi.org/10.1029/2021MS002647, 2021.
Mahowald, N., Albani, S., Kok, J. F., Engelstaeder, S., Scanza, R., Ward, D. S., and Flanner, M. G.: The size distribution of desert dust aerosols and its impact on the Earth system, Aeolian Res., 15, 53–71, https://doi.org/10.1016/j.aeolia.2013.09.002, 2014.
Mahowald, N. M., Muhs, D. R., Levis, S., Rasch, P. J., Yoshioka, M., Zender, C. S., and Luo, C.: Change in atmospheric mineral aerosols in response to climate: Last glacial period, preindustrial, modern, and doubled carbon dioxide climates, J. Geophys. Res.-Atmos., 111, D10202, https://doi.org/10.1029/2005JD006653, 2006.
Mallone, S., Stafoggia, M., Faustini, A., Gobbi, G. P., Marconi, A., and Forastiere, F.: Saharan Dust and Associations between Particulate Matter and Daily Mortality in Rome, Italy, Environ. Health Persp., 119, 1409–1414, https://doi.org/10.1289/ehp.1003026, 2011.
Mårtensson, E. M., Nilsson, E. D., de Leeuw, G., Cohen, L. H., and Hansson, H.-C.: Laboratory simulations and parameterization of the primary marine aerosol production, J. Geophys. Res.-Atmos., 108, 4297, https://doi.org/10.1029/2002JD002263, 2003.
Marticorena, B. and Bergametti, G.: Modeling the atmospheric dust cycle: 1. Design of a soil-derived dust emission scheme, J. Geophys. Res.-Atmos., 100, 16415–16430, https://doi.org/10.1029/95JD00690, 1995.
McNeill, V. F., Woo, J. L., Kim, D. D., Schwier, A. N., Wannell, N. J., Sumner, A. J., and Barakat, J. M.: Aqueous-Phase Secondary Organic Aerosol and Organosulfate Formation in Atmospheric Aerosols: A Modeling Study, Environ. Sci. Technol., 46, 8075–8081, https://doi.org/10.1021/es3002986, 2012.
Meskhidze, N., Xu, J., Gantt, B., Zhang, Y., Nenes, A., Ghan, S. J., Liu, X., Easter, R., and Zaveri, R.: Global distribution and climate forcing of marine organic aerosol: 1. Model improvements and evaluation, Atmos. Chem. Phys., 11, 11689–11705, https://doi.org/10.5194/acp-11-11689-2011, 2011.
Mokhtari, M., Gomes, L., Tulet, P., and Rezoug, T.: Importance of the surface size distribution of erodible material: an improvement on the Dust Entrainment And Deposition (DEAD) Model, Geosci. Model Dev., 5, 581–598, https://doi.org/10.5194/gmd-5-581-2012, 2012.
Monahan, E. C., Spiel, D. E., and Davidson, K. L.: A Model of Marine Aerosol Generation Via Whitecaps and Wave Disruption, in: Oceanic Whitecaps: And Their Role in Air-Sea Exchange Processes, edited by: Monahan, E. C. and Niocaill, G. M., Springer Netherlands, Dordrecht, 167–174, https://doi.org/10.1007/978-94-009-4668-2_16, 1986.
Murray, B. J., O'Sullivan, D., Atkinson, J. D., and Webb, M. E.: Ice nucleation by particles immersed in supercooled cloud droplets, Chem. Soc. Rev., 41, 6519–6554, https://doi.org/10.1039/C2CS35200A, 2012.
O'Dowd, C. D., Facchini, M. C., Cavalli, F., Ceburnis, D., Mircea, M., Decesari, S., Fuzzi, S., Yoon, Y. J., and Putaud, J.-P.: Biogenically driven organic contribution to marine aerosol, Nature, 431, 676–680, https://doi.org/10.1038/nature02959, 2004.
O'Dowd, C. D., Langmann, B., Varghese, S., Scannell, C., Ceburnis, D., and Facchini, M. C.: A combined organic-inorganic sea-spray source function, Geophys. Res. Lett., 35, L01801, https://doi.org/10.1029/2007GL030331, 2008.
Okin, G. S.: A new model of wind erosion in the presence of vegetation, J. Geophys. Res.-Earth Surf., 113, F02S10, https://doi.org/10.1029/2007JF000758, 2008.
Owen, P. R.: Saltation of uniform grains in air, J. Fluid Mech., 20, 225–242, https://doi.org/10.1017/S0022112064001173, 1964.
Park, S.-U. and In, H.-J.: Parameterization of dust emission for the simulation of the yellow sand (Asian dust) event observed in March 2002 in Korea, J. Geophys. Res.-Atmos., 108, 4618, https://doi.org/10.1029/2003JD003484, 2003.
Philip, S., Martin, R. V., Pierce, J. R., Jimenez, J. L., Zhang, Q., Canagaratna, M. R., Spracklen, D. V., Nowlan, C. R., Lamsal, L. N., Cooper, M. J., and Krotkov, N. A.: Spatially and seasonally resolved estimate of the ratio of organic mass to organic carbon, Atmos. Environ., 87, 34–40, https://doi.org/10.1016/j.atmosenv.2013.11.065, 2014.
Platnick, S. and Twomey, S.: Determining the Susceptibility of Cloud Albedo to Changes in Droplet Concentration with the Advanced Very High Resolution Radiometer, J. Appl. Meteorol. Climatol., 33, 334–347, https://doi.org/10.1175/1520-0450(1994)033<0334:DTSOCA>2.0.CO;2, 1994.
Platnick, S., King, M., and Hubanks, P.: MODIS Atmosphere L3 Daily Product, NASA MODIS Adaptive Processing System, Goddard Space Flight Center, USA [data set], https://doi.org/10.5067/MODIS/MYD08_D3.061, 2015.
Prospero, J. M., Bullard, J. E., and Hodgkins, R.: High-Latitude Dust Over the North Atlantic: Inputs from Icelandic Proglacial Dust Storms, Science, 335, 1078–1082, https://doi.org/10.1126/science.1217447, 2012.
Pu, B. and Ginoux, P.: How reliable are CMIP5 models in simulating dust optical depth?, Atmos. Chem. Phys., 18, 12491–12510, https://doi.org/10.5194/acp-18-12491-2018, 2018.
Quinn, P. K. and Bates, T. S.: Regional aerosol properties: Comparisons of boundary layer measurements from ACE 1, ACE 2, Aerosols99, INDOEX, ACE Asia, TARFOX, and NEAQS, J. Geophys. Res.-Atmos., 110, D14202, https://doi.org/10.1029/2004JD004755, 2005.
Ridley, D. A., Heald, C. L., Kok, J. F., and Zhao, C.: An observationally constrained estimate of global dust aerosol optical depth, Atmos. Chem. Phys., 16, 15097–15117, https://doi.org/10.5194/acp-16-15097-2016, 2016.
Rinaldi, M., Decesari, S., Finessi, E., Giulianelli, L., Carbone, C., Fuzzi, S., O'Dowd, C. D., Ceburnis, D., and Facchini, M. C.: Primary and Secondary Organic Marine Aerosol and Oceanic Biological Activity: Recent Results and New Perspectives for Future Studies, Adv. Meteorol., 2010, e310682, https://doi.org/10.1155/2010/310682, 2010.
Rinaldi, M., Fuzzi, S., Decesari, S., Marullo, S., Santoleri, R., Provenzale, A., von Hardenberg, J., Ceburnis, D., Vaishya, A., O'Dowd, C. D., and Facchini, M. C.: Is chlorophyll-a the best surrogate for organic matter enrichment in submicron primary marine aerosol?, J. Geophys. Res.-Atmos., 118, 4964–4973, https://doi.org/10.1002/jgrd.50417, 2013.
Roelofs, G. J.: A GCM study of organic matter in marine aerosol and its potential contribution to cloud drop activation, Atmos. Chem. Phys., 8, 709–719, https://doi.org/10.5194/acp-8-709-2008, 2008.
Schroth, A. W., Crusius, J., Sholkovitz, E. R., and Bostick, B. C.: Iron solubility driven by speciation in dust sources to the ocean, Nat. Geosci., 2, 337–340, https://doi.org/10.1038/ngeo501, 2009.
Sciare, J., Favez, O., Sarda-Estève, R., Oikonomou, K., Cachier, H., and Kazan, V.: Long-term observations of carbonaceous aerosols in the Austral Ocean atmosphere: Evidence of a biogenic marine organic source, J. Geophys. Res.-Atmos., 114, D15302, https://doi.org/10.1029/2009JD011998, 2009.
Shao, Y.: Simplification of a dust emission scheme and comparison with data, J. Geophys. Res.-Atmos., 109, D10202, https://doi.org/10.1029/2003JD004372, 2004.
Shrivastava, M., Cappa, C. D., Fan, J., Goldstein, A. H., Guenther, A. B., Jimenez, J. L., Kuang, C., Laskin, A., Martin, S. T., Ng, N. L., Petaja, T., Pierce, J. R., Rasch, P. J., Roldin, P., Seinfeld, J. H., Shilling, J., Smith, J. N., Thornton, J. A., Volkamer, R., Wang, J., Worsnop, D. R., Zaveri, R. A., Zelenyuk, A., and Zhang, Q.: Recent advances in understanding secondary organic aerosol: Implications for global climate forcing, Rev. Geophys., 55, 509–559, https://doi.org/10.1002/2016RG000540, 2017.
Song, Q., Zhang, Z., Yu, H., Ginoux, P., and Shen, J.: Global dust optical depth climatology derived from CALIOP and MODIS aerosol retrievals on decadal timescales: regional and interannual variability, Atmos. Chem. Phys., 21, 13369–13395, https://doi.org/10.5194/acp-21-13369-2021, 2021a.
Song, Q., Zhang, Z., Yu, H., Ginoux, P., and Shen, J.: Global dust optical depth climatology data, Google Drive [data set], https://drive.google.com/drive/folders/1aQVupe7govPwR6qmsqUbR4fJQsp1DBCX?usp=sharing (last access: 5 June 2024), 2021b.
Spada, M., Jorba, O., Pérez García-Pando, C., Janjic, Z., and Baldasano, J. M.: Modeling and evaluation of the global sea-salt aerosol distribution: sensitivity to size-resolved and sea-surface temperature dependent emission schemes, Atmos. Chem. Phys., 13, 11735–11755, https://doi.org/10.5194/acp-13-11735-2013, 2013.
Spracklen, D. V., Arnold, S. R., Sciare, J., Carslaw, K. S., and Pio, C.: Globally significant oceanic source of organic carbon aerosol, Geophys. Res. Lett., 35, L12811, https://doi.org/10.1029/2008GL033359, 2008.
Tegen, I. and Lacis, A. A.: Modeling of particle size distribution and its influence on the radiative properties of mineral dust aerosol, J. Geophys. Res.-Atmos., 101, 19237–19244, https://doi.org/10.1029/95JD03610, 1996.
Textor, C., Schulz, M., Guibert, S., Kinne, S., Balkanski, Y., Bauer, S., Berntsen, T., Berglen, T., Boucher, O., Chin, M., Dentener, F., Diehl, T., Easter, R., Feichter, H., Fillmore, D., Ghan, S., Ginoux, P., Gong, S., Grini, A., Hendricks, J., Horowitz, L., Huang, P., Isaksen, I., Iversen, I., Kloster, S., Koch, D., Kirkevåg, A., Kristjansson, J. E., Krol, M., Lauer, A., Lamarque, J. F., Liu, X., Montanaro, V., Myhre, G., Penner, J., Pitari, G., Reddy, S., Seland, Ø., Stier, P., Takemura, T., and Tie, X.: Analysis and quantification of the diversities of aerosol life cycles within AeroCom, Atmos. Chem. Phys., 6, 1777–1813, https://doi.org/10.5194/acp-6-1777-2006, 2006.
Thornhill, G., Collins, W., Olivié, D., Skeie, R. B., Archibald, A., Bauer, S., Checa-Garcia, R., Fiedler, S., Folberth, G., Gjermundsen, A., Horowitz, L., Lamarque, J.-F., Michou, M., Mulcahy, J., Nabat, P., Naik, V., O'Connor, F. M., Paulot, F., Schulz, M., Scott, C. E., Séférian, R., Smith, C., Takemura, T., Tilmes, S., Tsigaridis, K., and Weber, J.: Climate-driven chemistry and aerosol feedbacks in CMIP6 Earth system models, Atmos. Chem. Phys., 21, 1105–1126, https://doi.org/10.5194/acp-21-1105-2021, 2021.
Tian, R., Ma, X., and Zhao, J.: A revised mineral dust emission scheme in GEOS-Chem: improvements in dust simulations over China, Atmos. Chem. Phys., 21, 4319–4337, https://doi.org/10.5194/acp-21-4319-2021, 2021.
Tilmes, S., Hodzic, A., Emmons, L. K., Mills, M. J., Gettelman, A., Kinnison, D. E., Park, M., Lamarque, J. -F., Vitt, F., Shrivastava, M., Campuzano-Jost, P., Jimenez, J. L., and Liu, X.: Climate Forcing and Trends of Organic Aerosols in the Community Earth System Model (CESM2), J. Adv. Model. Earth Sy., 11, 4323–4351, https://doi.org/10.1029/2019MS001827, 2019.
Tsigaridis, K., Daskalakis, N., Kanakidou, M., Adams, P. J., Artaxo, P., Bahadur, R., Balkanski, Y., Bauer, S. E., Bellouin, N., Benedetti, A., Bergman, T., Berntsen, T. K., Beukes, J. P., Bian, H., Carslaw, K. S., Chin, M., Curci, G., Diehl, T., Easter, R. C., Ghan, S. J., Gong, S. L., Hodzic, A., Hoyle, C. R., Iversen, T., Jathar, S., Jimenez, J. L., Kaiser, J. W., Kirkevåg, A., Koch, D., Kokkola, H., Lee, Y. H., Lin, G., Liu, X., Luo, G., Ma, X., Mann, G. W., Mihalopoulos, N., Morcrette, J.-J., Müller, J.-F., Myhre, G., Myriokefalitakis, S., Ng, N. L., O'Donnell, D., Penner, J. E., Pozzoli, L., Pringle, K. J., Russell, L. M., Schulz, M., Sciare, J., Seland, Ø., Shindell, D. T., Sillman, S., Skeie, R. B., Spracklen, D., Stavrakou, T., Steenrod, S. D., Takemura, T., Tiitta, P., Tilmes, S., Tost, H., van Noije, T., van Zyl, P. G., von Salzen, K., Yu, F., Wang, Z., Wang, Z., Zaveri, R. A., Zhang, H., Zhang, K., Zhang, Q., and Zhang, X.: The AeroCom evaluation and intercomparison of organic aerosol in global models, Atmos. Chem. Phys., 14, 10845–10895, https://doi.org/10.5194/acp-14-10845-2014, 2014.
UCAR/NCAR: CESM v2.1.3, GitHub [code], https://github.com/ESCOMP/CESM/tree/release-cesm2.1.3 (last access: 5 June 2024), 2020.
USDA: Soil Texture Calculator, https://www.nrcs.usda.gov/resources/education-and-teaching-materials/soil-texture-calculator, last access: 23 August 2023.
Vignati, E., Facchini, M. C., Rinaldi, M., Scannell, C., Ceburnis, D., Sciare, J., Kanakidou, M., Myriokefalitakis, S., Dentener, F., and O'Dowd, C. D.: Global scale emission and distribution of sea-spray aerosol: Sea-salt and organic enrichment, Atmos. Environ., 44, 670–677, https://doi.org/10.1016/j.atmosenv.2009.11.013, 2010.
Wang, H., Easter, R. C., Zhang, R., Ma, P.-L., Singh, B., Zhang, K., Ganguly, D., Rasch, P. J., Burrows, S. M., Ghan, S. J., Lou, S., Qian, Y., Yang, Y., Feng, Y., Flanner, M., Leung, L. R., Liu, X., Shrivastava, M., Sun, J., Tang, Q., Xie, S., and Yoon, J.-H.: Aerosols in the E3SM Version 1: New Developments and Their Impacts on Radiative Forcing, J. Adv. Model. Earth Sy., 12, e2019MS001851, https://doi.org/10.1029/2019MS001851, 2020.
Wang, Y., Zhang, P., Li, J., Liu, Y., Zhang, Y., Li, J., and Han, Z.: An updated aerosol simulation in the Community Earth System Model (v2.1.3): dust and marine aerosol emissions and secondary organic aerosol formation, Zenodo [code], https://doi.org/10.5281/zenodo.11488849, 2024.
Weng, H., Lin, J., Martin, R., Millet, D. B., Jaeglé, L., Ridley, D., Keller, C., Li, C., Du, M., and Meng, J.: Global high-resolution emissions of soil NOx, sea salt aerosols, and biogenic volatile organic compounds, Sci. Data, 7, 148, https://doi.org/10.1038/s41597-020-0488-5, 2020.
Wu, C., Lin, Z., and Liu, X.: The global dust cycle and uncertainty in CMIP5 (Coupled Model Intercomparison Project phase 5) models, Atmos. Chem. Phys., 20, 10401–10425, https://doi.org/10.5194/acp-20-10401-2020, 2020.
Wu, C., Lin, Z., Liu, X., Ji, D., Zhang, H., Li, C., and Lin, G.: Description of Dust Emission Parameterization in CAS-ESM2 and Its Simulation of Global Dust Cycle and East Asian Dust Events, J. Adv. Model. Earth Sy., 13, e2020MS002456, https://doi.org/10.1029/2020MS002456, 2021.
Wu, M., Liu, X., Yang, K., Luo, T., Wang, Z., Wu, C., Zhang, K., Yu, H., and Darmenov, A.: Modeling Dust in East Asia by CESM and Sources of Biases, J. Geophys. Res.-Atmos., 124, 8043–8064, https://doi.org/10.1029/2019JD030799, 2019.
Xing, L., Wu, J., Elser, M., Tong, S., Liu, S., Li, X., Liu, L., Cao, J., Zhou, J., El-Haddad, I., Huang, R., Ge, M., Tie, X., Prévôt, A. S. H., and Li, G.: Wintertime secondary organic aerosol formation in Beijing–Tianjin–Hebei (BTH): contributions of HONO sources and heterogeneous reactions, Atmos. Chem. Phys., 19, 2343–2359, https://doi.org/10.5194/acp-19-2343-2019, 2019.
Yang, Y., Russell, L. M., Lou, S., Lamjiri, M. A., Liu, Y., Singh, B., and Ghan, S. J.: Changes in Sea Salt Emissions Enhance ENSO Variability, J. Climate, 29, 8575–8588, https://doi.org/10.1175/JCLI-D-16-0237.1, 2016.
Zender, C. S., Bian, H., and Newman, D.: Mineral Dust Entrainment and Deposition (DEAD) model: Description and 1990s dust climatology, J. Geophys. Res.-Atmos., 108, 4416, https://doi.org/10.1029/2002JD002775, 2003a.
Zender, C. S., Newman, D., and Torres, O.: Spatial heterogeneity in aeolian erodibility: Uniform, topographic, geomorphic, and hydrologic hypotheses, J. Geophys. Res.-Atmos., 108, 4543, https://doi.org/10.1029/2002JD003039, 2003b.
Zhang, K., Knipping, E., Wexler, A., Bhave, P., and Tonnesen, G.: Size distribution of sea-salt emissions as a function of relative humidity, Atmos. Environ., 39, 3373–3379, https://doi.org/10.1016/j.atmosenv.2005.02.032, 2005.
Zhang, K. M., Knipping, E. M., Wexler, A. S., Bhave, P. V., and Tonnesen, G. S.: Reply to comment on “Size distribution of sea-salt emissions as a function of relative humidity”, Atmos. Environ., 40, 591–592, https://doi.org/10.1016/j.atmosenv.2005.08.044, 2006.
Zhao, J., Qiu, Y., Zhou, W., Xu, W., Wang, J., Zhang, Y., Li, L., Xie, C., Wang, Q., Du, W., Worsnop, D. R., Canagaratna, M. R., Zhou, L., Ge, X., Fu, P., Li, J., Wang, Z., Donahue, N. M., and Sun, Y.: Organic Aerosol Processing During Winter Severe Haze Episodes in Beijing, J. Geophys. Res.-Atmos., 124, 10248–10263, https://doi.org/10.1029/2019JD030832, 2019.
Zhao, X., Liu, X., Burrows, S. M., and Shi, Y.: Effects of marine organic aerosols as sources of immersion-mode ice-nucleating particles on high-latitude mixed-phase clouds, Atmos. Chem. Phys., 21, 2305–2327, https://doi.org/10.5194/acp-21-2305-2021, 2021.