the Creative Commons Attribution 4.0 License.
the Creative Commons Attribution 4.0 License.
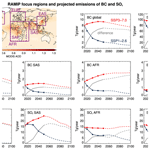
The Regional Aerosol Model Intercomparison Project (RAMIP)
Robert J. Allen
Bjørn H. Samset
Massimo A. Bollasina
Paul T. Griffiths
James Keeble
Marianne T. Lund
Risto Makkonen
Joonas Merikanto
Declan O'Donnell
David J. Paynter
Geeta G. Persad
Steven T. Rumbold
Toshihiko Takemura
Kostas Tsigaridis
Sabine Undorf
Daniel M. Westervelt
Changes in anthropogenic aerosol emissions have strongly contributed to global and regional trends in temperature, precipitation, and other climate characteristics and have been one of the dominant drivers of decadal trends in Asian and African precipitation. These and other influences on regional climate from changes in aerosol emissions are expected to continue and potentially strengthen in the coming decades. However, a combination of large uncertainties in emission pathways, radiative forcing, and the dynamical response to forcing makes anthropogenic aerosol a key factor in the spread of near-term climate projections, particularly on regional scales, and therefore an important one to constrain. For example, in terms of future emission pathways, the uncertainty in future global aerosol and precursor gas emissions by 2050 is as large as the total increase in emissions since 1850. In terms of aerosol effective radiative forcing, which remains the largest source of uncertainty in future climate change projections, CMIP6 models span a factor of 5, from −0.3 to −1.5 W m−2. Both of these sources of uncertainty are exacerbated on regional scales.
The Regional Aerosol Model Intercomparison Project (RAMIP) will deliver experiments designed to quantify the role of regional aerosol emissions changes in near-term projections. This is unlike any prior MIP, where the focus has been on changes in global emissions and/or very idealised aerosol experiments. Perturbing regional emissions makes RAMIP novel from a scientific standpoint and links the intended analyses more directly to mitigation and adaptation policy issues. From a science perspective, there is limited information on how realistic regional aerosol emissions impact local as well as remote climate conditions. Here, RAMIP will enable an evaluation of the full range of potential influences of realistic and regionally varied aerosol emission changes on near-future climate. From the policy perspective, RAMIP addresses the burning question of how local and remote decisions affecting emissions of aerosols influence climate change in any given region. Here, RAMIP will provide the information needed to make direct links between regional climate policies and regional climate change.
RAMIP experiments are designed to explore sensitivities to aerosol type and location and provide improved constraints on uncertainties driven by aerosol radiative forcing and the dynamical response to aerosol changes. The core experiments will assess the effects of differences in future global and regional (Africa and the Middle East, East Asia, North America and Europe, and South Asia) aerosol emission trajectories through 2051, while optional experiments will test the nonlinear effects of varying emission locations and aerosol types along this future trajectory. All experiments are based on the shared socioeconomic pathways and are intended to be performed with 6th Climate Model Intercomparison Project (CMIP6) generation models, initialised from the CMIP6 historical experiments, to facilitate comparisons with existing projections. Requested outputs will enable the analysis of the role of aerosol in near-future changes in, for example, temperature and precipitation means and extremes, storms, and air quality.
- Article
(20035 KB) - Full-text XML
-
Supplement
(336 KB) - BibTeX
- EndNote
Aerosols emitted from natural and anthropogenic sources exert strong influences on the Earth's climate. At the global mean scale, anthropogenic emissions of aerosols, such as black carbon (BC) from incomplete combustion, and of aerosol precursor gases, such as SO2 that leads to the formation of sulfate particles, currently induce a net, global annual mean cooling of around 0.4 ∘C (Masson-Delmotte et al., 2021). Aerosols cool the climate through their interaction with radiation and through their influence on cloud properties (Forster et al., 2021). Anthropogenic aerosols (AAs) also have a wide range of direct and indirect effects on the water and energy cycles across a range of spatio-temporal scales (Richardson et al., 2018; Samset et al., 2018a; Sand et al., 2020), on clouds (Amiri-Farahani et al., 2017; Allen et al., 2019a; Cherian and Quaas, 2020), and on extreme weather events (Samset et al., 2018c; Samset et al., 2018b; Fan et al., 2016; Sillmann et al., 2019; Wang et al., 2020; Chen et al., 2019; Luo et al., 2020), mediated by multiple physical mechanisms. However, while aerosol emissions are second only to greenhouse gases in contributing to anthropogenic climate change over the historical era (Forster et al., 2021), their influences are distinct and markedly more uncertain and spatially heterogeneous. This applies to the effective radiative forcing (ERF) induced by aerosol emissions, where aerosol is the largest uncertainty in the anthropogenic forcing of climate (Forster et al., 2021); to the resulting influence on global mean surface temperature and precipitation; and, in particular, to the influence on the regional and seasonal pattern of impact-relevant climate hazards. The regional response to aerosol changes has become an increasingly active topic of research in recent years (e.g. Nordling et al., 2019; Krishnan et al., 2020; Hari et al., 2020; Westervelt et al., 2020a; Wilcox et al., 2020; Fiedler and Putrasahan, 2021; Persad, 2023). This has been motivated by the recognition that a lack of understanding of regionally heterogeneous aerosol–precipitation interactions is hampering our understanding of historical climate change, during which greenhouse gas and aerosol emissions have broadly increased in lockstep. It also limits our confidence in future climate projections and the assessment of their impacts, as aerosol emissions are expected to rapidly decline over the coming decades.
Global anthropogenic emissions of a range of aerosol species and the resulting aerosol optical depth (AOD) increased through most the 20th century, levelled off in the early 1980s, and have recently begun to decline (Turnock et al., 2020; Dittus et al., 2020; Quaas et al., 2022). The geographical distribution of emissions and AOD has continued to evolve since 1980, with a gradual shift in the core emission region from Europe and the US to South and East Asia (Myhre et al., 2017a; Fiedler and Putrasahan, 2021), with a possible shift to Africa in the future (Lund et al., 2019). In the last decade Chinese SO2 emissions have been markedly reduced, while emissions of both SO2 and BC from India have increased, leading to a dipole change in AOD over South and East Asia (Samset et al., 2019). For the coming decades, the shared socioeconomic pathways (SSPs) used, e.g., by the Intergovernmental Panel on Climate Change (IPCC) in their 6th Assessment Report (AR6) project a wide range of possible trajectories of AA emissions from different regions, depending on national and international air quality policies, the pace of energy and transport technologies transitioning away from fossil fuel combustion, and other factors (Rao et al., 2017; Hoesly et al., 2018; Lund et al., 2019).
Recent literature has documented how both global and regional climate are highly sensitive to regional aerosol emissions and their rates of change, with aerosol influencing the atmosphere (Undorf et al., 2018; Westervelt et al., 2018; Tang et al., 2018; Wilcox et al., 2019; Luo et al., 2020; Persad, 2023) and ocean heat uptake and circulation (Ma et al., 2020; Menary et al., 2020; Hassan et al., 2021; Robson et al., 2022; Hassan et al., 2022). Aerosol–climate interactions are also strongly dependent on the physical and chemical properties of the aerosols themselves, notably whether or not they absorb shortwave radiation through the atmospheric column (like BC) or predominantly scatter it (e.g. sulfate) (Li et al., 2022).
Aerosols have been shown to influence relevant climate phenomena local to the emission sources, such as monsoons (Westervelt et al., 2020a; Xie et al., 2020), as well as to generate climate anomalies far downstream of the aerosol source regions via teleconnections (Smith et al., 2016; Undorf et al., 2018; Wilcox et al., 2019; Amiri-Farahani et al., 2020; Merikanto et al., 2021). For precipitation, the response of the Asian summer monsoon to aerosols has been found to be particularly strong (Levy et al., 2013; Westervelt et al., 2015; Acosta Navarro et al., 2017; Li et al., 2016; Bartlett et al., 2018; Samset et al., 2018b). Aerosols also affect global circulation patterns and the interhemispheric temperature contrast by affecting the albedo of the Northern Hemisphere (NH) more strongly (primarily via aerosol–cloud interactions; e.g. Wilcox et al., 2013). This has been linked to changes in the tropical rain belt (Allen et al., 2014; Allen et al., 2015; Wang et al., 2015; Allen and Ajoku, 2016; Westervelt et al., 2017) and the global (Polson et al., 2014; Shonk et al., 2020) and regional monsoons (Hari et al., 2020; Westervelt et al., 2020b; Xie et al., 2020). Aerosols have also been found to generate teleconnections from the tropics to the NH midlatitudes, affecting extratropical temperature and precipitation patterns and variability and storm tracks (Ming et al., 2011; Wilcox et al., 2019; Allen and Zhao, 2022). The frequency and intensity of extreme events have also been shown to have different sensitivities to aerosol emissions than to greenhouse-gas-induced global warming (e.g. Sillmann et al., 2019; Luo et al., 2020; Chen et al., 2019).
This all points to a strong need for improved understanding of the role of regional aerosol–climate interactions in historical and future changes in climate hazards and risk. There are, however, major known limitations, uncertainties, and gaps in current scientific knowledge related to both the interaction between aerosols and climate and between aerosol emissions and formation.
Firstly, representations of aerosol–climate interactions vary markedly between current global models (Turnock et al., 2020; Wilcox et al., 2015). Aerosols have been rudimentarily included since the 1990s, but the detail level of this implementation has greatly increased in recent years (Wilcox et al., 2013; Ekman, 2014; Chen et al., 2021). For the sixth phase of the Coupled Model Intercomparison Project (CMIP6; Eyring et al., 2016), most participating Earth system models included treatment of both anthropogenic and natural emissions, notably sulfate and black carbon aerosols and biomass burning, dust, and sea spray, respectively, with many models also treating secondary organic aerosols. Models use the same emission inputs for aerosols and aerosol precursors but simulate diverse aerosol loadings in the atmosphere due to the use of interactive schemes for aerosol species (e.g. Wilcox et al., 2013). Emissions of natural aerosols are climate dependent. Aerosol transport, removal, and deposition are treated, as is chemical processing and ageing and, in many cases, internal mixing, which impacts aerosol optical properties and their effectiveness as cloud condensation nuclei. All models now include a representation of aerosol–cloud interactions. However, the actual model parameterisations of this effect still vary substantially (Turnock et al., 2020). Representations of the effect of changing aerosol concentrations on cloud albedo (Twomey effect; Twomey, 1977) range from latitude- and longitude-dependent scalings of the cloud droplet number concentration (CDNC) through empirical relations between aerosol mass and CDNC to fully interactive, two-moment aerosol–cloud microphysics. Many models also represent aerosol effects on the precipitation formation rate (cloud lifetime or Albrecht effect; Albrecht, 1989). Crucially, the models also include treatment of the weather and climate effects of the radiative and microphysical aerosol interactions with clouds and precipitation. Over the historical era, CMIP6 models estimate a total aerosol effective radiative forcing ranging from −0.3 to −1.5 W m−2 (Forster et al., 2021), which is equivalent to an uncertainty in historical surface temperature change of over 1 K (Dittus et al., 2020). The majority of this uncertainty arises from the aerosol–cloud interactions (Forster et al., 2021). Consequently, the complexity of their climate interactions and their implementation in models introduce a major component of the remaining scientific uncertainty in both simulations of historical climate (Wilcox et al., 2013; Ekman, 2014; Zhang et al., 2021a) and future projections (Allen, 2015). Aerosol–cloud interactions are typically considered at the model grid scale, but super-parameterised models, where aerosol–cloud interactions are included in the subgrid cloud physics, can produce very different results (Wang et al., 2014; Terai et al., 2020), further adding to the uncertainty associated with the representation of aerosol processes in models.
Secondly, some aerosol emission inventories, notably for carbonaceous aerosols, are more uncertain than for greenhouse gases, both in abundance and in geographical distribution (Hoesly et al., 2018). Formation of secondary aerosols, condensational growth and coagulation, transport, removal, and ageing of aerosols are all complex processes that are difficult to model, as are all aspects of aerosol–cloud interactions (Stevens and Feingold, 2009; Boucher et al., 2013; Fan et al., 2016; Szopa et al., 2021). The optical properties of aerosols are also not fully constrained, meaning that their radiative interactions and resulting radiative forcing also have marked uncertainties. For these reasons, aerosols were highlighted in the IPCC AR6 as a major source of uncertainty in future climate projections, as they have also been in previous IPCC reports (Myhre et al., 2013; Szopa et al., 2021). Further, simulations of aerosol–climate interactions are also dependent on the model representation of the underlying climate, such as the geographical and temporal distribution of precipitation, monsoon dynamics, modes of variability, and cloud distributions and processes (Mülmenstädt and Wilcox, 2021).
Most existing literature regarding regionally heterogeneous climate responses to changing aerosol emissions has been based on idealised regional perturbations (e.g. Dong et al., 2014; Dong et al., 2016; Westervelt et al., 2017; Liu et al., 2018; Persad and Caldeira, 2018) or drawn from simulations where global emission changes are imposed (e.g. Song et al., 2014; Guo et al., 2021; Zhang et al., 2021b). While this has yielded a wide range of strong, fundamental insights, these approaches also have challenges. Idealised perturbations are usually artificially very large or applied in equilibrium simulations (e.g. Myhre et al., 2017b; Westervelt et al., 2020a), which makes the signal clearer and facilitates the analysis of the forced response and underlying mechanisms but makes connections to realistic, transient evolution challenging. Global perturbations, on the other hand, risk conflating the effects of emissions from a given region with long-range effects from another region. Comparing studies is also challenging since model setup and biases and emission pathways generally differ and can cause spurious variations between results. Hence, there is a need for a coordinated, multi-model intercomparison effort that uses (1) consistent emissions and model setup, (2) transient, realistic aerosol perturbations following established emission scenarios, and (3) individual simulations for regions of potentially strong and rapid near-term emission changes.
The Regional Aerosol Model Intercomparison Project (RAMIP) is a coordinated multi-model intercomparison project aimed at quantifying the climate and air quality responses to changing regional emissions in near-term projections. The MIP will draw on the availability of a new generation of higher-resolution models with improved representation of aerosol and related climate processes and on the activities already ongoing in CMIP6 and its range of endorsed MIPs. Thus, RAMIP seeks to target modellers and modelling groups that previously participated in CMIP6 exercises, such as DECK (Diagnostic, Evaluation and Characterization of Klima; Eyring et al., 2016), ScenarioMIP (O'Neill et al., 2016), and AerChemMIP (Collins et al., 2017). Among the key scientific outcomes expected from RAMIP, we include improved knowledge of near-term hazards, of dynamical and transient responses to heterogeneous climate forcing, and of the sensitivity of near-term climate and air quality evolution to emissions policies in key aerosol-emitting countries.
In the following sections, we introduce and describe RAMIP. We first describe and motivate the need for the MIP, then document the model protocol and setup and discuss relations to other ongoing MIPs and potential synergies from analyses combining RAMIP results with existing simulations from CMIP6. We then present a range of core findings expected from RAMIP and how they will advance our knowledge of near-term, regional climate evolution and risk. Finally, we show proof-of-principle results from three models.
RAMIP experiments focus on the transient climate evolution resulting from a range of plausible future emission changes in four key aerosol emission regions (South Asia, East Asia, Africa and the Middle East, and North America and Europe, shown in Fig. 2 and defined in figure and table captions). The core aim of the experiments is to give a more direct link to policy decisions than global emission perturbations and allow for studies of aerosol transport, air quality, regionally specific climate interactions, and teleconnections and remote impacts. Generating medium-sized initial condition ensembles of simulations from each participating model further allows for investigations of the role of aerosols relative to internal climate variability and projections of aerosol-induced climate forcing onto modes of variability. The main advance made possible by RAMIP is an evaluation of the full range of potential influences of realistic and regionally varied aerosol emission changes on near-term climate evolution, as projected by current state-of-the-art Earth system models, with fully comparable initial conditions, emissions, and experimental protocols.
All RAMIP experiments are based on the plausible future changes in regional anthropogenic aerosol emissions represented within the shared socioeconomic pathways (SSPs) used in ScenarioMIP and AR6 (O'Neill et al., 2016; Rao et al., 2017; Riahi et al., 2017). The SSPs explore a wide range of global aerosol emission trajectories, from rapid decreases in emissions of carbonaceous aerosol and sulfur dioxide in SSP1-1.9 and SSP1-2.6 to continued increases in emissions until the mid-21st century in SSP3-7.0 (Rao et al., 2017; Scannell et al., 2019; Fig. 1). The magnitude of the differences in global BC and SO2 emissions between these SSPs is comparable to their respective increases between 1850 and 2014. Within these scenarios, there are also significant differences between emission pathways in different regions to the extent that the sign of aerosol emission trends can differ between SSPs. Within a given region, emissions often vary strongly between scenarios. In particular, for Asia and Africa there are large differences between the emission pathways specified in the different SSPs (Lund et al., 2019), which are likely to result in significant uncertainties in local and remote climate hazards on 30-year timescales. The core RAMIP simulations will focus on anthropogenic emission changes in these regions (see Fig. 2).
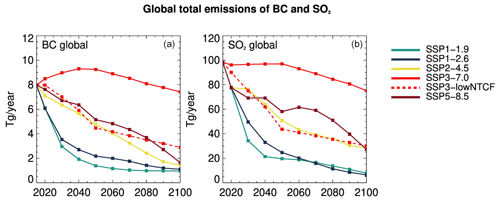
Figure 1Global total emissions of (a) black carbon and (b) sulfur dioxide from a range of SSPs, including SSP3-7.0 and SSP1-2.6, upon which the RAMIP experiments are based, and the AerChemMIP SSP3-7.0-lowNTCF pathway (Table 4).
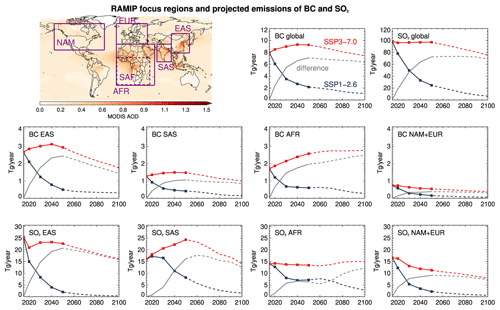
Figure 2RAMIP focus regions and projected emissions of black carbon (BC) and the sulfate aerosol precursor gas sulfur dioxide (SO2). The first row shows the regions where East Asian (EAS), South Asian (SAS), and African and Middle Eastern (AFR) emissions will be perturbed, along with 2015 aerosol optical depth at 550 nm from MODIS (combined Dark Target and Deep Blue) (Platnick, 2015), the time series of total global BC and SO2 emission rates from SSP3-7.0 (red) and SSP1-2.6 (navy), and the difference between them (grey). The second row shows BC emission rates for the four regions, and the third row shows SO2 emission rates. Africa and the Middle East is the region bounded by 35∘ S, 35∘ N, 20∘ W, and 60∘ E; East Asia is the region bounded by 20 and 53∘ N and 95 and 133∘ E; North America and Europe are the regions bounded by 35∘ N, 70∘ N, 20∘ W, and 45∘ E and 25∘ N, 70∘ N, 150∘ W, and 45∘ W; and South Asia is the region bounded by 5 and 35∘ N and 65 and 95∘ E.
Figure 2 shows the time series of total BC and SO2 emission rates over four regions from SSP3-7.0 and SSP1-2.6 from 2015 to 2100. In each case, these scenarios span the full range of aerosol and precursor emission uncertainty considered in ScenarioMIP. For the globe and Asia, differences in the emission rate between the two scenarios reach their maximum by the mid-21st century, when SSP3-7.0 emissions begin to decrease. Over Africa and the Middle East, emissions continue to increase in SSP3-7.0 until the end of the century, but growth in the difference between the two scenarios is slower in the second half of the century. Over North America and Europe, emissions decrease in both scenarios but decrease faster in SSP1-2.6. The maximum difference between the scenarios occurs in the 2050s but is small compared to the other regions. However, emission changes in this region have been shown to have a higher efficacy than lower-latitude perturbations (Shindell et al., 2015; Aamaas et al., 2016; Byrne and Schneider, 2018; Liu et al., 2018). RAMIP will include two sets of coupled transient experiments that will run from January 2015 to February 2051 to capture this period of rapid divergence between SSP3-7.0 and SSP1-2.6 aerosol pathways and a set of experiments with fixed sea surface temperatures and year 2050 emissions for the assessment of radiative forcing and rapid adjustments.
The SSPs span a wide range of emission pathways but cluster around three trajectories: rapid reductions until 2050, steady reductions throughout the 21st century, and sustained high emissions (Fig. 1). It is unlikely that the real world will follow one of these trajectories exactly. However, it may not be sufficient to interpolate between scenarios to understand the climate response in this case, particularly when there are large differences in the regional pattern of emissions between the pathways, as is the case for Asia (Samset et al., 2019). Here, emissions are projected to increase over East and South Asia together in SSP3-7.0, decrease together in SSP1-2.6 and SSP1-1.9, and continue the current pattern of East Asian reductions and South Asian increases in SSP2-4.5 and SSP5-8.5. Idealised simulations have shown that the Asian climate response to aerosol changes is strongly nonlinear due to interacting atmospheric circulation responses to emission changes in neighbouring regions (Herbert et al., 2021), and there is also a suggestion of such nonlinearities in the CMIP6 ensemble (Wilcox et al., 2020). There is similar potential for nonlinearities in the response to African emission changes, where northern emission changes are dominated by SO2 and southern emission changes are dominated by BC. Dedicated experiments, which will be included in RAMIP, are required to explore such nonlinearities fully.
2.1 Transient simulations
RAMIP includes two sets of coupled transient experiments that will be used to explore the responses to regional anthropogenic aerosol changes (Tier 1) and the sensitivities of these responses to emission location and aerosol type (Tier 2). RAMIP transient simulations will be initialised from the end of the CMIP6 historical simulations (Eyring et al., 2016). They will use the ScenarioMIP SSP3-7.0 simulation as a reference, as aerosol and precursor emissions continue to rise steadily in this scenario, both globally and in all the focus emission regions except North America and Europe (Fig. 2). In common with most CMIP6-related simulations, all RAMIP simulations will include prescribed concentrations of well-mixed greenhouse gases (GHGs) and land use changes, which will be taken from SSP3-7.0. The core (Tier 1) experiments are summarised in Table 1. These experiments use an identical setup to SSP3-7.0 but take anthropogenic aerosol and precursor emissions (SO2, SO4, and black and organic carbon) from SSP1-2.6 for four regions (depicted in Fig. 2): the globe, East Asia (EAS), South Asia (SAS), and Africa and the Middle East (AFR). In models using prescribed oxidants, these are also taken from SSP3-7.0. As such, we recommend that copies of SSP3-7.0 jobs are used as the basis for the RAMIP simulations so that only the aerosol and precursor emissions and requested output diagnostics need to be modified. An example method for producing the regional emission files from existing SSP3-7.0 and SSP1-2.6 files is included in the Supplement. The RAMIP data request is included as Appendix B and is discussed further in Sect. 2.4.
Table 1Tier 1 transient experiments: perturbations to regional anthropogenic aerosol and precursor emissions (SO2, SO4, black carbon, organic carbon). At least 10 members are requested for each experiment, initialised from the CMIP6 historical simulation. Africa and the Middle East is the region bounded by 35∘ S and 35∘ N and 20∘ W and 60∘ E; East Asia is the region bounded by 20 and 53∘ N and 95 and 133∘ E; North America and Europe are the regions bounded by 35∘ N, 70∘ N, 20∘ W, and 45∘ E and 25∘ N, 70∘ N, 150∘ W, and 45∘ W; and South Asia is the region bounded by 5 and 35∘ N and 65 and 95∘ E (Fig. 2).
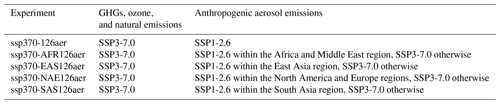
Transient simulations enable us to quantify the impact of aerosol emission uncertainty on the rate of change in climate variables and on the emergence of signals of regional climate change. Ten ensemble members are requested for both SSP3-7.0 and the RAMIP Tier 1 experiments to enable quantification of the role of internal variability and of its interaction with the forced response. Ten members is at the upper end of the ensemble size contributed by most modelling centres to the CMIP6 historical and ScenarioMIP experiments and represents a balance between computational effort and additional information gained per ensemble member (Monerie et al., 2022). In addition to the RAMIP experiments, participating models for which 10 SSP3-7.0 ensemble members are not already available will need to run additional SSP3-7.0 ensemble members to achieve the requested 10-member SSP3-7.0 reference ensemble.
In order to initialise the 10 ensemble members requested for the RAMIP experiments, participating models will need 10 historical ensemble members. Where it is not feasible to produce 10 members from 1850 to 2014, we recommend a procedure similar to that used to generate the CESM2 large ensemble (Rodgers et al., 2021) whereby additional historical members (of shorter duration) are produced by branching from existing historical members in 1950, after the application of a small random perturbation to their initial atmospheric temperature fields (known as a “micro-perturbation”). Thus, the ensemble spread results from internally generated climate variability, with some sampling of internal climate variability resulting from differing ocean states.
The optional Tier 2 experiments, summarised in Table 2, are used to explore potential nonlinear interactions between the response to emission changes in neighbouring regions and between the responses to particular aerosol species. SSP370-ASIA126aer is the basis for the assessment of the effect of changing emissions in both East and South Asia together. In combination with the Tier 1 experiments, SSP370-EAS126aer and SSP370-SAS126aer, it also enables an exploration of the potential nonlinearities in the climate response that may arise when emissions in East and South Asia follow different pathways.
Table 2Tier 2 transient experiments: coupled transient experiments from January 2015 to February 2051 designed to explore regional interactions and nonlinearities. At least 10 members are requested for each experiment, initialised from the end of the CMIP6 historical simulation. East Asia is the region bounded by 20 and 53∘ N and 95 and 133∘ E; South Asia is the region bounded by 5 and 35∘ N and 65 and 95∘ E; and sub-Saharan Africa is the region bounded by 35∘ S and 12∘ N and 20∘ W and 50∘ E (Fig. 2). Emissions of anthropogenic aerosol and precursor emissions (SO2, SO4, black carbon, organic carbon) are perturbed following SSP1-2.6 in each case for the specified region, except for ssp370-126aer_nh3nox, where NH3 and NOx should also follow SSP1-2.6. BC: black carbon; OC: organic carbon.
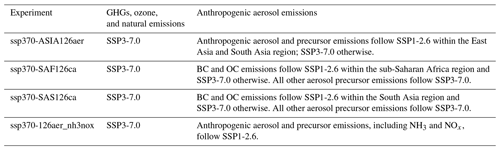
Carbonaceous aerosols are likely to become a more important component of the total aerosol burden in the future (Lund et al., 2019; Samset et al., 2019), and the climate response to changes in them is much more uncertain than the response to sulfate aerosol (Samset et al., 2016; Stjern et al., 2017). SSP370-SAF126ca and SSP370-SAS126ca isolate the impact of changes in carbonaceous aerosol emissions over sub-Saharan Africa and South Asia, respectively. Combined with the Tier 1 experiments, SSP370-AFR126aer and SSP370-SAS126aer, they also facilitate an assessment of interactions between the responses to scattering and absorbing aerosol for the two regions.
Tier 1 and Tier 2 each require around 1500 years of coupled transient simulations. Computational requirements will vary depending on the model and its resolution, but indicative computational requirements are given in Appendix A for models that have been used to perform proof-of-principle simulations. As all the RAMIP experiments are projections designed to be compared to an SSP3-7.0 baseline, 10 SSP3-7.0 simulations (860 years of coupled transient simulations) and thus also 10 historical simulations (1650 years) and a control run (> 1000 years) are required before the RAMIP simulations can be started.
2.2 Fixed sea surface temperature simulations
Simulations with fixed sea surface temperatures (fSST) are requested to accompany all Tier 1 experiments in order to provide additional data on forcing, rapid adjustments, and air quality impacts. These simulations will follow the RFMIP design (Pincus et al., 2016), specifying pre-industrial sea surface temperatures and sea ice concentrations and being run for at least 30 years (Tables 3, 4). All anthropogenic emissions will be taken from the corresponding Tier 1 experiments for the year 2050 in order to maximise the aerosol emission differences between experiments (Table 3).
Table 3Fixed-SST experiments: perturbations to regional anthropogenic aerosol and precursor emissions. All experiments use pre-industrial SSTs, sea ice extent, and land use, following the RFMIP convention (Pincus et al., 2016). Anthropogenic emissions are for the year 2050 and include the seasonal cycle. At least 30 years are requested for each experiment, and the first year is not included in analysis. Africa and the Middle East is the region bounded by 35∘ S, 35∘ N, 20∘ W, and 60∘ E; East Asia is the region bounded by 20 and 53∘ N and 95 and 133∘ E; North America and Europe are the regions bounded by 35∘ N, 70∘ N, 20∘ W, and 45∘ E and 25∘ N, 70∘ N, 150∘ W, and 45∘ W; and South Asia is the region bounded by 5 and 35∘ N and 65 and 95∘ E (Fig. 2). For all experiments, “anthropogenic aerosol emissions” are SO2, SO4, black carbon (BC), and organic carbon (OC). The optional (in italics) piClim-370-126aer_nh3nox also includes perturbations to NH3 and NOx emissions.
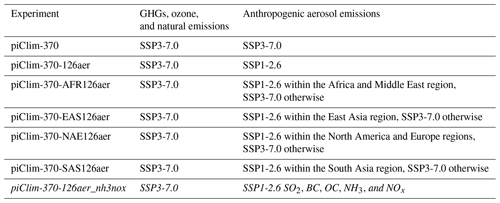
Table 4Synergies with DECK, CMIP6 historical, and other MIPs.
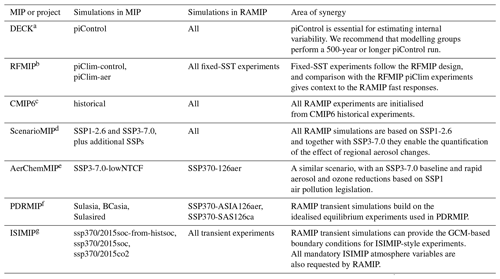
The full MIP names and associated reference papers are as follows: a Diagnostic, Evaluation and Characterization of Klima (Eyring et al., 2016); b Radiative Forcing Model Intercomparison Project (Pincus et al., 2016); c Sixth Coupled Model Intercomparison Project (Eyring et al., 2016); d Scenario Model Intercomparison Project (O'Neill et al., 2016); e Aerosols and Chemistry Model Intercomparison Project (Collins et al., 2017); f Precipitation Driver Response Model Intercomparison Project (Myhre et al., 2017b); g Inter-Sectoral Impact Model Intercomparison Project (Warszawski et al., 2014). GCM: general circulation model.
fSST simulations are highly useful for diagnosing rapid adjustments to changes in aerosol concentrations, such as atmospheric heating profiles and lapse rates, changes to relative humidity profiles, and cloud and precipitation changes. Coupled system effects, notably sea surface temperatures (SSTs) and ocean–atmosphere modes of variability will subsequently impact clouds, circulation, monsoon patterns, and the precipitation response on a slower, surface-temperature-dependent timescale. This will complicate the interpretations of the transient experiments, but the availability of well-diagnosed ERFs and precipitation response patterns from rapid adjustments from fSST simulations is expected to aid in disentangling these various aspects of the response. In total, 180 years of fSST simulations are requested by RAMIP, 30 of which are optional.
Aerosol direct and indirect radiative forcing (the sum equal to the aerosol instantaneous radiative forcing) can be calculated using the method of Ghan (2013) as the difference between two simulations with the same SSTs but different aerosol and precursor gas emissions (e.g. piClim-370 and piClim-370-126aer). Direct radiative forcing is estimated as Δ(F−Fclean), where Δ is the difference between simulations, F is the top-of-the-atmosphere (TOA) net radiative flux, and Fclean is the same flux calculated as a diagnostic but neglecting scattering and absorption by individual aerosol species. The cloud radiative forcing is calculated as Δ(Fclean−Fclear,clean), where ΔFclear,clean is the TOA radiative flux calculated as an additional diagnostic neglecting the scattering and absorbing by both aerosols and clouds. Archival of these “double radiation call” diagnostics for the fSST runs is encouraged for those models with this capability. The availability of model-specific instantaneous radiative forcing for all-sky and clear-sky conditions allows a complete decomposition of rapid adjustments from the fSST simulations.
2.3 Optional nitrate experiments
Particulate nitrate is a significant but poorly constrained fraction of the global aerosol burden (Myhre et al., 2013). Modelling of nitrate aerosol is made challenging by the difficulty in accurately representing the conditions for nitrate formation and by the strong temperature and humidity dependence of nitrate production and aerosol volatility.
As SO2 emissions are projected to decline in future, the nitrate burden is projected to be increasingly important, both relatively as the sulfate burden declines and absolutely as the decreasing H2SO4 burden leaves more NH3 available for reaction with HNO3. Regionally, however, local decreases in NOx emissions may lead to decreased nitrate and ozone burdens (Bauer et al., 2016). The effects of modification to the sink for NOx are also uncertain, with complex implications for ozone (Bauer et al., 2007) and OH.
The evolution of nitrate aerosol in the future and its effect on oxidants (and hence other short-lived climate forcers) remain largely unexplored, so far. There are important open questions regarding the role of emissions, temperature, and wet deposition (Szopa et al., 2021) in nitrate aerosol levels in the future, with its contribution to regional air quality being unknown, given that nitrate aerosol was excluded from future estimates of PM2.5 in AR6 due to lack of data. The evolution of nitrate loading is further complicated by the sensitivity of aerosol to biomass burning sources of NOx (Hickman et al., 2021), which may compensate for decreases in anthropogenic NOx emissions. A recent AerChemMIP study (Allen et al., 2021) showed large increases in nitrate aerosol based on SSP3-7.0 (∼ 50 % by mid-century) and moderate decreases (∼ 20 % by mid-century) under the mitigation pathway SSP3-7.0-lowNTCF. Moreover, these changes largely occur over two of our regions of interest: South and East Asia.
A small number of CMIP6 generation climate models include a representation of nitrate aerosol, and RAMIP makes use of this capability by including an optional pair of fSST and coupled transient experiments (piClim-370-126aer_nh3nox and ssp370-126aer_nh3nox) as the basis for multi-model exploration of the drivers of future changes in nitrate aerosol loading and quantification of the effects of nitrate aerosol on near-future aerosol forcing and regional climate change (Tables 2 and 3). We also request new diagnostics required to assess the nitrate budget, including the production and loss of precursor species (NH3, NO3) and ammonium nitrate (NH4NO3) and their deposition loss rates (Appendix B).
2.4 Diagnostics
The RAMIP data request is summarised in Appendix B. The requested output variables for all RAMIP experiments are listed in Table B1. This core output will be CMORized (by participating centres) and made available for community analysis via the Centre for Environmental Data Analysis (CEDA). Most diagnostics requested by RAMIP were included in the CMIP6 data request. However, a small number of variables have been defined specifically for RAMIP. Most of these variables are based on existing CMIP6 variables but have been modified either to reduce their vertical resolution to enable a larger number of daily variables to be archived or to extend them to new chemical species to enable the analysis of the nitrate budget. These variables are highlighted in Table B1 and defined in Table B2. We also request, where possible, cloud condensation nuclei at supersaturations of 0.02 % and 1 %, following Fanourgakis et al. (2019).
The RAMIP output protocols include sufficient fields at sufficient resolutions to disentangle the processes underlying the simulated responses to aerosol emission changes in individual models (Appendix B), including seasonal-mean changes; daily temperature, precipitation, and air quality extremes; and storms. These output fields will also enable an assessment of the influence of regional aerosol emissions on climate impacts, both via facilitation of the computation of Climate Impact Drivers (Ranasinghe et al., 2021), such as extreme heat-humidity events, flooding, or fire weather, and via scrutiny of the level of model consensus on these signals and by using them to drive climate impact models within the Inter-Sectoral Impact Model Intercomparison Project (ISIMIP; Warszawski et al., 2014; Table 4). RAMIP will be uniquely positioned to investigate these processes in a multi-model setting and to separate physical responses from internal variability.
RAMIP outputs will also enable the study of Earth system responses to future anthropogenic aerosol changes, such as natural aerosol feedbacks, cryosphere changes, and changes in atmospheric chemistry, in models that simulate them. Dust feedbacks, in particular, may be important. The RAMIP emission regions contain several large dust source regions, and there is some evidence that global dust burdens will increase in future (Allen et al., 2016; Tegen and Schepanski, 2018). However, any such changes will be strongly dependent on changes in precipitation and the atmospheric circulation, making the effect of dust feedbacks uncertain (Allen et al., 2016; Kok et al., 2018). RAMIP simulations may also be useful for studies of future air quality that may seek to quantify potential air quality and health improvements from specific emission pathways, including the air quality and health improvements due to regional emission changes.
2.5 Relations to other MIPs
RAMIP is designed around existing experiments from CMIP6 and its endorsed MIPs and builds on the coupled equilibrium experiments performed in PDRMIP (Table 4). Transient simulations will be initialised from the end of the CMIP6 historical simulations, and the SSP3-7.0 experiment from ScenarioMIP will be used as the reference simulation, following AerChemMIP. Due to the short time horizon and regional focus of the RAMIP experiments, at least 10 ensemble members per experiment are requested. As such, 10 member ensembles will also be required for the historical (used to initialise the RAMIP simulations) and SSP3-7.0 simulations (the RAMIP reference case) for participating models, which many modelling centres have already produced as part of their contribution to CMIP6.
SSP3-7.0, in which aerosol emissions continue to increase until the mid-21st century, is a natural reference experiment for RAMIP, as aerosol and precursor emissions decline in the other SSPs over this period (Figs. 1, 2). The Tier 1 SSP370-126aer is similar to the AerChemMIP SSP370-lowNTCF (Collins et al., 2017), in which all emissions follow SSP3-7.0 except for global emissions of near-term climate forcers (methane, tropospheric ozone and its precursors, tropospheric aerosols and their precursors, nitrous oxide, and ozone-depleting halocarbons), which are rapidly reduced following a dedicated pathway based on SSP1 (Gidden et al., 2019; Table 4). However, the magnitude of the aerosol reduction in SSP370-lowNTCF is only 50 %–60 % as large as that in SSP370-126aer by 2050 (Fig. 1).
RAMIP experiments will use the emission pathways from SSP3-7.0 and SSP1-2.6, as used in ScenarioMIP, to explore the effect of regional aerosol changes. SSP370-126aer isolates the role of global aerosol changes, and the remaining Tier 1 and 2 experiments explore the effects of emission location and aerosol type. Analysis of the Tier 1 experiments, SSP370-EAS126aer and SSP370-SAS126aer, and the Tier 2 experiment, SSP370-ASIA126aer, will also add to our understanding of the climate changes seen in SSP2-4.5 and SSP5-8.5, where emissions in East Asia decline in the early 21st century, while they continue to increase in South Asia (Samset et al., 2019). We also anticipate that the analysis of RAMIP simulations will be complemented by the analysis of a range of ScenarioMIP experiments, in order to quantify the role of regional aerosol emissions in the rate and magnitude of near-future climate changes and the time of emergence of regional signals. The latter will also require a quantification of internal climate variability, which will draw on the piControl simulation from the CMIP6 DECK (Table 4).
RAMIP fSST experiments follow the design used in the RFMIP piClim experiments (Table 4). RAMIP will follow RFMIP and use pre-industrial sea surface temperatures and sea ice concentrations. In RFMIP, 2014 emissions were applied for specified species in order to assess their impact over the historical period. For example, piClim-aer includes 2014 aerosol and precursor emissions, with all other forcing set to 1850 values. Comparison to the piClim-control experiment, where all forcings are set to 1850 values, shows the impact of historical aerosol changes. The equivalent RAMIP experiments focus instead on their potential future impact through the comparison of simulations with 2050 emissions from different scenarios (Table 3). The RAMIP fSST experiments can also be compared directly to the RFMIP piClim-control to show the fast response to the emission changes between 1850 and 2050.
Beyond CMIP6, RAMIP will also build on the coupled equilibrium experiments performed as part of PDRMIP (Myhre et al., 2017b) and by Westervelt et al. (2017, 2018, 2020a). The large, idealised aerosol perturbations (a multiplication or a total removal) and long coupled equilibrium simulations used in these studies resulted in valuable information about the forced climate response to regional aerosol changes, insights into the mechanisms underpinning the responses, and the sensitivity of the response to the model choice. RAMIP now offers a straightforward comparison of the climate response to regional aerosol changes and the response to total anthropogenic forcing on timescales relevant to climate mitigation and adaptation, which was not possible when using equilibrium experiments.
Danabasoglu et al. (2020)Danabasoglu et al. (2019)Danabasoglu (2019)Held et al. (2019)Paynter et al. (2018)Guo et al. (2018)Sellar et al. (2019)O'Connor et al. (2019)Tang et al. (2019)Table 5Models used to perform the test simulations shown in this work, their historical and future ERF, and references for RFMIP and piControl data used in this work. Historical ERFs [W m−2] are calculated as the difference between the RFMIP simulations piClim-aer and piClim-control and quantify the response to the increase in global aerosol emissions between 1850 and 2014. The 2050 ERFs [W m−2] are global mean values calculated as the difference between piClim-370 and piClim-370-126aer, piClim-370-AFR12aer, piClim-370-EAS126aer, and piClim-370-SAS126aer to quantify the effect of potential aerosol reductions in 2050. The spatial pattern of these forcings is shown in Fig. 3 for CESM2.
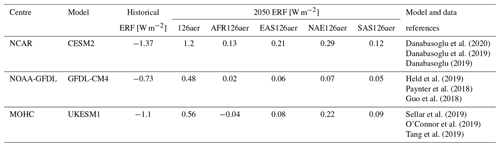
A possible concern with the RAMIP design is that small global mean forcings in the regional experiments will lead to responses that are difficult to detect in the 36-year transient experiments. However, earlier coupled transient experiments with comparable Asian aerosol perturbations to those included in RAMIP have shown significant and robust responses (e.g. Chen et al., 2019; Wilcox et al., 2019; Luo et al., 2020). Fixed-SST experiments have already been performed with three RAMIP models (Table 5), which enable the diagnosis of the effective radiative forcing (ERF) and the fast response to the changes in regional aerosol emissions (Table 3). These experiments provide an indication of the general model response that might be expected in the coupled experiments, lending further support to the suitability of the experiment design.
The models were run for 30 years, and the following analysis is based on all years except the first. The three models used here include one with a relatively strong historical aerosol ERF (CESM2, ERF W m−2), one with a relatively weak historical ERF (GFDL-CM4, ERF W m−2), and one with an ERF close to the CMIP6 mean historical aerosol ERF of −1.12 W m−2 (UKESM1-0-LL, ERF W m−2). The three models span the range of ERFs from models anticipated to participate in RAMIP, and all lie within the 68 % confidence interval of the most recent estimate of aerosol ERF (Bellouin et al., 2020). Model climatologies compared to the 5th ECMWF reanalysis (ERA5; Hersbach et al., 2020) are briefly discussed in Appendix A and shown in Figs. A1–A3.
Global differences in emissions of BC and SO2 between SSP3-7.0 and SSP1-2.6 in 2050 are comparable in size to their respective increases over the historical period (2014 vs. 1850). The global mean ERF due to global differences in aerosol emissions in 2050 between SSP3-7.0 and SSP1-2.6 has a magnitude of between 50 % and 88 % of the historical aerosol forcing for the three models, with the aerosol reductions in SSP1-2.6 relative to SSP3-7.0 leading to a positive ERF of up to 1.2 W m−2 (Table 5).
The regional emission perturbations in the Tier 1 experiments account for between 10 % and 40 % of the global emission differences in 2050 and, as expected, result in only small global mean forcing (Table 5). Each regional perturbation in Tier 1 results in global mean ERFs that are 1 order of magnitude smaller than in the experiment with global aerosol perturbations (Table 5, Fig. 3). Although such global mean forcings are unlikely to result in a detectable global response, the ERF is much larger near the emission region in each case (Fig. 3) and comparable to the magnitude of the historical forcing over Africa and South Asia. Such strongly heterogeneous forcing patterns can produce strong localised climate responses through their direct impact on the local radiation balance (Dong et al., 2014) and processes such as cloud and precipitation formation (Dong et al., 2019) and by inducing changes in the circulation of the atmosphere (Dong et al., 2016; Monerie et al., 2022; Wang et al., 2020) and the ocean (Menary et al., 2020; Allen et al., 2019b).
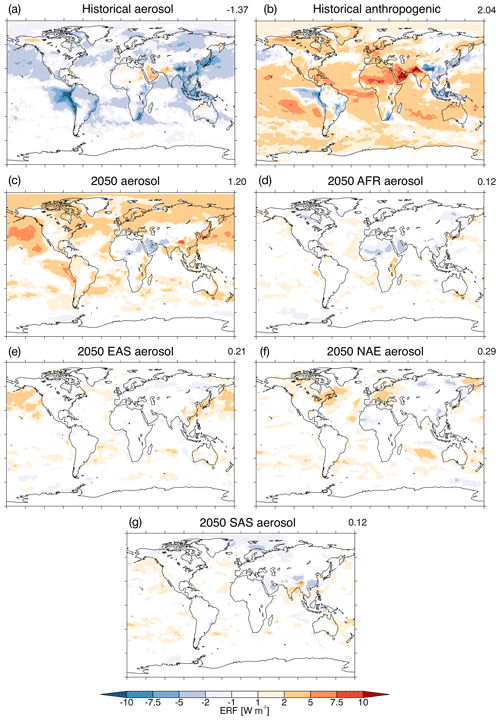
Figure 3Annual mean effective radiative forcing (ERF) from CESM2 for historical (2015 vs. 1850) (a) piClim-aer and (b) piClim-anthro relative to piClim-control, calculated from RFMIP data. RAMIP experiments (c) piClim-370-126aer, (d) piClim-370-AFR126aer, (e) piClim-370-EAS126aer, (f) piClim-370-NAE126aer, and (g) piClim-370-SAS126aer relative to piClim-370 are shown on the same colour scale. The global mean ERF [W m−2] is shown in the top right corner of each panel.
As the RAMIP emission regions and some of the main anticipated response regions experience large seasonal variations in precipitation, we show the June to August (JJA) mean response to capture the Northern Hemisphere summer monsoon season as an example of the potential climate responses in the RAMIP experiments. Annual mean anomalies largely reflect the JJA response (not shown). Figure 4 shows the JJA mean anomaly in clear-sky downwelling shortwave radiation at the surface for each of the perturbed aerosol fixed-SST simulations relative to the control experiment, piClim-370 (Table 3) from CESM2, GFDL-CM4, and UKESM1-0-LL. In all cases, a large, local increase in downwelling shortwave is seen due to the reduction in aerosol emissions. Increases are also seen in downstream regions resulting from the modulation of radiation by transported aerosol. The four regional experiments, piClim-370-AFR126aer, piClim-370-EAS126aer, piClim-370-NAE126aer, and piClim-370-SAS126aer, capture most of the large (>3 W m−2) anomalies seen in the global experiment and a large fraction of the North Pacific anomaly, which is primarily the result of East Asian emission changes (Fig. 4).
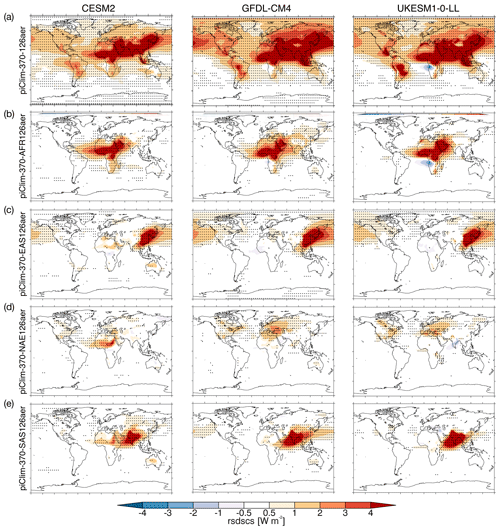
Figure 4Anomalies in June–August mean downwelling shortwave radiation (clear sky) at the surface (rsdscs) for (a) piClim-370-126aer, (b) piClim370-AFR126aer, (c) piClim-370-EAS126aer, (d) piClim-370-NAE126aer, and (e) piClim-370-SAS126aer relative to piClim-370 for CESM2, GFDL-CM4, and UKESM1-0-LL. Stippling indicates where the magnitude of the anomalies is larger than 0.5 times the interannual standard deviation.
Although the models are driven by the same emissions, inter-model differences in the response can be seen in the downwelling shortwave anomalies, which reflect differences in, e.g., aerosol transport, atmospheric lifetime, and radiative properties (Fig. 4). GFDL-CM4 simulates a larger increase in clear-sky downwelling shortwave radiation over the North Pacific in the two Asian experiments compared to UKESM1-0-LL and CESM2, while CESM2 simulates larger increases over the Arabian Peninsula and North Africa than the other models. In piClim-370-126aer and piClim-370-AFR126aer, both GFDL-CM4 and UKESM1-0-LL simulate large decreases in downwelling shortwave radiation relative to piClim-370, which are not seen in CESM2, reflecting the different aerosol properties in the three models. UKESM1-0-LL and GFDL-CM4 simulate large increases in downwelling shortwave radiation over North America and Europe in piClim-370-NAE126aer relative to piClim-370, while CESM2 also includes a large anomaly over North Africa due to dust feedbacks. Such differences in the pattern of the radiative response to regional emission perturbations may influence the dynamical response and demonstrate the need for RAMIP's consistent multi-model approach to be able to identify robust responses to regional aerosol changes.
CESM2, GFDL-CM4, and UKESM1-0-LL all simulate large Asian and African precipitation anomalies in each of the fixed-SST experiments (Fig. 5). A number of features are consistent between CESM2 and GFDL-CM4, including increased precipitation over east and west Africa and China in response to global aerosol reductions in SSP1-2.6 and drying over India. In all models, comparison of the SSP370-126aer and SSP370-AFR126aer anomalies suggests that the response to local aerosol changes dominates the fast African precipitation response to global aerosol reductions, while the Asian summer monsoon response is more sensitive to remote changes (Fig. 5).
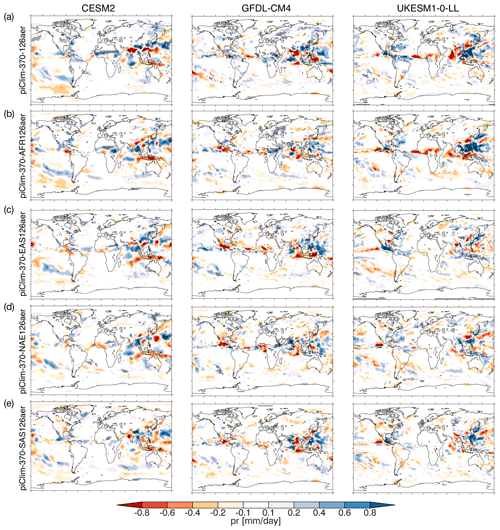
Figure 5Anomalies in June–August mean precipitation (pr) for (a) piClim-370-126aer, (b) piClim370-AFR126aer, (c) piClim-370-EAS126aer, (d) piClim-370-NAE126aer, and (e) piClim-370-SAS126aer relative to piClim-370 for CESM2, GFDL-CM4, and UKESM1-0-LL. Stippling indicates where the magnitude of the anomalies is larger than 0.5 times the interannual standard deviation.
CESM2 and GFDL-CM4 both simulate different patterns of precipitation anomalies over India and China depending on the aerosol emission source (Fig. 5). UKESM1-0-LL, however, simulates similar patterns but with different magnitudes, consistent with behaviour seen in earlier versions of this model (e.g. Dong et al., 2014, 2016; Kasoar et al., 2018). Such differences suggest that different cloud and radiative properties or different dynamical mechanisms are at play in this region in different models, possibly projecting onto and being modulated by each model's baseline climatology. The consistent experiment design in RAMIP will enable the exploration of such differences, enable the identification of responses that are robust to model differences, and further our understanding of how the long-standing model biases in this region might affect the simulated response to forcing.
Over Africa and East Asia, the precipitation anomaly from UKESM1-0-LL generally has the opposite sign to that from CESM2 and GFDL-CM4. Although an increase in precipitation, as simulated by the latter models, is generally the expected response to a decrease in aerosol emissions, the decrease simulated by UKESM1-0-LL is consistent within that model's climate response. In these fixed-SST experiments, UKESM1-0-LL is largely mirroring its response to historical increases in global aerosol emissions, seen in the difference between the RFMIP piClim-aer and piClim-control experiments (Fig. 6). However, historical experiments are unlikely to be reliable predictors of near-future responses to regional aerosol changes in all cases, as is suggested in the comparison between historical and future ERF for the three models (Table 5, Fig. 3). Aerosol forcing is mediated by clouds, which may change in response to increasing greenhouse gas (GHG) concentrations, influencing the pattern and magnitude of aerosol forcing. Furthermore, some anticipated near-future aerosol emission changes, such as those over Africa, have no historical equivalent.
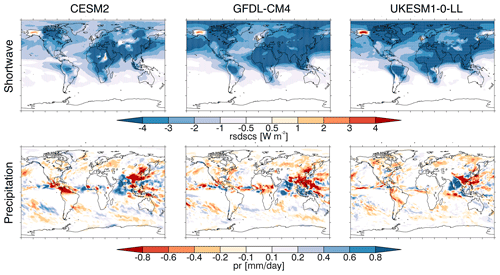
Figure 6Anomalies in JJA mean (a) downwelling shortwave radiation at the surface (clear sky) and (b) precipitation from piClim-aer relative to piClim-control for CESM2, GFDL-CM4, and UKESM1-0-LL.
Overall, the results of these fSST test simulations are highly promising for the ability of the RAMIP simulations to help answer our core science questions. All three models show robust responses, broadly as expected from previous literature, although with intriguing inter-model variability that illustrates the diversity in current aerosol–precipitation connections in Earth system models and motivates the need for transient coupled simulations in a large number of models. We note, however, that the model responses in an fSST setup are markedly different to what we can expect in fully coupled simulations. Firstly, our time slice simulations were performed at or near the time of the largest emission differences between signal and baseline, thus maximising the ERFs. Coupled transient simulations will instead track the gradual evolution of this forcing pattern and its subsequent climate responses. Secondly, fSST is a steady-state setup, where the models have time to equilibrate, while in transient simulations, the response time of the climate comes strongly into play. Thirdly, and most importantly, the ocean response present in coupled simulations will likely – even on the annual or decadal timescales of RAMIP – strongly modulate both the magnitude and the regional and seasonal patterns of the climate responses to aerosol emission changes. The results presented here are therefore expected to be quite different to those from the final, coupled RAMIP experiments but can still be interpreted as a first look at the potential span of rapid adjustments to future regional aerosol changes.
Confident climate projections at regional scales are essential for better-informed adaptation and mitigation policy measures. Such predictions will require progress both in constraining radiative forcing and in understanding the climate response to this forcing. Rapidly changing anthropogenic aerosol emissions represent a key uncertainty in such near-term projections due to the documented strong regional and global climate effects they have had over the historical period and the large uncertainties in near-term emission pathways, radiative forcing, and the dynamical responses to heterogeneous forcing.
There is a possibility of rapid changes in aerosol emissions over the next 30 years that are comparable in size to the increase in emissions over the historical era. The regional responses (and possible global responses via atmospheric teleconnections) to such changes are poorly understood. RAMIP will improve our physical understanding of how realistic regional aerosol emissions impact local as well as remote climate and provide the information needed to make direct links between regional climate policies and regional climate change.
CMIP6 models offer significant advances in the representation of aerosol and aerosol–climate interactions compared to CMIP5 (e.g. Bellouin et al., 2013; Mulcahy et al., 2018; Kirkevåg et al., 2018; Wyser et al., 2019). CMIP6 projections also explore a wider range of aerosol emission uncertainty than CMIP5 (Scannell et al., 2019), and many participating centres have produced several ensemble members, which better enable the study of regional climate change and the interactions between forced and internal climate variability. RAMIP builds on the advances made in CMIP6 and its endorsed MIPs to further our understanding and quantification of the climate response to regional forcing. Focusing on the regions with the largest near-term aerosol emission uncertainty in the shared socioeconomic pathways, the RAMIP Tier 1 experiments will enable the quantification of the effect of regional emission policies on changes in climate hazards local to and remote from the emission regions and of their influence on regional rates of change and emergence of climate signals. Tier 2 experiments are designed to explore the interactions in the climate response to emission changes in multiple regions and between different aerosol species. Fixed-SST simulations will enable the diagnosis of aerosol forcing and the quantification of the fast response to emission changes.
RAMIP will quantify the transient evolution of core climate determinants such as temperature, precipitation, cloud fraction, and humidity; of variability indicators such as the diurnal temperature range and seasonality; and of indicators of change such as extreme event intensities and occurrence rates and the variability in daily weather. These will be interpreted in light of forcing calculations (top-of-atmosphere, surface, and atmospheric) from fixed-SST simulations. The availability of a 10-member ensemble for each experiment from each model will provide a unique opportunity to separate the influence of internal variability within one model from the inter-model differences introduced by distinct climatologies, physical process representation, and responses to forcing. Core metrics will be regional rates of change under high or low near-term aerosol emission changes as well as changes in probability density functions of daily weather. RAMIP will also focus on transient evolutions of aerosol–cloud interactions over the regions of study, on aerosol transport and links to air pollution, and on atmospheric teleconnections (e.g. through influences on the Walker circulation) and ocean circulation and variability changes (such as the Indian Ocean Dipole and Atlantic Meridional Overturning Circulation).
The three models used to conduct fixed-SST test experiments – CESM2 (Danabasoglu et al., 2020), GFDL-CM4 (Held et al., 2019), and UKESM1-0-LL (Sellar et al., 2019) – were chosen as their historical aerosol ERF spans the range of ERFs seen in the initial models participating in RAMIP and the interquartile range of CMIP6 ERFs (−1.26 to −0.85 W m−2). They are also independent in terms of their components, using, for example, different atmosphere and aerosol schemes. CESM2 uses the Model Aerosol Module (MAM4) two-moment aerosol scheme and the CAM6 atmosphere (Danabasoglu et al., 2020), UKESM1-0-LL uses the Global Model of Aerosol Processes (GLOMAP-mode) two-moment aerosol scheme for anthropogenic aerosol and the HadGEM3-GA7.1 atmosphere (Sellar et al., 2019), and GFDL-CM4 uses a bulk mass-based scheme and the GFDL-AM4.0.1 atmosphere (Held et al., 2019).
A comparison of the 1950–2014 mean JJA mean near-surface temperature, downwelling shortwave radiation at the surface, and precipitation from the models and ERA5 is shown in Figs. A1 and A2. A long period is chosen to minimise the impact of internal variability on the comparison, and JJA is shown due to the importance of the monsoon in the RAMIP emission regions. CESM2 and UKESM1-0-LL overestimate downwelling shortwave radiation over the Atlantic by more than 15 W m−2 relative to ERA5 (Figs. A1 and A2). UKESM has additional positive biases over Africa, the Middle East, and Asia, while CESM2 has a negative bias over Africa. All three models overestimate the downwelling shortwave radiation over Europe, to varying extents, relative to ERA5. GFDL-CM4 and UKESM1-0-LL are 1–2 K cooler than ERA5 over the Northern Hemisphere midlatitudes to high latitudes, while CESM2 is warmer (Figs. A1 and A2). CESM2 and UKESM1-0-LL are both slightly warmer than ERA5 throughout the tropics.
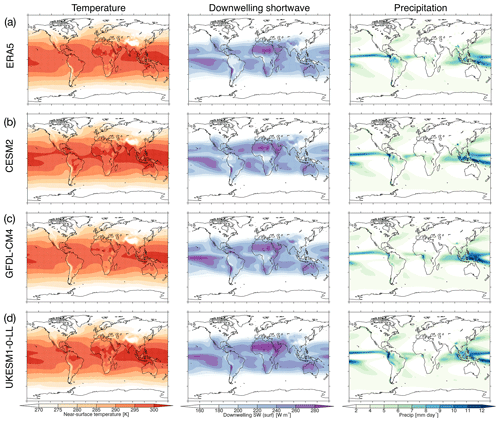
Figure A1Long-term (1950–2014) mean June–August near-surface temperature, downwelling shortwave radiation at the surface, and precipitation for (a) reanalysis data from ERA5 and the three models used in the test experiments, (b) CESM2, (c) GFDL-CM4, and (d) UKESM1-0-LL, calculated using the CMIP6 historical experiment.
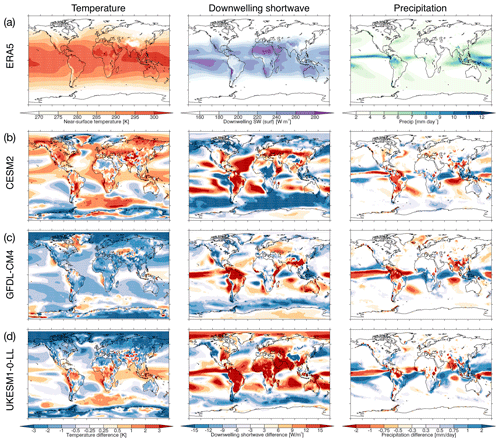
Figure A2(a) Long-term (1950–2014) mean JJA near-surface temperature, downwelling shortwave radiation at the surface, and precipitation from ERA5. The remaining panels show anomalies relative to ERA5 for (b) CESM2, (c) GFDL-CM4, and (d) UKESM1-0-LL, calculated using the CMIP6 historical experiment.
All three models underestimate the strength of the Asian summer monsoon, which is a common and long-standing bias amongst climate models (Sperber et al., 2013; Wilcox et al., 2020). UKESM1-0-LL has the largest bias over South Asia but performs better over East Asia (Figs. A1 and A2). All models show signs of an Atlantic Intertropical Convergence Zone (ITCZ) that is located too far to the south and a double ITCZ over the Pacific (which is also reflected in the interannual variability in the models, shown in Fig. A3). In all three models, Indian Ocean precipitation extends too far to the west and northwest compared to ERA5.
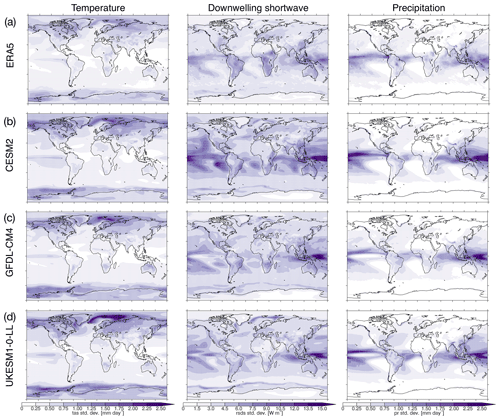
Figure A3Interannual standard deviation in near-surface temperature, downwelling shortwave radiation at the surface, and precipitation for (a) ERA5, (b) CESM2, (c) GFDL-CM4, and (d) UKESM1-0-LL. For ERA5, this is calculated from detrended data for 1950 to 2020. For the CMIP6 models, this is calculated from all available years of the piControl simulation.
The model differences shown here may play a role in the diverse responses to the emission changes imposed in the RAMIP experiments. Such dependencies will be explored further when the Tier 1 experiments are available for a larger selection of models.
Initial benchmarking runs performed with these models provide indicative computational requirements for participation in RAMIP. Simulations with CESM2.1 at 1∘ resolution on NCAR's computer cluster “Cheyenne” require approximately 100 000 core hours per coupled transient simulation. With 10 ensemble members per experiment and 4 (not counting the baseline) experiments for Tier 1, this equates to approximately 4 million core hours in total for the Tier 1 coupled transient experiments and 8 million core hours for all proposed RAMIP experiments. Simulations with UKESM1-0-LL run on the Met Office Cray XC40 supercomputer require approximately 400 000 core hours per coupled transient simulation. This equates to approximately 16 million core hours in total for the Tier 1 coupled transient experiments and 32 million core hours for all proposed RAMIP experiments.
The RAMIP data request is designed to include sufficient fields at sufficient temporal resolutions to disentangle the processes underlying the simulated responses to aerosol emission changes in individual models (Tables B1 and B2), including seasonal-mean changes; daily temperature, precipitation, and air quality extremes; and storms. These output fields will also enable assessment of the influence of regional aerosol emissions on climate impacts such as extreme heat-humidity events, flooding, or fire weather. RAMIP outputs will also enable the study of Earth system responses to future anthropogenic aerosol changes, such as natural aerosol feedbacks, cryosphere changes, and changes in atmospheric chemistry, in models that simulate them.
The majority of variables requested by RAMIP are standard CMIP6 variables. However, we also request new diagnostics required to assess the nitrate budget, including the production and loss of precursor species (NH3, NO3) and the ammonium nitrate (NH4NO3) and their deposition loss rates. These variables are highlighted in Table B1 and defined in Table B2. We also request, where possible, cloud condensation nuclei at supersaturations of 0.02 % and 1 %, following Fanourgakis et al. (2019).
Table B1Variables requested from participating centres (Climate Model Output Rewriter (CMOR) variable names). The variables listed in the “RAMIP” columns are new variables designed for RAMIP, largely based on existing CMOR variables and defined in Table B2.
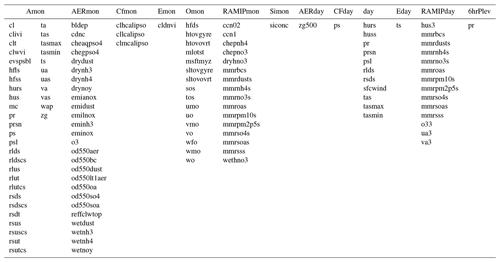
Where possible, we encourage the archival of double radiation call diagnostics for the fSST runs to enable a complete decomposition of rapid adjustments following the Ghan (2013) methodology. Direct radiative forcing is estimated as Δ(F−Fclean), where Δ is the difference between simulations, F is the top-of-the-atmosphere (TOA) net radiative flux, and Fclean is the same flux calculated as a diagnostic but neglecting scattering and absorption by individual aerosol species. The cloud radiative forcing is calculated as Δ(Fclean−Fclear,clean), where ΔFclear,clean is the TOA radiative flux calculated as an additional diagnostic neglecting the scattering and absorbing by both aerosols and clouds.
(Fanourgakis et al., 2019)(Fanourgakis et al., 2019)Table B2Monthly and daily variables defined for RAMIP. These variables are either reduced vertical-resolution versions of existing CMOR variables, new variables required to assess the nitrate budget based on existing CMOR variables for different species, or variables used in AeroCom analysis (Fanourgakis et al., 2019).
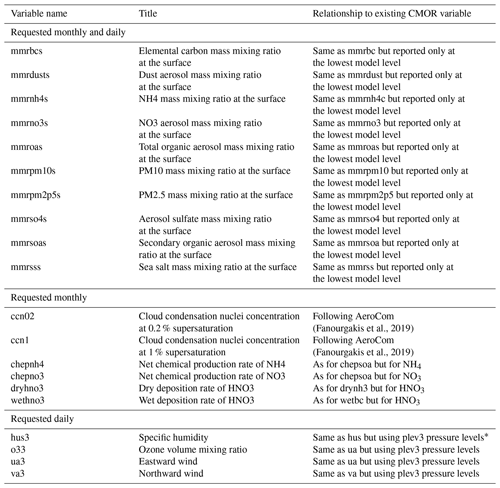
* plev3 data are requested at three pressure levels: 850, 500, and 250 hPa.
Emission data for the SSPs (Riahi et al., 2017) are publicly available. They can be found from the SSP database v2 (https://tntcat.iiasa.ac.at/SspDb, last access: 28 June 2023,) via the “CMIP6 Emissions” tab or from the ESGF Input4MIPs data repository (https://esgf-node.llnl.gov/projects/input4mips/, last access: 28 June 2023). RAMIP experiments are designed around the SSPs, which were originally produced for ScenarioMIP. Users wishing to access SSP emission data via Input4MIPs should use the following search constraints to see the files: MIP Era – “CMIP6”; Target MIP – “ScenarioMIP”; and Dataset Category – “emissions”. However, the RAMIP baseline experiment is the ScenarioMIP SSP3-7.0 experiment, and RAMIP participants will need to run (or use a model that has previously been used to run) 10 members of this experiment prior to running the RAMIP experiments. As input file requirements are model-specific, we recommend modifying existing emission files used to perform the ScenarioMIP experiments for your model to produce the necessary input files for RAMIP. A suggested method for making the regional emission perturbations required for RAMIP can be found in the Supplement, if required.
All data requested from the RAMIP simulations described in this paper will be CMORized and distributed through the Centre for Environmental Data Analysis (CEDA) with digital object identifiers (DOIs) assigned. As in CMIP6, the model output will be freely accessible after registration. In order to document RAMIP's scientific impact and enable ongoing support of RAMIP, users are asked to acknowledge CMIP6, RAMIP, the participating modelling groups, and CEDA.
The supplement related to this article is available online at: https://doi.org/10.5194/gmd-16-4451-2023-supplement.
All authors designed the experiments and wrote the paper. RJA, DJP, STR, and JK performed the simulations used in Sect. 3. LJW, MTL, RJA, and BHS performed the analysis.
The contact author has declared that none of the authors has any competing interests.
Publisher's note: Copernicus Publications remains neutral with regard to jurisdictional claims in published maps and institutional affiliations.
Laura J. Wilcox and Paul T. Griffiths are supported by the Natural Environment Research Council (NERC; grant NE/W004895/1, TerraFIRMA). Laura J. Wilcox, Paul T. Griffiths, James Keeble, and Steven T. Rumbold are additionally supported by the National Centre for Atmospheric Science. Robert J. Allen acknowledges support from NSF grant AGS-2153486. Robert J. Allen, Massimo A. Bollasina, Marianne T. Lund, Bjørn H. Samset, and Laura J. Wilcox acknowledge funding by the Research Council of Norway through grant no. 324182 (CATHY). Marianne T. Lund and Bjørn H. Samset also acknowledge funding through grant no. 248834 (QUISARC). Geeta G. Persad acknowledges support from NSF grant CNH-1715557.
We acknowledge the World Climate Research Programme, which, through its Working Group on Coupled Modelling, coordinated and promoted CMIP6. We thank the climate modelling groups for producing and making available their model output, the Earth System Grid Federation (ESGF) for archiving the data and providing access, and the multiple funding agencies who support CMIP6 and ESGF. We also acknowledge the use of ERA5 data produced by ECMWF. Additional details of ERA5 can be found at https://cds.climate.copernicus.eu/ (last access: 28 June 2023). MODIS level 3 data were downloaded from the NASA Giovanni interface. The analysis in this work was performed on the JASMIN super-data cluster (Lawrence et al., 2012). JASMIN is managed and delivered by the UK Science and Technology Facilities Council (STFC) Centre for Environmental Data Analysis (CEDA).
This research has been supported by the Natural Environment Research Council (grant no. NE/W004895/1), the National Science Foundation (grant nos. AGS-2153486 and CNH-171557), and the Norges Forskningsråd (grant nos. 324182 and 248834).
This paper was edited by Julia Hargreaves and reviewed by two anonymous referees.
Aamaas, B., Berntsen, T. K., Fuglestvedt, J. S., Shine, K. P., and Bellouin, N.: Regional emission metrics for short-lived climate forcers from multiple models, Atmos. Chem. Phys., 16, 7451–7468, https://doi.org/10.5194/acp-16-7451-2016, 2016. a
Acosta Navarro, J. C., Ekman, A. M. L., Pausata, F. S. R., Lewinschal, A., Varma, V., Seland, Ø., Gauss, M., Iversen, T., Kirkevåg, A., Riipinen, I., Hansson, H. C., Navarro, J. C. A., Ekman, A. M. L., Pausata, F. S. R., Lewinschal, A., Varma, V., Seland, Ø., Gauss, M., Iversen, T., Kirkevåg, A., Riipinen, I., and Hansson, H. C.: Future Response of Temperature and Precipitation to Reduced Aerosol Emissions as Compared with Increased Greenhouse Gas Concentrations, J. Climate, 30, 939–954, https://doi.org/10.1175/JCLI-D-16-0466.1, 2017. a
Albrecht, B. A.: Aerosols, Cloud Microphysics, and Fractional Cloudiness, Science, 245, 1227–1230, https://doi.org/10.1126/science.245.4923.1227, 1989. a
Allen, R. J.: A 21st century northward tropical precipitation shift caused by future anthropogenic aerosol reductions, J. Geophys. Res.-Atmos., 120, 9087–9102, https://doi.org/10.1002/2015JD023623, 2015. a
Allen, R. J. and Ajoku, O.: Future aerosol reductions and widening of the northern tropical belt, J. Geophys. Res.-Atmos., 121, 6765–6786, https://doi.org/10.1002/2016JD024803, 2016. a
Allen, R. J. and Zhao, X.: Anthropogenic aerosol impacts on Pacific Coast precipitation in CMIP6 models, Environ. Res., 1, 015005, https://doi.org/10.1088/2752-5295/ac7d68, 2022. a
Allen, R. J., Norris, J. R., and Kovilakam, M.: Influence of anthropogenic aerosols and the Pacific Decadal Oscillation on tropical belt width, Nat. Geosci., 7, 270–274, https://doi.org/10.1038/ngeo2091, 2014. a
Allen, R. J., Evan, A. T., and Booth, B. B. B.: Interhemispheric Aerosol Radiative Forcing and Tropical Precipitation Shifts during the Late Twentieth Century, J. Climate, 28, 8219–8246, https://doi.org/10.1175/JCLI-D-15-0148.1, 2015. a
Allen, R. J., Landuyt, W., and Rumbold, S. T.: An increase in aerosol burden and radiative effects in a warmer world, Nat. Clim. Change, 6, 269–274, https://doi.org/10.1038/nclimate2827, 2016. a, b
Allen, R. J., Amiri-Farahani, A., Lamarque, J.-F., Smith, C., Shindell, D., Hassan, T., and Chung, C. E.: Observationally constrained aerosol–cloud semi-direct effects, npj Clim. Atmos. Sci., 2, 16, https://doi.org/10.1038/s41612-019-0073-9, 2019a. a
Allen, R. J., Hassan, T., Randles, C. A., and Su, H.: Enhanced land–sea warming contrast elevates aerosol pollution in a warmer world, Nat. Clim. Change, 9, 300–305, https://doi.org/10.1038/s41558-019-0401-4, 2019b. a
Allen, R. J., Horowitz, L. W., Naik, V., Oshima, N., O'Connor, F. M., Turnock, S., Shim, S., Sager, P. L., van Noije, T., Tsigaridis, K., Bauer, S. E., Sentman, L. T., John, J. G., Broderick, C., Deushi, M., Folberth, G. A., Fujimori, S., and Collins, W. J.: Significant climate benefits from near-term climate forcer mitigation in spite of aerosol reductions, Environ. Res. Lett., 16, 034010, https://doi.org/10.1088/1748-9326/abe06b, 2021. a
Amiri-Farahani, A., Allen, R. J., Neubauer, D., and Lohmann, U.: Impact of Saharan dust on North Atlantic marine stratocumulus clouds: importance of the semidirect effect, Atmos. Chem. Phys., 17, 6305–6322, https://doi.org/10.5194/acp-17-6305-2017, 2017. a
Amiri-Farahani, A., Allen, R. J., Li, K.-F., Nabat, P., and Westervelt, D. M.: A La Niña-Like Climate Response to South African Biomass Burning Aerosol in CESM Simulations, J. Geophys. Res.-Atmos., 125, e2019JD031832, https://doi.org/10.1029/2019JD031832, e 2020. a
Bartlett, R. E., Bollasina, M. A., Booth, B. B. B., Dunstone, N. J., Marenco, F., Messori, G., and Bernie, D. J.: Do differences in future sulfate emission pathways matter for near-term climate? A case study for the Asian monsoon, Clim. Dynam., 50, 1863–1880, https://doi.org/10.1007/s00382-017-3726-6, 2018. a
Bauer, S. E., Koch, D., Unger, N., Metzger, S. M., Shindell, D. T., and Streets, D. G.: Nitrate aerosols today and in 2030: a global simulation including aerosols and tropospheric ozone, Atmos. Chem. Phys., 7, 5043–5059, https://doi.org/10.5194/acp-7-5043-2007, 2007. a
Bauer, S. E., Tsigaridis, K., and Miller, R.: Significant atmospheric aerosol pollution caused by world food cultivation, Geophys. Res. Lett., 43, 5394–5400, https://doi.org/10.1002/2016GL068354, 2016. a
Bellouin, N., Mann, G. W., Woodhouse, M. T., Johnson, C., Carslaw, K. S., and Dalvi, M.: Impact of the modal aerosol scheme GLOMAP-mode on aerosol forcing in the Hadley Centre Global Environmental Model, Atmos. Chem. Phys., 13, 3027–3044, https://doi.org/10.5194/acp-13-3027-2013, 2013. a
Bellouin, N., Quaas, J., Gryspeerdt, E., Kinne, S., Stier, P., Watson-Parris, D., Boucher, O., Carslaw, K. S., Christensen, M., Daniau, A.-L., Dufresne, J.-L., Feingold, G., Fiedler, S., Forster, P., Gettelman, A., Haywood, J. M., Lohmann, U., Malavelle, F., Mauritsen, T., McCoy, D. T., Myhre, G., Mülmenstädt, J., Neubauer, D., Possner, A., Rugenstein, M., Sato, Y., Schulz, M., Schwartz, S. E., Sourdeval, O., Storelvmo, T., Toll, V., Winker, D., and Stevens, B.: Bounding Global Aerosol Radiative Forcing of Climate Change, Rev. Geophys., 58, e2019RG000660, https://doi.org/10.1029/2019RG000660, 2020. a
Boucher, O., Randall, D., Artaxo, P., Bretherton, C., Feingold, G., Forster, P., Kerminen, V.-M., Kondo, Y., Liao, H., Lohmann, U., Rasch, P., Satheesh, S., Sherwood, S., Stevens, B., and Zhang, X.-Y.: Clouds and Aerosols. In: Climate Change 2013: The Physical Science Basis. Contribution of Working Group I to the Fifth Assessment Report of the Intergovernmental Panel on Climate Change, Climate Change 2013: The Physical Science Basis, Contribution of Working Group I to the Fifth Assessment Report of the Intergovernmental Panel on Climate Change, https://doi.org/10.1017/CBO9781107415324.016, 2013. a
Byrne, M. P. and Schneider, T.: Atmospheric Dynamics Feedback: Concept, Simulations, and Climate Implications, J. Climate, 31, 3249–3264, https://doi.org/10.1175/JCLI-D-17-0470.1, 2018. a
Chen, D., Rojas, M., Samset, B., Cobb, K., Niang, A. D., Edwards, P., Emori, S., Faria, S., Hawkins, E., Hope, P., Huybrechts, P., Meinshausen, M., Mustafa, S., Plattner, G.-K., and Tréguier, A.-M.: Framing, Context and Methods, in: Climate Change 2021: The Physical Science Basis, Contribution of Working Group I to the Sixth Assessment Report of the Intergovernmental Panel on Climate Change, Cambridge University Press, Cambridge, United Kingdom and New York, NY, USA, 147–286, https://doi.org/10.1017/9781009157896.003, 2021. a
Chen, W., Dong, B., Wilcox, L., Luo, F., Dunstone, N., and Highwood, E. J.: Attribution of Recent Trends in Temperature Extremes over China: Role of Changes in Anthropogenic Aerosol Emissions over Asia, J. Climate, 32, 7539–7560, https://doi.org/10.1175/JCLI-D-18-0777.1, 2019. a, b, c
Cherian, R. and Quaas, J.: Trends in AOD, Clouds, and Cloud Radiative Effects in Satellite Data and CMIP5 and CMIP6 Model Simulations Over Aerosol Source Regions, Geophys. Res. Lett., 47, e2020GL087132, https://doi.org/10.1029/2020GL087132, 2020. a
Collins, W. J., Lamarque, J.-F., Schulz, M., Boucher, O., Eyring, V., Hegglin, M. I., Maycock, A., Myhre, G., Prather, M., Shindell, D., and Smith, S. J.: AerChemMIP: quantifying the effects of chemistry and aerosols in CMIP6, Geosci. Model Dev., 10, 585–607, https://doi.org/10.5194/gmd-10-585-2017, 2017. a, b, c
Danabasoglu, G.: NCAR CESM2 model output prepared for CMIP6 RFMIP, Earth System Grid Federation [data set], https://doi.org/10.22033/ESGF/CMIP6.2199, 2019. a
Danabasoglu, G., Lawrence, D., Lindsay, K., Lipscomb, W., and Strand, G.: NCAR CESM2 model output prepared for CMIP6 CMIP piControl, Earth System Grid Federation [data set]m https://doi.org/10.22033/ESGF/CMIP6.7733, 2019. a
Danabasoglu, G., Lamarque, J.-F., Bacmeister, J., Bailey, D. A., DuVivier, A. K., Edwards, J., Emmons, L. K., Fasullo, J., Garcia, R., Gettelman, A., Hannay, C., Holland, M. M., Large, W. G., Lauritzen, P. H., Lawrence, D. M., Lenaerts, J. T. M., Lindsay, K., Lipscomb, W. H., Mills, M. J., Neale, R., Oleson, K. W., Otto-Bliesner, B., Phillips, A. S., Sacks, W., Tilmes, S., van Kampenhout, L., Vertenstein, M., Bertini, A., Dennis, J., Deser, C., Fischer, C., Fox-Kemper, B., Kay, J. E., Kinnison, D., Kushner, P. J., Larson, V. E., Long, M. C., Mickelson, S., Moore, J. K., Nienhouse, E., Polvani, L., Rasch, P. J., and Strand, W. G.: The Community Earth System Model Version 2 (CESM2), J. Adv. Model. Earth Sy., 12, e2019MS001916, https://doi.org/10.1029/2019MS001916, 2020. a, b, c
Dittus, A. J., Hawkins, E., Wilcox, L. J., Sutton, R. T., Smith, C. J., Andrews, M. B., and Forster, P. M.: Sensitivity of Historical Climate Simulations to Uncertain Aerosol Forcing, Geophys. Res. Lett., 47, e2019GL085806, https://doi.org/10.1029/2019GL085806, 2020. a, b
Dong, B., Sutton, R. T., Highwood, E., and Wilcox, L.: The Impacts of European and Asian Anthropogenic Sulfur Dioxide Emissions on Sahel Rainfall, J. Climate, 27, 7000–7017, https://doi.org/10.1175/JCLI-D-13-00769.1, 2014. a, b, c
Dong, B., Sutton, R., Highwood, E., and Wilcox, L.: Preferred response of the East Asian summer monsoon to local and non-local anthropogenic sulphur dioxide emissions, Clim. Dynam., 46, 1733–1751, https://doi.org/10.1007/s00382-015-2671-5, 2016. a, b, c
Dong, B., Wilcox, L. J., Highwood, E. J., and Sutton, R. T.: Impacts of recent decadal changes in Asian aerosols on the East Asian summer monsoon: roles of aerosol–radiation and aerosol–cloud interactions, Clim. Dynam., 53, 3235–3256, https://doi.org/10.1007/s00382-019-04698-0, 2019. a
Ekman, A. M. L.: Do sophisticated parameterizations of aerosol-cloud interactions in CMIP5 models improve the representation of recent observed temperature trends?, J. Geophys. Res.-Atmos., 119, 817–832, https://doi.org/10.1002/2013JD020511, 2014. a, b
Eyring, V., Bony, S., Meehl, G. A., Senior, C. A., Stevens, B., Stouffer, R. J., and Taylor, K. E.: Overview of the Coupled Model Intercomparison Project Phase 6 (CMIP6) experimental design and organization, Geosci. Model Dev., 9, 1937–1958, https://doi.org/10.5194/gmd-9-1937-2016, 2016. a, b, c, d, e
Fan, J., Wang, Y., Rosenfeld, D., and Liu, X.: Review of Aerosol–Cloud Interactions: Mechanisms, Significance, and Challenges, J. Atmos. Sci., 73, 4221–4252, https://doi.org/10.1175/JAS-D-16-0037.1, 2016. a, b
Fanourgakis, G. S., Kanakidou, M., Nenes, A., Bauer, S. E., Bergman, T., Carslaw, K. S., Grini, A., Hamilton, D. S., Johnson, J. S., Karydis, V. A., Kirkevåg, A., Kodros, J. K., Lohmann, U., Luo, G., Makkonen, R., Matsui, H., Neubauer, D., Pierce, J. R., Schmale, J., Stier, P., Tsigaridis, K., van Noije, T., Wang, H., Watson-Parris, D., Westervelt, D. M., Yang, Y., Yoshioka, M., Daskalakis, N., Decesari, S., Gysel-Beer, M., Kalivitis, N., Liu, X., Mahowald, N. M., Myriokefalitakis, S., Schrödner, R., Sfakianaki, M., Tsimpidi, A. P., Wu, M., and Yu, F.: Evaluation of global simulations of aerosol particle and cloud condensation nuclei number, with implications for cloud droplet formation, Atmos. Chem. Phys., 19, 8591–8617, https://doi.org/10.5194/acp-19-8591-2019, 2019. a, b, c, d, e
Fiedler, S. and Putrasahan, D.: How Does the North Atlantic SST Pattern Respond to Anthropogenic Aerosols in the 1970s and 2000s?, Geophys. Res. Lett., 48, e2020GL092142, https://doi.org/10.1029/2020GL092142, 2021. a, b
Forster, P., Storelvmo, T., Armour, K., Collins, W., Dufresne, J. L., Frame, D., Lunt, D. J., Mauritsen, T., Palmer, M. D., Watanabe, M., Wild, M., and Zhang, H.: The Earth's Energy Budget, Climate Feedbacks, and Climate Sensitivity, in: Climate Change 2021: The Physical Science Basis, Contribution of Working Group I to the Sixth Assessment Report of the Intergovernmental Panel on Climate Change, Cambridge University Press, https://doi.org/10.1017/9781009157896.009, 2021. a, b, c, d, e
Ghan, S. J.: Technical Note: Estimating aerosol effects on cloud radiative forcing, Atmos. Chem. Phys., 13, 9971–9974, https://doi.org/10.5194/acp-13-9971-2013, 2013. a, b
Gidden, M. J., Riahi, K., Smith, S. J., Fujimori, S., Luderer, G., Kriegler, E., van Vuuren, D. P., van den Berg, M., Feng, L., Klein, D., Calvin, K., Doelman, J. C., Frank, S., Fricko, O., Harmsen, M., Hasegawa, T., Havlik, P., Hilaire, J., Hoesly, R., Horing, J., Popp, A., Stehfest, E., and Takahashi, K.: Global emissions pathways under different socioeconomic scenarios for use in CMIP6: a dataset of harmonized emissions trajectories through the end of the century, Geosci. Model Dev., 12, 1443–1475, https://doi.org/10.5194/gmd-12-1443-2019, 2019. a
Guo, H., John, J. G., Blanton, C., McHugh, C., Nikonov, S., Radhakrishnan, A., Rand, K., Zadeh, N. T., Balaji, V., Durachta, J., Dupuis, C., Menzel, R., Robinson, T., Underwood, S., Vahlenkamp, H., Bushuk, M., Dunne, K. A., Dussin, R., Gauthier, P. P., Ginoux, P., Griffies, S. M., Hallberg, R., Harrison, M., Hurlin, W., Lin, P., Malyshev, S., Naik, V., Paulot, F., Paynter, D. J., Ploshay, J., Reichl, B. G., Schwarzkopf, D. M., Seman, C. J., Shao, A., Silvers, L., Wyman, B., Yan, X., Zeng, Y., Adcroft, A., Dunne, J. P., Held, I. M., Krasting, J. P., Horowitz, L. W., Milly, P., Shevliakova, E., Winton, M., Zhao, M., and Zhang, R.: NOAA-GFDL GFDL-CM4 model output piControl, Earth System Grid Federation [data set], https://doi.org/10.22033/ESGF/CMIP6.8666, 2018. a
Guo, L., Wilcox, L. J., Bollasina, M., Turnock, S. T., Lund, M. T., and Zhang, L.: Competing effects of aerosol reductions and circulation changes for future improvements in Beijing haze, Atmos. Chem. Phys., 21, 15299–15308, https://doi.org/10.5194/acp-21-15299-2021, 2021. a
Hari, V., Villarini, G., Karmakar, S., Wilcox, L. J., and Collins, M.: Northward Propagation of the Intertropical Convergence Zone and Strengthening of Indian Summer Monsoon Rainfall, Geophys. Res. Lett., 47, e2020GL089823, https://doi.org/10.1029/2020GL089823, 2020. a, b
Hassan, T., Allen, R. J., Liu, W., and Randles, C. A.: Anthropogenic aerosol forcing of the Atlantic meridional overturning circulation and the associated mechanisms in CMIP6 models, Atmos. Chem. Phys., 21, 5821–5846, https://doi.org/10.5194/acp-21-5821-2021, 2021. a
Hassan, T., Allen, R. J., Liu, W., Shim, S., van Noije, T., Le Sager, P., Oshima, N., Deushi, M., Randles, C. A., and O'Connor, F. M.: Air quality improvements are projected to weaken the Atlantic meridional overturning circulation through radiative forcing effects, Commun. Earth Environ., 3, 149, https://doi.org/10.1038/s43247-022-00476-9, 2022. a
Held, I. M., Guo, H., Adcroft, A., Dunne, J. P., Horowitz, L. W., Krasting, J., Shevliakova, E., Winton, M., Zhao, M., Bushuk, M., Wittenberg, A. T., Wyman, B., Xiang, B., Zhang, R., Anderson, W., Balaji, V., Donner, L., Dunne, K., Durachta, J., Gauthier, P. P. G., Ginoux, P., Golaz, J.-C., Griffies, S. M., Hallberg, R., Harris, L., Harrison, M., Hurlin, W., John, J., Lin, P., Lin, S.-J., Malyshev, S., Menzel, R., Milly, P. C. D., Ming, Y., Naik, V., Paynter, D., Paulot, F., Rammaswamy, V., Reichl, B., Robinson, T., Rosati, A., Seman, C., Silvers, L. G., Underwood, S., and Zadeh, N.: Structure and Performance of GFDL's CM4.0 Climate Model, J. Adv. Model. Earth Sy., 11, 3691–3727, https://doi.org/10.1029/2019MS001829, 2019. a, b, c
Herbert, R., Wilcox, L. J., Joshi, M., Highwood, E., and Frame, D.: Nonlinear response of Asian summer monsoon precipitation to emission reductions in South and East Asia, Environ. Res. Lett., 17, 014005, https://doi.org/10.1088/1748-9326/ac3b19, 2021. a
Hersbach, H., Bell, B., Berrisford, P., Hirahara, S., Horányi, A., Muñoz-Sabater, J., Nicolas, J., Peubey, C., Radu, R., Schepers, D., Simmons, A., Soci, C., Abdalla, S., Abellan, X., Balsamo, G., Bechtold, P., Biavati, G., Bidlot, J., Bonavita, M., De Chiara, G., Dahlgren, P., Dee, D., Diamantakis, M., Dragani, R., Flemming, J., Forbes, R., Fuentes, M., Geer, A., Haimberger, L., Healy, S., Hogan, R. J., Hólm, E., Janisková, M., Keeley, S., Laloyaux, P., Lopez, P., Lupu, C., Radnoti, G., de Rosnay, P., Rozum, I., Vamborg, F., Villaume, S., and Thépaut, J.-N.: The ERA5 global reanalysis, Q. J. Roy. Meteor. Soc., 146, 1999–2049, https://doi.org/10.1002/qj.3803, 2020. a
Hickman, J. E., Andela, N., Tsigaridis, K., Galy-Lacaux, C., Ossohou, M., and Bauer, S. E.: Reductions in NO2 burden over north equatorial Africa from decline in biomass burning in spite of growing fossil fuel use, 2005 to 2017, P. Natl. Acad. Sci. USA, 118, e2002579118, https://doi.org/10.1073/pnas.2002579118, 2021. a
Hoesly, R. M., Smith, S. J., Feng, L., Klimont, Z., Janssens-Maenhout, G., Pitkanen, T., Seibert, J. J., Vu, L., Andres, R. J., Bolt, R. M., Bond, T. C., Dawidowski, L., Kholod, N., Kurokawa, J.-I., Li, M., Liu, L., Lu, Z., Moura, M. C. P., O'Rourke, P. R., and Zhang, Q.: Historical (1750–2014) anthropogenic emissions of reactive gases and aerosols from the Community Emissions Data System (CEDS), Geosci. Model Dev., 11, 369–408, https://doi.org/10.5194/gmd-11-369-2018, 2018. a, b
Kasoar, M., Shawki, D., and Voulgarakis, A.: Similar spatial patterns of global climate response to aerosols from different regions, npj Clim. Atmos. Sci., 1, 12, https://doi.org/10.1038/s41612-018-0022-z, 2018. a
Kirkevåg, A., Grini, A., Olivié, D., Seland, Ø., Alterskjær, K., Hummel, M., Karset, I. H. H., Lewinschal, A., Liu, X., Makkonen, R., Bethke, I., Griesfeller, J., Schulz, M., and Iversen, T.: A production-tagged aerosol module for Earth system models, OsloAero5.3 – extensions and updates for CAM5.3-Oslo, Geosci. Model Dev., 11, 3945–3982, https://doi.org/10.5194/gmd-11-3945-2018, 2018. a
Kok, J. F., Ward, D. S., Mahowald, N. M., and Evan, A. T.: Global and regional importance of the direct dust-climate feedback, Nat. Commun., 9, 241, https://doi.org/10.1038/s41467-017-02620-y, 2018. a
Krishnan, S., Ekman, A. M. L., Hansson, H.-C., Riipinen, I., Lewinschal, A., Wilcox, L. J., and Dallafior, T.: The Roles of the Atmosphere and Ocean in Driving Arctic Warming Due to European Aerosol Reductions, Geophys. Res. Lett., 47, e2019GL086681, https://doi.org/10.1029/2019GL086681, 2020. a
Lawrence, B., Bennett, V. L., Churchill, J., Juckes, M., Kershaw, P., Oliver, P., Pritchard, M., and Stephens, A.: The JASMIN super-data-cluster, arXiv [preprint], https://doi.org/10.48550/arXiv.1204.3553, 2012. a
Levy, H., Horowitz, L. W., Schwarzkopf, M. D., Ming, Y., Golaz, J.-C., Naik, V., and Ramaswamy, V.: The roles of aerosol direct and indirect effects in past and future climate change, J. Geophys. Res.-Atmos., 118, 4521–4532, https://doi.org/10.1002/jgrd.50192, 2013. a
Li, J., Carlson, B. E., Yung, Y. L., Lv, D., Hansen, J., Penner, J. E., Liao, H., Ramaswamy, V., Kahn, R. A., Zhang, P., Dubovik, O., Ding, A., Lacis, A. A., Zhang, L., and Dong, Y.: Scattering and absorbing aerosols in the climate system, Nature Reviews Earth & Environment, 3, 363–379, https://doi.org/10.1038/s43017-022-00296-7, 2022. a
Li, Z., Lau, W. K., Ramanathan, V., Wu, G., Ding, Y., Manoj, M. G., Liu, J., Qian, Y., Li, J., Zhou, T., Fan, J., Rosenfeld, D., Ming, Y., Wang, Y., Huang, J., Wang, B., Xu, X., Lee, S., Cribb, M., Zhang, F., Yang, X., Zhao, C., Takemura, T., Wang, K., Xia, X., Yin, Y., Zhang, H., Guo, J., Zhai, P. M., Sugimoto, N., Babu, S. S., and Brasseur, G. P.: Aerosol and monsoon climate interactions over Asia, Rev. Geophys., 54, 866–929, https://doi.org/10.1002/2015RG000500, 2016. a
Liu, L., Shawki, D., Voulgarakis, A., Kasoar, M., Samset, B. H., Myhre, G., Forster, P. M., Hodnebrog, Ø., Sillmann, J., Aalbergsjø, S. G., Boucher, O., Faluvegi, G., Iversen, T., Kirkevåg, A., Lamarque, J.-F., Olivié, D., Richardson, T., Shindell, D., Takemura, T., Liu, L., Shawki, D., Voulgarakis, A., Kasoar, M., Samset, B. H., Myhre, G., Forster, P. M., Hodnebrog, Ø., Sillmann, J., Aalbergsjø, S. G., Boucher, O., Faluvegi, G., Iversen, T., Kirkevåg, A., Lamarque, J.-F., Olivié, D., Richardson, T., Shindell, D., and Takemura, T.: A PDRMIP Multimodel Study on the Impacts of Regional Aerosol Forcings on Global and Regional Precipitation, J. Climate, 31, 4429–4447, https://doi.org/10.1175/JCLI-D-17-0439.1, 2018. a, b
Lund, M. T., Myhre, G., and Samset, B. H.: Anthropogenic aerosol forcing under the Shared Socioeconomic Pathways, Atmos. Chem. Phys., 19, 13827–13839, https://doi.org/10.5194/acp-19-13827-2019, 2019. a, b, c, d
Luo, F., Wilcox, L., Dong, B., Su, Q., Chen, W., Dunstone, N., Li, S., and Gao, Y.: Projected near-term changes of temperature extremes in Europe and China under different aerosol emissions, Environ. Res. Lett., 15, 034013, https://doi.org/10.1088/1748-9326/ab6b34, 2020. a, b, c, d
Ma, X., Liu, W., Allen, R. J., Huang, G., and Li, X.: Dependence of regional ocean heat uptake on anthropogenic warming scenarios, Sci. Adv., 6, eabc0303, https://doi.org/10.1126/sciadv.abc0303, 2020. a
Masson-Delmotte, V., Zhai, P., Pirani, A., Connors, S., Péan, C., Berger, S., Caud, N., Chen, Y., Goldfarb, L., Gomis, M., Huang, M., Leitzell, K., Lonnoy, E., Matthews, J., Maycock, T., Waterfield, T., Yelekçi, O., Yu, R., and Zhou, B. (Eds.): IPCC, 2021: Summary for Policymakers, in: Climate Change 2021: The Physical Science Basis. Contribution of Working Group I to the Sixth Assessment Report of the Intergovernmental Panel on Climate Change, Cambridge University Press, https://doi.org/10.1017/9781009157896.001, 2021. a
Menary, M. B., Robson, J., Allan, R. P., Booth, B. B. B., Cassou, C., Gastineau, G., Gregory, J., Hodson, D., Jones, C., Mignot, J., Ringer, M., Sutton, R., Wilcox, L., and Zhang, R.: Aerosol-Forced AMOC Changes in CMIP6 Historical Simulations, Geophys. Res. Lett., 47, e2020GL088166, https://doi.org/10.1029/2020GL088166, 2020. a, b
Merikanto, J., Nordling, K., Räisänen, P., Räisänen, J., O'Donnell, D., Partanen, A.-I., and Korhonen, H.: How Asian aerosols impact regional surface temperatures across the globe, Atmos. Chem. Phys., 21, 5865–5881, https://doi.org/10.5194/acp-21-5865-2021, 2021. a
Ming, Y., Ramaswamy, V., and Chen, G.: A Model Investigation of Aerosol-Induced Changes in Boreal Winter Extratropical Circulation, J. Climate, 24, 6077–6091, https://doi.org/10.1175/2011JCLI4111.1, 2011. a
Monerie, P.-A., Wilcox, L. J., and Turner, A. G.: Effects of Anthropogenic Aerosol and Greenhouse Gas Emissions on Northern Hemisphere Monsoon Precipitation: Mechanisms and Uncertainty, J. Climate, 35, 2305–2326, https://doi.org/10.1175/JCLI-D-21-0412.1, 2022. a, b
Mulcahy, J. P., Jones, C., Sellar, A., Johnson, B., Boutle, I. A., Jones, A., Andrews, T., Rumbold, S. T., Mollard, J., Bellouin, N., Johnson, C. E., Williams, K. D., Grosvenor, D. P., and McCoy, D. T.: Improved Aerosol Processes and Effective Radiative Forcing in HadGEM3 and UKESM1, J. Adv. Model. Earth Sy., 10, 2786–2805, https://doi.org/10.1029/2018MS001464, 2018. a
Mülmenstädt, J. and Wilcox, L. J.: The Fall and Rise of the Global Climate Model, J. Adv. Model. Earth Sy., 13, e2021MS002781, https://doi.org/10.1029/2021MS002781, 2021. a
Myhre, G., Shindell, D., Bréon, F.-M., Collins, W., Fuglestvedt, J., Huang, J., Koch, D., Lamarque, J.-F., Lee, D., Mendoza, B., Nakajima, T., Robock, A., Stephens, G., Takemura, T., and Zhan, H.: Anthropogenic and Natural Radiative Forcing, in: Climate Change 2013: The Physical Science Basis, Contribution of Working Group I to the Fifth Assessment Report of the Intergovernmental Panel on Climate Change, Cambridge University Press, Cambridge, United Kingdom and New York, NY, USA, https://doi.org/10.1017/CBO9781107415324.018, 2013. a, b
Myhre, G., Aas, W., Cherian, R., Collins, W., Faluvegi, G., Flanner, M., Forster, P., Hodnebrog, Ø., Klimont, Z., Lund, M. T., Mülmenstädt, J., Lund Myhre, C., Olivié, D., Prather, M., Quaas, J., Samset, B. H., Schnell, J. L., Schulz, M., Shindell, D., Skeie, R. B., Takemura, T., and Tsyro, S.: Multi-model simulations of aerosol and ozone radiative forcing due to anthropogenic emission changes during the period 1990–2015, Atmos. Chem. Phys., 17, 2709–2720, https://doi.org/10.5194/acp-17-2709-2017, 2017a. a
Myhre, G., Forster, P. M., Samset, B. H., Hodnebrog, Ø., Sillmann, J., Aalbergsjø, S. G., Andrews, T., Boucher, O., Faluvegi, G., Fläschner, D., Iversen, T., Kasoar, M., Kharin, V., Kirkevåg, A., Lamarque, J.-F., Olivié, D., Richardson, T. B., Shindell, D., Shine, K. P., Stjern, C. W., Takemura, T., Voulgarakis, A., and Zwiers, F.: PDRMIP: A Precipitation Driver and Response Model Intercomparison Project – Protocol and Preliminary Results, B. Am. Meteorol. Soc., 98, 1185–1198, https://doi.org/10.1175/BAMS-D-16-0019.1, 2017b. a, b, c
Nordling, K., Korhonen, H., Räisänen, P., Alper, M. E., Uotila, P., O'Donnell, D., and Merikanto, J.: Role of climate model dynamics in estimated climate responses to anthropogenic aerosols, Atmos. Chem. Phys., 19, 9969–9987, https://doi.org/10.5194/acp-19-9969-2019, 2019. a
O'Connor, F., Dalvi, M., Kahana, R., Johnson, B., Mulcahy, J., Robertson, E., Shim, S., and Wiltshire, A.: MOHC UKESM1.0-LL model output prepared for CMIP6 RFMIP, Earth System Grid Federation [data set], https://doi.org/10.22033/ESGF/CMIP6.11061, 2019. a
O'Neill, B. C., Tebaldi, C., van Vuuren, D. P., Eyring, V., Friedlingstein, P., Hurtt, G., Knutti, R., Kriegler, E., Lamarque, J.-F., Lowe, J., Meehl, G. A., Moss, R., Riahi, K., and Sanderson, B. M.: The Scenario Model Intercomparison Project (ScenarioMIP) for CMIP6, Geosci. Model Dev., 9, 3461–3482, https://doi.org/10.5194/gmd-9-3461-2016, 2016. a, b, c
Paynter, D. J., Menzel, R., Jones, A., Schwarzkopf, D. M., Freidenreich, S., Wyman, B., Blanton, C., McHugh, C., Radhakrishnan, A., Vahlenkamp, H., Rand, K., Silvers, L., Guo, H., John, J. G., Ploshay, J., Balaji, V., and Wilson, C.: NOAA-GFDL GFDL-CM4 model output prepared for CMIP6 RFMIP, Earth System Grid Federation [data set], https://doi.org/10.22033/ESGF/CMIP6.1643, 2018. a
Persad, G. G.: The dependence of aerosols' global and local precipitation impacts on the emitting region, Atmos. Chem. Phys., 23, 3435–3452, https://doi.org/10.5194/acp-23-3435-2023, 2023. a, b
Persad, G. G. and Caldeira, K.: Divergent global-scale temperature effects from identical aerosols emitted in different regions, Nat. Commun., 9, 3289, https://doi.org/10.1038/s41467-018-05838-6, 2018. a
Pincus, R., Forster, P. M., and Stevens, B.: The Radiative Forcing Model Intercomparison Project (RFMIP): experimental protocol for CMIP6, Geosci. Model Dev., 9, 3447–3460, https://doi.org/10.5194/gmd-9-3447-2016, 2016. a, b, c
Platnick, S.: MODIS Atmosphere L3 Monthly Product, NASA MODIS Adaptive Processing System, Goddard Space Flight Center [data set], https://doi.org/10.5067/MODIS/MYD08_M3.006, 2015. a
Polson, D., Bollasina, M., Hegerl, G. C., and Wilcox, L. J.: Decreased monsoon precipitation in the Northern Hemisphere due to anthropogenic aerosols, Geophys. Res. Lett., 41, 6023–6029, https://doi.org/10.1002/2014GL060811, 2014. a
Quaas, J., Jia, H., Smith, C., Albright, A. L., Aas, W., Bellouin, N., Boucher, O., Doutriaux-Boucher, M., Forster, P. M., Grosvenor, D., Jenkins, S., Klimont, Z., Loeb, N. G., Ma, X., Naik, V., Paulot, F., Stier, P., Wild, M., Myhre, G., and Schulz, M.: Robust evidence for reversal of the trend in aerosol effective climate forcing, Atmos. Chem. Phys., 22, 12221–12239, https://doi.org/10.5194/acp-22-12221-2022, 2022. a
Ranasinghe, R., Ruane, A., Vautard, R., Arnell, N., Coppola, E., Cruz, F., Dessai, S., Islam, A., Rahimi, M., Carrascal, D. R., Sillmann, J., Sylla, M., Tebaldi, C., Wang, W., and Zaaboul, R.: Climate Change Information for Regional Impact and for Risk Assessment, in: Climate Change 2021: The Physical Science Basis, Contribution of Working Group I to the Sixth Assessment Report of the Intergovernmental Panel on Climate Change, Cambridge University Press, Cambridge, United Kingdom and New York, NY, USA, 1767–1926, https://doi.org/10.1017/9781009157896.014, 2021. a
Rao, S., Klimont, Z., Smith, S. J., Van Dingenen, R., Dentener, F., Bouwman, L., Riahi, K., Amann, M., Bodirsky, B. L., van Vuuren, D. P., Aleluia Reis, L., Calvin, K., Drouet, L., Fricko, O., Fujimori, S., Gernaat, D., Havlik, P., Harmsen, M., Hasegawa, T., Heyes, C., Hilaire, J., Luderer, G., Masui, T., Stehfest, E., Strefler, J., van der Sluis, S., and Tavoni, M.: Future air pollution in the Shared Socio-economic Pathways, Global Environ. Chang., 42, 346–358, https://doi.org/10.1016/j.gloenvcha.2016.05.012, 2017. a, b, c
Riahi, K., van Vuuren, D. P., Kriegler, E., Edmonds, J., O'Neill, B. C., Fujimori, S., Bauer, N., Calvin, K., Dellink, R., Fricko, O., Lutz, W., Popp, A., Cuaresma, J. C., KC, S., Leimbach, M., Jiang, L., Kram, T., Rao, S., Emmerling, J., Ebi, K., Hasegawa, T., Havlik, P., Humpenöder, F., Da Silva, L. A., Smith, S., Stehfest, E., Bosetti, V., Eom, J., Gernaat, D., Masui, T., Rogelj, J., Strefler, J., Drouet, L., Krey, V., Luderer, G., Harmsen, M., Takahashi, K., Baumstark, L., Doelman, J. C., Kainuma, M., Klimont, Z., Marangoni, G., Lotze-Campen, H., Obersteiner, M., Tabeau, A., and Tavoni, M.: The Shared Socioeconomic Pathways and their energy, land use, and greenhouse gas emissions implications: An overview, Global Environ. Chang., 42, 153–168, https://doi.org/10.1016/J.GLOENVCHA.2016.05.009, 2017. a, b
Richardson, T. B., Forster, P. M., Andrews, T., Boucher, O., Faluvegi, G., Fläschner, D., Hodnebrog, Ø., Kasoar, M., Kirkevåg, A., Lamarque, J.-F., Myhre, G., Olivié, D., Samset, B. H., Shawki, D., Shindell, D., Takemura, T., Voulgarakis, A., Richardson, T. B., Forster, P. M., Andrews, T., Boucher, O., Faluvegi, G., Fläschner, D., Hodnebrog, Ø., Kasoar, M., Kirkevåg, A., Lamarque, J.-F., Myhre, G., Olivié, D., Samset, B. H., Shawki, D., Shindell, D., Takemura, T., and Voulgarakis, A.: Drivers of Precipitation Change: An Energetic Understanding, J. Climate, 31, 9641–9657, https://doi.org/10.1175/JCLI-D-17-0240.1, 2018. a
Robson, J., Menary, M. B., Sutton, R. T., Mecking, J., Gregory, J. M., Jones, C., Sinha, B., Stevens, D. P., and Wilcox, L. J.: The role of anthropogenic aerosol forcing in the 1850–1985 strengthening of the AMOC in CMIP6 historical simulations, J. Climate, 35, 6843–6863, https://doi.org/10.1175/JCLI-D-22-0124.1, 2022. a
Rodgers, K. B., Lee, S.-S., Rosenbloom, N., Timmermann, A., Danabasoglu, G., Deser, C., Edwards, J., Kim, J.-E., Simpson, I. R., Stein, K., Stuecker, M. F., Yamaguchi, R., Bódai, T., Chung, E.-S., Huang, L., Kim, W. M., Lamarque, J.-F., Lombardozzi, D. L., Wieder, W. R., and Yeager, S. G.: Ubiquity of human-induced changes in climate variability, Earth Syst. Dynam., 12, 1393–1411, https://doi.org/10.5194/esd-12-1393-2021, 2021. a
Samset, B. H., Myhre, G., Forster, P. M., Hodnebrog, Ø., Andrews, T., Faluvegi, G., Fläschner, D., Kasoar, M., Kharin, V., Kirkevåg, A., Lamarque, J., Olivié, D., Richardson, T., Shindell, D., Shine, K. P., Takemura, T., and Voulgarakis, A.: Fast and slow precipitation responses to individual climate forcers: A PDRMIP multimodel study, Geophys. Res. Lett., 43, 2782–2791, https://doi.org/10.1002/2016GL068064, 2016. a
Samset, B. H., Myhre, G., Forster, P. M., Hodnebrog, Ø., Andrews, T., Boucher, O., Faluvegi, G., Fläschner, D., Kasoar, M., Kharin, V., Kirkevåg, A., Lamarque, J.-F., Olivié, D., Richardson, T. B., Shindell, D., Takemura, T., and Voulgarakis, A.: Weak hydrological sensitivity to temperature change over land, independent of climate forcing, npj Clim. Atmos. Sci., 1, 20173, https://doi.org/10.1038/s41612-017-0005-5, 2018a. a
Samset, B. H., Sand, M., Smith, C. J., Bauer, S. E., Forster, P. M., Fuglestvedt, J. S., Osprey, S., and Schleussner, C.-F.: Climate Impacts From a Removal of Anthropogenic Aerosol Emissions, Geophys. Res. Lett., 45, 1020–1029, https://doi.org/10.1002/2017GL076079, 2018b. a, b
Samset, B. H., Stjern, C. W., Andrews, E., Kahn, R. A., Myhre, G., Schulz, M., and Schuster, G. L.: Aerosol Absorption: Progress Towards Global and Regional Constraints, Curr. Clim. Change Rep., 4, 65–83, https://doi.org/10.1007/s40641-018-0091-4, 2018c. a
Samset, B. H., Lund, M. T., Bollasina, M., Myhre, G., and Wilcox, L.: Emerging Asian aerosol patterns, Nat. Geosci., 12, 582–584, https://doi.org/10.1038/s41561-019-0424-5, 2019. a, b, c, d
Sand, M., Samset, B. H., Tsigaridis, K., Bauer, S. E., and Myhre, G.: Black Carbon and Precipitation: An Energetics Perspective, J. Geophys. Res.-Atmos., 125, e2019JD032239, https://doi.org/10.1029/2019JD032239, 2020. a
Scannell, C., Booth, B. B. B., Dunstone, N. J., Rowell, D. P., Bernie, D. J., Kasoar, M., Voulgarakis, A., Wilcox, L. J., Acosta Navarro, J. C., Seland, Ø., and Paynter, D. J.: The Influence of Remote Aerosol Forcing from Industrialized Economies on the Future Evolution of East and West African Rainfall, J. Climate, 32, 8335–8354, https://doi.org/10.1175/JCLI-D-18-0716.1, 2019. a, b
Sellar, A. A., Jones, C. G., Mulcahy, J. P., Tang, Y., Yool, A., Wiltshire, A., O'Connor, F. M., Stringer, M., Hill, R., Palmieri, J., Woodward, S., de Mora, L., Kuhlbrodt, T., Rumbold, S. T., Kelley, D. I., Ellis, R., Johnson, C. E., Walton, J., Abraham, N. L., Andrews, M. B., Andrews, T., Archibald, A. T., Berthou, S., Burke, E., Blockley, E., Carslaw, K., Dalvi, M., Edwards, J., Folberth, G. A., Gedney, N., Griffiths, P. T., Harper, A. B., Hendry, M. A., Hewitt, A. J., Johnson, B., Jones, A., Jones, C. D., Keeble, J., Liddicoat, S., Morgenstern, O., Parker, R. J., Predoi, V., Robertson, E., Siahaan, A., Smith, R. S., Swaminathan, R., Woodhouse, M. T., Zeng, G., and Zerroukat, M.: UKESM1: Description and Evaluation of the U.K. Earth System Model, J. Adv. Model. Earth Sy., 11, 4513–4558, https://doi.org/10.1029/2019MS001739, 2019. a, b, c
Shindell, D. T., Faluvegi, G., Rotstayn, L., and Milly, G.: Spatial patterns of radiative forcing and surface temperature response, J. Geophys. Res.-Atmos., 120, 5385–5403, https://doi.org/10.1002/2014JD022752, 2015. a
Shonk, J. K. P., Turner, A. G., Chevuturi, A., Wilcox, L. J., Dittus, A. J., and Hawkins, E.: Uncertainty in aerosol radiative forcing impacts the simulated global monsoon in the 20th century, Atmos. Chem. Phys., 20, 14903–14915, https://doi.org/10.5194/acp-20-14903-2020, 2020. a
Sillmann, J., Stjern, C. W., Myhre, G., Samset, B. H., Hodnebrog, Ø., Andrews, T., Boucher, O., Faluvegi, G., Forster, P., Kasoar, M. R., Kharin, V. V., Kirkevåg, A., Lamarque, J.-F., Olivié, D. J. L., Richardson, T. B., Shindell, D., Takemura, T., Voulgarakis, A., and Zwiers, F. W.: Extreme wet and dry conditions affected differently by greenhouse gases and aerosols, npj Clim. Atmos. Sci., 2, 24, https://doi.org/10.1038/s41612-019-0079-3, 2019. a, b
Smith, D. M., Booth, B. B. B., Dunstone, N. J., Eade, R., Hermanson, L., Jones, G. S., Scaife, A. A., Sheen, K. L., and Thompson, V.: Role of volcanic and anthropogenic aerosols in the recent global surface warming slowdown, Nat. Clim. Change, 6, 936–940, https://doi.org/10.1038/nclimate3058, 2016. a
Song, F., Zhou, T., and Qian, Y.: Responses of East Asian summer monsoon to natural and anthropogenic forcings in the 17 latest CMIP5 models, Geophys. Res. Lett., 41, 596–603, https://doi.org/10.1002/2013GL058705, 2014. a
Sperber, K. R., Annamalai, H., Kang, I.-S., Kitoh, A., Moise, A., Turner, A., Wang, B., and Zhou, T.: The Asian summer monsoon: an intercomparison of CMIP5 vs. CMIP3 simulations of the late 20th century, Clim. Dynam., 41, 2711–2744, https://doi.org/10.1007/s00382-012-1607-6, 2013. a
Stevens, B. and Feingold, G.: Untangling aerosol effects on clouds and precipitation in a buffered system, Nature, 461, 607–613, https://doi.org/10.1038/nature08281, 2009. a
Stjern, C. W., Samset, B. H., Myhre, G., Forster, P. M., Hodnebrog, Ø., Andrews, T., Boucher, O., Faluvegi, G., Iversen, T., Kasoar, M., Kharin, V., Kirkevåg, A., Lamarque, J.-F., Olivié, D., Richardson, T., Shawki, D., Shindell, D., Smith, C. J., Takemura, T., and Voulgarakis, A.: Rapid Adjustments Cause Weak Surface Temperature Response to Increased Black Carbon Concentrations, J. Geophys. Res.-Atmos., 122, 11462–11481, https://doi.org/10.1002/2017JD027326, 2017. a
Szopa, S., Naik, V., Adhikary, B., Artaxo, P., Berntsen, T., Collins, W., Fuzzi, S., Gallardo, L., Kiendler-Scharr, A., Klimont, Z., Liao, H., Unger, N., and Zanis, P.: Short-Lived Climate Forcers, in: Climate Change 2021: The Physical Science Basis, Contribution of Working Group I to the Sixth Assessment Report of the Intergovernmental Panel on Climate Change, Cambridge University Press, Cambridge, United Kingdom and New York, NY, USA, 817–922, https://doi.org/10.1017/9781009157896.008, 2021. a, b, c
Tang, T., Shindell, D., Samset, B. H., Boucher, O., Forster, P. M., Hodnebrog, Ø., Myhre, G., Sillmann, J., Voulgarakis, A., Andrews, T., Faluvegi, G., Fläschner, D., Iversen, T., Kasoar, M., Kharin, V., Kirkevåg, A., Lamarque, J.-F., Olivié, D., Richardson, T., Stjern, C. W., and Takemura, T.: Dynamical response of Mediterranean precipitation to greenhouse gases and aerosols, Atmos. Chem. Phys., 18, 8439–8452, https://doi.org/10.5194/acp-18-8439-2018, 2018. a
Tang, Y., Rumbold, S., Ellis, R., Kelley, D., Mulcahy, J., Sellar, A., Walton, J., and Jones, C.: MOHC UKESM1.0-LL model output prepared for CMIP6 CMIP piControl, Earth System Grid Federation [data set], https://doi.org/10.22033/ESGF/CMIP6.6298, 2019. a
Tegen, I. and Schepanski, K.: Climate Feedback on Aerosol Emission and Atmospheric Concentrations, Curr. Clim. Change Rep., 4, 1–10, https://doi.org/10.1007/s40641-018-0086-1, 2018. a
Terai, C. R., Pritchard, M. S., Blossey, P., and Bretherton, C. S.: The Impact of Resolving Subkilometer Processes on Aerosol-Cloud Interactions of Low-Level Clouds in Global Model Simulations, J. Adv. Model. Earth Sy., 12, e2020MS002274, https://doi.org/10.1029/2020MS002274, 2020. a
Turnock, S. T., Allen, R. J., Andrews, M., Bauer, S. E., Deushi, M., Emmons, L., Good, P., Horowitz, L., John, J. G., Michou, M., Nabat, P., Naik, V., Neubauer, D., O'Connor, F. M., Olivié, D., Oshima, N., Schulz, M., Sellar, A., Shim, S., Takemura, T., Tilmes, S., Tsigaridis, K., Wu, T., and Zhang, J.: Historical and future changes in air pollutants from CMIP6 models, Atmos. Chem. Phys., 20, 14547–14579, https://doi.org/10.5194/acp-20-14547-2020, 2020. a, b, c
Twomey, S.: The Influence of Pollution on the Shortwave Albedo of Clouds, J. Atmos. Sci., 34, 1149–1152, https://doi.org/10.1175/1520-0469(1977)034<1149:TIOPOT>2.0.CO;2, 1977. a
Undorf, S., Polson, D., Bollasina, M. A., Ming, Y., Schurer, A., and Hegerl, G. C.: Detectable Impact of Local and Remote Anthropogenic Aerosols on the 20th Century Changes of West African and South Asian Monsoon Precipitation, J. Geophys. Res.-Atmos., 123, 4871–4889, https://doi.org/10.1029/2017JD027711, 2018. a, b
Wang, Y., Wang, M., Zhang, R., Ghan, S. J., Lin, Y., Hu, J., Pan, B., Levy, M., Jiang, J. H., and Molina, M. J.: Assessing the effects of anthropogenic aerosols on Pacific storm track using a multiscale global climate model, P. Natl. Acad. Sci. USA, 111, 6894–6899, https://doi.org/10.1073/pnas.1403364111, 2014. a
Wang, Y., Jiang, J. H., and Su, H.: Atmospheric responses to the redistribution of anthropogenic aerosols, J. Geophys. Res.-Atmos., 120, 9625–9641, https://doi.org/10.1002/2015JD023665, 2015. a
Wang, Y., Le, T., Chen, G., Yung, Y. L., Su, H., Seinfeld, J. H., and Jiang, J. H.: Reduced European aerosol emissions suppress winter extremes over northern Eurasia, Nat. Clim. Change, 10, 225–230, https://doi.org/10.1038/s41558-020-0693-4, 2020. a, b
Warszawski, L., Frieler, K., Huber, V., Piontek, F., Serdeczny, O., and Schewe, J.: The Inter-Sectoral Impact Model Intercomparison Project (ISI MIP): Project framework, P. Natl. Acad. Sci. USA, 111, 3228–3232, https://doi.org/10.1073/pnas.1312330110, 2014. a, b
Westervelt, D. M., Horowitz, L. W., Naik, V., Golaz, J.-C., and Mauzerall, D. L.: Radiative forcing and climate response to projected 21st century aerosol decreases, Atmos. Chem. Phys., 15, 12681–12703, https://doi.org/10.5194/acp-15-12681-2015, 2015. a
Westervelt, D. M., Conley, A. J., Fiore, A. M., Lamarque, J.-F., Shindell, D., Previdi, M., Faluvegi, G., Correa, G., and Horowitz, L. W.: Multimodel precipitation responses to removal of U.S. sulfur dioxide emissions, J. Geophys. Res.-Atmos., 122, 5024–5038, https://doi.org/10.1002/2017JD026756, 2017. a, b, c
Westervelt, D. M., Conley, A. J., Fiore, A. M., Lamarque, J.-F., Shindell, D. T., Previdi, M., Mascioli, N. R., Faluvegi, G., Correa, G., and Horowitz, L. W.: Connecting regional aerosol emissions reductions to local and remote precipitation responses, Atmos. Chem. Phys., 18, 12461–12475, https://doi.org/10.5194/acp-18-12461-2018, 2018. a, b
Westervelt, D. M., Mascioli, N. R., Fiore, A. M., Conley, A. J., Lamarque, J.-F., Shindell, D. T., Faluvegi, G., Previdi, M., Correa, G., and Horowitz, L. W.: Local and remote mean and extreme temperature response to regional aerosol emissions reductions, Atmos. Chem. Phys., 20, 3009–3027, https://doi.org/10.5194/acp-20-3009-2020, 2020a. a, b, c, d
Westervelt, D. M., You, Y., Li, X., Ting, M., Lee, D. E., and Ming, Y.: Relative Importance of Greenhouse Gases, Sulfate, Organic Carbon, and Black Carbon Aerosol for South Asian Monsoon Rainfall Changes, Geophys. Res. Lett., 47, e2020GL088363, https://doi.org/10.1029/2020GL088363, 2020b. a
Wilcox, L. J., Highwood, E. J., and Dunstone, N. J.: The influence of anthropogenic aerosol on multi-decadal variations of historical global climate, Environ. Res. Lett., 8, 24033, https://doi.org/10.1088/1748-9326/8/2/024033, 2013. a, b, c, d
Wilcox, L. J., Highwood, E. J., Booth, B. B. B., and Carslaw, K. S.: Quantifying sources of inter-model diversity in the cloud albedo effect, Geophysical Research Letters, 42, 1568–1575, https://doi.org/10.1002/2015GL063301, 2015. a
Wilcox, L. J., Dunstone, N., Lewinschal, A., Bollasina, M., Ekman, A. M. L., and Highwood, E. J.: Mechanisms for a remote response to Asian anthropogenic aerosol in boreal winter, Atmos. Chem. Phys., 19, 9081–9095, https://doi.org/10.5194/acp-19-9081-2019, 2019. a, b, c, d
Wilcox, L. J., Liu, Z., Samset, B. H., Hawkins, E., Lund, M. T., Nordling, K., Undorf, S., Bollasina, M., Ekman, A. M. L., Krishnan, S., Merikanto, J., and Turner, A. G.: Accelerated increases in global and Asian summer monsoon precipitation from future aerosol reductions, Atmos. Chem. Phys., 20, 11955–11977, https://doi.org/10.5194/acp-20-11955-2020, 2020. a, b, c
Wyser, K., van Noije, T., Yang, S., von Hardenberg, J., O'Donnell, D., and Döscher, R.: On the increased climate sensitivity in the EC-Earth model from CMIP5 to CMIP6, Geosci. Model Dev., 13, 3465–3474, https://doi.org/10.5194/gmd-13-3465-2020, 2020. a
Xie, X., Myhre, G., Liu, X., Li, X., Shi, Z., Wang, H., Kirkevåg, A., Lamarque, J.-F., Shindell, D., Takemura, T., and Liu, Y.: Distinct responses of Asian summer monsoon to black carbon aerosols and greenhouse gases, Atmos. Chem. Phys., 20, 11823–11839, https://doi.org/10.5194/acp-20-11823-2020, 2020. 2021a. a, b
Zhang, J., Furtado, K., Turnock, S. T., Mulcahy, J. P., Wilcox, L. J., Booth, B. B., Sexton, D., Wu, T., Zhang, F., and Liu, Q.: The role of anthropogenic aerosols in the anomalous cooling from 1960 to 1990 in the CMIP6 Earth system models, Atmos. Chem. Phys., 21, 18609–18627, https://doi.org/10.5194/acp-21-18609-2021, a
Zhang, L., Wilcox, L. J., Dunstone, N. J., Paynter, D. J., Hu, S., Bollasina, M., Li, D., Shonk, J. K. P., and Zou, L.: Future changes in Beijing haze events under different anthropogenic aerosol emission scenarios, Atmos. Chem. Phys., 21, 7499–7514, https://doi.org/10.5194/acp-21-7499-2021, 2021b. a
- Abstract
- Introduction
- Experimental design
- A first look at effective radiative forcing and fixed-SST responses in RAMIP
- Summary
- Appendix A: Models used in fixed-SST experiments
- Appendix B: RAMIP data request
- Data availability
- Author contributions
- Competing interests
- Disclaimer
- Acknowledgements
- Financial support
- Review statement
- References
- Supplement
- Abstract
- Introduction
- Experimental design
- A first look at effective radiative forcing and fixed-SST responses in RAMIP
- Summary
- Appendix A: Models used in fixed-SST experiments
- Appendix B: RAMIP data request
- Data availability
- Author contributions
- Competing interests
- Disclaimer
- Acknowledgements
- Financial support
- Review statement
- References
- Supplement